- 1National Clinical Research Center for Mental Disorders & National Center for Mental Disorders, Beijing Anding Hospital, Capital Medical University, Beijing, China
- 2Xiamen Xianyue Hospital, Xianyue Hospital Affiliated with Xiamen Medical College, Fujian Psychiatric Center, Fujian Clinical Research Center for Mental Disorders, Fujian, China
Objective: Limited research has explored the associations between sleep disturbances (SD) and cognitive impairment (CI) in elderly patients with depression, particularly by incorporating polysomnography (PSG) to assess sleep quality. This study was conducted to determine correlations between PSG-quantified sleep parameters and CI among individuals with late-life depression.
Methods: 65 elderly patients with depression were included in the study. The sleep status was assessed using PSG, while cognitive function was evaluated using the Mini-Mental State Examination (MMSE). The correlation between PSG-measured sleep parameters and cognitive function was analyzed.
Results: CI was observed in 31 (47.7%) individuals. Depressed elderly patients with CI exhibited a shorter total sleep time (TST) compared to those without CI. Furthermore, their sleep efficiency (SE) was reduced as evidenced by shortened durations and proportions of N1 and N3. Conversely, the proportion of non-rapid eye movement (NREM) and N2 increased in this group. Additionally, both the duration and proportion of rapid eye movement (REM) were decreased. Spearman correlation analysis revealed a linear relationship between the MMSE score and various sleep parameters. However, in the multiple linear regression model, only the proportions of NREM exhibited a significant linear relationship with the MMSE scores.
Conclusions: In elderly patients with depression, a significant linear relationship was observed between the MMSE score and various sleep parameters measured by PSG.
1 Introduction
Depression is the most prevalent mental disorder among older adults worldwide, affecting 7% of the global elderly population and contributing to 5.7% of years lived with disability in individuals aged over 60 years (1). The association between cognitive impairment (CI) and depression has been observed in recent years (2, 3). CI may represent a particularly significant aspect of depression from a practical standpoint (4). After reaching the age of 65, older adults who received an initial diagnosis of depression demonstrated a significantly heightened association with CI (OR = 6.65, p < 0.01) (5). Accumulated evidence underscores the pivotal role of systemic inflammation in both CI and depression (6). Furthermore, oxidative stress emerges as a shared mechanism underlying cognitive decline and depression (7). Consequently, the relationship between CI and depression exhibits complexity and bidirectionality.
The previous studies have demonstrated that the prevalence of sleep disturbances (SD) in patients with depression ranges from 60% to 90%, whereas the prevalence of SD among this patient population in China is reported as 85.22% (8). Dysregulation of monoamine neurotransmitters, reduced glutamate production in the brain, and disruption of sleep-wake rhythmicity by dysregulating the hypothalamic-pituitary-adrenal axis may underlie SD observed in patients with depression (9).
SD among older adults has consistently been linked to mental health issues and shown to be associated with cognitive decline (10, 11). In clinical practice, both CI and SD are associated with the progression of depression. SD and CI are prevalent non-affective manifestations in patients with depression (12). Shorter than recommended sleep is believed to reduce brain health and cognitive performance (13). It is important to emphasize that current knowledge is far from sufficient to understand the role of SD in elderly depressed patients with CI. SD can be a symptom, a risk factor, or an early marker in elderly depressed patients with CI. Gaining a more comprehensive understanding of the sequential two-mediator relationship between depression progression and SD in connecting depression and CI can provide valuable insights into the clinical significance of monitoring and managing mood and sleep among older adults, particularly highlighting the cognitive benefits of addressing late-life depression to mitigate CI.
In previous studies, researchers have employed a variety of methodologies to assess SD and CI. While the Pittsburgh Sleep Quality Index (PSQI) has commonly been utilized as a subjective tool, actigraphy devices have also been used. However, polysomnography (PSG), considered the gold standard for detecting SD (14), represents an objective and sensitive assessment of sleep status (15). To the best of our knowledge, limited research has explored the associations between SD and CI in elderly patients with depression, particularly by incorporating PSG to assess sleep quality. In non-rapid eye movement (NREM) stages N1, N2, and N3, a previous research suggested that slow wave sleep (SWS) is related to better cognitive function (16).Thus, there is a lack of research that has correlated neuropsychological test measures with PSG measurements (17). In light of this gap, we conducted this study to determine correlations between PSG-quantified sleep architecture disturbances and CI among individuals with late-life depression.
2 Materials and methods
2.1 Subjects
Participants were recruited from Beijing Anding Hospital between June 2021 and May 2024. The patients included in the study were diagnosed with depression based on the criteria outlined in the Diagnostic and Statistical Manual of Mental Disorders, 5th edition (DSM-5) criteria and Mini-International Neuropsychiatric Interview (M.I.N.I.). Eligible participants included individuals aged 60-85 years who exhibited moderate or greater severity of symptoms, as indicated by a score of ≥17 on the Hamilton Depression Scale (HAMD-17) (18). And they had not received antipsychotic treatment for at least 3 months. Patients with the following conditions were excluded: (1) Patients with a history of epilepsy or coronary heart disease or other serious unstable physical diseases; (2) visual and hearing loss, illiteracy, and the inability to complete the required tests; (3) a history of psychiatric diseases such as organic mental disorders, Alzheimer’s disease, secondary dementia of other causes, schizophrenia, schizoaffective disorder and bipolar disorder; (4) a history of substance abuse, including alcohol or active substance abuse within the past 12 months, except nicotine; and (5) use of medications that may affect cognition or sleep for nearly 2 weeks. Written informed consent was obtained from all participants. The study was approved by the Medical Ethical Committee in Beijing Anding Hospital of the Capital Medical University, China [(2021) Research No. (75)]. The demographic and clinical characteristics of each patient were systematically documented.
2.2 PSG examination
All the subjects were observed with overnight video PSG using a digital sleep laboratory system (Grael, Compumedics, Australia). All PSG sessions were meticulously supervised by a proficient technician in accordance with standardized criteria. The inter-rater reliability is regularly assessed and ensured to exceed 0.9 as an integral part of laboratory protocols. The following sleep characteristics were acquired and analyzed: total sleep time (TST); sleep efficiency (SE); the time and percentage of stage N1, N2, N3, NREM, and rapid eye movement (REM) sleep, and the apnea hypopnea index (AHI). Obstructive sleep apnea (OSA) is defined as the presence of an AHI ≥5/h.
2.3 Subjective sleep and neuropsychological evaluations
The PSQI, developed by Buysse et al. in 1989, was utilized to assess the subjective sleep statuses of all participants. This questionnaire consists of 19 items that evaluate seven subdomains including daytime dysfunction, sleep disturbance, subjective sleep quality, habitual sleep efficiency, sleep duration, sleep latency and hypnotics use on a 4-point scale (ranging from 0 to 3). The total score ranges from 0 to 21 points with higher scores indicating poorer sleep quality. Individuals scoring ≥5 are categorized as poor sleepers (19).
Hamilton Depression Rating Scale (HAMD‐17) were used to assess depressive symptoms, with a HAMD-17 score ≥ 24 indicating the presence of severe depression (20). Cognitive functioning was evaluated with the Chinese version of the Mini Mental State Examination (MMSE) (21). The MMSE comprises a variety of cognitive tasks, encompassing immediate and delayed word recall, animal naming, word recognition, symbol digit modalities test, immediate constructional praxis, digit span backwards test, immediate and delayed logical memory, number series and trail-making tests. These tasks collectively contribute to a maximum achievable score of 30 points. A MMSE score < 24 is indicative of CI (22, 23).
2.4 Statistical analysis
All statistical tests were performed with SPSS 26.0 software (IBM Corp, Armonk, NY, USA). Statistical significance was set as a p-value<0.05 (two-tailed). The Chi-square test was used to determine the distribution of categorical variables, and the Student’s t test or Mann-Whitney U test was used for continuous variables. The Spearman correlation analysis was employed to examine the correlation between PSG-measured sleep parameters and cognitive function. Only those variables that were identified as significant (p<0.05) in the univariate analysis were included in the multivariate analysis. The sleep parameters of the patients were included in the multiple linear regression model along with the patients’ educational level, degree of depression, and OSA. This allowed for a comprehensive examination of the independent effects of each sleep parameter on CI. Figures were created using GraphPad Prism Version 9 (San Diego, USA).
3 Results
3.1 Demographic and clinical characteristics of all participants
A total of 65 elderly patients with depression, comprising 24 males and 41 females, were included in the study. The median age of 67 years (interquartile range, [IQR]: 65- 70), with a mean body mass index (BMI) 25.2 ± 4.0 Kg/m2. The PSQI had a median score of 15 (IQR:10-18), while the AHI had a median value of 4.90/h (IQR: 0.45-11.65). Among these patients, CI was observed in 31 (47.7%) individuals based on a MMSE score <24, while senior high school education or above was reported by only 22(33.8%) patients. Additionally, severe depression was present in 17 (26%) patients, and OSA was diagnosed in 31 (47.7%) patients. Table 1 summarizes the socio-demographic and clinical characteristics between CI and non-CI groups. The results revealed that CI was more prevalent among patients with severe depression (39.6% vs 70.6%, p=0.028), those with lower than senior high school education (22.7% vs 60.5%, p=0.004), higher PSQI scores (10 vs 17, p<0.001), or OSA (32.4% vs 64.5%, p=0.010). No significant differences were found regarding age, gender, BMI, and AHI when comparing subgroups.
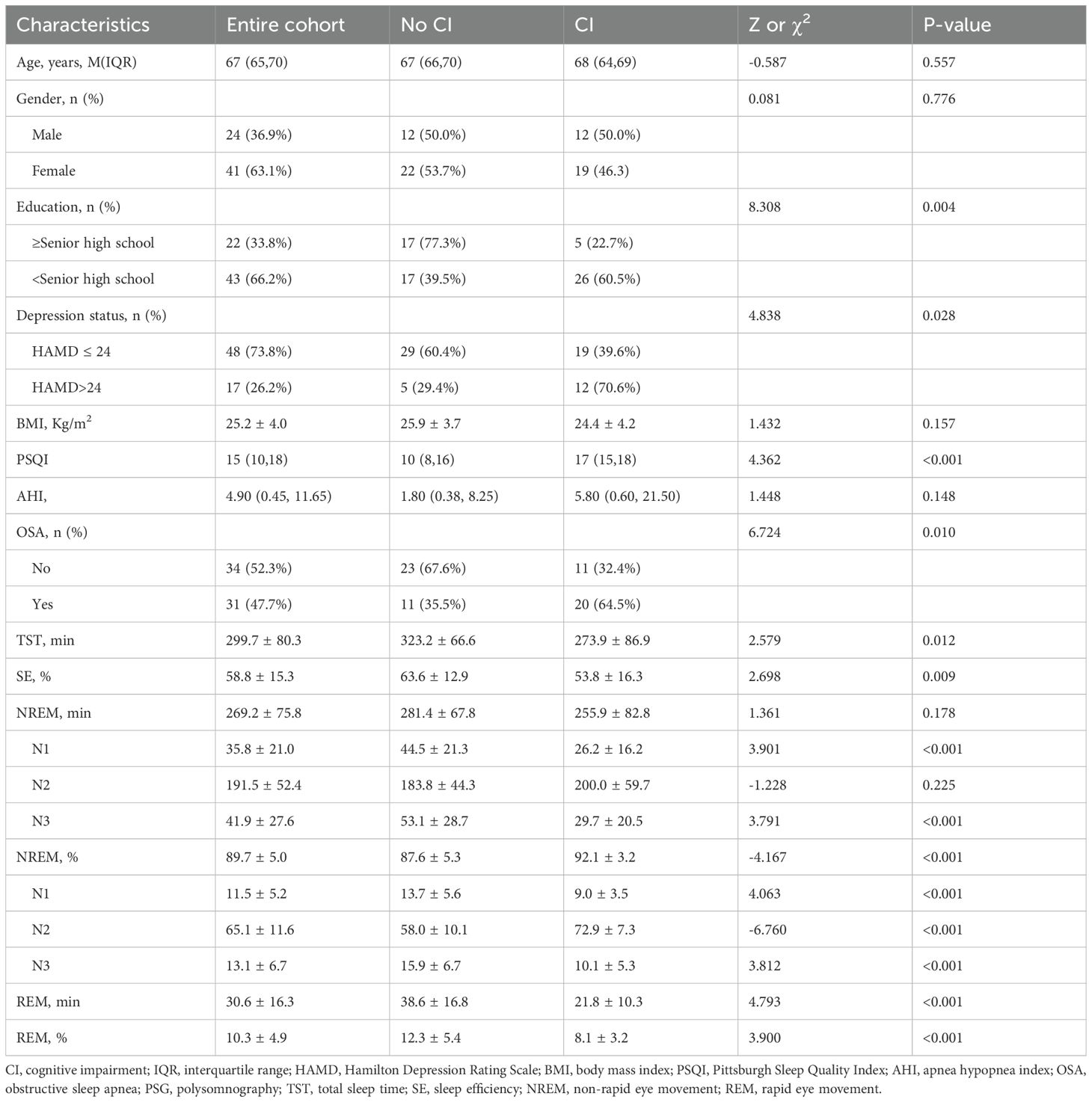
Table 1. General demographic, clinical characteristics and PSG-measured sleep parameters of patients with and without CI.
3.2 PSG-measured sleep parameters of all participants
The average TST of the 65 patients was 299.7 ± 80.3 min, with an average SE of 58.8 ± 15.3%. And 64 (98.5%) patients suffered from SD with a SE < 85% (24). The mean NREM sleep duration was 269.2 ± 7 5.8 min, comprising N1 (35.8 ± 21.0 min), N2 (191.5 ± 52.4 min), and N3 (41.9 ± 27.6 min). The proportion of NREM sleep accounted for an average of 89.7 ± 5.0%, with N1 representing 11.5 ± 5.2%, N2 representing 65.l ± 11.6%, and N3 representing 13.l ± 6.7%. On average, REM sleep lasted for 30.6 ± 16.3 min, constituting 10.3 ± 4.9%. A comparison between the two groups regarding PSG-measured sleep parameters is presented in Table 1. The results revealed that depressed elderly patients with CI exhibited a shorter TST compared to those without CI (323.2 ± 66.6 min vs 273.9 ± 86.9 min, t = 2.579, p = 0.012). Furthermore, their SE was reduced (63.6 ± 12.9% vs 53.8 ± 16.3%, t =2.698, p = 0.009), as evidenced by shortened N1 and N3 sleep durations (44.5 ± 21.3 min vs 26.2 ± 16.2 min, t =3.901, p < 0.001; 53.1 ± 28.7 min vs 29.7 ± 20.5 min, t = 3.791, p < 0.001, respectively) and proportions (13.7 ± 5.6% vs 9.0 ± 3.5%, t = 4.063, p < 0.001; 15.9 ± 6.7% vs 10.1 ± 5.3%, t = 3.812, p < 0.001, respectively). Conversely, the proportion of NREM sleep and N2 sleep increased in this group (87.6 ± 5.3% vs 92.1 ± 3.2%, t = -4.167, p < 0.001; 58.0 ± 10.1% vs 72.9 ± 7.3%, t = -6.760, p < 0.001, respectively). Additionally, both the duration and proportion of REM sleep were decreased (38.6 ± 16.8 min vs 21.8 ± 10.3 min, t = 4.793, p < 0.001; 12.3 ± 5.4% vs 8.1 ± 3.2%, t = 3.900, p < 0.001, respectively). Notably, there were no significant differences observed in the NREM or N2 sleep durations between the two groups.
3.3 Correlation between PSG-measured sleep parameters and cognitive function
The Spearman correlation analysis revealed a positive linear relationship between the MMSE score and various sleep parameters including TST (r = 0.325), SE (r = 0.330), N1 sleep time (r = 0.422), N1 sleep proportion (r = 0.381), N3 sleep time (r = 0.511), N3 sleep proportion (r = 0.510), REM sleep time (r = 0.467) and REM sleep proportion (r = 0.323) in patients (Figures 1A–D, F, H, J, L, respectively) (all p < 0.01). Conversely, there was a negative linear correlation observed between the MMSE score and the proportions of NREM (r = -0.346, p < 0.01) and N2 sleep (r = -0.650, p < 0.001) (Figures 1I, K). And there was no linear correlation between the MMSE score and NREM (r = 0.226, p > 0.05) or N2 sleep time (r = 0.068, p > 0.05) (Figures 1E, G). However, in the multiple linear regression model, only the proportions of NREM exhibited a significant linear relationship with the MMSE scores (p = 0.041) (Table 2).
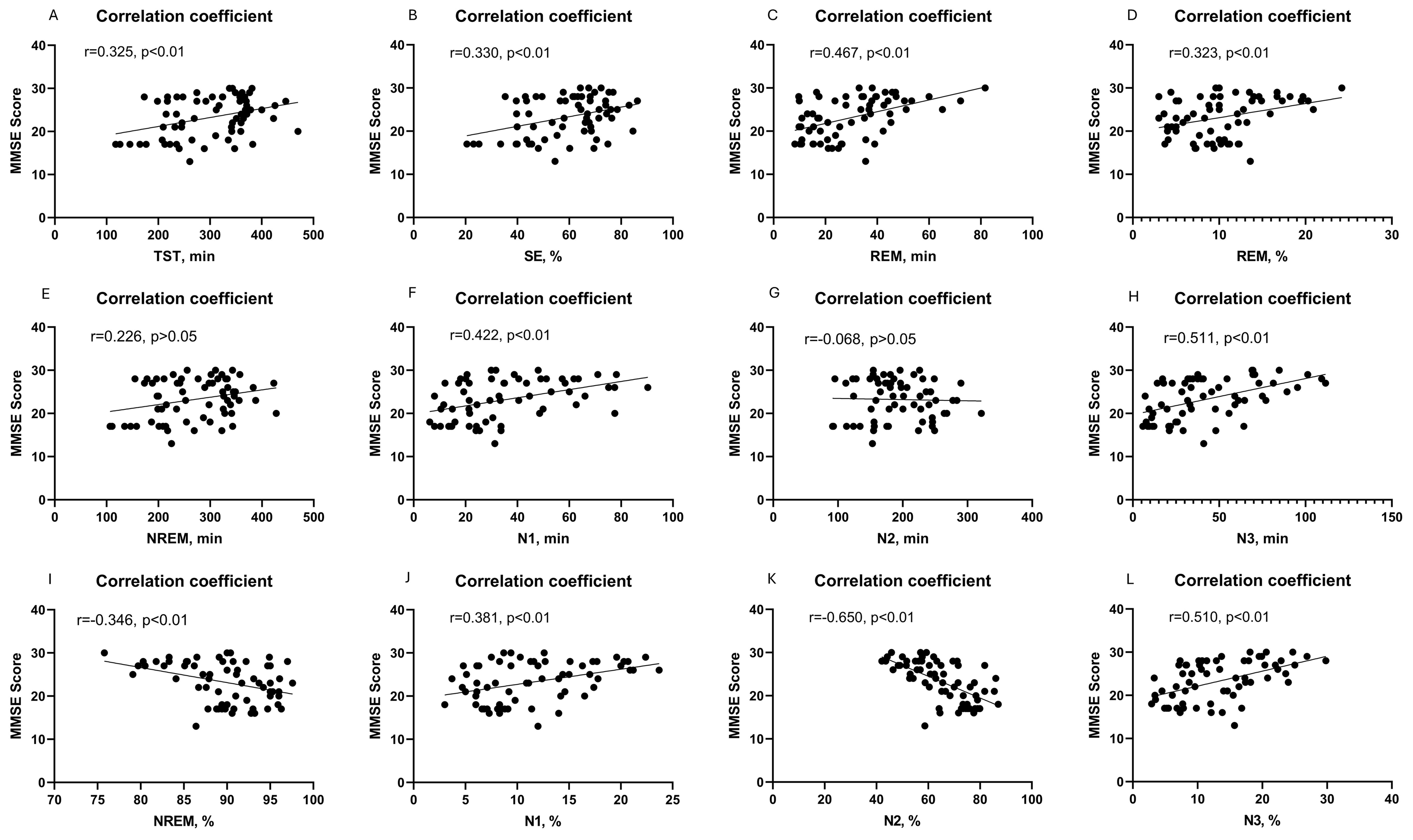
Figure 1. Correlation between cognitive function and PSG-measured sleep parameters, including TST sleep time (A); SE (B); REM sleep time (C); REM sleep proportion (D); NREM sleep time (E); N1 sleep time (F); N2 sleep time (G); N3 sleep time (H); NREM sleep proportion (I); N1 sleep proportion (J); N2 sleep proportion (K); and N3 sleep proportion (L).
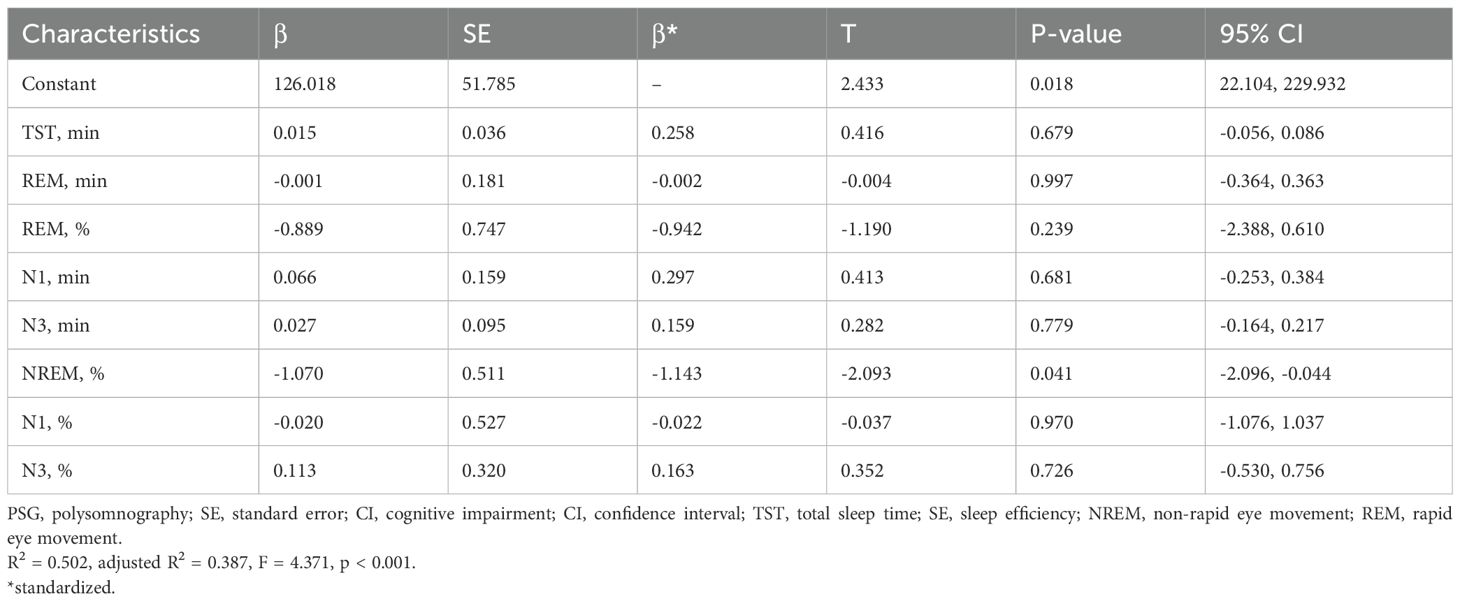
Table 2. The associations between PSG-measured sleep parameters and CI in late-life depression were analyzed using multiple linear regression.
4 Discussion
An increasing body of literature investigates the association between depression, sleep, and cognition; however, limited knowledge exists regarding older adults. Previous studies predominantly relied on questionnaires lacking objective evidence, which may raise concerns about recall bias and introduce uncertainty regarding participants’ actual sleep conditions (9). In our study, PSG was utilized to objectively evaluate sleep parameters in elderly patients with depression and their associations with cognitive impairment were examined. Among the 65 patients, CI was observed in 31 (47.7%) individuals. Depressed elderly patients with CI exhibited a shorter TST compared to those without CI. Furthermore, their SE was reduced as evidenced by shortened durations and proportions of N1 and N3. Conversely, the proportion of NREM and N2 increased in this group. Additionally, both the duration and proportion of REM were decreased. Spearman correlation analysis revealed a linear relationship between the MMSE score and various sleep parameters. However, in the multiple linear regression model, only the proportions of NREM exhibited a significant linear relationship with the MMSE scores. Those findings can shed light on the clinical relevance of monitoring and managing mental status and sleep for elderly patients with depression. These findings offer valuable insights into the clinical significance of monitoring and managing mental health status and sleep patterns in geriatric patients with depression.
Previous studies have demonstrated that the prevalence of sleep disorders in patients with depression ranges from 60% to 90% (8), which is comparatively lower than the observed rate of 98.5% reported in this study. This discrepancy may be attributed to the inclusion of exclusively elderly patients in our study cohort. Although aging per se does not lead to an escalation in SD, alterations in sleep architecture manifest with advancing age. A lot of empirical evidence suggests a high prevalence of SD within the elderly population (25, 26). In our study, the incidence of CI in elderly patients with depression was 47.7%, which was basically consistent with previous studies (27). Older adults with depression are prone to a progressive decline in mental health over time, which may contribute to age-related cognitive deterioration through pathogenetic mechanisms (28). This decline is characterized by an increased influence from subcortical emotion processing regions combined with attenuated top-down cognitive control (29). Recent studies have indicated that late-life depression also disrupts cognition through dysregulation of altered functional and structural brain connections (30).
A strong correlation has been observed between PSG-measured SD and CI in elderly patients with depression in our study. This is consistent with other studies that have shown impaired memory function due to disrupted sleep, either through a decreased proportion of REM sleep or reduced SE (31). In a previous study, the association between sleep duration and cognitive function was examined in a cohort of 1844 community-dwelling women; it was found that women who slept ≤5 h/night performed worse cognitively compared to those who slept 7 h/night (32). In NREM stages N1, N2, and N3, research focuses on the effects of SWS on cognition; results suggest that SWS is related to better cognitive function (16). In contrast to a previous study that, after controlling for multiple potential confounders, reported a U-shaped association between sleep duration and cognitive decline (33), our study was able to confirm only the declining trend in cognitive function with shorter sleep durations. This discrepancy may be attributed to the fact that all TST in our study were not higher than 10 hours. The reason for this phenomenon also might be Aβ deposition. Shorter sleep duration has been associated with increased Aβ deposition, indicating that SD contributes to CI rather than being solely a consequence of brain lesions. Moreover, Aβ accumulation in the regions involved in sleep regulation may exacerbate SD, leading to further elevation and accumulation of Aβ (34). In cognitively healthy older adults, both reduced sleep quality and shorter sleep duration have been linked to increased Aβ burden (35). Additionally, poor objectively measured sleep quality correlates with decreased CSF Aβ levels in healthy aging individuals (34). Earlier research using a mouse model of amyloidosis, which naturally develops Aβ plaques, demonstrated that extended periods of wakefulness were associated with higher ISF Aβ concentrations, whereas sleep deprivation hastened the formation of Aβ plaques (36, 37). Collectively, these findings suggest a potential connection between sleep and CI. Additional work is needed to determine whether these biological pathways mediate the association between sleep and cognitive function.
In contrast to other studies, we were not able to detect an influence of AHI on cognition. This might be due to the selection bias of patients with only very low AHI in this population, which is not representative for sleep disordered breathing patients. And we did not detect an association between cognition and BMI, underlining the assumption that the pathophysiology of cognition seems to differ between depression patients and the general population, which means that the clinical features pointing toward cognitive impairment in the general population seem not to be helpful in identifying patients at risk in the late-life depression population (38).
Several limitations should be acknowledged in this study. Firstly, PSG sleep tests were conducted without prior acclimatization of participants to the sleep laboratory, potentially introducing the first-night effect (i.e., differences observed in the initial PSG sleep recording compared to subsequent ones) (39), which has been shown to have a greater impact on older individuals than younger ones. However, it is less likely that this hypothesis holds true as the influence of the first-night effect on sleep structure would be expected to be similar in both cognitively impaired and non-impaired patients. Secondly, this work is limited by its relatively small sample size, which only allows for a limited number of statistical sub-analyses and lacks sufficient statistical power. Finally, the inclusion of certain confounding variables, such as socioeconomic status, was not accounted for in our study and should be considered as covariates in future research endeavors.
In conclusion, in elderly patients with depression, a significant linear relationship was observed between the MMSE score and various sleep parameters measured by PSG. Decreased sleep parameters, including TST, SE, duration of N1 stage, duration of N3 stage, proportion of N1 stage, and REM sleep time, along with an increased proportion of N2 stage, were identified as independent risk factors for CI.
Data availability statement
The raw data supporting the conclusions of this article will be made available by the authors, without undue reservation.
Ethics statement
The studies involving humans were approved by The Medical Ethical Committee in Beijing Anding Hospital of the Capital Medical University, China. The studies were conducted in accordance with the local legislation and institutional requirements. The participants provided their written informed consent to participate in this study.
Author contributions
JZ: Conceptualization, Validation, Writing – original draft, Supervision, Visualization. JQ: Validation, Writing – original draft. YW: Validation, Writing – original draft. LR: Supervision, Validation, Visualization, Writing – review & editing, Data curation. SZ: Data curation, Validation, Visualization, Writing – review & editing. XM: Data curation, Validation, Visualization, Writing – review & editing. YF: Formal analysis, Investigation, Validation, Visualization, Writing – review & editing. QZ: Conceptualization, Funding acquisition, Supervision, Validation, Visualization, Writing – review & editing. XC: Writing – review & editing.
Funding
The author(s) declare that financial support was received for the research and/or publication of this article. The study was supported by the Capital Health Development Research Special Fund (2022-3-2124); the Training Plan for High Level Public Health Technical Talents Construction Project (Discipline Backbones-02-38); Central Health Care Research Fund (2022YB68); the Researches project within the hospital (No. 35 of 2021 and YX2021-01).
Acknowledgments
The authors are appreciative of the donors, whose names are not included in the author list but who participated in this program. An unauthorized version of the Chinese MMSE was used by the study team without permission, however this has now been rectified with PAR. The MMSE is a copyrighted instrument and may not be used or reproduced in whole or in part, in any form or language, or by any means without written permission of PAR.
Conflict of interest
The authors declare that the research was conducted in the absence of any commercial or financial relationships that could be construed as a potential conflict of interest.
Publisher’s note
All claims expressed in this article are solely those of the authors and do not necessarily represent those of their affiliated organizations, or those of the publisher, the editors and the reviewers. Any product that may be evaluated in this article, or claim that may be made by its manufacturer, is not guaranteed or endorsed by the publisher.
References
1. Mlaki DA, Asmal L, Paddick SM, Gray WK, Dotchin C, Walker R. Prevalence and associated factors of depression among older adults in rural Tanzania. Int J Geriatr Psychiatry. (2021) 36:1559–66. doi: 10.1002/gps.5584
2. Liu X, Xia X, Hu F, Hao Q, Hou L, Sun X, et al. The mediation role of sleep quality in the relationship between cognitive decline and depression. BMC Geriatr. (2022) 22:178. doi: 10.1186/s12877-022-02855-5
3. Xu W, Lin L, Ding K, Ke Y, Huang J, Hou C, et al. The role of depression and anxiety in the relationship between poor sleep quality and subjective cognitive decline in Chinese elderly: Exploring parallel, serial, and moderated mediation. J Affect Disord. (2021) 294:464–71. doi: 10.1016/j.jad.2021.07.063
4. Yang X, Qi S, Wang M, Calhoun V, Sui J, Li T, et al. Subtypes of depression characterized by different cognitive decline and brain activity alterations. J Psychiatr Res. (2021) 138:413–9. doi: 10.1016/j.jpsychires.2021.04.023
5. Nazar G, Ulloa N, Martínez-Sanguinetti MA, Leiva AM, Petermann-Rocha F, Martínez XD, et al. Association between cognitive impairment and depression in Chilean older adults. Rev Med Chil. (2020) 148:947–55. doi: 10.4067/s0034-98872020000700947
6. Peiffer G, Underner M, Perriot J, Fond G. COPD, anxiety-depression and cognitive disorders: Does inflammation play a major role? Rev Mal Respir. (2021) 38:357–71. doi: 10.1016/j.rmr.2021.03.004
7. Ahmed T, Braidy N. Editorial: from oxidative stress to cognitive decline - towards novel therapeutic approaches. Front Mol Neurosci. (2021) 14:650498. doi: 10.3389/fnmol.2021.650498
8. Chen RF, Cai Y, Zhu ZH, Hou WL, Chen P, Wang J, et al. Sleep disorder as a clinical risk factor of major depression: associated with cognitive impairment. Asian J Psychiatr. (2022) 76:103228. doi: 10.1016/j.ajp.2022.103228
9. Liu J, Chen Q. Sequential link in depression, sleep and cognition: Longitudinal evidence from a serial multiple mediation analysis of older adults in China. Arch Gerontol Geriatr. (2024) 117:105249. doi: 10.1016/j.archger.2023.105249
10. Reid KJ, Martinovich Z, Finkel S, Statsinger J, Golden R, Harter K, et al. Sleep: a marker of physical and mental health in the elderly. Am J Geriatr Psychiatry. (2006) 14:860–6. doi: 10.1097/01.JGP.0000206164.56404.ba
11. Jaussent I, Bouyer J, Ancelin ML, Berr C, Foubert-Samier A, Ritchie K, et al. Excessive sleepiness is predictive of cognitive decline in the elderly. Sleep. (2012) 35:1201–7. doi: 10.5665/sleep.2070
12. Cha DS, Carmona N, Cha RH, Zhou AJ, Subramaniapillai M, Mansur RB, et al. Perceived sleep quality predicts cognitive function in adults with major depressive disorder independent of depression severity. Ann Clin Psychiatry. (2019) 31:17–26.
13. Zamore Z, Veasey SC. Neural consequences of chronic sleep disruption. Trends Neurosci. (2022) 45:678–91. doi: 10.1016/j.tins.2022.05.007
14. Li Y, Li J, Yan C, Dong K, Kang Z, Zhang H, et al. Sleep quality evaluation based on single-lead wearable cardiac cycle acquisition device. Sens (Basel). (2022) 23:328. doi: 10.3390/s23010328
15. Withrow D, Roth T, Koshorek G, Roehrs T. Relation between ambulatory actigraphy and laboratory polysomnography in insomnia practice and research. J Sleep Res. (2019) 28:e12854. doi: 10.1111/jsr.12854
16. Aricò D, Drago V, Foster PS, Heilman KM, Williamson J, Ferri R. Effects of NREM sleep instability on cognitive processing. Sleep Med. (2010) 11:791–8. doi: 10.1016/j.sleep.2010.02.009
17. Menon RN, Radhakrishnan A, Sreedharan SE, Sarma PS, Kumari RS, Kesavadas C, et al. Do quantified sleep architecture abnormalities underlie cognitive disturbances in amnestic mild cognitive impairment? J Clin Neurosci. (2019) 67:85–92. doi: 10.1016/j.jocn.2019.06.014
18. Hamilton M. A rating scale for depression. J Neurol Neurosurg Psychiatry. (1960) 23:56–62. doi: 10.1136/jnnp.23.1.56
19. Mollayeva T, Thurairajah P, Burton K, Mollayeva S, Shapiro CM, Colantonio A. The Pittsburgh sleep quality index as a screening tool for sleep dysfunction in clinical and non-clinical samples: A systematic review and meta-analysis. Sleep Med Rev. (2016) 25:52–73. doi: 10.1016/j.smrv.2015.01.009
20. Zimmerman M, Martinez JH, Young D, Chelminski I, Dalrymple K. Severity classification on the Hamilton depression rating scale. J Affect Disord. (2013) 150:384–8. doi: 10.1016/j.jad.2013.04.028
21. Cui GH, Yao YH, Xu RF, Tang HD, Jiang GX, Wang Y, et al. Cognitive impairment using education-based cutoff points for CMMSE scores in elderly Chinese people of agricultural and rural Shanghai China. Acta Neurol Scand. (2011) 124:361–7. doi: 10.1111/j.1600-0404.2010.01484.x
22. Gazibara T, Kisic-Tepavcevic D, Svetel M, Tomic A, Stankovic I, Kostic V, et al. Dynamics of change in self-reported disability among persons with Parkinson’s disease after 2 years of follow-up. Neurol Sci. (2017) 38:1415–21. doi: 10.1007/s10072-017-2967-9
23. Dana A, Shams A, Allafan N, Bahrami A. The relationship between attention and static balance disturbance in patients with Parkinson’s disease. Neurol Sci. (2021) 42:5107–15. doi: 10.1007/s10072-021-05184-4
24. Yu JM, Tseng IJ, Yuan RY, Sheu JJ, Liu HC, Hu CJ. Low sleep efficiency in patients with cognitive impairment. Acta Neurol Taiwan. (2009) 18:91–7.
25. Neikrug AB, Ancoli-Israel S. Sleep disorders in the older adult - a mini-review. Gerontology. (2010) 56:181–9. doi: 10.1159/000236900
26. Prinz PN, Vitiello MV, Raskind MA, Thorpy MJ. Geriatrics: sleep disorders and aging. N Engl J Med. (1990) 323:520–6. doi: 10.1056/nejm199008233230805
27. Hack LM, Tozzi L, Zenteno S, Olmsted AM, Hilton R, Jubeir J, et al. A cognitive biotype of depression and symptoms, behavior measures, neural circuits, and differential treatment outcomes: A prespecified secondary analysis of a randomized clinical trial. JAMA Netw Open. (2023) 6:e2318411. doi: 10.1001/jamanetworkopen.2023.18411
28. Akechi T, Sugishita K, Chino B, Itoh K, Ikeda Y, Shimodera S, et al. Whose depression deteriorates during acute phase antidepressant treatment? J Affect Disord. (2020) 260:342–8. doi: 10.1016/j.jad.2019.09.052
29. Disner SG, Beevers CG, Haigh EA, Beck AT. Neural mechanisms of the cognitive model of depression. Nat Rev Neurosci. (2011) 12:467–77. doi: 10.1038/nrn3027
30. Lee A, Fung A, Richards M, Chan WC, Chiu H, Lee R, et al. Risk of incident dementia varies with different onset and courses of depression. J Affect Disord. (2021) 282:915–20. doi: 10.1016/j.jad.2020.12.195
31. Scullin MK, Bliwise DL. Sleep, cognition, and normal aging: integrating a half century of multidisciplinary research. Perspect Psychol Sci. (2015) 10:97–137. doi: 10.1177/1745691614556680
32. Tworoger SS, Lee S, Schernhammer ES, Grodstein F. The association of self-reported sleep duration, difficulty sleeping, and snoring with cognitive function in older women. Alzheimer Dis Assoc Disord. (2006) 20:41–8. doi: 10.1097/01.wad.0000201850.52707.80
33. Pistacchi M, Gioulis M, Contin F, Sanson F, Marsala SZ. Sleep disturbance and cognitive disorder: epidemiological analysis in a cohort of 263 patients. Neurol Sci. (2014) 35:1955–62. doi: 10.1007/s10072-014-1870-x
34. Ju YE, McLeland JS, Toedebusch CD, Xiong C, Fagan AM, Duntley SP, et al. Sleep quality and preclinical Alzheimer disease. JAMA Neurol. (2013) 70:587–93. doi: 10.1001/jamaneurol.2013.2334
35. Spira AP, Gamaldo AA, An Y, Wu MN, Simonsick EM, Bilgel M, et al. Self-reported sleep and β-amyloid deposition in community-dwelling older adults. JAMA Neurol. (2013) 70:1537–43. doi: 10.1001/jamaneurol.2013.4258
36. Roh JH, Huang Y, Bero AW, Kasten T, Stewart FR, Bateman RJ, et al. Disruption of the sleep-wake cycle and diurnal fluctuation of β-amyloid in mice with Alzheimer’s disease pathology. Sci Transl Med. (2012) 4:150ra122. doi: 10.1126/scitranslmed.3004291
37. Kang J, Lim MM, Bateman RJ, Lee JJ, Smyth LP, Cirrito JR, et al. Amyloid-beta dynamics are regulated by orexin and the sleep-wake cycle. Science. (2009) 326:1005–7. doi: 10.1126/science.1180962
38. Mina T, Yew YW, Ng HK, Sadhu N, Wansaicheong G, Dalan R, et al. Adiposity impacts cognitive function in Asian populations: an epidemiological and Mendelian Randomization study. Lancet Reg Health West Pac. (2023) 33:100710. doi: 10.1016/j.lanwpc.2023.100710
Keywords: polysomnography, sleep, cognitive impairment, elderly patient, depression
Citation: Zhou J, Que J, Wang Y, Ren L, Zhang S, Ma X, Fan Y, Zhang Q and Chen X (2025) Association between polysomnography-measured sleep parameters and cognitive impairment in elderly patients with depression. Front. Psychiatry 16:1485127. doi: 10.3389/fpsyt.2025.1485127
Received: 23 August 2024; Accepted: 17 March 2025;
Published: 24 April 2025.
Edited by:
Gaelle Eve Doucet, Boys Town National Research Hospital, United StatesReviewed by:
Matisse Ducharme, University of Toronto, CanadaMaggie P. Rempe, University of Nebraska Medical Center, United States
Copyright © 2025 Zhou, Que, Wang, Ren, Zhang, Ma, Fan, Zhang and Chen. This is an open-access article distributed under the terms of the Creative Commons Attribution License (CC BY). The use, distribution or reproduction in other forums is permitted, provided the original author(s) and the copyright owner(s) are credited and that the original publication in this journal is cited, in accordance with accepted academic practice. No use, distribution or reproduction is permitted which does not comply with these terms.
*Correspondence: Qing’e Zhang, enFlODFAMTI2LmNvbQ==; Xueyan Chen, Y2hlbnh1ZXlhbkBtYWlsLmNjbXUuZWR1LmNu
†These authors have contributed equally to this work