- 1Department of Child and Adolescent Psychiatry, Psychotherapy and Psychosomatics, University Hospital Leipzig, Leipzig, Germany
- 2KIZMO GmbH - Clinical Innovation Center for Medical Technology, Oldenburg, Germany
- 3SpeechCare GmbH, Leverkusen, Germany
- 4Branch for Hearing, Speech and Audio Technology HSA, Fraunhofer Institute for Digital Media Technology IDMT, Oldenburg, Germany
- 5Research Unit of Language and Communication, Department of Rehabilitation Sciences, TU Dortmund University, Dortmund, Germany
Introduction: The diagnosis of autism spectrum disorder (ASD) is resource-intensive and associated with long waiting times. Digital screenings using facial expression recognition (FER) are a promising approach to accelerate the diagnostic process while increasing its sensitivity and specificity. The aim of this study is to examine whether the identification of smile events using FER in an autism diagnosis utilisation population is reliable.
Methods: From video recordings of children undergoing the Autism Diagnostic Observation Schedule (ADOS-2) due to suspected ASD, sequences showing smile and non-smile events were identified. It is being investigated whether the FER reliably recognizes smile events and corresponds to a human rating.
Results: The FER based on the facial action unit mouthSmile accurately identifies smile events with a sensitivity of 96.43% and a specificity of 96.08%. A very high agreement with human raters (κ = 0.918) was achieved.
Discussion: This study demonstrates that smile events can in principle be identified using FER in a clinical utilisation population of children with suspected autism. Further studies are required to generalise the results.
Introduction
Over the past two decades, considerable technical progress has been made in the automated analysis of facial expressions, language and gestures. Data processing tools are increasingly available on mobile devices and are therefore accessible to a wide range of users, opening up a variety of potential applications, for example in healthcare diagnostics and rehabilitation (1–4).
Autism spectrum disorder (ASD) is characterized by abnormalities in social communication (including reduced social initiative, social smile and eye contact, reduced gestures, reduced mimic), in speech and language and movement stereotypes (5). Currently, the diagnosis of ASD relies on the assessment of experts, with screening-instruments, interviews and structured behavioral observations, such as the Autism Diagnostic Observation Schedule (ADOS-2) (6, 7). The high demand for an ASD diagnostic, the low specificity of the screening systems (5) and the low relative availability of diagnostic resources result in long waiting times and late diagnosis internationally, which some authors refer to as a waiting list crisis (8). Automating parts of the diagnostic process through facial expression recognition (FER) could provide an objective, accessible, and resource-efficient screening tool, particularly in structurally weak regions (9).
This study is part of the IDEAS project (Identification of autism using speech and facial expression analysis) which examines the technical feasibility and clinical potential of automated analysis methods for ASD screening (10). It focuses on the identification of smile events, as social smiles play a crucial role in ASD diagnostics (5). Research indicates, that the non-verbal communication skills of autistic children differ from that of healthy controls, for instance through reduced social smiling, pointing gestures and eye contact (5, 11, 12). Therefore, one of the key points of the clinician’s evaluation during ADOS assessments, is to observe whether a child initiates, shares or reciprocates smiles, a fundamental skill for social interaction (5). This proof-of-principle study aims to determine whether smile events of an individual can be reliably automatically detected with FER.
Most facial expression analysis software [for an overview, see (12)] include an option for identifying facial Action Units (AU), a concept based on the Facial Action Coding System (FACS) developed by Ekman (13). The identification of smile events is based on AU 6 (cheek raiser) and AU 12 (lip corner puller), which are associated with the expression of joy (14, 15). While previous studies have demonstrated high accuracy in identifying smile events in controlled environments in healthy adults (14, 15), its application in naturalistic diagnostic settings presents challenges. These include variability in recording conditions, occlusions (e.g., hands or objects covering the face), movement artifacts, and the spontaneous nature of expressions, all of which can affect detection accuracy (15). It is therefore necessary to test the reliability of the FER in a challenging population, namely freely moving children with autistic symptoms.
In the current study, we address the following hypothesis: automated FER (based on AU 6 and AU 12 events) identifies smile events in video sequences of ADOS videos with high sensitivity/specificity and thus shows high agreement with human ratings.
Methods
Study participants and psychological assessment
Nine children (age: M = 10.24; SD = 3.84; range: 5.63-16.78; sex: 2 female, 7 male) were recruited via the outpatient ASD unit of the Department of Child and Adolescent Psychiatry, Psychotherapy and Psychosomatics, University Hospital Leipzig. All participants were presented at the clinic due to indications of ASD and were undergoing diagnostic clarification. All participants exhibited fluent speech.
The participants underwent standardized ASD diagnostics, with the results taken from the medical records. The ASD screening instrument Social Communication Questionnaire (SCQ; German version) (13) was completed for each participant. SCQ raw values ranged from 6 to 27 (M = 16.89; SD = 7.64). The ADOS-2 (German version) (6) was performed by an experienced certified examiner. One participant was assessed with ADOS module 2 (total score 6), 7 participants with module 3 (total score M = 6.14; SD = 2.91; range: 3-12), and one participant with module 4 (total score 5). This indicates that the autistic symptoms observed in this sample were of a moderate severity. The subject’s IQ ranged from 81 to 122 (M = 103.8; SD = 12.25). A single participant was diagnosed with ASD following the conclusion of the diagnostic process.
Video recording and processing
The children were video recorded during ADOS sessions. The video was recorded using the front camera of an Apple iPad Pro (4th generation, iOS 17.3.1), wall-mounted at eye level in a distance of around 160 cm to the child’s face (see Figure 1). The tablet screen was covered to ensure minimal distraction.
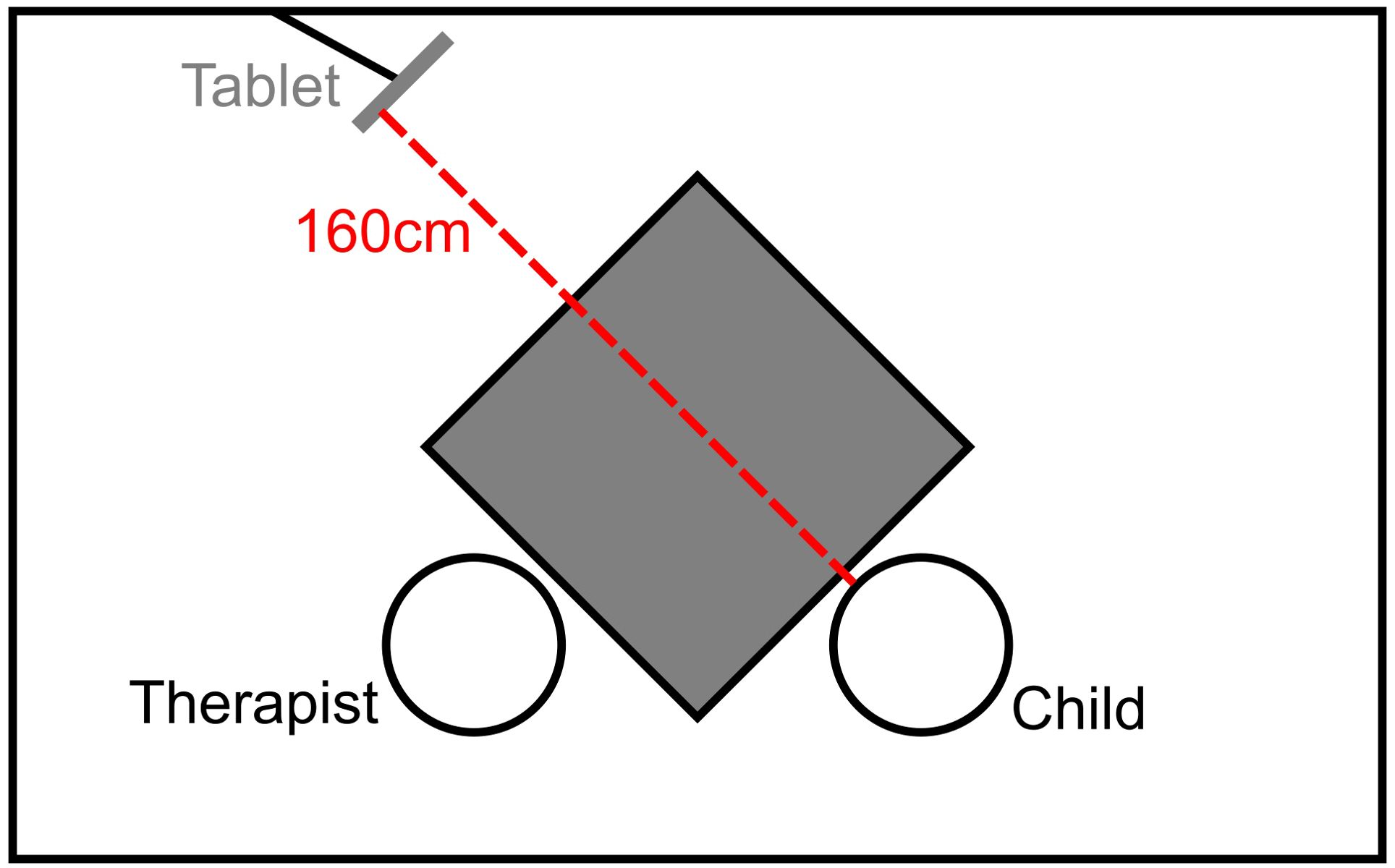
Figure 1. Schematic illustration of the recording setting. The therapist conducting the ADOS and the child were sitting across the corner of a table. The tablet was oriented towards the child’s face, and parallel to the edge of the table.
A FER software developed within the IDEAS project was applied for data acquisition. The software is based on the Apple ARKit, which enables real-time face tracking and identification of facial features in form of a 3D face mesh. This face mesh is normalized and converted into 57 blendshapes (representing 52 facial expressions) ranging in value from 0 (neutral position) to 1 (pronounced expression), as well as five head position parameters (see Figure 2). Blendshapes were recorded with 10 fps.
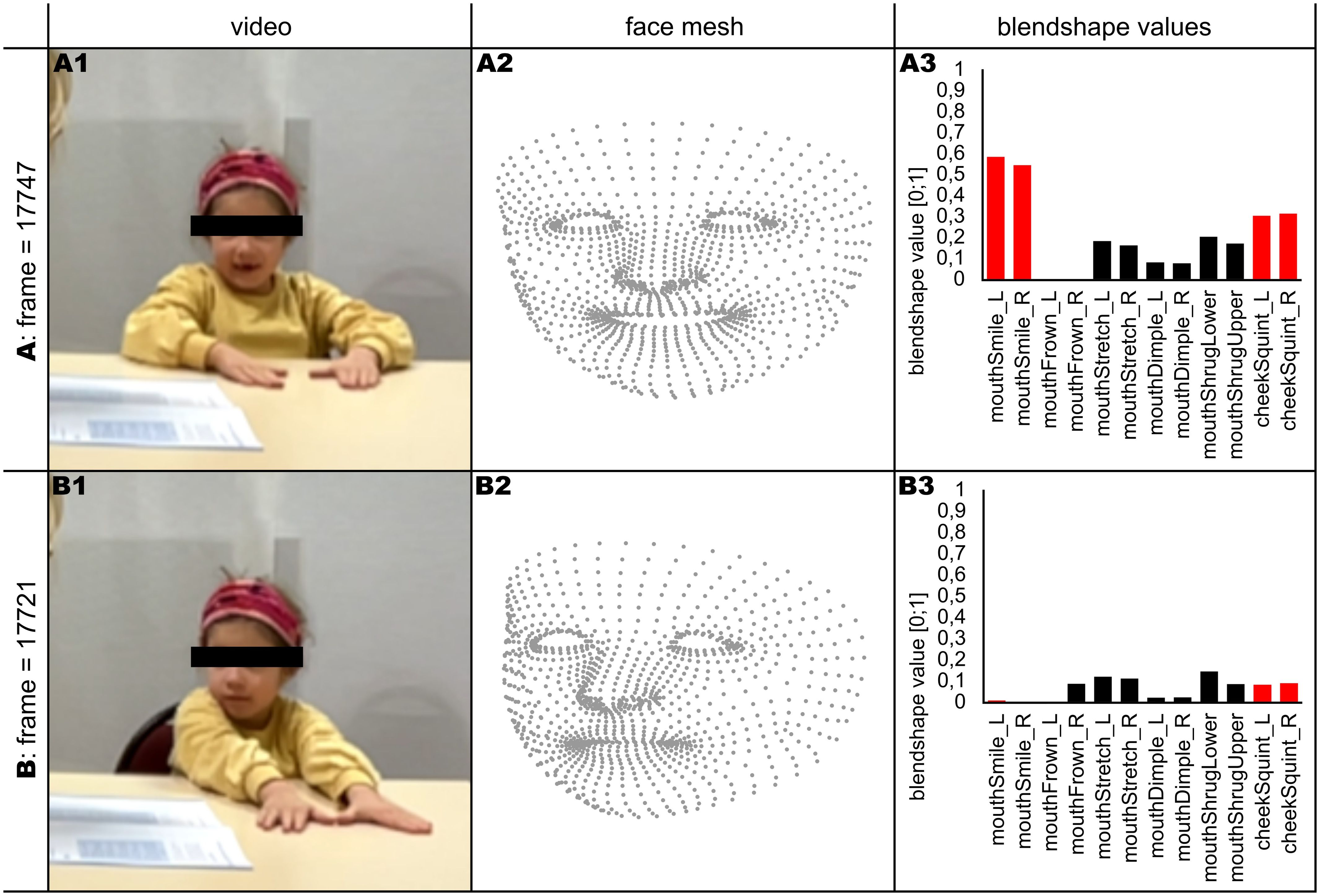
Figure 2. Data of two example frames (A, B) A1/B2 show the video image of the respective frame. A2/B2 show the mesh of the recorded face coordinates, from which blendshape values are derived. A3/B3 show a selection of blendshape values for the respective frame. Higher values indicate a more pronounced expression, lower values indicate a more neutral expression. The blendshapes of interest (mouthSmile_L, mouthSmile_R, cheekSquint_L and cheekSquint_R) are highlighted in red.
From the ADOS videos of our nine participants, 158 video segments with a length of 5 seconds were selected by one of the authors, including smiling and neutral expressions. In these segments, the participants’ faces and facial features were clearly visible, i.e. faces were not covered by hands or objects, with frontal to semi-profile head rotation. The video segments were randomly assembled into one video file, which resulted in a continuous sequence of scenes.
Five human raters – who had no insight into the study design – were instructed to indicate whether a smile event (1) or a non-smile event (0) occurred in each segment. In the written instructions for the raters, we explained that every smile should be reported, regardless of the social function (e.g. embarrassed, socially desirable, inappropriate, joyful smile). Inter-rater agreement was very high, as indicated by a Fleiss’ Kappa of κ = 0.9101 (95% CI [0.8620, 0.9582]; p < 0.001). In the case of disagreement (n = 16), the majority vote applied. Human rating was set as the true class label, resulting in a binary coding for every of the 158 cases (0 = non-smile event, 1 = smile event).
Threshold optimization for machine classification
The data were processed using Matlab (Version R2023a, MathWorks) (14). A random selection process was employed to allocate 50% of the smile and non-smile instances to the training data set and 50% to the test data set. This ensured that the participants and the smile/non-smile events in the two subsets were distributed as evenly as possible.
Studies on smile recognition in healthy individuals found that AU6 (cheek raiser) and AU12 (lip corner puller) are most strongly associated with the expression of joy (15, 16). In the further analysis, we thus focused on four blendshapes (cheekSquintLeft, cheekSquintRight, mouthSmileLeft and mouthSmileRight) representing bilateral AU6 and AU12. The blendshapes of the left and right halves of the face were linked by a logical ‘or’ operation to compute classifiers that are more robust to changes in head position. For instance, the mouthSmile classifier was true, if either mouthSmileLeft ‘or’ mouthSmileRight exceeded the threshold. The same operation was applied for the cheekSquint classifier. For the 79 training cases, the receiver operating characteristics (ROC) were calculated for each classifier (see Figure 3). The human rater consensus was used as the true class label (smile/non-smile event). For ROCs, threshold values from 0 to 1 were employed, with an increment of +0.001 per step. The optimal threshold was identified through the application of Youden’s J statistic. The area under the curve (AUC) was calculated using trapezoidal numerical integration.
Evaluation of the machine classification
The classifiers determined from the training data set were applied to the test data set. Sensitivity and specificity of the FER were calculated for each classifier. Cohen’s Kappa was used to assess the degree of agreement between the machine classification and the human rating.
Results
Receiver operating characteristics
ROCs (see Figure 3) show that a classification based on mouthSmile yielded a perfect classifier for the training data (AUC = 1). At the optimal threshold of 0.395 a true positive rate (TPR) of 1 was achieved, while the false positive rate (FPR) remained at 0. Classification based on cheekSquint demonstrated the capacity to differentiate between smile and non-smile events, but with lower accuracy (AUC = 0.966, TPR = 0.892, FPR = 0.098).
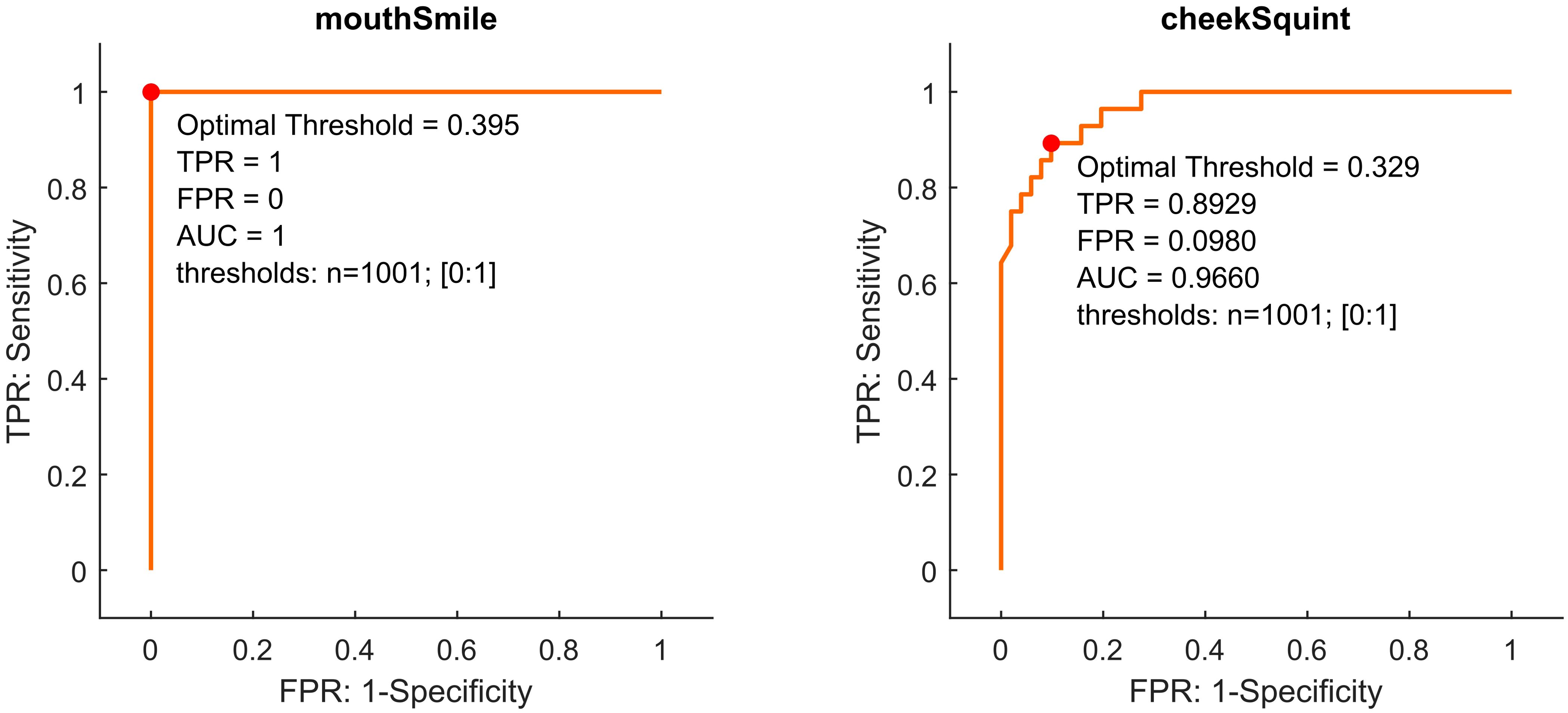
Figure 3. Receiver Operating Characteristics (ROC) of the classifiers mouthSmile and cheekSquint. The y-axis shows the true-positive rate (TPR) and the x-axis shows the false-positive rate (FPR) for each threshold. The area under the curve (AUC) of the ROC value of mouthSmile is equal to one and is therefore an excellent classifier for our training data.
Comparison of machine and human classification
The mouthSmile classifier (= either mouthSmileLeft or mouthSmileRight above threshold value) with the optimal threshold of 0.395 was employed to evaluate the test data set (n=79).
This resulted in a sensitivity of 0.964 and a specificity of 0.961, based on a confusion matrix with 49 true negatives, 27 true positives, 2 false positives, and 1 false negative. The degree of agreement between the machine classification and the human rating was assessed with Cohen’s Kappa, resulting in a value of κ = 0.9177 (95% CI [0.8263, 1.0090]; p < 0.001), indicating a very high level of agreement.
Discussion
The key findings of the study are the following: [i] Smile events can be identified automatically with high sensitivity/specificity in selected sequences from ADOS videos, comparable with human ratings. [ii] Blendshapes representing the mouth corner puller are particularly robust to identify smile events. These results confirm the above hypotheses.
The aim of this pilot study was to demonstrate that the identification of smile events in an ASD diagnostics utilization population work reliable with automated FER.
Applied in a naturalistic setting, FER is challenging because the recording conditions are constantly changing: some children show a strong restlessness of movement, faces are occasionally covered by objects, hair or hands, the distance between camera and child is inconstant, and finally facial expressions are more subtle and spontaneous.
Thus, in this first step, an experimental setup was chosen that reduced interfering variables on the measurement. Only verbalized children with age > 6 years were included; video sequences were selected showing the probands faces completely and at an angle favourable for data acquisition. Moreover, unambiguous facial expressions were chosen (smile event, non-smile event). The results are therefore not generalizable and more extensive data collection is needed.
There are several studies that have examined automated recognition of basic emotions in healthy, predominantly adult subjects (15, 16). The facial expression of joy was recognized most reliably compared to other basic emotions. An extensive analysis of different video databases revealed that technical characteristics of each database (e.g. size of the visual field, extent of head rotation and movement) had a significant impact on recognition accuracy (15). Posed, prototypical expressions were recognized more reliably than spontaneous expressions (15). A study with 30 participants reported predictive accuracy of automatic identification of spontaneous smile events (based on AU6 and AU12) with a specificity of 82.9% and a sensitivity of 89.7% (16). Misclassified smiles were mostly related to covering the mouth and due to yawning. A study examining the difference between spontaneous and posed smile events reported differences between minors and adults (17).
Compared to the aforementioned studies, the FER in our study recognized smile events with a higher specificity and sensitivity. This is probably due to the more restrictive experimental conditions in our study. We investigated the identification of the facial expression of joy (smile event) in contrast to a neutral facial expression (non-smile event). Since the facial expressions of individual basic emotions overlap to some extent, studies that include videos of all basic emotions might probably achieve poorer results. The use of Apple ARKit, which allows to measure facial expressions with particular precision, might as well have made a secondary contribution to the positive key values.
In the present study, video snippets displaying a prototypical facial expression were analysed. An alternative approach could be to analyse the complete ADOS video datasets after prior annotation of the smile events by human raters. A key benefit of the proposed approach is that the technical feasibility of identifying smile events by FER can be readily substantiated. Furthermore, the determination of threshold values for the FER is more straightforward in a favourable recording position. A disadvantage of the comparison of the approaches is the poor generalisability of the results to the full ADOS situation and the lack of evidence for the reliability of the FER under unfavourable recording conditions.
In some studies, FER was applied to patients with ASD. For instance, Bangerter et al. found reduced mimic response behaviour (AU12, AU6) to an amusing video task in individuals with ASD compared to healthy controls (18). The study used software based on Computer Expression Recognition Toolbox (CERT) which, according to the authors, achieved an accuracy of 90.1% for a database of posed facial expressions and almost 80% for a dataset of spontaneous facial expressions. Ahn et al. used a first-person video approach by means of data glasses worn by parents and examiners to investigate smile events of 61 infants with ASD during an ADOS session (19). These data were also analyzed using the CERT-toolbox. Children whose gaze at their parents included more smiles received lower social affect severity scores (19). In a multicentre study, Perochon et al. investigated whether 49 children with ASD out of N=475 infants can be correctly classified using the app SenseToKnow (4). In addition to many other parameters, the complexity of mouth movement, measured with automated FER, was included in the assessment. The classification algorithm, combining multiple digital phenotypes based on machine learning, showed high diagnostic accuracy (area under the receiver operating characteristic curve = 0.90, sensitivity = 87.8%, specificity = 80.8%). These encouraging results illustrate the high potential of FER for ASD diagnostics.
This proof-of-principle study has shown that iOS-based automated recognition of smile-events in sequences from naturalistic ADOS-2 videos is successful in principle. Further investigations are required to verify whether identification of smile-events is successful in ADOS-segments under difficult conditions (e.g. profile recordings, low resolution of the recordings, partial facial masking). Moreover, it will be investigated which contribution the presented FER may provide for the identification of children at risk of ASD. The identification and quantification of smile events in non-verbal communication (e.g. as reciprocal smiles) might be of particular importance. There are many conceivable areas of application for automated facial expression analysis in ASD diagnostics, for example as a supplement to the standard questionnaire-based autism screening or as an objective assistance system for analyzing ADOS examinations. The results described are preliminary and only partially utilize the possibilities of FER. In particular, a dedicated analysis of pooled data sets and AI-based identification of discrepant features of social communication open up new, promising approaches.
Strengths and limitations
This study demonstrated that automated identification of smile events in selected video sequences of a naturalistic ADOS-2 setting is both possible and reliable. This was the aim of this proof-of-principle study.
This pilot study is subject to various limitations: 1) Only video sequences with clear, prototypical smile events in a predominantly frontal view were selected, 2) narrow inclusion criteria (verbalization, age range, no cognitive impairment) were applied, 3) participants studied showed moderate ASD symptoms and did not meet ASD diagnostic criteria. Thus, results cannot be generalized all ADOS-2 participants or –ADOS-2 settings. Nevertheless, the results point to interesting opportunities and research desiderata regarding the use of advanced FER technology in the clinical setting.
Data availability statement
The raw data supporting the conclusions of this article will be made available by the authors, without undue reservation.
Ethics statement
The studies involving humans were approved by the Ethics Committee of Leipzig University, Faculty of Medicine (438/22-ek). The studies were conducted in accordance with the local legislation and institutional requirements. Written informed consent for participation in this study was provided by the participants’ legal guardians/next of kin. Written informed consent was obtained from the individual(s)'s legal guardians/next of kin for the publication of any identifiable images or data included in this article.
Author contributions
MD: Conceptualization, Data curation, Formal analysis, Investigation, Methodology, Validation, Writing – original draft, Writing – review & editing, Visualization, Software. UK: Conceptualization, Data curation, Formal analysis, Investigation, Methodology, Validation, Writing – review & editing. JH: Writing – review & editing, Methodology, Conceptualization, Software. HH: Writing – review & editing, Software. NR: Software, Writing – review & editing. SB: Writing – review & editing, Methodology, Conceptualization. KK: Software, Writing – review & editing, Funding acquisition. IN: Writing – review & editing. MB: Software, Writing – review & editing, Funding acquisition, Visualization. Gv: Writing – review & editing. DR: Writing – review & editing, Conceptualization, Data curation, Formal analysis, Funding acquisition, Investigation, Methodology, Project administration, Resources, Supervision, Validation, Visualization, Writing – original draft.
Funding
The author(s) declare that financial support was received for the research and/or publication of this article. The study was funded by the BMBF as part of the KMU-innovativ project IDEAS (grant number: 13GW0584A-E). This publication is supported by the Open Access Publishing Fund of Leipzig University.
Acknowledgments
We are grateful to the Federal Ministry of Education and Research of the Federal Republic of Germany (BMBF) for funding the IDEAS project.
Conflict of interest
Authors MB, HH, JH and NR were employed by the company KIZMO GmbH. The authors declare that KIZMO GmbH is interested in commercializing the software developed by the company. Author KK is employed by company Speechcare GmbH. The author declares that Speechcare GmbH is interested in commercializing the software developed by the company. As part of the IDEAS project, KIZMO GmbH and Speechcare GmbH developed software that was also used in this study. Employees of KIZMO GmbH and Speechcare GmbH were not involved in the study design or final data analysis.
The remaining authors declare that the research was conducted in the absence of any commercial or financial relationships that could be construed as a potential conflict of interest.
Generative AI statement
The author(s) declare that Generative AI was used in the creation of this manuscript. DeepL write was used to review text.
Publisher’s note
All claims expressed in this article are solely those of the authors and do not necessarily represent those of their affiliated organizations, or those of the publisher, the editors and the reviewers. Any product that may be evaluated in this article, or claim that may be made by its manufacturer, is not guaranteed or endorsed by the publisher.
References
1. Peach R, Friedrich M, Fronemann L, Muthuraman M, Schreglmann SR, Zeller D, et al. Head movement dynamics in dystonia: a multi-centre retrospective study using visual perceptive deep learning. NPJ Digit Med. (2024) 7:160. doi: 10.1038/s41746-024-01140-6
2. Friedrich MU, Roenn A-J, Palmisano C, Alty J, Paschen S, Deuschl G, et al. Validation and application of computer vision algorithms for video-based tremor analysis. NPJ Digit Med. (2024) 7:165. doi: 10.1038/s41746-024-01153-1
3. Fan Y, Wang H, Zhu X, Cao X, Yi C, Chen Y, et al. FER-PCVT: facial expression recognition with patch-convolutional vision transformer for stroke patients. Brain Sci. (2022) 12(12):1626. doi: 10.3390/brainsci12121626
4. Perochon S, Di Martino JM, Carpenter KLH, Compton S, Davis N, Eichner B, et al. Early detection of autism using digital behavioral phenotyping. Nat Med. (2023) 29:2489–97. doi: 10.1038/s41591-023-02574-3
5. DGKJP, DGPPN. AWMF S3 guideline. Autism spectrum disorders in childhood, adolescence and adulthood: Part 1: diagnostics(2016). Available online at: https://register.awmf.org/de/leitlinien/detail/028-018 (Accessed August 30, 2024).
6. Poustka L, Rühl D, Feineis-Matthews S, Poustka F, Hartung M, Bölte S. Diagnostische beobachtungsskala für autistische störungen-2 (ADOS-2). In: German Version of: Lord C, Rutter M, DiLavore PC, Risi S, Gotham K, Bishop S. Autism Diagnostic Observation Schedule, Second Edition. Göttingen: Hogrefe (2015).
7. Lord C, Rutter M, DiLavore P, Risi S, Gotham K, Bishop S. Autism Diagnostic Observation Schedule, Second Edition. Torrence (CA): Western Psychological Services. (2012).
8. Kanne SM, Bishop SL. Editorial Perspective: The autism waitlist crisis and remembering what families need. J Child Psychol Psychiatry. (2021) 62:140–2. doi: 10.1111/jcpp.13254
9. Neitzel I, Tuschen L, Ritterfeld U. Automatisierte Sprach-und Sprechanalyse in Forschung und Diagnostik bei Autismus-Spektrum-Störungen: Potenziale und Barrieren. Sprache· Stimme· Gehör. (2023) 27:84–8.
10. IDEAS. (2024). Available online at: www.ideas.care (Accessed August 21, 2024).
11. Ekman P, Friesen WV. Facial action coding system: A Technique for the Measurement of Facial Movement. Palo Alto (Calif: Consulting Psychologists Press (1978).
12. Cheong JH, Jolly E, Xie T, Byrne S, Kenney M, Chang LJ. Py-feat: python facial expression analysis toolbox. Affect Sci. (2023) 4:781–96. doi: 10.1007/s42761-023-00191-4
13. Bölte S, Poustka F. Fragebogen zur sozialen Kommunikation: Autismus-Screening; German Version of: Rutter M, Bailey F, Lord C. Social Communication Questionnaire (SCQ). Göttingen: Hogrefe. (2006).
14. The MathWorks Inc. MATLAB version: R2023a(2023). Available online at: https://www.mathworks.com (Accessed August 20, 2024).
15. Krumhuber EG, Küster D, Namba S, Skora L. Human and machine validation of 14 databases of dynamic facial expressions. Behav Res Methods. (2021) 53:686–701. doi: 10.3758/s13428-020-01443-y
16. Mohammed H, Kumar R, Bennani H, Halberstadt JB, Farella M. Automated detection of smiles as discrete episodes. J Oral Rehabil. (2022) 49:1173–80. doi: 10.1111/joor.13378
17. Dibeklioglu H, Salah AA, Gevers T. "Are you really smiling at me?", In: Fitzgibbon A, Lazebnik S, Perona P, Sato Y, Schmid C, editors. Spontaneous versus Posed Enjoyment Smiles. Computer Vision – ECCV 2012. Lecture Notes in Computer Science, vol 7574. Berlin, Heidelberg: Springer. (2012). doi: 10.1007/978-3-642-33712-3_38
18. Bangerter A, Chatterjee M, Manfredonia J, Manyakov NV, Ness S, Boice MA, et al. Automated recognition of spontaneous facial expression in individuals with autism spectrum disorder: parsing response variability. Mol Autism. (2020) 11:31. doi: 10.1186/s13229-020-00327-4
19. Ahn YA, Moffitt JM, Tao Y, Custode S, Parlade M, Beaumont A, et al. Objective measurement of social gaze and smile behaviors in children with suspected autism spectrum disorder during administration of the autism diagnostic observation schedule, 2nd edition. J Autism Dev Disord. (2024) 54:2124–37. doi: 10.1007/s10803-023-05990-z
Keywords: facial expression recognition, ADOS, autism diagnosis, digital diagnosis, ROC, smile recognition, diagnosis software, early autism diagnosis
Citation: Dotzer M, Kachel U, Huhsmann J, Huscher H, Raveling N, Kugelmann K, Blank S, Neitzel I, Buschermöhle M, von Polier GG and Radeloff D (2025) Identification of smile events using automated facial expression recognition during the Autism Diagnostic Observation Schedule (ADOS-2): a proof-of-principle study. Front. Psychiatry 16:1497583. doi: 10.3389/fpsyt.2025.1497583
Received: 12 November 2024; Accepted: 24 March 2025;
Published: 01 May 2025.
Edited by:
Michela Camia, University of Modena and Reggio Emilia, ItalyReviewed by:
Lisa Asta, University of Modena and Reggio Emilia, ItalySanna Stroth, University of Marburg, Germany
Copyright © 2025 Dotzer, Kachel, Huhsmann, Huscher, Raveling, Kugelmann, Blank, Neitzel, Buschermöhle, von Polier and Radeloff. This is an open-access article distributed under the terms of the Creative Commons Attribution License (CC BY). The use, distribution or reproduction in other forums is permitted, provided the original author(s) and the copyright owner(s) are credited and that the original publication in this journal is cited, in accordance with accepted academic practice. No use, distribution or reproduction is permitted which does not comply with these terms.
*Correspondence: Daniel Radeloff, ZGFuaWVsLnJhZGVsb2ZmQG1lZGl6aW4udW5pLWxlaXB6aWcuZGU=