- NASA Ames Research Center, Moffett Field, CA, United States
Diagnosis of disease from breath signatures comprised of volatile, mostly organic compounds is a promising field of research and medical application that, after more than 4 decades of active research, has yielded far fewer breatkthroughs than its potential initially appeared to promise. The recent pandemic spurred many teams, including ours at NASA, to investigate this approach for a particular application: determining COVID-19 infection status. In this Perspective, we examine the science and technology underlying one means of implementing such diagnostics, the so-called electronic nose, in an implementation named EnCOVID: Electronic nose for Coronavirus Organic Volatiles Infection Detection. We demonstrate an approach to developing application-specific arrays of sensors that balance chemical selectivity with reversibility in a manner well suited to chemically complex mixtures like human breath; the method differs markedly from how single, highly selective sensors are typically chosen. Using a 64-nanosensor array of carbon-nanotube-based chemiresistors, we used SIMCA (soft independent modeling by class analogy) on results from 63 volunteers to show that COVID-19 status, positive or negative, can be correctly classified, relative to gold-standard RT-PCR, 92% of the time. While the data set is too small to generate the critical independent training and testing sets, this classification success is a promising basis upon which to design a larger, more definitive study. We conclude that the portability and possibility of low-cost, high-volume manufacture of an electronic nose-based system may be most valuable for applications outside hospitals and clinics, including situations—like an epidemic—and venues, from airports to sports venues to schools, where large numbers of people have to be screened rapidly, in parallel.
1 Background and constraints
The possibility to identify disease in the human body from breath odor, recognized since ancient times (Hippocrates of Kos, 430 BCE), has been facilitated by the advent of modern oral hygiene: halitosis can complicate respiratory system volatile chemical signatures with unpleasant oral bacterial emissions (Van Den Velde et al., 2007). Detailed quantitative analysis by gas chromatography-mass spectrometry (GC-MS) reveals breath to be chemically complex, with hundreds of volatile organic compounds (VOCs) (Preti et al., 1988; Boots et al., 2012) plus multiple inorganic gases. (Breath also includes non-volatile species, from simple salts to complex proteins, carried within aerosol water droplets; in most breath-sampling systems, these are captured by particulate filters or condensation. Though they provide a wealth of information, the non-volatiles are not considered in this article.) The physiological mechanisms by which VOCs equilibrate between exhaled breath from the lungs and the rest of the body, principally via the circulatory system, has been studied extensively (King et al., 2013). Large differences in the human “volatile-om” or “breathprint,” even among cohorts of individuals with similar genotypes, phenotypes, and states of health, render unambiguous breath-based disease diagnosis highly challenging (Haworth et al., 2022).
Over the past 5 decades, a host of chemical sensors for gas-phase analysis have been researched, developed and demonstrated using a variety of transduction platforms. (Röck et al., 2008). Reversibility at operational temperature—key for sensors to perform continuous or repeated detection—necessitates comparatively weak physicochemical interactions between sensor transduction materials and analyte(s), compromising chemical specificity: chemically sensitive materials, even with features targeting a specific combination of molecular size, shape, polarity, hydrogen bonding, dispersive forces, and other distinguishing characteristics, will adsorb/absorb many if not most (semi)volatile species to some extent. While the extent of such sorption, including its concentration dependence, may differ markedly from compound to compound, a high concentration of an interfering compound that is poorly matched to the sensing material might yield the same response magnitude as a low concentration of the bespoke molecular target. Measuring multiple response characteristics beyond sorbed analyte quantity–e.g., analyte sorption/desorption response times (Post et al., 2022) or associated changes in sensing material mechanical, electrical, magnetic, or optical properties–may enhance chemical specificity without compromising reversibility (Ricco et al., 1985). Another effective selectivity approach is to include a chromatographic “front end” for chemical sensors, providing access to characteristic retention times for analytes at the cost of added calibration, complexity, system size, data processing, and power demand (Bryant-Genevier et al., 2014).
To convert mediocre selectivity from a bug to a feature, sensor arrays—in certain contexts called electronic noses—have been researched and developed extensively (Röck et al., 2008; Janata, 2009; Patel, 2014; Stetter and Penrose, 2002; Li T. et al., 2023). Because concentration-dependent responses are determined by the physicochemical characteristics of both the molecular target(s) and the sensing material, an array of such materials can provide a unique fingerprint for a given chemical compound, or a defined mixture of compounds (coffee, gasoline, wine, human breath, etc.) (Hierlemann and Gutierrez-Osuna, 2008). Importantly, arrays need not include large numbers of sensors: as few as two sensors can, in theory, provide an unlimited number of response combinations. In practice, however, two sensors are likely to be adequate only in certain scenarios (Hoyt et al., 1998), and unlikely to uniquely identify large numbers of compounds or mixtures of similar chemicals.
A broader variety of compounds and mixtures across wider concentration ranges may be identifiable by including more sensor elements, but only when each added element provides unique, or “chemically orthogonal,” information to all the others. Adding sensing elements that provide no unique chemical information is undesirable as each new sensing material costs time and money to develop and to manufacture, raising cost without improving performance. Furthermore, for some mathematical methods of analyzing multidimensional data, dispersing the same chemical information over more response dimensions can be tantamount to adding noise (Osbourn et al., 1998; Röck et al., 2008), leading to less reliable identification or poorer quantitation. Yet some sensor array platforms support tens or hundreds of transduction elements at reasonable cost, size and power and, fortunately, these can be effectively leveraged through “strategic redundancy”: by including three or more copies of each unique sensing material—even tailoring the numbers of redundant elements to signal:noise ratios per sensor element type by including more copies of noisier sensors—the median response per sensing material can provide a higher fidelity, higher reliability response that is also robust to single-sensor-element failure.
With an appropriate array of sensing materials, chemical sensors excel at detecting VOC mixtures, including those in breath, yet they provide no chemical bonding or molecularly specific information in the manner that mass spectrometry or vibrational spectroscopy can. Still, olfaction is not spectroscopy and, in analogy to olfaction (Persaud and Dodd, 1982; Gardner and Bartlett, 1994; Pearce, 1997; Kwon et al., 2015; Cheng et al., 2021), arrays can provide selectivity via multi-sensor response vectors based solely on the VOCs of a given breath mixture having low, moderate, or high affinity for each sensing material of the array. As explained above, application-appropriate chemical diversity of array elements is key (Ricco et al., 1998): by selecting/developing sensing materials with preferential affinity for the most important analytical targets (and, in some cases, choosing other elements with affinity for likely interferents), the output accuracy—clinically, the sensitivity (immunity to false negatives) and specificity (immunity to false positives)—can be enhanced.
Effective design of a sensor array for an application involving highly variable, chemically complex mixtures and variable backgrounds is, then, a balancing act: chemical selectivity and adequate sensitivity must be implemented without sacrificing reversibility. This feat may be difficult or impossible to achieve using “traditional” highly selective single-analyte sensors that require high binding constants to respond to a single low-concentration target in the presence of high-concentration interferents, making them into single-use disposables. But the desired balancing act can be accomplished using a well-chosen set of “sparingly selective” sensors to produce definitive response patterns that unambiguously identify a target analyte or mixture in a manner akin to how creatures such as humans and dogs interpret odors.
Despite the potential and promise of this approach, sensor arrays have found limited success for breath diagnostics (Haworth et al., 2022) High on the list of probable culprits is biological diversity. For example, if the objective is to learn if a person has COVID-19, and considering that the person has a unique genotype, phenotype, and time-varying state of respiratory chemical output, an effective strategy could be to sample and analyze the person’s breath under a wide range of physiologically impactful circumstances: tired vs well rested; thirsty or not; hungry or fed, with a multitude of food choices; healthy or with one or more afflictions, from a common cold to a stressful workday. These measurements would need to be made both when the person is shown by a “gold-standard” test (here, RT-PCR) not to have COVID-19, and then again when that same test returns a COVID-19 positive result. Analysis of the person’s data in n dimensions (for n sensing materials) would then provide the basis for future diagnoses from that lone person’s breath. But such an intensely personalized, intrusive, time-intensive “training” approach is impractical if not ridiculous.
A more reasonable clinical approach is to have a large cohort of genetically and phenotypically diverse volunteers—tens, hundreds, even thousands—stand in for all whose breath might be analyzed for disease by such a system. Testing must include comparable numbers of people confirmed to have the target affliction (at various stages of progression) and “healthy controls” confirmed not to be ill; for all, a wide range of genotypes and phenotypes should be included, as well as various conditions or diseases (comorbidities) that could interfere: respiratory afflictions of all types, diabetes, infections with a range of (non-target) viruses and bacteria, etc. In assessing the resulting data set, it can be productive to look for systematic differences among related sub-cohorts and account for these where practical. For example, if the breathprints of diabetics often include higher levels of ketones, the accompanying questionnaire would allow inclusion of diabetes status as a response parameter to supplement the sensor signals. This information could be used to only compare a diabetic’s breath with that of a cohort of other diabetics, or it could be the basis for modifying the sensitivity coefficients for sensor elements with high responses to ketones. Patient data can be shared across studies without compromising confidentiality.
In the next section, we summarize the approach and recent results of a pre-clinical study classifying breath samples from COVID-positive and -negative volunteers. The size of the study was too small (63 individuals) for an unqualified declaration of success, but the results are encouraging, and they suggest that continued exploration of gas sensor arrays for breath-based disease testing is well warranted.
2 Strategic approach
A direct and effective starting point is assessing the full set of VOCs in the breath of those with a target illness as well as healthy humans; ideally, such information is available, or can be obtained, from GC-MS studies (e.g., Hakim et al., 2012). Generally, the VOCs and inorganic gases for which the average or median difference between healthy and ill is largest, relative to sensor noise and drift, are likely to be (some of) the most helpful targets, though a few compounds that are relatively constant can be quite helpful too. Typical relevant compound classes include alcohols, aldehydes, esters, organic acids, alkanes, alkenes, aromatics, and thiols; of the inorganics, nitrogen oxides (esp. NO and NO2, which equilibrate via O2 reaction under typical breath and sampling conditions), CO, and H2S can be important. Managing humidity in breath is often critical as well, because the concentration of water vapor is much greater than any VOC. Fortunately, H2O interacts weakly with many of the VOC-affinity sensor materials, and water also is more neutral on the redox scale than NOx, CO, and H2S—relevant for sensors that respond via analytes’ electron donor/acceptor properties. A simple, practical approach to managing water vapor is presented with the details of COVID-19 breath analysis below.
Limits of detection (LODs) must reach ppb levels to provide reliable responses for most target VOCs and gases in breath. Choosing at least one sensing material expected to interact well with at least one of each of the dozen or so “most important” VOCs (as defined above) is a good start, recognizing that even a material targeting one particular compound or class (e.g., aldehydes) will usually respond to similar classes (ketones, esters, ethers), though with diminished sensitivity—if the material choice has been well made. Understanding which material provides a desired preferential response follows fundamental chemical principles: like dissolves like. This can be embodied with a single metric such as Hildebrand and Hansen solubility parameters (Barton, 1991; Hansen, 2007), but more sophisticated models, for example the linear solvation energy relationship (LSER) (Kamlet et al., 1983), can also be used. Analytical frameworks based on cluster analysis, neural networks, support vector machines, logistic regression, and a range of other methods can be effective for modeling and predicting responses (Scott et al., 2006; Tan and Xu, 2020); however, since the fundamental chemical interactions and properties of the analytes and sensing materials are not inputs to such models, they do not offer initial guidance (before data have been acquired) as to which materials should be best for which analytes.
Most sensors respond relative to a baseline that is often obtained by analysis of the environment in which the target(s) are to be measured in their absence. For breath, clean ambient air is suitable to generate a sensor blank or baseline. In potential screening applications like airports and sports venues, however, ambient air could include a significant background, much of it generated by humans, with further, unpredictable additions from cleaning chemicals, food preparation, and the like. The particular challenge is that mammalian respiratory systems not only add VOCs to exhaled air, they often also (partially) remove VOCs from inhaled ambient air. A sophisticated sensor system might therefore create its background or blank by scrubbing organics from ambient air in a manner analogous to the respiratory system. However, to the best of our knowledge, such systems have yet to be designed and demonstrated. Relatively clean air can be generated, nonetheless, from ambient air using scrubbing technology (e.g., activated carbon plus particle-capture filters). A silver lining to the pandemic cloud is that many public facilities now filter and exchange air much more thoroughly than pre-2020, making ambient air generally far cleaner than before.
3 Case study: COVID-19 breath assay using a chemiresistor array
The EnCOVID (Electronic nose for Coronavirus Organic Volatiles Infection Detection) (Li J. et al., 2023) is based on a predecessor system developed and refined over more than a decade at NASA’s Ames Research Center (ARC) (Li et al., 2003; 2005; 2006). Figure 1A synopsizes application of this system to the analysis of human breath for the presence of a mix of VOC markers signaling COVID-19 infection. Each volunteer, screened via RT-PCR, filled a single-use 1-L “breath bag” (Figure 1C). Sealed bags were removed to the laboratory before attaching and operating the E-nose analytical system, which is reported in detail elsewhere (Li J. et al., 2023).
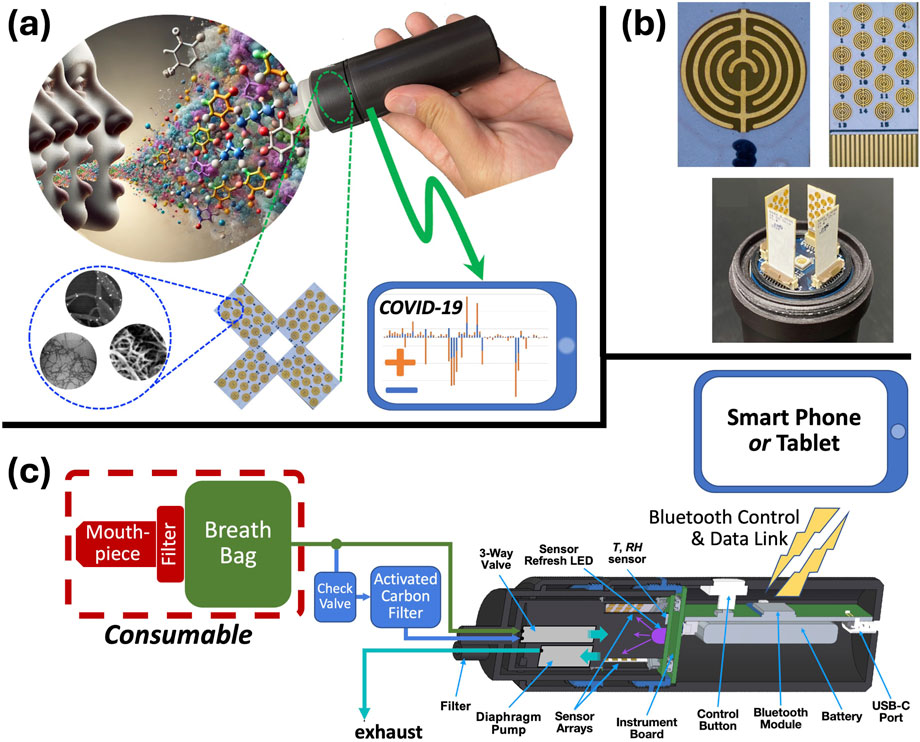
Figure 1. (A) EnCOVID system (black tube) operational approach, with zoomed-in views of four 16-chemiresistor arrays and carbon nanotubes. (B) Closeup of one interdigitated electrode supporting CNT-based thin-film sensors; 16 such IDEs on a ceramic substrate with edge connector; arrangement of four substrates in the sensor head. (C) Functional block diagram of latest EnCOVID system, incorporating pump, valve, filter, and UV LED within the sensor housing, and other components as shown. Graphics adapted from Li J. et al., 2023.
The process by which the EnCOVID system was configured for its target application is illustrative. First, a set of key target breath VOCs was identified; initially, a dozen were selected, along with the inorganic gas “NOx,” following published studies (Shan et al., 2020; Ruszkiewicz et al., 2020; Chen et al., 2021; Ibrahim et al., 2021; Degaudenzi et al., 2021; Snitz et al., 2021; Giovannini et al., 2021; Rodríguez-Aguilar et al., 2021; Wintjens et al., 2021). The organics included an alkane, a diene, an aromatic alkene, three alcohols, three esters, two aldehydes, and a ketone; their concentrations (by volume) in human breath were reported as ranges, varying from as low as 0.3 ppb (isoprene) to as high as 2 ppm (ethyl butanoate). That ester and two other VOCs were reported to increase in breath concentration of COVID-19-infected individuals relative to the uninfected; four VOCs were reported to decrease; the direction of change was not reported for the other compounds. Considering this context, from our array of over 200 available sensors, we selected 64 materials for which experience and fundamental chemical principles suggested differential responses to the target VOCs and NOx would be likely.
The sensing platform is an array of chemiresistors (Figure 1B), a transduction platform that has been utilized over decades in gas/vapor sensors by many researchers and developers, but nonetheless continues to advance due to creative, effective and targeted use of many, sometimes novel, types of materials. For the NASA array system, each interdigitated gold electrode was coated with a thin film comprised of a member of one of four classes of carbon nanotube (CNT)-based conductive material: chemically modified CNTs (e.g., fluorinated, sulfonated, etc.); molecular composites of CNTs and other electronic or redox conductors (e.g., polyaniline, ferrocene, noble metals); nanoparticle (NP)-modified CNTs (using, e.g., SnO2, Ag, and other NPs); and polymer-CNT blends [e.g., poly(epichlorohydrin), poly(isobutylene), hyperbranched fluoroalcohol polycarbosilanes]. This range of CNT-modifying materials all but ensures the existence of a material subset—ideally, a dozen sensors or more—for which the sensing elements are chemically orthogonal to one another in the context of the sensing challenge. The materials, deposited from organic solvent onto the interdigitated electrodes as micrometer-thick films, provide rapid (seconds) responses to the ad/absorption and desorption of VOCs and inorganic gases. All materials were screened for reversibility to ensure the sensors would be reusable for many samples.
Chemiresistors confer advantages of low power, compact size, inexpensive microfabrication, and support for a wide variety of sensing materials—which need not be conductive, provided their transduction process affects an integral material whose conductivity does change as a result. As Figure 1A shows, the compact nature of the sensor elements and their planar layout facilitates integration of tens of them into a flashlight-sized system. This device not only includes 64 chemiresistors and a combination temperature (T)-and-relative-humidity (RH) sensor, it also houses circuitry to monitor sensing element resistance, rechargeable batteries, a Bluetooth interface, and an ultraviolet (UV) light-emitting diode (LED) to help desorb analytes from the sensing elements if necessary. In the system’s most recent implementation, Figure 1C, which is a few cm longer than shown in Figure 1A, an internal 3-way valve and air pump are also included within the housing. The valve selects between the breath-sampling connection and a separate connection to background ambient (or “zero”) air, created from ambient air using an N99 spirometry filter (Li J. et al., 2023). The pump draws zero air across the sensor array and, when the valve changes state, provides 30 s of breath sample, followed by return to zero air.
Temptation to use such a device to directly sample breath blown at or into it must be tempered by the challenge presented: a reliable zero baseline vastly improves identification and quantitation of VOCs in samples, as does a repeatable, constant gas flow rate across all sensors for both the zero air and the breath sample. Further, with an infectious disease like COVID-19, disinfecting the sensor device after each use is challenging. These factors motivated implementation of a 1-L, single-use Tedlar “breath bag” to collect and store each volunteer’s breath sample. A particle filter at the mouthpiece blocks aerosols, bacteria, and viruses.
Using the combined filter-and-breath-bag approach provides pathogen control, allows near-real-time or delayed (up to several hours) analysis, even as it enables very reproducible flow rates and exposure times. It also provides an unanticipated benefit: relative humidity (RH) management. Storage of breath samples in Tedlar bags for 20 min to 24 h was found to provide consistent RH in a band from the upper 50%’s to the low 70%’s—a range of ∼15 RH percentage points—at ambient temperature. Storage in the bag equilibrates its surfaces with breath-sample RH, bringing it close to ambient RH (which, in our laboratories, usually ranges from 40% to 75%).
3.1 COVID-19 breath assay: Results and analysis
Initial test results for six CNT-based sensors are shown in Figure 2A for exposure to nitric oxide diluted in N2 to breath-relevant concentrations (20 ppb–1.5 ppm). Similar testing with the other 58 sensors confirmed significant sensitivity to NO, as well as reversibility, for many of the materials. Similar experiments with the 12 VOCs confirmed adequate sensitivity, dynamic range, and reversibility. A sequence of nine-VOC mixtures was tested with the sensors. The quantified responses of a 16-sensor subset is shown in Figure 2B for the mixtures defined in the figure. Altered responses occur even if just two of the nine VOCs in the mixture change concentration. The results typified by Figures 2A,B guided us in selecting the set of 64 sensors to use for human breath tests from more than 200 available sensing nanomaterials in our labs, the vast majority of them made from commercially available raw materials mixed or modified to produce the sensing materials detailed elsewhere (Li J. et al., 2023).
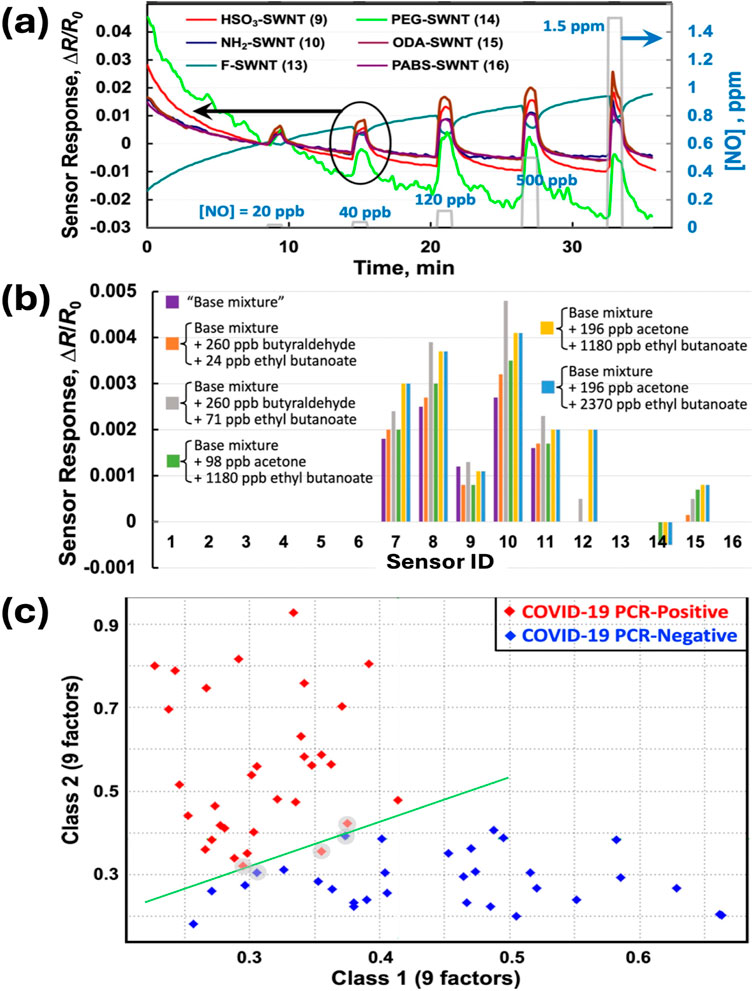
Figure 2. (A) Response, expressed as fractional change in sensor resistance (∆R/R0, left axis) of half a dozen of the most responsive CNT-based chemiresistors to various nitric oxide concentrations (right axis); sensor materials include bisulfato-, amino-, fluoro-, poly(ethylene glyco)-, octadecylamino (ODA)-, and polyaminobenzene sulfonic acid (PABS)-modified single-walled carbon nanotubes (SWNTs). (B) Responses from 16 sensors (eight of which provide responses exceeding three times noise) to six VOC mixtures; the 16 sensor materials are identified in Li J. et al., 2023. The “Base Mixture,” comprised of 10 ppm ethanol, 200 ppb styrene, 150 ppb n-propanol, 140 ppb 1,1-dipropoxypropane, 130 ppb isoprene, 130 ppb propyl acetate, and 10 ppb methyl methacrylate, remained constant throughout, while concentrations of butyraldehyde, ethyl butanoate, and acetone varied as shown in the figure. (C) Nine-factor SIMCA analysis of EnCOVID system response to COVID-19-positive (red) and -negative (blue) breath samples from 63 volunteers; green line separating the two classes was defined after removing five points (greyed in the figure) whose classification was ambiguous when using a simple linear class separator. Graphics adapted from Li J. et al., 2023.
Collection of breath data from healthy and COVID-19-infected volunteers using the EnCOVID system in cooperation with Stanford University Medicine (Li J. et al., 2023) resulted in a 64-dimensional response vector per sample that belonged to one of the two classes established by RT-PCR testing: COVID-19-positive or -negative. The number of mathematical methods suitable for analyzing such array responses are too many and varied to summarize here, but have been reviewed (Scott et al., 2006; Röck et al., 2008; Hierlemann et al., 1996; Raman et al., 2011). We evaluated SIMCA (soft independent modeling by class analogy), a supervised classification statistical method, based largely on principal-component analysis, as well as support-vector machine (SVM) and logistic regression approaches. Both SVM and SIMCA were found to be well suited to this challenge. These methods, like most others, provide better results if the S:G (“signal-to-garbage”) ratio is improved by a combination of signal processing and curation before analysis. Here, the term “garbage” includes both short-term noise (on the timescale of the measurement) and long-term drift, both of which can be minimized via conventional signal processing and averaging methods. A second, very important category of “garbage” is invalid responses, particularly those whose validity the operator has reason to question: e.g., the connecting tubing was found kinked after a run; the unit stopped functioning near the end of a run due to discharged batteries; a volunteer appeared to be hyperventilating just before filling the breath bag; and so on. The need to discard such data sets may appear obvious, but it is surprising how often this important screen-for-data-validity step is omitted. In a fully developed system, multiple sensors and tailored algorithms should “keep watch” on both hardware and response data to automatically reject such runs, but in preliminary testing, this falls to the operators, who must be made aware of this responsibility. Before analysis, EnCOVID data sets confirmed to be valid were then detrended and normalized to further improve the S:G ratio.
Figure 2C summarizes COVID-19 breath analysis results from 63 volunteers (by PCR, 32 of them COVID-19 positive, 31 of them COVID-19 negative) using nine-factor SIMCA analysis, demonstrating that 2D separation of the two classes is feasible (Figure 2C). Removing five “uncomfortably close” responses (greyed points) results in consistent classification for the remaining 58 of the 63 responses with a simple linear boundary between the two classes (green line), a promising 92% of the samples.
Qualitative analysis of the responses of the 64 CNT-based sensors revealed a 20-sensor subset doing a disproportionate share of the classification work: they were sensitive to at least several of the dozen VOCs over a broad range of ppb-level concentrations. Of those 20, ∼12 showed exceptional sensitivity to very low concentrations, detecting 2–5 ppb reliably. At the same time, an overlapping subset of nine materials provided atypically large responses to a few specific VOCs, bolstering array selectivity (Li J. et al., 2023). Overall, 21 of the 64 sensing materials were “exceptional performers” in one or more of the ways listed, suggesting that a smaller array size could be quite effective.
4 Conclusions: Room to improve and path forward
Making use of fewer unique sensing materials might not just be adequate, but better: given hardware to support 64 sensors (or another large number) without undue size and power requirements, a set of (say) 21 unique sensors could be implemented in triplicate. Such redundancy not only costs 3× less than developing 64 unique sensing materials for reproducible manufacture, it is robust to single-element failure: the median value of each triplicate set finds their most-agreed-upon response, which, on average, is closer to the true value than the response of a lone sensor, and single outliers are rejected. With larger arrays offering greater redundancy, outliers can be rejected first, and then the valid values per sensor type averaged to maximize accuracy. Importantly, this approach disperses the available chemical information across fewer dimensions than using a larger number of different but chemically redundant sensors, while also enabling materials that may contribute only noise to be eliminated. Our future work will focus on this approach.
The second ripe-for-improvement aspect of such analysis is common to many diagnostics that utilize biomarkers whose levels are determined both by a variety of human conditions and ailments, and by their naturally occurring analytical range within the healthy populace, owing to genotypical and phenotypical differences. A starting point is to gather information for personal factors likely to impact the respiratory system: co-morbidities such as diabetes, chronic obstructive pulmonary disease (COPD), asthma, and obesity; lifestyle factors like smoking, drinking alcohol, and diet; and basic human differences that often influence one’s normal range of a given biomarker, like age and gender. To understand how such factors, individually and in combination, impact where a “green line” is drawn to separate ill from healthy (as in Figure 2C), will require clinical testing of at least 10 times, if not 100 times, the 63-person sample reported here (Li J. et al., 2023).
The need for larger clinical studies is, arguably, a key factor obstructing widespread adoption of gas-sensor-array technology for medical diagnostics. Measuring the breath volatilome associated with a physiologically complex ailment such as cancer or viral infection, including pinpointing the type of cancer or specific virus, even the specific viral strain—and, often, estimating the severity of the ailment as well—and doing this in a manner relevant to a supermajority of the humans on Earth, is daunting. “Precision medicine” is not just a matter of tailoring treatments to individuals; it must also customize the diagnostic process that precedes therapy. To do this properly, hundreds to thousands of volunteers may have to contribute their breath and summarize key relevant aspects of their phenotype and genotype via a questionnaire. Sensor data must be carefully validated for each sample, followed by corrections for signal noise and drift, to obviate the “garbage-in/garbage-out” possibility that can obscure effective diagnostic potential.
A second, subtler factor limiting diagnostic adoption of gas sensor arrays may be failure to choose the right tool for the job. Breath-borne concentrations of gases such as hydrogen, nitric oxide, and various ketones are routinely measured as they are diagnostically informative in analogy to bloodstream levels of potassium, C-reactive protein, or creatinine, i.e. they must be considered in concert and in context with many other biochemical and physiological indicators. Measuring single VOCs or inorganic gases, however, requires neither the hardware cost and complexity of GC-MS, nor the signal-processing-and-analysis complexity of an electronic nose.
A system like EnCOVID is most promising when the diagnostic target is a complex mixture in which no single chemical compound is of particular interest, but the overall combination or pattern of VOCs matters most, and where this pattern differs meaningfully between those who are infected or ill and those who are not. Some types of cancer and certain classes of infections, arguably, are examples of this situation. For them, the case study described here is only one of many examples of the potential of breath-based diagnostics (Kim et al., 2012; King et al., 2013; Haworth et al., 2022; Dixit et al., 2021; Scheepers et al., 2022) that await validation via appropriately sized clinical studies with appropriate management of data and results. An assessment of the suitability of breath analysis for the management of Long COVID is a recent relevant example (Díaz de León-Martínez et al., 2024). The portability and potential for low-cost, high-volume manufacture of an electronic nose-based system may also prove to be of greater value when applications move out of hospitals and clinics to include situations—like an epidemic—and venues like airports, shopping malls, sports venues, office buildings, and schools, where large numbers of people may have to be screened rapidly, and in parallel.
Data availability statement
Publicly available datasets were analyzed in this study. This data can be found here: https://doi.org/10.1021/acssensors.3c00367.
Author contributions
AR: Conceptualization, Formal Analysis, Funding acquisition, Investigation, Methodology, Visualization, Writing–original draft, Writing–review and editing. AM: Conceptualization, Data curation, Formal Analysis, Investigation, Methodology, Validation, Visualization, Writing–review and editing. JL: Conceptualization, Data curation, Formal Analysis, Funding acquisition, Investigation, Methodology, Project administration, Resources, Supervision, Validation, Writing–original draft, Writing–review and editing.
Funding
The author(s) declare that financial support was received for the research and/or publication of this article. Technical work to develop and test the EnCOVID system was supported by the US Dept. of Health and Human Services under HHS-NASA interagency agreement No. SAA5-2020-4-I32973. Support for AJR to draft this Perspective was provided by NASA ARC’s Programs and Projects Directorate.
Acknowledgments
The authors gratefully acknowledge the additional NASA ARC team members who carried out the development, study and analysis summarized in this Perspective, including Luke A. Idziak, Adwait Sahasrabhojanee, Khoa Ngo, John P. Abdou, Nghia Mai, and Walter Alvarado.
Testing of the EnCOVID system for breath analysis of volunteers with and without active SARS-CoV-2 infection was conducted at Stanford Medicine under the leadership and direction of Prasanthi Govindarajan and Yvonne A. Maldonado of Stanford University’s School of Medicine, with additional supervision and participation by Rosita Thiessen, Jonathan Altamirano, Emma Stainton, Marcela Lopez, Grace K-Y Tam, Leanne Chun, Sherry Carrington, Frank Zhou, Youyou Duanmu, Milana Trounce, Simone Schubert, and Dolores B. Cunanan.
Design and fabrication of the hardware and integral software for the EnCOVID electronic nose system was carried out under NASA contract by Variable, Inc., under the leadership of George Yu.
Conflict of interest
The author(s) declared that they were an editorial board member of Frontiers, at the time of submission. This had no impact on the peer review process and the final decision.
Generative AI statement
The author(s) declare that no Generative AI was used in the creation of this manuscript.
Publisher’s note
All claims expressed in this article are solely those of the authors and do not necessarily represent those of their affiliated organizations, or those of the publisher, the editors and the reviewers. Any product that may be evaluated in this article, or claim that may be made by its manufacturer, is not guaranteed or endorsed by the publisher.
References
Barton, A. F. M. (1991). Handbook of solubility parameters and other cohesion parameters. 2nd Edn. Boca Raton: CRC Press.
Boots, A. W., van Berkel, J. J., Dallinga, J. W., Smolinska, A., Wouters, E. F., and van Schooten, F. J. (2012). The versatile use of exhaled volatile organic compounds in human health and disease. J. Breath. Res. 6, 027108. doi:10.1088/1752-7155/6/2/027108
Bryant-Genevier, J., Scholten, K., Kim, S. K., and Zellers, E. T. (2014). Multivariate curve resolution of co-eluting vapors from a gas chromatograph with microsensor array detector. Sens. Actuators B Chem. 202, 167–176. doi:10.1016/j.snb.2014.05.049
Chen, H., Qi, X., Zhang, L., Li, X., Ma, J., Zhang, C., et al. (2021). COVID-19 screening using breath-borne volatile organic compounds. J. Breath. Res. 15, 047104. doi:10.1088/1752-7163/ac2e57
Cheng, L., Meng, Q. H., Lilienthal, A. J., and Qi, P. F. (2021). Development of compact electronic noses: a review. Meas. Sci. Tech. 32, 062002. doi:10.1088/1361-6501/abef3b
Degaudenzi, A. V., Hernandez, D. M., Hidalgo, D., Contreras, E., Ceballos, M. L., Polanco, M., et al. (2021). SARS-CoV-2 breath tests implementation for the rapid COVID-19 surveillance: a game changer? – a review of existing data. Inter. Amer. J. Med. Health 4, 1–6. doi:10.31005/iajmh.v4i.198
Díaz de León-Martínez, L., Flores-Rangel, G., Alcántara-Quintana, L. E., and Mizaikoff, B. (2024). A review on long COVID screening: challenges and perspectives focusing on exhaled breath gas sensing. ACS Sens. 9, doi:10.1021/acssensors.4c02280
Dixit, K., Fardindoost, S., Ravishankara, A., Tasnim, N., and Hoorfar, M. (2021). Exhaled breath analysis for diabetes diagnosis and monitoring: relevance, challenges and possibilities. Biosens 11, 476. doi:10.3390/bios11120476
Gardner, J. W., and Bartlett, P. N. (1994). A brief history of electronic noses. Sens. Actuators B Chem. 18, 210–211. doi:10.1016/0925-4005(94)87085-3
Giovannini, G., Haick, H., and Garoli, D. (2021). Detecting COVID-19 from breath: a game changer for A big challenge. ACS Sens. 6, 1408–1417. doi:10.1021/acssensors.1c00312
Hakim, M., Broza, Y. Y., Barash, O., Peled, N., Phillips, M., Amann, A., et al. (2012). Volatile organic compounds of lung cancer and possible biochemical pathways. Chem. Rev. 112, 5949–5966. doi:10.1021/cr300174a
Haworth, J. J., Pitcher, C. K., Ferrandino, G., Hobson, A. R., Pappan, K. L., and Lawson, J. L. D. (2022). Breathing new life into clinical testing and diagnostics: perspectives on volatile biomarkers from breath. Crit. Rev. Clin. Lab. Sci. 59, 353–372. doi:10.1080/10408363.2022.2038075
Hierlemann, A., and Gutierrez-Osuna, R. (2008). Higher-order chemical sensing. Chem. Rev. 108, 563–613. doi:10.1021/cr068116m
Hierlemann, A., Schweizer-Berberich, M., Weimar, U., Kraus, G., Pfau, A., and Göpel, W. (1996). Pattern recognition and multicomponent analysis. Sens. Update 2, 119–180. doi:10.1002/1616-8984(199610)2:1<119::aid-seup119>3.0.co;2-6
Hippocrates of Kos, 430 BCE, in Goldberg, H. S. (1963). “Hippocrates, Father of Medicine.” (New York: Franklin Watts).
Hoyt, A. E., Ricco, A. J., Bartholomew, J. W., and Osbourn, G. C. (1998). SAW sensors for the room-temperature measurement of CO2 and relative humidity. Anal. Chem. 70, 2137–2145. doi:10.1021/ac971095z
Ibrahim, W., Cordell, R. L., Wilde, M. J., Richardson, M., Carr, L., Dasi, A. S. D., et al. (2021). Diagnosis of COVID-19 by exhaled breath analysis using gas chromatography−mass spectrometry. ERJ Open Res. 7, 00139–02021. doi:10.1183/23120541.00139-2021
Kamlet, M. J., Abboud, J. L. M., Abraham, M. H., and Taft, R. W. (1983). Linear solvation energy relationships. 23. A comprehensive collection of the solvatochromic parameters, pi.*, alpha., and. Beta., and some methods for simplifying the generalized solvatochromic equation. J. Org. Chem. 48, 2877–2887. doi:10.1021/jo00165a018
Kim, K. H., Jahan, S. A., and Kabir, E. (2012). A review of breath analysis for diagnosis of human health. Trend. Anal. Chem. 33, 1–8. doi:10.1016/j.trac.2011.09.013
King, J., Koc, H., Unterkofler, K., Teschl, G., Teschl, S., Mochalski, P., et al. (2013). “Physiological modeling for analysis of exhaled breath,” in Volatile biomarkers: non-invasive diagnosis in physiology and medicine. Editors A. Amann, and D. Smith (Amsterdam: Elsevier), 27–48.
Kwon, O. S., Song, H. S., Park, S. J., Lee, S. H., An, J. H., Park, J. W., et al. (2015). An ultrasensitive, selective, multiplexed superbioelectronic nose that mimics the human sense of smell. Nano Lett. 15, 6559–6567. doi:10.1021/acs.nanolett.5b02286
Li, J., Hannon, A., Yu, G., Idziak, L. A., Sahasrabhojanee, A., Govindarajan, P., et al. (2023). Electronic nose development and preliminary human breath testing for rapid, non-invasive COVID-19 detection. ACS Sens. 8, 2309–2318. doi:10.1021/acssensors.3c00367
Li, J., Lu, Y., and Meyyappan, M. (2006). Nano chemical sensors with polymer-coated carbon nanotubes. IEEE Sens. J. 6, 1047–1051. doi:10.1109/jsen.2006.881018
Li, J., Lu, Y., Ye, Q., Cinke, M., Han, J., and Meyyappan, M. (2003). Carbon nanotube sensors for gas and organic vapor detection. Nano. Lett. 3, 929–933. doi:10.1021/nl034220x
Li, J., Lu, Y., Ye, Q., Delzeit, L., and Meyyappan, M. (2005). A gas sensor array using carbon nanotubes and microfabrication technology. Electrochem. Sol. State Lett. 8, H100. doi:10.1149/1.2063289
Li, T., Zhu, X., Hai, X., Bi, S., and Zhang, X. (2023). Recent progress in sensor arrays: from construction principles of sensing elements to applications. ACS Sens. 8, 994–1016. doi:10.1021/acssensors.2c02596
Osbourn, G. C., Bartholomew, J. W., Ricco, A. J., and Frye, G. C. (1998). Visual-empirical region-of-influence pattern recognition applied to chemical microsensor array selection and chemical analysis. Accounts Chem. Res. 31, 297–305. doi:10.1021/ar970070j
Patel, H. K. (2014). The electronic nose: artificial olfaction technology. New Delhi: Springer India.
Pearce, T. C. (1997). Computational parallels between the biological olfactory pathway and its analogue ‘The Electronic Nose’: Part II. Sensor-based machine olfaction. Biosys 41, 69–90. doi:10.1016/s0303-2647(96)01660-7
Persaud, K., and Dodd, G. (1982). Analysis of discrimination mechanisms in the mammalian olfactory system using a model nose. Nature 299, 352–355. doi:10.1038/299352a0
Post, N., Bender, F., Josse, F., and Ricco, A. J. (2022). Application-specific adaptable coatings for sensors: using a single polymer–plasticizer pair to detect aromatic hydrocarbons, mixtures, and interferents in water with single sensors and arrays. ACS Sens. 7, 649–657. doi:10.1021/acssensors.1c02653
Preti, G., Labows, J. N., Kostelc, J. G., Aldinger, S., and Daniele, R. (1988). Analysis of lung air from patients with bronchogenic carcinoma and controls using gas chromatography-mass spectrometry. J. Chromatogr. B Biomed. Sci. App. 432, 1–11. doi:10.1016/s0378-4347(00)80627-1
Raman, B., Stopfer, M., and Semancik, S. (2011). Mimicking biological design and computing principles in artificial olfaction. ACS Chem. Neurosci. 2, 487–499. doi:10.1021/cn200027r
Ricco, A. J., Crooks, R. M., and Osbourn, G. C. (1998). Surface acoustic wave chemical sensor arrays: new chemically sensitive interfaces combined with novel cluster analysis to detect volatile organic compounds and mixtures. Accts. Chem. Res. 31, 289–296. doi:10.1021/ar9600749
Ricco, A. J., Martin, S. J., and Zipperian, T. E. (1985). Surface acoustic wave gas sensor based on film conductivity changes. Sen. Actuators 8, 319–333. doi:10.1016/0250-6874(85)80031-7
Röck, F., Barsan, N., and Weimar, U. (2008). Electronic nose: current status and future trends. Chem. Rev. 108, 705–725. doi:10.1021/cr068121q
Rodríguez-Aguilar, M., de León-Martínez, L. D., Zamora-Mendoza, B. N., Comas-García, A., Palomares, S. E. G., García-Sepúlveda, C. A., et al. (2021). Comparative analysis of chemical breath-prints through olfactory technology for the discrimination between SARS-CoV-2 infected patients and controls. Clin. Chim. Acta 519, 126–132. doi:10.1016/j.cca.2021.04.015
Ruszkiewicz, D. M., Sanders, D., O’Brien, R., Hempel, F., Reed, M. J., Riepe, A. C., et al. (2020). Diagnosis of COVID-19 by analysis of breath with gas chromatography-ion mobility spectrometry – a feasibility study. eClinicalMed 29-30, 100609. doi:10.1016/j.eclinm.2020.100609
Scheepers, M. H., Al-Difaie, Z., Brandts, L., Peeters, A., van Grinsven, B., and Bouvy, N. D. (2022). Diagnostic performance of electronic noses in cancer diagnoses using exhaled breath: a systematic review and meta-analysis. JAMA Net. Open 5, e2219372. doi:10.1001/jamanetworkopen.2022.19372
Scott, S. M., James, D., and Ali, Z. (2006). Data analysis for electronic nose systems. Microchim. Acta 156, 183–207. doi:10.1007/s00604-006-0623-9
Shan, B., Broza, Y. Y., Li, W., Wang, Y., Wu, S., Liu, Z., et al. (2020). Multiplexed nanomaterial-based sensor array for detection of COVID-19 in exhaled breath. ACS Nano 14, 12125–12132. doi:10.1021/acsnano.0c05657
Snitz, K., Andelman-Gur, M., Pinchover, L., Weissgross, R., Weissbrod, A., Mishor, E., et al. (2021). Proof of concept for real-time detection of SARS CoV-2 infection with an electronic nose. PLoS One 16, e0252121. doi:10.1371/journal.pone.0252121
Stetter, J. R., and Penrose, W. R. (2002). Understanding chemical sensors and chemical sensor arrays (electronic noses): past, present, and future. Sens. Update 10, 189–229. doi:10.1002/1616-8984(200201)10:1<189::aid-seup189>3.0.co;2-n
Tan, J., and Xu, J. (2020). Applications of electronic nose (e-Nose) and electronic tongue (e-Tongue) in food quality-related properties determination: a review. Artif. Intell. Agric. 4, 104–115. doi:10.1016/j.aiia.2020.06.003
Van Den Velde, S., Quirynen, M., and van Steenberghe, D. (2007). Halitosis associated volatiles in breath of healthy subjects. J. Chromatogr. B 853, 54–61. doi:10.1016/j.jchromb.2007.02.048
Keywords: breath diagnostic, electronic nose, nanosensor, chemical sensor array, COVID-19, nanomaterial, EnCOVID system
Citation: Ricco AJ, Hannon AM and Li J (2025) Chemical nanosensor arrays for human breath-based diagnostics: Perspective and a promising result for COVID-19 detection. Front. Sens. 6:1575769. doi: 10.3389/fsens.2025.1575769
Received: 12 February 2025; Accepted: 14 March 2025;
Published: 16 April 2025.
Edited by:
Dermot Diamond, Dublin City University, IrelandReviewed by:
Rosamaria Capuano, University of Rome Tor Vergata, ItalyIsabel M. Perez De Vargas, University of Granada, Spain
Copyright © 2025 Ricco, Hannon and Li. This is an open-access article distributed under the terms of the Creative Commons Attribution License (CC BY). The use, distribution or reproduction in other forums is permitted, provided the original author(s) and the copyright owner(s) are credited and that the original publication in this journal is cited, in accordance with accepted academic practice. No use, distribution or reproduction is permitted which does not comply with these terms.
*Correspondence: Antonio J. Ricco, YW50b25pby5qLnJpY2NvQG5hc2EuZ292; Jing Li, amluZ2xpODl1dUBnbWFpbC5jb20=