- 1National Renewable Energy Laboratory, Golden, CO, United States
- 2Department of Management, école des Hautes Études Commerciales Montréal, Montréal, QC, Canada
The goal of the circular economy (CE) is to transition from today's take-make-waste linear pattern of production and consumption to a circular system in which the societal value of products, materials, and resources is maximized over time. Yet circularity in and of itself does not ensure social, economic, and environmental performance (i.e., sustainability). Sustainability of CE strategies needs to be measured against their linear counterparts to identify and avoid strategies that increase circularity yet lead to unintended externalities. The state of the practice in quantitatively comparing sustainability impacts of circular to linear systems is one of experimentation with various extant methods developed in other fields and now applied here. While the proliferation of circularity metrics has received considerable attention, to-date, there is no critical review of the methods and combinations of methods that underlie those metrics and that specifically quantify sustainability impacts of circular strategies. Our critical review herein analyzes identified methods according to six criteria: temporal resolution, scope, data requirements, data granularity, capacity for measuring material efficiency potentials, and sustainability completeness. Results suggest that the industrial ecology and complex systems science fields could prove complementary when assessing the sustainability of the transition to a CE. Both fields include quantitative methods differing primarily with regard to their inclusion of temporal aspects and material efficiency potentials. Moreover, operations research methods such as multiple-criteria decision-making (MCDM) may alleviate the common contradictions which often exist between circularity metrics. This review concludes by suggesting guidelines for selecting quantitative methods most appropriate to a particular research question and making the argument that while there are a variety of existing methods, additional research is needed to combine existing methods and develop a more holistic approach for assessing sustainability impacts of CE strategies.
Introduction
Driven in part by the growth of renewable energy technologies and the expansion of information and communication technologies, the demand for critical materials is rising (Graedel and Cao, 2010; Knoeri et al., 2013; Deetman et al., 2018). It is estimated that between 2015 and 2060, demand for materials will increase by 87,000% for electric vehicles, 1,000% for wind power, and 3,000% for photovoltaics (PV) (Sovacool et al., 2020). Minerals and metals are essential to the low-carbon transition, but researchers and policy-makers warn that increased use of such materials should not aggravate social and ecological problems (Sovacool et al., 2020). In the information and communication technologies sector and others, growing demand is also seen as a primary cause of material scarcity (PWC, 2011). This is because the modus operandi of most industries and society are based on a linear view of the economy, following patterns of production and consumption where resources are extracted to manufacture products which are then discarded at the end of their useful life—the so-called “take-make-waste” linear economic model (Ellen MacArthur Foundation, 2020). The linear model is a cause of and exacerbates material scarcity (Lieder and Rashid, 2016; Sovacool et al., 2020). By contrast, a circular economy (CE) is viewed as an economic model capable of solving challenges of increasing demand and materials scarcity by departing from a linear view of the economy and encouraging the reduction and re-use of products and materials, therefore decoupling economic growth from resource depletion (Stahel, 2016; Linder et al., 2017). The successful implementation of the CE could, in principle, benefit both the global natural environment and economy. The CE aims to maximally retain economic value in products, materials, and resources over time (Kalmykova et al., 2018). It has been estimated, for instance, that CE could annually generate 1 trillion USD globally (over the linear economy) (Korhonen et al., 2018) and that in the European Union (EU) alone, resource efficiency stemming from applying CE concepts could save between 8 and 15% of EU primary energy consumption (Mehlhart et al., 2016).
While the origin of the CE concept is often assigned to D. W. Pearce and R. K. Turner book, Economics of natural resources and the environment in 1990 (Pearce and Turner, 1990), it has gained momentum since 2010 due to the efforts of the Ellen MacArthur Foundation (Ellen MacArthur Foundation, 2013) and others (World Business Council for Sustainable Development, 2020; World Economic Forum, 2020). Over the past decade, a growing body of research has been published on the topic of CE, and several literature reviews summarize research findings and provide further guidance. Ghisellini et al. (2016) were among the first to conduct an extensive review of the CE literature, including a review of CE metrics (Ghisellini et al., 2016). Many reviews have been performed since then and the most salient ones are presented here, along with their limitations.
Most reviews have focused on CE metrics proposing different ways of analyzing them. Iacovidou et al. (2017), for instance, reviewed CE metrics according to the environmental, economic, social, and technical dimensions of the CE while the taxonomy proposed by Saidani et al. (2019) involves 10 criteria such as the analysis scale, the intended use of the metric and its origin. Parchomenko et al. (2019), in turn, identified 63 metrics for assessing CE sustainability and defined their main characteristics. They further characterized the metrics with their relevance to 24 characteristics of the CE. For example, among the 24 key CE features identified, waste elimination is the most recurrent since 44 of the 63 evaluation methods and tools1 take waste into account in their calculation method. Taking a different approach, Moraga et al. (2019) proposed a classification framework to examine 20 product-level metrics. They conclude that existing metrics are limited because they do not include aspects related to consumer behavior (e.g., multifunctionality or product sharing) and the socio-institutional changes that are needed for certain CE strategies (e.g., product sharing). Although the above-highlighted reviews discuss methods that can be used to assess the CE to some degree, their main focus is on metrics: metric selection (Iacovidou et al., 2017; Moraga et al., 2019; Saidani et al., 2019) and guidance for further metrics development (Parchomenko et al., 2019). Metrics, however, are generated from methods and, thus, investigating the methods that have been applied to study the CE could provide further guidance for research and support the development of more adequate metrics. As the literature reviews show, different metrics have different dimensions (e.g., evaluating the environmental or social impacts of a system), which are rooted in the methods that generate them (Iacovidou et al., 2017; Sassanelli et al., 2019).
More recently, Kristensen and Mosgaard (2020) conducted a review of 30 micro-scale circularity measurement tools that can be applied at the product and enterprise-level (Kristensen and Mosgaard, 2020). Their results show that most of the metrics (21) focus on a selected aspect of CE and thus fail to capture the whole variety of strategies that could increase circularity. Conversely, they conclude that multidimensional methods lack pragmatism in their use (Kristensen and Mosgaard, 2020). Other literature reviews have focused on economic models that have been developed to study the CE (Bigano et al., 2016; Winning et al., 2017), on analyzing different circular business models (Bocken et al., 2019) and circular processes (Schetters et al., 2014), and on the different approaches employed by researchers when studying the CE (Merli et al., 2018). Although Merli et al. (2018) mention some of the methods and tools that have been used in CE research, their analysis is limited—e.g., to understand the strengths and weaknesses of each method—as they are not the primary focus of the study.
Focusing on methods—rather than metrics—Sassanelli et al. (2019) conducted the first and to-date, only systematic literature review of CE assessment methods. Their analysis included more than 10 different methods. The authors found that many CE studies strive to include several lifecycle stages in the analysis and that most methods are only focusing on the environmental aspect of sustainability. Moreover, their analysis confirms the findings of Parchomenko et al. (2019) that the use of materials is the variable that is most often studied in the literature on CE assessment. This result is explained both by the nature of the CE concept and the methods that have most often been used to investigate it: life cycle assessment and material flow analysis (Sassanelli et al., 2019). The authors also highlight the importance of CE assessment methods to enable analysis both on system and product levels and consider the large number of different variables that compose the entire lifecycle of a system. The study concludes that further research is therefore needed to determine how a holistic methodology could be developed to include all sustainability aspects of a given system (environmental, social and economic) including its full life cycle and assess its circularity. The review, however, does not provide relevant information such as the scope of the analysis covered by each method or combination of methods or whether dynamical aspects are included, which could help guide future CE research.
As already highlighted, because most reviews focus on metrics and tools [see, for instance (Iacovidou et al., 2017; Moraga et al., 2019; Saidani et al., 2019) rather than methods (with the exception of Sassanelli et al. (2019)], they fail to provide clear guidance for the selection of methods that underpin the metrics and tools used to study the CE. Given so many options of research methods to assess the sustainability of CE strategies, as well as the diversity of application scales and other elements such as temporal aspects, there is a need for guidance about which method (or combination of methods or tool) are best suited to assess particular CE-related research questions. It is relevant to ask, for instance, what is the scope of the analysis covered by each method, whether dynamical aspects are included, or their ability to model consumer behaviors and socio-institutional changes that are needed to accomplish the transition to a more circular economy. As shown herein, there is currently no approach specifically designed to quantitatively assess the sustainability of the transition toward a more circular economy and, thus, various methods from different fields have been applied for that purpose. It is quite a critical matter as increased circularity does not necessarily lead to sustainability benefits [e.g., if a material with recycled content cause more environmental impacts than another virgin material (Vendries et al., 2020) or if recycling is done informally, causing health and social impacts (Umair et al., 2013; Arushanyan et al., 2014)]. In light of these shortcomings, it is also pertinent to ask whether new methods should be developed to quantitively assess the benefits of the CE to sustainability [as Sassanelli et al. (2019) suggested] or if combining methods from different fields could be sufficient. Thus, the objectives of this paper are to:
• Critically analyze the literature to identify the strengths and weaknesses of the methods used to quantitatively assess the sustainability of CE strategies,
• Classify what types of research questions are best addressed by which methods
• Ascertain whether the development of new circularity assessment method(s) is needed.
Review Method and Analysis Framework
This article reviews methods that quantify the sustainability aspects of CE strategies. The scope of the review spans from 2000 to 2020 and comprises articles mainly from the industrial ecology and complex systems science fields (Figure 1). To collect the articles in this review, a Scopus search was performed using keyword combinations of the word “circular economy” and the names of the following eight main assessment methods: life cycle assessment, input-output analysis, material flow analysis, emergy/exergy analysis, system dynamics, discrete event simulation, agent-based modeling, and operations research (Figure 1). The industrial ecology and complex systems science fields were chosen based on a preliminary review of the CE literature (Ghali et al., 2017; Merli et al., 2018; Franco, 2019; Moraga et al., 2019; Sassanelli et al., 2019). The selection of keywords for the Scopus search also follows from this preliminary review and is based on the frequency of use and analysis of the methods mentioned in the reviewed publications.
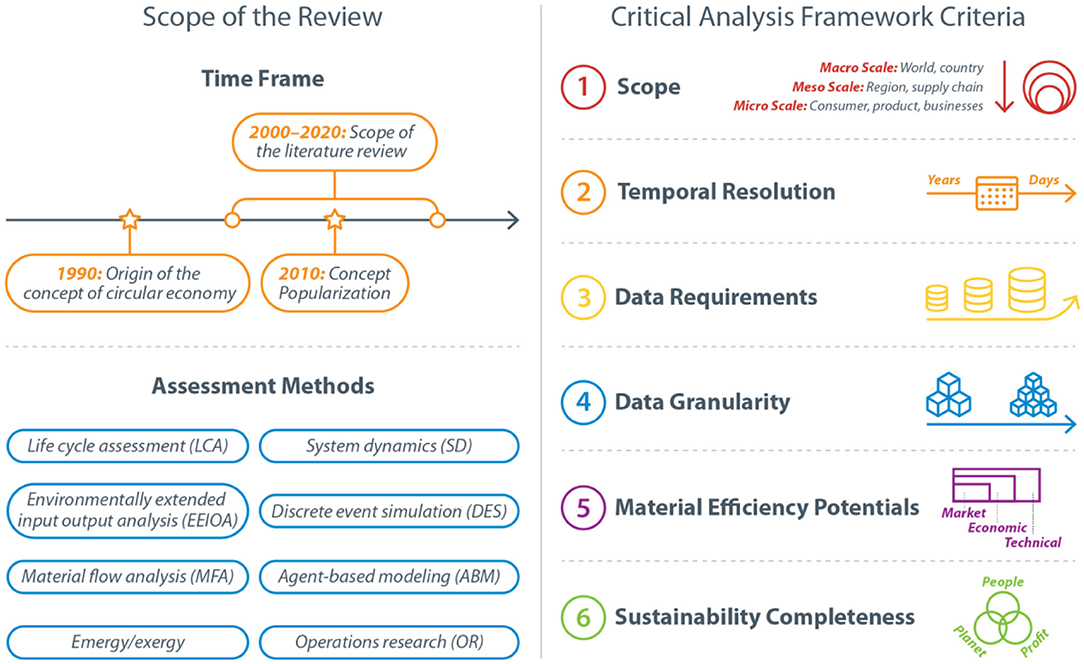
Figure 1. Scope of the literature review and critical analysis framework. The publication years of the articles in the review span from 2000 to 2020 and the articles reviewed focus primarily on assessment methods used in industrial ecology and complex systems science. The methods are analyzed according to six criteria, which affect the type of research questions that may be answered.
The reader is referred to the results section for a brief description of each assessment method, which is provided prior to an examination of examples from the CE literature for each assessment method. Moreover, the Supplementary Materials further provide conceptual representation and mathematical foundations of some of the reviewed methods. A total of 824 articles were found in Scopus. Although more than eight assessment methods have been applied to study circular strategies, the purpose of this review is not to be exhaustive but to give an overview and guidance about the methods that have been used most commonly to study circular strategies. Moreover, the review is not systematic as it would have been burdensome to analyze all published articles from specific fields (e.g., the keyword combination of “circular economy” and “life cycle assessment” yields 476 results on the Scopus database) without necessarily adding value to the analysis. Thus, for each method, after reviewing all abstracts identified in Scopus according to the keyword combinations above, the articles with the most relevance for the critical review were selected, for instance, the articles most cited and in journals with the highest impact factors. More articles were then found using the “snowballing” technique [i.e., using the reference list and citations of already reviewed papers to identify additional potentially relevant papers for review (Badampudi et al., 2015)]. At the end of this process, 92 articles were kept, which, in our opinion, best represented the eight methods and how they have been applied to study the CE.
In order to provide guidelines to CE stakeholders such as policy-makers, scholars, entrepreneurs, non-profits organizations, we analyzed the methods presented in each article we found through the literature review and we assessed their ability to model the CE. The capability of a method to adequately represent a CE system is considered herein the first step of a quantitative assessment of its sustainability and is, thus, the focus of this review. For more details on the consideration of the triple bottom line by assessment methods, the reader is directed to the review by Sassanelli et al. (2019).
To that end, each article was categorized according to six criteria: (1) scope, (2) temporal resolution, (3) data requirements, (4) data granularity, (5) material efficiency potentials, and (6) sustainability completeness. First, because CE needs to be implemented across the micro, meso, and macro scales (Stahel, 2016; Merli et al., 2018), we classified the scope of each method. Second, we assessed the temporal resolution of each method because the transition from a linear to a circular model of consumption and production occurs over time (Ghisellini and Ulgiati, 2020). Hence, it is important to understand the temporal aspects of the different methods used to analyze the CE. Next, because data requirements and data granularity may influence the choice of the assessment method, for instance, depending on what data are available, we evaluated the data required by each method and the granularity of data needed. Fifth, we assessed the method's approach to measuring material efficiency using three levels: technical, economic, and market potentials. The technical potential is the highest level and is not constrained by economics [in other words, measures that are not cost-effective are included in this potential (Nadel et al., 2004)]. The economic potential excludes economically ineffective measures and is, therefore, smaller than the technical potential. The market (or achievable) potential is often smaller than the economic potential, and better reflects observations from the real-world as it depends on the rate at which individuals and businesses adopt the CE strategies in practice (Nadel et al., 2004). This last level of analysis is crucial if the research question is related to the social changes implied by the CE. For instance, new business models such as those implied by the sharing economy (or collaborative consumption) may not be adopted by the overall population and therefore stay at a niche level or vanish (e.g., Car2Go failure in North America). Finally, the sustainability completeness criterium aims at evaluating to what extend each method can quantitatively assess CE strategies against the three pillars of sustainability (people, planet, and profit).
Review of Literature
Methods From Industrial Ecology
Life Cycle Assessment
Life cycle assessment (LCA) is a standardized method (ISO, 2006a,b) which aims to quantify environmental impacts of products and avoid burden shifting from one environmental impact or life cycle stage to another (Hellweg and i Canals, 2014). It requires identifying and quantifying material and energy flows throughout a product's life cycle, which consists of technological processes from raw materials extraction to the product's end-of-life processing (Hellweg and i Canals, 2014). The analyst must define the life cycle's boundaries during the initial scoping step of the LCA. For tractability, LCA analysts often exclude some of a product's background processes, which leads to the well-known limitation of truncation (Agez et al., 2019). Developing the life cycle inventory (LCI) is the second step of LCAs, which leads to quantifying environmental impacts in the life cycle impact assessment (LCIA) step (Hellweg and i Canals, 2014). The basis for an LCA is the functional unit—a measure that quantifies the product or service produced from the life cycle and over which a product's life cycle impacts are normalized. Methods have also been developed to evaluate the economic and social impacts of a product's life cycle, called life cycle costing (LCC) and social life cycle assessment (S-LCA), respectively (Fauzi et al., 2019). Those developments, along with others such as the inclusion of temporal and geographical aspects, enable LCA to answer a variety of sustainability-related questions arising from increasingly complex production and consumption systems (Hellweg and i Canals, 2014).
LCA is one of the most widely applied methodologies to assess circularity (Sassanelli et al., 2019) and aligns with the current focus of scholars toward the environmental dimension of the CE (Merli et al., 2018). For example, LCA has been used to identify potential environmental trade-offs of improving circularity (Lonca et al., 2018), and LCA combined with LCC has been used to evaluate the environmental and economic benefits of applying CE strategies (Laso et al., 2018). An important and perhaps counterintuitive conclusion from LCA studies is that circularity metrics, such as the material circularity index (MCI) from the Ellen MacArthur Foundation, may provide different conclusions than environmental indicators on which options are preferable (Lonca et al., 2018; Walker et al., 2018). In addition to the example given earlier, where a material with recycled content could be worse off than another virgin material (Vendries et al., 2020), two more examples can be mentioned. First, oxo-degrading plastic bags may seem more circular as they avoid the generation of visible waste. In reality, however, those plastic bags are more polluting to produce as they use more energy while still contributing to plastic waste [e.g., in the oceans, due to the microplastics they form when being degraded by UV light (Imbeault-Tétreault, 2017)]. However, the environmental impacts of oxo-degrading plastic bags could be reduced using renewables or due to economies of scale if demand were to increase. Second, a case study on tires showed that retreading (replacing the rubber bands on used tires) is the best option when compared to regrooving (re-carving new grooves in used tires) when assessed with the MCI (Lonca et al., 2018). This conclusion, however, does not hold in all the LCA environmental indicators (e.g., retreading increases environmental impacts in the resource indicator while regrooving reduces them) (Lonca et al., 2018). This is explained by the fact that circularity metrics do not model cause-effect chains of environmental impacts but rather focus on resource use. However, even in the resource impact category, LCA conclusions may be at odds with results from circularity metrics (Lonca et al., 2018). LCA alone, however, may not always be well-suited to quantifying the effects of CE. For instance, standardized LCA (ISO, 2006a,b) is typically conducted on a static system, and all impacts are aggregated (and averaged) over space and time, which limits its use in the case of the CE transition as it involves complex evolving systems (e.g., to study the changes in mobility patterns caused by new business models from the sharing economy such as Uber).
An essential step of LCA is to define the functional unit, providing a common ground to compare different impacts of different systems providing the same function. Indeed, the characteristics of the functional unit, such as geographical location and temporal scope, will drive the results of the entire study. This remark may be especially relevant in the case of the CE because new consumption patterns, such as those implied by product-service systems, may entail that the functional unit changes based on the introduction of the CE strategy. For instance, commuting to work in traditional mobility systems can be compared on the basis of a person-kilometer (displacement of one person over one kilometer) for each mode of transportation. However, CE systems such as carpooling and car-sharing may also involve other transportation modes. Moreover, the shares of transportation modes for a commute may vary with time (e.g., depending on the season). Thus, such CE systems may require a functional unit that encompasses those aspects rather than simply using the person-kilometer as the basis of the LCA. In a study of product-service systems, Goedkoop (1999) therefore proposes the concept of a narrow vs. a wide definition of the functional unit. For instance, a narrow functional unit definition for the mobility system mentioned above could be 1 person-kilometer, whereas a wide functional unit for the same system could be a household's monthly transportation activities. This concept was later applied to account for behavioral change in a model of smart-homes combining agent-based modeling and a dynamic LCA (Walzberg et al., 2019). The results of the LCA were highly dependent on temporal and behavioral aspects, the latter accounting for a third of the variance in climate change impact. This example suggests that LCA alone may not always be enough to study CE strategies involving social changes. The method is indeed primarily static, based on linear economy data, and therefore cannot endogenously capture the impacts of circular strategy implementation, which involves behavior changes from individuals and companies alike.
Environmentally Extended Input-Output Analysis
Environmentally extended input-output analysis (EEIOA) shares the same goal as LCA: to quantify environmental impacts linked directly or indirectly to a product or service, including production (but not always including the use or end-of-life phases) (Jeswani et al., 2010). However, LCA provides a detailed account of every process involved in the life cycle or a product system, while EEIOA regroups national inventories to describe the interdependence between economic sectors (Miller and Blair, 2009). EEIOA tables are based on national statistics on industries' trading information (input-output tables) as well as their emissions and resource use (environmental extensions). In comparison to LCA, EEIOA has the advantage of including every declared monetary transaction in an economy, avoiding the use of system boundaries and the ensuing truncations (Agez et al., 2019). Yet even EEIOA has limits as, for instance, the informal economy is not captured. Thus, in EEIOA, a broader representation of the supply chain is achieved. However, what EEIOA gains in breadth, it sacrifices in-depth, or resolution (i.e., the technological details of the assessed system). Environmentally extended multi-regional input-output extends even further the breadth of the analysis to the global economy (Stadler et al., 2018).
To a lesser extent than LCA, EEIOA has been used to study circular strategies (Sassanelli et al., 2019). Wiebe et al. (2019), for instance, used the multi-regional input-output database EXIOBASE in combination with a scenario from the International Energy Agency (IEA) to model the economy in 2030 and study the implementation of CE strategies (recycling, reducing, repair, and re-use). The study found that CE strategies could reduce raw material extraction by 10% while increasing employment by 2%. Although such studies provide useful insights on CE implementation, they do not enable researchers to quickly adapt the developed model to study different scenarios because of the complexity of manipulating the EEIOA database. Thus, CE scenarios need to be modeled exogenously before being imposed on the EEIO model. To solve the issue of quickly building and analyzing complex CE strategy scenarios, Donati et al. (2020) proposed an EEIOA-based tool capable of modeling two CE strategies: product lifetime extension and resource efficiency. The authors applied their tool to study the application of the CE strategies in the EU construction, transportation, and household goods sectors and found that those strategies could reduce climate change impact by 10%.
With respect to CE, the downside of EEIOA is that the technological “recipes” are heavily aggregated, representing production averages rather than the manufacture of a specific product. Thus, individual firms and their specific technological processes are not represented in EEIOA, making it impossible to realize a study on a specific product (Suh, 2004) or business model. Moreover, niche markets of the CE, such as second-hand products, are not represented in input-output tables; at best, they are included in a broader economic sector such as “other manufactured goods” if not excluded entirely. Finally, another limitation of the method is that it does not provide insights into temporal dynamics, such as the material stocks becoming available for re-use (Wiebe et al., 2019). Moreover, EEIO databases tend to be out of date (because of the amount of work involved in updating a database), so emerging sectors and technologies such as those of the sharing economy may not be represented. As an example, the latest available input-output tables from the Bureau of economic analysis are from 2012 (BEA, 2020). Finally, because EEIOA is a snapshot of the economy, it may miss or overrepresent punctual events such as droughts or recessions.
Material Flow Analysis
Material flow analysis (MFA) describes a system such as a city or a country in terms of its input and output physical flows of materials in space and time (Rincón et al., 2013). MFA starts with the definition of the system boundaries. Then, all relevant processes and flows of the system are modeled (Cencic and Rechberger, 2008). A process may be a transformation, transportation, or storage activity. Flows connect processes in the same way that intermediate product and economic flows link processes and economic sectors in LCA and EEIOA, respectively. The goal of MFA is to understand the material processes of a system to inform better decisions related, for instance, to waste management, material availability constraints, or material disposition (Ethan et al., 2000). While MFA shares similarities with LCA and EEIOA as models of the technosphere, the method also demonstrates some key divergence.
First, in MFA, processes are usually more aggregated than in LCA and EEIOA. Another difference with LCA is that, often, not much attention is given to the details of processes (they are considered black boxes) as researchers are more interested in the inputs and outputs of the system (Cencic and Rechberger, 2008). Moreover, MFA usually deals with a small set of materials rather than all related materials and energy flows of a product and does not usually focus on environmental impacts. However, similar to LCA, MFA may be adapted to account for costs rather than physical flows in so-called material flow cost analysis (MFCA) (Merli et al., 2018). One of the advantages of MFA, as compared to LCA and EEIOA, is that the method accounts for hidden flows of materials (however, depending on the study, some of those hidden flows may also be accounted for in LCA) (Rincón et al., 2013). Those hidden flows are constituted of the materials that are necessary to obtain final goods, but which are not visible in the economic account, such as wood harvesting losses (Rincón et al., 2013). The methodology also enables keeping track of material stocks, which is not the case of LCA or EEIOA (Lopes Silva et al., 2015). Moreover, similarly to input-output analysis, MFA can be extended to account for environmental impact [e.g., with the environmentally-weighted material consumption indicator (Krausmann et al., 2017)]. Finally, using time series and projection, temporal aspects can be included in MFA (Park et al., 2011). In the context of CE, Wiedenhofer et al. (2019), for instance, developed an economy-wide dynamic MFA to explore the effect of implementing strong re-use and recycling policies at the global scale between 2015 and 2050.
There is an obvious alignment between CE and MFA in the focus on materials, and indeed MFA has been used to study CE at the country, region, or city scale. Some researchers, for instance, have applied the method to develop a circularity metric (Franklin-Johnson et al., 2016), while others have assessed the circularity of the global economy, the EU, and a city using an economy-wide MFA framework (Haas et al., 2015; Voskamp et al., 2017). Voskamp et al. (2017), for instance, showed that because the material metabolism of the city of Amsterdam is dominated by trade flows and not consumer-related consumption flows, the CE will have a limited impact on the metabolism of the city. Haas et al. (2015) applied MFA to assess the circularity of global and European material flows. Both studies concluded that the world, and the EU, are still far away from achieving a CE as exemplified, for instance, by only 6 and 12.6% recycling rates, respectively. Moreover, a significant fraction of consumed materials accumulates as in-use stock and, thus, globally, stocks are growing. The authors critically conclude that even if recycling rates improve, growing stocks (i.e., materials reservoirs such as buildings) will make it difficult to close the loops (Haas et al., 2015). However, MFA does not enable modeling what happens with in-use stocks because processes are considered black-boxes (focusing on the mass balance, fewer details are given on how the input of processes are used to yield outputs). Thus, MFA is limited to represent some CE strategies operating within the in-use stocks such as repairing, refurbishing and sharing.
Emergy and Exergy
Another challenge of MFA is the use of mass as a metric, which neglects the relative values of materials with different qualitative energy contents; for instance, 1 kJ obtained from wood is different from 1 kJ from coal due to the renewable quality of the former. However, all flows of materials may be considered as energy flows from an ecological point of view (Huang et al., 2006). Thus, emergy and exergy have emerged as a solution to this issue. Rooted in ecology and thermodynamics, emergy is defined as the sum of all inputs of available energy directly or indirectly required in a system (or embodied energy) (Pan et al., 2016). With the help of conversion coefficients, all flows are accounted in the same unit, the solar equivalent joules (or emjoule), which refers to the available energy of one kind consumed in transformations. Therefore, the qualitative nature of the different types of energy is included in emergy analysis. In the context of CE, emergy analysis was applied to eco-industrial parks (Pan et al., 2016). In this study, the use of emergy enabled accounting for the ecosystem's contribution to the activities of the industrial park, such as the amount of energy required by ecological services to render polluting emissions harmless.
Exergy, on the other hand, is defined as the maximum usable energy of a material and can be computed for both combustible and non-combustible materials (Jamali-Zghal et al., 2015). Some researchers have suggested that exergy could be a relevant metric to assess circularity (Huysman et al., 2017), as this method has the advantage of accounting for the thermodynamic limits of circularity (Abadías Llamas et al., 2019). Contrary to emergy, the exergy concept does not allow, however, to qualitatively differentiate different types of energy and, as for emergy, is limited in its ability to grasp potential environmental impacts (Sassanelli et al., 2019). A few studies, however, have combined environmental impact methodologies with exergy analysis to solve that issue (Yousef and Hassan, 2019).
Methods From Complex Systems Science
System Dynamics
System dynamics (SD) is a computer simulation approach that aims at understanding complex systems by studying their feedback mechanisms and non-linear behaviors (Sterman, 2010). SD describes a system with stocks, flows, and parameters, while a corresponding set of differential and algebraic equations are solved through a simulation to get insights into the system's dynamics (Filippov, 2004). The method accounts for four characteristics of complex systems: accumulation of stocks, time delays, feedback loops, and non-linearities (Franco, 2019). One strength of SD is that, in addition to quantitative data, it may use qualitative data to generate robust and accurate models (Luna-Reyes and Andersen, 2003). One potential drawback is that SD cannot distinguish eventual heterogeneities in a stock, which in some cases may make a difference (Filippov, 2004). For instance, metal recycling leads to the presence of trace contaminants (due to the presence of different metals in scraps), which may ultimately limit recycling (Daehn et al., 2017), a fact that is difficult to model in SD. Moreover, SD usually does not account for the environmental aspects of sustainability. In the context of CE, SD has been used to study closed-loop supply chains, recycling, business models, and product-service systems (Asif et al., 2016; Franco, 2019).
Discrete Event Simulation
While system dynamics models usually involve a high degree of aggregation and seek to understand how various feedback loops affect the system, discrete event simulation (DES) models represent similar systems in more detail. DES focuses more on how various deterministic and stochastic events trigger the dynamics of the system rather than on feedback loops (Morgan et al., 2017). DES enables researchers to explore progression through a system—such as that of a product through an assembly line—and is often used to represent the business processes of a system. This method is especially suited when the temporal variation of the states of system constituents is an essential source of the variability in the system's outputs (Morgan et al., 2017). SD and DES may produce very similar results; however, the choice of the method may influence the system's boundaries and how a researcher approaches a problem (Morgan et al., 2017). In the context of the CE, this choice may be based, for instance, on the scale of the analysis.
DES has been used to study sustainable manufacturing and the CE (Gbededo et al., 2018; Charnley et al., 2019). One study also used DES to investigate conditions favoring remanufacturing in the UK (Charnley et al., 2019). In that study, the DES model represented the remanufacturing process, where the discrete events described various remanufacturing operations such as collection, disassembly, inspection, reconditioning, and reassembly. By focusing on processes, DES is well-suited to study circular strategies at a micro-scale but may be challenging to apply at the meso or macro scales (while the reverse is true for SD). This challenge could be overcome, however, if modeling and computational resources are sufficient. Finally, similarly to SD, DES provides a limited account of sustainability if not combined with other methods.
Agent-Based Modeling
In agent-based modeling (ABM), a system is represented through its various entities (called agents), much like in DES (Wilensky and Rand, 2015). Agents are heterogeneous individual entities that may represent various elements, such as organizations, households, or molecules (Wilensky and Rand, 2015). Moreover, agents are independent, interact with their environment as well as with each other, and make their own decisions (Grüne-Yanoff and Weirich, 2010; Wilensky and Rand, 2015). ABM diverges from SD and analytical modeling in three main aspects: (i) agents' interactions and their results are discrete, (ii) system's entities homogeneity is not assumed, (iii) model outputs can be examined at both individual agent and agent-aggregated levels (Wilensky and Rand, 2015). ABM is a suitable framework to study complex systems when the behavior of the modeled system emerges from the behaviors of the network of agents and their interactions, with no overall central control (Baustert and Benetto, 2017).
ABM has been applied in the context of CE, for instance, to study industrial symbiosis (IS) (Ghali et al., 2017; Chahla and Zoughaib, 2019; Fraccascia et al., 2019). Ghali et al. (2017) developed an ABM based on the theoretical framework of IS. The authors show how IS socio-technical attributes can emerge from the interactions and behavior of individual plants: they found, for instance, that both the social structure (i.e., the type of social network connecting firms) and social dynamics (trust and IS knowledge diffusion in the network) were important factors in the creation of industrial synergies (Ghali et al., 2017). While Ghali et al. (2017) make use of the concept of trust as a driver of IS, another study looks more precisely at the effect of non-cooperative behaviors (i.e., when IS actors are self-interested) (Chahla and Zoughaib, 2019). ABM has also been used to study recycling behaviors (Meng et al., 2018; Tong et al., 2018; Luo et al., 2019). In China, change in consumer behavior that leads to increased waste separation and recycling is seen as critical to the CE transition (Tong et al., 2018). Thus, Tong et al. (2018) developed an ABM based on a social psychology model—the theory of planned behaviors (Ajzen, 1991)—to identify critical factors in changing consumer behaviors. Finally, ABM has also been applied to study consumers' acceptance of new business models in the CE context (Lieder et al., 2017). Results from that study show that ABM is suited to analyze different marketing strategies, such as launching two circular business models at the same time or the effect of advertisement on the development of circular business models. While such studies do not provide information on sustainability metrics directly, they may help understand the conditions that would favor circularity in a broader manner.
Because the system's description is based on the description of its agents, a strength of the method is its ability to provide a detailed representation of the entities' decision process. As such, the method enables researchers to look for a behavioral model that more accurately represents the real-life decision process (Walzberg et al., 2019). When studying CE strategies, if economic agents always make rational decisions (maximizing their utility), traditional methods may be well-suited and would account for the technical, economic, and market potential of the CE strategies. Otherwise, those methods may fall short of representing the actual market potential. Indeed, the market potential may be constrained due to humans thinking with both a reflective system (leading to rational decisions) and an automatic system (leading to more instinctive decisions) (Thaler and Sunstein, 2008), which may divert from techno-economic optimum. Starting from the bottom-up, ABM often employs theories from social psychology such as the theory of planned behavior (Ajzen, 1991) to represent decisions involving both the reflective and automatic systems (Walzberg et al., 2019). Weaknesses of the ABM method are, however, the need for thorough validation and a significant amount of input data (Rand, 2019), the lack of consistency between how models are reported (Hinkelmann et al., 2011), and the difficulty to generalize some models (Hansen et al., 2019).
Operations Research
Operations research (OR) is a broad field that includes multi-criteria decision-making (MCDM) tools, game theory, and other optimization techniques (Laengle et al., 2017). OR aims at facilitating decisions by looking for the best solution to a given problem (Altay and Green, 2006). For instance, MCDM enables decision-makers to choose the best alternative based on quantitative scores when facing the issue of multiple criteria and disagreements between the alternative's relative performances on each criterion (Thies et al., 2019). In the context of CE, Xu et al. (2018), for instance, used MCDM to evaluate innovations strategy for wastes from electronic and electrical equipment (WEEE) recycling in Australia. The authors identified 28 criteria organized according to the benefits, opportunities, costs, and risks as well as the triple bottom line frameworks. Then, they identified 11 CE strategies available to a WEEE recycling company and found that strategies related to the product and service perspective (e.g., implement recovery schemes or information exchange networks) perform the best due to their ability to create profit and value to the company.
Another OR method used to study CE is game theory. The method enables describing situations of cooperation and conflict mathematically (games) and finding the state of equilibrium (if it exists) under which each player of the game maximizes its utility (Hargreaves-Heap and Varoufakis, 1995; Palafox-Alcantar et al., 2020). Although the study of cooperation and conflicts stemming from CE implementation is still mostly missing from the literature (Palafox-Alcantar et al., 2020), game theory could prove beneficial to study conflicts arising from CE actors and situations favoring cooperation (e.g., to implement industrial symbiosis). Tan and Guo also used the method to analyze the interactions between regulators, manufacturers, and recyclers (Tan and Guo, 2019). While the strength of OR methods is to provide an optimal (or as close as possible) answer to a CE research question, they are limited by high computational requirements and modeling time.
Other Methods
Methods from other fields than industrial ecology and complex systems science have also been applied to study CE, and each addresses a specific aspect of the concept. As those methods have been less used in the CE context, they are presented together in this section and are not included in the critical analysis. Some of those methods are also qualitative and, thus, outside the scope of the review.
First, qualitative research in socio-technical transitions may be used to frame the issue of implementing CE. The multi-level perspective framework, for instance, was used to assess the sustainability of the transition to a more circular economy in Australia's metals sector (Jackson et al., 2014). The study proposes ways to identify the three levels of the multi-level perspective (landscape, regime, and niche levels) and the tasks necessary to aid the transition in each of these levels. Next, in another study, the IPAT equation2 was combined with index decomposition analysis to assess the potential of the CE transition for environmental sustainability (Lonca et al., 2019). Various qualitative or semi-quantitative methods to implement circular business models have also been proposed (Bocken et al., 2019): backcasting techniques (which involves starting from the desired outcome and working backward to identify initiating conditions), screening tools, eco-design, and “serious games” (i.e., the use of games for another purpose than entertainment such as stirring innovation in a company) may help companies redefine their business models toward circularity. Finally, partial equilibrium and computable general equilibrium models have also been proposed to study the CE (Winning et al., 2017; García-Barragán et al., 2019). Winning et al. (2017), for instance, developed a computable general equilibrium model to study the economic effects of potential circular economy policies, suggesting that this type of model could be useful to assess CE at a macro-scale.
Circularity Metrics
A significant amount of research effort is also dedicated to designing appropriate metrics to assess CE (Corona et al., 2019). The future ISO TC323 standard aims to propose consensual ways of measuring CE (ISO, 2019) and will likely utilize metrics from existing methods. For instance, LCA metrics have been used to complement the material circularity indicator (MCI) and extend the scope of the CE assessment to identify potential environmental trade-offs that are not necessarily captured by the MCI (Lonca et al., 2018). Additionally, MFA is used to define general circularity metrics such as a cyclical use rate (measuring recycling) and the shares of secondary materials in the system's inputs and outputs (Tanzer and Rechberger, 2019) as well as according to the type of resource: energy, biomass or metals and minerals (Mayer et al., 2019). The EU is using several indicators grouped into three categories to measure its member's circularity performance: sustainable resource management, societal behavior, and business operations (European Commission, 2020a). The CE indicators are provided for each EU member, covering an even wider scope than of a country. Moreover, on a product level, the EU also developed sector-specific product environmental footprint category rules for companies to provide environmental information on their product in a harmonized way (European Commission, 2020b). Because of its broader scope, the CE indicators covering the material footprint of EU members is based on MFA; however, the product environmental footprint category rules are based on LCA and the circular footprint formula (Zampori and Pant, 2019). This discussion on metrics further highlights the critical role of applying and comparing diverse methods to study CE because each method provides specifics metrics. A complete review of circularity metrics is, however, out of the scope of this study.
Combination of Methods
Methods are often combined to alleviate each other's weaknesses. LCA has been used in combination with SD, ABM, EEIOA, and other methods to answer specific questions with more accuracy (Micolier et al., 2019; Yang, 2019). When SD is coupled with LCA, it adds temporal aspects to LCA (Peng et al., 2018), and LCA/SD coupling has been used to assess the economic and environmental performance of circular product systems (Asif et al., 2016). In another example of combined methods, EEIOA was used with ABM to study PV policies in Indonesia (Al Irsyad et al., 2019). SD and DES have also been combined, where SD enables the modeler to describe the system at a macro-scale, and DES can enrich this description with further details (Morgan et al., 2017). In some cases, different methods exhibit consistent mathematical structures, which help facilitate integration. Heijungs and Suh established such similar mathematical structures between EEIOA and LCA (and it follows that the methods may be integrated into so-called hybrid assessments) (Heijungs and Suh, 2002), while Davis et al. (2009) showed how the technology matrix could be represented by agents in ABM.
Our review of methods indicates that multiple methods have only been combined in specific instances (Table 1). Operations research methods have been extensively combined with methods from both the industrial ecology and complex systems science fields. Apart from operations research, it is evident from Table 1 that methods are mostly combined within their respective fields (i.e., industrial ecology and complex systems science). Moreover, while MCDM often uses results from other methods (e.g., LCA indicators), its use has been restrained to assessing specific life cycle stages and environmental or economic metrics within the context of the CE (Sassanelli et al., 2019).
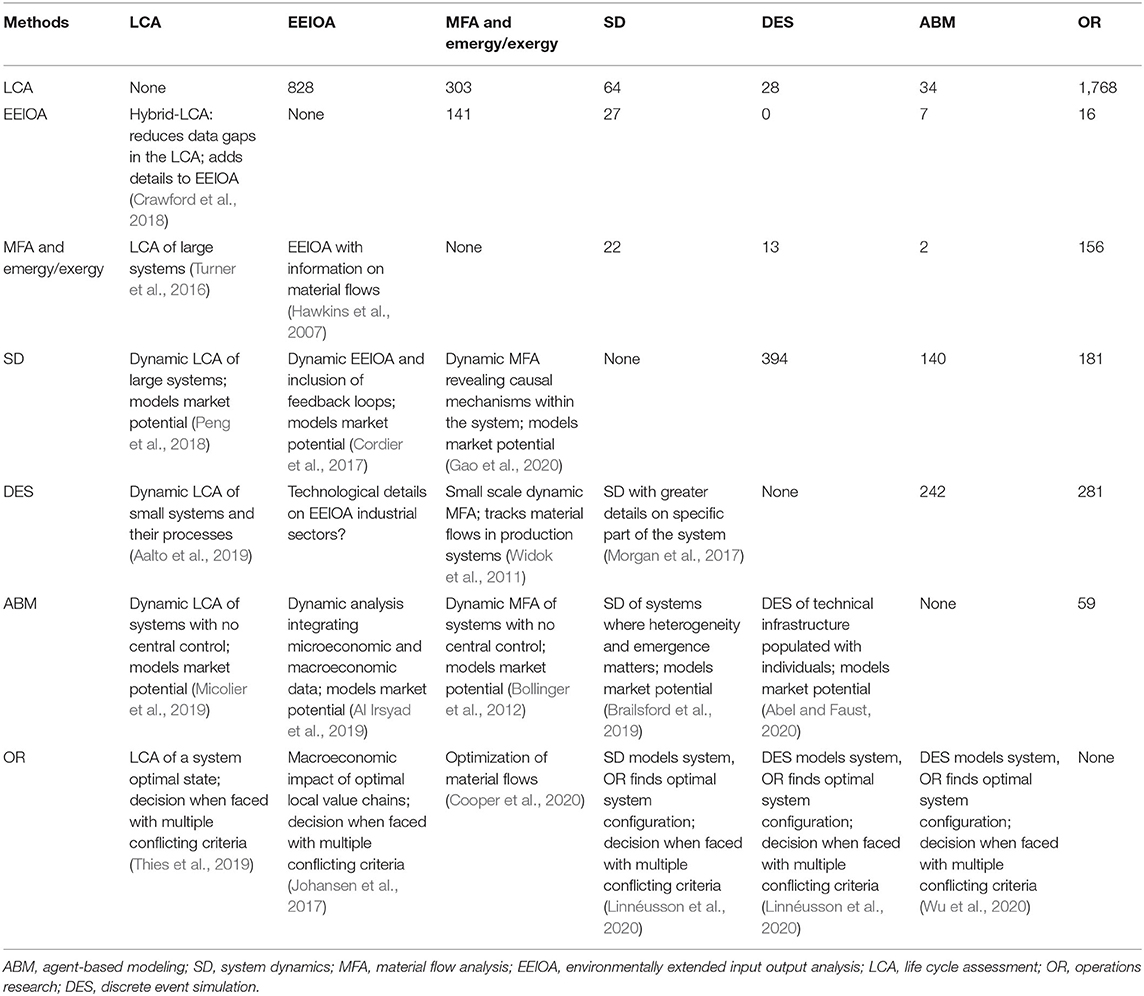
Table 1. Types of analysis covered when combining methods (and example from the literature) and number of results in the scopus database with query string “TITLE-ABS-KEY [“Method 1” AND (“Method 2”)].
However, a review of the strengths and weaknesses of each method (Table 2) highlights the need for additional work to combine methods to investigate specific research questions or case studies related to the CE. For example, in the context of the CE, ABM could be used to simulate consumers' waste management behaviors and determine which are the explanatory factors behind them, while LCA would inform on the environmental impacts of such behaviors (Figure 2).
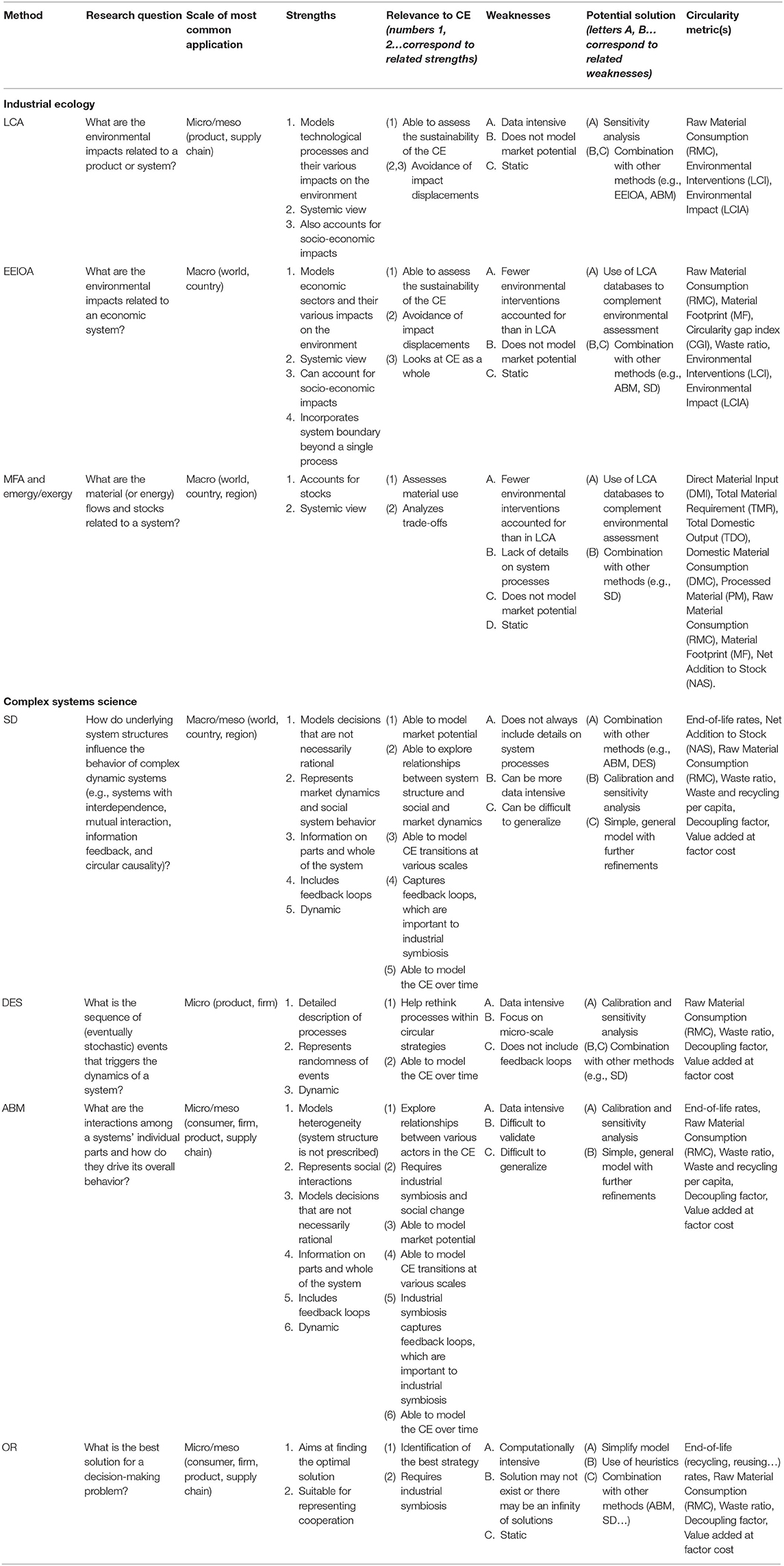
Table 2. Strengths and weaknesses of reviewed methods with respect to CE—the elements of the table (e.g., weaknesses) may not be exhaustive in order to stay concise and focus the analysis on CE.
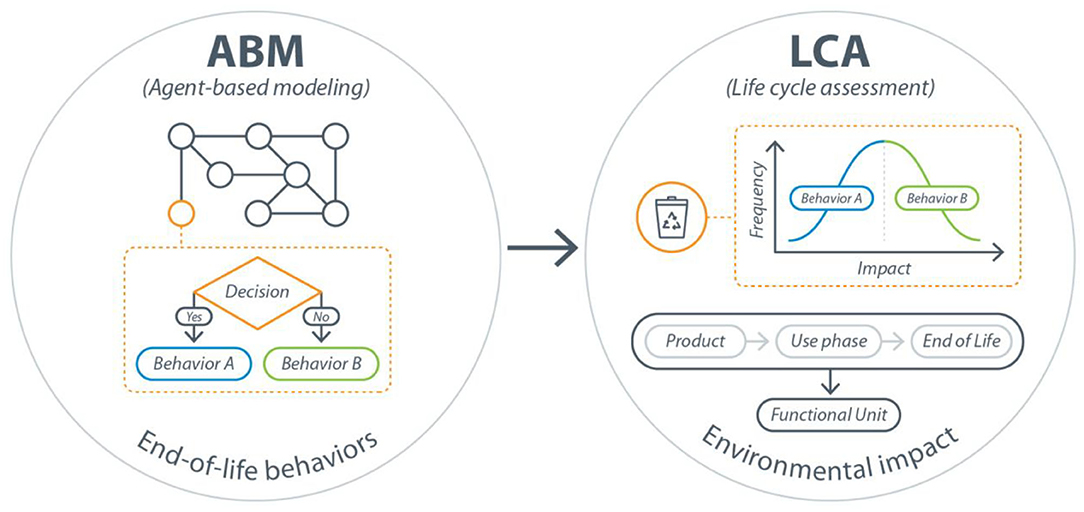
Figure 2. An example of the use of combined ABM and LCA in the context of the circular economy. The ABM method could enable representing the decisions of the various actors of the CE (e.g., consumers, manufacturers, and recyclers) while LCA allows the evaluations of the environmental impacts stemming from those decisions. Typical decisions for consumers would be, for instance, to recycle a product or dump it with the regular trash.
Critical Analysis
In this section, we analyze the methods presented above and assess their ability to model the CE, and quantitatively evaluate its contribution to sustainability. To that end, six criteria were used: (1) scope, (2) temporal resolution, (3) data requirements, (4) data granularity, (5) material efficiency potentials, and (6) sustainability completeness. Table 2 summarizes the results of the critical analysis.
Evaluating Material Efficiency Potentials and Sustainability Completeness
Figure 3 compares the methods that were evaluated according to their propensity to capture the different material efficiency potentials (technical, economic, and market; defined in section Review Method and Analysis Framework) and sustainability completeness that they perform. With respect to sustainability completeness, most methods are limited in their ability to account for all sustainability aspects, with the exceptions of EEIOA and LCA. Input-output analysis was first developed to provide an economic account of industrial production before being extended to include environmental and social aspects [e.g., to track greenhouse gas emissions and employment (Agez et al., 2019; Joyce et al., 2019)]. On the contrary, LCA was first developed to account for environmental impacts before being developed to include economic (with LCC) and social (with S-LCA) impacts (Fauzi et al., 2019). MFA can evaluate the contribution of a system to the environmental and economic pillars of sustainability but do not usually account for social aspects (Merli et al., 2018), while emergy and exergy focus on the environment through energy accounting. The literature analyzed herein also shows that methods from complex systems science usually focus on one of the sustainability pillars, tracking one or a few metrics at a time (e.g., rate of adoption of a technology, waste generated, or greenhouse gas emissions).
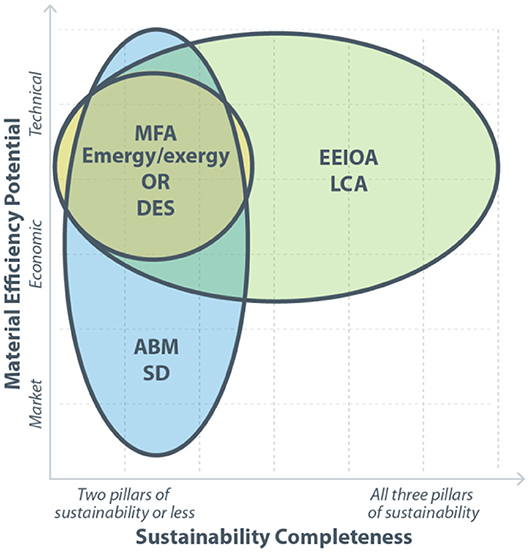
Figure 3. Capacity of reviewed methods to capture material efficiency potentials and sustainability completeness. Industrial ecology methods are limited to the techno-economic potential, while complex systems science methods allow studying the three material efficiency potentials (see ordinate). The directions of the figure's axis do not denote superiority but rather a logical order (e.g., the technical potential may be modeled before the economic and market potential). ABM, agent-based modeling; SD, system dynamics; MFA, material flow analysis; EEIOA, environmentally extended input-output analysis; LCA, life cycle assessment; OR, operations research; DES, discrete event simulation.
The ability of each method to capture material efficiency potentials also varies. For example, technical and economic potentials may be evaluated with LCA, EEIOA, and MFA/exergy/emergy. These methods, however, make it difficult to account for the market potential of CE strategies as they do not usually require collecting detailed data on the market level (consumers and individual firms), such as the rate of adoption of new technology. Methods from complex systems science, on the other hand, may be better adapted to represent market dynamics and, thus, evaluate the market potential of CE strategies. ABM and SD, for example, are the only methods that enable studying all three material efficiency potentials. However, their approaches for capturing these three potentials differ: SD is a top-down approach that defines a system in terms of flows and stocks, while ABM represents the system from a bottom-up perspective. These methods, however, are limited when studying environmental issues. Therefore, depending on the research question, it may be necessary to combine methods from different fields when assessing the CE contribution to sustainability.
Assessing Scope and Temporal Resolution
Methods also differ by their scope and temporal resolution (Figure 4). Regarding scope, LCAs are usually constructed at a meso scale, although they have been used to assess larger systems when combined with other approaches (Dandres et al., 2011; Querini and Benetto, 2015). EEIOA, however, covers a broader scope from a country to the global economy. MFA and emergy/exergy methods are usually applied to study regions or countries. Regarding the micro scale, DES looks primarily at industrial processes. Finally, ABM and SD are flexible in their scope, albeit the number of agents in practice limits ABM for computational reasons.
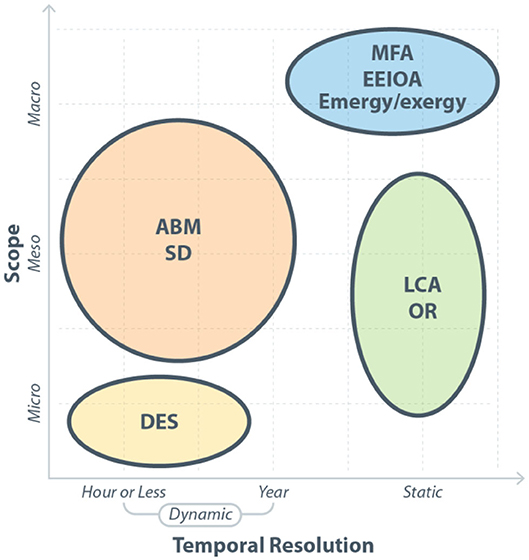
Figure 4. Scope and temporal resolution of reviewed methods. Industrial ecology methods are static methods, although they may be modified to include temporal aspects while complex systems science methods are dynamic. Both fields include methods that are more appropriate to the micro, meso, and macro scales. ABM, agent-based modeling; SD, system dynamics; MFA, material flow analysis; EEIOA, environmentally extended input-output analysis; LCA, life cycle assessment; OR, operations research; DES, discrete event simulation.
With respect to the temporal resolution, ABM and SD both consider time as discrete3, but they can include different temporal scales, from an hour, or less, to years. DES models may also have various temporal scales, although they are rarely used to study long-term phenomena. All other methods covered in the review are static (i.e., they do not account for time), although, in some cases, they can be modified to include temporal aspects. Levasseur et al. (2010), for instance, defined dynamic characterization factors to consider the fate of CO2 emissions in the atmosphere when performing LCA, and although EEIOA does not account for economic evolution, partial equilibrium and computable general equilibrium models may alleviate that issue. It is worth noting that although dynamic LCA is uncommon among practitioners, serious progress has been made by scholars toward incorporating dynamic aspects, including the publication of several frameworks (Beloin-Saint-Pierre et al., 2020). Finally, current industrial ecology methods may lead to errors in the estimation of landfills' impacts due to leachates, as the environmental models suffer from insufficient regionalization and compartmentation (Turner et al., 2017). Moreover, practitioners often adopt assumptions that underestimate long-term emissions (Turner et al., 2017). This is especially critical when assessing EOL options of products containing heavy metals such as electronics and batteries (Rydh and Karlström, 2002; Raugei et al., 2012). The regionalized IMPACT world+ method, however, alleviates some of those issues (Bulle et al., 2019).
Assessing Data Requirements and Granularity
The reviewed methods also differ in the amount and type of data they require (Figure 5). Due to its high level of detail, LCA requires a large amount of data (e.g., material and physical flows for the LCI and information on emissions fate for the LCIA). Comparatively, EEIOA only uses economic flows to model the system and does not account for as many environmental interventions as LCA (Huysman et al., 2016). MFA and emergy/exergy also require a moderate amount of data as they do not intend to model the system's processes in detail and focus on material and energy use rather than a broad range of environmental impacts (although exergy analysis may require a high quantity of data related to energy depending on the study). Although, in theory, OR methods could be used to study extensive systems in detail, in practice, this is not the case due to the high computational requirements and modeling time. Finally, other methods from complex systems science usually require a great amount of data.
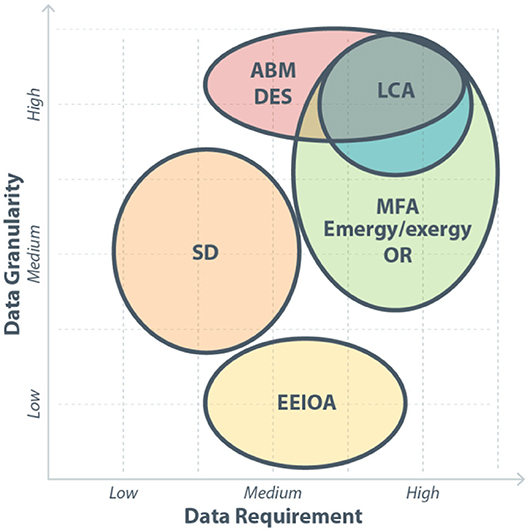
Figure 5. Type and amount of data required by reviewed methods. Data requirement (the amount of data needed by the method) is correlated to the data granularity (the level of precision of the data used by the method). ABM, agent-based modeling; SD, system dynamics; MFA, material flow analysis; EEIOA, environmentally extended input-output analysis; LCA, life cycle assessment; OR, operations research; DES, discrete event simulation.
With regards to the type of data, MFA and emergy/exergy use highly granular data, tracking all flows of a single material (or substance) and energy, respectively. In the same vein, LCA involves disaggregated data on intermediate products and environmental flows. Due to the scope of the method, EEIOA works with aggregated economic data (economic flows are tracked between broad economic sectors rather than processes), while complex systems science methods most often deal with highly granular data.
Strengths and Weaknesses of Each Method With Respect to CE Assessment
Table 2 summarizes the strengths and weaknesses of each of the reviewed methods with respect to its ability to assess the sustainability performance of the CE. We list the type of research questions of the eight main methods (or groups of methods) identified in section two, their strengths, their relevance to the CE, their weaknesses, potential solutions to overcome their weaknesses, and the main output metrics of each method.
Because the CE is a broad concept, we found that each method reviewed here is relevant for studying at least one aspect of the CE (refer to Table 2 for details). MFA approaches are relevant to study material use in a system while accounting for stocks and loops and are therefore particularly suited to examining circularity strategies related to closing the loops. In general, industrial ecology methods are suitable to assess the potential of the CE for sustainability, though they may not be best suited to studying market material efficiency potentials. There are two main reasons why industrial ecology methods fall short in doing so: (1) they are inherently static, and (2) they focus on the technological network rather than the social network of the socio-technical systems. Conversely, simulation methods such as ABM and SD are better suited to represent such market dynamics because they naturally include the system's temporal aspects and can represent social interactions. This strength is particularly relevant for the study of the CE transition. Indeed, the transition involves a wide range of actors (e.g., recyclers, producers, and consumers) that are connected through a social network. Moreover, the implementation of CE strategies occurs over time and depends on the interrelation of both the technological and social networks of the system. Simulation methods often require many different and complex types of data, but sensitivity analysis and calibration techniques may prove effective in mitigating this issue.
Overall, we find that no single method is currently able to capture all the important aspects of the CE. For example, traditional complex systems science methods lack metrics related to sustainability and industrial ecology methods lack dynamic modeling capabilities. These gaps highlight the need for combining multiple methods to better assess the sustainability performance of the CE. Several prior studies have done just that. They have used hybrid approaches, which combine multiple methods, to take advantage of the strengths of each and minimize their weaknesses (Table 1). For example, a combination of SD and DES presents the ability to model over multiple scales. In addition, hybrid assessment combining LCA and EEIOA may help alleviate the issue of data gaps in LCA (Agez et al., 2019), thus enabling the assessment of a CE strategy on a particular product as well as its economy-wide consequences. However, these methods have, thus far, not been applied as broadly as individual methods when it comes to analyzing the CE, and these combined approaches have generally only integrated methods within the same fields of research (e.g., EEIOA and LCA or SD and DES).
The results of this work indicate that combining methods across disciplines may prove particularly fruitful. An exciting avenue for future research could be to combine methods from complex systems science and industrial ecology with methods from operations research such as MCDM. In such an endeavor, the behavior of the studied system could be simulated with SD or ABM, while LCA or EEIOA (depending on the scope of the analysis) could provide information on the environmental impact of envisioned CE strategies. Lastly, MCDM could provide policy-makers with a fundamental basis to choose the best alternatives when disagreements occur within the included metrics. Another advantage of combining methods could be to extend the scope of the analysis. In doing so, unforeseen consequences that could dim the socioeconomic and environmental benefits of the CE, such as the rebound effect, could be captured. In collaborative consumption models, for instance, such environmental and socioeconomic rebound could occur (Walzberg et al., 2020a,b). As indicated in Table 1, further research is needed to streamline the combination of methods from different fields.
Limits of the Review
Other methods, such as integrated assessment models, have been used to study sustainability and the bioeconomy but have not been applied formally to CE yet (Lee, 2017). The purpose of this review was not to be exhaustive, however, but rather to give an overview and guidance over the methods that have mostly been used to study circular strategies. Moreover, for each method, we did not analyze all the articles applying the method to the study of circular strategies.
Summary and Analysis Implications
Based on our review of the literature, a variety of methods have been applied to assess the performance of the CE and its contribution to sustainability. We reviewed the approaches that have been used thus far and documented their strengths and weaknesses. The methods were further categorized into two fields: industrial ecology and complex systems science. Consistent with prior research (Merli et al., 2018), we find the highest number of articles related to CE assessment methods have come from the industrial ecology field. While methods from industrial ecology are able to characterize the sustainability of the CE, they are generally static and are, therefore, unable to capture market dynamics or social changes associated with CE transitions. However, the review also indicates that methods from complex systems science could play a critical role in helping CE stakeholders because these methods can incorporate the three material efficiency potentials along with temporal aspects, which allows for studying social changes that are essential for CE transitions. Despite the potential benefits of utilizing methods from complex systems science, we find that none of the methods that we reviewed can holistically assess the performance of a CE. It is also worth mentioning that most industrial ecology methods were developed based on the concept of sustainable production rather than sustainable consumption, which can create some constraints when evaluating some CE strategies. Complex systems science methods and further developments of industrial ecology methods (e.g., the development of Life cycle sustainability assessment combining LCA, LCC, and S-LCA) could, therefore, help study the consumption and production side of CE altogether.
The results of this work, particularly Tables 1, 2, will hopefully help guide CE researchers and policy-makers in their choice of an assessment method or combination of methods. First, the type of research question should provide a guideline toward what method to favor. Second, since assessment methods are more adapted to the macro scale (e.g., EEIOA and SD) or to the micro-scale (e.g., LCA and DES), the scope of the analysis should be defined. The temporal resolution should also be considered: one may opt for a static method (i.e., if the research question is to establish the degree of circularity of an existing system) or a dynamic method (i.e., to study the effect of specific policies or technological advancement). The types of metrics that are desired (e.g., environmental vs. circularity metrics), along with constraints on data availability and computational resources, may further limit the possible choices. The weaknesses of each method should also be understood and considered when choosing an assessment method. This work further indicates that combining multiple methods could be a powerful way to overcome these challenges because the strength of one method may be able to mitigate another's weakness (Table 1). Finally, although we did not review qualitative methods in detail, they could provide analytical frameworks for quantitative models. The multi-level perspective, for instance, can support the development of ABM when looking at transition phenomena (Hansen et al., 2019).
Finally, to answer the question in the title, it seems from the literature review that while a variety of existing methods could be used to assess the sustainability performance of the CE, they all have weaknesses that prevent them from addressing all of the components of CE transitions (e.g., the complex, dynamic, social, environmental, multi-actor, and multi-sector components of the CE). A great variety of methods can be (and have been) applied and combined to study circularity, and these existing methods can answer a significant number of research questions. However, no method is able to answer questions about the CE holistically. Thus, we find that while research on a single new method may not be needed, additional research is needed to develop a framework that combines existing methods to better accommodate all the necessary components for analyzing the CE.
It is also important to note that while this work did not perform a detailed assessment of CE metrics, better circularity metrics (e.g., that encompass environmental considerations) are also still being developed (Corona et al., 2019). A further discussion about metrics is, however, out of the scope of this review. What also seems paramount for CE, regardless of the focus (methods or metrics), is to improve communication and distillation of research results. This issue often arises when reporting sustainability studies such as LCA (Briem et al., 2019; Galindro et al., 2019) and should be avoided as CE transition occurs. Lastly, there is also a need for easy to use tools for practitioners [e.g., such as the EPA's sustainable materials management prioritization tools (EPA, 2020)] and improved data collection methods (e.g., through big data analysis) in order to support decision-making and modeling purposes (e.g., data on waste management are often lacking).
Conclusion
This review strives to identify the strengths and weaknesses of methods that are currently used to model and quantitatively assess the contribution of the CE to sustainability. We find that most of the methods that have been applied thus far have come from industrial ecology. However, the fields of complex systems science and operations research could add relevant dimensions when studying research questions involving, for instance, social changes or multiple metrics. Thus, combining methods may be a great way to gain more insights on how to transition effectively from a linear to a circular economy, and should include clear communication of study results and limitations.
Author Contributions
JW: conceptualization, methodology, investigation, and writing—original draft. GL and RH: conceptualization, investigation, and writing—review and editing. AE: writing—review and editing. AC and GH: supervision and writing—review and editing. All authors contributed to the article and approved the submitted version.
Conflict of Interest
The authors declare that the research was conducted in the absence of any commercial or financial relationships that could be construed as a potential conflict of interest.
Acknowledgments
This work was authored in part by the National Renewable Energy Laboratory, operated by Alliance for Sustainable Energy, LLC, for the U.S. Department of Energy (DOE) under Contract No. DE-AC36-08GO28308. Funding provided by the Advanced Manufacturing Office and the Office of Strategic Program. The views expressed in the article do not necessarily represent the views of the DOE or the U.S. Government. The U.S. Government retains and the publisher, by accepting the article for publication, acknowledges that the U.S. Government retains a non-exclusive, paid-up, irrevocable, worldwide license to publish or reproduce the published form of this work, or allow others to do so, for U.S. Government purposes. We would also like to thank the reviewers for their detailed feedback that helped us significantly improve this work.
Supplementary Material
The Supplementary Material for this article can be found online at: https://www.frontiersin.org/articles/10.3389/frsus.2020.620047/full#supplementary-material
Footnotes
1. ^Understood here as a collection of methods or a packaging of methods in a way that makes it easily accessible to others.
2. ^.
3. ^Although SD uses differential equations, they are solved numerically.
References
Aalto, M., Kc, R., Korpinen, O.-J., Karttunen, K., and Ranta, T. (2019). Modeling of biomass supply system by combining computational methods - a review article. Appl. Energy 243, 145–154. doi: 10.1016/j.apenergy.2019.03.201
Abadías Llamas, A., Valero Delgado, A., Valero Capilla, A., Torres Cuadra, C., Hultgren, M., Peltomäki, M., et al. (2019). Simulation-based exergy, thermo-economic and environmental footprint analysis of primary copper production. Minerals Eng. 131, 51–65. doi: 10.1016/j.mineng.2018.11.007
Abel, K. C., and Faust, K. M. (2020). Modeling complex human systems: an adaptable framework of urban food deserts. Sustain. Cities Soc. 52:101795. doi: 10.1016/j.scs.2019.101795
Agez, M., Majeau-Bettez, G., Margni, M., Strømman, A. H., and Samson, R. (2019). Lifting the veil on the correction of double counting incidents in hybrid life cycle assessment. J. Indus. Ecol. 24, 517–533. doi: 10.1111/jiec.12945
Ajzen, I. (1991). The theory of planned behavior. Organ. Behav. Hum. Decis. Process. 50, 179–211. doi: 10.1016/0749-5978(91)90020-T
Al Irsyad, M. I., Halog, A., and Nepal, R. (2019). Estimating the impacts of financing support policies towards photovoltaic market in Indonesia: a social-energy-economy-environment model simulation. J. Environ. Manage. 230, 464–473. doi: 10.1016/j.jenvman.2018.09.069
Altay, N., and Green, W. G. (2006). OR/MS research in disaster operations management. Eur. J. Operat. Res. 175, 475–493. doi: 10.1016/j.ejor.2005.05.016
Arushanyan, Y., Ekener-Petersen, E., and Finnveden, G. (2014). Lessons learned – review of LCAs for ICT products and services. Comput. Ind. 65, 211–234. doi: 10.1016/j.compind.2013.10.003
Asif, F. M. A., Lieder, M., and Rashid, A. (2016). Multi-method simulation based tool to evaluate economic and environmental performance of circular product systems. J. Cleaner Prod. 139, 1261–1281. doi: 10.1016/j.jclepro.2016.08.122
Badampudi, D., Wohlin, C., and Petersen, K. (2015). “Experiences from using snowballing and database searches in systematic literature studies,” in Proceedings of the 19th International Conference on Evaluation and Assessment in Software Engineering. Association for Computing Machinery, (Nanjing, China). doi: 10.1145/2745802.2745818
Baustert, P., and Benetto, E. (2017). Uncertainty analysis in agent-based modelling and consequential life cycle assessment coupled models: a critical review. J. Cleaner Prod. 156, 378–394. doi: 10.1016/j.jclepro.2017.03.193
BEA (2020). Bureau of Economic analysis - Input-Output Accounts Data. Available online at: https://www.bea.gov/ (accessed May 5, 2020).
Beloin-Saint-Pierre, D., Albers, A., Hélias, A., Tiruta-Barna, L., Fantke, P., Levasseur, A., et al. (2020). Addressing temporal considerations in life cycle assessment. Sci. Total Environ. 743:140700. doi: 10.1016/j.scitotenv.2020.140700
Bigano, A., Śniegocki, A., and Zotti, J. (2016). Policies for a more dematerialized eu economy. Theor. Underpin. Polit. Context Expect. Feasib. 8:717. doi: 10.3390/su8080717
Bocken, N., Strupeit, L., Whalen, K., and Nußholz, J. (2019). A review and evaluation of circular business model innovation tools. Sustainability 11:2210. doi: 10.3390/su11082210
Bollinger, L. A., Davis, C., Nikolić, I., and Dijkema, G. P. J. (2012). Modeling metal flow systems. J. Ind. Ecol. 16, 176–190. doi: 10.1111/j.1530-9290.2011.00413.x
Brailsford, S. C., Eldabi, T., Kunc, M., Mustafee, N., and Osorio, A. F. (2019). Hybrid simulation modelling in operational research: A state-of-the-art review. Euro. J. Oper. Res. 278, 721-737. doi: 10.1016/j.ejor.2018.10.025
Briem, A.-K., Betten, T., and Wehner, D. (2019). Personalized life cycle assessment – reflecting individuality within the methodological framework. Matér. Tech. 107:507. doi: 10.1051/mattech/2019030
Bulle, C., Margni, M., Patouillard, L., Boulay, A.-M., Bourgault, G., De Bruille, V., et al. (2019). IMPACT World+: a globally regionalized life cycle impact assessment method. Int. J. Life Cycle Assess. 24, 1653–1674. doi: 10.1007/s11367-019-01583-0
Cencic, O., and Rechberger, H. (2008). “Material flow analysis with software STAN,” in EnviroInfo, 440–447.
Chahla, G. A., and Zoughaib, A. (2019). Agent-based conceptual framework for energy and material synergy patterns in a territory with non-cooperative governance. Comput. Chem. Eng. 131:106596. doi: 10.1016/j.compchemeng.2019.106596
Charnley, F., Tiwari, D., Hutabarat, W., Moreno, M., Okorie, O., and Tiwari, A. (2019). Simulation to enable a data-driven circular economy. Sustainability 11:3379. doi: 10.3390/su11123379
Cooper, D. R., Ryan, N. A., Syndergaard, K., and Zhu, Y. (2020). The potential for material circularity and independence in the U.S. steel sector. J. Ind. Ecol. 24, 748–762. doi: 10.1111/jiec.12971
Cordier, M., Uehara, T., Weih, J., and Hamaide, B. (2017). An input-output economic model integrated within a system dynamics ecological model: feedback loop methodology applied to fish nursery restoration. Ecol. Econ. 140, 46–57. doi: 10.1016/j.ecolecon.2017.04.005
Corona, B., Shen, L., Reike, D., Rosales Carreón, J., and Worrell, E. (2019). Towards sustainable development through the circular economy—a review and critical assessment on current circularity metrics. Resourc. Conserv. Recycl. 151:104498. doi: 10.1016/j.resconrec.2019.104498
Crawford, R. H., Bontinck, P.-A., Stephan, A., Wiedmann, T., and Yu, M. (2018). Hybrid life cycle inventory methods - a review. J. Clean. Prod. 172, 1273-1288. doi: 10.1016/j.jclepro.2017.10.176
Daehn, K. E., Cabrera Serrenho, A., and Allwood, J. M. (2017). How will copper contamination constrain future global steel recycling? Environ. Sci. Technol. 51, 6599–6606. doi: 10.1021/acs.est.7b00997
Dandres, T., Gaudreault, C., Tirado-Seco, P., and Samson, R. (2011). Assessing non-marginal variations with consequential LCA: application to European energy sector. Renew. Sustain. Energy Rev. 15, 3121–3132. doi: 10.1016/j.rser.2011.04.004
Davis, C., Nikolić, I., and Dijkema, G. P. J. (2009). Integration of life cycle assessment into agent-based modeling. J. Ind. Ecol. 13, 306–325. doi: 10.1111/j.1530-9290.2009.00122.x
Deetman, S., Pauliuk, S., van Vuuren, D. P., van der Voet, E., and Tukker, A. (2018). Scenarios for demand growth of metals in electricity generation technologies, cars, and electronic appliances. Environ. Sci. Technol. 52, 4950–4959. doi: 10.1021/acs.est.7b05549
Donati, F., Aguilar-Hernandez, G. A., Sigüenza-Sánchez, C. P., de Koning, A., Rodrigues, J. F. D., and Tukker, A. (2020). Modeling the circular economy in environmentally extended input-output tables: methods, software and case study. Resourc. Conserv. Recycl. 152:104508. doi: 10.1016/j.resconrec.2019.104508
Ellen MacArthur Foundation (2013). Towards the Circular Economy: Opportunities for the consumer goods sector.
Ellen MacArthur Foundation (2020). Ellen MacArthur Foundation. Available online at: https://www.ellenmacarthurfoundation.org/ (accessed April 30, 2020).
EPA (2020). EPA's Sustainable Materials Management Prioritization Tools. Available online at: https://www.epa.gov/smm/sustainable-materials-management-smm-web-academy-webinar-sustainable-materials-management-smm (accessed May 05, 2020).
Ethan, H., Decker, S. E., Felisa, A., Smith, D. R., and Blake, R. F. S. (2000). Energy and material flow through the urban ecosystem. Annu. Rev. Energy Environ. 25, 685–740. doi: 10.1146/annurev.energy.25.1.685
European Commission (2020a). Circular Economy Indicators. Available online at: https://ec.europa.eu (accessed November 18, 2020).
European Commission (2020b). The Environmental Footprint Pilots. Available online at: https://ec.europa.eu (accessed November 18, 2020).
Fauzi, R. T., Lavoie, P., Sorelli, L., Heidari, M. D., and Amor, B. (2019). Exploring the current challenges and opportunities of life cycle sustainability assessment. Sustainability 11:636. doi: 10.3390/su11030636
Filippov, A. B. A. (2004). “From system dynamics and discrete event to practical agent based modeling: reasons, techniques, tools,” in The 22nd International Conference of the System Dynamics Society, (Oxford).
Fraccascia, L., Yazan, D. M., Albino, V., and Zijm, H. (2019). The role of redundancy in industrial symbiotic business development: a theoretical framework explored by agent-based simulation. Int. J. Prod. Econ. 221:107471. doi: 10.1016/j.ijpe.2019.08.006
Franco, M. A. (2019). A system dynamics approach to product design and business model strategies for the circular economy. J. Clean. Prod. 241:118327. doi: 10.1016/j.jclepro.2019.118327
Franklin-Johnson, E., Figge, F., and Canning, L. (2016). Resource duration as a managerial indicator for circular economy performance. J. Clean. Prod. 133, 589–598. doi: 10.1016/j.jclepro.2016.05.023
Galindro, B. M., Zanghelini, G. M., and Soares, S. R. (2019). Use of benchmarking techniques to improve communication in life cycle assessment: a general review. J. Clean. Prod. 213, 143–157. doi: 10.1016/j.jclepro.2018.12.147
Gao, C., Gao, C., Song, K., and Fang, K. (2020). Pathways towards regional circular economy evaluated using material flow analysis and system dynamics. Resourc. Conserv. Recycl. 154:104527. doi: 10.1016/j.resconrec.2019.104527
García-Barragán, J. F., Eyckmans, J., and Rousseau, S. (2019). Defining and measuring the circular economy: a mathematical approach. Ecol. Econ. 157, 369–372. doi: 10.1016/j.ecolecon.2018.12.003
Gbededo, M. A., Liyanage, K., and Garza-Reyes, J. A. (2018). Towards a life cycle sustainability analysis: a systematic review of approaches to sustainable manufacturing. J. Clean. Prod. 184, 1002–1015. doi: 10.1016/j.jclepro.2018.02.310
Ghali, M. R., Frayret, J.-M., and Ahabchane, C. (2017). Agent-based model of self-organized industrial symbiosis. J. Clean. Prod. 161, 452–465. doi: 10.1016/j.jclepro.2017.05.128
Ghisellini, P., Cialani, C., and Ulgiati, S. (2016). A review on circular economy: the expected transition to a balanced interplay of environmental and economic systems. J. Clean. Prod. 114, 11–32. doi: 10.1016/j.jclepro.2015.09.007
Ghisellini, P., and Ulgiati, S. (2020). Circular economy transition in Italy. Achievements, perspectives and constraints. J. Clean. Prod. 243:118360. doi: 10.1016/j.jclepro.2019.118360
Goedkoop, M. J. (1999). Product service systems, ecological and economic basics. Ministry of Housing, Spatial Planning and the Environment, Communications Directorate.
Graedel, T. E., and Cao, J. (2010). Metal spectra as indicators of development. Proc. Natl. Acad. Sci. U. S. A. 107, 20905–20910. doi: 10.1073/pnas.1011019107
Grüne-Yanoff, T., and Weirich, P. (2010). The philosophy and epistemology of simulation: a review. Simul. Gaming 41, 20–50. doi: 10.1177/1046878109353470
Haas, W., Krausmann, F., Wiedenhofer, D., and Heinz, M. (2015). How circular is the global economy? an assessment of material flows, waste production, and recycling in the European union and the world in 2005. J. Ind. Ecol. 19, 765–777. doi: 10.1111/jiec.12244
Hansen, P., Liu, X., and Morrison, G. M. (2019). Agent-based modelling and socio-technical energy transitions: a systematic literature review. Energy Res. Soc. Sci. 49, 41–52. doi: 10.1016/j.erss.2018.10.021
Hargreaves-Heap, S., and Varoufakis, Y. (1995). Game Theory: a Critical Introduction. Routledge. doi: 10.4324/9780203199275
Hawkins, T., Hendrickson, C., Higgins, C., Matthews, H. S., and Suh, S. (2007). A Mixed-Unit Input-Output Model for Environmental Life-Cycle Assessment and Material Flow Analysis. Environ. Sci. Technol. 41, 1024–1031. doi: 10.1021/es060871u
Heijungs, R., and Suh, S. (2002). The Computational Structure of Life Cycle Assessment. The Netherlands: Kluwer Academic Publisher Dordrecht. doi: 10.1007/978-94-015-9900-9
Hellweg, S., and i Canals, L. M. (2014). Emerging approaches, challenges and opportunities in life cycle assessment. Science 344, 1109–1113. doi: 10.1126/science.1248361
Hinkelmann, F., Murrugarra, D., Jarrah, A. S., and Laubenbacher, R. (2011). A mathematical framework for agent based models of complex biological networks. Bull. Math. Biol. 73, 1583–1602. doi: 10.1007/s11538-010-9582-8
Huang, S.-L., Lee, C.-L., and Chen, C.-W. (2006). Socioeconomic metabolism in Taiwan: emergy synthesis versus material flow analysis. Resourc. Conserv. Recycl. 48, 166–196. doi: 10.1016/j.resconrec.2006.01.005
Huysman, S., De Schaepmeester, J., Ragaert, K., Dewulf, J., and De Meester, S. (2017). Performance indicators for a circular economy: a case study on post-industrial plastic waste. Resourc. Conserv. Recycl. 120, 46–54. doi: 10.1016/j.resconrec.2017.01.013
Huysman, S., Schaubroeck, T., Goralczyk, M., Schmidt, J., and Dewulf, J. (2016). Quantifying the environmental impacts of a European citizen through a macro-economic approach, a focus on climate change and resource consumption. J. Clean. Prod. 124, 217–225. doi: 10.1016/j.jclepro.2016.02.098
Iacovidou, E., Velis, C. A., Purnell, P., Zwirner, O., Brown, A., Hahladakis, J., et al. (2017). Metrics for optimising the multidimensional value of resources recovered from waste in a circular economy: a critical review. J. Clean. Prod. 166, 910–938. doi: 10.1016/j.jclepro.2017.07.100
Imbeault-Tétreault, H. (2017). Analyse du Cycle de vie des Sacs d'emplettes au Québec. Recyc-Québec: Montréal.
Jackson, M., Lederwasch, A., and Giurco, D. (2014). Transitions in theory and practice: managing metals in the circular economy. Resources 3, 516–543. doi: 10.3390/resources3030516
Jamali-Zghal, N., Lacarrière, B., and Le Corre, O. (2015). Metallurgical recycling processes: sustainability ratios and environmental performance assessment. Resourc. Conserv. Recycl. 97, 66–75. doi: 10.1016/j.resconrec.2015.02.010
Jeswani, H. K., Azapagic, A., Schepelmann, P., and Ritthoff, M. (2010). Options for broadening and deepening the LCA approaches. J. Clean. Prod. 18, 120–127. doi: 10.1016/j.jclepro.2009.09.023
Johansen, U., Werner, A., and Nørstebø, V. (2017). Optimizing the wood value chain in northern norway taking into account national and regional economic trade-offs. Forests 8:172. doi: 10.3390/f8050172
Joyce, P. J., Finnveden, G., Håkansson, C., and Wood, R. (2019). A multi-impact analysis of changing ICT consumption patterns for Sweden and the EU: indirect rebound effects and evidence of decoupling. J. Clean. Prod. 211, 1154–1161. doi: 10.1016/j.jclepro.2018.11.207
Kalmykova, Y., Sadagopan, M., and Rosado, L. (2018). Circular economy – from review of theories and practices to development of implementation tools. Resourc. Conserv. Recycl. 135, 190–201. doi: 10.1016/j.resconrec.2017.10.034
Knoeri, C., Wäger, P. A., Stamp, A., Althaus, H.-J., and Weil, M. (2013). Towards a dynamic assessment of raw materials criticality: linking agent-based demand — with material flow supply modelling approaches. Sci. Total Environ. 461–462, 808–812. doi: 10.1016/j.scitotenv.2013.02.001
Korhonen, J., Honkasalo, A., and Seppälä, J. (2018). Circular economy: the concept and its limitations. Ecol. Econ. 143, 37–46. doi: 10.1016/j.ecolecon.2017.06.041
Krausmann, F., Schandl, H., Eisenmenger, N., Giljum, S., and Jackson, T. (2017). Material flow accounting: measuring global material use for sustainable development. Annu. Rev. Environ. Resourc. 42, 647–675. doi: 10.1146/annurev-environ-102016-060726
Kristensen, H. S., and Mosgaard, M. A. (2020). A review of micro level indicators for a circular economy - moving away from the three dimensions of sustainability? J. Clean. Prod. 243:118531. doi: 10.1016/j.jclepro.2019.118531
Laengle, S., Merigó, J. M., Miranda, J., Słowiński, R., Bomze, I., Borgonovo, E., et al. (2017). Forty years of the European journal of operational research: a bibliometric overview. Eur. J. Operat. Res. 262, 803–816. doi: 10.1016/j.ejor.2017.04.027
Laso, J., García-Herrero, I., Margallo, M., Vázquez-Rowe, I., Fullana, P., Bala, A., et al. (2018). Finding an economic and environmental balance in value chains based on circular economy thinking: an eco-efficiency methodology applied to the fish canning industry. Resourc. Conserv. Recycl. 133, 428–437. doi: 10.1016/j.resconrec.2018.02.004
Lee, D.-H. (2017). Econometric assessment of bioenergy development. Int. J. Hydr. Energy 42, 27701–27717. doi: 10.1016/j.ijhydene.2017.08.055
Levasseur, A., Lesage, P., Margni, M., Deschênes, L., and Samson, R. (2010). Considering time in LCA: dynamic LCA and its application to global warming impact assessments. Environ. Sci. Technol. 44, 3169–3174. doi: 10.1021/es9030003
Lieder, M., Asif, F. M. A., and Rashid, A. (2017). Towards Circular Economy implementation: an agent-based simulation approach for business model changes. Auton. Agents Multi-Agent Syst. 31, 1377–1402. doi: 10.1007/s10458-017-9365-9
Lieder, M., and Rashid, A. (2016). Towards circular economy implementation: a comprehensive review in context of manufacturing industry. J. Clean. Prod. 115, 36–51. doi: 10.1016/j.jclepro.2015.12.042
Linder, M., Sarasini, S., and van Loon, P. (2017). A metric for quantifying product-level circularity. J. Ind. Ecol. 21, 545–558. doi: 10.1111/jiec.12552
Linnéusson, G., Ng, A. H. C., and Aslam, T. (2020). A hybrid simulation-based optimization framework supporting strategic maintenance development to improve production performance. Euro. J. Oper. Res. 281, 402–414. doi: 10.1016/j.ejor.2019.08.036
Lonca, G., Bernard, S., and Margni, M. J. J. (2019). A versatile approach to assess circularity: the case of decoupling. J. Clean. Prod. 240:118174. doi: 10.1016/j.jclepro.2019.118174
Lonca, G., Muggéo, R., Imbeault-Tétreault, H., Bernard, S., and Margni, M. (2018). Does material circularity rhyme with environmental efficiency? Case studies on used tires. J. Clean. Prod. 183, 424–435. doi: 10.1016/j.jclepro.2018.02.108
Lopes Silva, D. A., de Oliveira, J. A., Saavedra, Y. M. B., Ometto, A. R., Rieradevall i Pons, J., and Gabarrell Durany, X. (2015). Combined MFA and LCA approach to evaluate the metabolism of service polygons: a case study on a university campus. Resourc. Conserv. Recycl. 94, 157–168. doi: 10.1016/j.resconrec.2014.11.001
Luna-Reyes, L. F., and Andersen, D. L. (2003). Collecting and analyzing qualitative data for system dynamics: methods and models. Syst. Dyn. Rev. 19, 271–296. doi: 10.1002/sdr.280
Luo, M., Song, X., Hu, S., and Chen, D. (2019). Towards the sustainable development of waste household appliance recovery systems in China: an agent-based modeling approach. J. Clean. Prod. 220, 431–444. doi: 10.1016/j.jclepro.2019.02.128
Mayer, A., Haas, W., Wiedenhofer, D., Krausmann, F., Nuss, P., and Blengini, G. A. (2019). Measuring progress towards a circular economy: a monitoring framework for economy-wide material loop closing in the EU28. J. Industr. Ecol. 23, 62–76. doi: 10.1111/jiec.12809
Mehlhart, G., Bakas, I., Herczeg, M., Strosser, P., Rynikiewicz, C., Agenais, A., et al. (2016). Study on the Energy Saving Potential of Increasing Resource Efficiency. Final Report, Study prepared for the European Commission, Directorate General Environment. doi: 10.2779/172229
Meng, X., Wen, Z., and Qian, Y. (2018). Multi-agent based simulation for household solid waste recycling behavior. Resourc. Conserv. Recycl. 128, 535–545. doi: 10.1016/j.resconrec.2016.09.033
Merli, R., Preziosi, M., and Acampora, A. (2018). How do scholars approach the circular economy? A systematic literature review. J. Clean. Prod. 178, 703–722. doi: 10.1016/j.jclepro.2017.12.112
Micolier, A., Loubet, P., Taillandier, F., and Sonnemann, G. (2019). To what extent can agent-based modelling enhance a life cycle assessment? Answers based on a literature review. J. Clean. Prod. 239:118123. doi: 10.1016/j.jclepro.2019.118123
Miller, R. E., and Blair, P. D. (2009). Input-Output Analysis: Foundations and Extensions. Cambridge: Cambridge University Press. doi: 10.1017/CBO9780511626982
Moraga, G., Huysveld, S., Mathieux, F., Blengini, G. A., Alaerts, L., Van Acker, K., et al. (2019). Circular economy indicators: what do they measure? Resourc. Conserv. Recycl. 146, 452–461. doi: 10.1016/j.resconrec.2019.03.045
Morgan, J. S., Howick, S., and Belton, V. (2017). A toolkit of designs for mixing discrete event simulation and system dynamics. Eur. J. Operat. Res. 257, 907–918. doi: 10.1016/j.ejor.2016.08.016
Nadel, S., Shipley, A., and Elliott, R. N. (2004). “The technical, economic and achievable potential for energy-efficiency in the US–A meta-analysis of recent studies,” in Proceedings of the 2004 ACEEE Summer Study on Energy Efficiency in Buildings. Citeseer. 215–218.
Palafox-Alcantar, P. G., Hunt, D. V. L., and Rogers, C. D. F. (2020). The complementary use of game theory for the circular economy: a review of waste management decision-making methods in civil engineering. Waste Manage. 102, 598–612. doi: 10.1016/j.wasman.2019.11.014
Pan, H., Zhang, X., Wang, Y., Qi, Y., Wu, J., Lin, L., et al. (2016). Emergy evaluation of an industrial park in sichuan province, China: a modified emergy approach and its application. J. Clean. Prod. 135, 105–118. doi: 10.1016/j.jclepro.2016.06.102
Parchomenko, A., Nelen, D., Gillabel, J., and Rechberger, H. (2019). Measuring the circular economy - a multiple correspondence analysis of 63 metrics. J. Clean. Prod. 210, 200–216. doi: 10.1016/j.jclepro.2018.10.357
Park, J.-A., Hong, S.-J., Kim, I., Lee, J.-Y., and Hur, T. (2011). Dynamic material flow analysis of steel resources in Korea. Resourc. Conserv. Recycl. 55, 456–462. doi: 10.1016/j.resconrec.2010.12.007
Pearce, D. W., and Turner, R. K. (1990). Economics of Natural Resources and the Environment. JHU Press.
Peng, S., Li, T., Wang, Y., Liu, Z., Tan, G. Z., and Zhang, H. (2018). Prospective life cycle assessment based on system dynamics approach: a case study on the large-scale centrifugal compressor. J. Manufact. Sci. Eng. 141:021003. doi: 10.1115/1.4041950
Querini, F., and Benetto, E. (2015). Combining agent-based modeling and life cycle assessment for the evaluation of mobility policies. Environ. Sci. Technol. 49, 1744–1751. doi: 10.1021/es5060868
Rand, W. (2019). “Theory-interpretable, data-driven agent-based modeling,” in Social-Behavioral Modeling for Complex Systems, eds P. K. Davis, A. O'Mahony and J. Pfautz (John Wiley & Sons), 337–357. doi: 10.1002/9781119485001.ch15
Raugei, M., Isasa, M., and Fullana Palmer, P. (2012). Potential Cd emissions from end-of-life CdTe PV. Int. J. Life Cycle Assess. 17, 192–198. doi: 10.1007/s11367-011-0348-9
Rincón, L., Castell, A., Pérez, G., Solé, C., Boer, D., and Cabeza, L. F. (2013). Evaluation of the environmental impact of experimental buildings with different constructive systems using material flow analysis and life cycle assessment. Appl. Energy 109, 544–552. doi: 10.1016/j.apenergy.2013.02.038
Rydh, C. J., and Karlström, M. (2002). Life cycle inventory of recycling portable nickel–cadmium batteries. Resourc. Conserv. Recycl. 34, 289–309. doi: 10.1016/S0921-3449(01)00114-8
Saidani, M., Yannou, B., Leroy, Y., Cluzel, F., and Kendall, A. (2019). A taxonomy of circular economy indicators. J. Clean. Prod. 207, 542–559. doi: 10.1016/j.jclepro.2018.10.014
Sassanelli, C., Rosa, P., Rocca, R., and Terzi, S. (2019). Circular economy performance assessment methods: a systematic literature review. J. Clean. Prod. 229, 440–453. doi: 10.1016/j.jclepro.2019.05.019
Schetters, M. J. A., van der Hoek, J. P., Kramer, O. J. I., Kors, L. J., Palmen, L. J., Hofs, B., et al. (2014). Circular economy in drinking water treatment: reuse of ground pellets as seeding material in the pellet softening process. Water Sci. Technol. 71, 479–486. doi: 10.2166/wst.2014.494
Sovacool, B. K., Ali, S. H., Bazilian, M., Radley, B., Nemery, B., Okatz, J., et al. (2020). Sustainable minerals and metals for a low-carbon future. Science 367, 30–33. doi: 10.1126/science.aaz6003
Stadler, K., Wood, R., Bulavskaya, T., Södersten, C.-J., Simas, M., Schmidt, S., et al. (2018). EXIOBASE 3: developing a time series of detailed environmentally extended multi-regional input-output tables. J. Ind. Ecol. 22, 502–515. doi: 10.1111/jiec.12715
Suh, S. (2004). Functions, commodities and environmental impacts in an ecological–economic model. Ecol. Econ. 48, 451–467. doi: 10.1016/j.ecolecon.2003.10.013
Tan, Y., and Guo, C. (2019). Research on two-way logistics operation with uncertain recycling quality in government multi-policy environment. Sustainability 11:882. doi: 10.3390/su11030882
Tanzer, J., and Rechberger, H. (2019). Setting the common ground: a generic framework for material flow analysis of complex systems. Recycling 4:23. doi: 10.3390/recycling4020023
Thaler, R. H., and Sunstein, C. R. (2008). Nudge : Improving Decisions About Health, Wealth, and Happiness. Yale University Press.
Thies, C., Kieckhäfer, K., Spengler, T. S., and Sodhi, M. S. (2019). Operations research for sustainability assessment of products: a review. Eur. J. Operat. Res. 274, 1–21. doi: 10.1016/j.ejor.2018.04.039
Tong, X., Nikolic, I., Dijkhuizen, B., van den Hoven, M., Minderhoud, M., Wäckerlin, N., et al. (2018). Behaviour change in post-consumer recycling: applying agent-based modelling in social experiment. J. Clean. Prod. 187, 1006–1013. doi: 10.1016/j.jclepro.2018.03.261
Turner, D. A., Beaven, R. P., and Woodman, N. D. (2017). Evaluating landfill aftercare strategies: a life cycle assessment approach. Waste Manage. 63, 417–431. doi: 10.1016/j.wasman.2016.12.005
Turner, D. A., Williams, I. D., and Kemp, S. (2016). Combined material flow analysis and life cycle assessment as a support tool for solid waste management decision making. J. Cleaner Prod. 129, 234–248. doi: 10.1016/j.jclepro.2016.04.077
Umair, S., Björklund, A., and Petersen, E. E. (2013). “Social life cycle inventory and impact assessment of informal recycling of electronic ICT waste in Pakistan,” in Proceedings of the First International Conference on Information and Communication Technologies for Sustainability ETH Zurich, eds L. Hilty, E. Aebischer, G. Andersson, and W. Lohmann (Zurich), 52–58.
Vendries, J., Sauer, B., Hawkins, T. R., Allaway, D., Canepa, P., Rivin, J., et al. (2020). The significance of environmental attributes as indicators of the life cycle environmental impacts of packaging and food service ware. Environ. Sci. Technol. 54, 5356–5364. doi: 10.1021/acs.est.9b07910
Voskamp, I. M., Stremke, S., Spiller, M., Perrotti, D., van der Hoek, J. P., and Rijnaarts, H. H. M. (2017). Enhanced performance of the eurostat method for comprehensive assessment of urban metabolism: a material flow analysis of amsterdam. J. Ind. Ecol. 21, 887–902. doi: 10.1111/jiec.12461
Walker, S., Coleman, N., Hodgson, P., Collins, N., and Brimacombe, L. (2018). Evaluating the environmental dimension of material efficiency strategies relating to the circular economy. Sustainability 10:666. doi: 10.3390/su10030666
Walzberg, J., Dandres, T., Merveille, N., Cheriet, M., and Samson, R. (2019). Assessing behavioural change with agent-based life cycle assessment: application to smart homes. Renew. Sustain. Energy Rev. 111, 365–376. doi: 10.1016/j.rser.2019.05.038
Walzberg, J., Dandres, T., Merveille, N., Cheriet, M., and Samson, R. (2020a). Should we fear the rebound effect in smart homes? Renew. Sustain. Energy Rev. 125:109798. doi: 10.1016/j.rser.2020.109798
Walzberg, J., Lonca, G., and Deschênes, J. (2020b). “Collaborative consumption and sustainability: lifting the veil on unforeseen adverse effects,” in International Conference on Resource Sustainability, (Dublin, Ireland).
Widok, A. H., Wohlgemuth, V., and Page, B. (2011). “Combining sustainability criteria with discrete event simulation,” in Proceedings of the 2011 Winter Simulation Conference (WSC), 859–870. doi: 10.1109/WSC.2011.6147812
Wiebe, K. S., Harsdorff, M., Montt, G., Simas, M. S., and Wood, R. (2019). Global circular economy scenario in a multi-regional input–output framework. Environ. Sci. Technol. 53, 6362–6373. doi: 10.1021/acs.est.9b01208
Wiedenhofer, D., Fishman, T., Lauk, C., Haas, W., and Krausmann, F. (2019). Integrating material stock dynamics into economy-wide material flow accounting: concepts, modelling, and global application for 1900–2050. Ecol. Econ. 156, 121–133. doi: 10.1016/j.ecolecon.2018.09.010
Wilensky, U., and Rand, W. (2015). An Introduction to Agent-Based Modeling: Modeling Natural, Social, and Engineered Complex Systems with NetLogo. MIT Press.
Winning, M., Calzadilla, A., Bleischwitz, R., Nechifor, V. J. I. E., and Policy, E. (2017). Towards a circular economy: insights based on the development of the global ENGAGE-materials model and evidence for the iron and steel industry. Int. Econ. Econ. Policy 14, 383–407. doi: 10.1007/s10368-017-0385-3
World Business Council for Sustainable Development (2020). Available online at: https://www.wbcsd.org/ (accessed October 13, 2020).
World Economic Forum (2020). Available online at: https://www.weforum.org/ (accessed October 13, 2020).
Wu, Z., Zhou, M., Zhang, T., Li, G., Zhang, Y., and Liu, X. (2020). Imbalance settlement evaluation for China's balancing market design via an agent-based model with a multiple criteria decision analysis method. Energy Policy 139:111297. doi: 10.1016/j.enpol.2020.111297
Xu, Y., Zhang, L., Yeh, C.-H., and Liu, Y. (2018). Evaluating WEEE recycling innovation strategies with interacting sustainability-related criteria. J. Clean. Prod. 190, 618–629. doi: 10.1016/j.jclepro.2018.04.078
Yang, Y. (2019). A unified framework of life cycle assessment. Int. J. Life Cycle Assess. 24, 620–626. doi: 10.1007/s11367-019-01595-w
Yousef, M. S., and Hassan, H. (2019). Assessment of different passive solar stills via exergoeconomic, exergoenvironmental, and exergoenviroeconomic approaches: a comparative study. Solar Energy 182, 316–331. doi: 10.1016/j.solener.2019.02.042
Keywords: circular economy, material efficiency, industrial ecology, circularity metrics, sustainability assessment, complex systems science
Citation: Walzberg J, Lonca G, Hanes RJ, Eberle AL, Carpenter A and Heath GA (2021) Do We Need a New Sustainability Assessment Method for the Circular Economy? A Critical Literature Review. Front. Sustain. 1:620047. doi: 10.3389/frsus.2020.620047
Received: 21 October 2020; Accepted: 07 December 2020;
Published: 07 January 2021.
Edited by:
Dingsheng Li, University of Nevada, Reno, United StatesReviewed by:
Joanna Kulczycka, AGH University of Science and Technology, PolandMohammad Ali Rajaeifar, Newcastle University, United Kingdom
Copyright © 2021 Walzberg, Lonca, Hanes, Eberle, Carpenter and Heath. This is an open-access article distributed under the terms of the Creative Commons Attribution License (CC BY). The use, distribution or reproduction in other forums is permitted, provided the original author(s) and the copyright owner(s) are credited and that the original publication in this journal is cited, in accordance with accepted academic practice. No use, distribution or reproduction is permitted which does not comply with these terms.
*Correspondence: Julien Walzberg, anVsaWVuLndhbHpiZXJnQG5yZWwuZ292