- 1Institute of Geosciences, University of Potsdam, Potsdam, Germany
- 2Human Origins Program, National Museum of Natural History, Smithsonian Institution, Washington, DC, United States
- 3Department of Biology, Faculty of Science, Muni University, Arua, Uganda
- 4Center for Conservation Genomics, Smithsonian National Zoological Park and Conservation Biology Institute, Smithsonian Institution, Washington, DC, United States
- 5Department of Biological Sciences, Chicago State University, Chicago, IL, United States
- 6Center for Species Survival, Smithsonian National Zoological Park and Conservation Biology Institute, Smithsonian Institution, Front Royal, VA, United States
- 7Department of Environmental Science and Public Policy, George Mason University, Fairfax, VA, United States
- 8School of Systems Biology, George Mason University, Fairfax, VA, United States
- 9Department of Chemical Analytics and Biogeochemistry, Leibniz-Institute of Freshwater Ecology and Inland Fisheries (IGB), Berlin, Germany
- 10Department of Biology, George Mason University, Fairfax, VA, United States
- 11Department of Biology, Faculty of Science, Mbarara University of Science and Technology, Mbarara, Uganda
Sedimentary ancient DNA has been proposed as a key methodology for reconstructing biodiversity over time. Yet, despite the concentration of Earth’s biodiversity in the tropics, this method has rarely been applied in this region. Moreover, the taphonomy of sedimentary DNA, especially in tropical environments, is poorly understood. This study elucidates challenges and opportunities of sedimentary ancient DNA approaches for reconstructing tropical biodiversity. We present shotgun-sequenced metagenomic profiles and DNA degradation patterns from multiple sediment cores from Mubwindi Swamp, located in Bwindi Impenetrable Forest (Uganda), one of the most diverse forests in Africa. We describe the taxonomic composition of the sediments covering the past 2200 years and compare the sedimentary DNA data with a comprehensive set of environmental and sedimentological parameters to unravel the conditions of DNA degradation. Consistent with the preservation of authentic ancient DNA in tropical swamp sediments, DNA concentration and mean fragment length declined exponentially with age and depth, while terminal deamination increased with age. DNA preservation patterns cannot be explained by any environmental parameter alone, but age seems to be the primary driver of DNA degradation in the swamp. Besides degradation, the presence of living microbial communities in the sediment also affects DNA quantity. Critically, 92.3% of our metagenomic data of a total 81.8 million unique, merged reads cannot be taxonomically identified due to the absence of genomic references in public databases. Of the remaining 7.7%, most of the data (93.0%) derive from Bacteria and Archaea, whereas only 0–5.8% are from Metazoa and 0–6.9% from Viridiplantae, in part due to unbalanced taxa representation in the reference data. The plant DNA record at ordinal level agrees well with local pollen data but resolves less diversity. Our animal DNA record reveals the presence of 41 native taxa (16 orders) including Afrotheria, Carnivora, and Ruminantia at Bwindi during the past 2200 years. Overall, we observe no decline in taxonomic richness with increasing age suggesting that several-thousand-year-old information on past biodiversity can be retrieved from tropical sediments. However, comprehensive genomic surveys of tropical biota need prioritization for sedimentary DNA to be a viable methodology for future tropical biodiversity studies.
Introduction
The tropics hold the greatest biodiversity on Earth (e.g., Gaston, 2000; Jenkins et al., 2013) containing about three quarters of all species (Barlow et al., 2018). It remains a major scientific challenge to unravel the processes that led to this exceptional diversity and to test existing models on the diversification of tropical biota (e.g., Stevens, 1989; Mittelbach et al., 2007; Pennington et al., 2015). Yet elucidating the evolution of tropical biodiversity and the assembly of tropical biotic communities over time is generally hampered by the lack of a taxonomically complete fossil record and the absence of exhaustive phylo- and biogeographic information. In particular tropical rainforests – the most diverse terrestrial ecosystems – are largely characterized by a poor fossil record (Wing et al., 2009; Jacobs et al., 2010), which severely limits the reconstruction of diversity patterns. For example, the mammalian fossil record for the past 20,000 years of Africa only contains few taxa from the large Congo Basin (Jousse, 2017) where several hundreds of mammal species occur today (Jenkins et al., 2013). Past diversity and community structure of small rainforest vertebrates or insects may be reconstructed from amber deposits (e.g., Wilson, 1985; Sherratt et al., 2015), which, however, are of very localized occurrence (Poinar, 1992). Floristic diversity can be reconstructed from pollen records, but generally suffer from limited taxonomic resolution in comparison to the existing tropical plant diversity (e.g., Morley, 2000). Hence, a comprehensive picture of the history of tropical biodiversity is still far from being established.
In addition to addressing this challenging scientific problem, the ongoing collapse in biodiversity in the tropics (Ceballos et al., 2017; Barlow et al., 2018) resulting from anthropogenically driven habitat degradation, deforestation, forest fragmentation, and defaunation necessitates well-documented species inventories for successful conservation management. Establishing baseline data on the “pre-disturbance” occurrence of species within a geographic area is relevant for protected area planning, ecosystem restoration and assessments on the global vulnerability of species by the International Union for the Conservation of Nature (IUCN). However, the species composition of most tropical regions prior to extensive modern human disturbance is often not known, except for few areas for which historic accounts or natural history museum specimens exist. Therefore, the development of independent means for reconstructing species assemblages would be of enormous help to conservationists and policy makers.
Besides conventional paleoecological tools and historical records, emerging ancient DNA approaches are likely to be very useful for elucidating the hyperdiversity conundrum of the tropics and in contributing to the urgent conservation needs of tropical countries (Andersen et al., 2012; Hofman et al., 2015; Thomsen and Willerslev, 2015; Díez-del-Molino et al., 2018; Epp, 2019). Ancient DNA (aDNA) can be directly extracted from fossil bones, teeth (e.g., Adler et al., 2011) and plant macro-remains (e.g., Estrada et al., 2018), thereby providing genetic information on individual organisms and species. Ancient DNA may also be present in sediments and, if sufficiently preserved can potentially open a window into the past faunal and floral composition of ecosystems (e.g., Willerslev et al., 2003; Slon et al., 2017). Sedimentary ancient DNA (sedaDNA) has been successfully used to detect the presence of extant, extinct and introduced species, including rare and large vertebrates, in various sedimentary environments (e.g., Haile et al., 2007, 2009; Boessenkool et al., 2012; Giguet-Covex et al., 2014; Graham et al., 2016; Slon et al., 2017).
Sedimentary DNA originates from traces of organisms left in the environment which are incorporated into the sediments of lakes, swamps or caves (e.g., Hofreiter et al., 2003; Willerslev et al., 2003; Haile et al., 2007; Andersen et al., 2012; Parducci et al., 2017; Slon et al., 2017). DNA-containing remains include hair, feces, urine, skin, eggs, feathers, tissue, and seeds. Sedimentary DNA may be deposited directly by the locally present biota such as aquatic taxa in lakes, cave-dwelling organisms or plants rooting in swamps. In addition, DNA may also be transported into a sedimentary basin from its catchment via rivers or runoff (Parducci et al., 2017). Depending on the stage of decay, nucleic acids may either be intra- or extracellular. The extracellular DNA is then microbially processed or adsorbed by cations, clay minerals, apatite, silica or other sedimentary matter (e.g., Lorenz and Wackernagel, 1987, 1994; Ogram et al., 1988; Armbrecht et al., 2019).
Thus far, sedaDNA has primarily been studied in cold climate regions, particularly northern Eurasia, North America and New Zealand where preservation conditions are ideal (e.g., Willerslev et al., 2014; Parducci et al., 2017; Slon et al., 2017; Kisand et al., 2018). For example, sediments from Arctic permafrost have yielded DNA up to 400,000 years old (Willerslev et al., 2003). The vast majority of sedaDNA studies applied metabarcoding, which is the amplification and sequencing of widely used mitochondrial or chloroplast markers such as mitochondrial 16S rRNA, cytochrome b, cytochrome oxidase I, and the chloroplast trnL P6 loop. However, this approach restricts the detection of taxa to selected groups and amplifies certain species more efficiently than others due to polymerase chain reaction (PCR) biases, thus providing a biased perspective on the past taxonomic composition of a site (e.g., Ziesemer et al., 2015; Parducci et al., 2018; Adams et al., 2019). Due to PCR biases and PCR template duplications, robust quantitative comparison of taxonomic profiles is difficult (Adams et al., 2019), even with gene copy normalization (Starke and Morais, 2019). Furthermore, metabarcoding approaches discard the majority of extracted DNA, problematic for conservation of non-replaceable aDNA samples. Moreover, the short length of degraded aDNA precludes the use of long barcoding markers (Willerslev et al., 2014), while the detection of rare taxa necessitates numerous runs of PCR. In comparison, shotgun sequencing reveals the entire metagenomic composition of a sediment sample and thus allows for the most comprehensive description of taxonomic diversity and community composition (e.g., Ziesemer et al., 2015; Slon et al., 2017; Pedersen et al., 2016). This method avoids PCR amplification, which reduces the risk of contamination with modern DNA (Armbrecht et al., 2019) but requires more comprehensive genomic databases to reliably identify obtained sequences (e.g., Rawlence et al., 2014; Cribdon et al., 2020). Nevertheless, reliable quantification of organism prevalence is difficult because metagenomic profiles are biased by the differing sizes of individual genomes – species with larger genomes generate more DNA sequences than ones with smaller genomes and thus appear to be more frequent (e.g., Segata et al., 2012). This bias can be corrected, but only if the genome sizes of all identified organisms are known.
SedaDNA studies of tropical regions are very rare and thus far principally absent from hyperdiverse tropical rainforests (Pedersen et al., 2015; Epp, 2019). For example, sub-Saharan Africa is understudied for aDNA as a whole (Campana et al., 2013) and the history of its rich vertebrate fauna has not been investigated from a sedaDNA perspective. We are aware of only six studies from tropical Africa that used sedaDNA either to explore floristic diversity or aquatic organisms such as diatoms and Daphnia (Mergeay et al., 2007; Epp et al., 2010, 2011; Stoof-Leichsenring et al., 2012; Boessenkool et al., 2014; Bremond et al., 2017). A possible reason for the scarcity of studies in the tropics is the assumption that high temperatures are not conducive for long-term DNA preservation (Kistler et al., 2017). Recently it has been shown, however, that DNA can persist in tropical lacustrine and marine sediments under high temperatures for hundreds to thousands of years (Bremond et al., 2017; Gomez Cabrera et al., 2019). However, the preservation of DNA in different sediment types in general, and in tropical systems in particular, is insufficiently known (e.g., Epp, 2019). Dry sediments in temperate regions seem to preserve DNA relatively well (Hofreiter et al., 2003), but vertical transport and leaching of DNA may lead to contamination within stratigraphic profiles (Haile et al., 2007). The role of DNA leaching in saturated sediments needs to be examined since the polarity of DNA molecules should promote its degradation by hydrolysis (Lindahl, 1993; Pääbo et al., 2004). Given these issues, a much better understanding of transport, deposition, preservation and degradation of sedimentary DNA in the tropics is needed (Domaizon et al., 2017; Giguet-Covex et al., 2019).
To address these open questions and to explore the value of sedaDNA as a tool for tropical conservation biology, we investigated the metagenomic composition and patterns of DNA degradation in several sediment cores collected from one of the most-diverse tropical rainforests of equatorial Africa – Bwindi Impenetrable Forest in Uganda (Kingdon, 1973; Butynski, 1984). Bwindi Forest is part of the Albertine Rift – a globally important conservation region which is characterized by high levels of endemism and the richest vertebrate diversity in Africa (Plumptre et al., 2007). It also belongs to the Eastern Afromontane Biodiversity hotspot (Brooks et al., 2004) highlighting its biogeographic uniqueness and vulnerability to anthropogenic impacts. Bwindi is the richest forest in Uganda with regard to the number of recorded mammal and plant species (Plumptre et al., 2007; Olupot and Plumptre, 2010). Importantly, Bwindi harbors one of the two globally surviving populations of mountain gorillas (Gorilla beringei beringei) with a population of about 400 individuals (Roy et al., 2014). This forest reserve is also the only place where mountain gorillas are sympatric with chimpanzees (Pan troglodytes schweinfurthii) (Stanford and Nkurunungi, 2003). Our study leveraged an undisturbed swamp located in the middle of Bwindi Forest that contains sediments dating back to the Pleistocene (Marchant et al., 1997). Bwindi thus provides an excellent opportunity to test whether tropical diverse rainforest biota can be detected with sedaDNA.
Here we present metagenomic assemblages across the tree of life (i.e., Bacteria, Archaea, and Eukaryota) retrieved by shotgun sequencing of sedaDNA covering the past 2200 years. The overall objectives of our study are (1) to investigate conditions of sedaDNA preservation and degradation in relation to chemical and physical sediment and water properties in a tropical environment and (2) to characterize taxonomic diversity and metagenomic composition of Bwindi over time. Specifically, we asked the following questions:
(1) Is endogenous ancient DNA preserved in tropical swamp sediments?
(2) How do temperature, acidity, nutrient content, elemental composition, and sediment lithology and age influence DNA preservation?
(3) How precisely can the taxonomic level of shotgun-sequenced sediments from a diverse tropical site be resolved at present?
To this end, we compare DNA concentration, molecular fragment length and cytosine deamination patterns as signals of DNA degradation with a multivariate dataset consisting of the age, type, chemistry and temperature of the sediments. Using our metagenomic approach, we compare past and present ecological communities preserved in the sediment and present taxonomic assignments using three metagenomic classifiers and four reference databases with a focus on plant and animal detections, assess the accuracy of identifications by comparing plant DNA signals with pollen data and animal DNA assignments with modern occurrence data and finally examine biases introduced by metagenomic reference databases and bioinformatic approaches in the detection of taxa.
Materials and Methods
Study Area
Bwindi Impenetrable National Park is a 331 km2 large forest (0°53′–1°08′ S; 29°35′–29°50′E) in southwest Uganda (Figure 1), which supports an exceptional diversity of at least 135 mammal species (10 primate species), 381 bird species, 34 reptile species, 29 amphibian species and 393 tree species (Plumptre et al., 2007). Fifty-six tetrapod species and 74 plant species of Bwindi are Albertine Rift endemics (Plumptre et al., 2007).
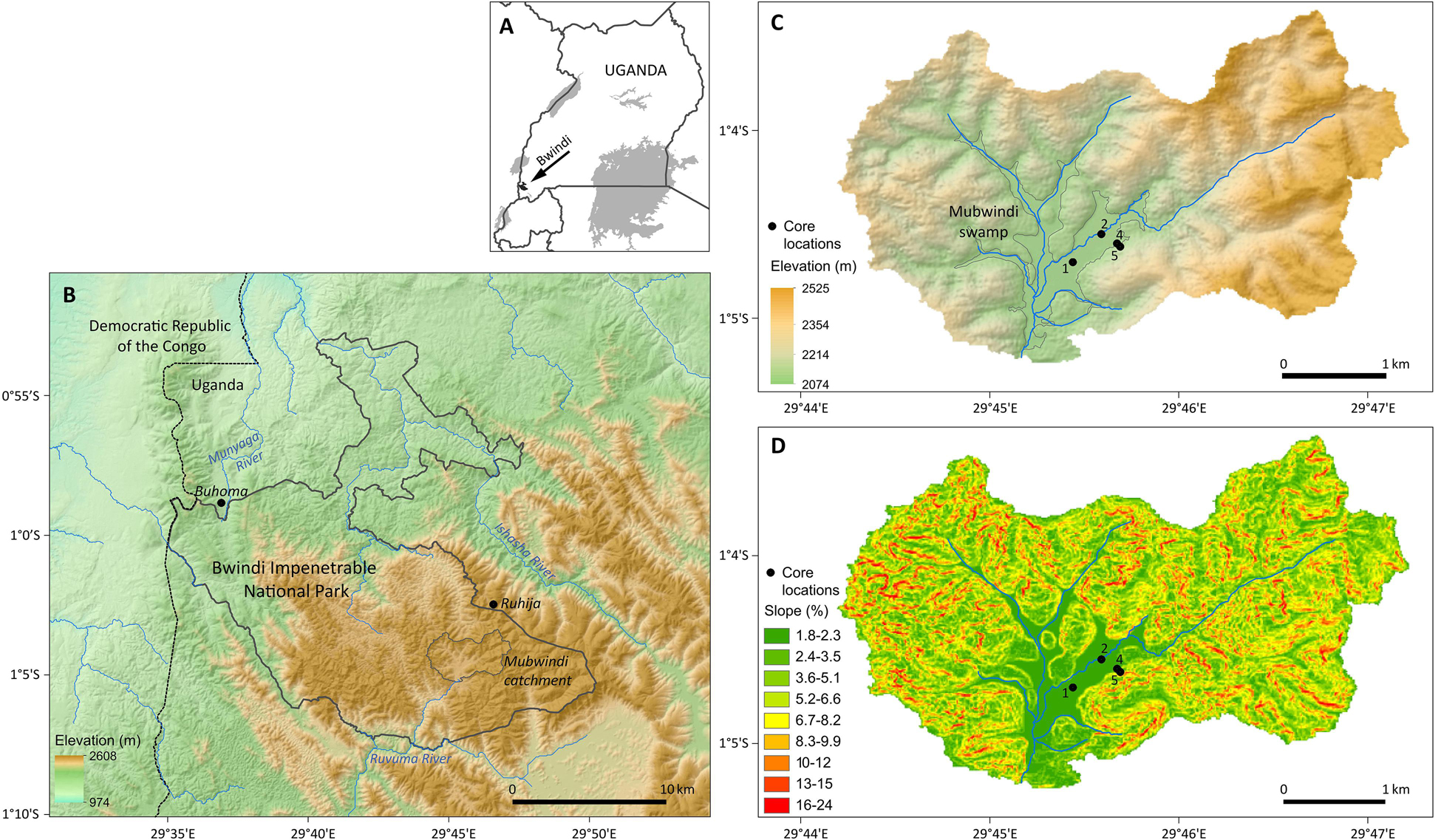
Figure 1. Study area: (A) location of Bwindi Impenetrable National Park in Uganda, (B) digital elevation model of the Bwindi region with the national park boundary shown as thick gray line and the boundary of the catchment of Mubwindi Swamp as thin gray line, (C) elevation map and drainage network of the catchment of Mubwindi Swamp (bordered by gray line) with locations of coring sites 1, 2, 4 and 5, and (D) slope map of the Mubwindi catchment and swamp. Maps 2-4 and catchment boundaries are based on the TanDEM-X digital elevation model (© DLR).
Bwindi is located in the Kigezi Highlands (Figure 1), which are part of the eastern shoulder of the Albertine Rift – the western arm of the East African Rift. The bedrock geology of Bwindi consists of Precambrian metamorphic rocks such as schist, quartzite, shale and granite and the soils are ferrallitic (Butynski, 1984). The elevational gradient of this relatively small reserve ranges from 1190 m in its northern most part to over 2600 m in the southeastern corner based on the digital elevation model of Figure 1. The land surface is strongly dissected, resulting in substantial relief differences and steep slopes.
At Ruhija (2350 m, Figure 1A) mean monthly temperatures are between 13.4 and 19.1°C (September 2001 to August 2002: monthly median 15.1°C; Nkurunungi et al., 2004) and mean annual precipitation is 1378 mm recorded over the period 1987–2006 (Kasangaki et al., 2012). Two rainy seasons occur between March to May and September to November associated with the biannual passage of the tropical rainbelt. Bwindi is covered with mid-altitude and montane rainforest and a small area in the southeast with bamboo (Arundinaria alpina) forest. The dominating tree species are Chrysophyllum gorungosanum, Entandrophragma excelsum, Neoboutonia macrocalyx, Newtonia buchanani, and Parinari excelsa (Butynski, 1984; Olupot and Plumptre, 2010).
In the southcentral part of Bwindi lies Mubwindi Swamp – a 1 km2 flat, slightly inclined peatland fed by several streams from a 12 km2 large, forested catchment (Figures 1, 2A). We cored Mubwindi Swamp due to its long sediment record and because it serves as habitat to various terrestrial and (semi) aquatic species, which enhances the possibility of DNA deposition. The swamp is mostly covered with sedge communities dominated by Cyperus latifolius and Cyperus denudatus, which are often associated with Thelypteris cf. confluens, Alchemilla johnstonii, Crassocephallum paludum, Lobellia mildbraedi, and other species.
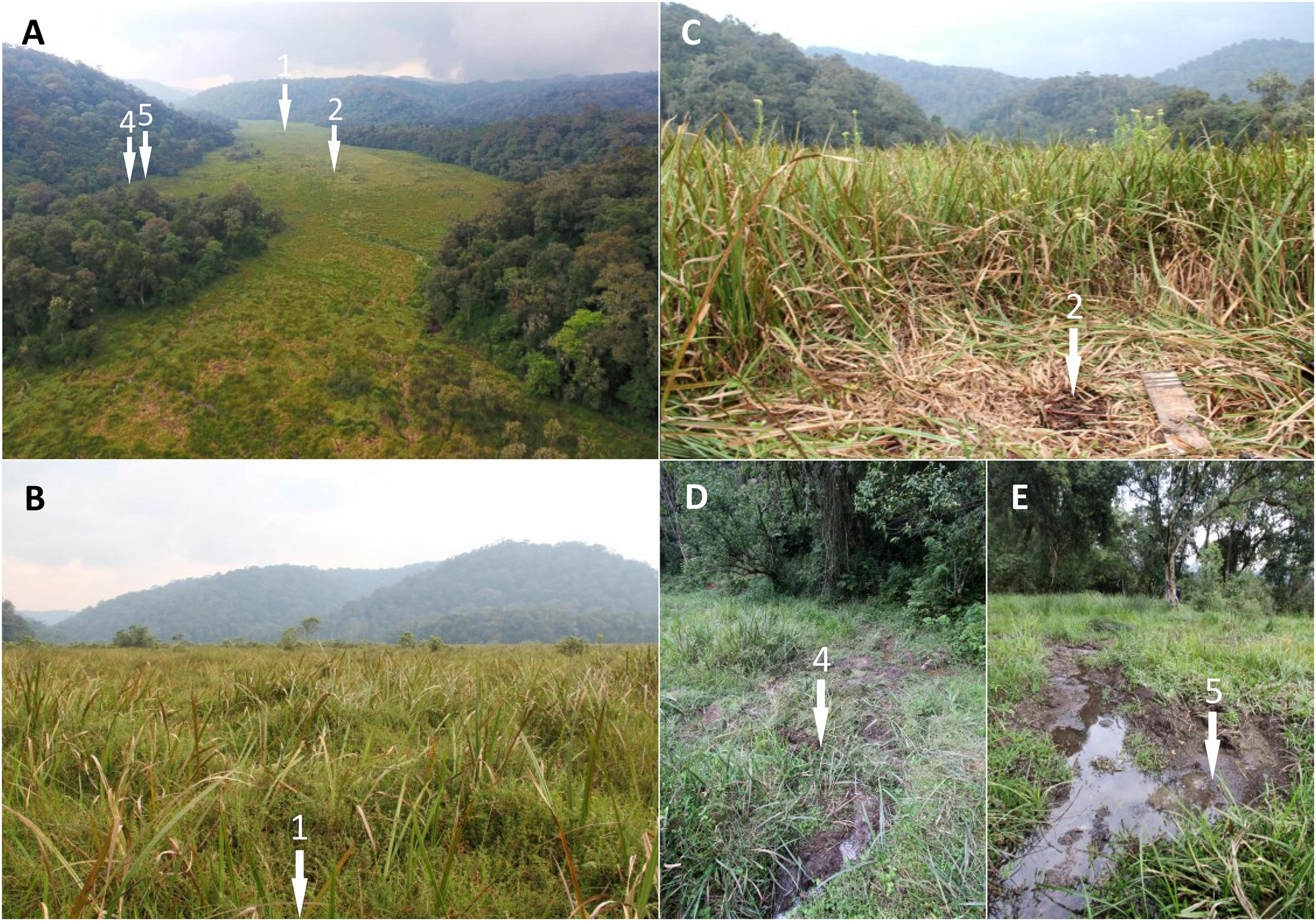
Figure 2. Photographs of Mubwindi Swamp and coring sites. (A) Aerial photograph of Mubwindi from northeast to southwest with white arrows marking the coring sites. Note the elephant trails across the swamp in the foreground. (B) Coring site 1 at the swamp center, (C) coring site 2 (master core site) at the swamp center, (D) coring site 4 (elephant dung site) and (E) coring site 5 (elephant wallow). Note the recent elephant tracks in (D,E).
Several large mammals use Mubwindi Swamp as a foraging or breeding site like Sitatunga (Tragelaphus spekei) and bushbuck (Tragelaphus scriptus) (Mugerwa et al., 2013; pers. obs.). Mountain gorillas visit the swamp’s edge to feed on the thistle Carduus kikuyorua (Ganas et al., 2009; Rothman et al., 2014; pers. obs.). The swamp is also home to breeding populations of several Albertine Rift endemics, including the globally endangered Grauer’s swamp warbler (Bradypterus graueri; Kahindo et al., 2017), Delany’s swamp mouse (Delanymys brooksi; Kasangaki et al., 2003), and several frog species such as Bururi long-fingered frog (Cardioglossa cyaneospila; Blackburn et al., 2016) and Ahl’s reed frog (Hyperolius castaneus; Drewes and Vindum, 1994). Bwindi forest apparently lies within the hybrid zone of forest and savanna elephants (Loxodonta cyclotis, L. africana; Mondol et al., 2015) and currently supports a population of less than 40 elephants (Kasangaki et al., 2012), which regularly visit the forests around Mubwindi and the swamp itself for foraging and drinking, particularly during the dry season (Babaasa, 2000, pers. obs.). In the catchment of Mubwindi Swamp occur other rare vertebrates such as African golden cat (Caracal aurata), yellow-backed duiker (Cephalophus silvicultor) and one of Africa’s rarest birds, the African green broadbill (Pseudocalyptomena graueri) (Olupot and Plumptre, 2010; Mugerwa et al., 2013; pers. obs.).
Core Collection, Logging, and Processing
Between August 4 and 7, 2017 we collected seven sediment cores from Mubwindi Swamp (1°4′ S, 29°45′ E, ca. 2090 m a.s.l.) with a modified Livingstone-type corer equipped with a square rod and steel barrel, which was 5 cm in diameter (Wright et al., 1984). Prior to collecting cores, the piston and core barrel were washed with boiling water to reduce DNA contamination. In the swamp center, we collected two long cores from two sites (site 1 and 2, Figure 1) that both reached a basal resistant gravel layer (cores designated MUB17-1A: 620 cm, MUB17-2C: 656 cm). To this end, we successively recovered one-meter-long core sections from the same bore hole and extruded the cores in the field. Site 1 was located in a mixed sedge-fern community dominated by Cyperus denudatus and Thelypteris cf. confluens (Figure 2B) and site 2 in a tall sedge community covered by dense stands of Cyperus latifolius and within 5 m of a stream (Figure 2C). At these sites we collected additional surface cores within 1 meter from the main cores for assessing DNA degradation and modern metagenomic composition (site 1: core 1E: 87 cm, site 2: cores 2A: 71 cm, 2D: 93 cm). For site 2, we combined core sections 2D-1 and sections 2C-2 through 2C-6 into a composite core (here referred to as “master core”) for studying long-term patterns (Figure 3), whereas for site 1 we only present data from surface samples. Furthermore, we collected two short cores (4A, 5A) at the eastern edge of the swamp where elephants had just been active prior to our visit. Core MUB17-4A (83 cm) was recovered from a site covered with elephant dung (here referred to as “elephant dung site”; Figure 2D) and core MUB17-5A (79 cm) retrieved from a flooded elephant wallow (here referred to as “elephant wallow site”; Figure 2E).
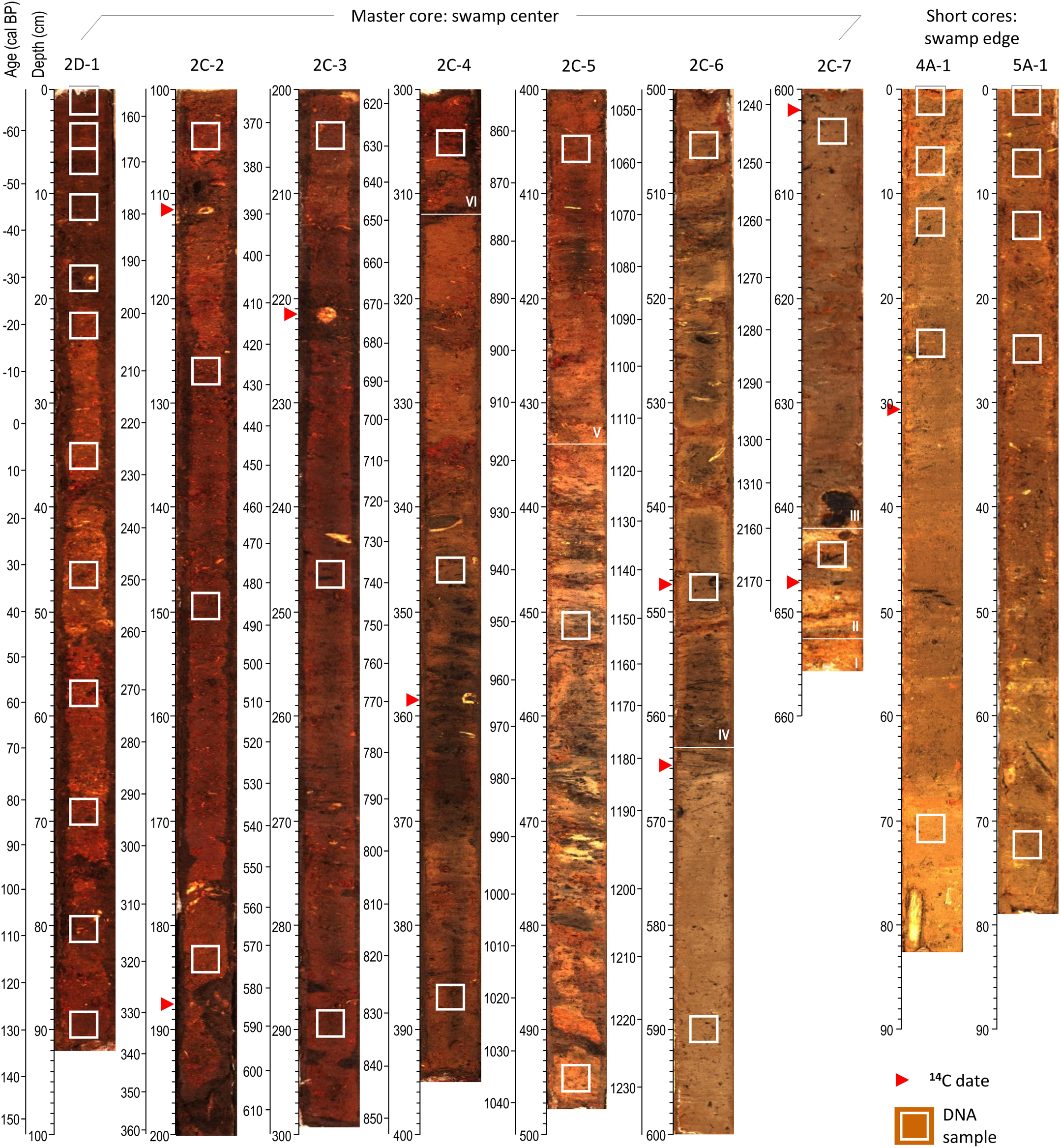
Figure 3. Images of the analyzed core sections with age (calibrated median ages before present, only master core) and depth scales to the left of each image. White squares mark locations of sedDNA samples and red triangles locations of radiocarbon dates. The master core (MUB17-2C/D) consists of seven sections, with the top section shown on the left and the bottom section on the right. Core 4A-1 is the elephant dung site and 5A-1 the elephant wallow site. Roman numerals denote stratigraphic units of the master core, which are separated by white lines.
All core sections were wrapped in plastic wrap and stored in ABS (acrylonitrile butadiene styrene) tubes for transport and permanent storage. Cores were shipped to the LacCore facility (University of Minnesota, MN, United States) for non-invasive analyses, sub-sampling and permanent cold storage. At LacCore, cores were first scanned non-invasively for wet bulk-density using gamma-ray attenuation with a Geotek multi-sensor core logger at 0.5 cm intervals (two runs per core section). Limited high-energy irradiation using Gamma or X-rays is unlikely to significantly impact sedimentary DNA preservation (M. Muschick, pers. com.; Wanek et al., 2013; Wanek and Rühli, 2016). Each core was split longitudinally with a bleached diamond-bladed band saw into a working split section used for destructive sub-sampling and an archive split section used for non-invasive analyses and permanent storage. Each fresh archive section was photographed with a GeoScan IV digital linescan camera at 300 dpi.
Under sterile conditions, we collected samples for sedimentary ancient DNA analyses from the freshly split working sections of cores 1A, 1E, 2A, 2C, 2D, 4A, 5A (Figure 3 and Supporting Data). Two-cm-thick samples were taken from the core’s center with bleached and acetone cleaned tools after removing one centimeter of sediment from the core surface to avoid any contamination (Figure 3). All samples were immediately frozen and shipped to the Smithsonian Conservation Biology Institute (Washington, DC) for metagenomic analyses. From the archive core sections we obtained point magnetic susceptibility profiles (0.5 cm resolution) with a Geotek XYZ core logger and X-ray fluorescence (XRF) elemental profiles (0.5 cm sampling resolution; 15 s dwell time) with an ITRAX X-ray core scanner at Large Lakes Observatory (Duluth, MN, United States).
Stratigraphic and Geochemical Core Analyses
The lithostratigraphy of the cores was determined by visual inspection of the core surfaces and with a dissecting scope. In addition, mineralogy was analyzed microscopically from smear slides and with a Hitachi TM 1000 tabletop scanning electron microscope. For measuring water and organic matter content we collected two-cm-thick samples contiguously from the master core and two-cm-thick samples parallel to every DNA sample location from the other analyzed cores. Water content was determined by drying sediment at 100°C for 12 h in an oven and organic matter (OM) content was determined by loss-on-ignition of the dried samples at 550°C for 4 h in a muffle furnace (Dean, 1974). In addition, 1-cm-thick samples were taken for elemental analyses (C, N, S, P) immediately adjacent to every sedDNA sample location. These samples were freeze-dried and ground to a powder. Total elemental contents of carbon (C), nitrogen (N), and sulfur (S) were determined by high-temperature combustion elemental analysis (infrared detection, Elementar Vario EL, IGB Berlin). Total phosphorus content was determined by molybdenum blue spectrometry (Murphy and Riley, 1962) after thermal combustion (550°C) and hot potassium peroxodisulfate hydrolysis (Andersen, 1976; Ebina et al., 1983).
Radiocarbon Dating
From cores 2C and 4A we collected plant macrofossils of non-aquatic plants and wood for AMS radiocarbon dating (Table 1). Site 5 (wallow) was not dated because of obvious bioturbation. Radiocarbon activity for 11 samples was determined by accelerator mass spectrometry at Lawrence Livermore National Laboratory (Table 1). We developed a Bayesian age-depth model for the master core with the BACON software package (Blaauw and Christen, 2011). All 14C dates were also calibrated into calendar years with the IntCal13 calibration curve (Reimer et al., 2013) in CALIB 7.1 (Stuiver and Reimer, 1993). Ages are presented as calendar years before present (i.e., present is 1950; “cal BP”) and on the common era (“CE”) notation.
Field Measurements
To assess the influence of the local environment on DNA preservation, we conducted field surveys on water chemistry and physical and chemical soil properties of Mubwindi Swamp in June and September 2018 and July 2019. At all our four coring locations we measured soil pH and temperature changes with depth. To this end we successively retrieved 50-cm-long sediment core sections with a D-section core sampler and immediately upon core retrieval measured soil temperature in intervals of between 1 and 10 cm with an Extech digital thermometer, followed by pH with a Lutron soil pH meter at the same intervals. Close to our four coring locations, we also collected surface water samples from open pools or dug pits for the analysis of major dissolved elements, anions, and nutrients. Water samples were filtered (0.45 μm) and preserved by acidification (2M HCl) for subsequent analysis in the laboratory at IGB Berlin. Water temperature, pH and EC were measured at each sampling location with a handheld multiparameter probe (WTW Multi 3530). Dissolved aluminum (Al), calcium (Ca), iron (Fe), potassium (K), magnesium (Mg), manganese (Mn), and sodium (Na) were determined by inductively coupled-optical emission spectroscopy (ICP-OES, Thermo Scientific iCAP 6300). Dissolved chloride (Cl–) and sulfate (SO42–) were determined in samples without acidification by ion chromatography (conductivity detection after chemical suppression, Metrohm CompactIC). Dissolved ammonia (NH4+) and nitrate (NO3–) were determined by flow-segmented analysis (FSA, SEAL AutoAnalyzer 3). Dissolved organic carbon (DOC) was determined by thermocatalytic conversion infrared spectroscopy (Shimadzu TOC-L). Soluble reactive phosphorus (SRP) was determined by molybdenum blue spectroscopy (Murphy and Riley, 1962).
Ancient DNA Extraction and Sequencing
We extracted DNA from 44 sediment samples (∼0.25 g per extraction), 30 from our master core and the remainder from the short cores (Figure 3 and Supporting Data), using DNeasy PowerSoil kits (Qiagen Inc., Germantown, MD, United States). To monitor DNA contamination during the coring and DNA extraction procedures, we also extracted DNA from two ∼0.25 g samples of Lux bar soap (Unilever) used to grease the corer’s piston and processed two additional sham extractions that contained only extraction reagents. DNA concentrations were measured using a Qubit® 2.0 fluorometer with the dsDNA HS assay kit (Thermo Fisher Scientific, Waltham, MA, United States).
We built double-indexed, double-stranded DNA libraries using KAPA Library Preparation kits – Illumina (Roche Sequencing and Life Science, Kapa Biosystems, Wilmington, MA, United States) with iNext adapters (Glenn et al., 2019). To render the iNext stub compatible with the KAPA kit’s A-tailing step, the stub sequence was modified by the addition of a thymine residue to the 3′-terminus (revised sequence: 5′-TCGTCGGCAGCGTCAGATGTGTATAAGAGACAGT-3′) and the stub complement sequence was extended by a guanine at the 5′-terminus (revised sequence: 5′-[phos]GACAGAGAATATGTGTAGAGGCTCGGGTGCTCTG-3′). In addition to the previously described DNA samples and controls, we added four control reactions (“Library Controls”) containing only water to monitor reagent contamination during library preparation. Libraries were amplified by 18 cycles of indexing PCR using KAPA HiFi Uracil + polymerase (Roche Sequencing and Life Science, Kapa Biosystems, Wilmington, MA,
United States) according to the manufacturer’s instructions. All purification steps during library preparation and amplification were performed using carboxyl paramagnetic beads (Rohland and Reich, 2012). Amplified libraries were visualized on 2% agarose gels stained with GelRed (Biotium Inc., Fremont, CA, United States) and quantified using a Qubit® 2.0 fluorometer with the dsDNA HS assay kit and quantitative PCR with the KAPA Library Quantification Kit (Roche Sequencing and Life Science, Kapa Biosystems, Wilmington, MA, United States). Libraries were pooled and submitted to Admera Health (South Plainfield, NJ, United States) where residual adapter-multimers were removed using AMPure XP beads (Beckman Coulter Life Sciences, Indianapolis, IN, United States) and final library pool quality was confirmed via visualization on a TapeStation (Agilent Technologies, Santa Clara, CA, United States). The quality-controlled pool was then 2 × 151 bp paired-end sequenced on a single lane of a Nextseq 500 (Illumina, Inc., San Diego, CA, United States).
DNA Sequence Quality Control
Library sequence qualities were inspected using FastQC 0.11.5 (Andrews, 2016). We trimmed residual adapter contaminants and low-quality bases from the sequences using Trimmomatic 0.39 (parameters LEADING:3, TRAILING:3, SLIDINGWINDOW:4:15, MINLEN:30, HEADCROP:1, CROP:149, ILLUMINACLIP:NexteraPE-A-tail.fa:2:30:10 where Nextera-PE-A-tail.fa includes the A-tailing Nextera adapter sequences; Bolger et al., 2014). We merged paired reads using FLASH 1.2.11 (parameter -M 149; Magoč and Salzberg, 2011) and removed PCR duplicates from merged reads using CD-HIT-EST 4.6 (parameter -c 1; Li and Godzik, 2006).
DNA Degradation and Cytosine Deamination
We estimated putative ancient DNA fragment length distributions from the deduplicated, merged reads using a previously developed custom Ruby script (Campana et al., 2014). While this estimate excludes a minority of library inserts longer than 288 bp, typical mean fragment lengths of ancient DNA are 150 bp or shorter (e.g., Green et al., 2009; Prüfer et al., 2010; Dabney et al., 2013a). We confirmed the accuracy of our fragment length estimates by inspection of library insert length distributions, both bioinformatically (Supporting Data) and by visualization on agarose gels.
We performed an ad hoc test to determine whether the Mubwindi Swamp sedimentary DNA demonstrated cytosine deamination patterns consistent with authentic ancient DNA preservation (Briggs et al., 2007). The sediment taxonomic profiles were dominated by Rhizobiales (see below). Many of these taxa, such as Bradyrhizobium japonicum, are plant symbionts providing functional benefits such as nitrogen fixation and nutrient provision (e.g., Kaneko et al., 2011; Erlacher et al., 2015). We observed intact plant tissues (roots, leaves and stems) primarily in the top layers of the sediment column. Therefore, we expect increased proportions of cytosine deamination in lower layers if “ancient” damaged Rhizobiales molecules are being preserved in situ (Briggs et al., 2007; Sawyer et al., 2012). Absence of an increasing cytosine deamination signal is consistent with these molecules originating from modern DNA, either from free-living taxa closely related to root-associated species or via leaching through the sediment column. To estimate the rates of terminal cytosine deamination, we aligned the deduplicated, merged reads against the Bradyrhizobium japonicum USDA6T reference genome (GenBank accession NC_017249.1; Kaneko et al., 2011) using BWA-MEM 0.7.17-r1188 (Li, 2013). The alignment was converted to bam format and sorted using SAMtools 1.9 (Li et al., 2009). Deamination patterns were analyzed using mapDamage2 2.0.8-dirty (Jónsson et al., 2013).
Metagenomic Characterization
We aligned the deduplicated, merged reads against the National Center for Biotechnology Information (NCBI) non-redundant nucleotide (hereafter “nt,” version dated 9 September 2019) and the Refseq Genomic (hereafter “Refseq,” version dated 3 February 2020) databases using megaBLAST 2.6.0+ (Camacho et al., 2009) under default settings. MegaBLAST results were analyzed using the naïve lowest common ancestor (LCA) algorithm in MEGAN Community Edition 6.17.0 (nt analysis) or 6.18.5 (Refseq analysis) under default settings (Huson et al., 2016) except that “MinSupportPercent” was set to 0.005. We compared the two databases’ read assignments using paired t tests in GraphPad QuickCalcs (GraphPad Software Inc.).1 Relative metagenome compositions were compared using normalized counts to control for variation in sequencing depth, maintaining at least one read per identified taxon, and discarding unidentified sequences. Community compositions at the ordinal rank were compared by Principal Coordinates Analysis (PCoA) and neighbor-net analysis based on Bray-Curtis distance in MEGAN. We compared animal (Metazoa) and land plant (Embryophyta) taxa (ordinal level or higher) presence/absence in the sedimentary DNA record against regional species (Butynski, 1984; Kasangaki et al., 2003, 2008; Stanford and Nkurunungi, 2003; Olupot and Plumptre, 2010; Mugerwa et al., 2013; Decru et al., 2019) and pollen records (Marchant et al., 1997; Marchant and Taylor, 1998). Pollen data were obtained from the African pollen database hosted at ftp://ftp.ncdc.noaa.gov/pub/data/paleo/pollen/tiliafiles/apd/ (accessed 3 November 2019). We excluded samples with fewer than 50,000 unique reads from the animal and plant record comparisons as these produced too few eukaryotic sequences for accurate identification.
To better characterize the sediment microbial communities, we also analyzed the deduplicated, merged reads using MetaPhlan2 2.9.21 (Truong et al., 2015) and QIIME 2 2019.7 (Bolyen et al., 2019) following Ferrari et al. (2018). We performed MetaPhlAn2 analyses under default settings and generated heat maps clustering sediment samples and taxa using Euclidean distances. In the QIIME 2 analyses, we closed-reference clustered the sequences against a previously trained (using QIIME 2 2018.4) SILVA 16S database (build 132; Pruesse et al., 2007) at 99% identity using VSEARCH (Rognes et al., 2016). Clustered sequences were aligned with MAFFT (Katoh and Standley, 2013), and a phylogenetic tree was built using FastTree (Price et al., 2010). Both phylogenetic and non-phylogenetic diversity metrics were calculated with rarefaction to 50 and 500 sequences. Communities were compared using PCoA using Bray-Curtis and Jaccard distances in EMPeror (Vázquez-Baeza et al., 2013, 2017).
Statistical Analyses
We examined the relation between DNA yield and environmental factors with exponential regression analysis and the linear dependence between multiple DNA quantity and degradation parameters by computing Pearson correlation coefficients in R 3.5.1 (R Core Team, 2018) using the PerformanceAnalytics 1.5.3 package (Peterson et al., 2019). Since we did not have an a priori model to explain DNA degradation in the Mubwindi Swamp sediments, we also assessed non-parametric relationships between environmental parameters and DNA yield, terminal deamination, and sequence length using Spearman’s ρ in R.
Results
Core Stratigraphy, Chemistry, and Age
The master core (MUB17-2C/D) contains a stratigraphic sequence which grades from clastic to organic sediment from bottom to top and is divided into six stratigraphic units based on patterns of sediment composition, texture and geochemistry (Figure 4). The core bottom from 656 to 653 cm is an unsorted gravel layer (unit I), high in density (1.9 g cm–3) and magnetic susceptibility, but low in water (<20%) and organic matter content of the dry mass fraction (<10%). High XRF counts were obtained for Ca, S, Al, and Fe (Figure 5). Unit II is a coarse sand layer from 653 to 642 cm containing wood and charcoal, but overall less than 10% organic matter. Its C (2.6%) and N (0.1%) content were the lowest of the entire core record, while XRF peaks occurred for Si, Al, Ti and Zn. The sediment type changes abruptly at 642 cm to a homogenous silty clay that continues until 563 cm (unit III). This sediment had, on average, a water content of ca. 50%, a dry mass organic matter content of ca. 20% and a C content of <10%, whereas average density is 1.3 g cm–3. The major elements recorded by XRF are K, Ti, Fe and Al. Unit IV from 563 to 434 cm is a clay deposit with numerous horizontal layers of charcoal, buried leaf fragments and some smaller wood pieces. Its sediment had a density of ∼1 g cm–3, an average sediment water content of 78%, a dry mass organic content of about 37%, a C content < 20% and a N content < 1%. The XRF elemental composition was similar to that of the previous unit, but showed higher counts for S, Co, and Ni. The sediment also contains pyrite and other Fe minerals. Unit V, which extends from 434 to 312 cm, is an organic-rich clay with nearly 60% average dry mass organic matter content and mean sediment water content of 86%. This sediment composition was generally reflected in slightly negative values for magnetic susceptibility, except for a high magnetic susceptibility interval between 372 and 340 cm corresponding to major peaks in XRF counts for Fe and Co and the occurrence of Fe oxides. In addition, relatively high XRF counts of S and a maximum S content of nearly 4% characterized this unit, consistent with the frequent occurrence of pyrite. The final unit VI from 312 to 0 cm is water-saturated, sedge peat (water content > 90%) with an average organic matter content of the dry mass of 84%, a wet bulk density of 0.9 g cm–3 and consistently negative magnetic susceptibility values. This stratigraphic unit has the highest C (average 42%) and N (average 2.1%) content of all units. The P content rises toward the top of the unit and is between 1.1 and 1.7 mg g–1 dry mass in the upper 25 cm. The peat consists mostly of sedge rootlets and contains few small wood pieces (Figure 3). Nearly all XRF elements show significantly lower counts in the XRF profiles (except Ni) and subdued variability in this unit. The stratigraphic units III–V (clay sediments) are interpreted to represent a shallow lake environment, which filled with sediment to form a peatland (unit VI). In contrast to the master core, the short cores from the swamp’s edge (4A, 5A, Figure 3) consist entirely of silty clay and the dry mass contained only between 15 and 50% organic matter. The elephant dung site (4A) had a water content of ca. 50–60% and about 20% dry mass organic matter. The elephant wallow core (5A) exhibited signs of bioturbation and consists of about 70–80% water and the dry mass had about 50% organic matter in the top 50 cm and about 30% below that depth. The most abundant element counts of the XRF analysis for these cores were Fe, Ti, and K.
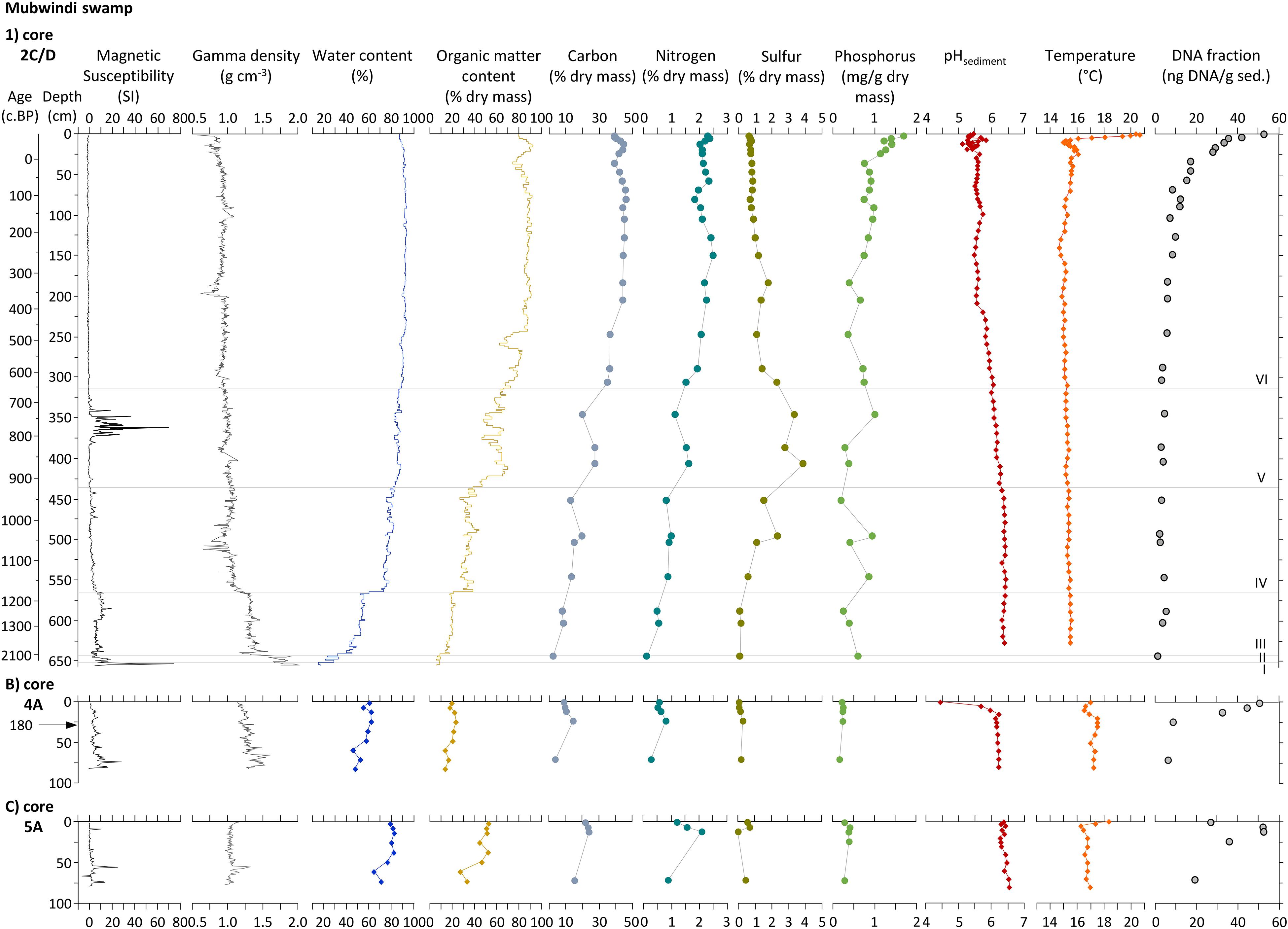
Figure 4. Physico-chemical sediment stratigraphy of cores MUB17-2C/D (master), -4E (dung), and -5A (wallow) together with the DNA fraction of the sediment and a calibrated age scale (years cal. BP) for core 2C/D. pH and temperature were measured in the field close to the original coring locations. Roman numerals denote stratigraphic units of the master core, which are separated by horizontal lines.
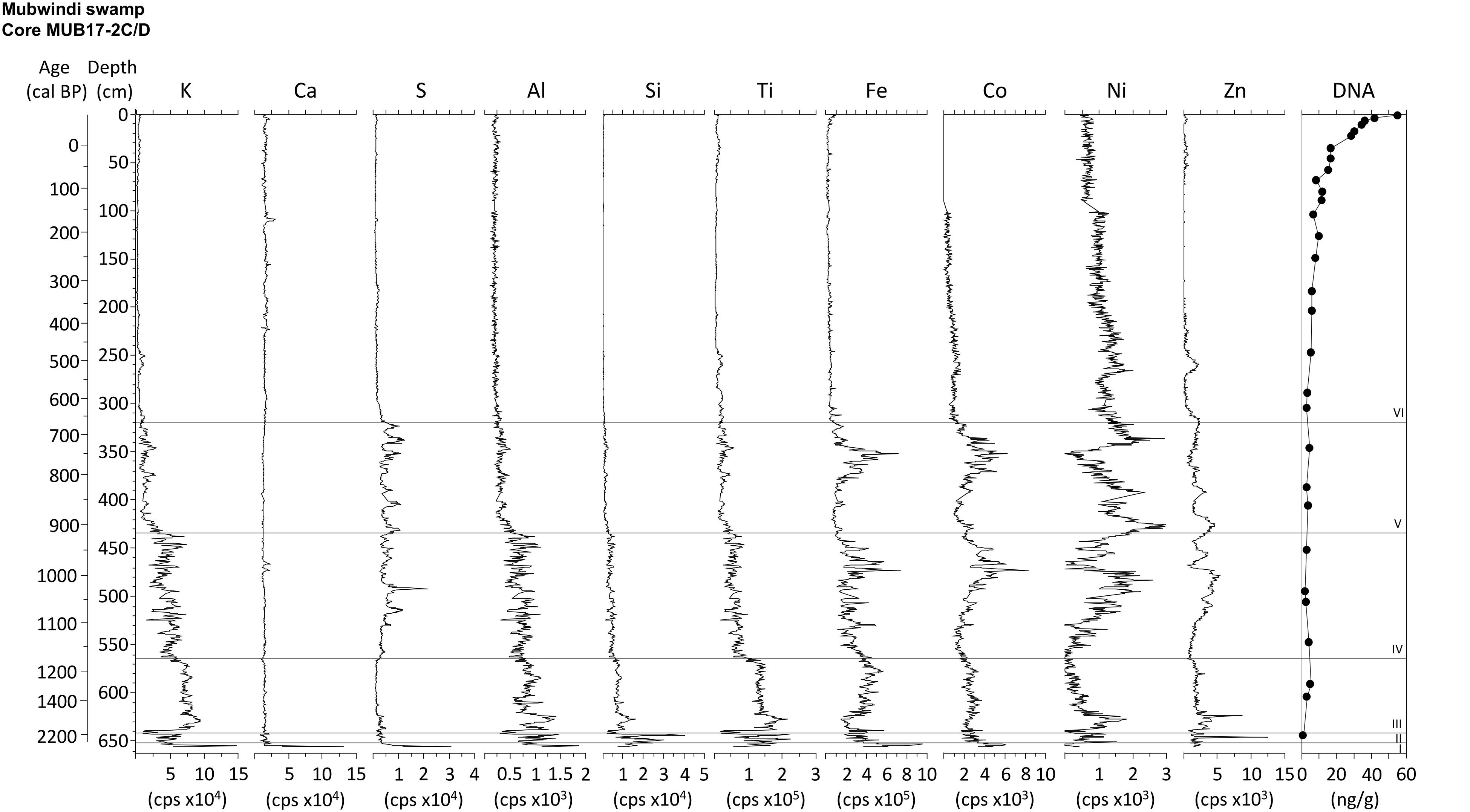
Figure 5. XRF elemental composition of core MUB17-2C/D (master) in comparison to DNA yield. XRF units are counts per second (cps). Roman numerals denote stratigraphic units, which are separated by horizontal lines.
Age of Sediments
The radiocarbon chronology of site 2 (master core) showed that this central part of Mubwindi Swamp was an accumulating basin since ∼2200 cal BP (Table 1). However, the difference of nearly 1000 years between the two deepest radiocarbon ages of the master core, less than 50 cm apart, and the abrupt change in sediment from coarse sand to clay at 642 cm (transition from unit II to III) suggests a possible erosional surface and an associated depositional hiatus. Marchant et al. (1997) also found episodic sedimentation and several hiatuses in their cores. We therefore implemented a hiatus at 642 cm in the BACON age model calculation (Supporting Data). The resulting age-depth model shows relatively constant and very fast deposition since ∼1320 cal BP (642–0 cm). The associated average long-term sedimentation rate is 4.9 mm/yr, which means that one centimeter of sediment was formed in only about two years. The master core therefore provides a temporal high-resolution record: excluding the oldest DNA sample, the average time between adjacent DNA samples is ∼50 years, decreasing to ∼20 years in the upper one meter (Figure 3). The elephant dung site at the edge of the swamp has a median age of ∼180 cal BP (∼1770 CE) at a depth of 30 cm, indicating a slower sedimentation rate of ca. 1 mm/yr at the swamp’s edge (Table 1).
Physico-Chemical Soil and Water Properties
Mubwindi Swamp is an acidic swamp in a relatively cool tropical climate. Daytime soil temperatures in the sediment profile of site 2 (master core) ranged from maximum 20.6°C (0 cm) to 14.6°C (150 cm) (Figure 4). The average soil temperature of the entire 630 cm profile was 15.5°C – very similar to mean monthly air temperatures. Within the upper 10 cm of the sediment column the soil temperature declined rapidly by over 5°C, then continued to drop slightly until 140 cm, but below this depth increased slightly to 15.4°C (Figure 4). At the elephant dung site (core 4A) soil temperatures showed subdued changes, varying between 17.5 and 16.5°C and lacking a maximum at the surface, which instead was recorded at 20–30 cm. In contrast, the elephant wallow site (core 5A) had a temperature maximum at the surface (18.4°C) whereas below 10 cm temperature remained relatively constant at ca. 17°C.
Soil acidity along a 630 cm deep sediment column of the master core site ranged from pH 5.1 to 6.4 (Figure 4). The peat in the upper 20 cm is acidic (average pH 5.4) and showed the greatest variation in pH values. Between 25 and 210 cm, constantly moderately acidic conditions prevailed (pH of ∼5.6) and below that depth pH continued to rise; the sediment was only slightly acidic below 330 cm (≥ 6.1). The surface of the elephant dung site (core 4A) had a pH of 4.4 and became only weakly acidic below 10 cm depth (≥6.1). The elephant wallow site (core 5A) exhibited very similar acidity of between pH 6.3 and 6.7 (slightly acidic to neutral) (Figure 4).
The low concentration of Ca and the moderately acidic pH values of the surface water classify Mubwindi Swamp as a transitional poor to intermediate fen (i.e., groundwater-fed peatland; Table 2). The water was generally depleted in nutrients, however the master core site (2) was slightly enriched in dissolved reactive phosphorus (SRP), ammonium (NH4+), and potassium (K). Both swamp center sites (1 and 2) were very rich in dissolved organic carbon (DOC). At the swamp edge (sites 4, 5), the surface water had elevated sulfate concentrations.
DNA Content, Preservation, and Authenticity
All sediment samples contained Qubit-quantifiable DNA (range: 0.9-86.1 ng DNA/g wet sediment; Mean ± SD: 22.2 ± 21.1 ng/g). Qubit-quantifiable DNA was not detected in negative controls verifying that this DNA derives from the sediments and not contaminants. Between 193 and 24,239,573 read pairs (Mean ± SD: 2,840,581 ± 5,273,296 read pairs) were sequenced for each sediment library, yielding between 126 and 15,458,842 unique merged reads per library (Mean ± SD: 1,859,731 ± 3,425,701 unique reads). Between 1 and 46,643 read pairs (Mean ± SD: 14,440 ± 15,484 read pairs) were sequenced for each negative control, yielding between 0 and 76 unique merged reads per library (Mean ± SD: 19 ± 25.8 unique reads). These low numbers of unique reads in the blanks indicated that our results were not strongly biased by reagent contamination.
We observed a clear trend of decreasing quantities of DNA with greater depths (older age) of the cores (Figures 4, 6, 7), consistent with the preservation of ancient DNA in situ. The surface samples (n = 7) of all our cores contained between 28.6 and 86.1 ng DNA/g sediment (Mean ± SD: 53 ± 17 ng/g, Figure 6). In the master core the amount of sedimentary DNA exponentially declined with depth (r2 = 0.755, p < 0.001) from a maximum amount of 54.7 ng/g at the surface (0–2 cm) to 0.9 ng/g at 645 cm in the basal sand (Figure 7). The largest decline in DNA mass occurred in the upper 100 cm with reductions of more than 10 ng/g between adjacent samples from 2 to 3 cm and 20 to 30 cm. Below 100 cm, the amount of DNA was consistently less than 10 ng/g sediment and, below 250 cm (sediment older than 500 years), it was generally less than 5 ng/g (≤10% of surface sample). Below this depth the mass of DNA remained relatively constant at an average fraction of 3 ng/g despite substantial changes in sediment type, water- and organic matter content and geochemistry in this part of the core (Figure 4).
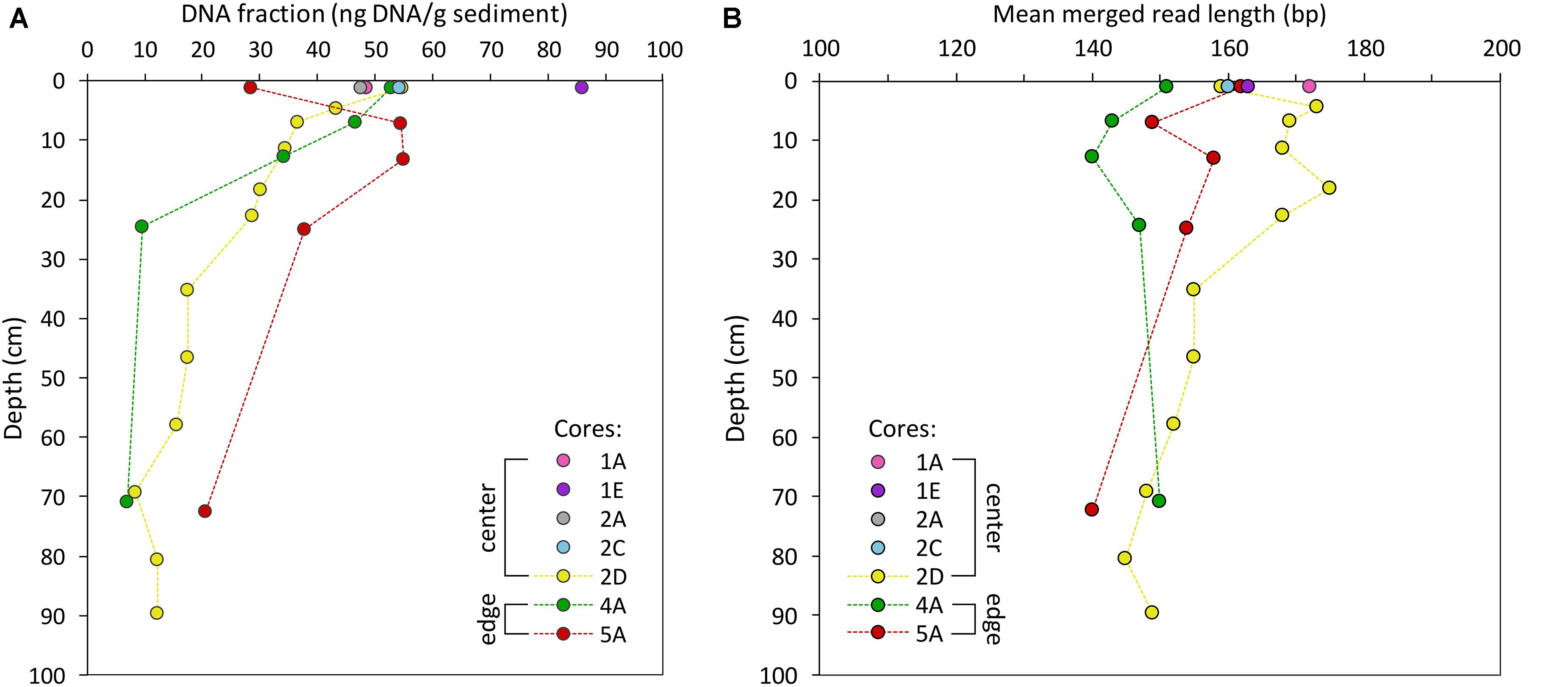
Figure 6. DNA yield of surface samples (cores: MUB17-1A, -1E, -2A, -2C) and samples of the first meter of cores MUB17-2D, -4A, and -5A. (A) DNA fraction per gram sediment, (B) DNA molecule fragmentation presented as mean merged DNA molecule length (bp).
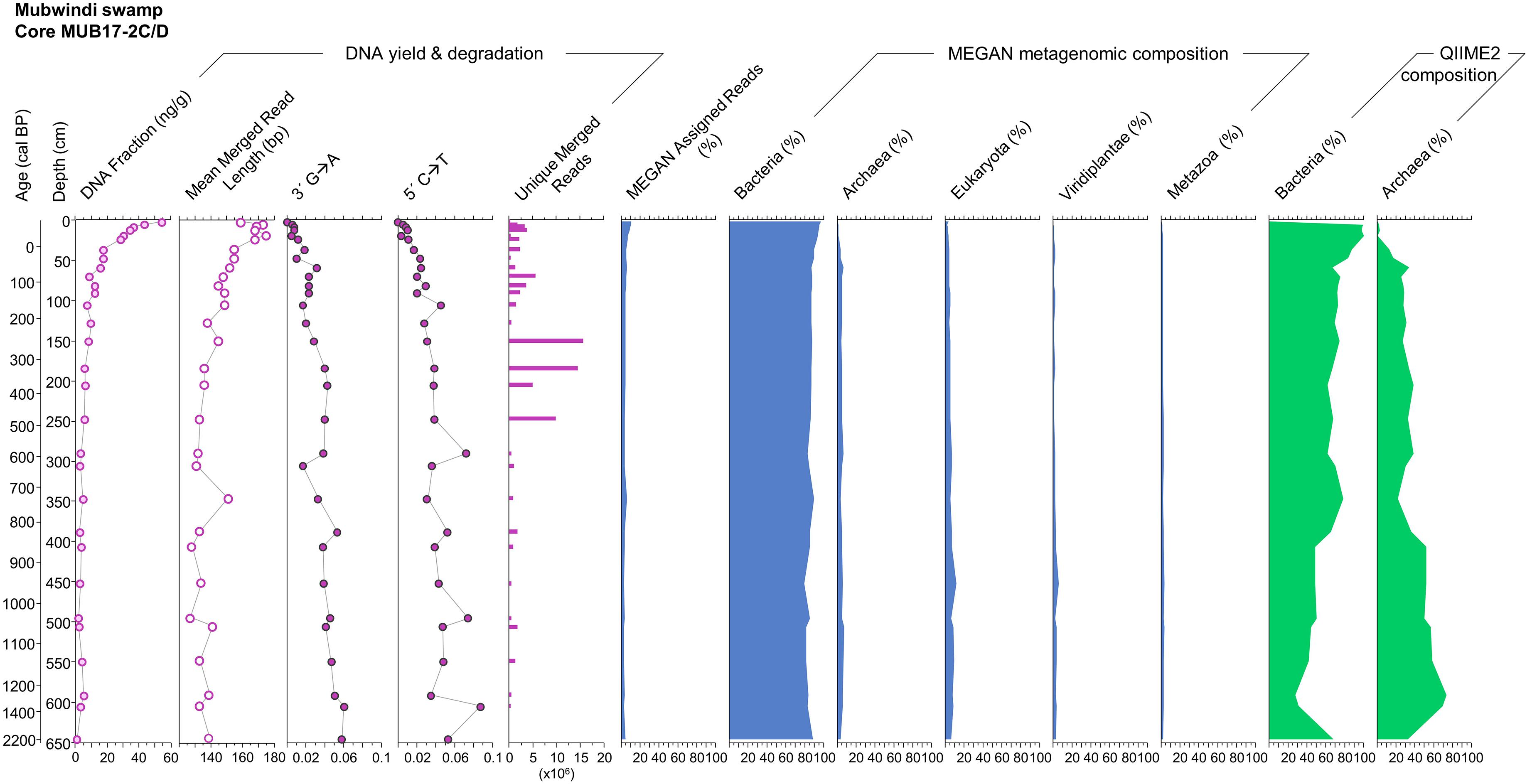
Figure 7. DNA quantity, degradation patterns and metagenomic compositions derived from the MEGAN and QIIME2 pipelines for core MUB17-2 (master core) on a depth and calibrated age scale. DNA degradation is indicated by DNA fragmentation (mean merged read length) and 5′ C→T and 3′ G→A substitutions. The percentage of MEGAN-assigned reads is calculated as the number of MEGAN-assigned reads divided by the number of unique merged reads. The low percentage emphasizes the large number of unidentifiable reads due to missing coverage in genomic databases. Similarly, the percentage of each taxonomic unit presented for the MEGAN metagenomic composition corresponds to the number of taxon-assigned reads divided by the total number of MEGAN-assigned reads. Viridiplantae (green plants) and Metazoa (animals) are clades within the Eukaryota domain. Note the differences in the proportion of Bacteria and Archaea between MEGAN and QIIME2.
The trend of rapidly declining DNA fraction in the upper meter was persistent across Mubwindi Swamp despite the obvious differences in sediment type and composition between swamp center and edge, i.e., peat vs. silty clay (Figure 6). In core 4A (elephant dung site) DNA declined exponentially (r2 = 0.683, p = 0.5322) from 52.9 at 0–2 cm to 7.0 ng/g at 70 cm depth (Figures 4, 6). In contrast, the surface sample of core 5A (wallow site) contained only half as much DNA as the samples from 5 to 7 and 10 to 12 cm which have 55 ng DNA/g sediment. Only below this depth did the DNA concentration decline, but the amount was still larger than in samples of similar depths in the other cores (Figure 6). Additional core samples are required to determine whether this variation is a result of local environmental conditions or simply variability in DNA taphonomy, which has been shown to differ even within a single bone (Green et al., 2010).
Fragment lengths and cytosine deamination patterns also supported the persistence of authentic ancient DNA in the sediment. Mean fragment lengths ranged from 127 to 175 bp (Mean ± SD: 146 ± 13 bp) and molecular fragment lengths were highest in the upper 30 cm of the cores (Figure 6). In the master core, fragment lengths declined with depth (exponential decline r2 = 0.543, p < 0.001), but not as strongly as the DNA fraction. Conversely, both 5′ C→T and 3′ G→A increased exponentially with sediment depth and age in this core (5′ C→T: r2 = 0.519, p < 0.001; 3′ G→A: r2 = 0.601, p < 0.001), strongly suggesting that a portion of the sediment sequences derived from preserved aDNA. The quantity of DNA and fragment length both decreased down core and were significantly correlated (r2 = 0.701, p < 0.001), whereas both parameters were significantly negatively correlated with both 5′ C→T and 3′ G→A as shown in Figure 8.
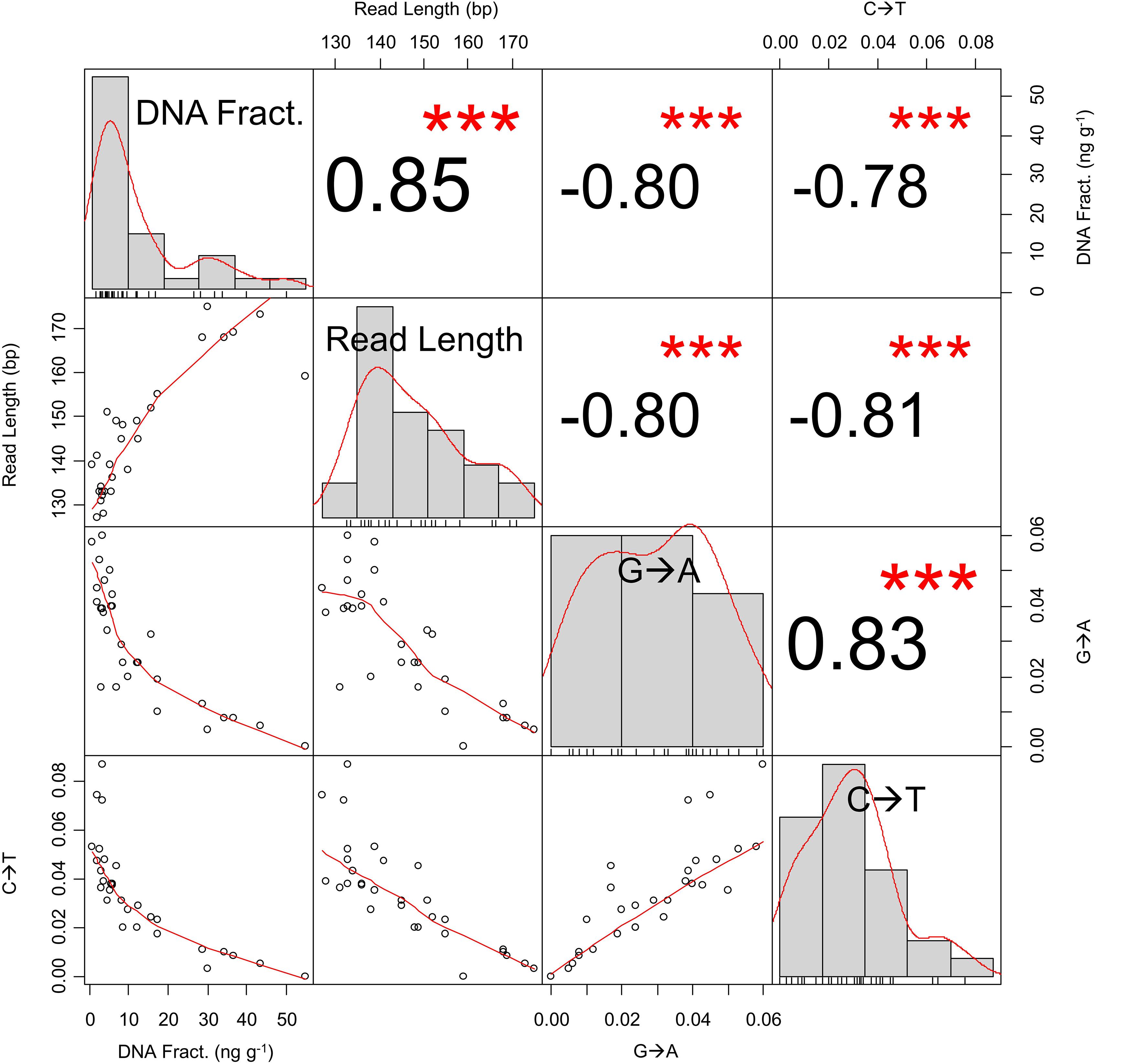
Figure 8. Correlation matrix of DNA fraction, mean read length, and terminal cytosine deamination from core MUB17-2C/D. The histograms depict the distribution of sample values. All correlations (r) are highly significant with estimated p-values of 0 (denoted by “***”).
MEGAN Metagenomic Characterization
The vast majority (nt database: 87.1–97.3%; Refseq database: 80.8–95.5%) of sediment sequences were not identifiable via megaBLAST and MEGAN analysis (nt: Mean ± SD: 94.1 ± 2.6%; Refseq: Mean ± SD: 90.9 ± 3.7%; Figure 7; Supporting Data). MEGAN-identified sequences were dominated by Bacteria (nt: range 79.0–96.9%, Mean ± SD: 88.2 ± 4.4%; Refseq: range 79.5–99.0%, Mean ± SD: 90.7 ± 4.4%), particularly Rhizobiales (nt: range 7.1–31.3%, Mean ± SD: 16.5 ± 4.8%: Refseq range 9.0–33.3%, Mean ± SD: 15.5 ± 4.8%) and Burkholderiales (nt: range 0.0–15.0%, Mean ± SD: 7.2 ± 3.0%; Refseq: range 1.3–14.1%, Mean ± SD: 6.2 ± 2.8%). The microbial profiling conducted in this project was undertaken to further our understanding of DNA preservation in this environment. So, while a full description of microbial profiles is beyond the scope of this paper, detailed microbial profiles and MEGAN results are available in the Supporting Data. The bacterial profiles were complex, representing 125 (nt database) to 156 (Refseq database) taxonomically described orders across all samples (Supporting Data: Taxonomic Profiles). Archaea, representing 15 (Refseq database) to 16 orders (nt database), comprised 0.0–7.5% of the nt assigned reads (0.0–6.9% of Refseq assigned reads) and increased with frequency with depth (nt: Mean ± SD: 4.2 ± 2.1%, r2 = 0.69; Refseq: Mean ± SD: 3.4 ± 1.8%, r2 = 0.28). The most frequent Archaea were Methanobacteriales (nt: range 0.0–4.3%, Mean ± SD: 1.1 ± 1.0%; Refseq: range 0.0–4.8%, Mean ± SD: 0.5 ± 1.0%), Methanomicrobiales (nt: range 0.0–7.4%, Mean ± SD: 1.0 ± 1.2%; Refseq: range 0.0–6.3%, Mean ± SD: 0.9 ± 1.0%), and Methanosarcinales (nt: range 0.0–5.7%, Mean ± SD: 0.9 ± 1.1%; Refseq: range 0.0–3.4%, Mean ± SD: 0.7 ± 0.8%). Eukaryotes were under-represented, comprising only 0.0–13.8% of the identified reads in total (nt: range 0.0–11.5%, Mean ± SD: 4.5 ± 2.3%; Refseq: range 0.0–13.8%, Mean ± SD: 4.2 ± 2.8%) with 0.0–5.8% (nt: range 0.0–3.1%, Mean ± SD: 1.6 ± 0.8%; Refseq: range 0.0–5.8%, Mean ± SD: 2.5 ± 1.6%) deriving from Metazoa and 0.0–6.9% (nt: range 0.0–5.8%, Mean ± SD: 1.4 ± 1.1%; Refseq: range: 0.0–6.9%, Mean ± SD: 1.2 ± 1.3%) from Viridiplantae (Figure 7). Other than the relative decrease in Bacteria and corresponding increase in Archaea with depth, we observed little evidence of temporal structure at an ordinal scale (Supporting Data: Taxonomic Profiles). The relative increase in methanogenic Archaea between strata may not necessarily indicate changes in the past community structure, but rather current structure due to differing microbiota in deeper Mubwindi Swamp sediments (Vuillemin et al., 2017). PCoA and neighbor-net analysis separated some of the surface samples from the short cores (samples 57, 59, 70, and 100) from the primary core samples. To a lesser extent, the short core samples 54, 56, 58, 60, 62, and 63 were also distinct. While these results were consistent with local community structure variation due to microenvironmental differences, the distinct samples were also the shallowest sequenced (120–37,261 unique sequences per sample), indicating that this pattern was probably a sampling artifact (Supporting Data).
The Refseq-aligned dataset assigned taxa to more sequences than the nt-aligned dataset (nt: Mean ± SD: 90,911 ± 150,070 sequences; Refseq: Mean ± SD: 142,931 ± 238,131 sequences; two-tailed p = 0.0003). The Refseq-aligned dataset assigned significantly more sequences to Eukaryota (nt: Mean ± SD: 4,042 ± 6,928 sequences; Refseq: Mean ± SD: 5,919 ± 10,897 sequences; two-tailed p = 0.0036), Bacteria (nt: Mean ± SD: 80,718 ± 132,524 sequences; Refseq: Mean ± SD: 129,720 ± 215,085 sequences; two-tailed p = 0.0003), and Archaea (nt: Mean ± SD: 3,598 ± 6,906 sequences; Refseq: Mean ± SD: 4,578 ± 8,665 sequences; two-tailed p = 0.0013). While the Refseq-aligned dataset assigned more reads to both Metazoa (nt: Mean ± SD: 1,569 ± 2,843 sequences; Refseq: Mean ± SD: 3,810 ± 7,390 sequences; two-tailed p = 0.0022) and Viridiplantae (nt: Mean ± SD: 1,038 ± 1,669 sequences; Refseq: Mean ± SD: 1,187 ± 1,911 sequences; two-tailed p = 0.0041) than the nt-aligned dataset, the effect was larger for Metazoa (mean 2.43 × more assigned sequences) than Viridiplantae (mean 1.14 ×). In fact, the increase in Metazoa was greater than the increase in total Eukaryota (mean 1.46 ×), likely reflecting the disproportionate number of animal genomes in the Refseq database (e.g., Brandies et al., 2019).
The taxonomic compositions of our MEGAN results were not significantly impacted by reagent contamination. The low contamination level (151 total unique reads in the negative controls) were consistent with rare cross-contaminations between sediment samples and negative controls and sporadic reagent contamination. For instance, the Schistosoma, Mustela and Percomorphaceae reads (see below) were probably the result of rare cross-contaminations from the sediment samples (e.g., via index switching: Kircher et al., 2012) as none are found frequently in reagents or were processed previously in our ancient DNA laboratory. Since our analyses were limited to high taxonomic ranks unlikely to be significantly biased by these rare contaminations, we list identified contaminants, rather than bias our dataset by excluding these taxa. Using the nt database, the majority of MEGAN-identified sequences (n = 32 of 37 assigned sequences; 86%) in the negative controls derived from common laboratory contaminants including Hominoids (n = 14), of which 12 were identified as human (Homo sapiens), Canids (n = 1), and Bacteria (n = 17). Identified bacterial species and strains in the blanks included Acinetobacter johnsonii XBB1 (n = 1), Alcaligenes faecalis (n = 1), Aquaspirillium sp. LM1 (n = 1), Brevundimonas sp. (n = 1), Citrobacter freundii complex (n = 1), Dietza sp. oral taxon 368 (n = 1), Fusobacterium sp. (n = 1), Pseudomonas putida (n = 1), Salincola tamaricis (n = 1), Sphingomonas hengshuiensis (n = 1), Staphylococcus saprophyticus subsp. saprophyticus (n = 1), and Stenotrophomonas sp. (n = 1). One contaminant sequence was identified as Schistosoma japonicum, and another three were identified as Percomorphaceae [including Dicentrarchus labrax (n = 1), Mastacembelus armatus (n = 1), and Scophthalmus maximus (n = 1)]. Using the Refseq database, MEGAN assigned taxa to 62 contaminant sequences, of which 33 derived from Bacteria. These included Acinetobacter sp. (n = 1), Alcaligenes faecalis subsp. faecalis NBRC 13111 (n = 1), Bradyrhizobium sp. LSPM299 (n = 1), Brevundimonas bullata (n = 1), Dietzia sp. (n = 1), Egibacter rhizosphaerae (n = 1), Fusobacterium sp. (n = 1), Henriciella litoralis (n = 1), Methylobacterium pseudosasicola (n = 1), Microbacterium sp. CGR2 (n = 1), Pedosphaera pravula Ellin514 (n = 1), Pseudomonas sp. (n = 2), Sphingobium phenoxybenzoativprans (n = 1), Sphingomonas sp. (n = 1), Staphylococcus sp. (n = 2), Streptomyces griseus subsp. griseus (n = 1), Terracidiphilus gabretensis (n = 1), and Williamsia sp. (n = 1). Eukaryotic contaminants identified using the Refseq database included Percomorphaceae (n = 7, including 1 assigned to Lates calcarifer), Primates (n = 14, including 8 assigned to Homininae), Canidae (n = 4, including 2 assigned to Canis lupus), and Mustela putorius furo (n = 1).
Plant and Animal Taxonomic Assignments
For land plants (Embryophyta) our MEGAN analysis against the nt database resulted in 18 taxonomic assignments, with 11 at ordinal rank. All 18 taxa were recorded in the master core, in which the richest samples occurred below 340 cm depth and single samples contained between 1 and 7 orders (Figure 9). The most commonly detected orders were in descending frequency Poales (100% presence), Fabales, Solanales, Rosales, and Brassicales. In comparison, the Refseq database yielded 21 plant taxonomic assignments with 14 orders. All these taxa were recorded in the master core, in which ordinal richness ranged from 0 to 10 orders per sample and was also highest below 340 cm depth (Figure 9). Fabales, Solanales, Poales, and Rosales were the most frequently detected plant orders with Refseq. Refseq identified all the taxa that were identified with the nt database, but in addition also Asparagales (1×), Gentianales (1×), and Caryophyllales (3×) (Figure 9).
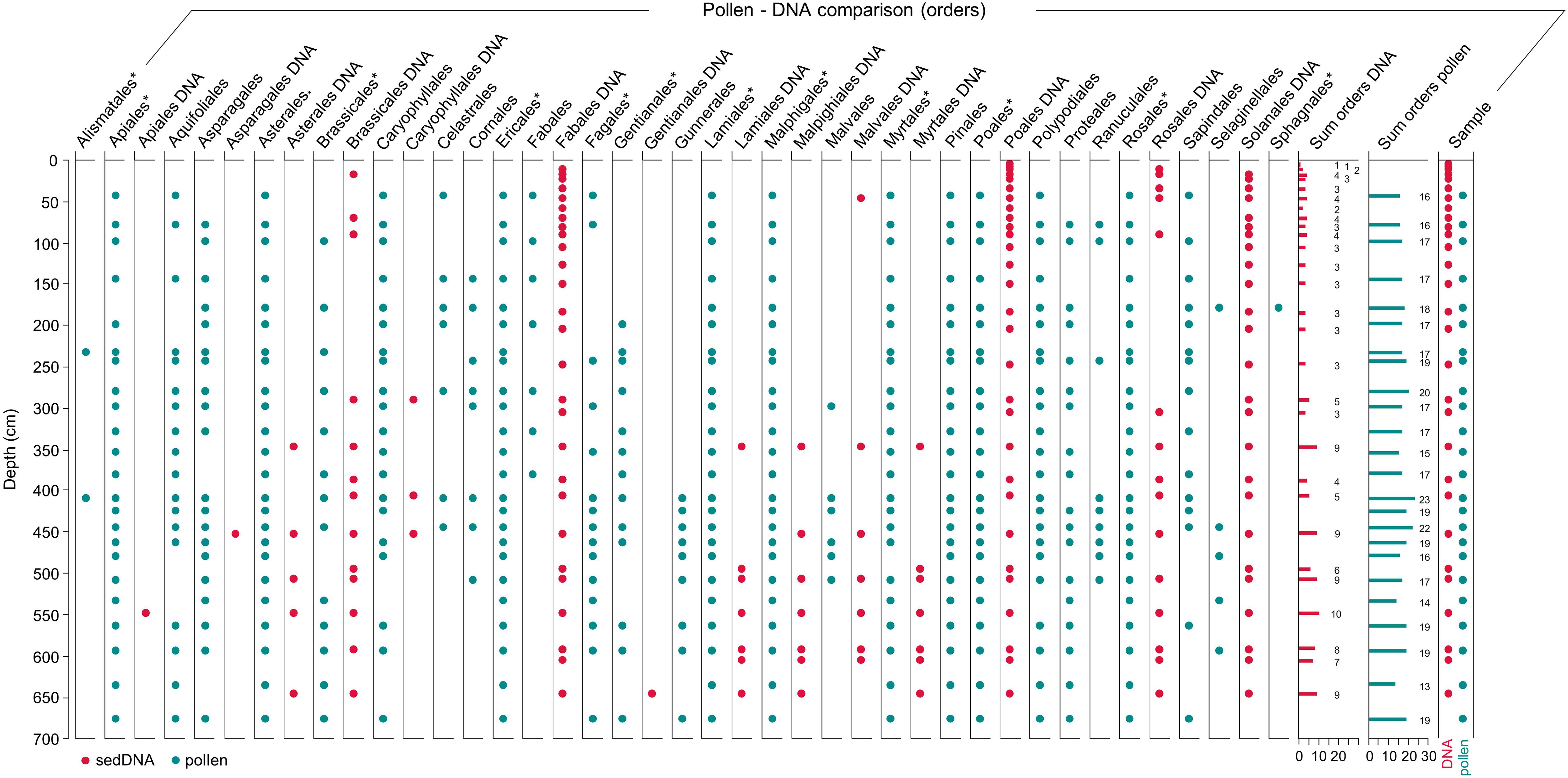
Figure 9. Comparison of pollen and DNA record from Mubwindi Swamp. Plant DNA is from the master core MUB17-2D/C. DNA taxonomic assignments are based on both nt and Refseq output. The pollen data are from core MUB 3 of Marchant et al. (1997) and pollen types have been combined into their corresponding plant orders. Plant orders that include local pollen taxa are denoted by an asterisk. Each dot represents a record in the sediment core, red dots DNA and cyan dots pollen.
Using the nt database, MEGAN assigned sedDNA sequences to 26 different animal taxa (Metazoa) of which 9 are at the level of order (Figure 10A). The most frequently recorded orders are Diptera (in all samples), Primates, Hymenoptera, and Cetartiodactyla. All of the detected taxa occur at present in Africa and members of all recorded orders in Bwindi Impenetrable Forest. In the master core between 1 and 5 orders/sample were detected and the number of assigned orders increased slightly with depth (age) (Figure 10A). In contrast, the Refseq database assigned sequences to 41 animal taxa representing 15 orders. Diptera, Hymenoptera, and Rodentia were detected in all samples, followed in frequency by Primates, Carnivora and Cetartiodactyla. In the master core, the number of detected orders per sample ranged from 3 to 13, with the minimum recorded at 4 cm and the maxima between 300 and 500 cm depth (Figure 10B). Four assigned taxa (Protacanthopterygii, Metatheria (= marsupials), Cetacea, Octopoda) do not occur in (tropical) Africa and we consequently considered them misidentifications. Cetacea and Octopoda were only detected once but Metatheria was detected regularly in the master core (Figure 10B).
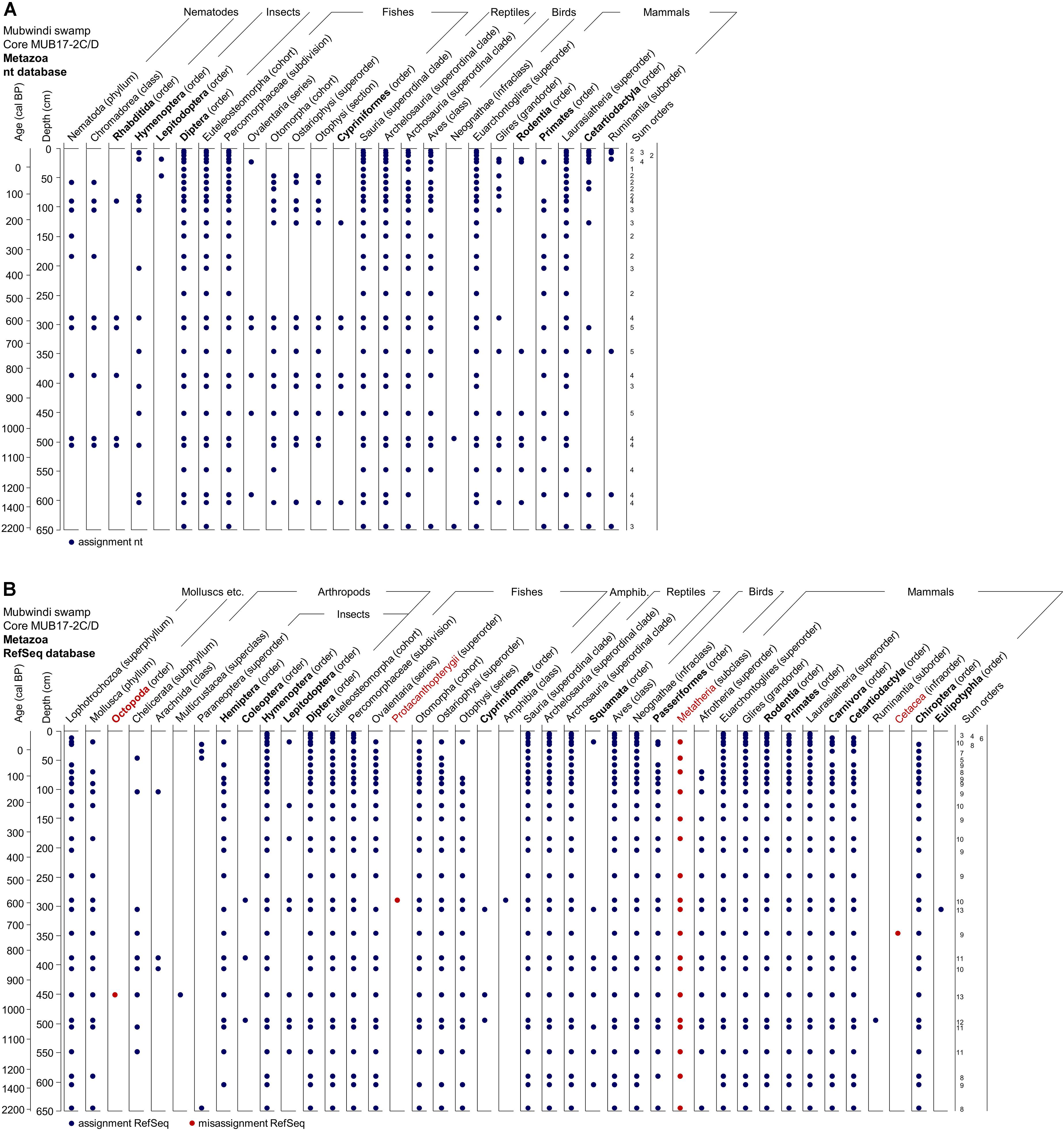
Figure 10. Metazoa taxa observed in DNA preserved in the Mubwindi Swamp sediments. Metazoa DNA is from the master core MUB17-2D/C. DNA taxonomic assignments are based on both nt (A) and Refseq (B) output. Orders are in bold. Each dot represents a record in the sediment core. Taxa in red indicate likely taxonomic misassignments since these taxa are unknown in the area.
The taxonomic assignments for Metazoa from both reference databases had 22 taxa with the following seven orders in common: Cypriniformes, Rodentia, Primates, Cetartiodactyla, Hymenoptera, Lepidoptera, and Diptera. Excluding misidentifications, Refseq uniquely identified the orders Squamata, Passeriformes, Eulipotyphla, Carnivora, Chiroptera, Hemiptera, and Coleoptera whereas the nt database uniquely yielded Cyprindontiformes and Rhabditida. Together both databases detected a total of 45 assigned animal taxa of which 17 are at ordinal rank.
Microbial Composition Using MetaPhlAn2 and QIIME 2
MetaPhlAn2 only identified a total of 11 bacterial (n = 7) and archaeal (n = 4) species/strains representing 5 bacterial and 1 archaeal orders. No taxa were identified for 30 of the sediment samples or for any of the negative controls (Supporting Data). We did not analyze these data further given that they were uninformative.
QIIME 2 identified between 0 and 1823 unique 16S sequences per sediment sample (Mean ± SD: 238 ± 412 sequences; Supporting Data). No 16S sequences were identified in the negative controls. Excluding samples with no retained reads, 27.3–100.0% were assigned to Bacteria (Mean ± SD: 74.6 ± 20.2%) and 0.0–72.7% were assigned to Archaea (Mean ± SD: 25.4 ± 20.2%). As in the MEGAN analyses, the percentage of reads assigned to Archaea increased with depth (r2 = 0.72). Community compositions were exceptionally complex, with no taxa dominating the assignments (Supporting Data). In the dataset rarified to 50 sequences, PCoA separated the samples from the first meter of sediment from the remaining sediment samples (Supporting Data). PCoA did not reveal informative patterns in dataset rarified to 500 sequences due to the low sequencing depth eliminating most samples (n = 8 after rarefaction; Supporting Data).
Discussion
Sedimentary ancient DNA has been proposed as a powerful tool in conservation biology and paleoecology (e.g., Boessenkool et al., 2014; Rawlence et al., 2014; Epp, 2019). However, many aspects on the production, dispersal, deposition and accumulation of DNA in sediments of terrestrial ecosystems and on the taphonomy of sedaDNA remain to be studied (Epp, 2019). This need is particularly true for the tropics where most of the world’s species occur. Our study highlights challenges and opportunities of sedaDNA approaches for reconstructing tropical biodiversity which we identify from the investigation of multiple profiles from a tropical swamp located within an exceptionally diverse African rainforest. The cores from Mubwindi Swamp allow us to examine sources of DNA, its taxonomic composition and the conditions of DNA preservation in different types of sediments. Our integrative approach allows for a comprehensive view of taphonomic processes, and cross-validation of our results, in the Mubwindi Swamp (Armbrecht et al., 2019; Giguet-Covex et al., 2019).
Sources of Metagenomic DNA
The deposition of DNA in a sedimentary basin and its representation of the locally present biota are influenced by the abundance of the locally present species, their biomass, their genome length, transport processes and by chance (but see Andersen et al., 2012; Yoccoz et al., 2012; Giguet-Covex et al., 2019). Four possible sources provide DNA to the sediments of Mubwindi Swamp including (1) DNA from micro- and macro-organisms living in the sediment, (2) DNA from organisms living on or utilizing the surface of the swamp, (3) DNA derived via streams and runoff from the catchment of the swamp (see Giguet-Covex et al., 2019), and (4) DNA derived by deposition from the air (e.g., pollen grains: Parducci et al., 2005). Given these sources, DNA extracted from the sediments will be a mixture of modern and ancient DNA.
Mubwindi Swamp was apparently an open water environment (shallow lake) from about about 1320 to ∼650 cal BP and then served as habitat for (semi)aquatic biota (e.g., Cypriniformes; Figure 10) and very likely as a water source to terrestrial fauna (e.g., Cetartiodactyla; Figure 10). At this stage the sediments received local authochtonous DNA and allochotonous DNA influx from the catchment that could be transferred to the central part of the basin. In the master core the taxonomic diversity is highest in the clayey sediment below ∼330 cm depth (older than ∼720 cal BP) corresponding to the shallow lake phase. Possibly the Mubwindi basin received more diverse DNA via erosion and runoff from its catchment during this phase than the peatland that formed later. Slightly higher sedimentation rates prior to 800 cal BP together with higher XRF counts of Al and Ti (Figure 5), which are indicators of erosion, support this interpretation. At about 650 cal BP the peatland had formed (Figure 3) and terrestrial habitat conditions became established that changed DNA transport pathways as a consequence.
Swamps and peatlands provide habitat to a diverse group of organisms that live in the organic deposits. Microbes will contribute modern DNA over the entire sediment column and thus disturb ancient DNA signals. They are expected to be most abundant in the aerobic zone of the peat surface of Mubwindi Swamp (water table: 0–14 cm measured during field work), where also high soil temperatures and in the center of the swamp also higher nutrient concentrations likely favor microbial activity (Dickinson, 1983; Dabney et al., 2013b; Table 2). Generally, the aerobic zone of peat deposits in swamps is habitat for various protozoa (e.g., amoeba) and soil fauna (e.g., mites, collembola, nematodes; Mason and Standen, 1983; Speight and Blackith, 1983) and also the rooting zone for most plants (Crawford, 1983). These organisms will add their DNA to the sediments as indicated by the detection of nematode DNA (Figure 10A). Deeper anaerobic conditions are likely to be associated with reduced microbial life but provide habitat for Archaea (Figure 7). Plants with anatomical transport mechanisms for oxygen will be able to survive in this waterlogged environment (Crawford, 1983). Radiocarbon dating of roots has shown that living herbaceous plants can insert their roots into waterlogged sediments of subtropical swamps to a depth of 60 cm (Glaser et al., 2012). Therefore, living plants may also be contributing DNA directly into the upper sediment column. We expect that the roots of the sedges Cyperus latifolius and Cyperus denudatus, which dominate most areas of Mubwindi Swamp and likely contribute a large proportion to the peat, represent a major source of sedDNA as indicated by the frequent detection of Poales (Figure 9).
Given these conditions most biotic activity should be concentrated in approximately the upper 25 cm of the sediment column. Indeed, from this zone most DNA was extracted from the cores and the DNA shows the lowest proportion of deamination and fragmentation (Figure 7). The extracted DNA pool from the upper sediment column thus contains a large contribution of modern DNA from living biota. Hence, the decline in the DNA yield with depth is not simply an indication of DNA degradation, but also of decreasing biotic activity in the sediment column. This high proportion of in situ derived DNA will also overwhelm signals of DNA derived from passing animals and influx from the swamp’s catchment. Moreover, the biotically active zone will contribute a certain signal of time-averaging by all those species that can exist across a wider zone (>20 cm) of sediment. Time-averaging of sedaDNA may therefore be a specific problem in slowly accumulating systems. Permanent and deep lakes with anoxic bottom waters may be better sources for catchment studies of past biota. Yet modern microbial DNA will always be a significant part of any sedaDNA profile.
Mubwindi Swamp sedaDNA Preservation
The collected sediment cores differ substantially in terms of their composition and the sediments show a large range in water content (15–94%), organic matter content (6–92%), nutrients (N, P, K), pH (4.4–6.7), and temperature (14.6–20.6°C; Figure 4). DNA is preserved in all sediment types and persists under various environmental conditions with few clear explanatory trends (Figure 11 and Supporting Information). DNA is thus degrading at similar rates in different sedimentary environments with varying nutrient, pH and temperature conditions. General trends in our data are (1) the exponential decline in the amount of DNA with sediment depth and age, (2) the increase in DNA fragmentation with depth and age, and (3) the increase in 5′ C→T and 3′ G→A substitutions with depth and age (Figure 7). Nevertheless, the preserved DNA’s read lengths, deamination rates, and quantities show significant monotonic relationships with almost all environmental factors within the master core, including those unlikely to have a direct causative relationship (e.g., sediment magnetic susceptibility: Figure 11 and Supporting Information). This finding suggests that there is not a single environmental driver or simple set of factors that influence the degradation of DNA in swamp sediments (Figure 11; Dabney et al., 2013b) and complicates the generation of a comprehensive statistical model for DNA preservation in the Mubwindi Swamp due to environmental parameter non-independence. The roles of local sedimentation patterns and sediment types in DNA preservation demand further study.
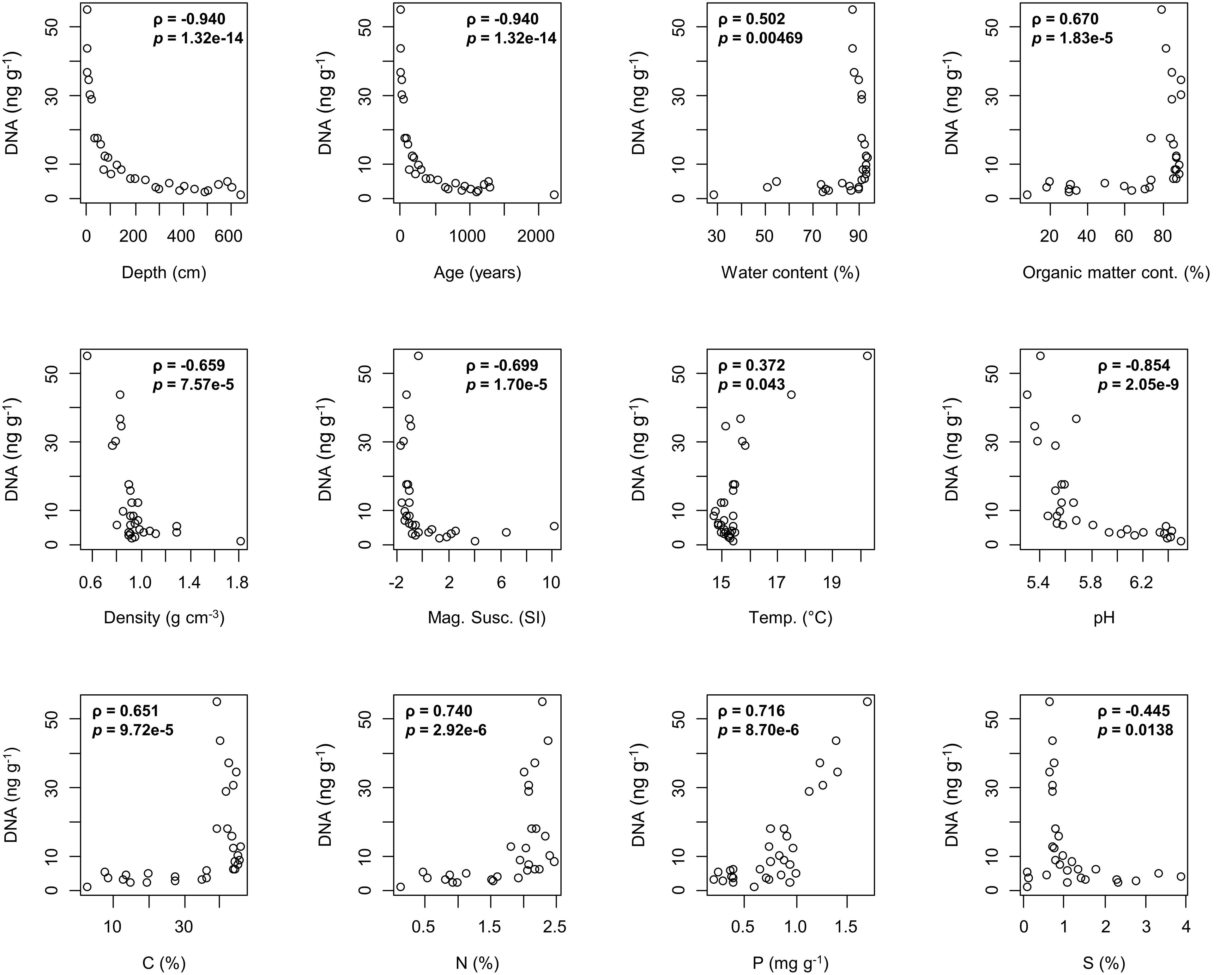
Figure 11. Relationships between DNA fraction and environmental parameters for the master core MUB17-2C/D. The strength (Spearman’s ρ) and statistical significance (uncorrected p-values) of non-parametric correlations are given for each comparison.
At the master core site, the DNA in the basal sand layer was apparently deposited under drier conditions with neutral pH and lower nutrient levels than those above (Figure 4), which likely limited hydrolysis immediately after deposition (Lindahl, 1993; Pääbo et al., 2004). Although such conditions have been associated with comparably “good” sedaDNA preservation in caves (e.g., Hofreiter et al., 2003; Slon et al., 2017), at Bwindi the amount of remaining DNA and its fragmentation in the basal sediments are very similar to that of the overlaying wetter acidic organic peat deposits (Figure 7). Although higher taxonomic diversity was detected in the deeper minerogenic sediments than in the peat, we assume that catchment-scale transport processes rather than differential lithologic control on preservation account for these findings. Organic matter content also appears to have limited effects on the quantity of preserved DNA and its fragmentation (Figure 4). In the master core, DNA yield and fragmentation reach background levels below about 200 cm, where organic matter is present in a wide range of values (6–88%). Thus, the deposition in clastic sediment (sandy, silty, or clay deposits) or in organic peat deposits seems not to determine how well DNA is preserved in the long-term. This conclusion is supported by similar initial amounts of DNA yield in the surface of all cores and the similar decline in DNA yield in the first meter of the short cores (4A, 5A) (Figure 6A), which entirely consist of clay in contrast to the master core’s upper peat layer (Figure 3).
In addition, the variation in the elemental composition of the master core is also not reflected in variations of DNA yield or preservation stage. Despite large changes in the quantity of cations such as K, Al, or Fe we find no indication for preferential preservation through DNA adsorption in zone of higher concentrations of metals (Figure 5). The DNA patterns of the short cores with their high Fe XRF counts and clay content corroborate this inference.
As predicted by current models of DNA preservation (e.g., Dabney et al., 2013b; Kistler et al., 2017), the vast majority of DNA content loss occurs rapidly after deposition in the Mubwindi Swamp (Figures 6, 7). Not only was there a sharp decline in DNA content below the top meter of bioactive sediment, we observed very few eukaryotic sequences, even in sites of recent elephant activity, suggesting that deposited DNA degrades rapidly in the acidic and warm (17–21°C) swamp environment (Giguet-Covex et al., 2019). Based on laboratory and field experiments, acidic, warm conditions are known to promote DNA hydrolysis and are non-conducive to long-term DNA preservation (Lindahl and Nyberg, 1972; Lindahl, 1993; Strickler et al., 2015; Kistler et al., 2017; Seymour et al., 2018). However, inference of the influence of pH and temperature on DNA degradation by direct comparison of DNA yield with these parameters is misleading. Most DNA is deposited at the sediment surface (where DNA yield, acidity, and temperature are highest), whereas DNA yield declines with depth as acidity and temperature decrease. This finding likely indicates the concentration of DNA from living biota in the sediment surface where an aerobic, warm, and more nutrient-rich environment facilitates abundant plant growth and microbial and soil faunal activity. DNA degradation under surface conditions only becomes apparent when deposition time is considered. In the swamp’s center, DNA is deposited at the sediment surface at > 20°C and then exposed to the maximum burial temperatures for over 50 years (= 25 cm depth) until temperature stabilizes at about 15.5°C (Figure 4). This time of exposure to high temperatures (and low pH) will likely drive rapid DNA degradation, while at the same time the in situ microbial contribution to the DNA pool should also decrease as the sediment changes from aerobic to anaerobic as inferred from the presence of Archaea and the measured minimum position of the water table at -14 cm. Furthermore, the higher concentrations of sedimentary nitrogen and phosphorus near the surface likely contribute to DNA degradation by stimulating microbial decay (Dabney et al., 2013b; Figure 4) and are thus not reflecting good preservation conditions as suggested in Figure 11.
Although the reduced DNA content in earlier (deeper) strata likely correlates with decreased biotic activity at these depths, statistically, age and depth are the best predictors for the remaining fraction of DNA in the sediment (age/depth: r2 = 0.755, p < 0.001). Furthermore, the decreasing fragment lengths and increased cytosine deamination of DNA sequences deriving from deeper, earlier sediments suggest that at least a portion of these molecules are preserved ancient DNA. Moreover, the pattern of increasing cytosine deamination with depth is not consistent with the DNA from deeper strata deriving solely from water-driven leaching of DNA from extant, living and recently deceased organisms through the sediment column despite up to > 90% sediment water content. In the case of the leaching scenario, we would expect all sediment DNA samples to exhibit roughly the same level of deamination since they would all be nearly of the same age. Furthermore, in the leaching scenario, we would expect a continuous gradient in DNA content down the core. Instead, we find that the DNA content stabilizes below the first meter of sediments. We conclude that, despite the acidic condition, genuine ancient DNA has survived in Mubwindi Swamp for over 2200 years and sedaDNA is therefore retrievable from tropical swamps.
Our data suggest a tropical sediment model in which most DNA degrades beyond recovery rapidly after deposition in the swamp (<200 years) (Bremond et al., 2017). The majority of DNA molecules in the most recent sediment layers likely derive from ongoing biotic activity rather than preserved ancient biomolecules. The small portion of DNA molecules that survive this initial stage of elimination become stabilized in the less acidic and cooler deeper sediment levels, which are more conducive to long-term DNA survival. These deeper sediments still preserve a sedaDNA record of diverse taxonomic assemblages. In contrast to other studies of African sedaDNA (Boessenkool et al., 2014; Bremond et al., 2017) we, in fact, observe no decline in the number of taxa with increasing sediment age. Instead the master core contains higher taxonomic diversity in sediment older than ca. 600 cal BP. The absence of a declining trend in taxonomic richness with age suggests that sedaDNA can provide information on past tropical biodiversity for several thousand years.
Challenges for sedaDNA Studies
DNA Recovery Biases
Recovered ancient DNA molecules will vary in length and quantity by DNA extraction and library preparation protocols (Rohland and Hofreiter, 2007; Meyer and Kircher, 2010; Dabney and Meyer, 2012; Dabney et al., 2013a; Gansauge and Meyer, 2013; Gansauge et al., 2017; Glocke and Meyer, 2017; Rohland et al., 2018). A wide-variety of aDNA extraction methods, including silica-based, alcoholic, and phenol-chloroform protocols, exist (Rohland and Hofreiter, 2007; Hagan et al., 2020), each optimized to various substrates. Similarly, a multitude of aDNA library preparation protocols have been developed, including double-stranded (e.g., Meyer and Kircher, 2010), single-stranded (Gansauge and Meyer, 2013; Gansauge et al., 2017) and single-tube approaches (Carøe et al., 2018). Even subtle experimental decisions can have significant downstream effects. For instance, within silica-based extractions, choices of extraction buffers (Rohland and Hofreiter, 2007), binding buffers and matrices (Dabney et al., 2013a; Rohland et al., 2018), and purification protocols (Rohland et al., 2018) have significant effects on DNA yields and length biases. The choice of library amplification polymerases has been shown to have significant impacts on molecular length and GC content (Dabney and Meyer, 2012).
Since sediments are heterogenous substrates, sedimentary DNA analyses may be prone to extraction and library preparation biases due to extraction and library protocols being insufficiently optimized across strata (e.g., sand vs. silty clay within the Mubwindi core). Moreover, community structure reconstructions will vary depending on chosen extraction method because these protocols vary in their recovery efficiency between intra- and extracellular DNA (e.g., Vuillemin et al., 2017). We used the PowerSoil extraction kit as it has been shown to be relatively efficient across a wide variety of substrates (including ancient samples: Hagan et al., 2020) and is therefore the recommended protocol of the Earth Microbiome Project (Marotz et al., 2017; Thompson et al., 2017) and the Human Microbiome Project (Aagaard et al., 2013). The microbial results are thus highly comparable to those of these large-scale projects. Nevertheless, the PowerSoil protocol has not been specifically optimized for sedaDNA extraction (e.g., Armbrecht et al., 2020) and is likely to have inefficiently extracted the shortest aDNA molecules (Rohland et al., 2018). This bias is compounded by our double-stranded library preparation protocol, which while more cost- and time-efficient, is less likely to recover the most damaged aDNA molecules than a single-stranded approach (Gansauge and Meyer, 2013; Gansauge et al., 2017; Glocke and Meyer, 2017).
Mixture of Modern and Ancient DNA
The mixture of modern and ancient DNA in sediments complicates the reconstruction of past ecosystems. Extracting authentic eukaryotic ancient DNA from a pool of DNA dominated by modern and ancient microbial DNA is a major challenge due to their rarity (e.g., Slon et al., 2017). Every sedimentary deposit is also a habitat to microbes and so there is no depositional environment that solely contains ancient DNA. Authenticating past microbial life is even more challenging as ancient microbes will be very similar to extant species currently inhabiting the sediment (e.g., Campana et al., 2014). DNA results from environments impacted by significant leaching will be even harder to authenticate (Haile et al., 2007).
In addition, determining both the drivers of DNA decay and the half-life of sedaDNA is exceptionally challenging with a mixed assemblage of ancient and modern DNA. For example, a low pH is expected to lead to both faster DNA degradation and reduced biotic activity and therefore lower modern DNA contribution, with both effects contributing to lower DNA yield. Unlike with samples of known species (such as bone: Allentoft et al., 2012), any approach to calculate DNA degradation half-life must incorporate the DNA contributions from both living or recently deceased organisms in a sediment context, which could render results ambiguous.
Limitations of Current Metagenomic Databases
Our ecological community reconstructions were limited by very low sequence identification rates (<13% in all sediment samples). Of a total 81.8 million unique, merged reads, only 6.3 million can be assigned to taxonomic entities (7.7%) with the Refseq database (4 million (4.9%) with the nt database). This result is unsurprising given that Ugandan taxa in general are nearly unrepresented in genomic reference databases. Nevertheless, low sequence identification rates from metagenomic sedaDNA studies are not limited to the tropics. Ahmed et al. (2018) and Parducci et al. (2019) were only able to assign ∼16 and 2.3% of reads, respectively, from Hässeldala Port (southern Sweden) sediments. Similarly, Slon et al. (2017) identified only between 4 and 21% of metagenomic reads from Eurasian Pleistocene cave sediments. Low identification rates are problematic for ancient DNA research as they increase experimental costs and decrease reliability in the generated community composition profiles.
Our data suggested systematic biases due to sequence database limitations in the reconstructed profiles. Eukaryotic sequences were very rare (nt: 4.4% of identified sequences, 0.2% of total merged reads; Refseq: 4.0% of identified sequences, 0.3% of total merged reads) in the Mubwindi Swamp sediments. The rarity of plant sequences is surprising given the dominance of plant biomass in the Mubwindi Swamp and the frequency of identified plant-associated microbial taxa. Similarly, Parducci et al. (2019) identified only 1,634 plant reads from ∼1 billion metagenomic sequences (representing < 0.1% of assigned reads) from Hässeldala Port sediments, indicating that poor plant identification is a common issue using current databases. Yet, at the taxonomic rank of order, the few plant DNA sequences evidently provide a reliable reconstruction of past floristic composition from the Mubwindi Swamp and its catchment. A comparison of the sedDNA plant data with published pollen records from Mubwindi Swamp (Marchant et al., 1997; Marchant and Taylor, 1998) showed good agreement between these independent datasets (Figure 9). Thirteen of the 14 orders detected by sedDNA were also recorded by pollen analysis (Marchant et al., 1997). The order Solanales not found as pollen, however, contains species that occur in Bwindi today (e.g., Stanford and Nkurunungi, 2003). One of the most frequently taxa detected by sedDNA is Poales, which includes the family Cyperaceae and which is the most common pollen type in the cores of Marchant et al. (1997). This observation is consistent with the dominance of sedges in Mubwindi Swamp and their addition of belowground biomass (roots, rhizomes) to the peat deposit.
Core MUB3 of Marchant et al. (1997) is closest to our master core allowing us to compare presence of taxa with depth (Figure 9). In general plant DNA detects about a quarter (∼15–60%) of the orders found as pollen in individual samples of similar depth. The majority of taxa identified by sedDNA matches pollen taxa that are of local origin (e.g., Poales, Rosales), that is pollen produced by plants that (potentially) grow in Mubwindi Swamp (Figure 9; Marchant et al., 1997). An exception is Fabales which were regularly detected by DNA, yet Newtonia buchananii a member of Fabales is a common tree in the swamp’s direct catchment and a possible past DNA source (Marchant et al., 1997). These observations generally suggest that most of the sedDNA was derived from local sources.
The relatively good taxonomic correspondence and similar detection with depth between DNA and pollen indicates that shotgun-sequenced sedaDNA is reliably recording plant history in the tropics at the ordinal level. Given the large proportion of unknown plant DNA in our data we expect increases in both the detection of taxa (diversity) and the resolution of taxonomic level with future improvements of species coverage in genomic databases. For sedaDNA to outperform the taxonomic resolution of pollen analysis first necessitates genome-sequencing of the majority of plant species in Bwindi Impenetrable Forest and in tropical forests in general. However, we advocate to apply both methods in combination when reconstructing past floristic diversity.
This study, the first examining Metazoan assemblages with sedaDNA in tropical Africa, successfully revealed the past occurrence of 16 native orders of animals at Mubwindi Swamp. Species belonging to all these orders occur in Bwindi today and members of most of them, including Rodentia, Primates, Carnivora, Cetartiodactyla, Eulipotyphla, Chiroptera, Passeriformes, Squamata, Lepidoptera, Diptera, Hymenoptera, Hemiptera, and Coleoptera visit or inhabit Mubwindi Swamp at present (e.g., Butynski, 1984; Kasangaki et al., 2003, 2008; Decru et al., 2019; pers. observ.), supporting the correctness of these taxonomic detections in the sediment record. The co-occurrence of most animal taxa in our sedaDNA data-set, in particular the frequent occurrence of Afrotheria, Euarchontoglires (including Rodentia and Primates), Carnivora, and Cetartiodactyla (Figure 10) suggest that Bwindi Impenetrable Forest was inhabited by typical African faunal assemblages during the past 2200 years. Intriguing is the missing or low detection of various wetland taxa that are common in Mubwindi Swamp today such as Odonata, Trichoptera, Anura, Gastropoda or Eulipotyphla and which should therefore be important DNA sources. This result could suggest that these important groups are particularly underrepresented in genomic surveys. The detection of vertebrates is moreover biased by species which have been genome sequenced – mostly large, charismatic taxa and model species (Brandies et al., 2019). Smaller and elusive animal species that constitute most of the diversity, such as shrews and frogs will be less likely detected. A comprehensive reconstruction of animal diversity of Bwindi Impenetrable Forest would therefore be premature at this stage and systematic genomic surveys of Bwindi taxa are required in order to produce more reliable, precise ancient DNA taxonomic profiles that can serve as baseline data for conservation.
Assigning taxa below ordinal rank is still prone to large uncertainties given the inadequate coverage of Afrotropical taxa in reference databases. This challenge is emphasized by the assignment of reads to several exotic animal taxa that either have no Quaternary record in tropical Africa (Metatheria, Protacanthopterygii) or inhabit the ocean (Cetacea, Octopoda, partly Protacanthopterygii) (Figure 10B). The issue of dealing with higher taxonomic levels and more importantly the 92% of unidentifiable data restrict clear insights into community dynamics over time. Moreover, the choice of reference database has also significant effects on the resulting taxonomic composition of metagenomic data. Whereas the nt database derived record shows a scattered presence without clear temporal trend for most animal orders at Mubwindi, the Refseq derived data indicate a nearly continuous presence for over half of the detected orders, particularly for mammals (Figure 10). These contrasting results emphasize that multiple genomic reference databases should be explored in tandem when working with shotgun-sequenced sedaDNA data-sets.
We also found systematic biases due to differences between metagenomic analysis pipelines. QIIME 2 analyses found that Archaea comprised a much larger portion of the microbial community than in the MEGAN dataset (Figure 7). This result likely reflects differences in taxonomic database biases. Far more complete bacterial genomes have been sequenced than archaeal ones (25,496 bacterial genomes vs. 1,680 archaeal in the NCBI Genome database as of 28 October 2019), resulting in a bias toward the preferential identification of bacterial sequences. Although the same bias exists in the 16S dataset (592,561 bacterial and 25,026 archaeal sequences in the SILVA build 132 non-redundant 16S database), 16S has been better characterized for both domains, reducing the bias’s impact. The MEGAN profile therefore probably undercounts the presence of Archaea relative to Bacteria, with the QIIME 2 profile being more representative of the true community. Similarly, MEGAN documented dominance of the microbial communities by Rhizobiales and Burkholderiales, which was not observed in the QIIME 2 dataset. In this case, it is difficult to determine which pipeline produced the more accurate community reconstructions. The choice of database for microbial detection is also critical: our MEGAN analyses found slight, but significant, taxonomic compositional differences between the nt- and Refseq-aligned datasets. As found previously (Ye et al., 2019), MetaPhlAn2 performed poorly as a metagenomic classifier. We recommend against its further use in sedaDNA research. The megaBLAST/MEGAN pipeline is common in sedaDNA analyses (e.g., Parducci et al., 2019), even being called the “gold standard” by Cribdon et al. (2020). QIIME (Caporaso et al., 2010) and QIIME 2 are common in sedaDNA analyses using metabarcoding (e.g., Ziesemer et al., 2015). We therefore employed these classifiers for comparability with extant datasets. Nevertheless, metagenomic standards and classification algorithms are advancing rapidly (Ye et al., 2019; Cribdon et al., 2020), which will necessitate development of more accurate ancient DNA classifiers such as PIA (Cribdon et al., 2020) and MALT (Vågene et al., 2018).
Conclusion
We present one of the richest biogeological and DNA datasets for tropical sediments yet published. Using this dataset, we model ancient DNA preservation in the Mubwindi Swamp, showing that age and depth are the strongest determinants of DNA preservation and fragmentation, but that almost all environmental parameters have monotonic relationships with DNA degradation. Surprisingly, microenvironmental conditions and lithology show few systematic impacts on DNA preservation in this sedimentary basin. We show that metagenomic sedimentary DNA can provide valuable insights into past tropical biodiversity, but that further development of genomic databases is necessary to provide robust, detailed community reconstructions. The actual taxonomic composition and resolution of our recovered DNA would likely change if all sequences could be identified. This problem of a skewed reconstruction of taxonomic composition will be present in any future sedaDNA study for the tropics until the genomes of most tropical species have been sequenced.
Besides a fundamental improvement of genomic databases, the transport, deposition, and ultimate representation of sedaDNA of different ecosystems need to be systematically investigated. Calibration studies should inventory a site’s extant diversity and compare its biota with the taxonomic composition of environmental DNA samples from various locations and transport pathways (streams etc.) within the site’s catchment and basin. Until taxonomically representative databases are generated and further DNA taphonomic studies are completed, sedaDNA cannot be fully utilized for biodiversity studies in the tropics.
Data Availability Statement
The datasets generated for this study can be found in the Sequence Read Archive accession PRJNA573108.
Author Contributions
RD, MM, RP, JM, and MC secured the funding. RD, MM, JM, and MC designed the study. RD, MA, MM, JN, and MC performed the field sampling. RD, MA, and JN secured the necessary permits for the study. RD, MA, NP, TG, and JN performed the experiments. RD and MC analyzed and archived the data. RD and MC wrote the manuscript with contributions from all co-authors. All authors contributed to the article and approved the submitted version.
Funding
This material was based upon work supported by the Global Genome Initiative under Grant No. (GGI-Exploratory Science-2016) awarded to RD. This work was financially also supported by Geo.X, the Research Network for Geosciences in Berlin anPotsdam. RD acknowledges a KoUP seed fund from the University of Potsdam for establishing collaboration with Mbarara University of Science and Technology. The Smithsonian Institution (Human Origins Program, National Museum of Natural History and the Smithsonian National Zoological Park and Conservation Biology Institute) supported this research. Elevation data in Figure 1 are based on the TanDEM-X Science DEM granted to RD by the German Space Agency (DLR 2017). We acknowledge the support of the open-access publication fund of the University of Potsdam.
Conflict of Interest
The authors declare that the research was conducted in the absence of any commercial or financial relationships that could be construed as a potential conflict of interest.
Acknowledgments
We acknowledge the LacCore facility for their support in equipment renting, core storage and processing. This research and core export were approved by the Uganda National Council of Science and Technology (Ref. No. NS13ES) and by the Uganda Wildlife Authority (Ref. No. UWA/COD96/05). We are grateful for the support from Lornah Nabukwasi, Jessica Quinta, Lydia Olaka, Casim Umba Tolo, Julius B. Lejju, Matt Tocheri, Bodo Bookhagen, Manfred Strecker, Paul Glaser, and Mark Shapley and from Jörg Gelbrecht, Grit Siegert, Constancia Levertz, Elisabeth Schuette, Sarah Krocker, and Thomas Rossoll of IGB Berlin. At Bwindi we received generous help from the Uganda Wildlife Authority, especially from wardens George Businge, Raymond Kato, Joseph Arinaitwe, and Pontius Ezuma as well as from all staff of the Institute of Tropical Forest Conservation, Chrispino Mugarura, the Uganda Police Force and from many porters at Ruhija. This research was conducted using the Smithsonian Institution High Performance Cluster (SI/HPC): https://doi.org/10.25572/SIHPC.
Supplementary Material
The Supplementary Material for this article can be found online at: https://www.frontiersin.org/articles/10.3389/fevo.2020.00218/full#supplementary-material
Footnotes
References
Aagaard, K., Petrosino, J., Keitel, W., Watson, M., Katancik, J., Garcia, N., et al. (2013). The Human Microbiome Project strategy for comprehensive sampling of the human microbiome and why it matters. FASEB J. 27, 1012–1022. doi: 10.1096/fj.12-220806
Adams, C. I. M., Knapp, M., Gemmell, N. J., Jeunen, G.-J., Bunce, M., Lamare, M. D., et al. (2019). Beyond biodiversity: Can environmental DNA (eDNA) cut it as a population genetics tool? Genes 10:192. doi: 10.3390/genes10030192
Adler, C. J., Haak, W., Donlon, D., and Cooper, A., and Genographic Consortium. (2011). Survival and recovery of DNA from ancient teeth and bones. J. Archaeol. Sci. 38, 956–964. doi: 10.1016/j.jas.2010.11.010
Ahmed, E., Parducci, L., Unneberg, P., Ågren, R., Schenk, F., Rattray, J. E., et al. (2018). Archaeal community changes in Lateglacial lake sediments: Evidence from ancient DNA. Quat. Sci. Rev. 181, 19–29. doi: 10.1016/j.quascirev.2017.11.037
Allentoft, M. E., Collins, M., Harker, D., Haile, J., Oskam, C. L., Hale, M. L., et al. (2012). The half-life of DNA in bone: measuring decay kinetics in 158 dated fossils. Proc. R. Soc. B Biol. Sci. 279, 4724–4733. doi: 10.1098/rspb.2012.1745
Andersen, J. (1976). An ignition method for determination of total phosphorus in lake sediments. Water Res. 10, 329–331. doi: 10.1016/0043-1354(76)90175-5
Andersen, K., Bird, K. L., Rasmussen, M., Haile, J., Bruening-Madsen, H., Kjær, K. H., et al. (2012). Meta-barcoding of ‘dirt’. DNA from soil reflects vertebrate biodiversity. Mol. Ecol. 21, 1966–1979. doi: 10.1111/j.1365-294X.2011.05261.x
Andrews, S. (2016). FastQC: A Quality Control Tool for high Throughput Sequence Data. Available at: http://www.bioinformatics.babraham.ac.uk/projects/fastqc (accessed July 15, 2016).
Armbrecht, L., Herrando-Pérez, S., Eisenhofer, R., Hallegraeff, G. M., Bolch, C. J. S., and Cooper, A. (2020). An optimized method for the extraction of ancient eukaryote DNA from marine sediments. Mol. Ecol. Resour. 1–14. doi: 10.1111/1755-0998.13162
Armbrecht, L. H., Coolen, M. J. L., Lejzerowicz, F., George, S. C., Negandhi, K., Suzuki, Y., et al. (2019). Ancient DNA from marine sediments: precautions and considerations for seafloor coring, sample handling and data generation. Earth Sci. Rev. 196:102887. doi: 10.1016/j.earscirev.2019.102887
Babaasa, D. (2000). Habitat selection by elephants in Bwindi Impenetrable National Park, south-western Uganda. Afr. J. Ecol. 38, 116–122. doi: 10.1046/j.1365-2028.2000.00226.x
Barlow, J., França, F., Gardner, T. A., Hicks, C. C., Lennox, G. D., Berenguer, E., et al. (2018). The future of hyperdiverse tropical ecosystems. Nature 559, 517–526. doi: 10.1038/s41586-018-0301-1
Blaauw, M., and Christen, J. A. (2011). Flexible paleoclimate age-depth models using an autoregressive gamma process. Bayesian Anal. 6, 457–474. doi: 10.1214/11-BA618
Blackburn, D. C., Boix, C., Greenbaum, E., Fabrezi, M., Meirte, D., Pumptre, A. J., et al. (2016). The distribution of the Bururi Long-fingered Frog (Cardioglossa cyaneospila, family Arthroleptidae), a poorly known Albertine Rift endemic. Zootaxa 4170, 355–364. doi: 10.11646/zootaxa.4170.2.8
Boessenkool, S., Epp, L. S., Haile, J., Bellemain, E., Edwards, M., Coissac, E., et al. (2012). Blocking human contaminant DNA during PCR allows amplification of rare mammal species from sedimentary ancient DNA. Mol. Ecol. 21, 1806–1815. doi: 10.1111/j.1365-294X.2011.05306.x
Boessenkool, S., McGlynn, G., Epp, L. S., Taylor, D., Pimentel, M., Gizaw, A., et al. (2014). Use of ancient sedimentary DNA as a novel conservation tool for high-altitude tropical biodiversity. Conserv. Biol. 28, 446–455.
Bolger, A. M., Lohse, M., and Usadel, B. (2014). Trimmomatic: a flexible trimmer for Illumina sequence data. Bioinformatics 30, 2114–2120. doi: 10.1093/bioinformatics/btu170
Bolyen, E., Rideout, J. R., Dillon, M. R., Bokulich, N. A., Abnet, C. C., Al-Ghalith, G. A., et al. (2019). Reproducible, interactive, scalable and extensible microbiome data science using QIIME 2. Nat. Biotechnol. 37, 852–857. doi: 10.1038/s41587-019-0209-9
Brandies, P., Peel, E., Hogg, C. J., and Belov, K. (2019). The value of reference genomes in the conservation of threatened species. Genes 10:846. doi: 10.3390/genes10110846
Bremond, L., Favier, C., Ficetola, G. F., Tossou, M. G., Akouégninou, A., Gielly, L., et al. (2017). Five thousand years of tropical lake sediment DNA records from Benin. Quat. Sci. Rev. 170, 203–211. doi: 10.1016/j.quascirev.2017.06.025
Briggs, A. W., Stenzel, U., Johnson, P. L. F., Green, R. E., Kelso, J., Prüfer, K., et al. (2007). Patterns of damage in genomic DNA sequences from a Neandertal. Proc. Natl. Acad. Sci. U.S.A. 104, 14616–14621. doi: 10.1073/pnas.0704665104
Brooks, T., Hoffmann, M., Burgess, N., Plumptre, A., Williams, S., Gereau, R. E., et al. (2004). “Eastern afromontane,” in Hotspots Revisited: Earth’s Biologically Richest and Most Endangered Ecoregions, 2nd Edn, eds R. A. Mittermeier, P. Robles-Gil, M. Hoffmann, J. D. Pilgrim, T. M. Brooks, C. G. Mittermeier, et al. (Mexico: Cemex), 241–242.
Butynski, T. M. (1984). Ecological Survey of the Impenetrable (Bwindi) Forest, Uganda, and Recommendations for its Conservation and Management. New York, NY: New York Zoological Society.
Camacho, C., Coulouris, G., Avagyan, V., Ma, N., Papadopolous, J., Bealer, K., et al. (2009). BLAST+: architecture and applications. BMC Bioinformatics 10:421. doi: 10.1186/1471-2105-10-421
Campana, M. G., Bower, M. A., and Crabtree, P. J. (2013). Ancient DNA for the archaeologist: the future of African research. Afr. Archaeol. Rev. 30, 21–37. doi: 10.1007/s10437-013-9127-2
Campana, M. G., Robles García, N., Rühli, F. J., and Tuross, N. (2014). False positives complicate ancient pathogen identification using high-throughput shotgun sequencing. BMC Res. Notes 7:111. doi: 10.1186/1756-0500-7-111
Caporaso, J. G., Kuczynski, J., Stombaugh, J., Bittinger, K., Bushman, F. D., Costello, E. K., et al. (2010). QIIME allows analysis of high-throughput community sequencing data. Nat. Methods 7, 335–336. doi: 10.1038/nmeth.f.303
Carøe, C., Gopalakrishnan, S., Vinner, L., Mak, S. S. T., Sinding, M. H. S., Samaniego, J. A., et al. (2018). Single-tube library preparation for degraded DNA. Methods Ecol. Evol. 9, 410–419. doi: 10.1111/2041-210X.12871
Ceballos, G., Ehrlich, P. R., and Dirzo, R. (2017). Biological annihilation via the ongoing sixth mass extinction signaled by vertebrate population losses and declines. Proc. Natl. Acad. Sci. U.S.A. 114, E6089–E6096. doi: 10.1073/pnas.1704949114
Crawford, R. M. M. (1983). “Root survival in flooded soils,” in Ecosystems of the World. 4A Mires: Swamp, Bog, Fen and Moor General Studies, ed. A. J. P. Gore (Amsterdam: Elsevier), 257–283.
Cribdon, B., Ware, R., Smith, O., Gaffney, V., and Allaby, R. G. (2020). PIA: More accurate taxonomic assignment of metagenomic data demonstrated on sedaDNA from the North Sea. Front. Ecol. Evol. 8:84. doi: 10.3389/fevo.2020.00084
Dabney, J., Knapp, M., Glocke, I., Gansauge, M. T., Weihmann, A., Nickel, B., et al. (2013a). Complete mitochondrial genome sequence of a Middle Pleistocene cave bear reconstructed from ultrashort DNA fragments. Proc. Natl. Acad. Sci. U.S.A. 110, 15758–15763. doi: 10.1073/pnas.1314445110
Dabney, J., and Meyer, M. (2012). Length and GC-biases during sequencing library amplification: a comparison of various polymerase-buffer systems with ancient and modern DNA sequencing libraries. BioTechniques 52, 87–94. doi: 10.2144/000113809
Dabney, J., Meyer, M., and Pääbo, S. (2013b). Ancient DNA damage. Cold Spring Harb. Perspect. Biol. 5:a012567. doi: 10.1101/cshperspect.a012567
Dean, W. E. (1974). Determination of carbonate and organic matter in calcareous sediments and sedimentary rocks by loss on ignition; comparison with other methods. J. Sediment. Res. 44, 242–248. doi: 10.1306/74D729D2-2B21-11D7-8648000102C1865D
Decru, E., Vranken, N., Bragança, P. H., Snoeks, J., and Van Steenberge, M. (2019). Where ichthyofaunal provinces meet: the fish fauna of the Lake Edward system, East Africa. J. Fish Biol. 96, 1186–1201. doi: 10.1111/jfb.13992
Dickinson, C. H. (1983). “Microorganisms in Peatlands,” in Ecosystems of the World. 4A Mires: Swamp, Bog, Fen and Moor General Studies, ed. A. J. P. Gore (Amsterdam: Elsevier), 225–243.
Díez-del-Molino, D., Sánchez-Barreiro, F., Barnes, I., Gilbert, M. T. P., and Dalén, L. (2018). Quantifying temporal genomic erosion in endangered species. Trends Ecol. Evol. 33, 176–185. doi: 10.1016/j.tree.2017.12.002
Domaizon, I., Winegardner, A., Capo, E., Gauthier, J., and Gregor-Eaves, I. (2017). DNA-based methods in paleolimnology: new opportunities for investigating long-term dynamics of lacustrine biodiversity. J. Paleolimnol. 58, 1–21. doi: 10.1007/s10933-017-9958-y
Drewes, R. C., and Vindum, J. V. (1994). Amphibians of the Impenetrable Forest, southwest Uganda. J. Afr. Zool. 108, 55–70.
Ebina, J., Tsutsui, T., and Shirai, T. (1983). Simultaneous determination of total nitrogen and total phosphorus in water using peroxodisulfate oxidation. Water Res. 17, 1721–1726. doi: 10.1016/0043-1354(83)90192-6
Epp, L. S. (2019). A global perspective for biodiversity history with ancient environmental DNA. Mol. Ecol. 28, 2456–2458. doi: 10.1111/mec.15118
Epp, L. S., Stoof, K. R., Trauth, M. H., and Tiedemann, R. (2010). Historical genetics on a sediment core from a Kenyan lake: intraspecific genotype turnover in a tropical rotifer is related to past environmental changes. J. Paleolimnol. 43, 939–954. doi: 10.1007/s10933-009-9379-7
Epp, L. S., Stoof-Leichsenring, K. R., Trauth, M. H., and Tiedemann, R. (2011). Molecular profiling of diatom assemblages in tropical lake sediments using taxon-specific PCR and Denaturing High-Performance Liquid Chromatography (PCR-DHPLC). Mol. Ecol. Resour. 11, 842–853. doi: 10.1111/j.1755-0998.2011.03022.x
Erlacher, A., Cernava, T., Cardinael, M., Soh, J., Sensen, C. W., Grube, M., et al. (2015). Rhizobiales as functional and enosymbiontic members in the lichen symbiosis of Lobaria pulmonaria L. Front. Microbiol. 6:53. doi: 10.3389/fmicb.2015.00053
Estrada, O., Breen, J., Richards, S. M., and Cooper, A. (2018). Ancient plant DNA in the genomic era. Nat. Plants 4, 394–396. doi: 10.1038/s41477-018-0187-9
Ferrari, G., Lischer, H. E. L., Neukamm, J., Rayo, E., Borel, N., Pospischil, A., et al. (2018). Assessing metagenomic signals recovered from Lyuba, a 42,000-year-old permafrost-preserved woolly mammoth calf. Genes 9:436. doi: 10.3390/genes9090436
Ganas, J., Nkurunungi, J. B., and Robbins, M. M. (2009). A preliminary study of the temporal and spatial biomass patterns of herbaceous vegetation consumed by mountain gorillas in an Afromontane rain forest. Biotropica 41, 37–46. doi: 10.1111/j.1744-7429.2008.00455.x
Gansauge, M.-T., Gerber, T., Glocke, I., Korlević, P., Lippik, L., Nagel, S., et al. (2017). Single-stranded DNA library preparation from highly degraded DNA using T4 DNA ligase. Nucleic Acids Res. 45:e79. doi: 10.1093/nar/gkx033
Gansauge, M.-T., and Meyer, M. (2013). Single-stranded DNA library preparation for the sequencing of ancient or damaged DNA. Nat. Protoc. 8, 737–748. doi: 10.1038/nprot.2013.038
Giguet-Covex, C., Ficetola, G. F., Walsh, K., Poulenard, J., Bajard, M., Fouinat, L., et al. (2019). New insights on lake sediment DNA from the catchment: importance of taphonomic and analytical issues on the record quality. Sci. Rep. 9:14676. doi: 10.1038/s41598-019-50339-1
Giguet-Covex, C., Pansu, J., Arnaud, F., Rey, P.-J., Griggo, C., Gielly, L., et al. (2014). Long livestock farming history and human landscape shaping revealed by lake sediment DNA. Nat. Commun. 5:3211. doi: 10.1038/ncomms4211
Glaser, P. H., Volin, J. C., Givnish, T. J., Hansen, B. C., and Stricker, C. A. (2012). Carbon and sediment accumulation in the Everglades (USA) during the past 4000 years: rates, drivers, and sources of error. J. Geophys. Res. Biogeosc. 117:G03026. doi: 10.1029/2011JG001821
Glenn, T. C., Nilsen, R. A., Kieran, T. J., Sanders, J. G., Bayona-Vásquez, N. J., and Finger, J. W. Jr., et al. (2019). Adapterama I: universal stubs and primers for 384 unique dual-indexed or 147,456 combinatorially-indexed Illumina libraries (iTru & iNext). bioRxiv [Preprint]. doi: 10.1101/049114
Glocke, I., and Meyer, M. (2017). Extending the spectrum of DNA sequences retrieved from ancient bones and teeth. Genome Res. 27, 1230–1237. doi: 10.1101/gr.219675.116
Gomez Cabrera, M. D. C., Young, J. M., Roff, G., Staples, T., Ortiz, J. C., Pandolfi, J. M., et al. (2019). Broadening the taxonomic scope of coral reef palaeoecological studies using ancient DNA. Mol. Ecol. 28, 2636–2652. doi: 10.1111/mec.15038
Graham, R. W., Belmecheri, S., Choy, K., Culleton, B. J., Davies, L. J., Froese, D., et al. (2016). Timing and causes of mid-Holocene mammoth extinction on St. Paul Island, Alaska. Proc. Natl. Acad. Sci. U.S.A. 113, 9310–9314. doi: 10.1073/pnas.1604903113
Green, R. E., Briggs, A. W., Krause, J., Prüfer, K., Burbano, H. A., Siebauer, M., et al. (2009). The Neandertal genome and ancient DNA authenticity. EMBO J. 28, 2494–2502. doi: 10.1038/emboj.2009.222
Green, R. E., Krause, J., Briggs, A. W., Maricic, T., Stenzel, U., Kircher, M., et al. (2010). A draft sequence of the Neandertal genome. Science 328, 710–722. doi: 10.1126/science.1188021
Hagan, R. W., Hofman, C. A., Hübner, A., Reinhard, K., Schnorr, S., and Lewis, C. M. Jr., et al. (2020). Comparison of extraction methods for recovering ancient microbial DNA from paleofeces. Am. J. Phys. Anthropol. 171, 275–284. doi: 10.1002/ajpa.23978
Haile, J., Froese, D. G., MacPhee, R. D. E., Roberts, R. G., Arnold, L. J., Reyes, A. V., et al. (2009). Ancient DNA reveals late survival of mammoth and horse in interior Alaska. Proc. Natl. Acad. Sci. U.S.A. 106, 22352–22357. doi: 10.1073/pnas.0912510106
Haile, J., Holdaway, R., Oliver, K., Bunce, M., Gilbert, M. T. P., Nielsen, R., et al. (2007). Ancient DNA chronology with sediment deposits: Are paleobiological reconstructions possible and is DNA leaching a factor? Mol. Biol. Evol. 24, 982–989. doi: 10.1093/molbev/msm016
Hofman, C. A., Rick, T. C., Fleischer, R. C., and Maldonado, J. E. (2015). Conservation archaeogenomics: ancient DNA and biodiversity in the Anthropocene. Trends Ecol. Evol. 30, 540–549. doi: 10.1016/j.tree.2015.06.008
Hofreiter, M., Mead, J. I., Martin, P., and Poinar, H. N. (2003). Molecular caving. Curr. Biol. 13, R693–R695. doi: 10.1016/j.cub.2003.08.039
Huson, D. H., Beier, S., Flade, I., Górska, A., El-Hadidi, M., Mitra, S., et al. (2016). MEGAN Community edition—interactive exploration and analysis of large-scale microbiome sequencing data. PLoS Comput. Biol. 12:e1004957. doi: 10.1371/journal.pcbi.1004957
Jacobs, B. F., Pan, A. D., and Scotese, C. R. (2010). “A review of the Cenozoic vegetation history of Africa,” in Cenozoic Mammals of Africa, eds L. Werdelin, and W. J. Sanders (Berkeley, CA: University of California Press), 57–72.
Jenkins, C. N., Pimm, S. L., and Joppa, L. N. (2013). Global patterns of terrestrial vertebrate diversity and conservation. Proc. Natl. Acad. Sci. U.S.A 110, E2602–E2610. doi: 10.1073/pnas.1302251110
Jónsson, H., Ginolhac, A., Schubert, M., Johnson, P. L., and Orlando, L. (2013). mapDamage2.0: fast approximate Bayesian estimates of ancient DNA damage parameters. Bioinformatics 29, 1682–1684. doi: 10.1093/bioinformatics/btt193
Jousse, H. (2017). Atlas of Mammal Distribution Through Africa from the LGM (˜18Ka) to Modern times. The Zooarchaeological Record. Oxford: Archaeopress Publishing Ltd.
Kahindo, C. M., Bates, J. M., and Bowie, R. C. K. (2017). Population genetic structure of Grauer’s Swamp Warbler Bradypterus graueri, an Albertine Rift endemic. Ibis 159, 415–429. doi: 10.1111/ibi.12453
Kaneko, T., Maita, H., Hirakawa, H., Uchiike, N., Minamisawa, K., Watanabe, A., et al. (2011). Complete genome sequence of the soybean symbiont Bradyrhizobium japonicum strain USDA6T. Genes 2, 763–787. doi: 10.3390/genes2040763
Kasangaki, A., Bitariho, R., Shaw, P., Robbins, M., and McNeilage, A. (2012). “7. Long-term ecological and socio-economic changes in and around Bwindi Impenetrable National Park, south-western Uganda,” in The Ecological Impact of Long-term Changes in Africa’s Rift Valley, ed. A. J. Plumptre (Hauppauge, NY: Nova Science Publishers), 106–124.
Kasangaki, A., Chapman, L. J., and Balirwa, J. (2008). Land use and the ecology of benthic macroinvertebrate assemblages of high-altitude rainforest streams in Uganda. Freshw. Biol. 53, 681–697. doi: 10.1111/j.1365-2427.2007.01925.x
Kasangaki, A., Kityo, R., and Kerbis, J. (2003). Diversity of rodents and shrews along an elevational gradient in Bwindi Impenetrable National Park, south-western Uganda. Afr. J. Ecol. 41, 115–123. doi: 10.1046/j.1365-2028.2003.00383.x
Katoh, K., and Standley, D. M. (2013). MAFFT multiple sequence alignment software version 7: improvements in performance and usability. Mol. Biol. Evol. 30, 772–780. doi: 10.1093/molbev/mst010
Kingdon, J. (1973). Endemic mammals and birds of western Uganda: measuring Uganda’s biological wealth and a plea for supra-economic values. Uganda J. 37, 1–8.
Kircher, M., Sawyer, S., and Meyer, M. (2012). Double indexing overcomes inaccuracies in multiplex sequencing on the Illumina platform. Nucleic Acid Res. 40:e3. doi: 10.1093/nar/gkr771
Kisand, V., Talas, L., Kisand, A., Stivrins, N., Reitalu, T., Alliksaar, T., et al. (2018). From microbial eukaryotes to metazoan vertebrates: wide spectrum paleo-diversity in sedimentary ancient DNA over the last ˜14,500 years. Geobiology 16, 628–639. doi: 10.1111/gbi.12307
Kistler, L., Ware, R., Smith, O., Collins, M., and Allaby, R. G. (2017). A new model for ancient DNA decay based on paleogenomic meta-analysis. Nucleic Acids Res. 45, 6310–6320. doi: 10.1093/nar/gkx361
Li, H. (2013). Aligning Sequence Reads, Clone Sequences and Assembly Contigs Using BWA-MEM. Cambridge, MA: MIT.
Li, H., Handsaker, B., Wysoker, A., Fennell, T., Ruan, J., Homer, N., et al. (2009). The Sequence Alignment/Map format and SAMtools. Bioinformatics 25, 2078–2079. doi: 10.1093/bioinformatics/btp352
Li, W., and Godzik, A. (2006). Cd-hit: a fast program for clustering and comparing large sets of protein or nucleotide sequences. Bioinformatics 22, 1658–1659. doi: 10.1093/bioinformatics/btl158
Lindahl, T. (1993). Instability and decay of the primary structure of DNA. Nature 362, 709–715. doi: 10.1038/362709a0
Lindahl, T., and Nyberg, B. (1972). Rate of depurination of native deoxyribonucleic acid. Biochemistry 11, 3610–3618. doi: 10.1021/bi00769a018
Lorenz, M. G., and Wackernagel, W. (1987). Adsoprtion of DNA to sand and variable degradation rates of adsorbed DNA. Appl. Environ. Microbiol. 53, 2948–2952. doi: 10.1128/aem.53.12.2948-2952.1987
Lorenz, M. G., and Wackernagel, W. (1994). Bacterial gene transfer by natural genetic transformation in the environment. Microbiol. Rev. 58, 563–602. doi: 10.1128/mmbr.58.3.563-602.1994
Magoč, T., and Salzberg, S. L. (2011). FLASH: fast length adjustment of short reads to improve genome assemblies. Bioinformatics 27, 2957–2963. doi: 10.1093/bioinformatics/btr507
Marchant, R., and Taylor, D. (1998). Dynamics of montane forest in central Africa during the late Holocene: a pollen-based record from western Uganda. Holocene 8, 375–381. doi: 10.1191/095968398672993971
Marchant, R., Taylor, D., and Hamilton, A. (1997). Late Pleistocene and Holocene history at Mubwindi Swamp, southwest Uganda. Quat. Res. 47, 316–328. doi: 10.1006/qres.1997.1887
Marotz, C., Amir, A., Humphrey, G., Gaffney, J., Gogul, G., and Knight, R. (2017). DNA extraction for streamlined metagenomics of diverse environmental samples. BioTechniques 62, 290–293. doi: 10.2144/000114559
Mason, C. F., and Standen, V. (1983). “Aspects of secondary production,” in Ecosystems of the World. 4A Mires: Swamp, Bog, Fen and Moor General Studies, ed. A. J. P. Gore (Amsterdam: Elsevier), 367–382.
Mergeay, J., Vanoverbeke, J., Verschuren, D., and de Meester, L. (2007). Extinction, recolonization, and dispersal through time in a planktonic crustacean. Ecology 88, 3032–3043. doi: 10.1890/06-1538.1
Meyer, M., and Kircher, M. (2010). Illumina sequencing library preparation for highly multiplexed target capture and sequencing. Cold Spring Harb. Protoc. 2010:pdb.prot5448. doi: 10.1101/pdb.prot5448
Mittelbach, G. G., Schemske, D. W., Cornell, H. V., Allen, A. P., Brown, J. M., Bush, M. B., et al. (2007). Evolution and the latitudinal diversity gradient: speciation, extinction and biogeography. Ecol. Lett. 10, 315–331. doi: 10.1111/j.1461-0248.2007.01020.x
Mondol, S., Moltke, I., Hart, J., Keigwin, M., Brown, L., Stephens, M., et al. (2015). New evidence for hybrid zones of forest and savanna elephants in Central and West Africa. Mol. Ecol. 24, 6134–6147. doi: 10.1111/mec.13472
Mugerwa, B., Sheil, D., Ssekiranda, P., van Heist, M., and Ezuma, P. (2013). A camera trap assessment of terrestrial vertebrates in Bwindi Impenetrable National Park, Uganda. Afr. J. Ecol. 51, 21–31. doi: 10.1111/aje.12004
Murphy, J., and Riley, J. P. (1962). A modified single solution method for the determination of phosphate in natural waters. Anal. Chim. Acta 27, 31–36. doi: 10.1016/S0003-2670(00)88444-5
Nkurunungi, J. B., Ganas, J., Robbins, M. M., and Stanford, C. B. (2004). A comparison of two mountain gorilla habitats in Bwindi Impenetrable National Park, Uganda. Afr. J. Ecol. 42, 289–297. doi: 10.1111/j.1365-2028.2004.00523.x
Ogram, A., Sayler, G. S., Gustin, D., and Lewis, R. J. (1988). DNA adsorption to soils and sediments. Environ. Sci. Technol. 22, 982–984. doi: 10.1021/es00173a020
Olupot, P., and Plumptre, A. (2010). Conservation research in Uganda’s Forests. Hauppauge, NY: Nova Science Publishers, Inc.
Pääbo, S., Poinar, H., Serre, D., Jaenicke-Després, V., Hebler, J., Rohland, N., et al. (2004). Genetic analyses from ancient DNA. Annu. Rev. Genet. 38, 645–679. doi: 10.1146/annurev.genet.37.110801.143214
Parducci, L., Alsos, I. G., Unneberg, P., Pedersen, M. W., Han, L., Lammers, Y., et al. (2019). Shotgun environmental DNA, pollen, and macrofossil analysis of Lateglacial lake sediments from southern Sweden. Front. Ecol. Evol. 7:189. doi: 10.3389/fevo.2019.00189
Parducci, L., Bennett, K. D., Ficetola, G. F., Alsos, I. G., Suyama, Y., Wood, J. R., et al. (2017). Ancient plant DNA in lake sediments. New Phytol. 214, 924–942. doi: 10.1111/nph.14470
Parducci, L., Nota, K., and Wood, J. (2018). “Reconstructing past vegetation communities using ancient DNA from lake sediments,” in Paleogenomics. Population Genomics, eds C. Lindqvist and O. Rajora (Cham: Springer), doi: 10.1007/13836_2018_38
Parducci, L., Suyama, Y., Lascoux, M., and Bennett, K. D. (2005). Ancient DNA from pollen: a genetic record of population history in Scots pine. Mol. Ecol. 14, 2873–2882. doi: 10.1111/j.1365-294X.2005.02644.x
Pedersen, M. W., Overballe-Petersen, S., Ermini, L., der Sarkissian, C., Haile, J., Hellstrom, M., et al. (2015). Ancient and modern environmental DNA. Philos. Trans. R. Soc. B. 370:20130383.
Pedersen, M. W., Ruter, A., Schweger, C., Friebe, H., Staff, R. A., Kjeldsen, K. K., et al. (2016). Postglacial viability and colonization in North America’s ice-free corridor. Nature 537, 45–49. doi: 10.1038/nature19085
Pennington, R. T., Hughes, M., and Moonlight, P. W. (2015). The origins of tropical rainforest hyperdiversity. Trends Plant Sci. 20, 693–695. doi: 10.1016/j.tplants.2015.10.005
Peterson, B. G., Carl, P., Boudt, K., Bennett, R., Ulrich, J., Zivot, E., et al. (2019). PerformanceAnalytics: Econometric Tools for Performance and Risk Analysis. R package version 1.5.3. Available at: https://CRAN.R-project.org/package=PerformanceAnalytics (accessed October 18, 2019).
Plumptre, A. J., Davenport, T. R. B., Behangana, M., Kityo, R., Eilu, G., Ssegawa, P., et al. (2007). The biodiversity of the Albertine Rift. Biol. Conserv. 134, 178–194. doi: 10.1016/j.biocon.2006.08.021
Price, M. N., Dehal, P. S., and Arkin, A. P. (2010). FastTree 2—Approximately maximum-likelihood trees for large alignments. PLoS One 5:e9490. doi: 10.1371/journal.pone.0009490
Pruesse, E., Quast, C., Knittel, K., Fuchs, B. M., Ludwig, W., Peplies, J., et al. (2007). SILVA: a comprehensive online resource for quality checked and aligned ribosomal RNA sequence data compatible with ARB. Nucleic Acids Res. 35, 7188–7196. doi: 10.1093/nar/gkm864
Prüfer, K., Stenzel, U., Hofreiter, M., Pääbo, S., Kelso, J., and Green, R. E. (2010). Computational challenges in the analysis of ancient DNA. Genome Biol. 11:R47. doi: 10.1186/gb-2010-11-5-r47
R Core Team (2018). R: A Language and Environment for Statistical Computing. Vienna: R Foundation for Statistical Computing.
Rawlence, N. J., Lowe, D. J., Wood, J. R., Young, J. M., Churchman, G. J., Huang, Y.-T., et al. (2014). Using palaeoenvironmental DNA to reconstruct past environments: progress and prospects. J. Quat. Sci. 29, 610–626. doi: 10.1002/jqs.2740
Reimer, P. J., Bard, E., Baylis, A., Beck, J. W., Blackwell, P. G., Bronk Ramsey, C., et al. (2013). IntCal13 and Marine13 radiocarbon age calibration curves 0–50,000 years cal BP. Radiocarbon 55, 1869–1887. doi: 10.2458/azu_js_rc.55.16947
Rognes, T., Flouri, T., Nichols, B., Quince, C., and Mahé, F. (2016). VSEARCH: a versatile open source tool for metagenomics. PeerJ 4:e2584. doi: 10.7717/peerj.2584
Rohland, N., Glocke, I., Aximu-Petri, A., and Meyer, M. (2018). Extraction of highly degraded DNA from ancient bones, teeth and sediments for high-throughput sequencing. Nat. Protoc. 2, 1756–1762. doi: 10.1038/nprot.2007.247
Rohland, N., and Hofreiter, M. (2007). Comparison and optimization of ancient DNA extraction. BioTechniques 42, 343–352. doi: 10.2144/000112383
Rohland, N., and Reich, D. (2012). Cost-effective, high-throughput DNA sequencing libraries for multiplexed target capture. Genome Res. 22, 939–946. doi: 10.1101/gr.128124.111
Rothman, J. M., Nkurunungi, J. B., Shannon, B. F., and Bryer, M. A. (2014). “High altitude diets: implications for the feeding and nutritional ecology of mountain gorillas,” in High Altitude Primates, eds N. B. Grow, S. Gursky-Doyen, and A. Krzton (New York, NY: Springer), 247–264. doi: 10.1007/978-1-4614-8175-1_14
Roy, J., Arandjelovic, M., Bradley, B. J., Guschanski, K., Stephens, C. R., Bucknell, D., et al. (2014). Recent divergences and size decreases of eastern gorilla populations. Biol. Lett. 10:20140811. doi: 10.1098/rsbl.2014.0811
Sawyer, S., Krause, J., Guschanski, K., Savolainen, V., and Pääbo, S. (2012). Temporal patterns of nucleotide misincorporations and DNA fragmentation in ancient DNA. PLoS One 7:e34131. doi: 10.1371/journal.pone.0034131
Segata, N., Waldron, L., Ballarini, A., Narasimhan, V., Jousson, O., and Huttenhower, C. (2012). Metagenomic microbial community profiling using unique clade-specific marker genes. Nat. Methods 9, 811–814. doi: 10.1038/nmeth.2066
Seymour, M., Durance, I., Cosby, B. J., Ransom-Jones, E., Deiner, K., Ormerod, S. J., et al. (2018). Acidity promotes degradation of multi-species environmental DNA in lotic mesocosms. Commun. Biol. 1:4.
Sherratt, E., del Rosario Castañeda, M., Garwood, R. J., Mahler, D. L., Sanger, T. J., Herrel, A., et al. (2015). Amber fossils demonstrate deep-time stability of Caribbean lizard communities. Proc. Natl. Acad. Sci. U.S.A. 112, 9961–9966. doi: 10.1073/pnas.1506516112
Slon, V., Hopfe, C., Weiß, C. L., Mafessoni, F., de la Rasilla, M., Lalueza-Fox, C., et al. (2017). Neandertal and Denisovan DNA from Pleistocene sediments. Science 356, 605–608. doi: 10.1126/science.aam9695
Speight, M. C. D., and Blackith, R. E. (1983). “The animals,” in Ecosystems of the World. 4A Mires: Swamp, Bog, Fen and Moor General Studies, ed. A. J. P. Gore (Amsterdam: Elsevier), 349–365.
Stanford, C. B., and Nkurunungi, J. B. (2003). Behavioral ecology of sympatric chimpanzees and gorillas in Bwindi Impenetrable National Park, Uganda: diet. Int. J. Primatol. 24, 901–918. doi: 10.1023/A:1024689008159
Starke, R., and Morais, D. (2019). Gene copy of the 16S rRNA gene cannot outweigh methodological biases of sequencing. bioRxiv [Preprint]. doi: 10.1101/813477
Stevens, G. C. (1989). The latitudinal gradient in geographical range: how so many species coexist in the tropics. Am. Nat. 133, 240–256. doi: 10.1086/284913
Stoof-Leichsenring, K. R., Epp, L. S., Trauth, M. H., and Tiedemann, R. (2012). Hidden diversity in diatoms of Kenyan Lake Naivasha: a genetic approach detects temporal variation. Mol. Ecol. 21, 1918–1930. doi: 10.1111/j.1365-294X.2011.05412.x
Strickler, K. M., Fremier, A. K., and Goldberg, C. S. (2015). Quantifying effects of UV-B, temperature, and pH on eDNA degradation in aquatic microcosms. Biol. Conserv. 183, 85–92. doi: 10.1016/j.biocon.2014.11.038
Stuiver, M., and Reimer, P. J. (1993). Extended 14C data base and revised CALIB 3.0 14C age calibration program. Radiocarbon 35, 215–230. doi: 10.1017/S0033822200013904
Thompson, L. R., Sanders, J. G., McDonald, D., Amir, A., Ladau, J., Locey, K. J., et al. (2017). A communal catalogue reveals Earth’s multiscale microbial diversity. Nature 551, 457–463. doi: 10.1038/nature24621
Thomsen, P. F., and Willerslev, E. (2015). Environmental DNA – an emerging tool in conservation for monitoring past and present biodiversity. Biol. Conserv. 183, 4–18. doi: 10.1016/j.biocon.2014.11.019
Truong, D. T., Franzosa, E. A., Tickle, T. L., Scholz, M., Weingart, G., Pasolli, E., et al. (2015). MetaPhlAn2 for enhanced metagenomic taxonomic profiling. Nat. Methods 12, 902–903. doi: 10.1038/nmeth.3589
Vågene, Å. J., Herbig, A., Campana, M. G., Robles García, N. M., Warinner, C., Sabin, S., et al. (2018). Salmonella enterica genomes from victims of a major sixteenth-century epidemic in Mexico. Nat. Ecol. Evol. 2, 520–538. doi: 10.1038/s41559-017-0446-6
Vázquez-Baeza, Y., Gonzalez, A., Smarr, L., McDonald, D., Morton, J. T., Navas-Molina, J. A., et al. (2017). Bringing the dynamic microbiome to life with animations. Cell Host Microbe 21, 7–10. doi: 10.1016/j.chom.2016.12.009
Vázquez-Baeza, Y., Pirrung, M., Gonzalez, A., and Knight, R. (2013). EMPeror: a tool for visualizing high-throughput microbial community data. Gigascience 2:16. doi: 10.1186/2047-217X-2-16
Vuillemin, A., Horn, F., Alawi, M., Henny, C., Wagner, D., Crowe, S. A., et al. (2017). Preservation and significance of extracellular DNA in ferruginous sediments from late Towuti, Indonesia. Front. Microbiol. 8:1440. doi: 10.3389/fmicb.2017.01440
Wanek, J., and Rühli, F. (2016). Risks to fragmented DNA in dry, wet, and frozen states from computed tomography: a comparative theoretical study. Radiat. Environ. Biophys. 55, 229–241. doi: 10.1007/s00411-016-0637-6
Wanek, J., Speller, R., and Rühli, F. (2013). Direct action of radiation on mummified cells: modeling of computed tomography by Monte Carlo algorithms. Radiat. Environ. Biophys. 52, 397–410. doi: 10.1007/s00411-013-0471-z
Willerslev, E., Davison, J., Moora, M., Zobel, M., Coissac, E., Edwards, M. E., et al. (2014). Fifty thousand years of Arctic vegetation and megafaunal diet. Nature 506, 47–51. doi: 10.1038/nature12921
Willerslev, E., Hansen, A. J., Binladen, J., Brand, T. B., Gilbert, M. T. P., Shapiro, B., et al. (2003). Diverse plant and animal genetic records from Holocene and Pleistocene sediments. Science 300, 791–795. doi: 10.1126/science.1084114
Wilson, E. O. (1985). Invasion and extinction in the West Indian ant fauna: evidence from the Dominican amber. Science 229, 265–267. doi: 10.1126/science.229.4710.265
Wing, S. L., Herrera, F., Jaramillo, C. A., Gómez-Navarro, C., Wilf, P., and Labandeira, C. C. (2009). Late Paleocene fossils from the Cerrejón Formation, Colombia, are the earliest record of Neotropical rainforest. Proc. Natl. Acad. Sci. U.S.A. 106, 18627–18632. doi: 10.1073/pnas.0905130106
Wright, H. E., Mann, D. H., and Glaser, P. H. (1984). Piston corers for peat and lake sediments. Ecology 65, 657–659. doi: 10.2307/1941430
Ye, S. H., Siddle, K. J., Park, D. J., and Sabeti, P. C. (2019). Benchmarking metagenomics tools for taxonomic classification. Cell 178, 779–794. doi: 10.1016/j.cell.2019.07.010
Yoccoz, N. G., Bråthen, K. A., Gielly, L., Haile, J., Edwards, M. E., Goslar, T., et al. (2012). DNA from soil mirrors plant taxonomic and growth from diversity. Mol. Ecol. 21, 3647–3655. doi: 10.1111/j.1365-294X.2012.05545.x
Keywords: sedimentary ancient DNA, tropical biodiversity, DNA preservation, sediment, tropical swamp, shotgun sequencing, metagenomic analysis
Citation: Dommain R, Andama M, McDonough MM, Prado NA, Goldhammer T, Potts R, Maldonado JE, Nkurunungi JB and Campana MG (2020) The Challenges of Reconstructing Tropical Biodiversity With Sedimentary Ancient DNA: A 2200-Year-Long Metagenomic Record From Bwindi Impenetrable Forest, Uganda. Front. Ecol. Evol. 8:218. doi: 10.3389/fevo.2020.00218
Received: 18 November 2019; Accepted: 12 June 2020;
Published: 10 July 2020.
Edited by:
Nathan Wales, University of York, United KingdomReviewed by:
Mikkel Winther Pedersen, University of Cambridge, United KingdomCharline Giguet-Covex, UMR5204 Environnements, DYnamiques et TErritoires de la Montagne (EDYTEM), France
Copyright © 2020 Dommain, Andama, McDonough, Prado, Goldhammer and Nkurunungi. At least a portion of this work is authored by Michael G. Campana, Richard Potts and Jesús E. Maldonado on behalf of the U.S. Government and, as regards Drs. Campana, Potts and Maldonado and the U.S. Government are not subject to copyright protection in the United States. Foreign and other copyrights may apply. This is an open-access article distributed under the terms of the Creative Commons Attribution License (CC BY). The use, distribution or reproduction in other forums is permitted, provided the original author(s) and the copyright owner(s) are credited and that the original publication in this journal is cited, in accordance with accepted academic practice. No use, distribution or reproduction is permitted which does not comply with these terms.
*Correspondence: René Dommain, ZG9tbWFpbkB1bmktcG90c2RhbS5kZQ==