- 1Department of Radiation Oncology, Memorial Sloan Kettering Cancer Center, New York, NY, United States
- 2Department of Medical Physics, Memorial Sloan Kettering Cancer Center, New York, NY, United States
- 3Department of Laboratory Medicine, Memorial Sloan Kettering Cancer Center, New York, NY, United States
Background: To investigate the impact of alpha-2-macroglobulin (A2M), a suspected intrinsic radioprotectant, on radiation pneumonitis and esophagitis using multifactorial predictive models.
Materials and Methods: Baseline A2M levels were obtained for 258 patients prior to thoracic radiotherapy (RT). Dose-volume characteristics were extracted from treatment plans. Spearman's correlation (Rs) test was used to correlate clinical and dosimetric variables with toxicities. Toxicity prediction models were built using least absolute shrinkage and selection operator (LASSO) logistic regression on 1,000 bootstrapped datasets.
Results: Grade ≥2 esophagitis and pneumonitis developed in 61 (23.6%) and 36 (14.0%) patients, respectively. The median A2M level was 191 mg/dL (range: 94–511). Never/former/current smoker status was 47 (18.2%)/179 (69.4%)/32 (12.4%). We found a significant negative univariate correlation between baseline A2M levels and esophagitis (Rs = −0.18/p = 0.003) and between A2M and smoking status (Rs = 0.13/p = 0.04). Further significant parameters for grade ≥2 esophagitis included age (Rs = −0.32/p < 0.0001), chemotherapy use (Rs = 0.56/p < 0.0001), dose per fraction (Rs = −0.57/p < 0.0001), total dose (Rs = 0.35/p < 0.0001), and several other dosimetric variables with Rs > 0.5 (p < 0.0001). The only significant non-dosimetric parameter for grade ≥2 pneumonitis was sex (Rs = −0.32/p = 0.037) with higher risk for women. For pneumonitis D15 (lung) (Rs = 0.19/p = 0.006) and D45 (heart) (Rs = 0.16/p = 0.016) had the highest correlation. LASSO models applied on the validation data were statistically significant and resulted in areas under the receiver operating characteristic curve of 0.84 (esophagitis) and 0.78 (pneumonitis). Multivariate predictive models did not require A2M to reach maximum predictive power.
Conclusion: This is the first study showing a likely association of higher baseline A2M values with lower risk of radiation esophagitis and with smoking status. However, the baseline A2M level was not a significant risk factor for radiation pneumonitis.
Introduction
Advances in radiation technology like intensity modulated radiation therapy (IMRT) and image guided RT (IGRT) have facilitated improved sparing of healthy surrounding tissues and organs. Nonetheless, radiation pneumonitis and esophagitis remain the most common dose-limiting toxicities in thoracic RT (1–6). Concurrent chemoradiation significantly increases the risk of developing pneumonitis or esophagitis compared to radiation alone (7, 8).
The reported incidence of pneumonitis after definitive thoracic RT ranges from 10 to 20%, although figures can vary greatly (3, 9–13). This is partly because pneumonitis remains a clinical diagnosis; there are no biomarkers or radiological findings that unequivocally confirm its presence. Medical intervention is required for patients with grade two or higher radiation pneumonitis, and severe cases can lead to fatal outcomes [Common Terminology Criteria for Adverse Events (CTCAE) v4.03, Supplementary Material 1]. Significant esophageal toxicity (grade 3–5) occurs in around 4% of patients with sequential chemotherapy and RT and in 18–22% of patients with concurrent chemoradiation (5, 14). Most patients experience mild symptoms like dysphagia and odynophagia while still undergoing radiation. Commonly opioids are used to control symptoms, but in severe cases, tube feeding or surgical intervention can be necessary (Supplementary Material 1).
To reduce dose-limiting toxicity in thoracic radiation, efforts have been made to adhere to normal tissue constraints derived from dose volume correlations with clinical toxicities (15). However, dose volume histograms do not fully predict clinical toxicities, as great interindividual variation remains. Intrinsic predictors of normal tissue radiation response may explain the variation and should be further analyzed.
Radioprotective agents, both natural and synthetic, can present an alternative method to prevent radiation-induced toxicity. Although this has been an active field of research for decades, only two compounds, amifostine and palifermine, are FDA-approved for the use in radiation therapy and neither is commonly used in routine thoracic RT (16–19). Another compound under investigation is alpha-2-Macroglobulin (A2M), a postulated intrinsic radioprotector. Human A2M is a glycoprotein and the largest non-immunoglobulin serum protein. In animal studies, A2M has exhibited radioprotective effects in healthy irradiated tissue. In studies with rats that underwent full body irradiation to 6.7 Gy, rats with endo- or exogenously increased levels of A2M had a higher rate of survival, regained their baseline body weight and lymphocyte count faster, and displayed normal proliferative ability of the liver tissue compared to the control groups with normal A2M levels (20–22). Suggested key mechanisms supporting the potential of A2M as a radioprotector include promoting expression of antioxidant enzymes, inhibiting fibroblast activation thus preventing fibrosis, deactivating pro-inflammatory cytokines, and enhancing DNA and cell repair mechanisms (23). Our previous study in a small cohort showed a correlation of A2M with radiation pneumonitis (9). Smoking can potentially increase A2M levels. However, literature specifically on A2M in smokers remains rare. Some studies confirmed higher A2M levels in smokers compared to non-smokers (24–26).
We investigated whether pre-treatment serum A2M levels are an independent predictive variable for the development of post-radiation toxicity in the lung and esophagus in a large cohort of patients receiving thoracic RT.
Materials and Methods
Patients
Clinical, laboratory, treatment and toxicity data were systematically collected in a series of thoracic RT patients between 2012 and 2016 during standard treatment and follow-up procedures. Patients were treated with either conventionally fractionated RT using 3D conformal RT (3DCRT) or intensity-modulated RT (IMRT), or with stereotactic body RT (SBRT). Patients with any prior thoracic RT were excluded. Serum samples for A2M analysis were collected at baseline prior to fraction #1 of RT. We obtained a retrospective institutional review board waiver to analyze the data. Toxicity data consist of radiation pneumonitis and esophagitis rates graded per CTCAE v4.03. Data were obtained at baseline and at routine follow-up visits every 3 months for the first 2 years.
Alpha-2-Macroglobulin
Serum samples were taken ≤ 30 days prior to RT start, typically at the time of simulation. The mean and standard deviation between A2M measurement date and RT start date were 14 and 6 days, respectively. CLIA (Clinical Laboratory Improvement Amendments) approved A2M testing was performed at Quest Diagnostics Nichols Institute (San Juan Capistrano, CA). A2M levels were given in mg/dL; the normal range was defined as 100–280 mg/dL.
Treatment Plans
For patients treated before 2014, treatment plans were retrieved from our in-house planning system (27). From 2014 onwards, treatments were planned in the Eclipse treatment planning system (Varian Medical Systems, Palo Alto, CA). To analyze dosimetric data, treatment plans were imported to the research platform CERR (Computational Environment for Radiological Research) for computing dosimetric variables (28). Dosimetric variables were extracted from target structures: complete esophagus for esophagitis and “lung minus gross tumor volume (GTV)” and heart for pneumonitis. Before that, plan doses were converted to equivalent dose in 2 Gy fractions (EQD2) with α/β ratio of 10 for esophagus and 3 for lung minus GTV and heart (29). As radiation esophagitis tends to develop acutely during radiotherapy, one more set of dosimetric variables for esophagus was extracted in addition to the planned doses. For these fractional variables (denoted by the prefix “f,” e.g., fmax dose) we divided the dose volume histogram (DVH) bins by the number of treatment days between the start of RT and the end of RT including weekends.
Statistical Methods
Univariate and multivariate analyses were performed to investigate associations between toxicity (esophagitis and pneumonitis) and A2M levels, clinical, and dosimetric variables. Patients were categorized into two groups for each endpoint: non-toxicity (grade <2) and clinically significant toxicity (grade ≥2).
A Wilcoxon rank-sum test was used to find a difference in A2M expression between the two groups. Spearman's correlation (Rs) test was used to assess associations between endpoints, Dx values (minimum dose to the volume with the x% hottest dose in the organ of interest), computed from x = 5% to x = 100% in intervals of 5%, mean dose, max dose, clinical variables, and A2M. For this test CTCAE grades 0–5 were used instead of dichotomized values (grade <2 and grade ≥2).
Multivariate analysis using the least absolute shrinkage and selection operator (LASSO) logistic regression was performed using features with p < 0.1 that resulted from the univariate Spearman's correlation test. To avoid variable instability due to high collinearity, Pearson's correlation test was conducted among all dosimetric variables before the multivariate analysis. A cutoff of Pearson's correlation coefficient >0.75 was used to determine a relatively small group of variables for further LASSO modeling, by selecting a single variable that has the best correlation with the endpoint among a set of correlated variables after hierarchical clustering.
To rigorously verify model validity, the data were split into two groups (training data with 2/3 and validation data with 1/3 of samples). The training and validation data were balanced with cancer subtypes and outcomes. This split was performed separately for pneumonitis and esophagitis. The model building process was carried out using only the training data. Furthermore, to examine the stability of LASSO variable selection, the model building process was conducted using a bootstrapped dataset generated from the training data. Finally, the validation data were tested on the resulting model, quantified by the area under the receiver operating characteristic curve (AUC) as a function of true positive rate (sensitivity) and false positive rate (1-specificity). The final reported results represent the average performance on the validation data for predictive models built using 1,000 bootstrapped datasets.
For statistical analyses, R language (version 3.2.4), MATLAB (version 8.6.0; MathWorks. Natick, MA) and SPSS (version 24; IBM. Armonk, NY) were used.
Results
Patient Characteristics
In total, 258 patients were eligible for analysis. Most patients were former (n = 179, 69.4%) or current smokers (n = 32, 12.4%). One hundred and thirty-four patients (51.9%) underwent chemotherapy in addition to RT and the median total RT dose was 54 Gy (range: 27–74 Gy) for conventional fractionation and 50 Gy (range: 30–70 Gy) for SBRT. The median A2M level was 191 mg/dL (range: 94–511 mg/dL). The median follow-up was 8.9 months (range: 0.2–40.2; calculated from the start of RT). More details are available in Table 1.
Toxicities
Fifty-third patients (20.5%) experienced grade two and eight (3.1%) grade three radiation esophagitis. No grade four or five esophagitis was observed. Median time to development of esophagitis was 0.85 months after the start of RT (range: 0.2–6.47 months). Grade two radiation pneumonitis developed in 26 patients (10.1%), grade three in nine (3.5%) and grade four in one patient (0.4%). No grade five pneumonitis was observed. Median time to development of pneumonitis was 4.7 months after the start of RT (range: 1.3–8.1 months).
Of the patients who developed grade ≥2 esophagitis, 8 (13.1%) were never, 43 (70.5%) former and 10 (16.4%) current smokers whereas in pneumonitis 9 (25%) were never, 24 (66.7%) former and 3 (8.3%) current smokers.
Univariate Analysis
Alpha-2-Macroglobulin
A significant correlation between baseline A2M values and esophagitis was found (Rs = −0.18/p = 0.003). Using a Wilcoxon rank-sum test, we found that patients with grade <2 had significantly higher baseline serum A2M levels than patients with grade ≥2 esophagitis (p = 0.015) as shown in Table 2. No statistically significant difference was found between baseline A2M levels and grade ≥2 pneumonitis (p = 0.84).
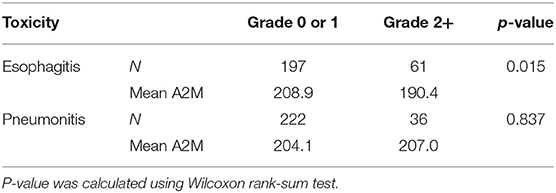
Table 2. Comparison of mean A2M serum levels [mg/dL] between grade <2 and ≥2 esophagitis and pneumonitis.
A trend between smoking status and A2M levels was observed. Current smokers had higher levels (217.3 mg/dl) compared to former (207.3 mg/dl) and never smokers (185.4 mg/dl), and former smokers had higher levels compared to never smokers. The A2M level had a significant correlation with a status of former/current smoker when compared to never smokers (Rs = 0.13/p = 0.04).
Clinical Factors
Among standard clinical variables, the following variables showed significant correlations with grade ≥2 esophagitis: age (Rs = −0.32/p < 0.0001), fraction number (Rs = 0.64/p < 0.0001), treatment days (Rs = 0.60/p < 0.0001), chemotherapy use (Rs = 0.56/p < 0.0001), dose per fraction (Rs = −0.57/p < 0.0001), and total dose (Rs = 0.35/p < 0.0001), whereas for grade ≥2 pneumonitis, the only significant clinical variable was sex (Rs = −0.32/p = 0.037) with a higher risk for women.
Dosimetric Factors
Spearman's correlation test between dosimetric variables in esophagus and esophagitis showed that all variables had Rs > 0.60 (p < 0.0001) as shown in Figure 1A. For the fractional dose, fD40 was the highest correlated variable (Rs = 0.58/p < 0.0001) as shown in Figure 1B.
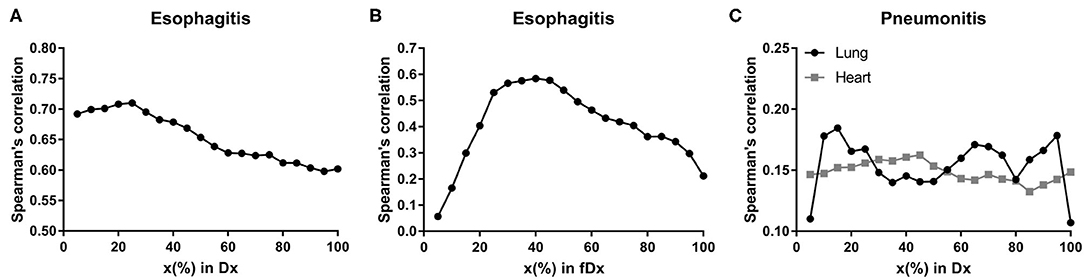
Figure 1. Spearman's correlation coefficients. Spearman's correlation coefficients between radiation-induced injuries (≥ grade 2) and Dx in esophagus for (A) esophagitis, fDx in esophagus for (B) esophagitis, and Dx in lung and heart for (C) pneumonitis.
D15 (Rs = 0.19/p = 0.006) in lung and D45 (Rs = 0.16/p = 0.016) in heart were assessed as the highest correlated variables with pneumonitis for each organ (Figure 1C). Maximum dose in heart was also significantly correlated with Rs = 0.14 (p = 0.043).
Multivariate Analysis and Validation Testing
Hierarchical clustering coupled with Pearson's correlation test using training data was performed on dosimetric variables of each organ to measure variable similarity. Many dosimetric variables were highly correlated (Supplementary Material 2). Using a threshold of 0.75 in Pearson's correlation the variable with the highest Rs value for the endpoint among the variables in each cluster was selected. Clinical variables with p < 0.1 in the univariate analysis and dosimetric variables left after the clustering test were used in the LASSO logistic regression: D25, D40, D50, D65, D85, fD10, fD25, fD35 in esophagus, age, total dose, and A2M for esophagitis; D10, D15, D65, D95 in lung, D20, D45, max dose in heart and sex for pneumonitis. For both endpoints, treatment days, SBRT (yes/no) and chemotherapy (yes/no) were used. Two variables including dose per fraction and number of fractions were excluded due to their high correlation with the number of treatment days.
LASSO logistic regression models were trained using bootstrapped datasets generated from training data and were tested on the validation data, resulting in an average AUC of 0.84 (standard deviation [SD] = 0.03) and 0.78 (SD = 0.06) for esophagitis and pneumonitis, respectively. Additional modeling was performed for esophagitis without A2M resulting in the same average AUC (0.84). This appears to be due to more significant dosimetric and clinical variables used in the modeling. To assess the importance of features, the frequency of occurrence of each feature during the model building process was counted (Figure 2). For the esophagitis model, chemotherapy and treatment days were most frequently selected with 770 and 758 times from 1,000 different models, respectively. It is worth noting that A2M was selected 610 times, implying its likely association with esophagitis. For the pneumonitis model, D65 in lung and max dose in heart were most frequently selected with 865 and 798 times, respectively. Patients were sorted based on predicted outcomes on the validation data and grouped into six equal bins with one being the lowest risk group and six being the highest risk group. When comparing observed and predicted incidence, we found a high conformity of both endpoints, meaning that the predictive models are highly robust (Figure 3). Final predictive models built using all training data are shown in Table 3. Interestingly, a variable of treatment days (between the start of RT and the end of RT including weekends) was selected in both models.
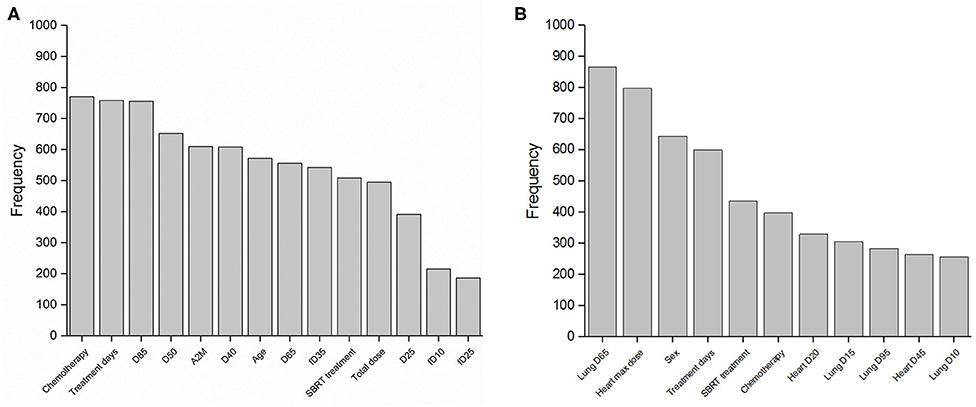
Figure 2. Features in predictive models. Frequency of occurrence of each feature used in 1,000 predictive models for (A) esophagitis and (B) pneumonitis.
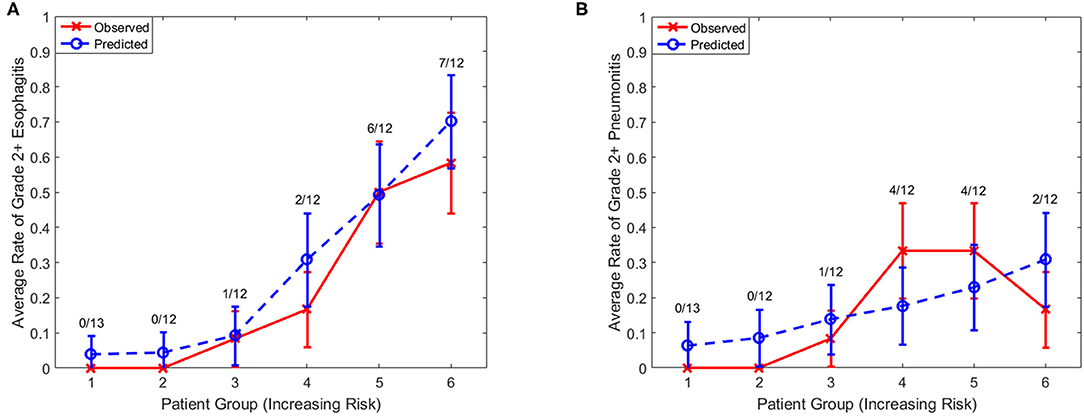
Figure 3. Observed and predicted incidence. Comparison of observed and predicted incidence on validation data (1/3 of samples) for (A) esophagitis and (B) pneumonitis. Numerator, number of events in each bin; Denominator, number of samples in each bin.
In addition, the frequency of occurrence of each pair of features used in the LASSO logistic regression model was investigated (Figure 4), which provides the information of interaction effects of features in the predictive model.
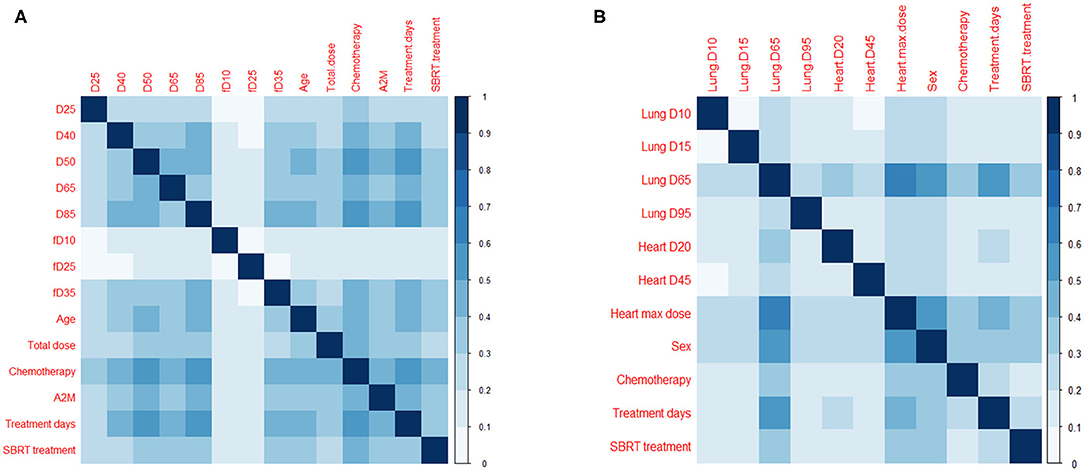
Figure 4. Pairs of features in predictive models. Frequency of occurrence of a pair of features (divided by 1,000) used in 1,000 predictive models for (A) esophagitis and (B) pneumonitis.
Discussion
Taking into account the multifactorial etiology of radiation toxicity (30), it is essential to look at different predictive factors in the development of lung and esophageal injury after RT. Dosimetric parameters are most commonly included in predictive models but biological and genetic determinants are also under investigation (9, 11, 30–37). In our analysis, we focused on dose-volume metrics, age, chemotherapy, and other clinical variables in addition to the intrinsic radioprotectant A2M.
As we identified in our correlative analysis, baseline serum A2M levels appear to be influenced by patients' smoking status. Former and current smokers displayed higher A2M values than patients that had never smoked. Active and former smoking has been associated with lower rates of grade ≥3 radiation pneumonitis compared to never smokers in patients with NSCLC after 3DCRT or IMRT (38). The effect of smoking on the immune system has been studied extensively. Paradoxically, smoking results in immunosuppression as well as aggravated autoimmunity. Altered levels of inflammatory cytokines like TNF-α, IFN-γ, IL-1β, IL-6, IL-8, IL-10, and others have been reported in healthy smokers (39–42). A possible explanation for the connection between active smoking and a lower risk for esophagitis or pneumonitis is that long-term cigarette smoking leads to an increased immune response in the lung and surrounding tissues due to the damage it inflicts on the lung parenchyma. Although the mechanisms resulting in normal tissue injury after RT are still under investigation, the release of reactive oxygen species (ROS) as well as proinflammatory and profibrotic cytokines is thought to have a central role in the process (30). Higher baseline levels of acute-phase proteins like A2M may have a protective effect on the irradiated tissue by binding proinflammatory and profibrotic cytokines, thus reducing the acute cytokine toxicity, and inducing an upregulation of antioxidant enzymes like manganese superoxide dismutase (MnSOD) (23, 30).
Our study suggests that there may be an association between natural pre-treatment baseline levels of the intrinsic radioprotectant serum A2M in patients with thoracic malignancies and an increased risk of developing radiation esophagitis. This association reached univariate statistical significance for esophagitis, but not for the pneumonitis endpoint. This finding indicates that higher levels of A2M may have a protective effect in patients undergoing thoracic RT. The high selection frequency of A2M in the model building process confirms our primary univariate analyses implying a likely correlation of A2M levels with esophagitis rates. Factors that have repeatedly shown significant correlation with esophagitis include V40–V60 (6, 43–46) (Vx: percentage volume receiving at least x Gy), mean esophageal dose (47–49), as well as sequential and especially concurrent chemoradiation in comparison to RT alone (5, 6, 50–53).
For pneumonitis, we validated the correlation with radiation dose received by the heart. Different lung dose volumes (V5–V40 and mean dose in lung) have been found to predict the development of pneumonitis (7, 10, 54). In addition, the dose received by the heart during thoracic radiation seems to be an accurate predictor (55, 56). The best fitting predictive model reported by Huang et al. included D10 (heart), D35 (lung) and max dose (lung), and had an AUC of 0.72 (55). Although the ideal dosimetric variable(s) for predicting pneumonitis across all patient subgroups may not yet be known, it is evident that heart doses are an essential part of any model built for this cause. Though we could not confirm the impact of A2M on pneumonitis with our data, a correlation between them has been previously described (9, 56). We may have been limited by the lower incidence of grade ≥2 pneumonitis (14.0%) and the low rate of current smokers in our patient cohort. In the previously published study on A2M and pneumonitis, pneumonitis rates were between 19 and 35%. The variable of sex was found to be correlated with pneumonitis in univariate analysis and had the third-highest frequency in 1,000 model runs (Figure 2B), consistent with another study (57). However, its role as a risk factor for pneumonitis is controversial (58).
While all patients had A2M collected within 30 days prior to the start of RT and toxicity data were systematically prospectively graded per our clinical standard, caution is warranted regarding the interpretation of these results. In particular, including a factor like chemotherapy in predictive models should be considered carefully as different regimens, doses and timings, depending on the patient population, make it a very heterogeneous variable. Similarly, the patient cohort we studied was diverse regarding diagnosis and treatment. Although requirements for eligibility included no prior RT, patients underwent different modes of RT (3DCRT/SBRT/IMRT) which may have an impact on the toxicity profile. Furthermore, smoking status as a variable was not evenly distributed. Given its proposed link to serum A2M levels, this could be a cause for certain discrepancies in our results. A2M levels in humans reported in previous studies range on average between 100 and 450 mg/dL with a mean of around 215 mg/dL. Age and gender are known to influence this value with females generally showing around 20% higher levels than same-aged males (59, 60). Given the lack of data thus far concerning other factors that may influence intrinsic A2M levels, further analyses with serum levels recorded immediately prior to and while receiving RT are necessary.
In summary, the analysis of our institutional dataset has produced predictive models for both esophagitis and pneumonitis. Although the addition of A2M did not increase the predictive power of multivariate predictive models, this is the first report on the possible association of higher levels of A2M with a lower risk of radiation esophagitis and with smoking, warranting further investigation and independent validation.
Data Availability Statement
The datasets generated for this study are available on request to the corresponding author.
Ethics Statement
The studies involving human participants were reviewed and approved by Memorial Sloan Kettering Cancer Center. The patients/participants provided their written informed consent to participate in this study.
Author Contributions
JD, JO, AR, DR, AW, and EY contributed to conception and design of the study. MF, EG, AR, and DR acquired and organized the data. AA, JD, JO, HP, MT, and JY analyzed the data and performed the statistical analysis. JO, AR, and DR drafted the manuscript. All authors contributed to the critical revision and approved the submission of the manuscript.
Funding
This research was funded in part through the NIH/NCI Cancer Center Support Grant Nos. P30 CA008748 and R21 CA234752.
Conflict of Interest
JD has research contracts with Varian Medical Systems and Philips. AR has grants from Varian Medical Systems, Boehringer Ingelheim, Pfizer, Astrazeneca, Merck, and personal fees from Astrazeneca, Merck, Cybrexa, MoreHealth, and ResearchtoPractice as well as non-financial support from Philips/Elekta. AW has a grant from CivaTech Oncology, personal fees from AstraZeneca, and a travel grant from AlphaTau Medical.
The remaining authors declare that the research was conducted in the absence of any commercial or financial relationships that could be construed as a potential conflict of interest.
The handling editor declared a past collaboration with two of the authors MT and JD.
Supplementary Material
The Supplementary Material for this article can be found online at: https://www.frontiersin.org/articles/10.3389/fonc.2020.01395/full#supplementary-material
References
1. Marks L, Yu X, Vujaskovic Z, Small W Jr, Folz R, Anscher M. Radiation-induced lung injury. Semin Radiat Oncol. (2003) 13:333–45. doi: 10.1016/S1053-4296(03)00034-1
2. Bradley J, Movsas B. Radiation esophagitis: predictive factors and preventive strategies. Semin Radiat Oncol. (2004) 14:280–6. doi: 10.1016/j.semradonc.2004.06.003
3. Kocak Z, Evans ES, Zhou SM, Miller KL, Folz RJ, Shafman TD, et al. Challenges in defining radiation pneumonitis in patients with lung cancer. Int J Radiat Oncol Biol Phys. (2005) 62:635–8. doi: 10.1016/j.ijrobp.2004.12.023
4. Mehta V. Radiation pneumonitis and pulmonary fibrosis in non-small-cell lung cancer: pulmonary function, prediction, and prevention. Int J Radiat Oncol Biol Phys. (2005) 63:5–24. doi: 10.1016/j.ijrobp.2005.03.047
5. Werner-Wasik M, Paulus R, Curran WJ Jr, Byhardt R. Acute esophagitis and late lung toxicity in concurrent chemoradiotherapy trials in patients with locally advanced non-small-cell lung cancer: analysis of the radiation therapy oncology group (RTOG) database. Clin Lung Cancer. (2011) 12:245–51. doi: 10.1016/j.cllc.2011.03.026
6. Kwint M, Uyterlinde W, Nijkamp J, Chen C, Bois J de, Sonke JJ, et al. Acute esophagus toxicity in lung cancer patients after intensity modulated radiation therapy and concurrent chemotherapy. Int J Radiat Oncol Biol Phys. (2012) 84:e223–8. doi: 10.1016/j.ijrobp.2012.03.027
7. Palma DA, Senan S, Tsujino K, Barriger RB, Rengan R, Moreno M, et al. Predicting radiation pneumonitis after chemoradiation therapy for lung cancer: an international individual patient data meta-analysis. Int J Radiat Oncol Biol Phys. (2013) 85:444–50. doi: 10.1016/j.ijrobp.2012.04.043
8. Palma DA, Senan S, Oberije C, Belderbos J, Dios NR de, Bradley JD, et al. Predicting esophagitis after chemoradiation therapy for non-small cell lung cancer: an individual patient data meta-analysis. Int J Radiat Oncol Biol Phys. (2013) 87:690–6. doi: 10.1016/j.ijrobp.2013.07.029
9. Oh JH, Craft JM, Townsend R, Deasy JO, Bradley JD, El Naqa I. A bioinformatics approach for biomarker identification in radiation-induced lung inflammation from limited proteomics data. J Proteome Res. (2011) 10:1406–15. doi: 10.1021/pr101226q
10. Rodrigues G, Lock M, D'souza D, Yu E, van Dyk J. Prediction of radiation pneumonitis by dose - volume histogram parameters in lung cancer–a systematic review. Radiother Oncol. (2004) 71:127–38. doi: 10.1016/j.radonc.2004.02.015
11. Tsujino K, Hirota S, Endo M, Obayashi K, Kotani Y, Satouchi M, et al. Predictive value of dose-volume histogram parameters for predicting radiation pneumonitis after concurrent chemoradiation for lung cancer. Int J Radiat Oncol Biol Phys. (2003) 55:110–5. doi: 10.1016/S0360-3016(02)03807-5
12. Wang JY, Chen KY, Wang JT, Chen JH, Lin JW, Wang HC, et al. Outcome and prognostic factors for patients with non-small-cell lung cancer and severe radiation pneumonitis. Int J Radiat Oncol Biol Phys. (2002) 54:735–41. doi: 10.1016/S0360-3016(02)02994-2
13. Kong F-M, Haken RT, Eisbruch A, Lawrence TS. Non-small cell lung cancer therapy-related pulmonary toxicity: an update on radiation pneumonitis and fibrosis. Semin Oncol. (2005) 32:42–54. doi: 10.1053/j.seminoncol.2005.03.009
14. Auperin A, Le Pechoux C, Rolland E, Curran WJ, Furuse K, Fournel P, et al. Meta-analysis of concomitant versus sequential radiochemotherapy in locally advanced non-small-cell lung cancer. J Clin Oncol. (2010) 28:2181–90. doi: 10.1200/JCO.2009.26.2543
15. Bentzen SM, Constine LS, Deasy JO, Eisbruch A, Jackson A, Marks LB, et al. Quantitative Analyses of Normal Tissue Effects in the Clinic (QUANTEC): an introduction to the scientific issues. Int J Radiat Oncol Biol Phys. (2010) 76:S3–9. doi: 10.1016/j.ijrobp.2009.09.040
16. Movsas B, Scott C, Langer C, Werner-Wasik M, Nicolaou N, Komaki R, et al. Randomized trial of amifostine in locally advanced non–small-cell lung cancer patients receiving chemotherapy and hyperfractionated radiation: radiation therapy oncology group trial 98-01. J Clin Oncol. (2005) 23:2145–54. doi: 10.1200/JCO.2005.07.167
17. Hensley ML, Hagerty KL, Kewalramani T, Green DM, Meropol NJ, Wasserman TH, et al. American Society of Clinical Oncology 2008 clinical practice guideline update: use of chemotherapy and radiation therapy protectants. J Clin Oncol. (2009) 27:127–45. doi: 10.1200/JCO.2008.17.2627
18. Buentzel J, Micke O, Adamietz IA, Monnier A, Glatzel M, de Vries A. Intravenous amifostine during chemoradiotherapy for head-and-neck cancer: a randomized placebo-controlled phase III study. Int J Radiat Oncol Biol Phys. (2006) 64:684–91. doi: 10.1016/j.ijrobp.2005.08.005
19. Spielberger R, Stiff P, Bensinger W, Gentile T, Weisdorf D, Kewalramani T, et al. Palifermin for oral mucositis after intensive therapy for hematologic cancers. N Engl J Med. (2004) 351:2590–8. doi: 10.1056/NEJMoa040125
20. Mihailovic M, Dobric S, Poznanovic G, Petrovic M, Uskokovic A, Arambasic J, et al. The acute-phase protein alpha2-macroglobulin plays an important role in radioprotection in the rat. Shock. (2009) 31:607–14. doi: 10.1097/SHK.0b013e31818bb625
21. Mihailović M, Poznanović G, Grdović N, Vidaković M, Dinić S, Grigorov I, et al. The rat acute-phase protein α 2 -macroglobulin plays a central role in amifostine-mediated radioprotection. J Radiol Prot. (2010) 30:567–83. doi: 10.1088/0952-4746/30/3/011
22. Bogojevic D, Poznanovic G, Grdovic N, Grigorov I, Vidakovic M, Dinic S, et al. Administration of rat acute-phase protein alpha(2)-macroglobulin before total-body irradiation initiates cytoprotective mechanisms in the liver. Radiat Environ Biophys. (2011) 50:167–79. doi: 10.1007/s00411-010-0331-z
23. Chen X, Kong X, Zhang Z, Chen W, Chen J, Li H, et al. Alpha-2-macroglobulin as a radioprotective agent: a review. Chin J Cancer Res. (2014) 26:611–21. doi: 10.3978/j.issn.1000-9604.2014.09.04
24. Tappia PS, Troughton KL, Langley-Evans SC, Grimble RF. Cigarette smoking influences cytokine production and antioxidant defences. Clin Sci. (1995) 88:485–9. doi: 10.1042/cs0880485
25. Suriyaprom K, Harnroongroj T, Namjuntra P, Chantaranipapong Y, Tungtrongchitr R. Effects of tobacco smoking on alpha-2-macroglobulin and some biochemical parameters in Thai males. Southeast Asian J Trop Med Public Health. (2007) 38:918–26.
26. Mocchegiani E, Giacconi R, Costarelli L. Metalloproteases/anti-metalloproteases imbalance in chronic obstructive pulmonary disease: genetic factors and treatment implications. Curr Opin Pulm Med. (2011) 17(Suppl. 1):S11–9. doi: 10.1097/01.mcp.0000410743.98087.12
27. Mohan R, Barest G, Brewster LJ, Chui CS, Kutcher GJ, Laughlin JS, et al. A comprehensive three-dimensional radiation treatment planning system. Int J Radiat Oncol Biol Phys. (1988) 15:481–95. doi: 10.1016/S0360-3016(98)90033-5
28. Deasy JO, Blanco AI, Clark VH. CERR: a computational environment for radiotherapy research. Med Phys. (2003) 30:979–85. doi: 10.1118/1.1568978
29. Duijm M, van der Voort van Zyp NC, Granton PV, van de Vaart P, Mast ME, Oomen-de Hoop E, et al. Predicting high-grade esophagus toxicity after treating central lung tumors with stereotactic radiation therapy using a normal tissue complication probability model. Int J Radiat Oncol Biol Phys. (2020) 106:73–81. doi: 10.1016/j.ijrobp.2019.08.059
30. Anscher MS, Chen L, Rabbani Z, Kang S, Larrier N, Huang H, et al. Recent progress in defining mechanisms and potential targets for prevention of normal tissue injury after radiation therapy. Int J Radiat Oncol Biol Phys. (2005) 62:255–9. doi: 10.1016/j.ijrobp.2005.01.040
31. Zhao L, Pu X, Ye Y, Lu C, Chang JY, Wu X. Association between genetic variants in DNA double-strand break repair pathways and risk of radiation therapy-induced pneumonitis and esophagitis in non-small cell lung cancer. Cancers. (2016) 8:23. doi: 10.3390/cancers8020023
32. Kim KS, Jeon SU, Lee CJ, Kim YE, Bok S, Hong BJ, et al. Radiation-induced esophagitis in vivo and in vitro reveals that epidermal growth factor is a potential candidate for therapeutic intervention strategy. Int J Radiat Oncol Biol Phys. (2016) 95:1032–41. doi: 10.1016/j.ijrobp.2016.02.051
33. Sprung CN, Forrester HB, Siva S, Martin OA. Immunological markers that predict radiation toxicity. Cancer Lett. (2015) 368:191–7. doi: 10.1016/j.canlet.2015.01.045
34. Kerns SL, Kundu S, Oh JH, Singhal SK, Janelsins M, Travis LB, et al. The prediction of radiotherapy toxicity using single nucleotide polymorphism-based models: a step toward prevention. Semin Radiat Oncol. (2015) 25:281–91. doi: 10.1016/j.semradonc.2015.05.006
35. Bradley J, Deasy JO, Bentzen S, El-Naqa I. Dosimetric correlates for acute esophagitis in patients treated with radiotherapy for lung carcinoma. Int J Radiat Oncol Biol Phys. (2004) 58:1106–13. doi: 10.1016/j.ijrobp.2003.09.080
36. Seppenwoolde Y, Lebesque JV, Jaeger K de, Belderbos JS, Boersma LJ, Schilstra C, et al. Comparing different NTCP models that predict the incidence of radiation pneumonitis. Normal tissue complication probability. Int J Radiat Oncol Biol Phys. (2003) 55:724–35. doi: 10.1016/S0360-3016(02)03986-X
37. Yorke ED, Jackson A, Rosenzweig KE, Merrick SA, Gabrys D, Venkatraman ES, et al. Dose-volume factors contributing to the incidence of radiation pneumonitis in non-small-cell lung cancer patients treated with three-dimensional conformal radiation therapy. Int J Radiat Oncol Biol Phys. (2002) 54:329–39. doi: 10.1016/S0360-3016(02)02929-2
38. Jin H, Tucker SL, Liu HH, Wei X, Yom SS, Wang S, et al. Dose-volume thresholds and smoking status for the risk of treatment-related pneumonitis in inoperable non-small cell lung cancer treated with definitive radiotherapy. Radiother Oncol. (2009) 91:427–32. doi: 10.1016/j.radonc.2008.09.009
39. van Keulen HV, Gomes AS, Toffolo MC, Oliveira EE, Silva LC, Alves CC, et al. Serum levels of nitric oxide and cytokines in smokers at the beginning and after 4 months of treatment for smoking cessation. Int J Cardiol. (2017) 230:327–31. doi: 10.1016/j.ijcard.2016.12.111
40. Shiels MS, Katki HA, Freedman ND, Purdue MP, Wentzensen N, Trabert B, et al. Cigarette smoking and variations in systemic immune and inflammation markers. J Natl Cancer Inst. (2014) 106:dju294. doi: 10.1093/jnci/dju294
41. Arnson Y, Shoenfeld Y, Amital H. Effects of tobacco smoke on immunity, inflammation and autoimmunity. J Autoimmun. (2010) 34:J258–65. doi: 10.1016/j.jaut.2009.12.003
42. Levitzky YS, Guo CY, Rong J, Larson MG, Walter RE, Keaney JF, et al. Relation of smoking status to a panel of inflammatory markers: the framingham offspring. Atherosclerosis. (2008) 201:217–24. doi: 10.1016/j.atherosclerosis.2007.12.058
43. Rodriguez N, Algara M, Foro P, Lacruz M, Reig A, Membrive I, et al. Predictors of acute esophagitis in lung cancer patients treated with concurrent three-dimensional conformal radiotherapy and chemotherapy. Int J Radiat Oncol Biol Phys. (2009) 73:810–7. doi: 10.1016/j.ijrobp.2008.04.064
44. Zhu J, Zhang Z-C, Li B-S, Liu M, Yin Y, Yu J-M, et al. Analysis of acute radiation-induced esophagitis in non-small-cell lung cancer patients using the Lyman NTCP model. Radiother Oncol. (2010) 97:449–54. doi: 10.1016/j.radonc.2010.09.025
45. Zhang Z, Xu J, Zhou T, Yi Y, Li H, Sun H, et al. Risk factors of radiation-induced acute esophagitis in non-small cell lung cancer patients treated with concomitant chemoradiotherapy. Radiat Oncol. (2014) 9:54. doi: 10.1186/1748-717X-9-54
46. Caglar HB, Othus M, Allen AM. Esophagus in-field: a new predictor for esophagitis. Radiother Oncol. (2010) 97:48–53. doi: 10.1016/j.radonc.2010.07.024
47. Watkins JM, Wahlquist AE, Shirai K, Garrett-Mayer E, Aguero EG, Fortney JA, et al. Factors associated with severe acute esophagitis from hyperfractionated radiotherapy with concurrent chemotherapy for limited-stage small-cell lung cancer. Int J Radiat Oncol Biol Phys. (2009) 74:1108–13. doi: 10.1016/j.ijrobp.2008.09.013
48. Ozgen A, Hayran M, Kahraman F. Mean esophageal radiation dose is predictive of the grade of acute esophagitis in lung cancer patients treated with concurrent radiotherapy and chemotherapy. J Radiat Res. (2012) 53:916–22. doi: 10.1093/jrr/rrs056
49. Grant JD, Shirvani SM, Tang C, Juloori A, Rebueno NC, Allen PK, et al. Incidence and predictors of severe acute esophagitis and subsequent esophageal stricture in patients treated with accelerated hyperfractionated chemoradiation for limited-stage small cell lung cancer. Pract Radiat Oncol. (2015) 5:e383–91. doi: 10.1016/j.prro.2015.01.005
50. Singh AK, Lockett MA, Bradley JD. Predictors of radiation-induced esophageal toxicity in patients with non-small-cell lung cancer treated with three-dimensional conformal radiotherapy. Int J Radiat Oncol Biol Phys. (2003) 55:337–41. doi: 10.1016/S0360-3016(02)03937-8
51. Ahn S-J, Kahn D, Zhou S, Yu X, Hollis D, Shafman TD, et al. Dosimetric and clinical predictors for radiation-induced esophageal injury. Int J Radiat Oncol Biol Phys. (2005) 61:335–47. doi: 10.1016/j.ijrobp.2004.06.014
52. Huang EX, Bradley JD, El Naqa I, Hope AJ, Lindsay PE, Bosch WR, et al. Modeling the risk of radiation-induced acute esophagitis for combined Washington University and RTOG trial 93-11 lung cancer patients. Int J Radiat Oncol Biol Phys. (2012) 82:1674–9. doi: 10.1016/j.ijrobp.2011.02.052
53. Huang EX, Robinson CG, Molotievschi A, Bradley JD, Deasy JO, Oh JH. Independent test of a model to predict severe acute esophagitis. Adv Radiat Oncol. (2017) 2:37–43. doi: 10.1016/j.adro.2016.11.003
54. Zhang XJ, Sun JG, Sun J, Ming H, Wang XX, Wu L, et al. Prediction of radiation pneumonitis in lung cancer patients: a systematic review. J Cancer Res Clin Oncol. (2012) 138:2103–16. doi: 10.1007/s00432-012-1284-1
55. Huang EX, Hope AJ, Lindsay PE, Trovo M, El Naqa I, Deasy JO, et al. Heart irradiation as a risk factor for radiation pneumonitis. Acta Oncol. (2011) 50:51–60. doi: 10.3109/0284186X.2010.521192
56. Lee S, Ybarra N, Jeyaseelan K, Faria S, Kopek N, Brisebois P, et al. Bayesian network ensemble as a multivariate strategy to predict radiation pneumonitis risk. J Med Phys. (2015) 42:2421–30. doi: 10.1118/1.4915284
57. Das SK, Zhou S, Zhang J, Yin FF, Dewhirst MW, Marks LB. Predicting lung radiotherapy-induced pneumonitis using a model combining parametric Lyman probit with nonparametric decision trees. Int J Radiat Oncol Biol Phys. (2007) 68:1212–21. doi: 10.1016/j.ijrobp.2007.03.064
58. Kong F-M, Wang S. Non-dosimetric risk factors for radiation-induced lung toxicity. Semin Radiat Oncol. (2014) 25:100–9. doi: 10.1016/j.semradonc.2014.12.003
59. Ritchie RF, Palomaki GE, Neveux LM, Navolotskaia O, Ledue TB, Craig WY. Reference distributions for alpha2-macroglobulin: a practical, simple and clinically relevant approach in a large cohort. J Clin Lab Anal. (2004) 18:139–47. doi: 10.1002/jcla.20012
60. Jespersen MH, Jensen J, Rasmussen LH, Ejlersen E, Møller-Petersen J, Sperling-Petersen HU. The reference range for complexed α2-macroglobulin human plasma: development of a new enzyme linked in immunosorbent assay (ELISA) for quantitation of complexed α2-macroglobulin. Scand J Clin Lab Invest. (1993) 53:639–48. doi: 10.3109/00365519309092565
Keywords: alpha-2-macroglobulin (A2M), thoracic radiation, toxicity, radioprotection, predictive modeling
Citation: von Reibnitz D, Yorke ED, Oh JH, Apte AP, Yang J, Pham H, Thor M, Wu AJ, Fleisher M, Gelb E, Deasy JO and Rimner A (2020) Predictive Modeling of Thoracic Radiotherapy Toxicity and the Potential Role of Serum Alpha-2-Macroglobulin. Front. Oncol. 10:1395. doi: 10.3389/fonc.2020.01395
Received: 15 January 2020; Accepted: 02 July 2020;
Published: 06 August 2020.
Edited by:
Claudio Fiorino, San Raffaele Hospital (IRCCS), ItalyCopyright © 2020 von Reibnitz, Yorke, Oh, Apte, Yang, Pham, Thor, Wu, Fleisher, Gelb, Deasy and Rimner. This is an open-access article distributed under the terms of the Creative Commons Attribution License (CC BY). The use, distribution or reproduction in other forums is permitted, provided the original author(s) and the copyright owner(s) are credited and that the original publication in this journal is cited, in accordance with accepted academic practice. No use, distribution or reproduction is permitted which does not comply with these terms.
*Correspondence: Jung Hun Oh, T2hKQG1za2NjLm9yZw==