- 1Department of Clinical Science, Center for Diabetes Research, University of Bergen, Bergen, Norway
- 2Medical Laboratory CSD, Kyiv, Ukraine
- 3Department of Clinical Sciences, Lund University Diabetes Centre, Skåne University Hospital, Malmö, Sweden
Perinatal exposure to starvation is a risk factor for development of severe retinopathy in adult patients with diabetes. However, the underlying mechanisms are not completely understood. In the present study, we shed light on molecular consequences of exposure to short-time glucose starvation on the transcriptome profile of mouse embryonic retinal cells. We found a profound downregulation of genes regulating development of retinal neurons, which was accompanied by reduced expression of genes encoding for glycolytic enzymes and glutamatergic signaling. At the same time, glial and vascular markers were upregulated, mimicking the diabetes-associated increase of angiogenesis—a hallmark of pathogenic features in diabetic retinopathy. Energy deprivation as a consequence of starvation to glucose seems to be compensated by upregulation of genes involved in fatty acid elongation. Results from the present study demonstrate that short-term glucose deprivation during early fetal life differentially alters expression of metabolism- and function-related genes and could have detrimental and lasting effects on gene expression in the retinal neurons, glial cells, and vascular elements and thus potentially disrupting gene regulatory networks essential for the formation of the retinal neurovascular unit. Abnormal developmental programming during retinogenesis may serve as a trigger of reactive gliosis, accelerated neurodegeneration, and increased vascularization, which may promote development of severe retinopathy in patients with diabetes later in life.
Introduction
Intrauterine or fetal programming has in the past years been connected to age-related metabolic diseases in adults (Vaiserman and Lushchak, 2019; Hsu and Tain, 2020). Thus, there is striking evidence indicating that 25–63% of diabetes, cardiovascular disease, hypertension, and obesity can be attributed to the perinatal factors and features such as low weight at birth (Tian et al., 2006). Notably, pediatric ophthalmologists were the first to observe that preterm and low-birthweight infants exhibited a lasting abnormal retinal architecture, suggesting a key role of the intrauterine environment for vascular development in the retina and retinal neurovascular unit (RNVU) formation (Swan et al., 2018). Retinopathy is a progressive complication of diabetes with a global prevalence of 35.4% among diabetic patients (Solomon et al., 2017). Proliferative diabetic retinopathy (PDR) is the most severe form, which is characterized by progressive neovascularization leading to severe vision loss and blindness (Wong et al., 2018). Presently, there are only a few treatment alternatives for severe retinopathy targeting vascular pathology such as laser photocoagulation and anti-VEGF therapy (Wong et al., 2018). However, these treatments are not affordable in every country, and the prevalence of PDR is reported to be higher in developing countries as compared to developed countries (Ruta et al., 2013).
In support of the fetal programming hypothesis, recent epidemiological observational studies from the Ukrainian and the Hong Kong Diabetes Registries demonstrated disproportionally elevated risk for severe diabetic retinopathy in offspring to parents exposed to famine (Fedotkina et al., 2021). This evidence indicated that fetal exposure to starvation can be a triggering risk factor for vision-threatening diabetic retinopathy in adults, which might, independently or synergistically with diabetes-related metabolic risk factors, aggravate disease progression (Fedotkina et al., 2021). Understanding mechanisms involved in early retinogenesis during starvation insults, which could increase preponderance to diabetes retinopathy later in life, might aid in discovering fundamental cues for novel treatment strategies. In the present study, we investigated the effects of short-term exposure to glucose starvation on global transcriptome changes of embryonic retinal cells comprising mostly neuronal, glial, and vascular cells.
Materials and Methods
Retinal Cell Sample Preparation and Culture, RNA Isolation, and Sequencing
Isolation and Culture of Retinal Cells. C57BL/6J mice were purchased from Charles River. The retinas were isolated from E18.5 mouse embryos and digested with 0.05% trypsin (ready-made, Gibco) for 15 min at 37°C. The digestion was terminated by adding Dulbecco’s modified Eagle’s medium (Gibco) supplemented with 25 mM sodium bicarbonate (Gibco), 25 mM HEPES (Gibco), 10% fetal bovine serum (v/v, HyClone), and 1% penicillin and streptomycin solution (v/v, Gibco). The cell suspension was filtered through a 70-μM filter and centrifuged at 1,300 rpm for 5 min, resuspended in the medium, and centrifuged. This was repeated twice, and the cells were plated on poly-l-lysine-coated plates at a density of 2.0 × 106 cells/cm2. Next day, the cells were washed two times with phosphate-buffered saline (PBS) and starved for glucose in the Neurobasal medium supplemented with B27 supplement lacking insulin, with 0.06 g/l-glutamine, 1% penicillin–streptomycin (v/v, Gibco), and 11 mM HEPES for 6 h. The cells were further cultured for 6 days in the normal glucose medium (complete Neurobasal medium). The cells were harvested after 6 days of culturing for RNA isolation to obtain information on the long-term effects of starvation exposure.
Total RNA was isolated by using the miRNeasy micro kit (Qiagen), and reverse transcription was done by using the RevertAid first-strand cDNA synthesis kit (Thermo Fisher). RNA extracts were isolated by using TruSeq Stranded Total RNA with Ribo-Zero. Sequencing was done paired-end on the Illumina NextSeq 500.
The study was approved by the local ethics committee (Regional Ethics Review Board, Lund, Sweden, 2018-579, 2016/891), and the experiments were performed in compliance with the ARRIVE guidelines (Kilkenny et al., 2010).
Processing and Analysis of RNAseq Data
A total of 12 samples were sequenced (n = 6). One of the control samples was excluded from further analysis since it was not clustering with other control samples on the principal component analysis (PCA) biplot. The quality of paired-end RNAseq files was checked with MultiQC v1.0 by using fastq files. A Phred score greater than 30 was achieved for each sequencing position for all samples. There was no adapter contamination shown in QC (adapter contamination <0.1%), so trimming was not applied in order not to lose information. The alignment of transcripts was done by using Kallisto v0.43.1 (Bray et al., 2016) with the GRCh38.p10 reference assembly with the Ensembl Mus musculus v90 annotation as the reference transcriptome (Yates et al., 2016).
On average, we obtained 17.2 ± 6.7 (mean ± SD) million paired-end reads mapped to the mouse genome. After excluding lowly expressed genes (expressed in less than 20% of the samples), a total of 22,978 genes were used in the downstream analyses. The downstream analysis from this level was done by using the R statistical environment (www.r-project.org) and R Studio (www.rstudio.com). The estimated count values of the transcripts given as Kallisto output were converted to gene-level expression values by using Tximport v1.6.04 (Soneson et al., 2015). The genes that had low counts were excluded from the expression matrix with the inclusion criteria of a CPM higher than 0.5 for at least two of the samples.
PCA was applied to explore the differences between libraries. A PCA biplot and sample heatmap were prepared by using a regularized log transformation (rlog) function in the DESeq2 package (Love et al., 2014). Based on exploratory data analysis, one of the control samples was excluded from further analysis since it stood as an outlier on the PCA biplot showing the first two principal components based on gene expression counts. The PCA biplot showing the first two principal components that explain 64% of variation across the samples and the sample clustering based on the gene expression are presented in Supplementary Figure S1. The starved and control samples were separated along the first principal component axis, which shows that the gene expression was a determinant for the separation of the conditions and that most of the explained variance is primarily due to the difference between two conditions. The heatmap shows the clustering of the samples based on Euclidean distances between the samples. The distances are smaller within the conditions; hence, the samples are clustered based on the conditions.
The differential expression analysis was done with using edgeR v3.20.73 (Robinson et al., 2010; McCarthy et al., 2012). The raw count values were provided to edgeR since the tool handles the normalizations for sequencing depth, gene length, and RNA composition of the libraries (TMM normalization). Condition is used as a predictor of gene expression in a quasi-likelihood negative binomial generalized log-linear model (glmQLFit function). Empirical Bayes quasi-likelihood F-tests were used to assess the differential expression (glmQLFTest function). Batch was not included as a covariate in the final analysis since there was no obvious batch effect seen in the data exploration, and above 90% of the differentially expressed genes remained significant when the batch was added in the formula. Sex adjustment was not done via adding sex as an explicit covariate, but a robust algorithm was used to down-weight sex-linked genes (Phipson et al., 2016). False discovery rate (FDR) correction was done by using the Benjamini–Hochberg method. The gene was considered differentially expressed in case of FDR <0.05.
Cell Marker Selection
Marker genes for different cell types were selected based on literature (Fruttiger, 2002; Haverkamp et al., 2003; Casini et al., 2006; Ponomarev et al., 2006, 40; Kim et al., 2008; Johansson et al., 2010; Sanes and Zipursky, 2010; Winkler et al., 2010; Armulik et al., 2011; Kay et al., 2011; de Melo et al., 2011; Bassett et al., 2012; de Melo et al., 2012; Wu et al., 2013; Seung and Sümbül, 2014; Vlasits et al., 2014; Zhao et al., 2014; Darmanis et al., 2015; Karlstetter et al., 2015; Macosko et al., 2015; Maddox et al., 2015; Sanes and Masland, 2015; Zeisel et al., 2015; Boije et al., 2016; Gill et al., 2016; Shekhar et al., 2016; Struebing et al., 2016; Tasic et al., 2016; Vecino et al., 2016; Yu et al., 2016; Hoshino et al., 2017; Lee et al., 2017; Welby et al., 2017; Zeng and Sanes, 2017; Keeley and Reese, 2018; McDowell et al., 2018; Rheaume et al., 2018; Roesch et al., 2008). Genes were considered as marker genes of a relevant cell type upon demonstrating cell-specific expression in at least two separate publications. Cell type-specific expression has been confirmed using immunostaining or single-cell analysis in previous studies. Hence, many of them are overlapping between cell types, especially the ones that belong to the same cell classification as neuronal, glial, or vascular.
Gene Ontology and Pathway Enrichment Analyses
GO and pathway enrichments were done by using goana and kegga functions in edgeR. The genes in the relevant GO terms were extracted from the main page of gene ontology (www.geneontology.org) and KEGG (genome.jp) databases (Ashburner et al., 2000; Kanehisa and Goto, 2000; Mi et al., 2017; The Gene Ontology Consortium, 2017). The following packages were used for the creation of figures: GOplot (Walter et al., 2015) and ggplot2 (Wickham, 2009).
Results
Embryonic retinal cells were exposed to short-term 6-h glucose starvation to study the effects of perinatal glucose starvation on the global transcriptomic profile (n = 6) (Figure 1A) (Methods). Control cells were cultured under normal culture environment (n = 5). RNA was extracted for bulk RNA sequencing, and differential expression in the starved cells compared to controls was analyzed. A gene was labeled as differentially expressed between starved and control cells after multiple testing adjustment of p-values using FDR <0.05. We detected over 5,000 genes differentially expressed in response to exposure starvation; of those, 3,051 were upregulated and 2,533 were downregulated (FDR <0.05, Figure 1B and Supplementary Table S1). About 77% of upregulated genes (2,341) and 13% of downregulated genes (318) had at least a twofold change in the starved-for-glucose samples compared to non-treated controls (Figure 1C).
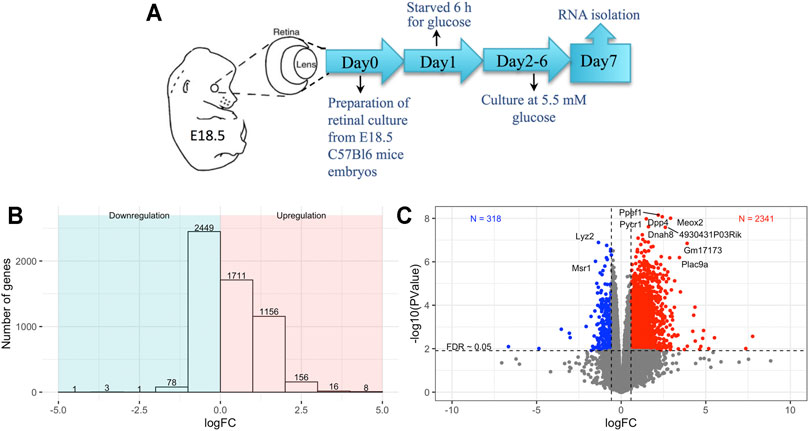
FIGURE 1. (A) Schematics of experimental setup, (B) barplot showing the number of differentially expressed genes for different log-fold-change bins, (C) volcano plot showing the variation of significance with log-fold-change; blue and red colors show downregulated and upregulated genes in the starved conditions with a log-fold-change greater than 50% between conditions and FDR <0.05.
Exposure to Glucose Starvation Results in Downregulation of Neuronal Retinal Marker Gene Expression
To study the effect of exposure to glucose starvation on transcriptomic profiles of different retinal cells, we analyzed expression of various retinal cell markers previously described in the literature (see Methods) (Figure 2 and Supplementary Figure S3 and Supplementary Table S2). It is worth mentioning that some of these genes were reported to be expressed in several retinal cell types and were not specific to a single cell type although they were still used as markers.
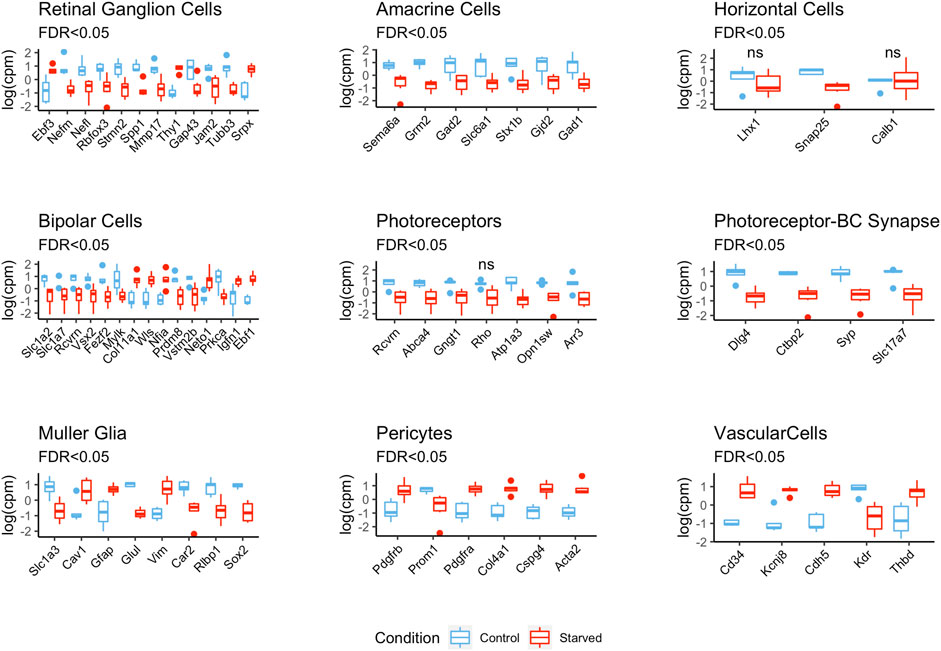
FIGURE 2. Differential expression of retinal cell markers between starved and control conditions. The expression values are centered and scaled (Supplementary Figure S4 shows unscaled plots with expression levels).
There were 23 retinal ganglion cell (RGC) markers detected, of which 10 (Gap43, Jam2, Nefl, Nefm, Mmp17, Rbfox3, Spp1, Stmn2, and Tubb3) were downregulated, while Thy1 and Ebf3 were highly upregulated as a result of starvation. The differential expressions of other RGC markers such as Atoh7, Cartpt, Slc17a6, and Pou4f1 were not statistically significant.
Expression of amacrine cell (AC) markers (7 out of 16) was significantly downregulated in the starved samples. These were markers related with GABAergic cells: glutamate decarboxylase genes Gad1 and Gad2, GABA transporter Slc6a1, and metabotropic glutamate receptor gene Grm2. Sema6a, which is an important gene for starburst ACs (SACs) and for stratification of the retinal layers (Seung and Sümbül, 2014), was also one of the downregulated AC markers.
The expression levels of horizontal cell marker genes Lhx1, Snap25, and Calb1 were decreased in the starved cells, but only Snap25 was found to be significantly differentially expressed with FDR <0.05.
General bipolar cell (BC) markers such as Slc1a7, which encode glutamate transporter; Prdm8; and Vsx2 showed decreased expression after starvation exposure as well as rod BC markers Prkca and Vstm2b, which are important for BC survival and differentiation during embryogenesis. In contrast, Col11a1, Ebf1, Igfn1, Neto1, Nfia, and Wls were the upregulated BC markers probably reflecting disproportion in On and Off BC subtypes.
Photoreceptor (PR) marker genes Abca4, Arr3, Atp1a3, Gngt1, Rcvrn, and Opn1sw were downregulated in the starved samples, while the downregulation of rhodopsin (Rho) was not statistically significant. Synaptic markers such as Ctbp2, Dlg4, Slc17a7, and Syp were also highly and negatively affected by the starvation.
For the Müller cells (MC), Rlbp1, Glul, Slc1a3, and Car2 genes, involved in neuroglial interplay, were downregulated with starvation, while Cav1, Gfap, and Vim were upregulated. Astrocyte markers such as Aldh1l1, S100b, and Fgfr3 were highly upregulated in the starved cells, while Slc1a2 was downregulated. For microglia, Adgre1, Cd40, Cd68, Aif1, Ptprc, Itgam, and Cx3cr1 were used as markers, and only Cd68 was found to be differentially expressed and downregulated in the starved cells.
Notably, we have also detected a decrease in the expression levels of neuronal cell markers that belong to different lineages, such as Sox2, Notch1, and also Pax6—an established developmental marker of progenitor cells in the retina (Supplementary Table S3).
Starvation Induces Proangiogenic Reprograming of the Developing Retina
Markers of pericytes and vascular endothelial cells such as Cd34, Cdh5, Cspg4, Thbd, Pdgfrb, and Acta2 showed upregulation after starvation exposure, while Vwf and Des were not significantly differentially expressed. Additionally, some genes associated with vascularization such as Tek and Angptl1 were also upregulated in the starved samples. VEGF genes (Vegfa, Vegfc, and Vegfd) were significantly upregulated after starvation exposure, but the Vegf receptor gene Kdr is found to be highly downregulated in the starved samples.
Given that VEGFs have been ascribed to play a pivotal role in co-patterning of nerves and vessels, we performed co-expression analysis of differentially expressed genes with Vegfa, Vegfc, and Vegfd in order to investigate potential VEGF-related candidates for therapeutic interrogation. Almost half of the differentially expressed genes were found to be commonly correlated across all Vegfs (n = 25,12, adjusted p-value ≤ 0.05) (Supplementary Table S5). Furthermore, to focus on vascularization-related genes in the co-expression analysis, we employed the gene list from the MGI database including many blood vessel development- and angiogenesis-related terms (Supplementary Table S6). Out of 262 differentially expressed genes related with these terms, 200 had common correlation across all three VEGF genes (adjusted p-value ≤ 0.05) (Supplementary Table S7).
Increased Vascularization Was Associated With Compromised Neuronal Development in the Starved Retina
For the investigation of the biological landscape affected by the short-term starvation exposure, we performed enrichment analysis using GO terms and KEGG pathways. Figure 3 shows specific GO biological process (BP) terms enriched for the differentially expressed genes (Supplementary Figure S4). Two of the top GO terms were nervous system development and blood vessel development, which were enriched for the downregulated (p = 1.4 · 10–42) and upregulated (p = 3.8 · 10–18) directions, respectively (Figure 3).
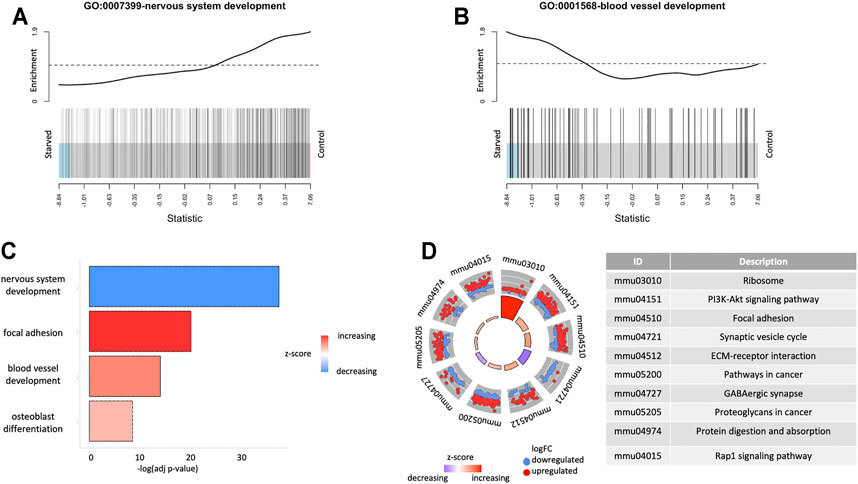
FIGURE 3. Functional enrichment results for expressed genes. (A) Gene Set Enrichment Analysis (GSEA) for GO terms GO: 0001568-blood vessel development and GO:0007399-nervous system development. (B) Overrepresentation analysis of GO terms for differentially expressed genes. (C) Overrepresentation analysis of KEGG pathways for differentially expressed genes.
Short-Term Glucose Starvation Had a Lasting Effect On Various BPs and Signaling Pathways
Figure 3D shows the KEGG pathway enrichments for the differentially expressed genes. One of the top enriched pathways is the PI3K-Akt signaling pathway (pup = 2.6 · 10–11), which is among the most important signaling pathways for metabolic control, cell survival, and proliferation, demonstrating enrichment of overexpressed genes in the starved cells. Among other upregulated KEGG pathways (p < 10–6) as a result of starvation exposure were ribosome (p = 3.2 · 10–30), olfactory transduction (p = 7.1 · 10–14), focal adhesion (p = 1.4 · 10–10), ECM–receptor interaction (p = 7.0 · 10–10), and those related to cancer (p = 6.3 · 10–8). On the contrary, synaptic vesicle cycle (p = 6.5 · 10–13) and GABAergic synapse (p = 5.6 · 10–9) pathways were downregulated, which can imply altering of neuroglial relations and synaptic transduction in the retina after glucose deprivation.
Genes Regulating Fatty Acid Elongation Were Upregulated After Glucose Starvation to Compensate for Reduced Expression of Glycolytic Enzymes
To shed light on the processes involved in energy balance affected by exposure to starvation, we analyzed genes involved in glucose, lipid, and amino acid metabolism. As expected in the condition of starvation for glucose, there was decreased expression of the genes involved in several steps of glycolysis including glucose transport (Figure 4A). These genes encode enzymes in the pathway from glucose to pyruvate metabolism, as well as Ldha and Ldhb encoding for l-lactate dehydrogenase, which catalyzes pyruvate-to-lactate conversion (Kanehisa and Goto, 2000; Slenter et al., 2018). In line with decreased expression of glycolytic genes, glucose transporters such as Slc2a1 (GLUT1) and Slc2a3 (GLUT3) were downregulated after starvation (FDR <0.05).
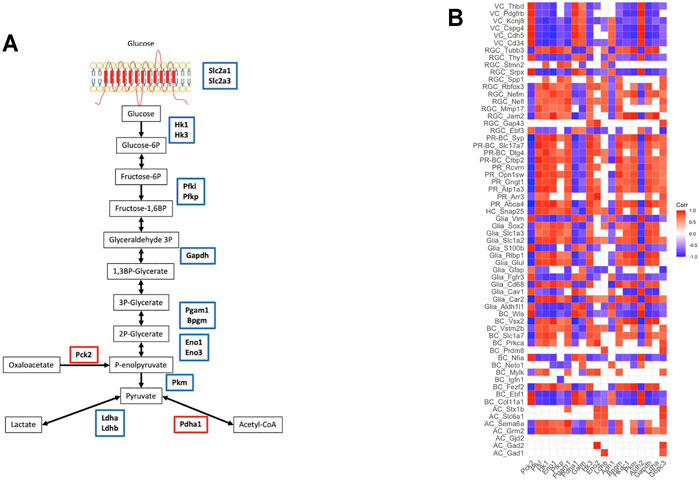
FIGURE 4. Metabolic changes after starvation exposure: (A) glycolysis pathway—the genes encoding the enzymes functioning in the pathway were downregulated, (B) the correlation between retinal cell markers and glycolysis/gluconeogenesis genes (VC: vascular cells, RGC: retinal ganglion cells, PR-BC: photoreceptor-bipolar cell synapses, PR: photoreceptor cells, HC: horizontal cells, BC: bipolar cells, AC: amacrine cells).
On the contrary, genes encoding for enzymes in the fatty-acid elongation pathway in the endoplasmic reticulum such as Elovl1, Elovl3, Elovl5, Fads1, Scd1, Hacd4, Acot1, and Acot2 were highly upregulated in the starved samples indicative of increased biosynthesis of fatty acids. Similarly, genes involved in amino acid transport and metabolism such as Dpp4, Xpnpep1, Xpnpep2, Mme, and Slc1a5 are highly upregulated in the starved cells (Supplementary Table S1).
Finally, to investigate the glycolytic and metabolic states of different retinal cell types, we assessed correlations of neuronal markers with glycolysis genes (Figure 4B). Assessment of the links between metabolic characteristics and cell-specific markers demonstrated a positive correlation between expressions of Ldha and PR-specific genes (Abca4, Arr3, Atp1a3, Gngt1, and Rcvrn) involved in different aspects of PR functioning including all-trans-retinal aldehyde and cation transport, regulation of rhodopsin activity, proper propagation, and termination of signaling. Moreover, glycolytic enzymes and Ldha repression were associated with downregulation of signaling molecules involved in neuronal subtype-specific patterning and axonal growth, as well as in synaptic plasticity regulation. Downregulation of Hk1, Hk3, Hkdc1, Pfk, and Pkm positively correlated with lower expression of genes encoding adhesion molecules and intercellular junctions (Jam2 and Mmp17), proteins regulating neuronal differentiation (Rbfox3) and growth (Stmn2), axonal transport (Nefl, Nefm, and Tubb3), and neuroprotection (Spp1) in RGC. Glycolytic enzymes and Ldha expression were in synchrony with downregulation of glutamate and GABA signaling and transport in both neurons and glial cells. Additionally, glycolytic enzyme expressions showed profound negative correlation with vascular marker expressions.
Discussion
The main findings in the present study provide evidence of differential changes in expression of genes contributing to retinal development and functioning after short-term metabolic disruption. Obtained data allow us to generate a hypothesis of irreversible and detrimental reprogramming of neurovascular unit (RNVU) formation during retinal development after early-life exposure to glucose starvation. Even after a “relief” period of normal conditions, the effects on the transcriptomic landscape of the retina were still immense. The key features appear to include multiple cellular pathways highlighting disrupted gene expression in the retina. Specifically, transcriptomic analysis has revealed a profound decrease in the expression of neuronal markers, while genes encoding for vascular markers were upregulated, similar to the diabetes-associated increase of angiogenesis in diabetic retinopathy.
Investigation of the molecular mechanisms underlying retinogenesis is important in order to gain knowledge on how to enlighten the path ahead for prevention or treatment of retinopathy in adults with diabetes. The top differentially expressed genes affected by starvation comprised Ppef1, which encodes for the protein product suggested to play a role in sensory neuron function and development; Dpp4, encoding a protease enzyme involved in the cleavage of a broad range of vasoactive peptides, which is also an established drug target for type 2 diabetes (Dicker, 2011); Meox2 gene, known to regulate angiogenesis and myogenesis; and Pycr1 and Dnah8, involved in the ATP-related processes. These findings provide further support for involvement of multiple mechanisms including neuronal, vascular, and energy metabolism that together may contribute to compromise early development of the entire RNVU.
A major pathological feature of advanced and severe forms of retinopathy in patients with diabetes is characterized by accelerated proliferative angiogenesis. This gives rise to an increased growth of small and immature vessels in the retina, which are susceptible to breakage and bleeding. In the present experiments using embryonic retinal cells, the vascular cell markers showed overall upregulation after exposure to glucose starvation, which could be a consequence of lack of necessary nutrition. The restructure of the vascular network might therefore be needed to reach resources even after a short-term glucose deprivation. In support of this, expression of other genes important for blood vessel development (Tek and Angptl1) was also upregulated along with the retinal vascular markers. As was previously reported, starvation causes acute energy depletion that can create a hypoxia-like condition (Rego et al., 1998). As a consequence of this, triggering of mechanisms to promote increased blood supply takes place, typically involving activation of HIF—a major driver for the transcription of VEGFs and overall over 60 genes adjusting cells to a hypoxic state (Sapieha et al., 2010). In this study, we have demonstrated that all VEGF genes (Vegfa, Vegfc, and Vegfd) were significantly upregulated after starvation exposure. Notably, elevated VEGF-C and VEGF-D levels were found in the retinal pigment epithelium (RPE) of patients with age-related macular degeneration (Vellanki et al., 2016). There are also data confirming a significant role of VEGF-D in retinal angiogenesis and ganglion cell protection under excitotoxic injury (Schlüter et al., 2020). In addition, it was demonstrated that hypoxia-induced expression of VEGF-C in the retina is as potent as VEGF-A in inducing pathological retinal neovascularization in PDR and retinopathy of prematurity (Campochiaro, 2015; Singh et al., 2015; Vellanki et al., 2016). In the present study, positive correlations between Vegf’s expression and vascular markers indicate strong promotion of angiogenesis.
Kdr (or Vegfr2) that binds Vegfa, Vegfc, and Vegfd in the retina was strongly downregulated in starved samples. In contrast to Flt1 (Vegfr1) that is restricted to endothelial cells, Kdr is abundantly expressed in the neuroretina (Penn et al., 2008). Importantly, during retinal neurogenesis, Kdr is also expressed by neural progenitor cells and retinal neurons (Hashimoto et al., 2006). High Kdr expression in embryonic retinal nerve cells under physiological conditions titrates VEGF to moderate spatial patterning of angiogenesis and limits internal retinal vascularization. It was shown that loss of Kdr in neurons caused misdirected angiogenesis toward neurons, resulting in abnormally increased vascular density around neurons (Okabe et al., 2014). Interestingly, Müller cell survival and proliferation during retinal development depend on VEGFR- and MAPK-related signaling (Liu et al., 2019). Mice with conditional knockout of Kdr demonstrated significant loss of Müller cells under diabetes/hypoxia, which accelerated retinal degeneration. These show the critical role of VEGF signaling in glial cells’ viability and neuronal integrity (Fu et al., 2018). Thus, increased VEGF expression after glucose deprivation may reflect activation of proangiogenic activity of retinal glial cells and provokes abnormal angiogenesis in the inner retinal compartment, whereas downregulation of Kdr can be related with reduced neurogenesis. A simultaneous increase of vascular markers supports this idea. The fact of increased expression of VEGF and other vascular markers reflects strengthening of the glial–vascular relationship, while neuroglial relations were compromised.
The key master regulator of metabolic relationships between different retinal cells in RNVU is macroglia (Müller cells and astrocytes). Müller cells are the main contributors of glutamate and GABA recycling in the retina and responsible for glutamate uptake from the synaptic cleft to prevent neurotoxicity (Bringmann et al., 2013). As glutamate and GABA are principal neurotransmitters ensuring the radial and lateral synaptic pathways, respectively (Yang, 2004), their downregulation could lead to the decrease of the retinal functional integration and contribute to neuronal degeneration (Bringmann et al., 2013). Similar to GABA, observed downregulation of Glul may reflect impaired regulatory function of Müller cells in neurotransmission and weakening of the neuroglial relationship. On the other hand, this may be related with reactive glial cells (de Melo et al., 2012) and the breakdown of the blood–retinal barrier (Shen et al., 2009), considering detected upregulation of Gfap and Vim. Reactive glia in turn may contribute to neuronal death via glutamate excitotoxicity (Sundstrom et al., 2018).
Notably, decreases in expression of some genes such as Sema6a, which has an important function in stratification of retinal layers (Seung and Sümbül, 2014), may furthermore indicate the overall dissociating effect of starvation exposure on the retinal structure. We observed a strong decrease in expression of marker genes for photoreceptors and photoreceptor-to-bipolar cell synapses, indicating that these cells and their connections are among the most vulnerable to abnormal metabolic conditions. Concomitant downregulation of other neuronal markers, especially the markers for progenitors and RGCs, and the upregulation of vascular and reactive glial markers can suggest that the short-term starvation for glucose may not only cause a retardation in the temporal development but might also give rise to the events promoting future neurodegeneration.
Interestingly, the expression of glucose transporters and glycolytic enzyme genes after exposure to glucose starvation correlated positively with photoreceptors and RGC, while it negatively correlated with VEGF expression in the retina. The similar changes were revealed under retinopathy of prematurity. Increased angiogenesis was associated with decreased retinal Glut1 and glycolytic enzyme expressions. In line with this, it was demonstrated that improvement of glucose uptake and glycolysis may restore retinal neuron formation and normalize retinal angiogenesis (Han et al., 2019).
When interpreting reciprocal changes in vascular drivers and glycolytic enzyme expression, it is important to underline the spatial distribution of distinct expressions and various responses of different retinal cells to hypoxia. The main site of aerobic glycolysis enzyme expression and lactate production is PRs cells located in the outer retina, whereas the main source of VEGF is Müller and ganglionic cells located in the inner retina. Within the retina, PRs rank among the highest-energy-consuming systems. Although PRs are rich in mitochondria and oxidative phosphorylation (OXPHOS) enzymes, these cells convert most of their glucose to lactate through aerobic glycolysis—a process known as the Warburg effect and accounts for about 80–90% of glucose metabolism in adult PRs (Chinchore et al., 2017; Narayan et al., 2017). It is speculated that aerobic glycolysis provides sufficient glucose influx to the pentose-phosphate pathway (PPP) with the subsequent generation of NAPH and lipid synthesis, allowing the recycle of the appropriate amounts of visual pigment (Sun et al., 2008). Lactate production depends on the expression and activity of LDHA, the enzyme critical for the Warburg effect, which was also downregulated in the retina due to starvation. The lack of LDH-A expression was detected in rats with inherited “retinitis pigmentosa,” associated with pathological loss of the photoreceptors (Narayan et al., 2019). This finding supports the notion that the PRs are particularly susceptible to inhibition of glycolysis. Lactate production by PRs is also important for the retinal glial cell functioning. In contrast to PRs, Müller glial cells do not express hexokinase or any pyruvate kinase isoform required for glycolysis (Lindsay et al., 2014; Rueda et al., 2016). Lactate provided by aerobic glycolysis in PRs is used as a fuel by Müller cells and RPE. Additionally, lactate, rather than glucose, is the most effective source of carbon for glutamine synthesis by Müller cells (Gardner and Davila, 2017). Naturally, downregulation of glycolytic enzymes, such as Hk1/3 and Impdh, in the starved retina was associated with a decline in expression of other molecules involved in glutamate turnover, neuronal differentiation, axonal growth, and synaptic transduction. This highlights the importance of a tight interface between retinal neurons and Müller cells that work together as an ecosystem to build metabolically specialized and interdependent RNVUs.
Importantly, the KEGG pathway analysis demonstrated upregulation of gene clusters related to PI3K-Akt and cancer-associated pathways in addition to the regulation of cell-matrix interplay, cell adhesion and migration, protein synthesis, and proteolysis (Figure 3D). Akt was found to be increased as a result of hyperglycemia, which is regarded as the primary cause of the development of retinopathy in the patients with diabetes (Qin et al., 2015). The increase in the signaling through PI3K promotes fibrosis in the retina, which in turn aggravates development of retinopathy. In support of activation of fibrosis in the samples starved for glucose, the extracellular matrix genes such as collagens (such as Col4a1 and Col1a1), fibronectin (Fn1), and laminins (such as Lamb1 and Lama4) were significantly differentially expressed with high fold changes. This signaling pathway may be a target for therapeutic intervention of pathogenic angiogenesis similar to those being developed in cancer (Sasore et al., 2014).
Limitations
The global expression analyses in the present study are performed using mRNA sequencing, and the corresponding protein data and morphological evidence are lacking. Therefore, additional experiments would be needed to expand current results for further validation at the protein expression level. Nevertheless, our observations on downregulation of glycolytic enzymes and their strong correlation predominantly with PR markers are in support of the suggested critical importance of main regulators of aerobic glycolysis in PR not only in the enzymatic reactions but also possibly in acting as neuroprotective mechanisms critical in maintaining PR health, viability, and survival (Weh et al., 2020). It is important to note that current results were generated using mouse embryonic retinal cells, and validation in humans would be warranted. To this extent, a genome-wide association study for severe retinopathy is ongoing in our cohort of Ukrainian patients with type 2 diabetes who were exposed to famine at birth, as a part of the National Ukrainian Registry (Fedotkina et al., 2021).
In summary, the present data provide hypothesis-generating evidence that perinatal glucose deprivation may cause metabolic adaptations in different compartments of the embryonic retina, leading to alterations in the developmental program of the entire RNVU. As was shown in the present study by experimental modeling of starvation for glucose of embryonic retinal cells, early-life metabolic adaptations might trigger reprogramming of retinogenesis with alteration of retinal neurogenesis towards abnormal angiogenesis. Thus, a negative correlation between the expression of glycolytic enzymes and VEGFs in the retina after starvation may reflect the desynchronization of neuroglial interplay and dissociation between outer and inner retinal functions and therefore choroid and retinal vasculature formation. These findings highlight the crucial importance of restoring the balance between neuroglial and glio-vascular function. A combined strategy including antiangiogenic drugs and metabolic correction could be proposed as a beneficial therapeutic approach. The latter could include governed stimulation of aerobic glycolysis and activation of the glutamate transporters to improve neuroglial coupling through lactate production supporting metabolic processes, neurotransmitter exchange, and synaptic transduction.
Data Availability Statement
The datasets presented in this study can be found in online repositories. The names of the repository/repositories and accession number(s) can be found below: Experiment ArrayExpress accession: E-MTAB-10705.
Ethics Statement
The study was approved by the local ethics committee (Regional Ethics Review Board, Lund, Sweden, 2018-579, 2016/891), and the experiments were performed in compliance with the ARRIVE guidelines (Kilkenny et al., 2010).
Author Contributions
TO did the statistical analyses, interpreted the data, and drafted the manuscript. RJ developed an experimental in vitro model. OS and VL interpreted the data and drafted the manuscript. RJ, IA, and VL developed the study design and collected the data. All authors contributed to the interpretation of the data and approved the final version of the manuscript.
Funding
This project is supported by the Swedish Research Council (Dnr 2015-03574 and Dnr349-2006-237), Strategic Research Area Exodiab (Dnr 2009-1039), Novo Nordisk Foundation (NNF12OC1016467), Swedish Foundation for Strategic Research (DnrIRC15-0067), Steno Diabetes Center Copenhagen, Bergen Research Foundation (BFS811294), and University of Bergen.
Conflict of Interest
The authors declare that the research was conducted in the absence of any commercial or financial relationships that could be construed as a potential conflict of interest.
Publisher’s Note
All claims expressed in this article are solely those of the authors and do not necessarily represent those of their affiliated organizations, or those of the publisher, the editors and the reviewers. Any product that may be evaluated in this article, or claim that may be made by its manufacturer, is not guaranteed or endorsed by the publisher.
Supplementary Material
The Supplementary Material for this article can be found online at: https://www.frontiersin.org/articles/10.3389/fcell.2021.726852/full#supplementary-material
References
Armulik, A., Genové, G., and Betsholtz, C. (2011). Pericytes: Developmental, Physiological, and Pathological Perspectives, Problems, and Promises. Develop. Cel 21, 193–215. doi:10.1016/j.devcel.2011.07.001
Ashburner, M., Ball, C. A., Blake, J. A., Botstein, D., Butler, H., Cherry, J. M., et al. (2000). Gene Ontology: Tool for the Unification of Biology. Nat. Genet. 25, 25–29. doi:10.1038/75556
Bassett, E. A., Korol, A., Deschamps, P. A., Buettner, R., Wallace, V. A., Williams, T., et al. (2012). Overlapping Expression Patterns and Redundant Roles for AP-2 Transcription Factors in the Developing Mammalian Retina. Dev. Dyn. 241, 814–829. doi:10.1002/dvdy.23762
Boije, H., Shirazi Fard, S., Edqvist, P.-H., and Hallböök, F. (2016). Horizontal Cells, the Odd Ones Out in the Retina, Give Insights into Development and Disease. Front. Neuroanat. 10, 77. doi:10.3389/fnana.2016.00077
Bray, N. L., Pimentel, H., Melsted, P., and Pachter, L. (2016). Near-Optimal Probabilistic RNA-Seq Quantification. Nat. Biotechnol. 34, 525–527. doi:10.1038/nbt.3519
Bringmann, A., Grosche, A., Pannicke, T., and Reichenbach, A. (2013). GABA and Glutamate Uptake and Metabolism in Retinal Glial (Müller) Cells. Front. Endocrinol. 4, 48. doi:10.3389/fendo.2013.00048
Campochiaro, P. A. (2015). Molecular Pathogenesis of Retinal and Choroidal Vascular Diseases. Prog. Retin. Eye Res. 49, 67–81. doi:10.1016/j.preteyeres.2015.06.002
Casini, G., Rickman, D. W., and Brecha, N. C. (2006). Expression of the γ-Aminobutyric Acid (GABA) Plasma Membrane Transporter-1 in Monkey and Human Retina. Invest. Ophthalmol. Vis. Sci. 47, 1682–1690. doi:10.1167/iovs.05-1117
Chinchore, Y., Begaj, T., Wu, D., Drokhlyansky, E., and Cepko, C. L. (2017). Glycolytic reliance Promotes Anabolism in Photoreceptors. Elife 6, e25946. doi:10.7554/eLife.25946
Darmanis, S., Sloan, S. A., Zhang, Y., Enge, M., Caneda, C., Shuer, L. M., et al. (2015). A Survey of Human Brain Transcriptome Diversity at the Single Cell Level. Proc. Natl. Acad. Sci. USA 112, 7285–7290. doi:10.1073/pnas.1507125112
de Melo, J., Miki, K., Rattner, A., Smallwood, P., Zibetti, C., Hirokawa, K., et al. (2012). Injury-Independent Induction of Reactive Gliosis in Retina by Loss of Function of the LIM Homeodomain Transcription Factor Lhx2. Proc. Natl. Acad. Sci. 109, 4657–4662. doi:10.1073/pnas.1107488109
de Melo, J., Peng, G.-H., Chen, S., and Blackshaw, S. (2011). The Spalt Family Transcription Factor Sall3 Regulates the Development of Cone Photoreceptors and Retinal Horizontal Interneurons. Development 138, 2325–2336. doi:10.1242/dev.061846
Dicker, D. (2011). DPP-4 Inhibitors: Impact on Glycemic Control and Cardiovascular Risk Factors. Diabetes Care 34, S276–S278. doi:10.2337/dc11-s229
Fedotkina, O., Luk, A., Jain, R., Prasad, R. B., Shungin, D., Simó‐Servat, O., et al. (2021). Perinatal Famine Is Associated with Excess Risk of Proliferative Retinopathy in Patients with Type 2 Diabetes. Acta Ophthalmol. doi:10.1111/aos.14948
Fruttiger, M. (2002). Development of the Mouse Retinal Vasculature: Angiogenesis Versus Vasculogenesis. Invest. Ophthalmol. Vis. Sci. 43, 522–527. Available at: https://iovs.arvojournals.org/article.aspx?articleid=2162512 (Accessed November 13, 2018).
Fu, S., Dong, S., Zhu, M., and Le, Y.-Z. (2018). VEGF as a Trophic Factor for Müller Glia in Hypoxic Retinal Diseases. Adv. Exp. Med. Biol. 1074, 473–478. doi:10.1007/978-3-319-75402-4_58
Gardner, T. W., and Davila, J. R. (2017). The Neurovascular Unit and the Pathophysiologic Basis of Diabetic Retinopathy. Graefes Arch. Clin. Exp. Ophthalmol. 255, 1–6. doi:10.1007/s00417-016-3548-y
Gill, K. P., Hung, S. S. C., Sharov, A., Lo, C. Y., Needham, K., Lidgerwood, G. E., et al. (2016). Enriched Retinal Ganglion Cells Derived from Human Embryonic Stem Cells. Sci. Rep. 6, 30552. doi:10.1038/srep30552
Han, X., Kong, J., Hartnett, M. E., and Wang, H. (2019). Enhancing Retinal Endothelial Glycolysis by Inhibiting UCP2 Promotes Physiologic Retinal Vascular Development in a Model of Retinopathy of Prematurity. Invest. Ophthalmol. Vis. Sci. 60, 1604–1613. doi:10.1167/iovs.19-26553
Hashimoto, T., Zhang, X.-M., Chen, B. Y.-K., and Yang, X.-J. (2006). VEGF Activates Divergent Intracellular Signaling Components to Regulate Retinal Progenitor Cell Proliferation and Neuronal Differentiation. Development 133, 2201–2210. doi:10.1242/dev.02385
Haverkamp, S., Ghosh, K. K., Hirano, A. A., and Wässle, H. (2003). Immunocytochemical Description of Five Bipolar Cell Types of the Mouse Retina. J. Comp. Neurol. 455, 463–476. doi:10.1002/cne.10491
Hoshino, A., Ratnapriya, R., Brooks, M. J., Chaitankar, V., Wilken, M. S., Zhang, C., et al. (2017). Molecular Anatomy of the Developing Human Retina. Develop. Cel 43, 763–779.e4. doi:10.1016/j.devcel.2017.10.029
Hsu, C.-N., and Tain, Y.-L. (2020). Developmental Origins of Kidney Disease: Why Oxidative Stress Matters? Antioxidants 10, 33. doi:10.3390/antiox10010033
Johansson, U. E., Eftekhari, S., and Warfvinge, K. (2010). A Battery of Cell- and Structure-Specific Markers for the Adult Porcine Retina. J. Histochem. Cytochem. 58, 377–389. doi:10.1369/jhc.2009.954933
Kanehisa, M., and Goto, S. (2000). KEGG: Kyoto Encyclopedia of Genes and Genomes. Nucleic Acids Res. 28, 27–30. doi:10.1093/nar/28.1.27
Karlstetter, M., Scholz, R., Rutar, M., Wong, W. T., Provis, J. M., and Langmann, T. (2015). Retinal Microglia: Just Bystander or Target for Therapy? Prog. Retin. Eye Res. 45, 30–57. doi:10.1016/j.preteyeres.2014.11.004
Kay, J. N., De la Huerta, I., Kim, I.-J., Zhang, Y., Yamagata, M., Chu, M. W., et al. (2011). Retinal Ganglion Cells with Distinct Directional Preferences Differ in Molecular Identity, Structure, and Central Projections. J. Neurosci. 31, 7753–7762. doi:10.1523/JNEUROSCI.0907-11.2011
Keeley, P. W., and Reese, B. E. (2018). DNER and NFIA Are Expressed by Developing and Mature AII Amacrine Cells in the Mouse Retina. J. Comp. Neurol. 526, 467–479. doi:10.1002/cne.24345
Kilkenny, C., Browne, W. J., Cuthill, I. C., Emerson, M., and Altman, D. G. (2010). Improving Bioscience Research Reporting: The ARRIVE Guidelines for Reporting Animal Research. Plos Biol. 8, e1000412. doi:10.1371/journal.pbio.1000412
Kim, D. S., Ross, S. E., Trimarchi, J. M., Aach, J., Greenberg, M. E., and Cepko, C. L. (2008). Identification of Molecular Markers of Bipolar Cells in the Murine Retina. J. Comp. Neurol. 507, 1795–1810. doi:10.1002/cne.21639
Lee, S., Elaskandrany, M., Lau, L. F., Lazzaro, D., Grant, M. B., and Chaqour, B. (2017). Interplay between CCN1 and Wnt5a in Endothelial Cells and Pericytes Determines the Angiogenic Outcome in a Model of Ischemic Retinopathy. Sci. Rep. 7, 1405. doi:10.1038/s41598-017-01585-8
Lindsay, K. J., Du, J., Sloat, S. R., Contreras, L., Linton, J. D., Turner, S. J., et al. (2014). Pyruvate Kinase and Aspartate-Glutamate Carrier Distributions Reveal Key Metabolic Links between Neurons and Glia in Retina. Proc. Natl. Acad. Sci. 111, 15579–15584. doi:10.1073/pnas.1412441111
Liu, Y., Wang, C., and Su, G. (2019). Cellular Signaling in Müller Glia: Progenitor Cells for Regenerative and Neuroprotective Responses in Pharmacological Models of Retinal Degeneration. J. Ophthalmol. 2019, 5743109. doi:10.1155/2019/5743109
Love, M. I., Huber, W., and Anders, S. (2014). Moderated Estimation of Fold Change and Dispersion for RNA-Seq Data with DESeq2. Genome Biol. 15, 550. doi:10.1186/s13059-014-0550-8
Macosko, E. Z., Basu, A., Satija, R., Nemesh, J., Shekhar, K., Goldman, M., et al. (2015). Highly Parallel Genome-wide Expression Profiling of Individual Cells Using Nanoliter Droplets. Cell 161, 1202–1214. doi:10.1016/j.cell.2015.05.002
Maddox, D. M., Collin, G. B., Ikeda, A., Pratt, C. H., Ikeda, S., Johnson, B. A., et al. (2015). A Mutation inSyne2Causes Early Retinal Defects in Photoreceptors, Secondary Neurons, and Müller Glia. Invest. Ophthalmol. Vis. Sci. 56, 3776–3787. doi:10.1167/iovs.14-16047
McCarthy, D. J., Chen, Y., and Smyth, G. K. (2012). Differential Expression Analysis of Multifactor RNA-Seq Experiments with Respect to Biological Variation. Nucleic Acids Res. 40, 4288–4297. doi:10.1093/nar/gks042
McDowell, R. E., Barabas, P., Augustine, J., Chevallier, O., McCarron, P., Chen, M., et al. (2018). Müller Glial Dysfunction during Diabetic Retinopathy in Rats Is Reduced by the Acrolein-Scavenging Drug, 2-Hydrazino-4,6-Dimethylpyrimidine. Diabetologia 61, 2654–2667. doi:10.1007/s00125-018-4707-y
Mi, H., Huang, X., Muruganujan, A., Tang, H., Mills, C., Kang, D., et al. (2017). PANTHER Version 11: Expanded Annotation Data from Gene Ontology and Reactome Pathways, and Data Analysis Tool Enhancements. Nucleic Acids Res. 45, D183–D189. doi:10.1093/nar/gkw1138
Narayan, D. S., Chidlow, G., Wood, J. P., and Casson, R. J. (2017). Glucose Metabolism in Mammalian Photoreceptor Inner and Outer Segments. Clin. Exp. Ophthalmol. 45, 730–741. doi:10.1111/ceo.12952
Narayan, D. S., Chidlow, G., Wood, J. P. M., and Casson, R. J. (2019). Investigations into Bioenergetic Neuroprotection of Cone Photoreceptors: Relevance to Retinitis Pigmentosa. Front. Neurosci. 13, 1234. doi:10.3389/fnins.2019.01234
Okabe, K., Kobayashi, S., Yamada, T., Kurihara, T., Tai-Nagara, I., Miyamoto, T., et al. (2014). Neurons Limit Angiogenesis by Titrating VEGF in Retina. Cell 159, 584–596. doi:10.1016/j.cell.2014.09.025
Penn, J. S., Madan, A., Caldwell, R. B., Bartoli, M., Caldwell, R. W., and Hartnett, M. E. (2008). Vascular Endothelial Growth Factor in Eye Disease. Prog. Retin. Eye Res. 27, 331–371. doi:10.1016/j.preteyeres.2008.05.001
Phipson, B., Lee, S., Majewski, I. J., Alexander, W. S., and Smyth, G. K. (2016). Robust Hyperparameter Estimation Protects Against Hypervariable Genes and Improves Power to Detect Differential Expression. Ann. Appl. Stat. 10, 946–963. doi:10.1214/16-AOAS920
Ponomarev, E. D., Shriver, L. P., and Dittel, B. N. (2006). CD40 Expression by Microglial Cells Is Required for Their Completion of a Two-Step Activation Process during Central Nervous System Autoimmune Inflammation. J. Immunol. 176, 1402–1410. doi:10.4049/jimmunol.176.3.1402
Qin, D., Zhang, G.-M., Xu, X., and Wang, L.-Y. (2015). The PI3K/Akt Signaling Pathway Mediates the High Glucose-Induced Expression of Extracellular Matrix Molecules in Human Retinal Pigment Epithelial Cells. J. Diabetes Res. 2015, 1–11. doi:10.1155/2015/920280
Rego, A. C., Areias, F. M., Santos, M. S., and Oliveira, C. R. (1998). Effect of Glucose Deprivation and Acute Glutamate Exposure in Cultured Retinal Cells. Exp. Neurol. 153, 128–134. doi:10.1006/exnr.1998.6865
Rheaume, B. A., Jereen, A., Bolisetty, M., Sajid, M. S., Yang, Y., Renna, K., et al. (2018). Single Cell Transcriptome Profiling of Retinal Ganglion Cells Identifies Cellular Subtypes. Nat. Commun. 9, 2759. doi:10.1038/s41467-018-05134-3
Robinson, M. D., McCarthy, D. J., and Smyth, G. K. (2010). edgeR: A Bioconductor Package for Differential Expression Analysis of Digital Gene Expression Data. Bioinformatics 26, 139–140. doi:10.1093/bioinformatics/btp616
Roesch, K., Jadhav, A. P., Trimarchi, J. M., Stadler, M. B., Roska, B., Sun, B. B., et al. (2008). The Transcriptome of Retinal Müller Glial Cells. J. Comp. Neurol. 509, 225–238. doi:10.1002/cne.21730
Rueda, E. M., Johnson, J. E., Giddabasappa, A., Swaroop, A., Brooks, M. J., Sigel, I., et al. (2016). The Cellular and Compartmental Profile of Mouse Retinal Glycolysis, Tricarboxylic Acid Cycle, Oxidative Phosphorylation, and ∼P Transferring Kinases. Mol. Vis. 22, 847–885.
Ruta, L. M., Magliano, D. J., LeMesurier, R., Taylor, H. R., Zimmet, P. Z., and Shaw, J. E. (2013). Prevalence of Diabetic Retinopathy in Type 2 Diabetes in Developing and Developed Countries. Diabet. Med. 30, 387–398. doi:10.1111/dme.12119
Sanes, J. R., and Masland, R. H. (2015). The Types of Retinal Ganglion Cells: Current Status and Implications for Neuronal Classification. Annu. Rev. Neurosci. 38, 221–246. doi:10.1146/annurev-neuro-071714-034120
Sanes, J. R., and Zipursky, S. L. (2010). Design Principles of Insect and Vertebrate Visual Systems. Neuron 66, 15–36. doi:10.1016/j.neuron.2010.01.018
Sapieha, P., Hamel, D., Shao, Z., Rivera, J. C., Zaniolo, K., Joyal, J. S., et al. (2010). Proliferative Retinopathies: Angiogenesis that Blinds. Int. J. Biochem. Cel Biol. 42, 5–12. doi:10.1016/j.biocel.2009.10.006
Sasore, T., Reynolds, A. L., and Kennedy, B. N. (2014). “Targeting the PI3K/Akt/mTOR Pathway in Ocular Neovascularization,” in Retinal Degenerative Diseases Advances in Experimental Medicine and Biology. Editors J. D. Ash, C. Grimm, J. G. Hollyfield, R. E. Anderson, M. M. LaVail, and C. Bowes Rickman (New York, NY: Springer), 805–811. doi:10.1007/978-1-4614-3209-8_101
Schlüter, A., Aksan, B., Diem, R., Fairless, R., and Mauceri, D. (2020). VEGFD Protects Retinal Ganglion Cells and, Consequently, Capillaries against Excitotoxic Injury. Mol. Ther. - Methods Clin. Develop. 17, 281–299. doi:10.1016/j.omtm.2019.12.009
Seung, H. S., and Sümbül, U. (2014). Neuronal Cell Types and Connectivity: Lessons from the Retina. Neuron 83, 1262–1272. doi:10.1016/j.neuron.2014.08.054
Shekhar, K., Lapan, S. W., Whitney, I. E., Tran, N. M., Macosko, E. Z., Kowalczyk, M., et al. (2016). Comprehensive Classification of Retinal Bipolar Neurons by Single-Cell Transcriptomics. Cell 166, 1308–1323.e30. doi:10.1016/j.cell.2016.07.054
Shen, W., Li, S., Chung, S. H., and Gillies, M. C. (2009). Retinal Vascular Changes after Glial Disruption in Rats. J. Neurosci. Res. 88, 1485–1499. doi:10.1002/jnr.22317
Singh, N. K., Kotla, S., Kumar, R., and Rao, G. N. (2015). Cyclic AMP Response Element Binding Protein Mediates Pathological Retinal Neovascularization via Modulating DLL4-NOTCH1 Signaling. EBioMedicine 2, 1767–1784. doi:10.1016/j.ebiom.2015.09.042
Slenter, D. N., Kutmon, M., Hanspers, K., Riutta, A., Windsor, J., Nunes, N., et al. (2018). WikiPathways: A Multifaceted Pathway Database Bridging Metabolomics to Other Omics Research. Nucleic Acids Res. 46, D661–D667. doi:10.1093/nar/gkx1064
Solomon, S. D., Chew, E., Duh, E. J., Sobrin, L., Sun, J. K., VanderBeek, B. L., et al. (2017). Diabetic Retinopathy: A Position Statement by the American Diabetes Association. Dia Care 40, 412–418. doi:10.2337/dc16-2641
Soneson, C., Love, M. I., and Robinson, M. D. (2015). Differential Analyses for RNA-Seq: Transcript-Level Estimates Improve Gene-Level Inferences. F1000Res 4, 1521. doi:10.12688/f1000research.7563.1
Struebing, F. L., Lee, R. K., Williams, R. W., and Geisert, E. E. (2016). Genetic Networks in Mouse Retinal Ganglion Cells. Front. Genet. 7, 169. doi:10.3389/fgene.2016.00169
Sun, L., Shukair, S., Naik, T. J., Moazed, F., and Ardehali, H. (2008). Glucose Phosphorylation and Mitochondrial Binding Are Required for the Protective Effects of Hexokinases I and II. Mol. Cel Biol 28, 1007–1017. doi:10.1128/MCB.00224-07
Sundstrom, J. M., Hernández, C., Weber, S. R., Zhao, Y., Dunklebarger, M., Tiberti, N., et al. (2018). Proteomic Analysis of Early Diabetic Retinopathy Reveals Mediators of Neurodegenerative Brain Diseases. Invest. Ophthalmol. Vis. Sci. 59, 2264–2274. doi:10.1167/iovs.17-23678
Swan, R., Kim, S. J., Campbell, J. P., Chan, R. V. P., Taylor, K. D., Li, X., et al. (2018). The Genetics of Retinopathy of Prematurity: A Model for Neovascular Retinal Disease. Ophthalmol. Retina 2, 949–962. doi:10.1016/j.oret.2018.01.016
Tasic, B., Menon, V., Nguyen, T. N., Kim, T. K., Jarsky, T., Yao, Z., et al. (2016). Adult Mouse Cortical Cell Taxonomy Revealed by Single Cell Transcriptomics. Nat. Neurosci. 19, 335–346. doi:10.1038/nn.4216
The Gene Ontology Consortium (2017). Expansion of the Gene Ontology Knowledgebase and Resources. Nucleic Acids Res. 45, D331–D338. doi:10.1093/nar/gkw1108
Tian, J.-Y., Cheng, Q., Song, X.-M., Li, G., Jiang, G.-X., Gu, Y.-Y., et al. (2006). Birth Weight and Risk of Type 2 Diabetes, Abdominal Obesity and Hypertension Among Chinese Adults. Eur. J Endocrinol. 155, 601–607. doi:10.1530/eje.1.02265
Vaiserman, A., and Lushchak, O. (2019). Developmental Origins of Type 2 Diabetes: Focus on Epigenetics. Ageing Res. Rev. 55, 100957. doi:10.1016/j.arr.2019.100957
Vecino, E., Rodriguez, F. D., Ruzafa, N., Pereiro, X., and Sharma, S. C. (2016). Glia-Neuron Interactions in the Mammalian Retina. Prog. Retin. Eye Res. 51, 1–40. doi:10.1016/j.preteyeres.2015.06.003
Vellanki, S., Ferrigno, A., Alanis, Y., Betts-Obregon, B. S., and Tsin, A. T. (2016). High Glucose and Glucose Deprivation Modulate Müller Cell Viability and VEGF Secretion. Int. J. Ophthalmol. Eye Sci. 4, 178–183. doi:10.19070/2332-290X-1900080
Vlasits, A. L., Bos, R., Morrie, R. D., Fortuny, C., Flannery, J. G., Feller, M. B., et al. (2014). Visual Stimulation Switches the Polarity of Excitatory Input to Starburst Amacrine Cells. Neuron 83, 1172–1184. doi:10.1016/j.neuron.2014.07.037
Walter, W., Sánchez-Cabo, F., and Ricote, M. (2015). GOplot: an R Package for Visually Combining Expression Data with Functional Analysis: Fig. 1. Bioinformatics 31, 2912–2914. doi:10.1093/bioinformatics/btv300
Weh, E., Lutrzykowska, Z., Smith, A., Hager, H., Pawar, M., Wubben, T. J., et al. (2020). Hexokinase 2 Is Dispensable for Photoreceptor Development but Is Required for Survival during Aging and Outer Retinal Stress. Cell Death Dis 11, 1–15. doi:10.1038/s41419-020-2638-2
Welby, E., Lakowski, J., Di Foggia, V., Budinger, D., Gonzalez-Cordero, A., Lun, A. T. L., et al. (2017). Isolation and Comparative Transcriptome Analysis of Human Fetal and iPSC-Derived Cone Photoreceptor Cells. Stem Cel Rep. 9, 1898–1915. doi:10.1016/j.stemcr.2017.10.018
Wickham, H. (2009). Ggplot2: Elegant Graphics for Data Analysis. 2nd ed. New York, NY: Springer Publishing Company, Incorporated.
Winkler, E. A., Bell, R. D., and Zlokovic, B. V. (2010). Pericyte-specific Expression of PDGF Beta Receptor in Mouse Models with normal and Deficient PDGF Beta Receptor Signaling. Mol. Neurodegeneration 5, 32. doi:10.1186/1750-1326-5-32
Wong, T. Y., Sun, J., Kawasaki, R., Ruamviboonsuk, P., Gupta, N., Lansingh, V. C., et al. (2018). Guidelines on Diabetic Eye Care. Ophthalmology 125, 1608–1622. doi:10.1016/j.ophtha.2018.04.007
Wu, F., Li, R., Umino, Y., Kaczynski, T. J., Sapkota, D., Li, S., et al. (2013). Onecut1 Is Essential for Horizontal Cell Genesis and Retinal Integrity. J. Neurosci. 33, 13053–13065. doi:10.1523/JNEUROSCI.0116-13.2013
Yang, X. (2004). Characterization of Receptors for Glutamate and GABA in Retinal Neurons. Prog. Neurobiol. 73, 127–150. doi:10.1016/j.pneurobio.2004.04.002
Yates, A., Akanni, W., Amode, M. R., Barrell, D., Billis, K., Carvalho-Silva, D., et al. (2016). Ensembl 2016. Nucleic Acids Res. 44, D710–D716. doi:10.1093/nar/gkv1157
Yu, Q. C., Song, W., Wang, D., and Zeng, Y. A. (2016). Identification of Blood Vascular Endothelial Stem Cells by the Expression of Protein C Receptor. Cell Res 26, 1079–1098. doi:10.1038/cr.2016.85
Zeisel, A., Muñoz-Manchado, A. B., Codeluppi, S., Lönnerberg, P., La Manno, G., Juréus, A., et al. (2015). Cell Types in the Mouse Cortex and hippocampus Revealed by Single-Cell RNA-Seq. Science 347, 1138–1142. doi:10.1126/science.aaa1934
Zeng, H., and Sanes, J. R. (2017). Neuronal Cell-Type Classification: Challenges, Opportunities and the Path Forward. Nat. Rev. Neurosci. 18, 530–546. doi:10.1038/nrn.2017.85
Keywords: transcriptomics, retinogenesis, starvation, neurovascular unit, retinopathy, transcriptomic (RNA-seq)
Citation: Özgümüs T, Sulaieva O, Jain R, Artner I and Lyssenko V (2021) Starvation to Glucose Reprograms Development of Neurovascular Unit in Embryonic Retinal Cells. Front. Cell Dev. Biol. 9:726852. doi: 10.3389/fcell.2021.726852
Received: 17 June 2021; Accepted: 25 October 2021;
Published: 18 November 2021.
Edited by:
Roland Wohlgemuth, Lodz University of Technology, PolandReviewed by:
Yehong Du, Chongqing Medical University, ChinaMaria Cecilia Sanchez, CONICET Centre for Research in Clinical Biochemistry and Immunology (CIBICI), Argentina
Copyright © 2021 Özgümüs, Sulaieva, Jain, Artner and Lyssenko. This is an open-access article distributed under the terms of the Creative Commons Attribution License (CC BY). The use, distribution or reproduction in other forums is permitted, provided the original author(s) and the copyright owner(s) are credited and that the original publication in this journal is cited, in accordance with accepted academic practice. No use, distribution or reproduction is permitted which does not comply with these terms.
*Correspondence: Valeriya Lyssenko, dmFsZXJpeWEubHlzc2Vua29AdWliLm5v