Early-Onset Preeclampsia Is Associated With Gut Microbial Alterations in Antepartum and Postpartum Women
- 1The First Affiliated Hospital of Jinan University, Guangzhou, China
- 2Medical Genetic Center, Guangdong Women and Children Hospital, Guangzhou, China
- 3Promegene Institute, Shenzhen, China
- 4Department of Obstetrics, Guangdong Women and Children Hospital, Guangzhou, China
- 5Tianjin Key Laboratory of Cardiovascular Remodeling and Target Organ Injury, Pingjin Hospital Heart Center, Tianjin, China
- 6Public Health Sciences Division, Fred Hutchinson Cancer Research Center, Seattle, WA, United States
Background: Imbalances in gut microbiota composition are linked to hypertension, host metabolic abnormalities, systemic inflammation, and other conditions. In the present study, we examined the changes of gut microbiota in women with early-onset preeclampsia (PE) and in normotensive, uncomplicated pregnant women during late pregnancy and at 1 and 6 weeks postpartum.
Methods: Gut microbiota profiles of women with PE and healthy pregnant women in the third trimester and at 1 and 6 weeks postpartum were assessed by 16S rRNA gene amplicon sequencing. Plasma levels of interleukin-6 (IL-6), intestinal fatty acid-binding protein (I-FABP), zonulin, and lipopolysaccharide (LPS) were measured in the third trimesters.
Results: At the genus level, 8 bacterial genera were significantly enriched in the antepartum samples of PE patients compared to healthy controls, of which Blautia, Ruminococcus2, Bilophila, and Fusobacterium represented the major variances in PE microbiomes. Conversely, 5 genera, including Faecalibacterium, Gemmiger, Akkermansia, Dialister, and Methanobrevibacter, were significantly depleted in antepartum PE samples. Maternal blood pressure and liver enzyme levels were positively correlated to the PE-enriched genera such as Anaerococcus, Ruminococcus2, Oribacterium, and Bilophila, while the fetal features (e.g., Apgar score and newborn birth weight) were positively correlated with PE-depleted genera and negatively correlated with PE-enriched genera. Moreover, maternal blood IL-6 level was positively associated with gut Bilophila and Oribacterium, whereas LPS level was negatively associated with Akkermansia. In terms of postpartum women, both the gut microbial composition and the PE-associated microbial alterations were highly consistent with those of the antepartum women.
Conclusion: PE diagnosed in the third trimester of pregnancy is associated with a disrupted gut microbiota composition compared with uncomplicated pregnant women, which are associated with maternal clinical features (blood pressure level and liver dysfunction) and newborn birth weight. Moreover, these antepartum alterations in gut microbiota persisted 6 weeks postpartum.
Introduction
Preeclampsia (PE), the second leading cause of maternal mortality worldwide (Huppertz, 2008; Ghulmiyyah and Sibai, 2012; Mol et al., 2016), is characterized by severe hypertension and multiple organ damage (Brown et al., 2018), and it can result in fetal intrauterine growth retardation, premature birth, or fetal death (Kovo et al., 2015). Untreated preeclampsia can be lethal with complications such as eclampsia, liver rupture, stroke, and kidney failure (Souza et al., 2013). In 2010, preeclampsia complicated 3–5% of pregnancies in Western countries and a higher percentage in low- and middle-income countries (Hutcheon et al., 2011; Ananth et al., 2013). Early-onset preeclampsia, which usually occurs between 20 and 34 weeks of gestation, leads to approximately 4 times more maternal death (Lisonkova et al., 2014) and 8 times more perinatal death or severe neonatal morbidity compared with mothers without preeclampsia (Lisonkova and Joseph, 2013; Khader et al., 2018). The specific etiology of preeclampsia is still unclear, and the combination of known risk factors for preeclampsia (such as women's age, body weight, previous preeclampsia, gestational hypertension, and first pregnancy) can only predict 30% of women who develop preeclampsia in clinical practice (Leslie et al., 2011; Mol et al., 2016).
Gut microbiota has profound effects on regulating host metabolism (Pedersen et al., 2016; Liu R. et al., 2017). It also plays an important role in blood pressure elevation during pregnancies (the hallmark of preeclampsia) (Gomez-Arango et al., 2016a). However, the interconnection between gut microbiota and preeclampsia is still unknown. Byproducts of gut microbial metabolism such as formate, hydrogen sulfate and toxic molecules (e.g., trimethylamine N-oxide), can directly induce elevation in blood pressure (Holmes et al., 2008; Tang et al., 2015; Tomasova et al., 2016). In contrast, the microbial flora generated short chain fatty acids can affect immune, epithelial, nervous system, and blood vessel functions to modulate blood pressure (Krautkramer et al., 2016; Schiering et al., 2017; Yan et al., 2017). Increasing evidence has revealed that gut microbiota is crucial to the development and maturation of host immune components, such as gut-associated lymphoid tissue and immunocytes (Kamada et al., 2013; Pickard et al., 2017), and also contributes to immune responses and to inhibiting inflammation (Wesemann et al., 2013). Notably, the mucosal surfaces of the gut experience low-grade inflammation with rising levels of pro-inflammatory cytokines and white blood cells as pregnancy advances, which also contributes to disturbances in intestinal flora (Koren et al., 2012). The above immune responses play a leading role in two main pathophysiological processes occurring in PE patients: (1) poor trophoblastic invasion resulted from the altered production of immunoregulatory cytokines and angiogenic factors and (2) a systemic inflammatory response (Laresgoiti-Servitje, 2013). Thus, these evidences highlight the potential and important association between gestational gut microbiota and preeclampsia.
A recent study suggested a significant shift of gut microbial composition in PE patients in late pregnancy (Liu J. et al., 2017); however, their findings remain limited due to the limitation of sample size and the lack of longitudinal investigation. Here, we conducted 16S rRNA gene sequencing to analyze the gut microbiota of 101 fecal samples of PE patients and 79 samples of healthy controls, separately in antepartum, 1 and 6 weeks postpartum. We identified the bacterial taxa associated with preeclampsia and revealed dynamic changes in the gut microbiota of patients from late pregnancy to postpartum. We also detected potential correlations between preeclampsia-associated microbes and host clinical characteristics, providing pieces for understanding the underlying mechanisms of preeclampsia.
Methods
Ethics Statement
This study was approved by the Ethics Committee of Guangdong Women and Children Hospital, and informed consent was obtained from all subjects in accordance with the Declaration of Helsinki (World Medical Association, 2013).
Study Cohort and Sample Collection
All pregnant women who planned to delivery at Guangdong Women and Children Hospital from January 2017 to December 2017, were recruited for our study. After consenting and excluding those with comorbidities, multiple pregnancies, gestational diabetes and chronic hypertension, 150 singleton pregnant women with a live birth were included for analysis. In total, 78 cases newly diagnosed with preeclampsia with severe effect in their third trimesters were categorized as the PE group, while 72 normotensive and uncomplicated women were designated as the normal controls (NC group). All enrolled participants were re-invited for a follow-up visit on an average of 1 and 6 weeks postpartum. At last, a total of 101 fecal samples of the PE group (number of samples: n = 48, 35, and 18 at antepartum, 1 and 6 weeks postpartum, respectively) and 79 samples of the NC group (n = 51, 17, and 11 at the above three time points, respectively) were collected. The phenotypic characteristics of the participants are summarized in Table 1, and detailed information is given in Table S1. No smoking among participants and their family members during pregnancy was reported. All participants were of Han nationality, the ethnic majority in China. Antenatal and postnatal clinical information and pregnancy outcomes were measured and collected by well-trained staffs according to standard procedures (see below sections for details).
The first fecal specimens (antepartum) were collected after hospitalization but before childbirth. All pregnant women delivered by cesarean section. At 1 and 6 weeks postpartum, fecal samples were collected at home by the participants, following a standardized procedure including antiseptic handling, collection in sterile tubes and immediate freezing at −20°C. The samples were then transferred to the laboratory immediately on ice and stored at −80°C until DNA extraction.
Biochemistry and Derived Traits
Prepregnancy body weight was extracted from their pregnancy health records. Body mass index (BMI) was calculated by dividing the weight in kilograms by the square of height in meters. Office blood pressure during antepartum evaluations was measured by trained research nurses using a mercury sphygmomanometer with an appropriate cuff size, auscultating the Korotkoff sounds with the participant in the sitting position (Lei et al., 2017). Office hypertension was defined by a systolic blood pressure (SBP) ≥ 140 mmHg and/or diastolic blood pressure (DBP) ≥ 90 mmHg on three visits or by current treatment with antihypertensive medications. Preeclampsia was diagnosed according to the current guidelines (American College of Obstetricians Gynecologists Task Force on Hypertension in Pregnancy, 2013): (1) SBP/DBP ≥ 140/90 mmHg on two occasions for at least 4 h with previously normal blood pressure; (2) proteinuria ≥ 300 mg/24-h urine collection; and (3) in the absence of proteinuria, new onset of any of the following: platelet count < 100,000/μL; serum creatinine concentration > 1.1 mg/dL or a doubling in the absence of other renal disease; elevated blood concentrations of liver transaminases to twice normal concentration; pulmonary edema; and cerebral or visual symptoms. Additionally, preeclampsia with severe features was diagnosed with any of the following findings: SBP/DBP ≥ 160/110 mmHg on two occasions at least 4 h apart; platelet count < 100,000/μL; elevated blood concentrations of liver transaminases to twice normal concentration; severe persistent right upper quadrant or epigastric pain; serum creatinine concentration > 1.1 mg/dL or a doubling in the absence of other renal disease; pulmonary edema; and new-onset cerebral or visual disturbances.
Fasting blood and 24-h urine samples in parallel to the BP monitoring were performed. Biochemical measurements included fasting plasma glucose level, plasma levels of total cholesterol, high density lipoprotein cholesterol (HDL), low density lipoprotein cholesterol (LDL), triglycerides, creatinine, 24-h urinary protein excretion, interleukin-6 (IL-6), intestinal fatty acid-binding protein (I-FABP), zonulin, and lipopolysaccharide (LPS). In the second visit, which was performed 1 week after childbirth, the subjects' body weight, blood pressure and diet questionnaire were also recorded. Plasma IL-6 (Thermo/eBiosecience) concentrations were quantified using a double antibody sandwich enzyme-linked immunosorbent assay (ELISA). Plasma I-FABP (R&D Systems) concentrations were quantified by specific ELISA. Zonulin in plasma was estimated by competitive ELISA (Alpha Diagnoestic International). Plasma LPS (antibodies online) was estimated by sandwich ELISA.
DNA Extraction and 16S rRNA Gene Sequencing
The microbial genomic DNA was extracted according to the MOBIO PowerSoil® DNA Isolation Kit 12888-100 protocol, and DNA was stored at −80°C in Tris-EDTA buffer solution before use. To enable amplification of the V4 region of the 16S rRNA gene and add barcode sequences, unique fusion primers were designed based on the universal primers set, 515F (5′-GTGYCAGCMGCCGCGGTAA-3′) and 806R (5′-GGACTACNVGGGTWTCTAAT-3′), along with barcode sequences. PCR mixtures contained 1 μL of each forward and reverse primer (10 μM), 1 μL of template DNA, 4 μL of dNTPs (2.5 mM), 5 μL of 10 × EasyPfu Buffer, 1 μL of Easy Pfu DNA Polymerase (2.5 U/μL), and 1 μL of double distilled water in a 50 μL reaction volume. Thermal cycling consisted of an initial denaturation step at 95°C for 5 min, followed by 30 cycles of denaturation at 94°C for 30 s, annealing at 60°C for 30 s, and extension at 72°C for 40 s, with a final extension step at 72°C for 4 min. Amplicons from each sample were run on an agarose gel. The expected band size for 515F-806R is ~300–350 bp. Amplicons were quantified with the Quant-iT PicoGreen dsDNA Assay Kit (ThermoFisher/ Invitrogen cat. no. P11496; following the manufacturer's instructions).
The amplicon library was combined in equal amount and subsequently quantified (KAPA Library Quantification Kit KK4824) according to the manufacturer's instructions, and high-throughput sequencing on the Illumina MiniSeq platform at Promegene Co. Ltd. (Shenzhen, China) was performed to generate 150 bp paired-end reads (exclude the primer sequences) for each sample.
Microbiome Analyses
Raw sequencing reads were eliminated from analysis if they produce >8 homopolymers, >2 mismatches in the primers, or >1 mismatches in the barcode. High-quality paired-end sequencing reads were analyzed based on the quantitative insights into microbial ecology (QIIME2, https://qiime2.org/) platform (Kuczynski et al., 2012), and the standard tools/plugins provided by QIIME2. First, raw 16S sequences were performed for quality control and to feature table construction using the DADA2 algorithm (Callahan et al., 2016). Possible phiX reads and chimeric sequences were removed, and the remaining reads were truncated from 0 to 140 bases (for both forward and reverse reads) to avoid the sequencing errors at the end of the reads. Paired-end reads were overlapped at the maximum mismatch parameter of 6 bases, which means a minimum similarity threshold of 90% on the overlap zone of the forward and reverse reads. The representative sequences (named “feature” in QIIME2 nomenclature) were then generated by removing the redundant and low occurrence (n < 5 in pool samples) sequences. We used the term “operational taxonomic unit (OTU)” instead of “feature” in the whole article for convenience. Then, taxonomic assignment of the OTUs were determined based on a pretrained Naive Bayes classifier (trained on the Greengenes 13_8 99% OTUs DeSantis et al., 2006) via the q2-feature-classifier plugin, and the taxonomic composition at the phylum, class, order, family, genus, and species levels were generated based on OTU annotation. To avoid sampling depth bias, 20,000 reads were randomly selected from each sample when calculating the OTU and taxa relative abundances.
Phylogenetic analyses were realized via the q2-phylogeny plugin, which performed multiple sequence alignment on the OTU sequences and generated phylogenetic trees of the OTUs from the alignment result. Four estimators of the alpha diversity, including Shannon's diversity index, observed OTUs, Faith's phylogenetic diversity (a qualitative measure of community richness that incorporates the phylogenetic relationships between the OTUs) and Pielou's evenness, and four estimator of the beta diversity, including Jaccard distance, Bray-Curtis distance, unweighted UniFrac distance, and weighted UniFrac distance, were used in this study and calculated based on the QIIME2 q2-diversity plugin.
Enterotype of the fecal samples were determined based on their genus level composition using a reference-based alignment algorithm (http://enterotypes.org/) (Costea et al., 2018).
Functional composition of the samples was generated using the PICRUSt2 algorithm (Langille et al., 2013). For each sample, the composition of the Kyoto Encyclopedia of Genes and Genomes (KEGG) (Kanehisa et al., 2017) orthologs (KOs) was predicted based on the functional information of the reference OTUs. KEGG modules and pathways composition were generated according to the assignment of KOs at https://www.kegg.jp/.
Statistical Analyses
Statistical analyses were implemented at the R v3.3.2 platform (https://www.r-project.org/). Permutational multivariate analysis of variance (effect size analysis) was performed with the adonis function of the R vegan package (https://cran.r-project.org/web/packages/vegan/index.html), and the adonis P-value was generated based on 1,000 permutations. Distance-based redundancy analysis (dbRDA) was performed on the OTUs and taxonomic composition profiles with the vegan package, based on the Bray-Curtis distance, and visualized via the R ade4 package. The PE-associated OTUs and taxa were identified based on the Wilcoxon rank-sum test. Random forest models were analyzed with the R randomForest package (1,000 trees). The performance of the predictive model was evaluated with the leave-one-out cross validation. Receiver operator characteristic (ROC) analysis was performed using the R pROC package. The ROC curve was created by plotting the true positive rate (sensitivity) against the false positive rate (1- sensitivity), and the area under the curve (AUC) was calculated to assess the ensemble. Procrustes analysis was performed with the R vegan package, and the Procrustes P-value was generated based on 1,000 permutations. P-value < 0.05 was considered statistically significant. The q was used to evaluate the false discovery rate for correction of multiple comparisons, and was calculated based on the R fdrtool package.
Results
Study Cohort and Sequencing Summary
To evaluate the associations between PE and the composition of women's gut microbiome in the perinatal/postnatal period, we analyzed the fecal samples of PE patients and healthy controls at antepartum (n = 48 vs. 51), 1 week postpartum (n = 35 vs. 17), and 6 weeks postpartum (n = 18 vs. 11). Patients and controls were matched according their body weight parameters including prepregnancy weight, antenatal weight, height and BMI at each time point (Table 1); on average, the PE patients were 3 years older than the controls (P < 0.05 at 1 and 6 weeks postpartum). PE patients and healthy controls were differed in their PE-associated clinical status at antepartum (Table 1) and after delivery (Table S1). Based on their self-reports and questionnaires, all participants were similar in their dietary habit and lifestyle (data not shown). To avoid the effect of delivery mode on gut microbiota, only pregnant women with cesarean section were included in this study.
The gut microbiotas of 180 fecal samples of pregnant women were characterized by sequencing the V4 variable region of the bacterial 16S rRNA gene, generating a total of 8,136,758 high quality sequences (45,204 ± 11,017 per sample; Table S1). A total of 2,293 OTUs were identified and taxonomically annotated based on an open source, universal microbiome bioinformatics platform, QIIME2 (Kuczynski et al., 2012). Of which, 74% could be annotated into specific genus (representing 81% of total sequences; Figure 1A), and 68% could be annotated into specific species (representing 77% of total sequences).
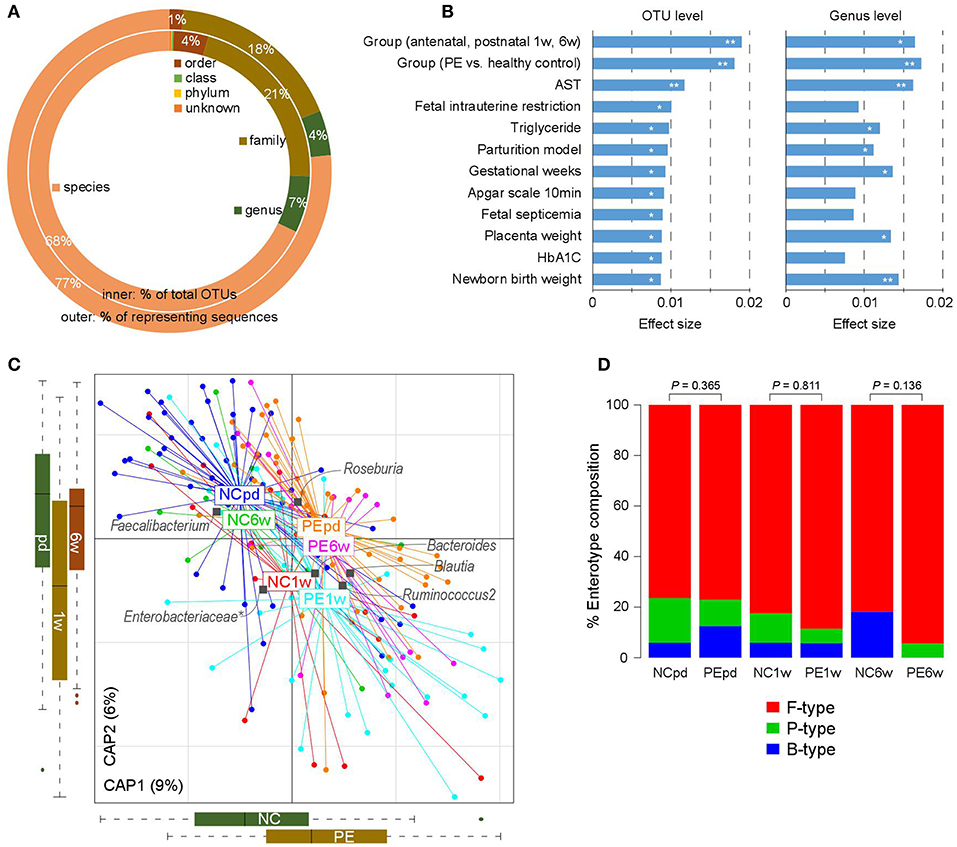
Figure 1. Overview of the gut microbial community in PE patients and healthy controls. (A) Summary of the taxonomic assignment of the OTUs. Inner circle, the percentage of OTUs that assigned into a taxon at the species, genus, family, order, class, and phylum levels. Outer circle, the percentage of representing sequences of the OTUs at all taxonomic levels. (B) Host factors that significantly affected the gut microbiota. The 12 factors associated with the variation of the gut microbiota at the OTU level are shown. Bar plots indicate the explained variation (effect size) of each factor at the OTU level (left panel) and genus level (right panel). **, permutated P < 0.01; *, permutated P < 0.05. (C) dbRDA plot based on the Bray-Curtis distances between microbial genera, revealing PE microbial dysbiosis at the antepartum, 1 and 6 weeks postpartum periods. Patient and control samples were mainly separated in the primary constrained axis (PE separation), and samples at different time points were mainly separated in the second constrained axis (time point separation). Lines connect samples (colored points) in the same group, and circles cover samples near the center of gravity for each group. Genera (yellow squares) as the main contributors are plotted by their loadings in these two components. (D) Constitution of enterotypes in all groups. P-values between PE patients and healthy controls at three time points were calculated based on Fisher's exact test.
Diversity and Overall Structure of Gut Microbiota
The intrasample richness and intersample relationship of the gut microbiota of all participates were assessed by alpha and beta diversity indexes, respectively. No significant differences in alpha diversity were detected during the antepartum and postpartum periods (P > 0.05 for all four indexes of alpha diversity; Figure S1). Similarly, the beta diversity between different groups did not differ significantly (P > 0.05 for all four indexes of beta diversity).
We then tested if PE was associated with the holistic community structure over the entire cohort. PE accounted for 1.8% (adonis P < 0.001) and 1.7% (adonis P = 0.002) of the gut microbiota variance at the OTU and genus levels, respectively. This effect size was relatively larger than other collected confounding factors, including the intrinsic parameters of pregnant women, fetal features, and antenatal clinical immune indexes (Figure 1B; Table S2), indicating that PE stratification was one of the main reasons in our cohort. Likewise, the grouping of antenatal, 1 and 6 weeks postpartum also accounted for an approximate proportion of microbial variances (1.9% at the OTU level and 1.6% at the genus level) with PE.
Bray-Curtis distance based redundancy analysis (dbRDA, analyzed at the genus level) captured visible separation of both PE stratification and sample time grouping on the overall gut microbiota (Figure 1C). PE significantly acted on the primary constrained axis of the dbRDA plot, while the genera Faecalibacterium, Blautia, and Ruminococcus2 represented the major contributors in the axis. Likewise, sample time grouping affected the second constrained axis, while Roseburia, Enterobacteriaceae, and Ruminococcus2 were the major contributors. Noticeably, despite the samples at 1 week postpartum were separated with others, the antenatal and 6 weeks postpartum samples were closely related in the second constrained axis, suggesting a remarkable shift of gut microbiota at 1 week postpartum but the majority recovered by 6 weeks postpartum.
The primary structure of the human gut microbiome is described by enterotypes (Costea et al., 2018). In our samples, the enterotype stratification was clearly driven by the abundance of several dominate genera such as Bacteroides (B-type), Prevotella (P-type), and Ruminococcus (F-type). PE patients exhibited consistent enterotype patterns with healthy controls from late pregnancy to postpartum (Figure 1D), despite the PE samples seeming to have a slight increase of F-type and decrease of B-type.
Microbial Taxa Signatures
We compared the gut microbial composition of PE patients and healthy controls at each time point to investigate microbial signatures of PE. At the phylum level, PE patients had a similar composition in the dominant phyla compare to the healthy controls, except several low abundant phyla (Fusobacteria, Tenericutes, and Verrucomicrobia) were significantly depleted in PE patients at the antenatal time point (Table S3). At the genus level, 8 genera were significant enriched in antenatal PE samples (Figure 2A), of which Blautia and Ruminococcus2, followed by Bilophila and Fusobacterium represented the major variances in PE microbiomes. The main species-level members of these genera, including an unclassified Blautia spp. (consisted 84.9% of Blautia), R. gnavus (consisted 54.7% of Ruminococcus2), B. wadsworthia (consisted 100% of Bilophila), and F. nucleatum (consisted 100% of Fusobacterium), were also significantly increased in PE patients (Table S3). Inversely, 5 genera, including Faecalibacterium, Gemmiger, Akkermansia, Dialister, and Methanobrevibacter, were significantly depleted in antenatal PE samples, which mostly consisted of the species F. prausnitzii (100%), G. formicilis (100%), A. muciniphila (100%), an unclassified Dialister spp. (100%), and M. smithii (100%), respectively. Moreover, several genera and species were significantly altered between the PE and control microbiota at 1 and 6 weeks postpartum (Table S3).
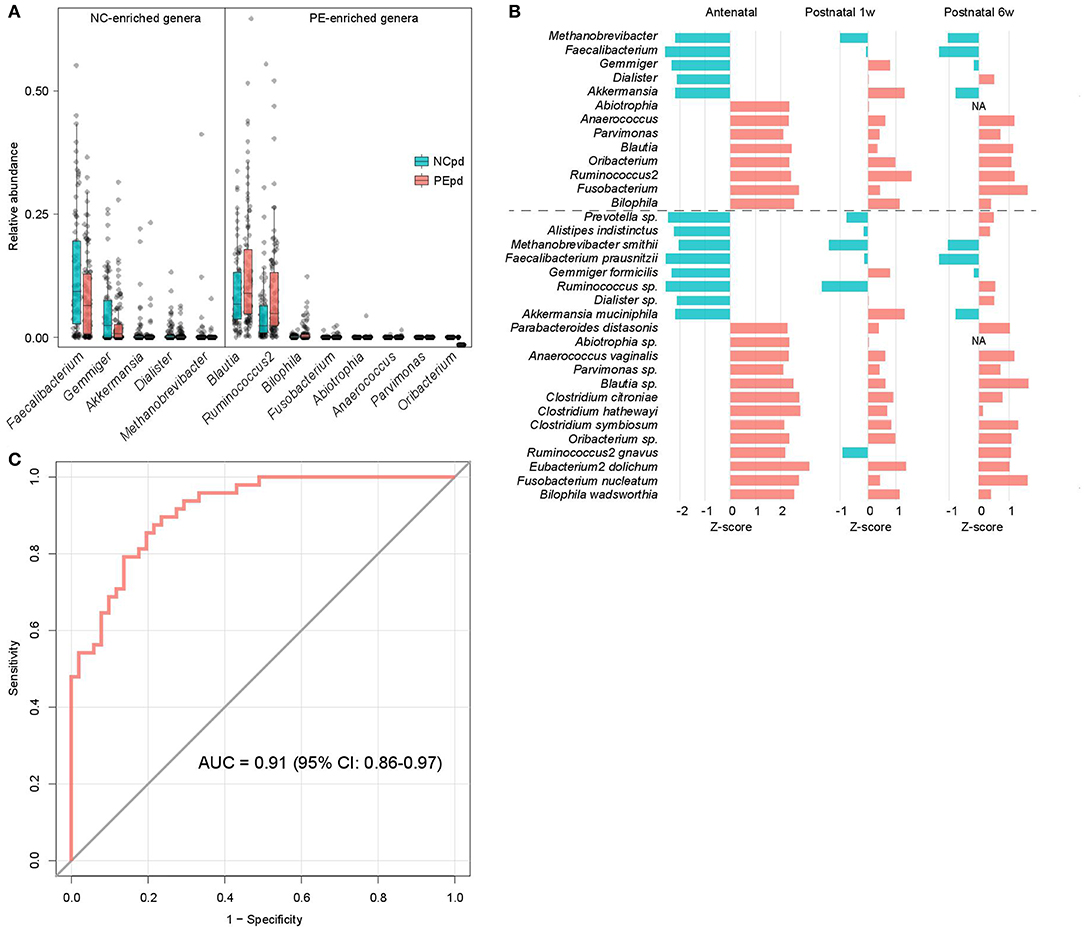
Figure 2. Difference of gut microbiota between PE patients and healthy controls. (A) Boxplot shows the significantly different genera between patients and controls. Genera with P < 0.05 (Wilcoxon rank-sum test) of samples at antepartum are shown. The boxes represent the interquartile range (IQR) between the first and third quartiles and the line inside represents the median. The whiskers denote the lowest and highest values within 1.5 times IQR from the first and third quartiles, respectively. (B) The PE-associated genera and species of samples at antepartum, and their tendencies in samples at 1 and 6 weeks postpartum. The bar lengths indicate the Z-score of a genus or species at different time points, and the colors represent enrichment in patients (red, Z-score > 0) or controls (blue, Z-score < 0). (C) ROC analysis for classification of PE status by the relative abundances of PE-associated genera, assessed by AUC.
The majority of taxonomic alterations in antenatal PE microbiota pertains to 1 or 6 weeks postpartum when 79.4% and 84.4% of antenatal-altered clades (at the genus and species levels) were within concordant tendency in samples of 1 and 6 weeks postpartum samples (Figure 2B), respectively. This finding indicated that the antenatal-altered taxa might be reliable microbial markers for PE across the antenatal and postnatal stages.
Using the random forest model, we evaluated the performance of gut microbial composition to predict PE status based on the relative abundance of 13 antenatal-altered genera. The model achieved an AUC of 0.91 (95% confidence interval, 0.86–0.97) for the discrimination of PE patients and healthy controls in antenatal samples (Figure 2C).
PE-Associated Microbes Correlate to Clinical and Immune Parameters
To investigate the interassociations between gut microbial composition and host clinical status, we identified statistical correlations between 13 PE-associated genera and the pregnant women's parameters, including blood indexes, fetal features, and antenatal clinical immune indexes. Significant associations were observed in the separate patient and control groups (Figure 3). For example, in PE patients, the women's DBP and SBP levels were positively correlated with PE-enriched genera, such as Anaerococcus, Ruminococcus2, Fusobacterium, and Oribacterium, while the fetal features (e.g., birth weight) were positively correlated with PE-depleted genera. One of the pregnant women's immune parameters, IL-6 was positively associated with Blautia (ρ = 0.36, q = 0.04; in PE patients) and Bilophila (Spearman's ρ = 0.37, q = 0.01; in healthy controls), and negatively associated with Faecalibacterium (ρ = −0.27, q = 0.04; in PE patients).
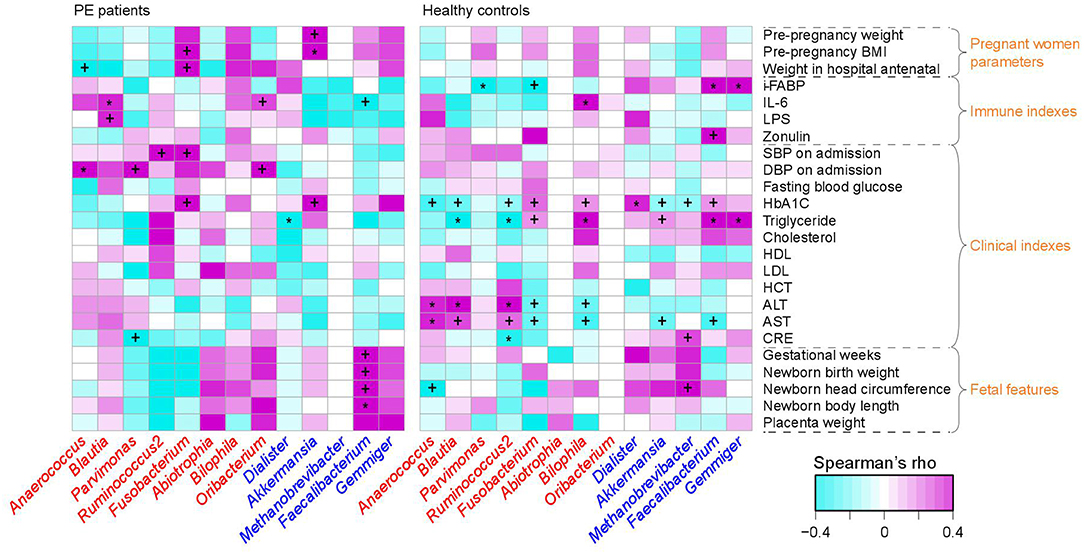
Figure 3. Correlation between PE-associated genera and the host parameters. The heatmap panel shows the Spearman correlation coefficient between the genera (text color: red, enriched in PE patients; blue, enriched in healthy controls) and host parameters. Significance levels in correlation tests are denoted: +,q < 0.10; *,q < 0.05.
KEGG Functional Signatures
The functional capacity of the gut microbiota of PE patients and healthy controls were predicted by PICRUSt analysis (Langille et al., 2013) based on their 16S OTU profiles, which led us to quantify the relative abundance of 494 KEGG modules. In all, 66 modules (13.4%) significantly differed in their abundance between PE patients and healthy controls (q < 0.05; Table S4), including 46 PE-reduced and 20 PE-enriched modules. The majority of the PE-reduced modules involved to central and other carbohydrate metabolism (n = 9, including 2 terpenoid biosynthesis modules), carbon fixation (n = 4), amino acid, cofactor, and vitamin metabolism (n = 5), ATP synthesis and photosynthesis (n = 7), two-component regulatory system (n = 8), and the transport systems of several small molecules (n = 7), while the PE-enriched modules involved various functional sets including 4 saccharide transport systems. Thirty-six (78.3%) PE-reduced modules, as well as all the PE-enriched modules, were significantly correlated with one or more PE-associated genera (Figure 4). In PE-enriched genera, Blautia and Ruminococcus2 were associated with the majority of PE-enriched functional modules, suggesting their central role in the PE microbiome. In addition, Fusobacterium and Bilophila had complementary roles, as they were uniquely positively correlated with the adverse modules involved in bile acid biosynthesis and nicotinate degradation. In PE-reduced genera, Faecalibacterium, Methanobrevibacter, and Akkermansia played central roles.
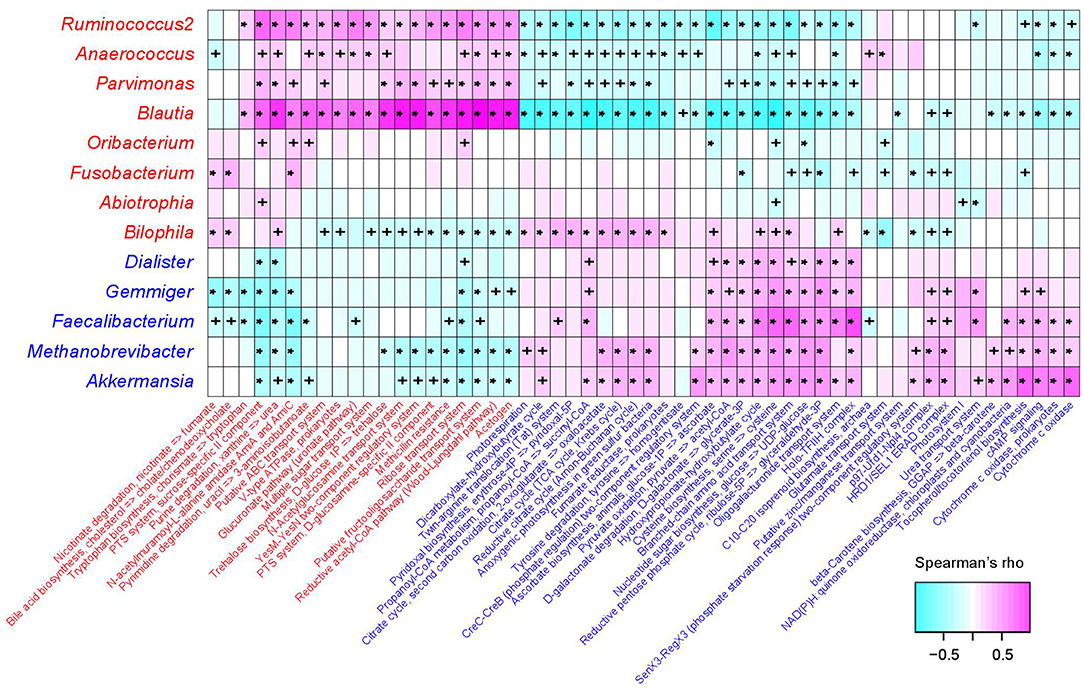
Figure 4. Correlation between PE-associated genera and functional modules. The heatmap panel shows the Spearman correlation coefficient between the genera and KEGG functional modules. Text color for genera and modules: red, enriched in PE patients; blue, enriched in healthy controls. Significance levels in correlation tests are denoted: +,P < 0.05; *,P < 0.01.
Longitudinal Variation of Gut Microbial Diversity and Composition
In our cohort, the fecal samples of 15 women were collected at antepartum and 1 week postpartum, and 11 women provided samples at antepartum and 6 weeks postpartum, which allowed us to investigate the longitudinal changes. From antepartum to 1 week postpartum, the observed OTUs and phylogenetic diversity (two indexes of microbial richness) in women's gut microbiota were consistent (Figure 5A), but their microbial evenness was disturbed. From antepartum to 6 weeks postpartum, all microbial alpha diversity indexes were correlated between the two time points. With regard to the aspect of microbial composition, we found that samples at both 1 and 6 weeks postpartum were highly consistent with samples at antepartum (Figure 5B; P < 0.05 for two comparisons).
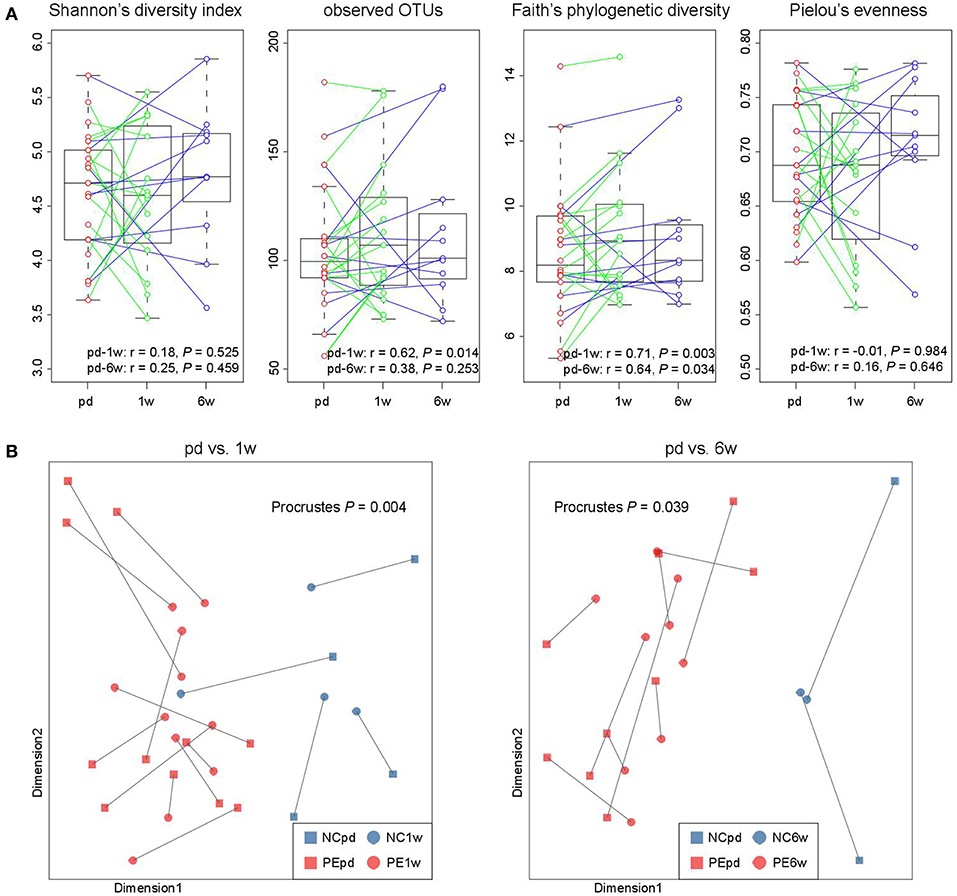
Figure 5. Longitudinal comparison of gut microbial diversity and composition between antepartum, 1 and 6 weeks postpartum. (A) Change in alpha diversity from the antepartum to 1 and 6 weeks postpartum. The boxes represent the interquartile range (IQR) between the first and third quartiles and the line inside represents the median. The whiskers denote the lowest and highest values within 1.5 times IQR from the first and third quartiles, respectively. Pearson correlation coefficient of samples between two time points was calculated, and P-value was tested using the correlation test. (B) Procrustes analysis reveals the covariation between samples at the antepartum, 1 and 6 weeks postpartum periods.
Discussion
In this study, the changes of gut microbiota of pregnant women with PE before and after delivery were analyzed and compared with those of normotensive, uncomplicated pregnant women in the antepartum, 1 and 6 weeks postpartum periods. Our study demonstrated that the composition of gut microbiota in PE patients remarkably differed from that in normal pregnant women throughout the evaluation periods.
Currently, the exact cause of PE remains elusive. Previous studies have suggested that metabolic abnormalities, systemic inflammatory reactions, oxidative stress, and vascular endothelial damage are involved in the pathogenesis of PE (Catalano et al., 2012; Paauw et al., 2016). Our study suggested that gut microbiota may also be one of the participants. Similar studies on gestational hypertension and gestational diabetes mellitus (GDM) supported the hypothesis that gestational diseases are associated with changes in gut microbiota (Kuang et al., 2017; Crusell et al., 2018). Gomez-Arango et al. (2016a) found that the abundance of Odoribacter, a bacteria producing butyric acid, was negatively correlated with the SBP of pregnant women at 16 weeks of pregnancy. Crusell et al. (2018) found that GDM was associated with a change in the gut microbiota composition in both the third trimester of pregnancy and postpartum. They especially reported an enrichment in the abundance of Blautia and Ruminococcus2 in diabetic patients, which was also observed in the PE patients in our cohort. Studies on reducing the risk of PE through probiotic supplementation (Brantsaeter et al., 2011) confirmed that PE is associated with gut microbes. For years, the strategies for managing PE have been limited to symptomatic therapy or the termination of pregnancy (Xia and Kellems, 2013). This suggests that regulating intestinal microbiota through probiotics may play a role in the prevention of PE. Thus, exploring the changes and functions of intestinal microbiota in PE may provide new ideas for the prevention and treatment of PE.
We found that PE stratification accounted for nearly 2% of gut microbial variation (Figure 1B), which was relatively larger than other host parameters. As revealed by previous population-based reports (Falony et al., 2016; Zhernakova et al., 2016), a large proportion (80–90%) of variation of human gut microbiome was explained by unexplained or intrinsic factors (e.g., enterotypes), whereas the host parameters including various kinds of diseases only explained a limited proportion of variations (usually <1%). In addition, we identified 13 PE-associated genera (Figure 2A), which achieved a higher discriminatory power (AUC = 0.91) for discriminating PE and control samples at the antenatal stage (Figure 2C) compared to that of other diseases such as diabetes (Qin et al., 2012) and hypertension (Yan et al., 2017). Thus, these findings highlighted the remarkable dysbiosis of gut microbiota in PE patients.
Literature searches of the 13 PE-associated genera showed that these bacteria were also associated with other host diseases including obesity, higher glucose metabolic disorders, pro-inflammatory effects, intestinal barrier dysfunction, and bile acid dysmetabolism (see discussion below). In addition, these microbes were able to be correlated with host immune parameters and function markers, such as IL-6 and LPS (Signat et al., 2011; Hunter and Jones, 2015), and such correlations were also found in the current study (Figure 3A). Overall, these findings suggested that PE patients harbor an inflammation-associated microbiota. The schematic of PE-microbiota is summarized in Figure 6, and the potential relevance of mechanisms is described below.
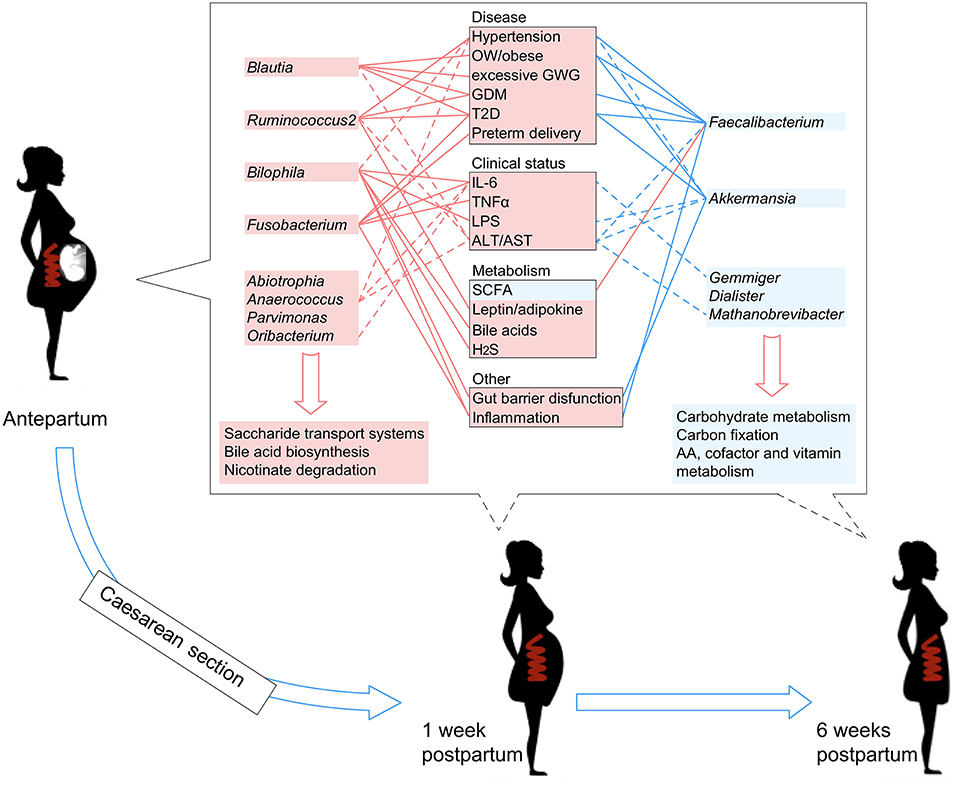
Figure 6. A schematic diagram showing the PE-associated bacteria and functions that had predicted effects on the host disease, clinical status, and metabolism. The PE-enriched species and functions are shown in the left red columns, and the PE-depleted species and functions are shown in the right blue columns. Center boxes show the host properties. Lines connect the PE-associated species and host properties with potential associations, while the dotted lines indicate the correlations which were identified in this study.
In PE-enriched genera, Blautia and Ruminococcus2 were associated with the majority of PE-enriched functional modules, suggesting their central role in the PE microbiome. Members of the genus Blautia are generally gram-positive bacteria that produce acetate, ethanol, hydrogen, lactate, or succinate as the end products of glucose fermentation (Liu et al., 2008). Blautia has been associated with obesity and type 2 diabetes (Cani et al., 2012; Kasai et al., 2015), and is enriched in prepregnancy overweight/obese and excessive gestational weight gain women (Stanislawski et al., 2017) and glucose-intolerant individuals (Egshatyan et al., 2016). These findings are in line with the results of Crusell et al. (2018) who showed increased abundances associated with GDM, suggesting that enriched Blautia abundance goes together with a nonfavorable metabolic profile. Similarly, Ruminococcus2 was also enriched in women with GDM (Zacarías et al., 2018) as well as type 2 diabetic patients (Zhang et al., 2013), and R. gnavus has been reported to be enriched in people with dysmetabolism and low microbial gene count (Le Chatelier et al., 2013). In addition, Ruminococcaceae are strongly correlated with the leptin level (an adipocyte-derived hormone that plays a direct role in the pathogenesis of PE) in the human body (Miehle et al., 2012; Taylor et al., 2015; Gomez-Arango et al., 2016b). Furthermore, we revealed that Ruminococcus2 abundance in the intestinal tract of PE patients was positively correlated with SBP/DBP on admission and ALT/AST levels in pregnant women (Figure 3A), suggesting that the Ruminococcus2 may also be directly related to the incidence of PE via its effect on the host's blood pressure or liver function.
Bilophila (B. wadsworthia) and Fusobacterium (F. nucleatum) also represented the major variance in PE microbiomes. B. wadsworthia can promote higher inflammation via producing hydrogen sulfide (da Silva et al., 2008), intestinal barrier dysfunction and bile acid dysmetabolism (Devkota et al., 2012; Natividad et al., 2018). Moreover, higher amounts of B. wadsworthia can release LPS and IL-6 (Hunter and Jones, 2015), which is in agreement with the observation in this study showing a positive correlation between the prenatal plasma IL-6 level and the abundance of intestinal Bilophila. F. nucleatum is one of the most prevalent gut and oral species that is associated with a wide spectrum of human diseases such as adverse pregnancy outcomes (e.g., preterm birth and neonatal sepsis) (Barak et al., 2007; Han et al., 2010; Bohrer et al., 2012; Wang et al., 2013) and gastrointestinal tract disorders (e.g., colorectal cancer and inflammatory bowel disease) (Han, 2015). The virulence mechanisms include its colonization, systemic dissemination, and induction of host inflammatory and tumorigenic responses (Han, 2015). Additionally, F. nucleatum is also a potent stimulator of inflammatory cytokines, such as IL-6, IL-8, and TNFα (Han et al., 2000; Park et al., 2014).
Except for the PE-enriched bacteria, several species including Faecalibacterium, Methanobrevibacter, and Akkermansia were depleted in PE patients. Faecalibacterium, one of the most abundant and important commensal bacteria in the human gut microbiota, produces short-chain fatty acids (SCFAs, especially butyrate) (Machiels et al., 2014), and protects the intestines, and is involved the reduction of obesity, diabetes and inflammation (Sokol et al., 2008; Miquel et al., 2013). Lower production capacity of SCFAs may therefore contribute to higher blood pressure, and thus increase the risk of PE in pregnant women (Pevsner-Fischer et al., 2017; Yan et al., 2017). In addition, Akkermansia muciniphila is associated with a low risk of diabetes (Shin et al., 2014), obesity (Everard et al., 2013), and high inflammation (Ganesh et al., 2013), based on its unique mucin-degrading capacity that functions to strengthen the integrity of the host's gut barrier (Guo et al., 2017).
Despite the pregnant women's gut microbiota being largely separated by the division of three time stages, the microbial alterations of the PE microbiome at the antenatal stage could also reflect on the samples at 1 and 6 weeks postpartum (Figure 2B). Particularly, but not significantly, the gut microbiomes of samples at 6 weeks postpartum were closer to the antenatal samples than that of the 1 week postpartum samples, either in the whole microbial composition (Figure 1C) or in the PE-associated taxa (Figure 2C). A similar phenomenon was also revealed in previous studies showing that the women's gut microbial structure dramatically changed during delivery and sustained recovery after a long period of time (DiGiulio et al., 2015; Wang et al., 2018). As a supplement, our intraindividual samples of antepartum-1 week-postpartum pairs and antepartum-6 weeks-postpartum pairs also showed highly microbial diversity and compositional consistency (Figure 5).
One of the limitations of the current study was sample size in postpartum for both week 1 and 6. The lack of close matching in individual confounding factors, such as genetic background, host geography, diet and lifestyle could also limit interpretations of this study results. Another limitation was that samples of women during 1st or 2nd trimester were not included in the study. Thus, our study could not suggest any causal relationship between the altered gut microbiota and PE pathogenicity. Future studies with a larger cohort from early pregnancy to postpartum will be needed to further understand the relationship between gut microbiome and PE. Testing specific microbiota in animal models is also beneficial to elucidate the mechanism of interaction between gut microbiome and preeclampsia during pregnancy.
Conclusion
To our knowledge, this is the first study investigating the gut microbiota composition in PE patients at both the time points of antepartum and postpartum. Our findings add more important information of correlation between gut microbiota and PE and extend the previous knowledge. The taxonomic signatures, microbe-clinical associations, and function signatures identified in this study suggested possible pathways for PE pathogenicity and provided potential markers for PE prediction and intervention.
Data Availability
The raw sequencing dataset acquired in this study has been deposited to the European Bioinformatics Institute (EBI) database under the accession code PRJEB33074 (https://www.ebi.ac.uk/ena/data/view/PRJEB33074). The sample metadata, OTU and taxonomic composition data, and the statistical scripts are available from the corresponding author on reasonable request.
Author Contributions
A-HY substantially contributed to the conception of the work, experimental, and manuscript guidance. L-JL mainly carried out the cohort research performance, data analysis, and drafted the manuscript. S-HL was responsible for statistics, analysis, and functional annotation of bioinformatics data. S-CL, CT, and Z-CZ participated in the implementation of the experiment. H-LD, HL, WH, M-CC, T-WH, and Y-NW were responsible for samples storage and fellow-up of the cohort. S-HL, XZ, and LY critically revised the manuscript for important intellectual content. All the participants provided approval for publication of the content, agreed to be accountable for all aspects of the work in ensuring that questions related to the accuracy or integrity of any part of the work are appropriately investigated and resolved.
Conflict of Interest Statement
The authors declare that the research was conducted in the absence of any commercial or financial relationships that could be construed as a potential conflict of interest.
Supplementary Material
The Supplementary Material for this article can be found online at: https://www.frontiersin.org/articles/10.3389/fcimb.2019.00224/full#supplementary-material
Table S1. Basic information and sequencing summary of 180 analyzed samples.
Table S2. PERMANOVA (adonis) analysis of host parameters on their gut microbiotas. Analysis were performed on the OTU and genus profiles to test whether the individuals' parameters have significant impact on the gut microbiota meta.
Table S3. Detailed information of the PE-associated taxa at the phylum, genus and species levels. Genera with P < 0.05 (Wilcoxon rank-sum test) at antepartum, 1 or 6weeks postpartum are shown.
Table S4. Detailed information of the PE-associated functional modules. Note: green, significantly enriched in healthy controls; red, significantly enriched in PE patients.
Figure S1. Comparison of alpha and beta diversity between PE patients and healthy controls. (A) Difference of four alpha diversity indexes between patients and controls. The boxes represent the interquartile range (IQR) between the first and third quartiles and the line inside represents the median. The whiskers denote the lowest and highest values within 1.5 times IQR from the first and third quartiles, respectively. (B) PCoA plot based on four beta diversity indexes. The first and second principal coordinates are shown. Lines connect samples (colored points) in the same group, and circles cover samples near the center of gravity for each group.
References
American College of Obstetricians and Gynecologists and Task Force on Hypertension in Pregnancy. (2013). Hypertension in pregnancy. Report of the American college of obstetricians and gynecologists' task force on hypertension in pregnancy. Obstet. Gynecol. 122, 1122–1131. doi: 10.1097/01.AOG.0000437382.03963.88
Ananth, C. V., Keyes, K. M., and Wapner, R. J. (2013). Pre-eclampsia rates in the United States, 1980-2010: age-period-cohort analysis. BMJ 347:f6564. doi: 10.1136/bmj.f6564
Barak, S., Oettinger-Barak, O., Machtei, E. E., Sprecher, H., and Ohel, G. (2007). Evidence of periopathogenic microorganisms in placentas of women with preeclampsia. J. Periodontol. 78, 670–676. doi: 10.1902/jop.2007.060362
Bohrer, J. C., Kamemoto, L. E., Almeida, P. G., and Ogasawara, K. K. (2012). Acute chorioamnionitis at term caused by the oral pathogen Fusobacterium nucleatum. Hawaii J. Med. Public Health 71, 280–281.
Brantsaeter, A. L., Myhre, R., Haugen, M., Myking, S., Sengpiel, V., Magnus, P., et al. (2011). Intake of probiotic food and risk of preeclampsia in primiparous women: the Norwegian Mother and Child Cohort Study. Am. J. Epidemiol. 174, 807–815. doi: 10.1093/aje/kwr168
Brown, M. A., Magee, L. A., Kenny, L. C., Karumanchi, S. A., McCarthy, F. P., Saito, S., et al. (2018). Hypertensive disorders of pregnancy: ISSHP classification, diagnosis, and management recommendations for international practice. Hypertension 72, 24–43. doi: 10.1161/HYPERTENSIONAHA.117.10803
Callahan, B. J., McMurdie, P. J., Rosen, M. J., Han, A. W., Johnson, A. J., and Holmes, S. P. (2016). DADA2: high-resolution sample inference from Illumina amplicon data. Nat. Methods 13, 581–583. doi: 10.1038/nmeth.3869
Cani, P. D., Osto, M., Geurts, L., and Everard, A. (2012). Involvement of gut microbiota in the development of low-grade inflammation and type 2 diabetes associated with obesity. Gut Microbes 3, 279–288. doi: 10.4161/gmic.19625
Catalano, P. M., McIntyre, H. D., Cruickshank, J. K., McCance, D. R., Dyer, A. R., Metzger, B. E., et al. (2012). The hyperglycemia and adverse pregnancy outcome study: associations of GDM and obesity with pregnancy outcomes. Diabetes Care 35, 780–786. doi: 10.2337/dc11-1790
Costea, P. I., Hildebrand, F., Arumugam, M., Backhed, F., Blaser, M. J., Bushman, F. D., et al. (2018). Enterotypes in the landscape of gut microbial community composition. Nat. Microbiol. 3, 8–16. doi: 10.1038/s41564-017-0072-8
Crusell, M. K. W., Hansen, T. H., Nielsen, T., Allin, K. H., Rühlemann, M. C., Damm, P., et al. (2018). Gestational diabetes is associated with change in the gut microbiota composition in third trimester of pregnancy and postpartum. Microbiome 6:89. doi: 10.1186/s40168-018-0472-x
da Silva, S. M., Venceslau, S. S., Fernandes, C. L., Valente, F. M., and Pereira, I. A. (2008). Hydrogen as an energy source for the human pathogen Bilophila wadsworthia. Antonie Van Leeuwenhoek 93, 381–390. doi: 10.1007/s10482-007-9215-x
DeSantis, T. Z., Hugenholtz, P., Larsen, N., Rojas, M., Brodie, E. L., Keller, K., et al. (2006). Greengenes, a chimera-checked 16S rRNA gene database and workbench compatible with ARB. Appl. Environ. Microbiol. 72, 5069–5072. doi: 10.1128/AEM.03006-05
Devkota, S., Wang, Y., Musch, M. W., Leone, V., Fehlner-Peach, H., Nadimpalli, A., et al. (2012). Dietary-fat-induced taurocholic acid promotes pathobiont expansion and colitis in Il10-/- mice. Nature 487, 104–108. doi: 10.1038/nature11225
DiGiulio, D. B., Callahan, B. J., McMurdie, P. J., Costello, E. K., Lyell, D. J., Robaczewska, A., et al. (2015). Temporal and spatial variation of the human microbiota during pregnancy. Proc. Natl. Acad. Sci. U.S.A. 112, 11060–11065. doi: 10.1073/pnas.1502875112
Egshatyan, L., Kashtanova, D., Popenko, A., Tkacheva, O., Tyakht, A., Alexeev, D., et al. (2016). Gut microbiota and diet in patients with different glucose tolerance. Endocr. Connect. 5, 1–9. doi: 10.1530/EC-15-0094
Everard, A., Belzer, C., Geurts, L., Ouwerkerk, J. P., Druart, C., Bindels, L. B., et al. (2013). Cross-talk between Akkermansia muciniphila and intestinal epithelium controls diet-induced obesity. Proc. Natl. Acad. Sci. U.S.A. 110, 9066–9071. doi: 10.1073/pnas.1219451110
Falony, G., Joossens, M., Vieira-Silva, S., Wang, J., Darzi, Y., Faust, K., et al. (2016). Population-level analysis of gut microbiome variation. Science 352, 560–564. doi: 10.1126/science.aad3503
Ganesh, B. P., Klopfleisch, R., Loh, G., and Blaut, M. (2013). Commensal Akkermansia muciniphila exacerbates gut inflammation in Salmonella Typhimurium-infected gnotobiotic mice. PLoS ONE 8:e74963. doi: 10.1371/journal.pone.0074963
Ghulmiyyah, L., and Sibai, B. (2012). Maternal mortality from preeclampsia/eclampsia. Semin. Perinatol. 36, 56–59. doi: 10.1053/j.semperi.2011.09.011
Gomez-Arango, L. F., Barrett, H. L., McIntyre, H. D., Callaway, L. K., Morrison, M., Dekker Nitert, M., et al. (2016a). Increased systolic and diastolic blood pressure is associated with altered gut microbiota composition and butyrate production in early pregnancy. Hypertension 68, 974–981. doi: 10.1161/HYPERTENSIONAHA.116.07910
Gomez-Arango, L. F., Barrett, H. L., McIntyre, H. D., Callaway, L. K., Morrison, M., Dekker Nitert, M., et al. (2016b). Connections between the gut microbiome and metabolic hormones in early pregnancy in overweight and obese women. Diabetes 65, 2214–2223. doi: 10.2337/db16-0278
Guo, X., Li, S., Zhang, J., Wu, F., Li, X., Wu, D., et al. (2017). Genome sequencing of 39 Akkermansia muciniphila isolates reveals its population structure, genomic and functional diverisity, and global distribution in mammalian gut microbiotas. BMC Genomics 18:800. doi: 10.1186/s12864-017-4195-3
Han, Y. W. (2015). Fusobacterium nucleatum: a commensal-turned pathogen. Curr. Opin. Microbiol. 23, 141–147. doi: 10.1016/j.mib.2014.11.013
Han, Y. W., Fardini, Y., Chen, C., Iacampo, K. G., Peraino, V. A., Shamonki, J. M., et al. (2010). Term stillbirth caused by oral Fusobacterium nucleatum. Obstet. Gynecol. 115(2 Pt 2), 442–445. doi: 10.1097/AOG.0b013e3181cb9955
Han, Y. W., Shi, W., Huang, G. T., Kinder Haake, S., Park, N. H., Kuramitsu, H., et al. (2000). Interactions between periodontal bacteria and human oral epithelial cells: Fusobacterium nucleatum adheres to and invades epithelial cells. Infect. Immun. 68, 3140–3146. doi: 10.1128/IAI.68.6.3140-3146.2000
Holmes, E., Loo, R. L., Stamler, J., Bictash, M., Yap, I. K., Chan, Q., et al. (2008). Human metabolic phenotype diversity and its association with diet and blood pressure. Nature 453, 396–400. doi: 10.1038/nature06882
Hunter, C. A., and Jones, S. A. (2015). IL-6 as a keystone cytokine in health and disease. Nat. Immunol. 16, 448–457. doi: 10.1038/ni.3153
Huppertz, B. (2008). Placental origins of preeclampsia: challenging the current hypothesis. Hypertension 51, 970–975. doi: 10.1161/HYPERTENSIONAHA.107.107607
Hutcheon, J. A., Lisonkova, S., and Joseph, K. S. (2011). Epidemiology of pre-eclampsia and the other hypertensive disorders of pregnancy. Best Pract. Res. Clin. Obstet. Gynaecol. 25, 391–403. doi: 10.1016/j.bpobgyn.2011.01.006
Kamada, N., Seo, S. U., Chen, G. Y., and Núñez, G. (2013). Role of the gut microbiota in immunity and inflammatory disease. Nat. Rev. Immunol. 13, 321–335. doi: 10.1038/nri3430
Kanehisa, M., Furumichi, M., Tanabe, M., Sato, Y., and Morishima, K. (2017). KEGG: new perspectives on genomes, pathways, diseases and drugs. Nucleic Acids Res. 45, D353–D361. doi: 10.1093/nar/gkw1092
Kasai, C., Sugimoto, K., Moritani, I., Tanaka, J., Oya, Y., Inoue, H., et al. (2015). Comparison of the gut microbiota composition between obese and non-obese individuals in a Japanese population, as analyzed by terminal restriction fragment length polymorphism and next-generation sequencing. BMC Gastroenterol. 15:100. doi: 10.1186/s12876-015-0330-2
Khader, Y. S., Batieha, A., Al-Njadat, R. A., and Hijazi, S. S. (2018). Preeclampsia in Jordan: incidence, risk factors, and its associated maternal and neonatal outcomes. J. Matern. Fetal. Neonatal. Med. 31, 770–776. doi: 10.1080/14767058.2017.1297411
Koren, O., Goodrich, J. K., Cullender, T. C., Spor, A., Laitinen, K., Backhed, H. K., et al. (2012). Host remodeling of the gut microbiome and metabolic changes during pregnancy. Cell 150, 470–480. doi: 10.1016/j.cell.2012.07.008
Kovo, M., Schreiber, L., Elyashiv, O., Ben-Haroush, A., Abraham, G., and Bar, J. (2015). Pregnancy outcome and placental findings in pregnancies complicated by fetal growth restriction with and without preeclampsia. Reprod. Sci. 22, 316–321. doi: 10.1177/1933719114542024
Krautkramer, K. A., Kreznar, J. H., Romano, K. A., Vivas, E. I., Barrett-Wilt, G. A., Rabaglia, M. E., et al. (2016). Diet-microbiota interactions mediate global epigenetic programming in multiple host tissues. Mol. Cell. 64, 982–992. doi: 10.1016/j.molcel.2016.10.025
Kuang, Y. S., Lu, J. H., Li, S. H., Li, J. H., Yuan, M. Y., He, J. R., et al. (2017). Connections between the human gut microbiome and gestational diabetes mellitus. Gigascience 6, 1–12. doi: 10.1093/gigascience/gix058
Kuczynski, J., Stombaugh, J., Walters, W. A., Gonzalez, A., Caporaso, J. G., and Knight, R. (2012). Using QIIME to analyze 16S rRNA gene sequences from microbial communities. Curr. Protoc. Microbiol. Chapter 1:Unit 1E.5. doi: 10.1002/9780471729259.mc01e05s27
Langille, M. G., Zaneveld, J., Caporaso, J. G., McDonald, D., Knights, D., Reyes, J. A., et al. (2013). Predictive functional profiling of microbial communities using 16S rRNA marker gene sequences. Nat. Biotechnol. 31, 814–821. doi: 10.1038/nbt.2676
Laresgoiti-Servitje, E. (2013). A leading role for the immune system in the pathophysiology of preeclampsia. J. Leukoc. Biol. 94, 247–257. doi: 10.1189/jlb.1112603
Le Chatelier, E., Nielsen, T., Qin, J., Prifti, E., Hildebrand, F., Falony, G., et al. (2013). Richness of human gut microbiome correlates with metabolic markers. Nature 500, 541–546. doi: 10.1038/nature12506
Lei, Q., Zhou, X., Duan, D. M., Lv, L. J., Lin, X. H., Ji, W. J., et al. (2017). Trimester-specific weight gain and midpregnancy diastolic blood pressure rebound during normotensive pregnancy. Hypertension 70, 804–812. doi: 10.1161/HYPERTENSIONAHA.117.09760
Leslie, K., Thilaganathan, B., and Papageorghiou, A. (2011). Early prediction and prevention of pre-eclampsia. Best Pract. Res. Clin. Obstet. Gynaecol. 25, 343–354. doi: 10.1016/j.bpobgyn.2011.01.002
Lisonkova, S., and Joseph, K. S. (2013). Incidence of preeclampsia: risk factors and outcomes associated with early- versus late-onset disease. Am. J. Obstet. Gynecol. 209, 544.e1–544.e12. doi: 10.1016/j.ajog.2013.08.019
Lisonkova, S., Sabr, Y., Mayer, C., Young, C., Skoll, A., and Joseph, K. S. (2014). Maternal morbidity associated with early-onset and late-onset preeclampsia. Obstet. Gynecol. 124, 771–781. doi: 10.1097/AOG.0000000000000472
Liu, C., Finegold, S. M., Song, Y., and Lawson, P. A. (2008). Reclassification of Clostridium coccoides, Ruminococcus hansenii, Ruminococcus hydrogenotrophicus, Ruminococcus luti, Ruminococcus productus and Ruminococcus schinkii as Blautia coccoides gen. nov., comb. nov., Blautia hansenii comb. nov., Blautia hydrogenotrophica comb. nov., Blautia luti comb. nov., Blautia producta comb. nov., Blautia schinkii comb. nov. and description of Blautia wexlerae sp. nov., isolated from human faeces. Int. J. Syst. Evol. Microbiol. 58(Pt 8), 1896–1902. doi: 10.1099/ijs.0.65208-0
Liu, J., Yang, H., Yin, Z., Jiang, X., Zhong, H., Qiu, D., et al. (2017). Remodeling of the gut microbiota and structural shifts in Preeclampsia patients in South China. Eur. J. Clin. Microbiol. Infect. Dis. 36, 713–719. doi: 10.1007/s10096-016-2853-z
Liu, R., Hong, J., Xu, X., Feng, Q., Zhang, D., Gu, Y., et al. (2017). Gut microbiome and serum metabolome alterations in obesity and after weight-loss intervention. Nat. Med. 23, 859–868. doi: 10.1038/nm.4358
Machiels, K., Joossens, M., Sabino, J., De Preter, V., Arijs, I., Eeckhaut, V., et al. (2014). A decrease of the butyrate-producing species Roseburia hominis and Faecalibacterium prausnitzii defines dysbiosis in patients with ulcerative colitis. Gut 63, 1275–1283. doi: 10.1136/gutjnl-2013-304833
Miehle, K., Stepan, H., and Fasshauer, M. (2012). Leptin, adiponectin and other adipokines in gestational diabetes mellitus and pre-eclampsia. Clin. Endocrinol. 76, 2–11. doi: 10.1111/j.1365-2265.2011.04234.x
Miquel, S., Martín, R., Rossi, O., Bermúdez-Humarán, L. G., Chatel, J. M., Sokol, H., et al. (2013). Faecalibacterium prausnitzii and human intestinal health. Curr. Opin. Microbiol. 16, 255–261. doi: 10.1016/j.mib.2013.06.003
Mol, B. W. J., Roberts, C. T., Thangaratinam, S., Magee, L. A., de Groot, C. J. M., and Hofmeyr, G. J. (2016). Pre-eclampsia. Lancet 387, 999–1011. doi: 10.1016/S0140-6736(15)00070-7
Natividad, J. M., Lamas, B., Pham, H. P., Michel, M. L., Rainteau, D., Bridonneau, C., et al. (2018). Bilophila wadsworthia aggravates high fat diet induced metabolic dysfunctions in mice. Nat. Commun. 9:2802. doi: 10.1038/s41467-018-05249-7
Paauw, N. D., Luijken, K., Franx, A., Verhaar, M. C., and Lely, A. T. (2016). Long-term renal and cardiovascular risk after preeclampsia: towards screening and prevention. Clin. Sci. 130, 239–246. doi: 10.1042/CS20150567
Park, S. R., Kim, D. J., Han, S. H., Kang, M. J., Lee, J. Y., Jeong, Y. J., et al. (2014). Diverse Toll-like receptors mediate cytokine production by Fusobacterium nucleatum and Aggregatibacter actinomycetemcomitans in macrophages. Infect. Immun. 82, 1914–1920. doi: 10.1128/IAI.01226-13
Pedersen, H. K., Gudmundsdottir, V., Nielsen, H. B., Hyotylainen, T., Nielsen, T., Jensen, B. A., et al. (2016). Human gut microbes impact host serum metabolome and insulin sensitivity. Nature 535, 376–381. doi: 10.1038/nature18646
Pevsner-Fischer, M., Blacher, E., Tatirovsky, E., Ben-Dov, I. Z., and Elinav, E. (2017). The gut microbiome and hypertension. Curr. Opin. Nephrol. Hypertens. 26, 1–8. doi: 10.1097/MNH.0000000000000293
Pickard, J. M., Zeng, M. Y., Caruso, R., and Núñez, G. (2017). Gut microbiota: role in pathogen colonization, immune responses, and inflammatory disease. Immunol. Rev. 279, 70–89. doi: 10.1111/imr.12567
Qin, J., Li, Y., Cai, Z., Li, S., Zhu, J., Zhang, F., et al. (2012). A metagenome-wide association study of gut microbiota in type 2 diabetes. Nature 490, 55–60. doi: 10.1038/nature11450
Schiering, C., Wincent, E., Metidji, A., Iseppon, A., Li, Y., Potocnik, A. J., et al. (2017). Feedback control of AHR signalling regulates intestinal immunity. Nature 542, 242–245. doi: 10.1038/nature21080
Shin, N. R., Lee, J. C., Lee, H. Y., Kim, M. S., Whon, T. W., Lee, M. S., et al. (2014). An increase in the Akkermansia spp. population induced by metformin treatment improves glucose homeostasis in diet-induced obese mice. Gut 63, 727–735. doi: 10.1136/gutjnl-2012-303839
Signat, B., Roques, C., Poulet, P., and Duffaut, D. (2011). Fusobacterium nucleatum in periodontal health and disease. Curr. Issues Mol. Biol. 13, 25–36.
Sokol, H., Pigneur, B., Watterlot, L., Lakhdari, O., Bermúdez-Humarán, L. G., Gratadoux, J. J., et al. (2008). Faecalibacterium prausnitzii is an anti-inflammatory commensal bacterium identified by gut microbiota analysis of Crohn disease patients. Proc. Natl. Acad. Sci. U.S.A. 105, 16731–16736. doi: 10.1073/pnas.0804812105
Souza, J. P., Gülmezoglu, A. M., Vogel, J., Carroli, G., Lumbiganon, P., Qureshi, Z., et al. (2013). Moving beyond essential interventions for reduction of maternal mortality (the WHO Multicountry Survey on Maternal and Newborn Health): a cross-sectional study. Lancet 381, 1747–1755. doi: 10.1016/S0140-6736(13)60686-8
Stanislawski, M. A., Dabelea, D., Wagner, B. D., Sontag, M. K., Lozupone, C. A., and Eggesbø, M. (2017). Pre-pregnancy weight, gestational weight gain, and the gut microbiota of mothers and their infants. Microbiome 5:113. doi: 10.1186/s40168-017-0332-0
Tang, W. H., Wang, Z., Kennedy, D. J., Wu, Y., Buffa, J. A., Agatisa-Boyle, B., et al. (2015). Gut microbiota-dependent trimethylamine N-oxide (TMAO) pathway contributes to both development of renal insufficiency and mortality risk in chronic kidney disease. Circ. Res. 116, 448–455. doi: 10.1161/CIRCRESAHA.116.305360
Taylor, B. D., Ness, R. B., Olsen, J., Hougaard, D. M., Skogstrand, K., Roberts, J. M., et al. (2015). Serum leptin measured in early pregnancy is higher in women with preeclampsia compared with normotensive pregnant women. Hypertension 65, 594–599. doi: 10.1161/HYPERTENSIONAHA.114.03979
Tomasova, L., Dobrowolski, L., Jurkowska, H., Wróbel, M., Huc, T., Ondrias, K., et al. (2016). Intracolonic hydrogen sulfide lowers blood pressure in rats. Nitric Oxide 60, 50–58. doi: 10.1016/j.niox.2016.09.007
Wang, J., Zheng, J., Shi, W., Du, N., Xu, X., Zhang, Y., et al. (2018). Dysbiosis of maternal and neonatal microbiota associated with gestational diabetes mellitus. Gut 67, 1614–1625. doi: 10.1136/gutjnl-2018-315988
Wang, X., Buhimschi, C. S., Temoin, S., Bhandari, V., Han, Y. W., and Buhimschi, I. A. (2013). Comparative microbial analysis of paired amniotic fluid and cord blood from pregnancies complicated by preterm birth and early-onset neonatal sepsis. PLoS ONE 8:e56131. doi: 10.1371/journal.pone.0056131
Wesemann, D. R., Portuguese, A. J., Meyers, R. M., Gallagher, M. P., Cluff-Jones, K., Magee, J. M., et al. (2013). Microbial colonization influences early B-lineage development in the gut lamina propria. Nature 501, 112–115. doi: 10.1038/nature12496
World Medical Association. (2013). World Medical Association Declaration of Helsinki: ethical principles for medical research involving human subjects. JAMA 310, 2191–2194. doi: 10.1001/jama.2013.281053
Xia, Y., and Kellems, R. E. (2013). Angiotensin receptor agonistic autoantibodies and hypertension: preeclampsia and beyond. Circ. Res. 113, 78–87. doi: 10.1161/CIRCRESAHA.113.300752
Yan, Q., Gu, Y., Li, X., Yang, W., Jia, L., Chen, C., et al. (2017). Alterations of the gut microbiome in hypertension. Front. Cell, Infect. Microbiol. 7:381. doi: 10.3389/fcimb.2017.00381
Zacarías, M. F., Collado, M. C., Gómez-Gallego, C., Flinck, H., Aittoniemi, J., Isolauri, E., et al. (2018). Pregestational overweight and obesity are associated with differences in gut microbiota composition and systemic inflammation in the third trimester. PLoS ONE 13:e0200305. doi: 10.1371/journal.pone.0200305
Zhang, X., Shen, D., Fang, Z., Jie, Z., Qiu, X., Zhang, C., et al. (2013). Human gut microbiota changes reveal the progression of glucose intolerance. PLoS ONE 8:e71108. doi: 10.1371/journal.pone.0071108
Keywords: gut microbiota, preeclampsia, pregnancy, 16S rRNA gene sequencing, microbial dysbiosis
Citation: Lv L-J, Li S-H, Li S-C, Zhong Z-C, Duan H-L, Tian C, Li H, He W, Chen M-C, He T-W, Wang Y-N, Zhou X, Yao L and Yin A-H (2019) Early-Onset Preeclampsia Is Associated With Gut Microbial Alterations in Antepartum and Postpartum Women. Front. Cell. Infect. Microbiol. 9:224. doi: 10.3389/fcimb.2019.00224
Received: 06 March 2019; Accepted: 11 June 2019;
Published: 26 June 2019.
Edited by:
Moez Rhimi, INRA Centre Jouy-en-Josas, FranceReviewed by:
Natalia Shulzhenko, Oregon State University, United StatesJunguk Hur, University of North Dakota, United States
Copyright © 2019 Lv, Li, Li, Zhong, Duan, Tian, Li, He, Chen, He, Wang, Zhou, Yao and Yin. This is an open-access article distributed under the terms of the Creative Commons Attribution License (CC BY). The use, distribution or reproduction in other forums is permitted, provided the original author(s) and the copyright owner(s) are credited and that the original publication in this journal is cited, in accordance with accepted academic practice. No use, distribution or reproduction is permitted which does not comply with these terms.
*Correspondence: Ai-Hua Yin, yinaiwa@vip.126.com