- 1Department of Laboratory Medicine, and Beijing Key Laboratory for Mechanisms Research and Precision Diagnosis of Invasive Fungal Diseases, Peking Union Medical College Hospital, Chinese Academy of Medical Sciences, Beijing, China
- 2Graduate School, Peking Union Medical College, Beijing, China
- 3Department of Infectious Diseases and Clinical Microbiology, Beijing Chaoyang Hospital, Capital Medical University, Beijing, China
- 4Department of Laboratory Medicine, Beijing Friendship Hospital, Capital Medical University, Beijing, China
- 5Centre for Infectious Diseases and Microbiology Laboratory Services, Institute of Clinical Pathology and Medical Research, New South Wales Health Pathology, Westmead Hospital, The University of Sydney, Westmead, NSW, Australia
- 6Department of Clinical Laboratory, Charles Sturt University, Orange, NSW, Australia
- 7New South Wales Health Pathology, Regional and Rural, Orange Hospital, NSW, Australia
Matrix-assisted laser desorption ionization-time of flight mass spectrometry (MALDI-TOF MS) has been accepted as a rapid, accurate, and less labor-intensive method in the identification of microorganisms in clinical laboratories. However, there is limited data on systematic evaluation of its effectiveness in the identification of phylogenetically closely-related yeast species. In this study, we evaluated two commercially available MALDI-TOF systems, Autof MS 1000 and Vitek MS, for the identification of yeasts within closely-related species complexes. A total of 1,228 yeast isolates, representing 14 different species of five species complexes, including 479 of Candida parapsilosis complex, 323 of Candida albicans complex, 95 of Candida glabrata complex, 16 of Candida haemulonii complex (including two Candida auris), and 315 of Cryptococcus neoformans complex, collected under the National China Hospital Invasive Fungal Surveillance Net (CHIF-NET) program, were studied. Autof MS 1000 and Vitek MS systems correctly identified 99.2% and 89.2% of the isolates, with major error rate of 0.4% versus 1.6%, and minor error rate of 0.1% versus 3.5%, respectively. The proportion of isolates accurately identified by Autof MS 1000 and Vitek MS per each yeast complex, respectively, was as follows; C. albicans complex, 99.4% vs 96.3%; C. parapsilosis complex, 99.0% vs 79.1%; C glabrata complex, 98.9% vs 94.7%; C. haemulonii complex, 100% vs 93.8%; and C. neoformans, 99.4% vs 95.2%. Overall, Autof MS 1000 exhibited good capacity in yeast identification while Vitek MS had lower identification accuracy, especially in the identification of less common species within phylogenetically closely-related species complexes.
Introduction
Invasive fungal diseases (IFD) have become an emerging healthcare problem worldwide. It is associated with high rates of morbidity and mortality in immunocompromised individuals and critically ill patients (Miceli et al., 2011; Lockhart et al., 2017). Rapid, reliable, and species-specific diagnosis of fungal pathogens causing IFD is a prerequisite for cost-effective and successful therapy. However, some closely-related yeast species complexes are difficult to identify by conventional morphological or biochemical methods, and several of these cryptic species have distinct antifungal susceptibility profiles associated with specific clinical settings. These include Candida parapsilosis sensu stricto, Candida metapsilosis, Candida orthopsilosis, Lodderomyces elongisporus of the C. parapsilosis complex, Candida albicans and Candida dubliniensis of the C. albicans complex, Candida glabrata sensu stricto, Candida nivariensis, and Candida bracarensis of the C. glabrata complex, Cryptococcus neoformans, and Cryptococcus gattii of the C. neoformans complex, and finally, Candida haemulonii, Candida duobushaemulonii, and Candida auris of the C. haemulonii complex (also refered as multidrug-resistant [MDR] complex) (Muñoz et al., 2018).
Within less than a decade, the introduction of MALDI-TOF MS has enabled rapid and accurate identification of microorganisms including bacteria, mycobacteria, yeasts and filamentous fungi in clinical laboratories (Odds et al., 2007; Rychert et al., 2018) Autof MS 1000 (Autobio Diagnostics, Zhengzhou, China), a commercial MALDI-TOF MS system, has been available for routine pathogen identification in many clinical laboratories in China since 2018 (Wang et al., 2019). However, its performance and application in the identification of yeasts has not been fully evaluated. The purpose of this study was to evaluate the accuracy of Autof MS 1000 and Vitek MS in the identification of yeasts causing IFDs, especially for pathogens within closely-related species complexes.
Materials and Methods
Yeast Isolates
A total of 1,228 yeast isolates causing IFDs, and collected from 57 hospitals in China during the period August 2016 to July 2017, under the CHIF-NET program, were studied. Isolates were initially inoculated on a chromogenic agar (Brilliance C., Oxoid Ltd., Hampshire, United Kingdom) then subcultured on Sabouraud dextrose agar (SDA), and incubated at 35°C for 24 to 48 h.
Sequencing-Based Identification
All isolates were identified by DNA sequencing of the internal transcribed spacer (ITS) region, which was considered the “gold standard”. Primers ITS1 (5’-TCC GTA GGT GAA CCT GCG G-3’) and ITS4 (5’-TCC TCC GCT TAT TGA TAT GC-3’) were used to amplify the full-length ITS region. Amplification of the ITS region was carried out under the following conditions: denaturation at 94°C for 5 min, followed by 30 cycles of denaturation at 94°C for 30 s, annealing at 56°C for 90 s, and elongation at 72°C for 75 s, with a final extension step of 10 min at 72°C. Strain species identification was performed as described in a previous study (Wang et al., 2016).
Vitek MS Identification
A thin smear of a freshly cultured isolate was deposited onto a target plate, and 1 μl of formic acid (70%) was added. After air-drying, each spot was overlaid with 1 μl of α-cyano-4-hydroxycinnamic acid (HCCA) matrix (bioMérieux). The matrix was then allowed to dry at room air. E.coli ATCC 8739 was utilized as a calibrant and quality control strain per acquisition group on each slide. Measurement was then performed following the manufacturer’s suggested setting using automated collecting spectra. Captured spectra were analyzed based on IVD database version 3.0. For data analysis, a “green frame” indicator with a reliability (probability) of between 60.0% and 99.9% indicated sufficient species level identification, and a “yellow triangle” indicator denoted low resolution. If no identification (NoID) was provided, the isolate was considered unidentified and presented as a “red circle”.
Autof MS 1000 Identification
Autof MS 1000 is a new Chinese brand of MALDI-TOF MS platform for identification of micro-organisms which was approved for clinical use by China National Medical Products Administration in 2020. The operation process of this platform is similar to that of Vitek MS. A thin smear of a freshly cultured isolate was deposited onto a target plate, and 1 μl of formic acid (70%) was added. After air-drying, each spot was overlaid with 1 μl of α-cyano-4-hydroxycinnamic acid (HCCA) matrix. But without a fixed calibration point, Autof MS 1000 system runs identification immediately after sampling. The database (Autof Acquirer Version V2.0.18) contains 14,125 microbial strains comprising 4,226 species, 360 of which are fungal species. Results were interpreted based on the log score value of the first best match following manufacturer’s instructions as follows: 0.0≤score<6.0, not reliable identification; 6.0≤score<9.0, genus-level reliable identification and probable species-level identification; score≥9.0 species-level reliable identification.
Criteria for Identification and Discrepant Analysis
In the case of discrepant results or no identification result for one or both methods, the result of ITS sequencing was considered the final correct identification. A major error (ME) in identification by each of the methods studied (compared to the gold standard ITS) was defined as the incorrect genus identification (“no identification” not included). A minor error (MIE) was defined as the correct genus identification with incorrect species identification.
Results
Correct Identification by Vitek MS and Autof MS 1000
A total of 1,228 isolates representing 14 different yeast species within five closely-related species complexes (ie. C. albicans complex, C. parapsilosis complex, C. glabrata complex, C. haemulonii complex and C. neoformans complex), were evaluated in this study. Out of these isolates, 1,218 (99.2%) were correctly identified to species level by the Autof MS 1000 system, while 1,095 (89.2%) were correctly identified by Vitek MS (Table 1).
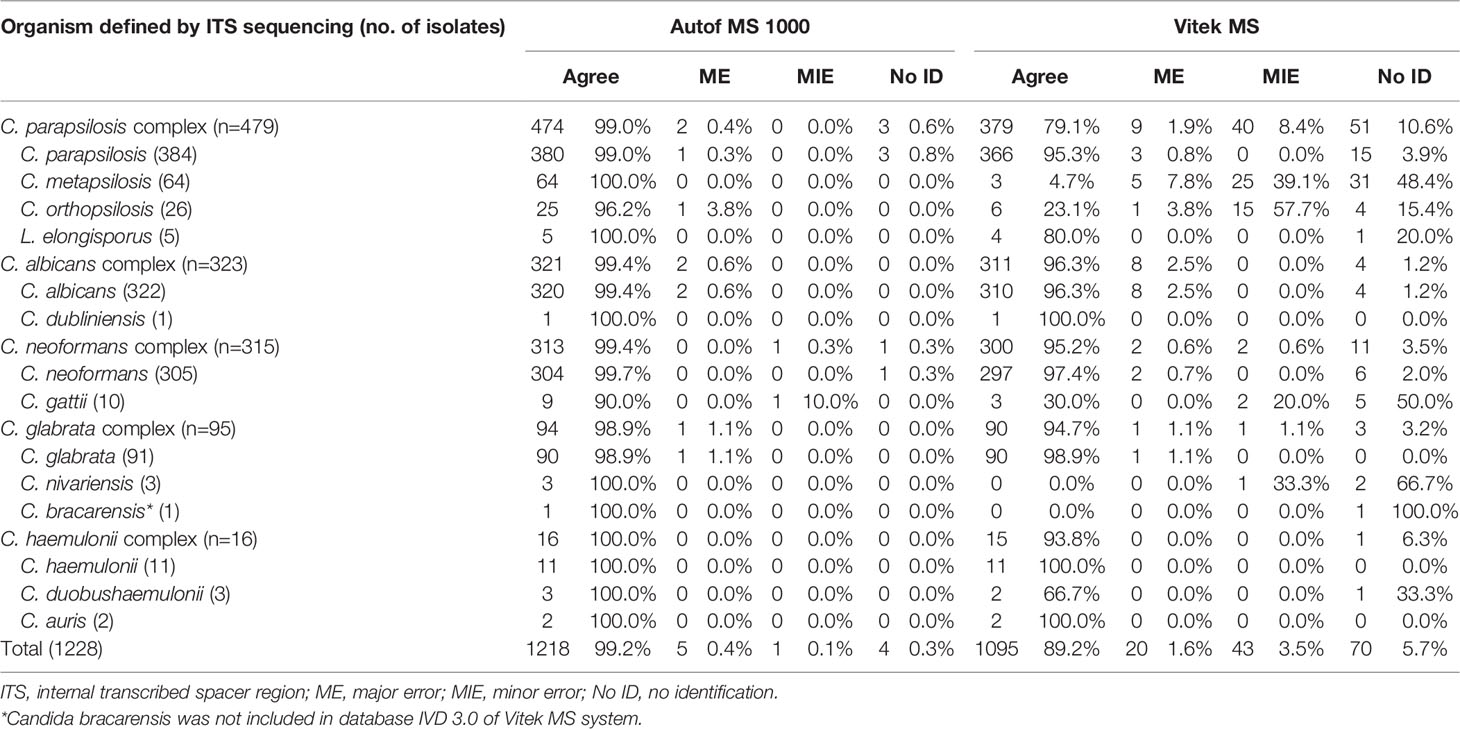
Table 1 Results of matrix-assisted laser desorption ionization-time of flight mass spectrometry (MALDI-TOF MS) identification by Autof MS 1000 and Vitek MS.
A majority (96.3%, 311/323) of C. albicans complex isolates were correctly identified to the species level by Vitek MS. For Autof MS 1000, the identification accuracy rate for C. albicans complex isolates was 99.4% (321/323). For C. glabrata complex isolates, 98.9% (94/95) were correctly identified to the species level by Autof MS 1000, which was higher than that of Vitek MS at 94.7% (90/95). For C. parapsilosis complex, 79.1% (379/479) of the isolates were correctly identified to species level by Vitek MS, which was much less than that of Autof MS 1000 at 99.0% (474/479). For C. neoformans complex, 95.2% (300/315) were correctly identified to the species level by Vitek MS while for Autof MS 1000, 99.4% (313/315) were correctly identified to species level (Table 1).
Comparison of “No Identification” Results
Four isolates (0.3%) yielded “no identification” result by Autos MS 1000, and 70 (5.7%) by Vitek MS (Table 1).
For species included in both systems’ identification databases, there were two (2/3, 66.6%) C. nivariensis isolates and 31 (31/64, 48.4%) of C. metapsilosis that were not identified by Vitek MS, which was a direct opposite to Autof MS 1000 which correctly identified both species. There were 5 (5/10, 50.0%) C. gattii isolates that were unidentified by Vitek MS.
In addition, C. bracarensis (n=1) was correctly identified by Autof MS 1000, but unidentified by Vitek MS as this species is not included in the Vitek MS database IVD 3.0.
Misidentifications by Vitek MS and Autof MS 1000
Autof MS 1000 identification results of five isolates (0.4%) were defined as major error while for Vitek MS it was for 20 isolates (1.6%) (Table 2). Only one identification result from an isolate was defined as a minor error for Autof MS 1000, while for Vitek MS, there were 43 (3.5%) isolates whose identification results were considered minor errors. One C. nivariensis (n=3) isolate was misidentified as C. glabrata; in addition, among C. metapsilosis (n=64) isolates, the identification results of five isolates were considered as ME, and 25 as MIE by Vitek MS. For C. gattii (n=10) there was one identification result that was considered as MIE by Autof MS 1000, and two by Vitek MS.
Discussion
Accurate identification of pathogens is important to ensure accurate diagnosis of diseases and subsequent proper treatment of the condition. As a newly licensed MALDI-TOF MS platform, few comparisons have been done between Autof MS 1000 and other systems. Recent studies have evaluated the performance of Autof MS 1000 in the identification of Bacteroides fragilis group isolates (Wang et al., 2019) and clinically relevant filamentous fungi (Sun et al., 2020). In this study, we evaluated the performance of MALDI-TOF MS systems, Autof MS 1000 and Vitek MS, in the identification of closely-related yeast species causing IFDs, using ITS sequencing as the reference method. Previous studies have shown that the accuracy for yeast identification ranges from 76.5% to 96.2% for Vitek MS (Wang et al., 2016). In this study, performance of Vitek MS in the identification of yeasts showed an overall accuracy of 89.2%. Misidentifications or no identifications were more likely to occur in less-common species within each species complexes. In comparison, Autof MS 1000 system exhibited higher identification accuracy in all species complexes (>98% for all species with overall accuracy of 99.2%).
C. albicans is the most frequent cause of superficial and systemic candidiasis. Before the introduction of further genetic studies, its closely related species, C. dubliniensis, was commonly misidentified as C. albicans (Sullivan et al., 1995). However, unlike C. albicans, C. dubliniensis causes fewer cases of systemic candidiasis (Odds et al., 2007). In the present study, both Vitek MS and Autof MS 1000 correctly identified >96% of C. albicans isolates, and correctly assigned C. dubliniensis to species-level.
C. parapsilosis has become the second to fourth most prevalent Candida species worldwide, and even surpasses C. albicans in some geographic regions (Harrington et al., 2017; Tóth et al., 2019; Xiao et al., 2020). Before the introduction of molecular tests and further biochemical investigations, C. orthopsilosis, and C. metapsilosis used to be classified into the C. parapsilosis complex (Tavanti et al., 2005). In addition, L. elongisporus, which may be misidentified as C. parapsilosis by convientional methods, remains underestimated in its clinical significance (Lockhart et al., 2008; Al-Obaid et al., 2018). However, the incidence, pathogenicity, and drug resistance of species within the C. parapsilosis complex has been shown to be different (Neji et al., 2017; Xiao et al., 2020). Overall, there was a significant difference in the performance of Autof MS 1000 (99.0%) and Vitek MS (79.1%) in assigning C. parapsilosis complex isolates to the correct species-level. Of note, Vitek MS system exhibited significant limitations in correctly identifying C. orthopsilosis (accuracy 23.1%) and C. metapsilosis (accuracy 4.7%), while Autof MS 1000 correctly identified all 64 C. metapsilosis and 96.2% of C. orthopsilosis isolates.
C. glabrata has emerged as the second most common cause of invasive candidiasis in the United States (Pfaller and Diekema, 2007), while in China it ranks fourth among clinically invasive yeast infections (Xiao et al., 2015). Its phylogenetically related species, C. nivariensis and C. bracarensis, were previously identified as C. glabrata by routine identification methods (Alcoba-Flórez et al., 2005; Correia et al., 2006). However their clinical significance and epidemiological role in candidiasis is different (Bishop et al., 2008). In this study, both systems correctly identified 98.9% of C. glabrata isolates. Autof MS 1000 system correctly identified all three C. nivariensis and one C. bracarensis isolates. However, no C. nivariensis or C. bracarensis isolates were correctly identified by Vitek MS. Of note, C. bracarensis is not included in the Vitek MS database IVD 3.0.
The basidiomycetous yeasts of the C. neoformans-C. gattii species complex are the causative agents of cryptococcosis with different pathogenicity (Samarasinghe and Xu, 2018). C. neoformans is more common in AIDS patients, whereas infections caused by C. gattii are more often reported in immunocompetent patients (Kwon-Chung et al., 2015). Previously, conventional L-canavanine glycine bromothymol blue (CGB) agar was used to distinguish the two species (Chen et al., 2014). In this study, Vitek MS and Autof MS 1000 correctly assigned 97.4% and 99.7% of C. neoformans isolates to species level, respectively. However, in 10 C. gattii isolates, the Vitek MS came up with a “ no identification” result in three isolates and misidentified two isolates as C. neoformans. In contrast, Autof MS 1000 accurately identified 90% of the C. gattii isolates with only one isolate misidentified as C. neoformans.
C. auris has recently drawn much attention clinically due to its multidrug resistant characteristics and fast spread worldwide (Spivak and Hanson, 2018). C. auris, and its closely-related species C. haemulonii, C. duobushaemulonii, and C. pseudohaemulonii, are difficult to identify using standard laboratory methods (Muñoz et al., 2018). In the identification of this species complex, both Autof MS 1000 and Vitek MS performed well, with only one isolate of C. duobushaemulonii not identified by Vitek MS.
The principle of MALDI-TOF MS in microbial identification is based on using scoring algorithms to match analyzed spectra with reference spectra (Freiwald and Sauer, 2009; Jang and Kim, 2018). Regardless of the instruments used, distinct algorithms in the same platform result in different identification reports (Panagea et al., 2015; Leyer et al., 2017). For Autof MS 1000, just like MALDI BioTyper (Bruker, Billerica, MA), the database is based on an isolate-specific references approach, while for Vitek MS, it is based on a taxonomical group-specific approach (Cassagne et al., 2016). In this study, Autof MS 1000 performed better than Vitek MS in the identification of closely-related yeasts causing invasive fungal diseases. We suppose that differences in reference spectral databases and scoring algorithms of these two platforms may contribute to the performance discrepancies.
Our study has some limitations. We only evaluated the systems’ performance on closely-related yeast species commonly seen in patients, it is not clear whether similar results would have been obtained on other yeast and filamentous fungal species. On the other hand, Vitek MS v3.0 System has shown its excellent accuracy for the identification of filamentous fungi (Rychert et al., 2018). Therefore, further investigations are needed for validating the performance of Autof MS 1000 (compared to other widely-used MALDI-TOF MS systems), in the identifitication of a broader range of bacterial and fungal species.
Conclusion
MALDI-TOF MS has previously proven to be valuable in the routine identification of yeast species. In this study, Autof MS 1000 exhibited higher identification accuracy than Vitek MS system in the identification of yeast isolates within different species complexes. The identification capacity of Vitek MS, especially in identifying less-common species within phylogenetically closely-related species complexes, still needs to be improved.
Data Availability Statement
The raw data supporting the conclusions of this article will be made available by the authors, without undue reservation.
Author Contributions
QY processed the experimental data, performed the analysis, drafted the manuscript, designed the tables, and revised the manuscript. MX, the main conceptual ideas and proof outline, performed the analysis and revised the manuscript. XF, GZ, YY, J-JZ, and S-MD contributed to sample preparation, performed MALDI-TOF MS identification. J-WC, YL, M-LZ, S-YY, J-JH, X-FC, and XH carried out the experiments, performed 16S rDNA sequencing identification. FK, TK, and Y-CX revised the manuscript, and Y-CX was involved in planning and supervised the work. All authors contributed to the article and approved the submitted version.
Funding
This work was supported by a National Major Science and Technology Project (grant number 2018ZX10712001); Beijing Hospitals Authority Youth Programe (QML20190301); the Natural Science Foundation of China (81802042, 81971979, 81802049); Beijing Nova Program (Z201100006820127); Outstanding Young Talents Cultivation Program in Dongcheng District (2018 grant to MX); Beijing Key Clinical Specialty for Laboratory Medicine - Excellent Project (No. ZK201000).
Conflict of Interest
The authors declare that the research was conducted in the absence of any commercial or financial relationships that could be construed as a potential conflict of interest.
Acknowledgments
We would like to acknowledge the co-principal investigators in the 57 hospitals participating in the China Hospital Invasive Fungal Surveillance Net (CHIF-NET) program for offering the yeast isolates. They are: (1) Zhang Qiang-Qiang, Huashan Hospital, Fudan University; (2) Liu Yun, Shanghai Changhai Hospital; (3) Xu Ying-Chun, Peking Union Medical College Hospital, Chinese Academy of Medical Sciences; (4) Chu Yun-Zhuo, First Hospital of China Medical University; (5) Liao Kang, the First Affiliated Hospital of Zhongshan University; (6) Sun Zi-Yong, Tongji Hospital, Tongji Medical College Huazhong University of Science & Technology; (7) Yu Hua, Sichuan Academy of Medical Sciences & Sichuan Provincial People’s Hospital; (8) Liu Peng-Peng, Affiliated Hospital of Medical College, Qingdao University; (9) Hu Xin-Lan, Fujian Provincial Hospital; (10) Kong Hai-Shen, Sir Run Run Shaw Hospital School of Medicine, Zhejiang University; (11) Mei Ya-Ning, Jiangsu Province People’s Hospital; (12) Li Qing-Feng, Public Health Clinical Medical Center of Chengdu; (13) Luo Yan-Ping, the General Hospital of PLA; (14) Meng Ling, Second Affiliated Hospital of Lanzhou University; (15) Kang Mei, Department of Laboratory Medicine, West China Hospital, Sichuan University; (16) Liu Zhi-Yong, Southwest Hospital Affiliated the Third Military Medical University; (17) Yu Yun-Song, Sir Run Run Shaw Hospital; (18) Jia Wei, The General Hospital Affiliated to Ningxia Medical University; (19) Pan Yu-Hong, Fujian Medical University Union Hospital; (20) Xi Hai-Yan, General Hospital of Nanjing Military Area Command; (21) Hu Zhi-Dong, General Hospital of Tianjin Medical University; (22) Fei Ying, The Affiliated Hospital of Guiyang Medical College; (23) Wang Shan-Mei, Henan Provincial People’s Hospital; (24) Zhang Li-Ping, the First Affiliated Hospital of Chongqing Medical Hospital; (25) Yang Bin, First Affiliated Hospital of Fujian Medical University; (26) Liu Wen-En, Xiangya Hospital Central South University; (27) Li Yi-Min, The First Affiliated Hospital of Guangzhou Medical Collage; (28) Liang Jia-Yin, The Third Hospital Attached to Zhongshan University; (29) Huang Jian-Sheng, Central Hospital of Lishui City; (30) Ma Ling, Union Hospital Tongji Medical College of Huazhong University of Science and Technology; (31) Xu Xiu-Li, Xining Hospital, Fourth Military Medical University; (32) Yang Yin-Mei, Guangzhou First People's Hospital Nansha Hospital; (33) Wu Jin-Ying, The Affiliated Yantai Yuhuangding Hospital of Qingdao University; (34) Shan Bin, the First Affiliated Hospital, Kunming Medical University; (35) Li Ruo-Yu, Peking University First Hospital; (36) Zhang Qing, Third Affiliated Hospital of Wenzhou Medical College; (37) Cao Wei, The Second Xiangya Hospital of Central South University; (38) Lu Wei-Ping, Daping Hospital, The Third Affiliated Hospital of Third Military Medical University; (39) Zhang Rong, the Second Affiliated Hospital of Zhejiang University School of Medicine; (40) Zhang Jian-Lei, Tianjin First Centre Hospital; (41) Lei Jin-E, the First Affiliated Hospital of Xi’an Jiaotong University; (42) Ma Xiao-Ling, the First Affiliated Hospital of University of Science and Technology of China; (43) Wang Shu-Feng, First Hospital of Shanxi Medical University; (44) Liang Hong-Jie, the First Affiliated Hospital of Guangxi Medical University; (45) Zhou Ting-Yin, Shanghai Changzheng Hospital; (46) Chen Xiang-Yang, People’s Hospital of Zhengzhou; (47) Li Juan, People’s Hospital of Xinjiang Uygur Autonomous Region; (48) Jin Yan, Shandong Provincial Hospital; (49) Ha Xiao-Qin, General Hospital of Lanzhou Military Region; (50) Ji Ping, the First Hospital of Xinjiang Medical University; (51) Yu Su-Fei, Taizhou Hospital of Zhejiang Province; (52) Wang Xiao-Ning, the First Hospital of Jilin University; (53) Gu Yi-Hai, 3201 Hospitals of Hanzhong; (54) Wang Hai-Bin, First Affiliated Hospital of PLA General Hospital; (55) Zhang Xian-Feng, The First Affiliated Hospital of Suzhou; (56) Kang Jian-Bang, The Second Hospital of Shanxi Medical University; (57) Xiao Qun, the 94th Hospital of Chinese People’s Liberation Army.
References
Alcoba-Flórez J., Méndez-Álvarez S., Cano J., Guarro J., Pérez-Roth E., Del Pilar Arévalo M. (2005). Phenotypic and molecular characterization of Candida nivariensis sp. nov., a possible new opportunistic fungus. J. Clin. Microbiol. 43, 4107–4111. doi: 10.1128/JCM.43.8.4107-4111.2005
Al-Obaid K., Ahmad S., Joseph L., Khan Z. (2018). Lodderomyces elongisporus: a bloodstream pathogen of greater clinical significance. New Microbes New Infect. 26, 20–24. doi: 10.1016/j.nmni.2018.07.004
Bishop J. A., Chase N., Magill S. S., Kurtzman C. P., Fiandaca M. J., Merz W. G. (2008). Candida bracarensis detected among isolates of Candida glabrata by peptide nucleic acid fluorescence in situ hybridization: Susceptibility data and documentation of presumed infection. J. Clin. Microbiol. 46, 443–446. doi: 10.1128/JCM.01986-07
Cassagne C., Normand A. C., L’Ollivier C., Ranque S., Piarroux R. (2016). Performance of MALDI-TOF MS platforms for fungal identification. Mycoses 59, 678–690. doi: 10.1111/myc.12506
Chen S. C., Meyer W., Sorrell C. (2014). Cryptococcus gattii Infections. Cryptococcus Gattii Infect. 27, 980–1024. doi: 10.1128/CMR.00126-13
Correia A., Sampaio P., James S., Pais C. (2006). Candida bracarensis sp. nov., a novel anamorphic yeast species phenotypically similar to Candida glabrata. Int. J. Syst. Evol. Microbiol. 56, 313–317. doi: 10.1099/ijs.0.64076-0
Freiwald A., Sauer S. (2009). Phylogenetic classification and identification of bacteria by mass spectrometry. Nat. Protoc. 4, 732–742. doi: 10.1038/nprot.2009.37
Harrington R., Kindermann S. L., Hou Q., Taylor R. J., Azie N., Horn D. L. (2017). Candidemia and invasive candidiasis among hospitalized neonates and pediatric patients. Curr. Med. Res. Opin. 33, 1803–1812. doi: 10.1080/03007995.2017.1354824
Jang K. S., Kim Y. H. (2018). Rapid and robust MALDI-TOF MS techniques for microbial identification: a brief overview of their diverse applications. J. Microbiol. 56, 209–216. doi: 10.1007/s12275-018-7457-0
Kwon-Chung K. J., Fraser J. A., Doering T. Á. L., Wang Z. A., Janbon G., Idnurm A., et al. (2015). Cryptococcus neoformans and Cryptococcus gattii, the etiologic agents of cryptococcosis. Cold Spring Harb. Perspect. Med. 4, a019760. doi: 10.1101/cshperspect.a019760
Leyer C., Gregorowicz G., Mougari F., Raskine L., Cambau E., De Briel D. (2017). Comparison of saramis 4.12 and IVD 3.0 vitek MS matrix-assisted laser desorption ionization-time of flight mass spectrometry for identification of mycobacteria from solid and liquid culture media. J. Clin. Microbiol. 55, 2045–2054. doi: 10.1128/JCM.00006-17
Lockhart S. R., Messer S. A., Pfaller M. A., Diekema D. J. (2008). Lodderomyces elongisporus masquerading as Candida parapsilosis as a cause of bloodstream infections. J. Clin. Microbiol. 46, 374–376. doi: 10.1128/JCM.01790-07
Lockhart S. R., Etienne K. A., Vallabhaneni S., Farooqi J., Chowdhary A., Govender N. P., et al. (2017). Simultaneous emergence of multidrug-resistant candida auris on 3 continents confirmed by whole-genome sequencing and epidemiological analyses. Clin. Infect. Dis. 64, 134–140. doi: 10.1093/cid/ciw691
Miceli M. H., Díaz J. A., Lee S. A. (2011). Emerging opportunistic yeast infections. Lancet Infect. Dis. 11, 142–151. doi: 10.1016/S1473-3099(10)70218-8
Muñoz J. F., Gade L., Chow N. A., Loparev V. N., Juieng P., Berkow E. L., et al. (2018). Genomic insights into multidrug-resistance, mating and virulence in Candida auris and related emerging species. Nat. Commun. 9. doi: 10.1038/s41467-018-07779-6
Neji S., Hadrich I., Trabelsi H., Abbes S., Cheikhrouhou F., Sellami H., et al. (2017). Virulence factors, antifungal susceptibility and molecular mechanisms of azole resistance among Candida parapsilosis complex isolates recovered from clinical specimens. J. Biomed. Sci. 24, 1–16. doi: 10.1186/s12929-017-0376-2
Odds F. C., Hanson M. F., Davidson A. D., Jacobsen M. D., Wright P., Whyte J. A., et al. (2007). One year prospective survey of Candida bloodstream infections in Scotland. J. Med. Microbiol. 56, 1066–1075. doi: 10.1099/jmm.0.47239-0
Panagea T., Pincus D. H., Grogono D., Jones M., Bryant J., Parkhill J., et al. (2015). Mycobacterium abscessus complex identification with matrix-assisted laser desorption ionization - Time of flight mass spectrometry. J. Clin. Microbiol. 53, 2355–2358. doi: 10.1128/JCM.00494-15
Pfaller M. A., Diekema D. J. (2007). Epidemiology of invasive candidiasis: A persistent public health problem. Clin. Microbiol. Rev. 20, 133–163. doi: 10.1128/CMR.00029-06
Rychert J., Slechta E. S., Barker A. P., Miranda E., Babady N. E., Tang Y. W., et al. (2018). Multicenter Evaluation of the Vitek MS v3.0 System for the Identification of Filamentous Fungi. J. Clin. Microbiol. 56, 1–11. doi: 10.1128/JCM.01353-17
Samarasinghe H., Xu J. (2018). Hybrids and hybridization in the Cryptococcus neoformans and Cryptococcus gattii species complexes. Infect. Genet. Evol. 66, 245–255. doi: 10.1016/j.meegid.2018.10.011
Spivak E. S., Hanson K. E. (2018). Candida auris: an Emerging Fungal Pathogen. J. Clin. Microbiol. 56, 1–10. doi: 10.1128/JCM.01588-17
Sullivan D. J., Westerneng T. J., Haynes K. A., Bennett D. E., Coleman D. C. (1995). Candida dubliniensis sp. nov.: phenotypic and molecular characterization of a novel species associated with oral candidosis in HIV-infected individuals. Microbiology 141, 1507–1521. doi: 10.1099/13500872-141-7-1507
Sun Y., Guo J., Chen R., Hu L., Xia Q., Wu W., et al. (2020). Multicenter evaluation of three different MALDI-TOF MS systems for identification of clinically relevant filamentous fungi. Med. Mycol. 59, 1–6. doi: 10.1093/mmy/myaa037
Tavanti A., Davidson A. D., Gow N. A. R., Maiden M. C. J., Odds F. C. (2005). Candida orthopsilosis and Candida metapsilosis spp. nov. to replace Candida parapsilosis groups II and III. J. Clin. Microbiol. 43, 284–292. doi: 10.1128/JCM.43.1.284-292.2005
Tóth R., Nosek J., Mora-Montes H. M., Gabaldon T., Bliss J. M., Nosanchuk J. D., et al. (2019). Candida parapsilosis: From genes to the bedside. Clin. Microbiol. Rev. 32, 1–38. doi: 10.1128/CMR.00111-18
Wang H., Fan Y. Y., Kudinha T., Xu Z. P., Xiao M., Zhang L., et al. (2016). A Comprehensive Evaluation of the Bruker Biotyper MS and Vitek MS Matrix-Assisted Laser Desorption Ionization-Time of Flight Mass Spectrometry Systems for Identification of Yeasts, Part of the National China Hospital Invasive Fungal Surveillance Net (CHIF). J. Clin. Microbiol. 54, 1376–1380. doi: 10.1128/JCM.00162-16
Wang Y., Chen X. F., Xie X. L., Xiao M., Yang Y., Zhang G., et al. (2019). Evaluation of VITEK MS, Clin-ToF-II MS, Autof MS 1000 and VITEK 2 ANC card for identification of Bacteroides fragilis group isolates and antimicrobial susceptibilities of these isolates in a Chinese university hospital. J. Microbiol. Immunol. Infect. 52, 456–464. doi: 10.1016/j.jmii.2018.12.009
Xiao M., Fan X., Chen S. C. A., Wang H., Sun Z. Y., Liao K., et al. (2015). Antifungal susceptibilities of Candida glabrata species complex, Candida krusei, Candida parapsilosis species complex and Candida tropicalis causing invasive candidiasis in China: 3 year national surveillance. J. Antimicrob. Chemother. 70, 802–810. doi: 10.1093/jac/dku460
Keywords: MALDI-TOF MS, closely-related yeasts, invasive fungal diseases, Autof MS 1000, Vitek MS
Citation: Yi Q, Xiao M, Fan X, Zhang G, Yang Y, Zhang J-J, Duan S-M, Cheng J-W, Li Y, Zhou M-L, Yu S-Y, Huang J-J, Chen X-F, Hou X, Kong F, Kudinha T and Xu Y-C (2021) Evaluation of Autof MS 1000 and Vitek MS MALDI-TOF MS System in Identification of Closely-Related Yeasts Causing Invasive Fungal Diseases. Front. Cell. Infect. Microbiol. 11:628828. doi: 10.3389/fcimb.2021.628828
Received: 13 November 2020; Accepted: 04 January 2021;
Published: 18 February 2021.
Edited by:
Yi-Wei Tang, Cepheid, United StatesReviewed by:
Bryan Schmitt, Indiana University Bloomington, United StatesCharles William Stratton, Vanderbilt University Medical Center, United States
Copyright © 2021 Yi, Xiao, Fan, Zhang, Yang, Zhang, Duan, Cheng, Li, Zhou, Yu, Huang, Chen, Hou, Kong, Kudinha and Xu. This is an open-access article distributed under the terms of the Creative Commons Attribution License (CC BY). The use, distribution or reproduction in other forums is permitted, provided the original author(s) and the copyright owner(s) are credited and that the original publication in this journal is cited, in accordance with accepted academic practice. No use, distribution or reproduction is permitted which does not comply with these terms.
*Correspondence: Ying-Chun Xu, eHljcHVtY2hAMTM5LmNvbQ==
†These authors have contributed equally to this work