- Nutrition Genetics and Biotechnology Division, Indian Council of Agricultural Research-Central Institute of Brackishwater Aquaculture, Chennai, India
The prevalence of bacterial diseases and the application of probiotics to prevent them is a common practice in shrimp aquaculture. A wide range of bacterial species/strains is utilized in probiotic formulations, with proven beneficial effects. However, knowledge of their role in inhibiting the growth of a specific pathogen is restricted. In this study, we employed constraint-based genome-scale metabolic modeling approach to screen and identify the beneficial bacteria capable of limiting the growth of V. harveyi, a common pathogen in shrimp culture. Genome-scale models were built for 194 species (including strains from the genera Bacillus, Lactobacillus, and Lactococcus and the pathogenic strain V. harveyi) to explore the metabolic potential of these strains under different nutrient conditions in a consortium. In silico-based phenotypic analysis on 193 paired models predicted six candidate strains with growth enhancement and pathogen suppression. Growth simulations reveal that mannitol and glucoronate environments mediate parasitic interactions in a pairwise community. Furthermore, in a mannitol environment, the shortlisted six strains were purely metabolite consumers without donating metabolites to V. harveyi. The production of acetate by the screened species in a paired community suggests the natural metabolic end product’s role in limiting pathogen survival. Our study employing in silico approach successfully predicted three novel candidate strains for probiotic applications, namely, Bacillus sp 1 (identified as B. licheniformis in this study), Bacillus weihaiensis Alg07, and Lactobacillus lindneri TMW 1.1993. The study is the first to apply genomic-scale metabolic models for aquaculture applications to detect bacterial species limiting Vibrio harveyi growth.
Introduction
Microbes naturally exist as a community with a complex web of interactions that define the microbial community structure. The coordinated interactions between microbes enable metabolic processes such as cross-feeding, degradation of complex saccharides (Freilich et al., 2011), or synthesis of complex molecules (McCarty and Ledesma-Amaro, 2019). Advancement in sequencing has provided a potential resource for constructing genome-scale metabolic models (GSM) that serve as a basis to explore metabolic capabilities in microbial communities. Constraint-based modeling is a well-established approach that has been successfully applied for in silico prediction of microbial interactions (Henson et al., 2019; Fang et al., 2020). This approach has been applied from individual to consortium of species with the potential to discern the metabolic capabilities and interactions that operate in a microbial community (Heinken and Thiele, 2015b; Magnúsdóttir et al., 2017).
Shrimp aquaculture is among the fastest-growing farming sectors globally in the coastal regions (FAO, 2020). However, the shrimp farming suffers from frequent bacterial, fungal, and viral outbreaks (Seibert and Pinto, 2012; Alfiansah et al., 2018). According to a survey of key shrimp farming states in India, economic losses owing to shrimp infections were 0.21 million tons worth $1.02 billion in the 2018–2019 fiscal year (Patil et al., 2021). Among the bacterial pathogens, the major Vibrio species affecting shrimp aquaculture include V. parahaemolyticus, V. vulnificus, V. furnissii, V. campbellii, V. harveyi, V. alginolyticus, and V. anguillarum (Saulnier et al., 2000; Kumar et al., 2020), leading to vibriosis.
A challenging problem that arises in this domain is the possible unscientific use of antibiotics and chemotherapeutic agents leading to drug or antibiotic-resistant bacteria in aquaculture (Kang et al., 2014). In the aquaculture sector, probiotics, prebiotics, and synbiotics are widely used to improve growth performance and enhance immunity and disease resistance (Hoseinifar et al., 2018). The probiotic organisms produce natural antimicrobial compounds such as organic acids, fatty acids, hydrogen peroxide, and bacteriocins that prevent pathogenic organism’s growth and confer positive health benefits to the host (Knipe et al., 2020). The application of probiotic in shrimp farming is a key factor implicated in bioremediation, water quality improvement (Verschuere et al., 2000), enhancing the nutritional benefit and antimicrobial activity against pathogenic microorganisms (Tamilarasu et al., 2021).
Earlier studies have reported the use of beneficial bacteria for their ability to inhibit pathogen through co-culture experiments supplemented with carbohydrates or prebiotics (Fooks and Gibson, 2002; Rurangwa et al., 2009). In a study, Fierro-Coronado et al. (2018) have investigated the role of fulvic acid in improving the survival of Litopenaeus vannamei challenged with Vibrio parahaemolyticus. However, the exact molecular mechanism by which the probiotic species suppress pathogen growth remain unknown, but their role in improving the health of shrimp is well established (García-Medel et al., 2020; Wang et al., 2020; Nair et al., 2021). Moreover, screening bacterial strains for probiotic properties on a large scale via conventional in vivo and in vitro methods is labor-intensive, time-consuming, and expensive. Further, screening at the species level is not sufficient as the beneficial properties are strain-dependent (Campana et al., 2017; McFarland et al., 2018). Therefore, it is essential to delineate the strains limiting the survival of pathogens through in silico approaches. These challenges, in turn, have rendered constraint-based genome-scale modeling approach as a choice for the selection of beneficial bacterial strains by analyzing the metabolic capabilities.
We developed a strain-specific genome-scale metabolic model concentrating on Lactobacilli, Bacilli, and Lactococci genera having closed-genome sequences. Moreover, the species from these genera have demonstrated health advantages (Sahandi et al., 2019). The pathogenic strain V. harveyi employed in this study is associated with infections in shellfish, finfish, corals, and molluscs, leading to substantial economic loss to the farmed species in both brackish water and marine aquaculture (Darshanee Ruwandeepika et al., 2012). The study aims to predict the potential species limiting the growth of V. harveyi and analyzes the metabolic interactions and exchanges operating under different nutrient environments in the microbial communities using in silico-based genome-scale metabolic models. The contributions made here accelerate the screening process by identifying the candidate strains for experimental validation to evaluate their efficacy against pathogenic strains. This study represents a pioneering work in screening the species through a constraint-based approach against a shrimp pathogen.
Methods
Dataset
The strains belonging to the genus, namely, Bacillus, Lactobacillus, and Lactococcus, for which the whole genome sequences were available, were used in the present study. The genome and protein sequences corresponding to three genera comprising 193 different strains were downloaded from NCBI RefSeq (as of 11/02/2020). This includes 106 strains of Bacillus, 81 strains of Lactobacillus, and 6 strains of Lactococcus. The dataset also contains a pathogenic strain V. harveyi QT520, linked to pathogenesis in marine organism (Supplementary Table 1).
Model Reconstruction
The genome-scale metabolic models were built with CarveMe v1.2.2. CarveMe generates automated models using a top-down approach for single and community species (Machado et al., 2018). CarveMe converts the universal metabolic model into an organism-specific model by removing reactions and metabolites that are unlikely to be present in the given organism. Each of the 194 protein sequences retrieved was given as an input in CarveMe to build a draft SBML model. The model statistics of 194 GSM generated is summarized in Supplementary Table 2. The resulting SBML models were gap filled and grown in a defined medium (Supplementary Table 3) in anaerobic conditions with biomass components specific to Gram-negative and Gram-positive bacteria to make the draft model functional. The individual models were merged in CarveMe using the merge community function into groups of varying sizes, and each strain was assigned its own compartment and a shared extracellular environment.
Computing Growth Using Flux Balance Analysis
Simulations were performed with COBRA toolbox v3.0 (Heirendt et al., 2011) in Matlab R2018b using the linear programming solver glpk. Each microbial consortium was subjected to flux balance analysis (FBA), a constraint-based approach to predict the flow of metabolites through a metabolic network (Orth et al., 2010). FBA uses linear programming formulation and operates under steady-state conditions using stoichiometric matrix obtained from metabolic models, mathematically expressed as follows:
where S is a stoichiometric matrix of size m×n, m is the number of metabolites, and n is the number of reactions, v represents the flux through all reactions, Lj and Uj are the lower and upper bound flux of each reaction j.
Simulations were performed by maximizing the objective function, i.e., the biomass reaction, while constraining the uptake rates of amino acids and essential vitamins/nutrients to −1 mmol/gDw/h. A substrate uptake of −10 mmol/gDw/h was constrained for glucose, fructose, mannitol, galactose, mannose, N-acetyl-D-glucosamine (N-acgam), ribose, glucoronate, and arabinose, and uptake of −5 mmol/gDw/h for sucrose, maltose, cellobiose, trehalose, lactose, and maltotriose environments (Supplementary Table 4). All the models were simulated in an anaerobic environment by setting the lower bounds of oxygen exchange to zero. An in silico growth rate of at least 0.01/h was considered the organism’s ability to take up the carbon source.
Flux Variability Analysis
Flux variability analysis (FVA) computes the flux range through each reaction while maintaining maximum biomass production (Mahadevan and Schilling, 2003).
where v represents the maximum and minimum flux through each reaction j. Due to the long computation time, we performed FVA only on the shortlisted models to determine the production of major short-chain fatty acids (SCFA), such as acetate, lactate, ethanol, formate, succinate, and butyrate. The flux value above 1e-06 mmol/gDW/h was considered an organism’s ability to produce the metabolites while maximizing the biomass reaction.7
Categorizing Paired Communities
We classified the strains into three categories as suggested by Heinken and Thiele (2015b), summarized as follows: (i) An increase in the growth rate of at least 10% under pairwise community compared to a single strain grown in the same environment, and unchanged growth of the pathogen in relation to a single strain. (ii) A strain with the same growth rate in paired and single in the same environment and a 10% decrease in growth for the pathogen over the single strain. (iii) A minimum of 10% increase in growth rate in paired over single in the same environment and a 10% decrease in growth of pathogen over single in same environment.
Computing Metabolic Exchanges
Species Metabolic Interaction Analysis (SMETANA v1.2.0), a mixed-integer linear programming method, was used to compute the metabolites exchanged between species in a paired community under a defined medium (Zelezniak et al., 2015). The smetana command was used with the flags “–flavor cobra” with detailed mode using the default CPLEX solver. Among the different scores generated, we analyzed only the SMETANA score, as this potentially indicates the strength of metabolic interactions between organisms. SMETANA score varies between 0 and 1, wherein 0 represents no interaction and 1 represents certain interaction between species.
Phylogenetic Analysis
The phylogenetic analysis was performed with MEGA7.0 software using the 16S rRNA genes of shortlisted species to understand their phylogenetic relationship (Kumar et al., 2016). We used MEGA software’s ClustalW program for multiple sequence alignment with bootstrap set to 1,000 using the maximum likelihood method to generate phylogenetic trees.
Average Nucleotide Identity
The average nucleotide identity (ANI) indices were computed using the Python library pyANI v0.20 to study genome level similarities among the selected species (Pritchard, 2015). PyANI uses Mummer or Blast to compute similarity indices. ANI was run with the argument “ANIm”, which calculates indexes with Mummer.
Statistical Analysis
The growth values derived from FBA were subjected to non-parametric one-way ANOVA with Kruskal-Wallis test using the R package for all combinations of shortlisted six species across four different media. The data visualization was performed with ggplot2.
Results
Screening Potential Species Based on Growth Benefit and Suppression of Pathogen
As an initial core analysis, each model was subject to FBA in an anaerobic media with single carbon source (15 different carbon sources were used). As shown in Supplementary Figure 1, the single models of 193 strains exhibited a wide range of simulated growth rates in each of the 15 nutrient environments. About carbon source utilization, 32 strains comprising 20 Bacilli, 11 Lactobacilli, and 1 Lactococci showed fermentation in all 15 environments. All 193 individual models showed in silico growth in maltose and glucose environments. Most strains (40–70%) fermented the carbon sources glucoronate, arabinose, and galactose. The strains L.kunkeei MP2 and L. sp. BHWM 4 are the least fermenting ones that utilized only five carbon sources. Additionally, phenotypic prediction on the pathogenic strain V. harveyi revealed no in silico growth under arabinose (Supplementary Figure 2).
Next, the single 193 models were merged with the pathogenic strain V. harveyi to perform pairwise community simulations. Only 48% of the strains in a paired community showed in silico growth, with only 30% showing a 10% increase in growth compared to single strains under diverse nutrient environments, as shown in Figure 1. Moreover, only 30% of strains in the paired community grew on the sole carbon sources, namely, glucose and maltose, compared to the single strains, where all 193 strains grew. In a paired community, three strains, namely, B. thermoamylovorans SSBM, B. weihaiensis Alg07, and L. sp. Koumiss, could survive across all nutrient environments.
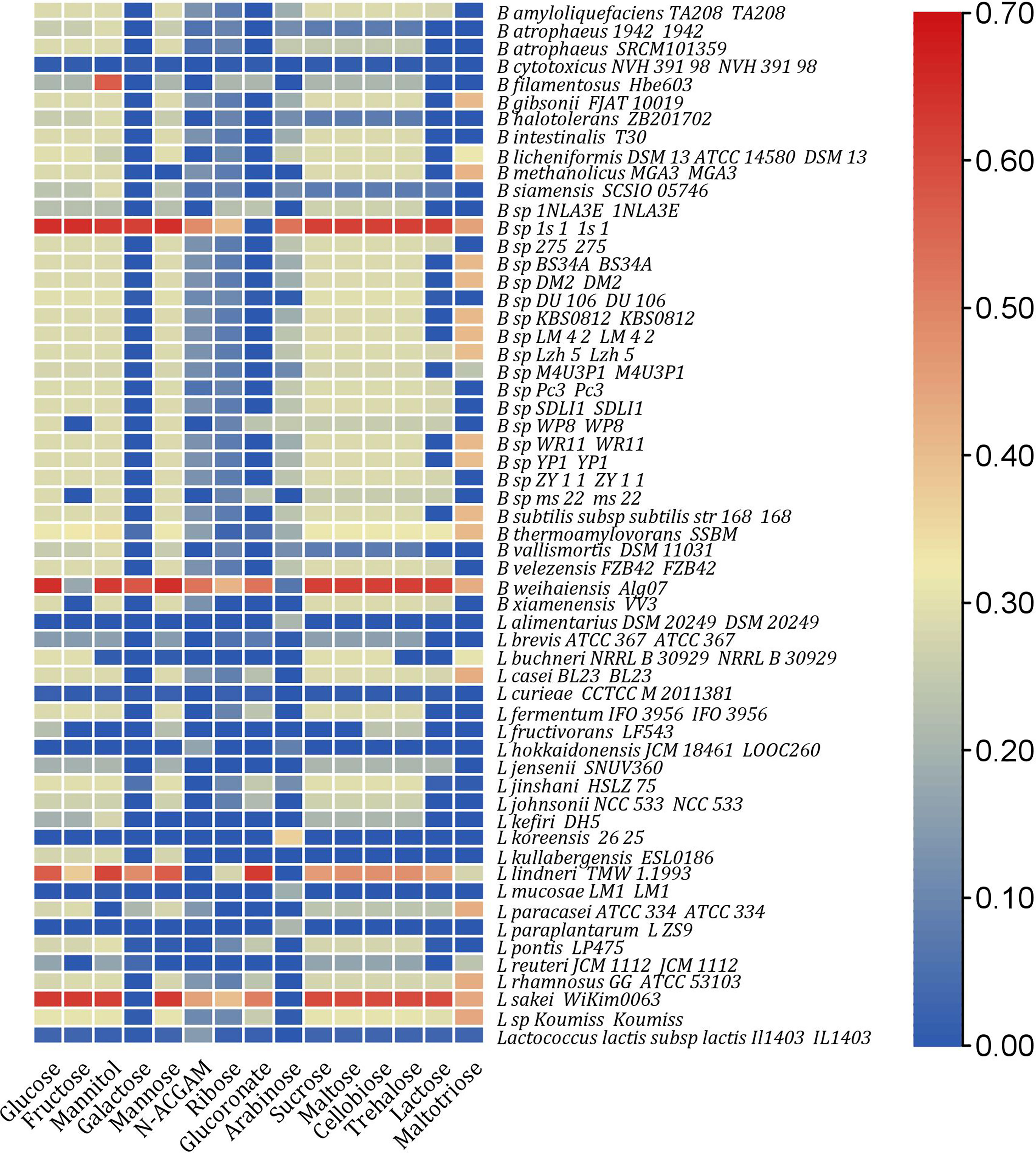
Figure 1 Heat map representing computed growth rates of strains with significant increase in growth rates (at least 10% uplift in in silico growth rate than single species) in a paired community with 15 different nutrient environments.
In paired communities, V. harveyi could grow with 186 out of 193 strains, as shown in Supplementary Figure 3, on 15 different nutrient environments. Under galactose environment, V. harveyi showed significant growth in silico. It was noted that only nine strains, namely, Bacillus sp 1s 1, B. thermoamylovorans SSBM, B. weihaiensis Alg07, L. jinshani HSLZ 75, L. lindneri TMW 1.1993, L. paracasei ATCC 334, L. pontis LP475, L. reuteri JCM 1112, and L. sp Koumiss, limited the growth of the pathogenic strain under galactose environment. Additionally, growth simulation revealed a limited growth for V. harveyi under mannitol environment in a paired community.
Mannitol Exhibits Parasitic Interaction in Pairwise Community Models
Based on the in silico phenotypic analysis, we categorized the strains as tabulated in Supplementary Table 5. We focussed our analysis on the paired models where an increase in growth rate was observed for the strains while a limited in silico growth rate for pathogen. A total of 48 strains, comprising 30 bacilli and 18 lactobacilli, fall into this category.
Prior work by Heinken and Thiele (2015a) has classified microbial communities into six types of interactions based on growth rates: parasitism, amensalism, commensalism, mutualism, neutralism, and competition. As the study’s goal was to identify the strains that limit the growth of the pathogen as well as enhance the growth of the strains, we were interested in parasitic interaction. Our analysis revealed that only 15% of the strains accounted for parasitic interactions (Supplementary Figure 4). The six strains, namely, Bacillus sp 1s 1, B. weihaiensis Alg07, L. sakei WiKim0063, L. sp Koumiss, L. lindneri TMW 1.1993, and L. buchneri NRRL B 30929, which showed parasitic interaction, also outperformed the other species in at least five nutrient environments, both improving growth and limiting pathogen survival (Figure 2). The different interaction types exhibited by 48 strains are tabulated in Supplementary Table 6.
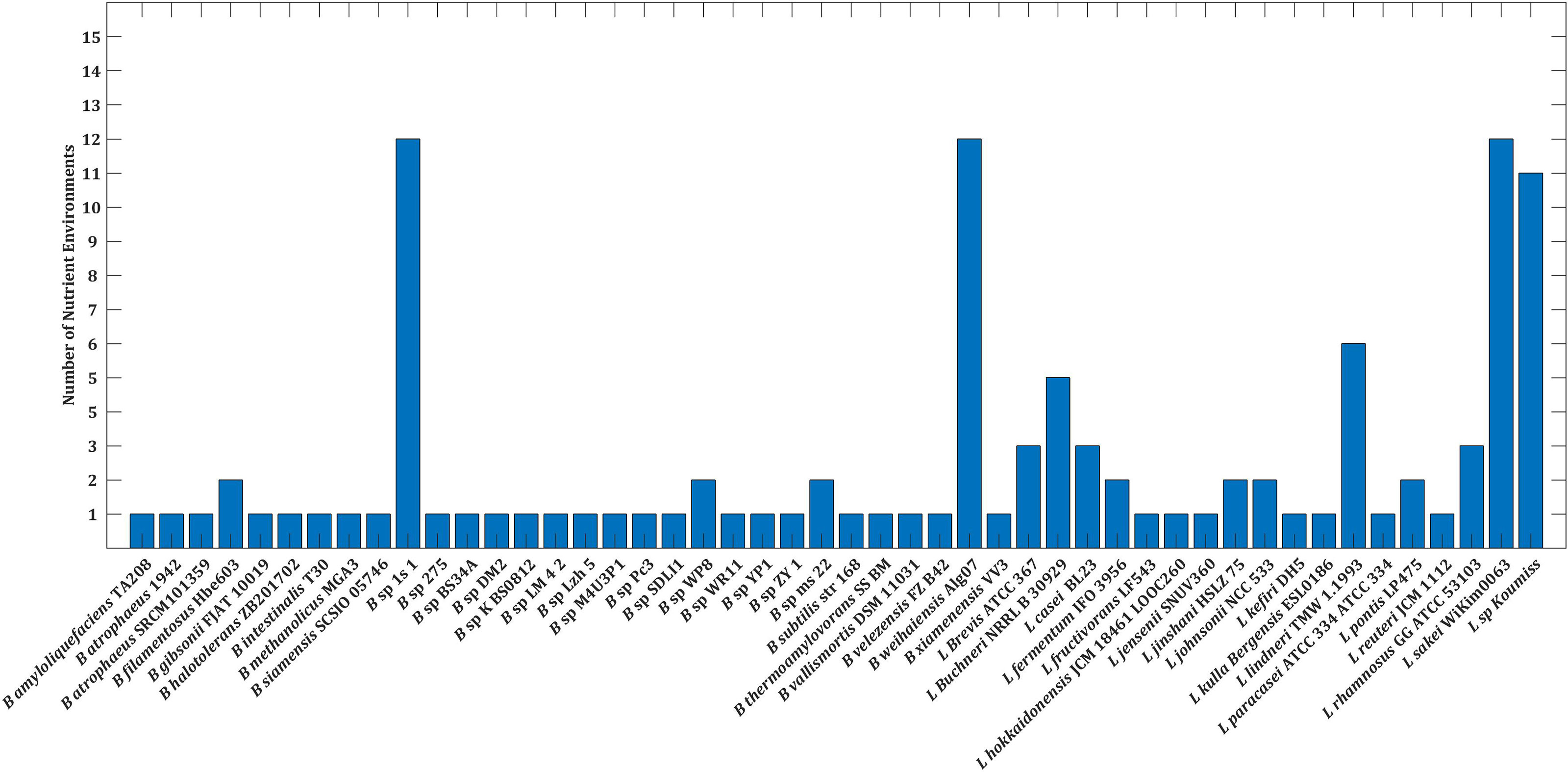
Figure 2 Barplot depicting the number of nutrient environments in which strains limits growth of V. harveyi.
Out of the 15 nutrient environments used in this study, pathogen survival was limited majorly in the mannitol environment, as shown in Supplementary Figure 5. Analysis of the 48 strains reveals metabolic gains on four species, namely, B. thermoamylovorans SSBM, B. weihaiensis Alg07, L. jinshani HSLZ 75, and L. lindneri TMW 1.1993. Among the four species, the highest metabolic gain is achieved by L. lindneri TMW 1.1993, upon paired with the pathogen (Supplementary Figure 6).
Cross-Fed Metabolites in Pairwise Community
SMETANA identified 87 metabolites cross-fed across the pairwise community for the 48 filtered species (Supplementary Table 7). Among the metabolites predicted, minerals and amino acids were frequently exchanged. In addition, lactate, acetate, ethanol, fumarate, and succinate have also been predicted to be exchanged. Out of the 87 pan metabolites predicted in silico, 58 metabolites were environment independent, with exchange occurring in all nutrient environments.
Moreover, out of the 48 strains, eight strains, namely, L. fermentum IFO 3956, L. hokkaidonensis JCM 18461 LOOC260, L. kullabergensis ESL0186, L. lindneri TMW 1.1993, L. pontis LP475, L. reuteri JCM 1112, L. sakei WiKim0063, and L. sp Koumiss, were only consumers, i.e., receiving metabolites from the pathogen based on our in silico predictions. All these strains have shown the ability to benefit from pathogen across all the nutrient environments by consuming metabolites. Among the metabolites cross-fed in the shortlisted strains (Figure 3), L. lindneri TMW 1.1993 achieved significant in silico growth benefits by uptaking carbon sources, namely, glucose and ribose, predicted to be secreted from the pathogen.
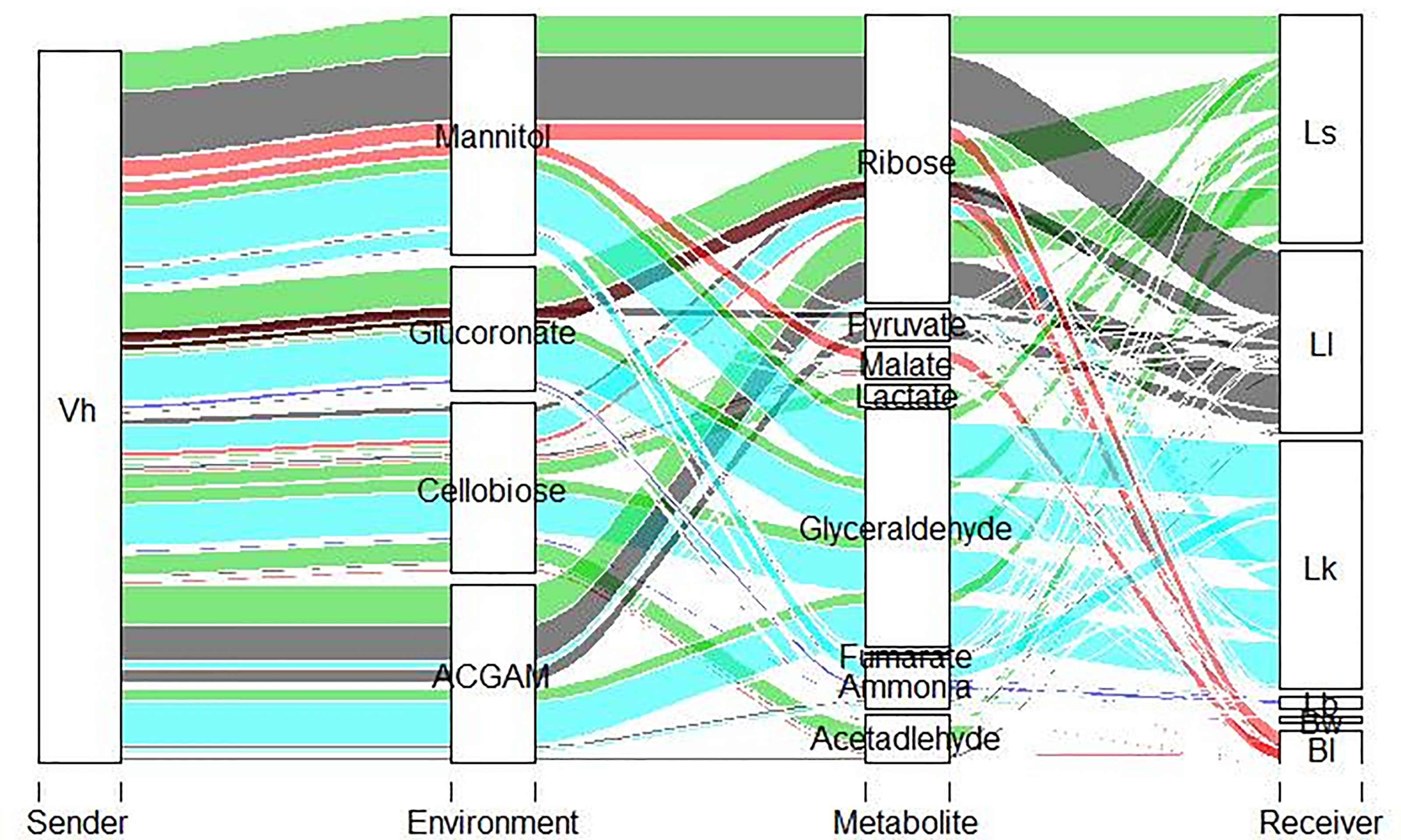
Figure 3 Alluvial plot depicting top metabolites exchanged between shortlisted species and V. harveyi with thickness of the line denoting the SMETANA scores, which range between 0 and 1.
Short-Chain Fatty Acids Profile Across Nutrient Environments
The flux profiles, i.e., the qualitative increase or decrease of SCFA, were inferred using FVA in single and paired models to contextualize the pairwise community models towards production of metabolites across environments. When comparing pairwise communities to single species, acetate and ethanol fluxes increased. The lactate production potential of 48 species varied significantly, with single models exhibiting higher flux than pairwise community models. Similarly, the ability of the organism to produce succinate and fumarate was higher in single species. All 48 paired species were unable to produce butyrate. A total of 10 species, as listed in Supplementary Table 8, could synthesize five of the six metabolites in at least one of the nutritional conditions.
Community Simulation With Potential Species
To unravel how the interactions among shortlisted species limit the growth of V. harveyi, we made merged models from all possible combinations of six strains for generating different consortia and subjected to FBA in four different nutrient environments. The four nutrients, namely, mannitol, N-acgam, glucoronate, and cellobiose, were chosen based on strain survival and limiting pathogen growth in these environments. The in silico growth rate listed in Supplementary Table 9 is the sum of the biomass reactions of the potential strains under each environment in different consortia. The results indicate that the maximum simulated growth rate of strains under mannitol, N-acgam, glucoronate, and cellobiose environment were 0.17, 0.14, 0.17, and 0.16/h, respectively. The consortium consisting of Bacillus sp 1s, B. weihaiensis Alg07, and L. lindneri TMW 1.1993 was the most effective combinations in growth enhancement and limiting pathogen survival.
Non-parametric one-way ANOVA revealed a significant difference between the groups tested in community simulations. The test resulted in Kruskal-Wallis chi-square value of 10.10 at p-value <0.05, indicating the significance between the species combinations. The top 10 groups that exhibited the highest average scores are depicted in Figure 4. Among the total combinations, B. licheniformis, B. weihaiensis, and L. lindneri were the best combinations that exhibited the highest in silico growth rates in the presence of V. harveyi.
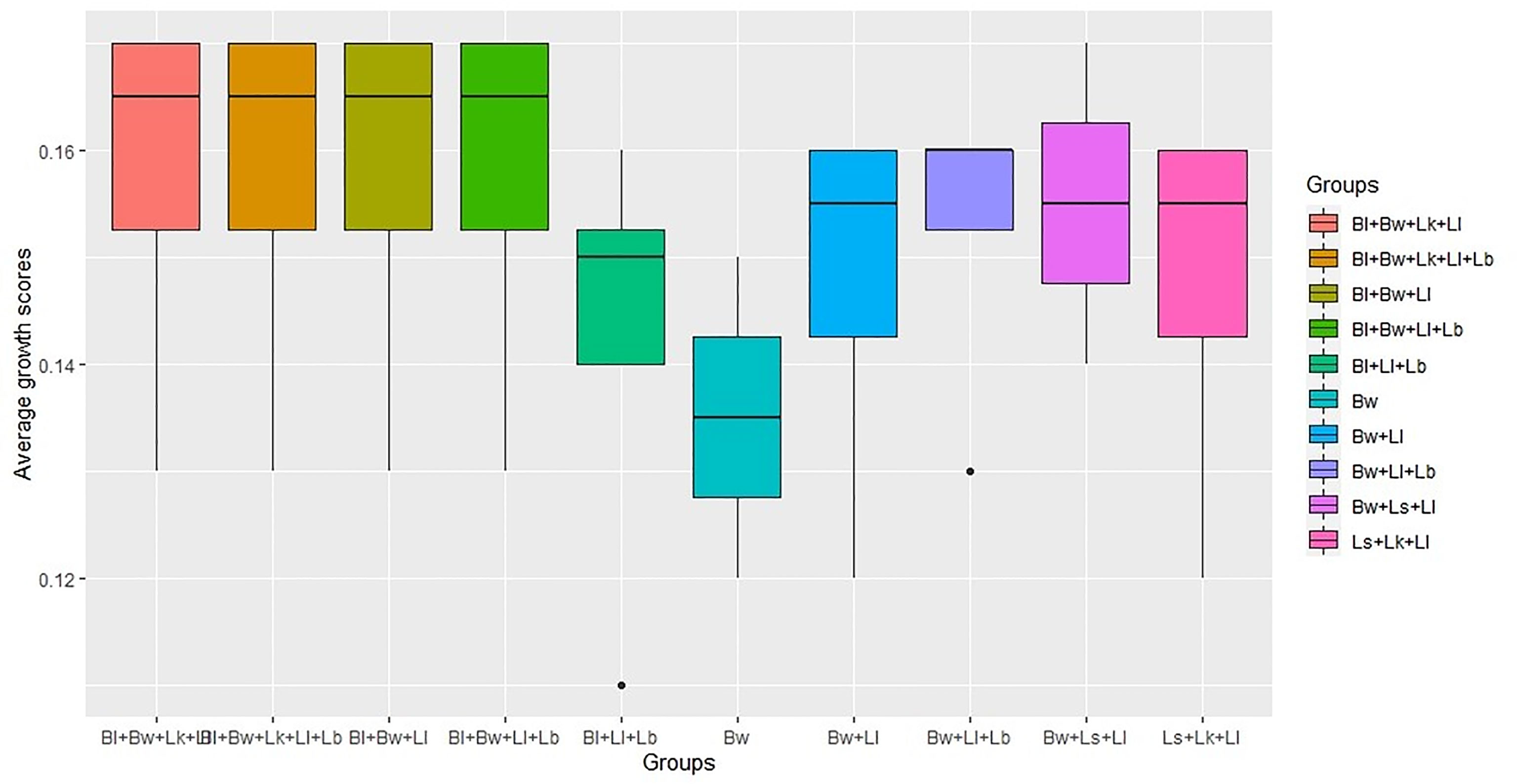
Figure 4 Top 10 community model groups with highest average growth scores (Bw, B. weihaiensis; Ll, L. lindneri; Bl, B. licheniformis; Ls, L. sakei; Lk, L. Koumiss; Lb, L. buchneri).
Discussion
This study reports the constraint-based metabolic modelling approach applied for the first time in the aquaculture ecosystem to screen potential strains limiting the growth of the pathogen. The individual models constructed from the genome of 193 strains exhibited better in silico growth in over 15 different nutrient environments. In contrast, limited in silico growth was observed with the paired models. The in silico growth impediment of strains observed in the paired models attributes to the metabolites derived from V. harveyi, a known property of the Vibrio spp. (Balakrishnan et al., 2014).
We identified 48 out of 193 strains through a flux-based approach to be ideal candidates limiting the growth of V. harveyi in specific nutrient environments. It is important to note that many strains out of these 48, like B. subtilis, L. casei, L. rhamnosus, L. paracasei, L. sakei, L. sp Koumiss, and L. buchneri, have already reported to be probiotic species (Lim et al., 2014; Hill et al., 2018; Westerik et al., 2018; Rhayat et al., 2019; Xu et al., 2019; García-Medel et al., 2020; Liao et al., 2020; Tang et al., 2020). Flux variability analysis revealed the production of SCFA, mainly acetate, by a majority of the strains in paired models, signifying the role of this natural by-product towards growth inhibition of the pathogen. Earlier, Mine and Boopathy (2011) also reported the role of organic acids such as formic acid, acetic acid, propionic acid, and butyric acid in inhibiting the V. harveyi.
The constraint-based approach employed in the current study screened several Lactobacilli strains as potential candidates limiting V. harveyi. The shortlisted strain L. buchneri NRRL B 30929 was originally isolated from an ethanol fermentation plant which has broad substrate utilization capability (Heinl and Grabherr, 2017).
In our in silico analysis, L. buchneri exhibited parasitic interaction limiting the pathogen surivival. Another shortlisted species, L. sakei, isolated from fish and meat products (Lim et al., 2014), reduce the count of Vibrio species in different live prey such as Artemia franciscana, Brachionus plicatilis, and Tigriopus japonicas (Sahandi et al., 2019). It has been reported that L. sakei showed a stronger killing effect on pathogenic V. parahaemolyticus, making it a promising candidate in controlling vibriosis (Le and Yang, 2018). The in silico analysis presented in this study revealed that L. sakei strain benefited from metabolites generated from V. harveyi, exhibiting a parasitic relationship by experiencing growth benefit.
L. lindneri is one of the novel Lactobacillus strains identified through constraint-based approach as a potential candidate against V. harveyi in our study. Interestingly, L. lindneri is associated with the following metabolic capabilities: (i) Flux-based Analysis revealed the ability to limit V. harveyi in many of the nutrient environments. (ii) Metabolic gain achieved by L. lindneri, which signifies a species’ ability to utilize a nutrient in a paired model where the same nutrient cannot be catabolized alone by the species. (iii) SMETANA-based analysis reveal L. lindneri as the consumer of metabolites donated by V.harveyi. In addition to the distinguished metabolic capabilities exhibited by L. lindneri in simulations performed in this study, it has also exhibited a close phylogenetic affiliation to known probiotic strains. The 16s rRNA-based phylogeny with a bootstrap value of 93% places greater confidence that L. lindneri is closely related to L. fructivorans (Supplementary Figure 7). The strain L. fructivorans is previously reported to significantly decrease larval mortality in sea bream (Carnevali et al., 2004).
Among the Bacillus strains shortlisted in this study, B. weihaiensis Alg07 exhibited parasitic interactions in the pairwise community. The B. weihaiensis Alg07 strain is associated with polysaccharide degradation and promotes the nutrient cycle and other essential functions in the marine ecosystem (Zhu et al., 2016). Another shortlisted strain, Bacillus sp 1s, exhibited proximity with B. licheniformis DSM 13 ATCC 14580 using ANI analysis (Supplementary Table 10). Several extracellular bio-compounds, including vitamins and enzymes, are produced by B. licheniformis, improving nutrient digestion and innate immunity (Tachibana et al., 2021). Moreover, the probiotic effect of B. licheniformis BCR 4-3 has been reported to increase resistance in Litopenaeus vannamei challenged with V. parahaemolyticus (García-Medel et al., 2020). In another study, the hemolytic activity of V. harveyi has been reported to be reduced by B. licheniformis (Nakayama et al., 2009).
In a microbial community, the nutrient environment significantly influences the interactions between species (Freilich et al., 2011). Our study highlighted the role of sugar alcohol mannitol, which retained its influence on growth benefit on beneficial species and limiting pathogen in different communities generated. The growth improvement effect of mannitol on Lactobacillus reported by Liong and Shah (2005) and limiting effect on V. harveyi observed in our study indicate the role of this sugar alcohol in the parasitic interactions. Simulations on multiple consortia generated with all possible combinations of potential strains revealed the community comprising Bacillus sp 1s, B. weihaiensis Alg07, and L. lindneri TMW 1.1993 to be the ideal combination in reducing the pathogen’s survival in the studied nutrient environments. This study also demonstrated the potential of genome-scale model to identify the ideal consortia of compatible potential strains for further testing and applications in the field.
The present study was conducted with the automated genome-scale model generated due to the non-availability of readily available manually curated published models. In addition, the scope of the study is limited to monosaccharide and disaccharide nutrient environments only. Future works with similar methods as followed in the study could provide further insights into the metabolic interactions between the probiotic and the pathogen if curated models with diverse nutrient environments are used and validated through experiments.
Conclusion
Large-scale screening for identifying beneficial microbes using experimental methods is difficult and time-consuming. However, the genome-scale modeling work presented here provides a feasible, effective, and alternative approach to accelerate the screening of larger microbial communities and shorten the path to experiments. Through the metabolic modelling approach, we can narrow down the search of potential probiotic candidates from the huge pool of species with closed genome availability, reducing experimental efforts and saving time and resources. The study also suggests the importance of nutrient environments in driving the parasitic interactions facilitating the growth of beneficial microbes. Moreover, with the exchange of metabolites observed in the paired models, the beneficial bacteria might gain an advantage and inhibit pathogen growth. Collectively, this work illustrated the importance of constraint-based genome-scale modeling to shortlist potential strains that could go for experimental validation.
Data Availability Statement
The original contributions presented in the study are included in the article/Supplementary Material. Further inquiries can be directed to the corresponding author.
Author Contributions
ND performed the study and analyzed the data and prepared the original draft of the manuscript. AJ, VK, and PP conceived, designed, and analyzed the study and read, revised, and approved the manuscript. SN performed bioinformatics analysis, and MS revised the manuscript. All authors contributed to the article and approved the submitted version.
Conflict of Interest
The authors declare that the research was conducted in the absence of any commercial or financial relationships that could be construed as a potential conflict of interest.
Publisher’s Note
All claims expressed in this article are solely those of the authors and do not necessarily represent those of their affiliated organizations, or those of the publisher, the editors and the reviewers. Any product that may be evaluated in this article, or claim that may be made by its manufacturer, is not guaranteed or endorsed by the publisher.
Acknowledgments
The authors are thankful to Indian Council of Agriculture Research (ICAR), New Delhi, for providing financial support to carry out this research under the “Network Project on Agricultural Bioinformatics and Computational Biology”. Authors are also thankful to Director, ICAR-CIBA for providing necessary support for carrying out this research.
Supplementary Material
The Supplementary Material for this article can be found online at: https://www.frontiersin.org/articles/10.3389/fcimb.2021.752477/full#supplementary-material
Supplementary Figure 1 | In silico growth rate of 193 species on 15 different nutrient environments.
Supplementary Figure 2 | In silico growth rate of Vibrio harveyi on 15 different nutrient environments.
Supplementary Figure 3 | Heat map representing the in silico growth of V. harveyi QT520 in a pairwise community across 15 different nutrient environments.
Supplementary Figure 4 | Interaction types exhibited by 48 species under 15 different nutrient environments. Red represents the maximum number of environments in which organisms present different types of interaction.
Supplementary Figure 5 | In silico nutrient uptake by screened 48 species in pairwise community models.
Supplementary Figure 6 | Barplot representing metabolic gains achieved by single and paired species.
Supplementary Figure 7 | Phylogenetic tree of 16S rRNA gene of screened species generated using maximum likelihood method in MEGA 7.0 with bootstrap set to 1,000.
References
Alfiansah, Y. R., Hassenrück, C., Kunzmann, A., Taslihan, A., Harder, J., Gärdes, A. (2018). Bacterial Abundance and Community Composition in Pond Water From Shrimp Aquaculture Systems With Different Stocking Densities. Front. Microbiol. 9, 2457. doi: 10.3389/fmicb.2018.02457
Balakrishnan, B., Ranishree, J. K., Thadikamala, S., Panchatcharam, P. (2014). Purification, Characterization and Production Optimization of a Vibriocin Produced by Mangrove Associated Vibrio Parahaemolyticus. Asian Pac. J. Trop. Biomed. 4, 253–261. doi: 10.12980/APJTB.4.2014C947
Campana, R., Van Hemert, S., Baffone, W. (2017). Strain-Specific Probiotic Properties of Lactic Acid Bacteria and Their Interference With Human Intestinal Pathogens Invasion. Gut Pathog. 9, 1–12. doi: 10.1186/s13099-017-0162-4
Carnevali, O., Zamponi, M. C., Sulpizio, R., Rollo, A., Nardi, M., Orpianesi, C., et al. (2004). Administration of Probiotic Strain to Improve Sea Bream Wellness During Development. Aquac. Int. 12, 377–386. doi: 10.1023/B:AQUI.0000042141.85977.bb
Darshanee Ruwandeepika, H. A., Sanjeewa Prasad Jayaweera, T., Paban Bhowmick, P., Karunasagar, I., Bossier, P., Defoirdt, T. (2012). Pathogenesis, Virulence Factors and Virulence Regulation of Vibrios Belonging to the Harveyi Clade. Rev. Aquac. 4, 59–74. doi: 10.1111/j.1753-5131.2012.01061.x
Fang, X., Lloyd, C. J., Palsson, B. O. (2020). Reconstructing Organisms in Silico: Genome-Scale Models and Their Emerging Applications. Nat. Rev. Microbiol. 12, 731–743. doi: 10.1038/s41579-020-00440-4
FAO. (2020). The 2020 Edition of The State of World Fisheries and Aquaculture. Nat. Resour. 35, 4–13. doi: 10.4060/ca9229en
Fierro-Coronado, J. A., Angulo, C., Rubio-Castro, A., Luna-González, A., Cáceres-Martínez, C. J., Ruiz-Verdugo, C. A., et al. (2018). Dietary Fulvic Acid Effects on Survival and Expression of Immune-Related Genes in Litopenaeus Vannamei Challenged With Vibrio Parahaemolyticus. Aquac. Res. 49, 3218–3227. doi: 10.1111/are.13789
Fooks, L. J., Gibson, G. R. (2002). In Vitro Investigations of the Effect of Probiotics and Prebiotics on Selected Human Intestinal Pathogens. FEMS Microbiol. Ecol. 39, 67–75. doi: 10.1016/S0168-6496(01)00197-0
Freilich, S., Zarecki, R., Eilam, O., Segal, E. S., Henry, C. S., Kupiec, M., et al. (2011). Competitive and Cooperative Metabolic Interactions in Bacterial Communities. Nat. Commun. 2, 589. doi: 10.1038/ncomms1597
García-Medel, D. I., Angulo, C., Escamilla-Montes, R., Fierro-Coronado, J. A., Diarte-Plata, G., Gámez-Jiménez, C., et al. (2020). Bacillus Licheniformis BCR 4-3 Increases Immune Response and Survival of Litopenaeus Vannamei Challenged With Vibrio Parahaemolyticus IPNGS16. Aquac. Int. 28, 2303–2318. doi: 10.1007/s10499-020-00585-2
Heinken, A., Thiele, I. (2015a). Anoxic Conditions Promote Species-Specific Mutualism Between Gut Microbes In Silico. Appl. Environ. Microbiol. 81, 4049–4061. doi: 10.1128/AEM.00101-15
Heinken, A., Thiele, I. (2015b). Systematic Prediction of Health - Relevant Humanmicrobial Co-Metabolism Through a Computational Framework. Gut Microbes 6, 85–92. doi: 10.1080/19490976.2015.1023494
Heinl, S., Grabherr, R. (2017). Systems Biology of Robustness and Flexibility: Lactobacillus Buchneri—A Show Case. J. Biotechnol. 257, 61–69. doi: 10.1016/j.jbiotec.2017.01.007
Heirendt, L., Arreckx, S., Pfau, T., Mendoza, S. N., Richelle, A., Heinken, A., et al. (2011). Creation and Analysis of Biochemical Constraint-Based Models Using the COBRA Toolbox V.3.0. Nat. Protoc. 6, 1290–1307. doi: 10.1038/nprot.2007.99
Henson, M. A., Orazi, G., Phalak, P., O’Toole, G. A. (2019). Metabolic Modeling of Cystic Fibrosis Airway Communities Predicts Mechanisms of Pathogen Dominance. mSystems 4, 1–20. doi: 10.1128/msystems.00026-19
Hill, D., Sugrue, I., Tobin, C., Hill, C., Stanton, C., Ross, R. P. (2018). The Lactobacillus Casei Group: History and Health Related Applications. Front. Microbiol. 9:2107. doi: 10.3389/fmicb.2018.02107
Hoseinifar, S. H., Sun, Y. Z., Wang, A., Zhou, Z. (2018). Probiotics as Means of Diseases Control in Aquaculture, a Review of Current Knowledge and Future Perspectives. Front. Microbiol. 9, 2429. doi: 10.3389/fmicb.2018.02429
Kang, C. H., Kim, Y. G., Oh, S. J., Mok, J. S., Cho, M. H., So, J. S. (2014). Antibiotic Resistance of Vibrio Harveyi Isolated From Seawater in Korea. Mar. Pollut. Bull. 86, 261–265. doi: 10.1016/j.marpolbul.2014.07.008
Knipe, H., Temperton, B., Lange, A., Bass, D., Tyler, C. R. (2020). Probiotics and Competitive Exclusion of Pathogens in Shrimp Aquaculture. Rev. Aquac. 13, 324–352. doi: 10.1111/raq.12477
Kumar, J. A., Kumar, K. V., Avunje, S., Akhil, V., Ashok, S., Kumar, S., et al. (2020). Phylogenetic Relationship Among Brackishwater Vibrio Species. Evol. Bioinform. 16, 1–8. doi: 10.1177/1176934320903288
Kumar, S., Stecher, G., Tamura, K. (2016). MEGA7: Molecular Evolutionary Genetics Analysis Version 7.0 for Bigger Datasets. Mol. Biol. Evol. 33, 1870–1874. doi: 10.1093/molbev/msw054
Le, B., Yang, S. H. (2018). Probiotic Potential of Novel Lactobacillus Strains Isolated From Salted-Fermented Shrimp as Antagonists for Vibrio Parahaemolyticus. J. Microbiol. 56, 138–144. doi: 10.1007/s12275-018-7407-x
Liao, C., Wang, T., Maslov, S., Xavier, J. B. (2020). Modeling Microbial Cross-Feeding at Intermediate Scale Portrays Community Dynamics and Species Coexistence. PloS Comput. Biol. 16, e1008135. doi: 10.1371/journal.pcbi.1008135
Lim, H. I., Lee, J., Jang, J. Y., Park, H. W., Choi, H. J., Kim, T. W., et al. (2014). Draft Genome Sequence of Lactobacillus Sakei Strain Wikim 22, Isolated From Kimchi in Chungcheong Province, South Korea. Genome Announc. 2, 195–196. doi: 10.1128/genomeA.01296-14
Liong, M. T., Shah, N. P. (2005). Optimization of Cholesterol Removal by Probiotics in the Presence of Prebiotics by Using a Response Surface Method. Appl. Environ. Microbiol. 71, 1745–1753. doi: 10.1128/AEM.71.4.1745-1753.2005
Machado, D., Andrejev, S., Tramontano, M., Raosaheb, K. (2018). Fast Automated Reconstruction of Genome-Scale Metabolic Models for Microbial Species and Communities. Nucleic Acids Res. 46, 7542–7553. doi: 10.1101/223198
Magnúsdóttir, S., Heinken, A., Kutt, L., Ravcheev, D. A., Bauer, E., Noronha, A., et al. (2017). Generation of Genome-Scale Metabolic Reconstructions for 773 Members of the Human Gut Microbiota. Nat. Biotechnol. 35, 81–89. doi: 10.1038/nbt.3703
Mahadevan, R., Schilling, C. H. (2003). The Effects of Alternate Optimal Solutions in Constraint-Based Genome-Scale Metabolic Models. Metab. Eng. 5, 264–276. doi: 10.1016/j.ymben.2003.09.002
McCarty, N. S., Ledesma-Amaro, R. (2019). Synthetic Biology Tools to Engineer Microbial Communities for Biotechnology. Trends Biotechnol. 37, 181–197. doi: 10.1016/j.tibtech.2018.11.002
McFarland, L. V., Evans, C. T., Goldstein, E. J. C. (2018). Strain-Specificity and Disease-Specificity of Probiotic Efficacy: A Systematic Review and Meta-Analysis. Front. Med. 5, 124. doi: 10.3389/fmed.2018.00124
Mine, S., Boopathy, R. (2011). Effect of Organic Acids on Shrimp Pathogen, Vibrio Harveyi. Curr. Microbiol. 63, 1–7. doi: 10.1007/s00284-011-9932-2
Nair, A. V., Leo Antony, M., Praveen, N. K., Sayooj, P., Raja Swaminathan, T., Vijayan, K. K. (2021). Evaluation of In Vitro and In Vivo Potential of Bacillus Subtilis MBTDCMFRI Ba37 as a Candidate Probiont in Fish Health Management. Microb. Pathog. 152, 104610. doi: 10.1016/j.micpath.2020.104610
Nakayama, T., Lu, H., Nomura, N. (2009). Inhibitory Effects of Bacillus Probionts on Growth and Toxin Production of Vibrio Harveyi Pathogens of Shrimp. Lett. Appl. Microbiol. 49, 679–684. doi: 10.1111/j.1472-765X.2009.02725.x
Orth, J. D., Thiele, I., Palsson, B.Ø. (2010). What is Flux Balance Analysis? Nat. Biotechnol. 28, 245–248. doi: 10.1038/nbt.1614.What
Patil, P. K., Geetha, R., Ravisankar, T., Avunje, S., Solanki, H. G., Abraham, T. J., et al. (2021). Economic Loss Due to Diseases in Indian Shrimp Farming With Special Reference to Enterocytozoon Hepatopenaei (EHP) and White Spot Syndrome Virus (WSSV). Aquaculture 533, 736231. doi: 10.1016/j.aquaculture.2020.736231
Pritchard, L. (2015). Genomics and Taxonomy in Diagnostics for Food Security : Soft-Rotting Enterobacterial Plant Pathogens Analytical Methods. Anal. Methods 00, 1–13. doi: 10.1039/C5AY02550H
Rhayat, L., Maresca, M., Nicoletti, C., Perrier, J., Brinch, K. S., Christian, S., et al. (2019). Effect of Bacillus Subtilis Strains on Intestinal Barrier Function and Inflammatory Response. Front. Immunol. 10, 564. doi: 10.3389/fimmu.2019.00564
Rurangwa, E., Laranja, J. L., Van Houdt, R., Delaedt, Y., Geraylou, Z., Van De Wiele, T., et al. (2009). Selected Nondigestible Carbohydrates and Prebiotics Support the Growth of Probiotic Fish Bacteria Mono-Cultures In Vitro. J. Appl. Microbiol. 106, 932–940. doi: 10.1111/j.1365-2672.2008.04034.x
Sahandi, J., Sorgeloos, P., Xiao, H., Wang, X., Qi, Z., Zheng, Y., et al. (2019). The Use of Selected Bacteria and Yeasts to Control Vibrio Spp. In Live Food. Antibiotics 8, 1–16. doi: 10.3390/antibiotics8030095
Saulnier, D., Haffner, P., Goarant, C., Levy, P., Ansquer, D. (2000). Experimental Infection Models for Shrimp Vibriosis Studies: A Review. Aquaculture 191, 133–144. doi: 10.1016/S0044-8486(00)00423-3
Seibert, C. H., Pinto, A. R. (2012). Challenges in Shrimp Aquaculture Due to Viral Diseases: Distribution and Biology of the Five Major Penaeid Viruses and Interventions to Avoid Viral Incidence and Dispersion. Braz. J. Microbiol. 43, 857–864. doi: 10.1590/S1517-83822012000300002
Tachibana, L., Telli, G. S., Dias, D., de, C., Gonçalves, G. S., Guimarães, M. C., et al. (2021). Bacillus Subtilis and Bacillus Licheniformis in Diets for Nile Tilapia (Oreochromis Niloticus): Effects on Growth Performance, Gut Microbiota Modulation and Innate Immunology. Aquac. Res. 52, 1630–1642. doi: 10.1111/are.15016
Tamilarasu, A., Ahilan, B., Gopalakannan, A., Somu Sunder Lingam, R. (2021). Evaluation of Probiotic Potential of Bacillus Strains on Growth Performance and Physiological Responses in Penaeus Vannamei. Aquac. Res. 52, 3124–3136. doi: 10.1111/are.15159
Tang, H., Ma, H., Hou, Q., Li, W., Xu, H., Liu, W., et al. (2020). Profiling of Koumiss Microbiota and Organic Acids and Their Effects on Koumiss Taste. BMC Microbiol. 20, 1–11. doi: 10.1186/s12866-020-01773-z
Verschuere, L., Rombaut, G., Sorgeloos, P., Verstraete, W. (2000). Probiotic Bacteria as Biological Control Agents in Aquaculture. Microbiol. Mol. Biol. Rev. 64, 655–671. doi: 10.1128/mmbr.64.4.655-671.2000
Wang, R., Guo, Z., Tang, Y., Kuang, J., Duan, Y., Lin, H., et al. (2020). Effects on Development and Microbial Community of Shrimp Litopenaeus Vannamei Larvae With Probiotics Treatment. AMB Express 10, 109. doi: 10.1186/s13568-020-01041-3
Westerik, N., Kort, R., Sybesma, W., Reid, G. (2018). Lactobacillus Rhamnosus Probiotic Food as a Tool for Empowerment Across the Value Chain in Africa. Front. Microbiol. 9, 1501. doi: 10.3389/fmicb.2018.01501
Xu, Y., Tian, Y., Cao, Y., Li, J., Guo, H., Su, Y., et al. (2019). Probiotic Properties of Lactobacillus Paracasei Subsp. Paracasei L1 and its Growth Performance-Promotion in Chicken by Improving the Intestinal Microflora. Front. Physiol. 10, 937. doi: 10.3389/fphys.2019.00937
Zelezniak, A., Andrejev, S., Ponomarova, O., Mende, D. R., Bork, P., Patil, K. R. (2015). Metabolic Dependencies Drive Species Co-Occurrence in Diverse Microbial Communities. Proc. Natl. Acad. Sci. U. S. A. 112, 6449–6454. doi: 10.1073/pnas.1421834112
Keywords: constraint-based, genome-scale model, microbial community, probiotics, vibriosis, shrimp
Citation: Devika NT, Jangam AK, Katneni VK, Patil PK, Nathamuni S and Shekhar MS (2021) In Silico Prediction of Novel Probiotic Species Limiting Pathogenic Vibrio Growth Using Constraint-Based Genome Scale Metabolic Modeling. Front. Cell. Infect. Microbiol. 11:752477. doi: 10.3389/fcimb.2021.752477
Received: 03 August 2021; Accepted: 02 September 2021;
Published: 29 September 2021.
Edited by:
Cristian Oliver, Austral University of Chile, ChileReviewed by:
Ansel Hsiao, University of California, Riverside, United StatesHector Abelardo González-Ocampo, CIIDIR Unidad Sinaloa, Mexico
Copyright © 2021 Devika, Jangam, Katneni, Patil, Nathamuni and Shekhar. This is an open-access article distributed under the terms of the Creative Commons Attribution License (CC BY). The use, distribution or reproduction in other forums is permitted, provided the original author(s) and the copyright owner(s) are credited and that the original publication in this journal is cited, in accordance with accepted academic practice. No use, distribution or reproduction is permitted which does not comply with these terms.
*Correspondence: Ashok Kumar Jangam, QXNob2suSmFuZ2FtQGljYXIuZ292Lmlu