- 1School of Life Sciences, Anhui Medical University, Hefei, Anhui, China
- 2Division of Gastroenterology, Union Hospital, Tongji Medical College, Huazhong University of Science and Technology, Wuhan, China
Faecalibacterium prausnitzii is a beneficial human gut microbe and a candidate for next-generation probiotics. With probiotics now being used in clinical treatments, concerns about their safety and side effects need to be considered. Therefore, it is essential to obtain a comprehensive understanding of the genetic diversity, functional characteristics, and potential risks of different F. prausnitzii strains. In this study, we collected the genetic information of 84 F . prausnitzii strains to conduct a pan-genome analysis with multiple perspectives. Based on single-copy genes and the sequences of 16S rRNA and the compositions of the pan-genome, different phylogenetic analyses of F. prausnitzii strains were performed, which showed the genetic diversity among them. Among the proteins of the pan-genome, we found that the accessory clusters made a greater contribution to the primary genetic functions of F. prausnitzii strains than the core and specific clusters. The functional annotations of F. prausnitzii showed that only a very small number of proteins were related to human diseases and there were no secondary metabolic gene clusters encoding harmful products. At the same time, complete fatty acid metabolism was detected in F. prausnitzii. In addition, we detected harmful elements, including antibiotic resistance genes, virulence factors, and pathogenic genes, and proposed the probiotic potential risk index (PPRI) and probiotic potential risk score (PPRS) to classify these 84 strains into low-, medium-, and high-risk groups. Finally, 15 strains were identified as low-risk strains and prioritized for clinical application. Undoubtedly, our results provide a comprehensive understanding and insight into F. prausnitzii, and PPRI and PPRS can be applied to evaluate the potential risks of probiotics in general and to guide the application of probiotics in clinical application.
Introduction
Faecalibacterium prausnitzii is a gram-negative and strictly anaerobic rod-shaped bacterium that is one of the most abundant commensal bacterial species in the colons of healthy humans (Duncan et al., 2002; Hold et al., 2003). F. prausnitzii was initially classed as a member of the Fusobacterium genus of the Firmicutes phylum. However, guided by phylogenetic analysis based on 16S rRNA sequencing, F. prausnitzii was identified as a new genus and can be classified into two phylogroups and three clusters (Duncan et al., 2002; Lopez-Siles et al., 2012; Benevides et al., 2017; Lopez-Siles et al., 2017). The importance of this commensal bacterium as a component of the healthy human microbiota has been highlighted by an increasing number of studies (Passera et al., 2016; Martín et al., 2017).
F. prausnitzii is well known as one of the most abundant butyrate-producing bacteria in the gastrointestinal tract (Flint et al., 2014). Butyrate plays a major role in gut physiology, and it has pleiotropic effects in the intestinal cell life cycle and numerous beneficial effects for health through protection against pathogen invasion, modulation of the immune system, and reduction of cancer progression (Macfarlane and Macfarlane, 2011). The metabolic activity of F. prausnitzii is not restricted to the production of butyrate; it can also consume acetate and produce carbon dioxide, formate, and D-lactate. Additionally, F. prausnitzii can hydrolyze fructose, oligosaccharides, pectin, starch, and even inulin (Lopez-Siles et al., 2012). The high metabolic activity of F. prausnitzii is beneficial not only for colonocytes but also for host metabolism. F. prausnitzii, as a commensal bacterium, has an anti-inflammatory property that has been identified based on human clinical data (Miquel et al., 2015). This protective mechanism presumably involves the inhibition of proinflammatory cytokines, such as nuclear factor-κB, and has a key role in the stimulation of anti-inflammatory cytokine secretion (such as IL-10) by active molecules (Zhang et al., 2014). Changes in the abundance of F. prausnitzii have been linked to dysbiosis not only in gastrointestinal disorders but also in metabolic syndromes and Alzheimer’s disease (Ueda et al., 2021). Investigations have revealed that the abundance of F. prausnitzii is low in both ulcerative colitis (UC) and Crohn’s disease (CD) patients compared with healthy people (Varela et al., 2013; Radhakrishnan et al., 2022). A reduction in Faecalibacterium spp. in fecal samples has been reported to be associated with an increase in irritable bowel syndrome (IBS) symptoms (Miquel et al., 2016). A decrease in Faecalibacterium spp. has been observed in colorectal cancer patients in comparison with healthy controls (Wu et al., 2013). Unlike the changes in gastrointestinal disorders, there were inconsistent trends in metabolic syndromes. For example, a previous study reported that the relative abundance of F. prausnitzii is increased in obese patients in contrast to healthy individuals (Furet et al., 2010). Lower abundances of Faecalibacterium were reported in patients with type 2 diabetes and non-alcoholic fatty liver disease (Qin et al., 2012; Zhu et al., 2013). There was a higher abundance of proinflammatory bacteria (e.g., Escherichia/Shigella) and a lower distribution of anti-inflammatory bacteria (e.g., Bacillus fragilis, F. prausnitzii) in amyloid-positive Alzheimer’s disease patients than in healthy subjects (Lee et al., 2018). It can be concluded that the population level and functions of F. prausnitzii are associated with host health. However, their causes and consequences are not clearly understood, and much information is lacking regarding which subspecies of F. prausnitzii are important under which conditions.
Given the important role of F. prausnitzii in human health and the fact that there have been no previous reports of its pathogenic characteristics, there is a clear potential for this species as a next-generation probiotic. Despite its importance in human health, only a few microbiological studies have been performed to isolate novel F. prausnitzii strains to better understand the biodiversity and phylogenetic diversity of this beneficial commensal species. With regard to the phylogenetic relationships of F. prausnitzii strains, several previous studies have found that they can be classified into two phylogroups and three clusters according to their 16S rRNA sequences, and the results support their membership of two different genomospecies or genomovars (Duncan et al., 2002; Lopez-Siles et al., 2012; Benevides et al., 2017; Lopez-Siles et al., 2017). Differences in enzyme production, antibiotic resistance, and immunomodulatory properties were found to be strain dependent (Martín et al., 2017). As of 15 July 2021, the genomes of 84 F . prausnitzii strains have been sequenced; six strains have been completely sequenced, and the rest have been mainly assembled at the contig and scaffold levels (Bag et al., 2017). Moreover, the genetic diversity and functional traits of F. prausnitzii, especially the CRISPR/Cas system, which is widely used in editing the genetic elements of microbiota (Horvath and Barrangou, 2010), and the compositions of virulence factors (VFs), antibiotic resistance genes (ARGs), and pathogenic genes (PGs), remain unclear. Therefore, a comprehensive analysis of the characteristics of F. prausnitzii is urgently needed to support to its clinical application.
In this study, we collected the available genomic datasets of 84 F . prausnitzii strains and conducted a comparative analysis to investigate the strains’ genetic diversity and functional traits, including cluster of orthologous group (COG) analysis, VFDB database annotation, CARD database annotation, PHI database annotation, KEGG database annotation, metabolic pathways analysis, and CRISPR/Cas composition analysis. Importantly, we proposed two indices, namely, the probiotic potential risk index (PPRI) and probiotic potential risk score (PPRS), based on the profiles of ARGs, VFs, and PGs, to estimate the potential risks of the clinical application of 84 F . prausnitzii strains, and these indices can be applied to other suitable probiotics.
Material and methods
Collection of genomic datasets of F. prausnitzii
To obtain the available genetic information of F. prausnitzii, we used F. prausnitzii as a keyword and searched the genome/RefSeq databases of NCBI on 15 July 2021. As a result, we collected the genome and protein sequences of 84 F . prausnitzii strains and downloaded them from the RefSeq database of NCBI for comparative genomic analysis. The assembly levels, genome assembly lengths, GC content (%), the numbers of proteins or genes, and the sources of these strains were obtained from NCBI and recorded in Supplementary Table 1.
Identification of protein orthologs of F. prausnitzii
To obtain an in-depth understanding of the pan-genome of F. prausnitzii, all proteins derived from 84 F . prausnitzii strains were used to construct protein families using OrthoMCL (version: 2.0.9) (Li et al., 2003) with BLAST. The E-value threshold was set to 1e-5 and the inflation parameter threshold was set to 1.5 (Zhong et al., 2018). Subsequently, a total of 8,420 homologous clusters were generated. To better understand the functional compositions of F. prausnitzii, these homologous clusters were divided into three categories, namely, core (representing the proteins shared by 84 F . prausnitzii strains), specific (representing the proteins detected only in one F. prausnitzii strain), and accessory groups (the rest of the proteins shared by at least two strains but not by all). Finally, 375 and 303 homologous clusters were classified into core groups and single-copy gene families, respectively. Additionally, detailed information about the reconstructed pan-genome of F. prausnitzii was obtained. Each identified orthogroup protein, including their original F. prausnitzii strain, accession number, protein sequence, and cluster number in the 84 F. prausnitzii strains, can be downloaded from the website: https://github.com/Basspoom/Detailed-information-on-the-reconstructed-pangenome-of-Faecalibacterium-prausnitzii.git.
Construction of phylogenetic trees for F. prausnitzii
To determine the evolutionary relationships of the 84 F . prausnitzii strains, three types of phylogenetic tree were constructed using different strategies. First, 16S rRNA sequences of the 84 F . prausnitzii strains were used to generate a phylogenetic tree with the maximum likelihood method. Then, the protein sequences for 303 single-copy gene families were aligned using MUSCLE (version 3.8.31) (Edgar, 2004) with default parameters, and then these alignment results were concatenated and used as an import sequence document with MEGA-X (version 10.2.5) (Kumar et al., 2018) to construct the phylogenetic tree of 84 F . prausnitzii strains using the neighbor-joining (NJ) method (Saitou and Nei, 1987). Additionally, the Manhattan distance was calculated based on the pan-genome composition of F. prausnitzii, which was used to generate a phylogenetic tree with the unweighted pair group average method (UPGMA).
Functional annotations of F. prausnitzii
To obtain an in-depth understanding of the functional compositions of F. prausnitzii, the proteins of homologous clusters were annotated against the COG and CAZyme databases. First, the representative protein sequences of 8,240 homologous clusters were annotated with the COG database (https://www.ncbi.nlm.nih.gov/COG/) (Galperin et al., 2019) using BLASTP with the following parameters: the E-value threshold was set to 1e-5 and the top annotation result was selected as the best functional trait for each homologous cluster and assigned to one of 25 functional categories (Han et al., 2020). Second, these representative protein sequences were annotated against the CAZyme database (Lombard et al., 2014), downloaded from dbCAN (Huang et al., 2018), using hmmscan of HMMER (version 3.1b1) (Makela et al., 2018) with default settings. The annotated results were summarized as glycosyltransferase (GT), glycoside hydrolase (GH), carbohydrate esterase (CE), carbohydrate-binding module (CBM), auxiliary activity (AA), polysaccharide lyase (PL), and S-layer homology (SLH).
Detection of secondary metabolism gene clusters and pathway construction in F. prausnitzii
KOALA (KEGG Orthology And Links Annotation) (Kanehisa et al., 2017) and AntiSMASH 6.0 (Medema et al., 2011) were used to gain more insight into the metabolic pathways, in particular, the secondary metabolism gene clusters of F. prausnitzii. Specifically, the proteins of each F. prausnitzii strain were annotated with the KEGG database using two online tools, namely, GhostKOALA and KofamKOALA under KOALA, to obtain detailed annotation results (Kanehisa et al., 2016b). Furthermore, EnrichM (version 0.64) (Liu et al., 2021) was used to obtain the KO matrix of F. prausnitzii to construct the metabolic pathways, such as the pathway of the metabolism of fatty acids of F. prausnitzii, which was constructed using the reconstruction function of KEGG Mapper (https://www.genome.jp/kegg/mapper/), a program that provides an in-depth understanding of the synthesis of fatty acids. In addition, AntiSMASH (https://antismash.secondarymetabolites.org/) was used to detect the potential secondary metabolite biosynthesis gene clusters of the 84 F. prausnitzii strains with the following parameters: detection strictness and relaxed to identify well-defined clusters (Blin et al., 2021).
Prediction of the CRISPR/Cas system of F. prausnitzii
To explore the profiles of the CRISPR/Cas system and obtain a better genetic background of F. prausnitzii strains, we investigated the components of the CRISPR/Cas system, including CRISPR arrays and Cas genes, using the online tool CrisprCasFinder (https://crisprcas.i2bc.paris-saclay.fr/) (Couvin et al., 2018). In particular, the size of the flanking region of each analyzed CRISPR array was set to 100, and the remaining parameters were set to default values. Furthermore, 10 strains that contained more sequences than CrisprCasFinder could deal with were analyzed using the CRISRPCasMeta tool with default settings, namely, F. prausnitzii KLE1255, F. prausnitzii 2789STDY5834930, F. prausnitzii CNCM | 4542, F. prausnitzii CNCM | 4546, F. prausnitzii BIOML-B15, F. prausnitzii BIOML-B16, F. prausnitzii BIOML-B6, F. prausnitzii BIOML-B1, F. prausnitzii SSTS Bg7063, and F. prausnitzii JG BgPS064.
Profiles of virulence factors, antibiotic resistance genes, and pathogenic genes of F. prausnitzii
To obtain an in-depth understanding of the genetic background of F. prausnitzii, the composition of virulence factors (VFs), antibiotic resistance genes (ARGs), and pathogenic genes (PGs) of F. prausnitzii were profiled. In brief, the setA database of the pathogenic Virulence Factor Database (VFDB) was downloaded from the official website (http://www.mgc.ac.cn/VFs/) (Chen et al., 2005). Then, the setA database was locally distributed and used for protein annotations of F. prausnitzii with DIAMOND (version: 0.8.36) (Buchfink et al., 2021). The annotation results were screened with a similarity of ≥80% and a coverage of ≥70% to obtain a more accurate annotation of virulence factors (Han et al., 2022). Then, eight kinds of virulence factor were filtered. Second, a famous ARG database, the Comprehensive Antibiotic Resistance Database (CARD) (McArthur et al., 2013), was downloaded (https://card.mcmaster.ca/) and used to detect potential ARGs from 8,240 homologous protein clusters with the default parameters; then, the results were filtered using a similarity of ≥80% and a coverage of ≥70% (Han et al., 2022). Third, to obtain the pathogenicity of F. prausnitzii, the Pathogen Host Interactions database (PHI-base, http://www.phi-base.org/) (Urban et al., 2020), a web-accessible database that focuses on pathogenicity, virulence, and effector genes from different pathogens, was applied to identify potential pathogenic genes. The results were filtered using the following parameters: similarity of ≥80% and coverage of ≥70%.
Estimation of the potential risks of F. prausnitzii strains
Previous studies have demonstrated that the beneficial human gut microbe F. prausnitzii is a candidate ‘probiotic of the future’ or ‘next-generation probiotic’ because it can produce high amounts of butyrate and several anti-inflammatory compounds (Khan et al., 2014; He et al., 2021; Maioli et al., 2021). In recent years, increasing applications of probiotics for the clinical treatment of diseases have been reported. Nevertheless, dangerous problems caused by probiotics occasionally occur, such as the adverse effects of the probiotic Lactobacilli precautions (Castro-Gonzalez et al., 2019) in recent case reports, including bacteremia and/or sepsis (Passera et al., 2016), abscesses (Pararajasingam and Uwagwu, 2017), and endocarditis (Boumis et al., 2018), suggesting that the safety of probiotics should be considered. However, the safety issues of probiotics have not been directly addressed, and the potential risk of probiotics has not been evaluated. Hence, to evaluate the potential risk of F. prausnitzii strains, we proposed two indices, namely, the PPRI and PPRS, based on the composition of ARGs, VFs, and PGs, which can provide guidance for choosing probiotics for the clinical treatment of diseases. PPRI was designed as a one-dimensional combination vector with three variables with the following equation:
where NARGs represents the number of ARGs of a strain, NVFs represents the number of VFs of a strain, and NPGs represents the number of PGs of a strain. To intuitively assess the potential risk of probiotic strains, including F. prausnitzii strains, we designed a PPRS that calculated the Euclidean distance of F. prausnitzii strains based on the PPRI with the following equation:
Results and discussion
Pan-genome construction and analysis
In the present study, to conduct a comparative analysis of F. prausnitzii, 84 available genome sequences, including complete and draft genomes, as well as their corresponding protein sequences for F. prausnitzii strains, were collected and downloaded from NCBI. The detailed information of these 84 F . prausnitzii strains is summarized in Supplementary Table 1, including the levels of assembly, the sizes of the genomes, the counts of genes and proteins, and their separation sources. With regard to the sources of these 84 F . prausnitzii strains, we found that a majority of strains were isolated from the feces of humans, such as F. prausnitzii KLE1255, F. prausnitzii APC918/95b, and F. prausnitzii A2165, which was consistent with the finding that F. prausnitzii is dominant in the intestinal tract of humans (Miquel et al., 2013). Although the genomes of 84 F . prausnitzii strains have been sequenced, only six strains have been completely sequenced, and the rest of the strains have been mainly assembled at the contig and scaffold levels. The number of scaffolds and contigs ranged from 1 to 1,135, and the sizes of the assembled genomes of F. prausnitzii strains ranged from 2.66 to 3.42 Mb. The GC content (%) ranged from 52.9% to 58.1%, and the numbers of proteins ranged from 1,991 to 3,264 (Supplementary Table 1).
To characterize the differences in genomic features among F. prausnitzii strains, we generated protein orthologs based on 194,877 high-quality proteins from 84 F . prausnitzii strains, and a total of 8,240 homologous clusters were identified. In addition, 375 homologous clusters were detected in 84 F . prausnitzii strains (Figure 1A), which were defined as the core genomes. In particular, 303 homologous clusters of core genomes were identified as single-copy core protein families. Additionally, 207 homologous clusters that were detected in only one strain of the 84 F . prausnitzii strains were classified into specific genes (unique genes), and the number of specific genes ranged from 1 to 149 in these 84 F . prausnitzii strains. Specifically, the number of specific genes in most F. prausnitzii strains was between 1 and 12, except for F. prausnitzii 2789STDY5834930, which contained 149 unique genes. In addition, the number of accessory families of F. prausnitzii strains ranged from 739 to 2,609 (Supplementary Table 2).
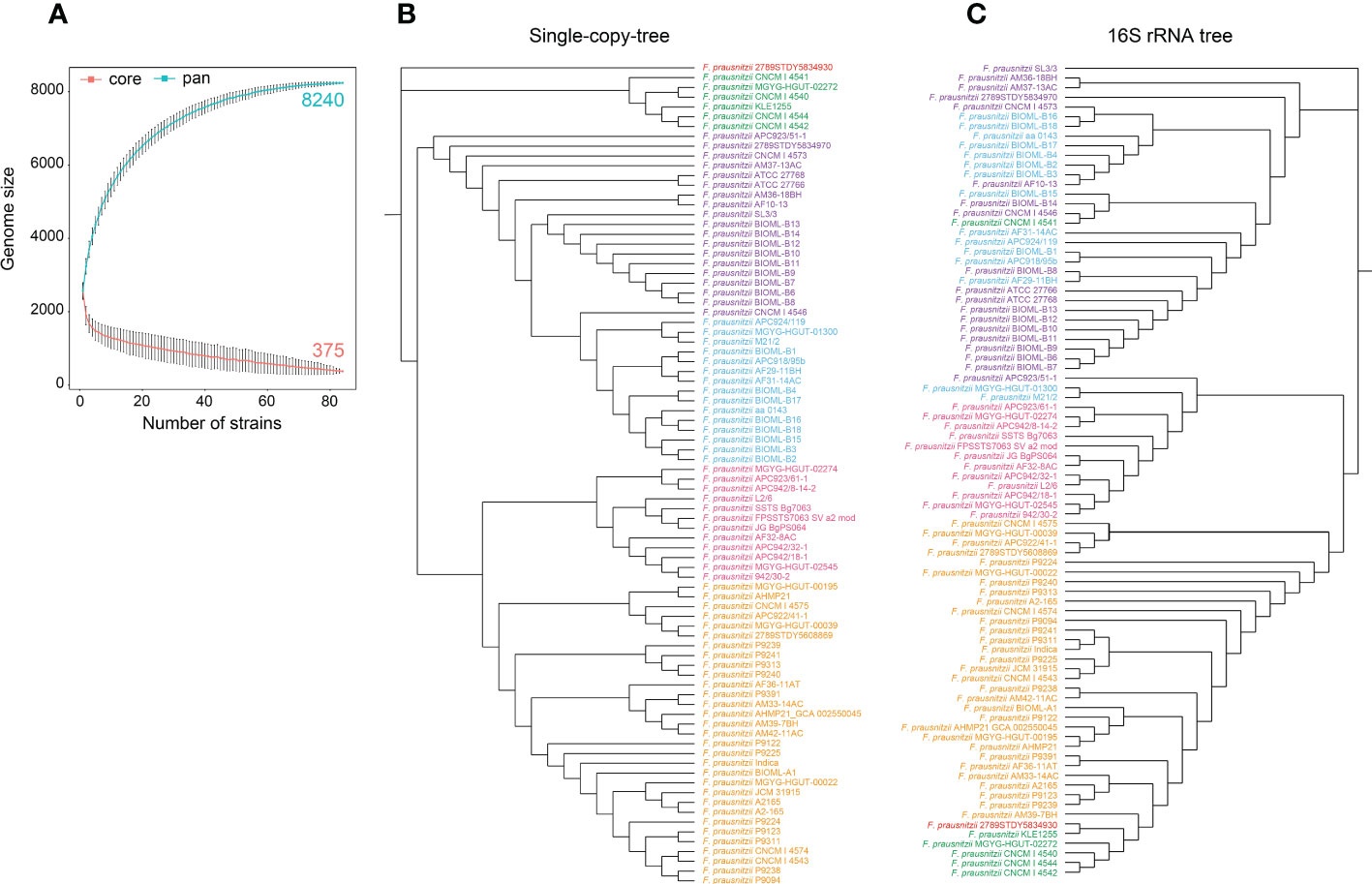
Figure 1 Pan-genome structure and phylogenetic analysis of F. prausnitzii. (A) Pan-genome structure of F. prausnitzii, showing increases and decreases in gene families in the core genome (blue) and pan-genome (red). The core geome of F. prausnitzii showed an open trend compared with its pan-genome. (B) A neighbor-joining phylogenetic tree was constructed based on the protein sequences for 303 single-copy gene families of these 84 F. prausnitzii strains, and they could be divided into six groups according to their evolutionary relationships. (C) A maximum likelihood phylogenetic tree was constructed using the 16S rRNA sequences of the 84 F. prausnitzii strains. Strains of the same name were marked with the same color in (B, C).
The core genome of F. prausnitzii showed an open trend compared with its pan-genome (Figure 1A). With the increase in the number of strains, the genome size of the core genes gradually decreased; finally, 375 core genes were identified. In addition, we found that only 4.5% of the pan-genome remained unchanged, while the remaining 95.5% was variable between strains, indicating that the pan-genome showed a high degree of genomic variability (Figure 1A). These results revealed that the genetic elements and functional traits of 84 F . prausnitzii strains were varied and contributed to the adaptation of F. prausnitzii strains to the intestinal niche.
Phylogenetic analysis of F. prausnitzii strains
To compare the similarity and genetic distance of the 84 F. prausnitzii strains, three types of phylogenetic trees were constructed based on the protein sequences for 303 single-copy gene families, their 16S rRNA sequences (Figures 1B, C), and the pan-genome of the 84 F . prausnitzii strains. Specifically, the protein sequences for 303 single-copy gene families were aligned, and then these alignment results were concatenated and used to construct the phylogenetic tree of 84 F . prausnitzii strains using the NJ method (Figure 1B). In addition, based on the sequences of 16S rRNA, we used MEGA-X to construct a phylogenetic tree using the maximum likelihood method (Figure 1C). As shown in Figures 1B, C, we found that these 84 F. prausnitzii strains could be divided into six groups according to their evolutionary relationships based on the two different construction strategies. Importantly, we compared these two phylogenetic trees with those of F. prausnitzii from studies by Fitzgerald et al. (2018) and Benevides et al. (2017). Fitzgerald et al. (2018) constructed a maximum likelihood phylogenetic tree of the family Ruminococcaceae based on concatenated alignments of 245 highly conserved proteins. In their study, F. prausnitzii could be divided into three groups, namely, I, IIa, and IIb. Compared with our single-copy tree (Figure 1B), group I corresponds to our blue and purple groups, group IIa corresponds to our green group, and group IIb corresponds to our pink and yellow groups. Benevides et al. (2017) also grouped Faecalibacterium into three different groups using 16S rRNA sequence clustering. As more F. prausnitzii strains were isolated and sequenced, more genomes were used in the present study and more detailed classification results were obtained. These results suggest that the evolutionary relationships of F. prausnitzii strains are complex, indicating the need for a pan-genomic analysis of F. prausnitzii.
Meanwhile, there were great discrepancies in the composition of the phylogenetic trees constructed using the two different strategies (Figures 1B, C). With regard to F. prausnitzii 2789STDY5834930, we found that it was separately grouped in the single-copy tree, revealing that it has the longest evolutionary time and may be the oldest species in the genus Faecalibacterium. Additionally, based on the composition of the pan-genome of the 84 F. prausnitzii strains, the UPGMA method was used to construct a phylogenetic tree to reveal the relationships between the F. prausnitzii strains (Supplementary Figure 1). The UPGMA tree was also significantly different from the phylogenetic trees constructed using the previous two strategies.
Overall, these results showed that the genetic elements and the evolutionary relationships of the F. prausnitzii strains were varied and complex, suggesting that a pan-genome analysis is essential to obtain an in-depth understanding of F. prausnitzii that benefits its applications as a new probiotic. Hence, we evaluated the functional compositions of F. prausnitzii strains from different perspectives, including COG annotations, CAZyme, metabolic pathways, secondary metabolism (in particular, fatty acid metabolism), the composition of CRISPR/Cas systems, and the composition of ARGs, VFs, and PGs, and constructed two indices, namely, the PPRI and PPRS, to evaluate the potential risk of F. prausnitzii strains and provide guidance for their application.
COG annotations for F. prausnitzii strains
To gain insight into the functional characteristics of F. prausnitzii strains, we annotated the 8,420 homologous clusters against the COG database (Galperin et al., 2019) (Figure 2A) and reorganized the functional traits based on the groups of accessory, core, and specific clusters (Figure 2B). As shown in Figure 2A, we found that several homologous clusters were annotated as the categories ‘only general function prediction’ (13.1%) and ‘function unknown’ (7.5%), which suggested that the functional traits of F. prausnitzii strains are unknown.
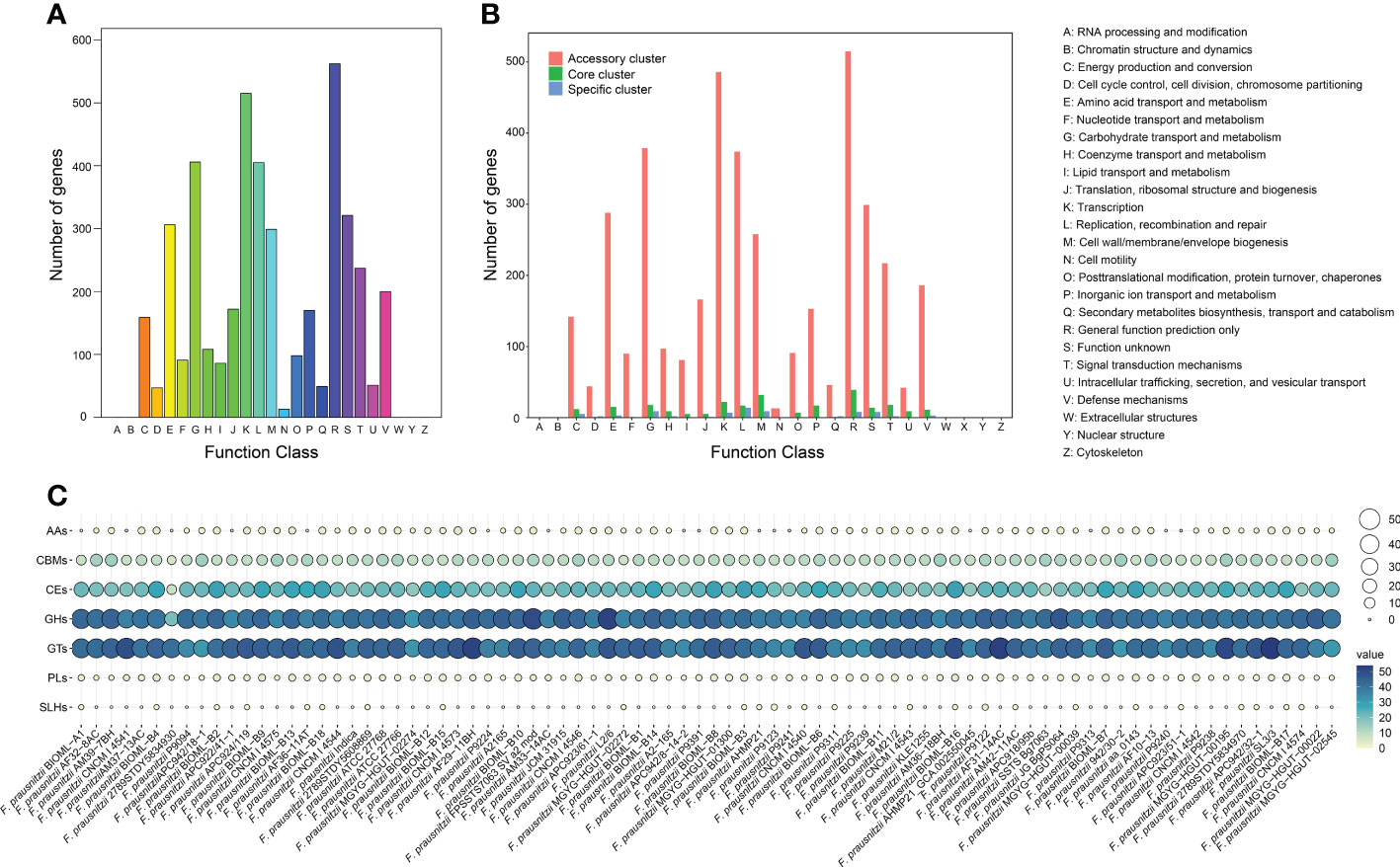
Figure 2 Pan-genome function of F. prausnitzii. (A) COG function classification of expressed contig sequences. (B) Distribution of COG categories in F. prausnitzii accessory, core, and unique genomes. The numbers of genes assigned by COG categories in the accessory cluster (red bars), core cluster (green bars), and unique cluster (blue bars) are shown. The functions of these genes are summarized on the right. (C) Distribution of CAZymes of pan-genome in 84 F. prausnitzii strains. The CAZymes classified from the pan-genome clusters were mainly divided into carbohydrate esterase (CE), glycoside hydrolase (GH), and glycosyltransferase (GT).
Subsequently, the annotations were divided into three groups according to their affiliation. Their numbers and proportions are presented in detail in Supplementary Table 3. We found that the functional traits of the core cluster contain diverse functions and play a basic role in the maintenance of primary cellular processes, including ‘DNA replication and transcription’, ‘cell signal transduction’, and ‘information storage and handling’ (Figure 2B). The functional annotations of unique genes were mainly annotated to ‘energy production and conversion’, ‘amino acid transport and metabolism’, ‘transcription’, and other similar categories (Figure 2B). We found that a proportion of unique genes (1.7%) were annotated. By contrast to core and unique clusters, a high proportion of the proteins (92.4%) classified into accessory clusters could be assigned to ‘transcription’, ‘replication, recombination, and repair’, ‘cell wall/membrane/envelope biogenesis’, ‘signal transduction mechanisms’, and the metabolism of biological macromolecules (Figure 2B). Moreover, the distributions of these accessory proteins in the 84 F. prausnitzii strains was varied. For example, the category ‘replication, recombination, and repair’ contained genes involved in mobile elements (transposase, recombinase, and integrase genes), indicating the presence of potential horizontal gene transfer events (Soucy et al., 2015). Overall, these results suggest that the composition of the accessory cluster contributes to the diversity of functional traits of different F. prausnitzii strains.
Identification of CAZymes for F. prausnitzii strains
To obtain a comprehensive understanding of the catalytic abilities of carbohydrates and carbohydrate metabolism in F. prausnitzii, we annotated the 8,240 homologous clusters of the 84 F . prausnitzii strains against the CAZyme database and explored the distribution pattern of carbohydrate metabolism in these strains (Figure 2C; Supplementary Tables 4 and 5). We found that the functional traits associated with carbohydrate metabolism in the F. prausnitzii strains were mainly annotated as carbohydrate esterase (CE), glycoside hydrolase (GH), and glycosyltransferase (GT, Figure 2C). Specifically, in the present study, sixteen kinds of GTs were detected in the 84 F . prausnitzii strains (Supplementary Figure 2; Supplementary Table 5). In particular, GT2 and GT4 were predominant in F. prausnitzii (Supplementary Figure 2). In general, the primary function of GTs is associated with glycoside synthesis, and their substrates are diverse (Lairson et al., 2008). According to the description of GT2 and GT4 in a previous study, the GT2 and GT4 families mainly contain several glycosyltransferases, such as chitin synthase, cellulose synthase, and α-glucose transferase (Bohra et al., 2019). Additionally, a report by Martinez-Fleites et al. (2006) pointed out that WaaG in the GT4 family catalyzes a key step in lipopolysaccharide synthesis. Therefore, given the high proportions of GT2 and GT4 in the F. prausnitzii strains, we speculated that these strains have the potential metabolic ability of chitin, cellulose, and lipopolysaccharide.
Additionally, 10 kinds of CEs were detected in 84 F. prausnitzii strains (Supplementary Figure 2, Supplementary Table 5). CE1 and CE10 were present in slightly higher numbers in F. prausnitzii. The function of CEs is to participate in the metabolism of carbohydrate esters (Armendariz-Ruiz et al., 2018). Genes putatively coding for the CAZyme families of carbohydrate esterases CE1 and CE10 have been suggested to have lifestyle-specific gene expression patterns (de Vries and de Vries, 2020).
Moreover, 34 kinds of GHs were detected in 84 F. prausnitzii strains (Supplementary Figure 2, Supplementary Table 5). The content of each GH in F. prausnitzii was similar and low. The function of GHs is to carry out the hydrolysis reaction of glycosides (Janecek and Svensson, 2016).
Construction of potential metabolic pathways for F. prausnitzii strains
To obtain an in-depth understanding of the potential metabolic pathways of F. prausnitzii, we profiled the functional traits of F. prausnitzii strains with different strategies, including using the annotations obtained from KEGG databases (Kanehisa et al., 2016a) and secondary metabolic gene clusters predicted by AntiSMASH (Medema et al., 2011), as well as constructing the fatty acid metabolism pathway. First, we applied two tools, namely, GhostKOALA and KofamKOALA, to annotate the proteins of the 84 strains against the KEGG database (Kanehisa et al., 2016a; Kanehisa et al., 2017). Based on the annotations of these two tools for the 84 F . prausnitzii strains, the results are summarized in Supplementary Tables 5, 6. Specifically, the annotation results showed that the proteins of the homologous clusters of F. prausnitzii were mainly involved in ‘metabolic process’ (77%), ‘genetic information processing’ (7.6%), ‘environmental information processing’ (7.3%), ‘cellular processes’ (4.0%), ‘organismal systems’ (1.3%), and ‘human diseases’ (2.8%, Figures 3A, B). Furthermore, among 8,240 homologous clusters of 84 F . prausnitzii strains, a total of 2,420 homologous clusters (29.4%) were annotated. These functional categories can be divided into ‘protein families: signaling and cellular process’, ‘protein families: genetic information process’, ‘environmental information processing’, and so on (Figure 3B). The details of these functional categories are provided in Supplementary Table 7.
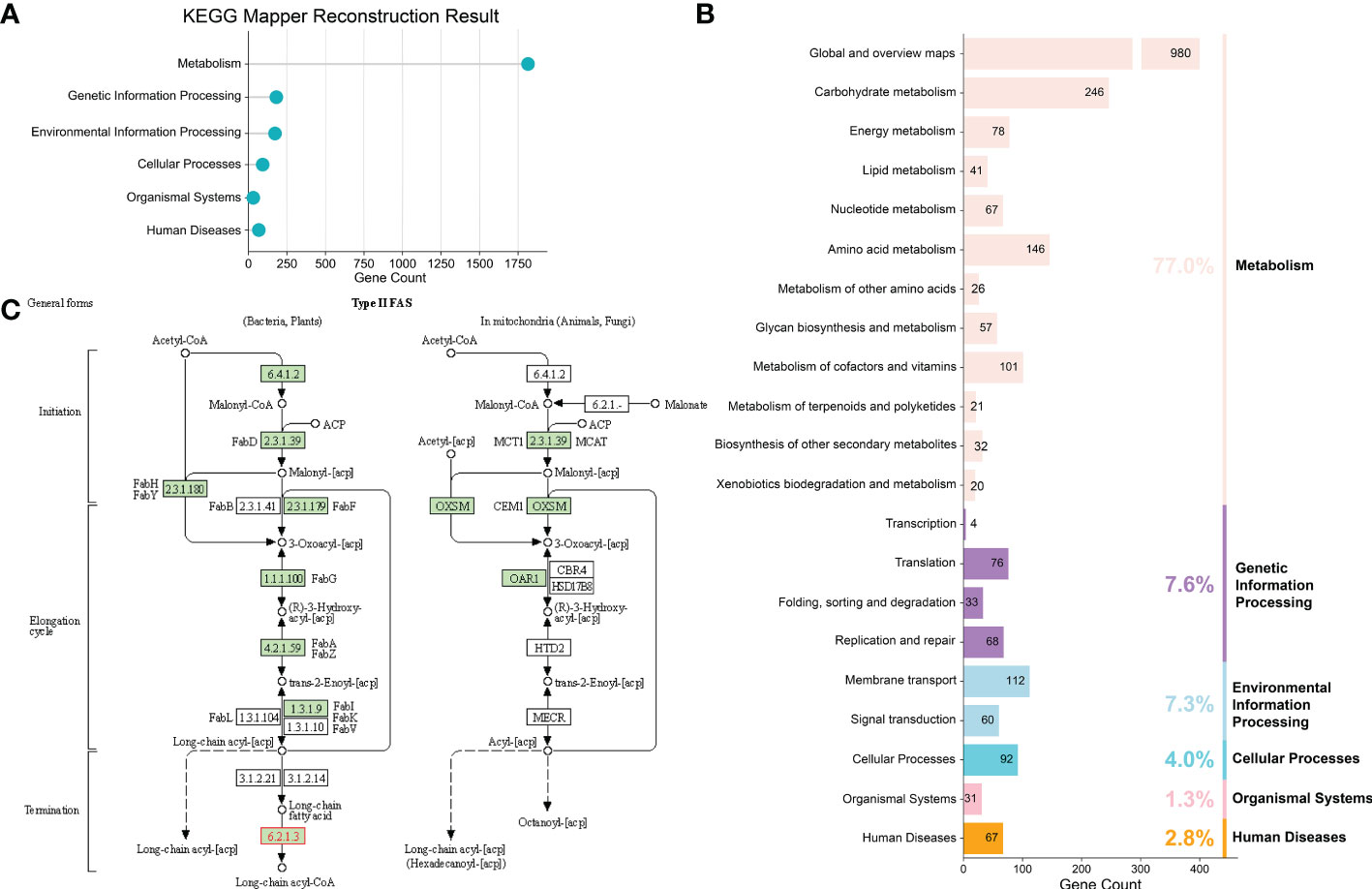
Figure 3 Construction of potential metabolic pathways for F. prausnitzii strains. (A) KEGG reconstruction of F. prausnitzii. The annotation results showed that the proteins of the homologous clusters of F. prausnitzii were mainly involved in ‘metabolic process’, ‘genetic information processing’, ‘environmental information processing’, ‘cellular processes’, ‘organismal systems’, and ‘human diseases’. (B) Detailed functional categories of KEGG reconstruction. (C) Fatty acid metabolism pathway of F. prausnitzii constructed via KEGG Mapper – Reconstruct Pathway. F. prausnitzii relies on the enzymes marked in green for fatty acid metabolism.
Second, we used AntiSMASH (Medema et al., 2011) to detect the secondary metabolic gene clusters (referred to as biosynthesis gene clusters, BGCs) of F. prausnitzii strains (Supplementary Table 8). Seven categories of BGCs were detected from 84 F . prausnitzii strains (ranthipeptide, cyclic-lactone-autoinducer, RiPP-like, lantipeptide class I, lassopeptide, RRE-containing, and lantipeptide class II) (Figure 4). In particular, we found that a majority of F. prausnitzii strains (85.7%) harbor the ranthipeptide and cyclic-lactone-autoinducer gene clusters, but only a few strains (39.2%) contain RiPP-like, lantipeptide class I, lassopeptide, RRE-containing, and lantipeptide class II gene clusters (Figure 4).
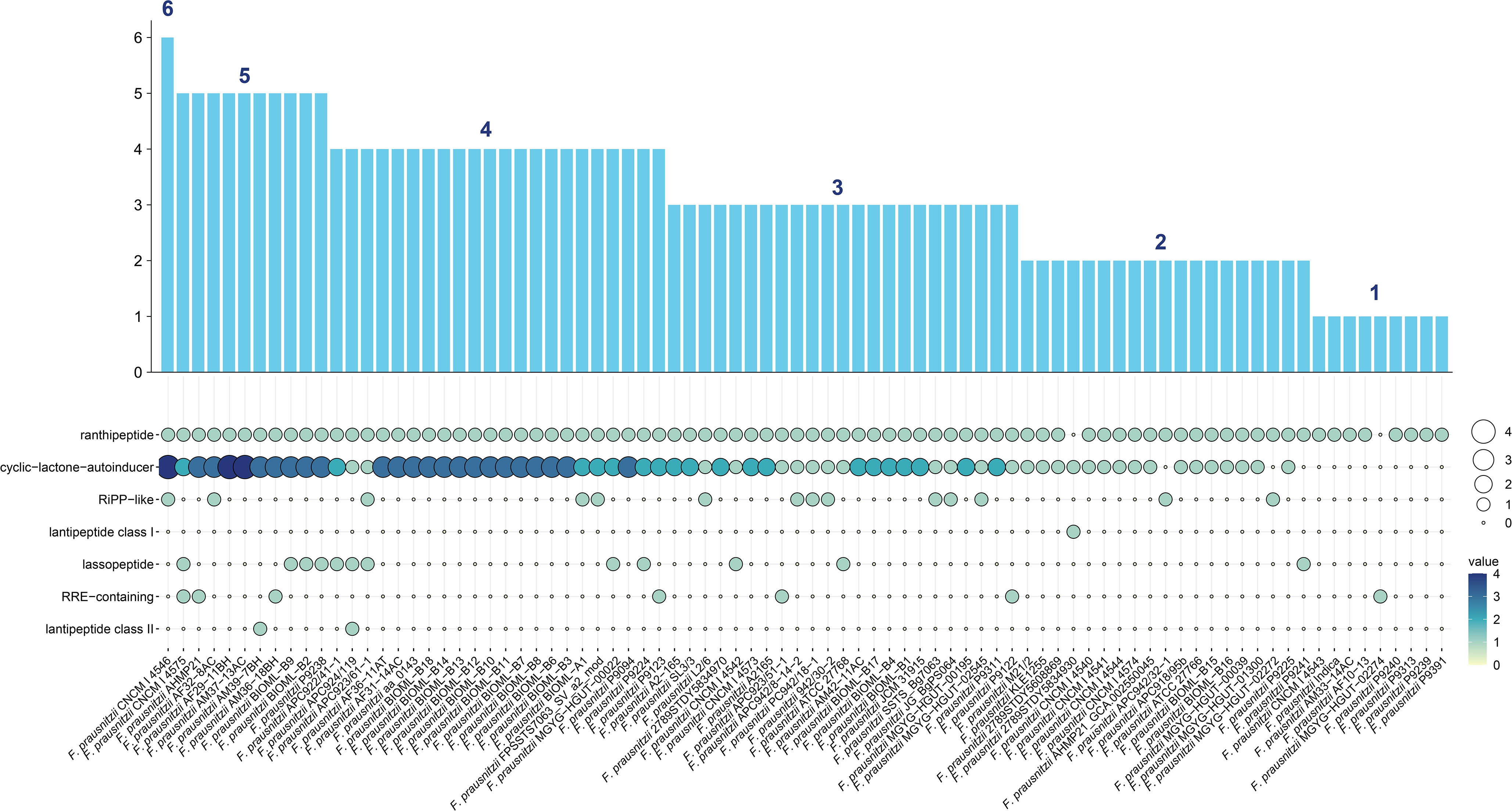
Figure 4 BGC carrying status of F. prausnitzii. Seven categories of secondary metabolism gene clusters (referred to as biosynthesis gene clusters, BGCs) from 84 F. prausnitzii strains were discovered: ranthipeptide, cyclic-lactone-autoinducer, RiPP-like, lantipeptide class I, lassopeptide, RRE-containing, and lantipeptide class II. The ordinate of the bar graph is the sum of the number of BGCs carried by each strain. Strains were arranged from high to low by the quantity of BGCs they carried.
Third, several previous studies have reported that the F. prausnitzii strain is a potential probiotic because it can produce short-chain fatty acids (Quevrain et al., 2016; Lenoir et al., 2020). Therefore, in the present study, we conducted annotations of all the proteins of F. prausnitzii strains and paid more attention to the metabolic pathways of fatty acids. The pathway of fatty acid metabolism was constructed to provide more valuable information about the beneficial functions of F. prausnitzii strains. EnrichM (Liu et al., 2021) was used to classify and obtain the KO IDs of the F. prausnitzii strains. These KO IDs were then used to construct the fatty acid metabolism pathway via KEGG Mapper – Reconstruct Pathway (Kanehisa et al., 2017) (Figure 3C). As shown in Figure 3C; Supplementary Figure 3, and Supplementary Table 9, the complete metabolic pathway of F. prausnitzii strains consists of different enzymes that transform different substrates. The initial substrate of this process is acetyl-CoA, which undergoes the addition of a molecule of malonyl-[acp] and sequentially reduces, oxidizes, and reduces to eliminate carbonyl, thus introducing two carbon atoms to synthesize butanoyl-[acp] containing four carbon atoms. The introduction of two carbon atoms occurs every time these four steps of the reaction are carried out until stearoyl-CoA/ACP with 18 carbon atoms is synthesized (Kindt et al., 2018).
All enzymes involved in fatty acid metabolism and their expression levels in each strain of F. prausnitzii are summarized in Supplementary Tables 9, 10. K00208 (FabI), K01961 (accC), and K16363 (IpxC-FabZ) were not used to construct the pathway because they were only found in F. prausnitzii BIOML-B16 and F. prausnitzii 2789STDY5834930 (Supplementary Table 10). Other enzymes with the same functions as these three enzymes can be found in other F. prausnitzii strains. For instance, K00208 (FabI) and K02371 (FabK) are both enoyl-[acyl-carrier protein] reductases. Therefore, the pathway constructed after discarding these three enzymes can more reasonably show the fatty acid metabolism of F. prausnitzii.
Several previous studies have demonstrated the great potential of F. prausnitzii strains for treating intestinal diseases due to the ability of their fatty acid metabolism (Quevrain et al., 2016; Lenoir et al., 2020). Overall, these results provide an in-depth understanding of F. prausnitzii strains and suggest diverse functions, in particular with regard to their abundant BGCs and their fatty acid metabolic pathway, which strongly prove their potential in the treatment of diseases related to gut microbiota.
CRISPR/Cas system components in F. prausnitzii strains
To obtain an in-depth understanding of the genome and efficiently edit the genetic elements of F. prausnitzii strains, we detected the components of the CRISPR/Cas systems, including the Cas proteins and the CRISPR arrays, for all 84 F . prausnitzii strains using the online tool CRISPRCasFinder (the results are summarized in Supplementary Table 11). Specifically, four types of Cas proteins with clear classifications, including CAS-Type IE, CAS-Type IC, CAS-Type IIIA, and CAS-Type ID, and one predicted Cas protein (CAS_putative), were identified in most F. prausnitzii strains, except F. prausnitzii A2-165, F. prausnitzii CNCM | 4540, F. prausnitzii A2165, F. prausnitzii APC942/8-14-2, F. prausnitzii AM36-18BH, F. prausnitzii JCM 31915, F. prausnitzii MGYG-HGUT-00022, F. prausnitzii MGYG-HGUT-02272, and F. prausnitzii 2789STDY5834930 (Supplementary Table 11). After checking the sizes and assembly levels of the genomes for these strains, we speculated that the presence of incomplete genomes affects the identification results. Of these five kinds of Cas proteins, CAS_putative was identified in most F. prausnitzii strains, while most F. prausnitzii strains (52.3%) contained CAS-Type IE, and only four strains contained CAS-Type ID. In addition, the number of Cas proteins in the 84 F . prausnitzii strains ranged from one to seven (Supplementary Table 11). The distribution of Cas proteins in the 84 F . prausnitzii strains may be associated with extensive horizontal gene transfer and rapid evolution (Soucy et al., 2015), suggesting a large variation of the CRISPR/Cas system among the strains.
In addition, CRISPR arrays of the 84 F . prausnitzii strains were also identified, which can indicate their ability to accept foreign DNA. Our results showed that the number of CRISPR arrays in F. prausnitzii varied. For example, F. prausnitzii FPSSTS7063_SV_a2_mod has the largest number of CRISPR arrays (nine). By contrast, fifteen strains, namely, F. prausnitzii APC923/51-1, F. prausnitzii AM37-13AC, F. prausnitzii AM36-18BH, F. prausnitzii 2789STDY5834930, F. prausnitzii CNCM | 4546, F. prausnitzii BIOML-B7, F. prausnitzii BIOML-B8, F. prausnitzii BIOML-B9, F. prausnitzii BIOML-B10, F. prausnitzii BIOML-B11, F. prausnitzii BIOML-B12, F. prausnitzii BIOML-B13, F. prausnitzii BIOML-B14, F. prausnitzii BIOML-B15, and F. prausnitzii BIOML-B16 have only one CRISPR array (Supplementary Table 11). These results provide a more in-depth understanding of the genetic elements of F. prausnitzii and its potential clinical applications.
Evaluation of the potential risk of F. prausnitzii strains based on the profiles of ARGs, VFs, and PGs
In recent years, the safety problems and adverse effects caused by using probiotics have been considered (Marissen et al., 2019; Rittiphairoj et al., 2019). Moreover, previous studies have pointed out that F. prausnitzii can be used for microbiological therapies to treat several intestinal diseases, such as IBS and CD (Quevrain et al., 2016). Therefore, when considering F. prausnitzii as a potential next-generation probiotic, it is essential to determine the potential risk of these F. prausnitzii strains to guide the selection of high-security strains. To solve this issue, we profiled the composition of ARGs, VFs, and PGs. Specifically, eight types of ARGs were identified in F. prausnitzii strains, namely, aadE, ermC, tet40, tetW, catD, tetA, ermB, and aph (3’)-I (Figure 5; Supplementary Tables 12, 13), suggesting that the F. prausnitzii strains possess resistance to aminoglycosides, macrolide-lincosamide-streptogramin, tetracycline, and chloramphenicol. Similarly, eight types of VFs were detected in the F. prausnitzii strains, ranging from one to six per strain (Figure 5; Supplementary Tables 14, 15). Eight types of VFs were detected in F. prausnitzii strains, namely, putative galactosyltransferase, polysaccharide biosynthesis protein_CpsF, capsular polysaccharide biosynthesis protein Cps4J, elongation factor Tu, chaperonin GroEL, ATP-dependent Clp protease proteolytic subunit, glucose-1-phosphate thymidylyltransferase, and UDP-N-acetylglucosamine 2-epimerase (Figure 5; Supplementary Table 14). In addition, 12 types of PGs were identified in F. prausnitzii strains: TufA, Cps2J, ClpP, ClpX, PstB, RmlA, RecA, OadB, Cps2E, ArgG, MsaB, and HpHSP60 (Figure 5; Supplementary Tables 16, 17). The distributions of ARGs, VFs, and PGs in the 84 F . prausnitzii strains were diverse, which revealed that the potential risks of F. prausnitzii strains are varied and that these factors can be used to reflect the risk of each strain.
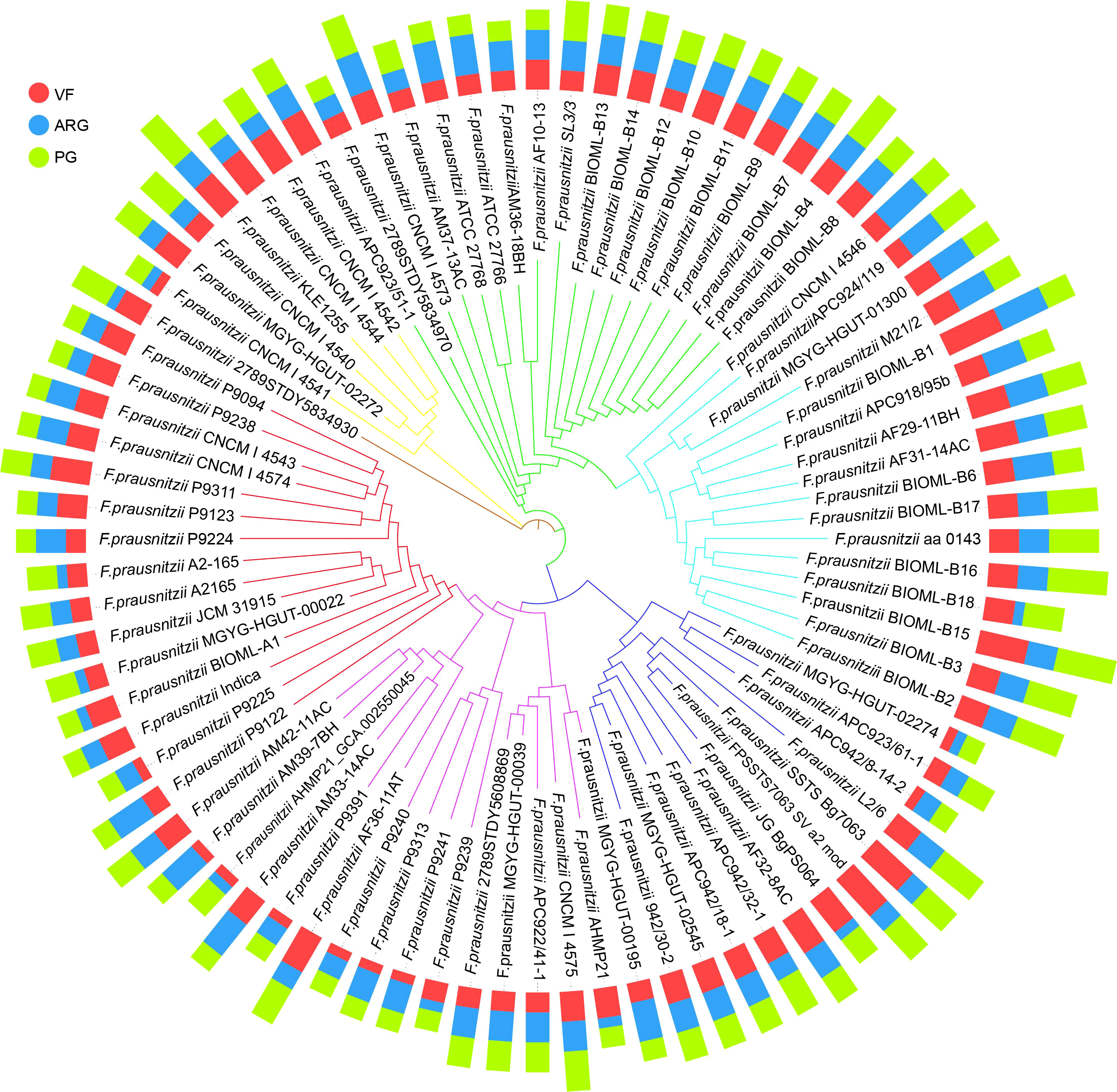
Figure 5 The profiles of VFs, ARGs, and PGs in 84 F. prausnitzii strains. Based on the sequences of 303 single-copy proteins, we constructed the phylogenetic tree (the inner circle) using the neighbor joining method. The outer circle is the bar graph of the three risk factors VF (red), ARG (blue), and PG (green). The types of risk factors are shown in Supplementary Tables 12, 14, and 16. The specific number of each risk factor carried by each strain is shown in Supplementary Tables 13, 15, and 17.
Therefore, based on the composition of ARGs, VFs, and PGs, we proposed two indices, namely, the PPRI and the PPRS. To show the composition of risk factors, PPRI was designed as a one-dimensional combination vector with three variables. To make PPRI more intuitive, we designed a PPRS that calculated the Euclidean distance of F. prausnitzii strains based on their PPRI. The PPRI and PPRS of each F. prausnitzii strain are summarized in Supplementary Table 18 and visualized in Figure 6. As shown in Figure 6A, the three coordinate axes represent the PPRI of the three indicators, ARGs, VFs, and PGs, for each F. prausnitzii strain. Subsequently, we calculated the PPRS of each F. prausnitzii strain (visualized in Figure 6B). The PPRS values ranged from 2.5 to 8.4 (Supplementary Table 18). Combining these two indices, we classified these 84 F . prausnitzii strains into three groups, namely, low-risk (green, PPRS ≤4), medium-risk (yellow, 4< PPRS<6), and high-risk groups (red, PPRS ≥6).
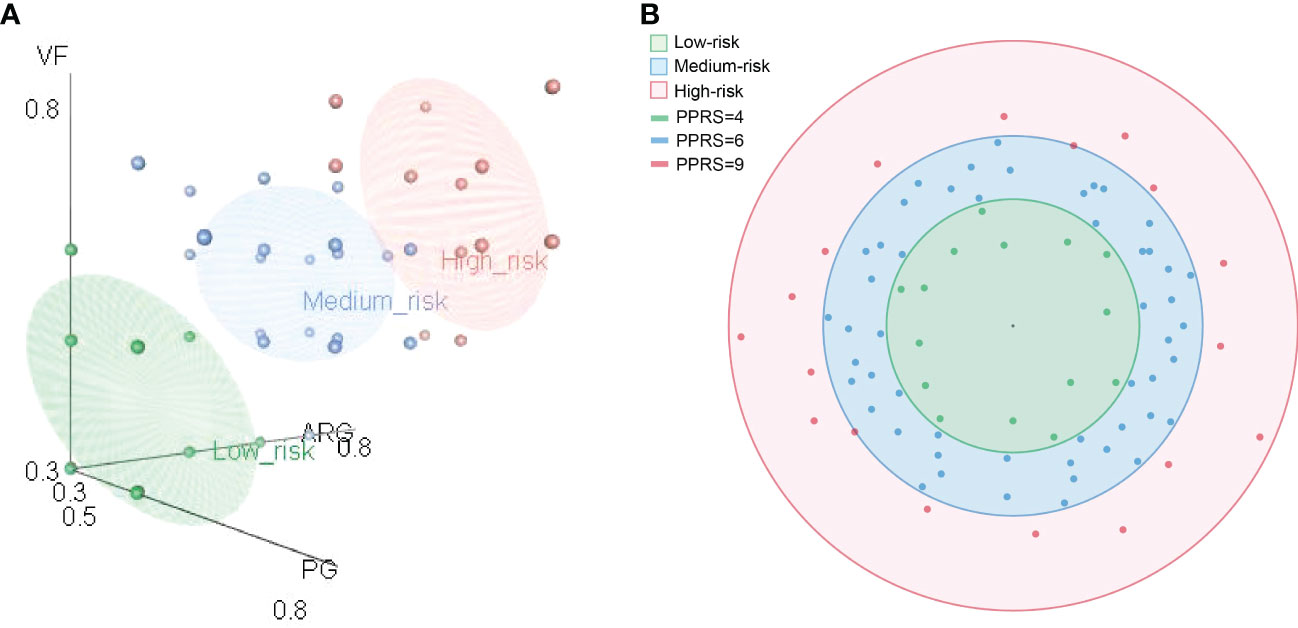
Figure 6 Comprehensive probiotic potential risk model of F. prausnitzii. (A) Eighty-four F. prausnitzii strains were classified as either low risk (green), medium risk (blue), or high risk (red) according to their probiotic potential risk index (PPRI). (B) Eighty-four F. prausnitzii strains were classified as either low risk (green), medium risk (blue), or high risk (red) according their probiotic potential risk score (PPRS).
Finally, F. prausnitzii CNCM | 4541, F. prausnitzii MGYG-HGUT-02274, F. prausnitzii APC942/8-14-2, F. prausnitzii P9391, F. prausnitzii P9225, F. prausnitzii P9239, F. prausnitzii P9240, F. prausnitzii AHMP21_GCA.002550045, F. prausnitzii 2789STDY5834970, F. prausnitzii BIOML-A1, F. prausnitzii A2-165, F. prausnitzii AHMP21, F. prausnitzii P9241, F. prausnitzii P9313, and F. prausnitzii MGYG-HGUT-00022 were classified into the low-risk group (Supplementary Table 18). These results suggest that these 15 bacteria can be selected as potential candidate strains for use in clinical treatment.
These two indices, PPRI and PPRS, were first proposed in the present study. They were designed not only for F. prausnitzii strains but also for other probiotics. These two indices are only indicators for risk assessment based on the genetic features of the strains. It should be noted that three features, including ARGs, VFs, and PGs, were selected for the construction of the PPRI and PPRS. If necessary, more multiple features, such as phenotypic data and wet experimental data, can be used as supplemental features to implement the PPRI and PPRS approaches. Of course, our assessment method can only provide guidance for the selection of probiotics, including F. prausnitzii strains.
Conclusion
In the present study, to obtain a comprehensive understanding of the genetic diversity, functional characteristics, and potential risks of F. prausnitzii, we collected the available genetic information of 84 F . prausnitzii strains to conduct a pan-genome analysis. We generated 8,420 and 375 homologous clusters as the pan-genome and core genome for F. prausnitzii, respectively. Phylogenetic analysis of the 84 strains revealed that although these strains can be divided into six groups, there were great discrepancies in the composition of the phylogenetic trees constructed by three different strategies, which indicated that the compositions of genetic elements of the 84 F . prausnitzii strains were varied. Based on the pan-genome of F. prausnitzii, we profiled the composition of functional traits against the COG, CAZyme, KEGG, ARG, VF, and PHI databases, predicted the BGCs, and constructed the fatty acid metabolism pathway for F. prausnitzii strains. Our results showed that the proteins of the accessory group made a greater contribution to the primary genetic functions of the F. prausnitzii strains than those of the core and specific groups, based on the COG annotations. Only a small number of proteins related to human diseases were identified according to the KEGG annotations, and no secondary metabolic gene clusters encoding harmful products were detected in the F. prausnitzii strains. In particular, the construction of a complete fatty acid metabolism pathway showed the metabolic ability of the synthesis of fatty acids. To obtain an in-depth understanding of the genome and efficiently edit the genetic elements of F. prausnitzii strains, the components of the CRISPR/Cas system were identified. Four types of Cas proteins with a clear classification and one predicted Cas protein were identified in most F. prausnitzii strains, and our results showed that the number of CRISPR arrays in F. prausnitzii varied. To reveal the potential risks of F. prausnitzii strains, we detected harmful elements; eight types of ARGs, eight types of VFs, and 12 types of PGs were detected. To evaluate the potential risks, we first proposed the PPRI and PPRS to classify these 84 strains into low-, medium-, and high-risk groups and identified 15 strains as low-risk strains that should be prioritized for clinical application. Overall, the present study provides a comprehensive understanding of the genetic diversity, functional characteristics, and metabolic pathways of F. prausnitzii. In particular, we propose the PPRI and PPRS for use in evaluating the potential risks of probiotics in general (not just F. prausnitzii) and guiding their clinical treatment. Our results provide insight into the understanding and application of F. prausnitzii as a next-generation probiotic.
Data availability statement
The original contributions presented in the study are included in the article/Supplementary Material. Further inquiries can be directed to the corresponding authors.
Author contributions
GL and MH designed the study. NZ, YJ, FF, and YL collected the data. ZB and MH conducted the pan-genome analysis. ZB, NZ, LC, and MH drew the figures. ZB, MH, and GL wrote the initial draft of the manuscript. All authors read, modified, and approved the final manuscript.
Funding
This work was supported by the National Natural Science Foundation of China (81800465), Grants for Scientific Research of BSKY (XJ201916) from Anhui Medical University, the Natural Science Foundation in Higher Education of Anhui (KJ2021A0244), the Anhui Provincial Natural Science Foundation (2208085QH231), and the Young Foundation of Anhui Medical University (2020xkj015).
Conflict of interest
The authors declare that the research was conducted in the absence of any commercial or financial relationships that could be construed as a potential conflict of interest.
Publisher’s note
All claims expressed in this article are solely those of the authors and do not necessarily represent those of their affiliated organizations, or those of the publisher, the editors and the reviewers. Any product that may be evaluated in this article, or claim that may be made by its manufacturer, is not guaranteed or endorsed by the publisher.
Supplementary material
The Supplementary Material for this article can be found online at: https://www.frontiersin.org/articles/10.3389/fcimb.2022.919701/full#supplementary-material
References
Armendariz-Ruiz, M., Rodriguez-Gonzalez, J. A., Camacho-Ruiz, R. M., Mateos-Diaz, J. C. (2018). Carbohydrate esterases: An overview. Methods Mol. Biol. 1835, 39–68. doi: 10.1007/978-1-4939-8672-9_2
Bag, S., Ghosh, T. S., Das, B. (2017). Complete genome sequence of faecalibacterium prausnitzii isolated from the gut of a healthy Indian adult. Genome Announcements 5 (46), e01286-17. doi: 10.1128/genomeA.01286-17
Benevides, L., Burman, S., Martin, R., Robert, V., Thomas, M., Miquel, S., et al. (2017). New insights into the diversity of the genus faecalibacterium. Front. Microbiol. 8. doi: 10.3389/fmicb.2017.01790
Blin, K., Shaw, S., Kloosterman, A. M., Charlop-Powers, Z., van Wezel, G. P., Medema, M. H., et al. (2021). AntiSMASH 6.0: Improving cluster detection and comparison capabilities. Nucleic Acids Res. 49, W29–W35. doi: 10.1093/nar/gkab335
Bohra, V., Dafale, N. A., Purohit, H. J. (2019). Understanding the alteration in rumen microbiome and CAZymes profile with diet and host through comparative metagenomic approach. Arch. Microbiol. 201, 1385–1397. doi: 10.1007/s00203-019-01706-z
Boumis, E., Capone, A., Galati, V., Venditti, C., Petrosillo, N. (2018). Probiotics and infective endocarditis in patients with hereditary hemorrhagic telangiectasia: A clinical case and a review of the literature. BMC Infect. Dis. 18, 65. doi: 10.1186/s12879-018-2956-5
Buchfink, B., Reuter, K., Drost, H. G. (2021). Sensitive protein alignments at tree-of-life scale using DIAMOND. Nat. Methods 18, 366–368. doi: 10.1038/s41592-021-01101-x
Castro-Gonzalez, J. M., Castro, P., Sandoval, H., Castro-Sandoval, D. (2019). Probiotic lactobacilli precautions. Front. Microbiol. 10. doi: 10.3389/fmicb.2019.00375
Chen, L., Yang, J., Yu, J., Yao, Z., Sun, L., Shen, Y., et al. (2005). VFDB: A reference database for bacterial virulence factors. Nucleic Acids Res. 33, D325–D328. doi: 10.1093/nar/gki008
Couvin, D., Bernheim, A., Toffano-Nioche, C., Touchon, M., Michalik, J., Neron, B., et al. (2018). CRISPRCasFinder, an update of CRISRFinder, includes a portable version, enhanced performance and integrates search for cas proteins. Nucleic Acids Res. 46, W246–W251. doi: 10.1093/nar/gky425
de Vries, S., de Vries, J. (2020). A global survey of carbohydrate esterase families 1 and 10 in oomycetes. Front. Genet. 11. doi: 10.3389/fgene.2020.00756
Duncan, S. H., Hold, G. L., Harmsen, H. J. M., Stewart, C. S., Flint, H. J. (2002). Growth requirements and fermentation products of fusobacterium prausnitzii, and a proposal to reclassify it as faecalibacterium prausnitzii gen. nov., comb. Nov. Int. J. Syst. Evol. Micr. 52, 2141–2146. doi: 10.1099/00207713-52-6-2141
Edgar, R. C. (2004). MUSCLE: Multiple sequence alignment with high accuracy and high throughput. Nucleic Acids Res. 32, 1792–1797. doi: 10.1093/nar/gkh340
Fitzgerald, C. B., Shkoporov, A. N., Sutton, T. D.S., Chaplin, A. V., Velayudhan, V., Ross, R. P., et al. (2018). Comparative analysis of Faecalibacterium prausnitzii genomes shows a high level of genome plasticity and warrants separation into new species-level taxa. BMC Genomics 19, 931. doi: 10.1186/s12864-018-5313-6
Flint, H. J., Scott, K. P., Duncan, S. H., Louis, P., Forano, E. (2014). Microbial degradation of complex carbohydrates in the gut. Gut Microbes 3, 289–306. doi: 10.4161/gmic.19897
Furet, J., Kong, L., Tap, J., Poitou, C., Basdevant, A., Bouillot, J., et al. (2010). Differential adaptation of human gut microbiota to bariatric surgery–induced weight loss. Diabetes 59, 3049–3057. doi: 10.2337/db10-0253
Galperin, M. Y., Kristensen, D. M., Makarova, K. S., Wolf, Y. I., Koonin, E. V. (2019). Microbial genome analysis: The COG approach. Brief. Bioinform. 20, 1063–1070. doi: 10.1093/bib/bbx117
Han, M., Liu, G., Chen, Y., Wang, D., Zhang, Y. (2020). Comparative genomics uncovers the genetic diversity and characters of veillonella atypica and provides insights into its potential applications. Front. Microbiol. 11, 1219. doi: 10.3389/fmicb.2020.01219
Han, M., Zhang, L., Zhang, N., Mao, Y., Peng, Z., Huang, B., et al. (2022). Antibiotic resistome in a large urban-lake drinking water source in middle China: Dissemination mechanisms and risk assessment. J. Hazard. Mater. 424, 127745. doi: 10.1016/j.jhazmat.2021.127745
He, X., Zhao, S., Li, Y. (2021). Faecalibacterium prausnitzii: A next-generation probiotic in gut disease improvement. Can. J. Infect. Dis. Med. Microbiol. 2021, 1–10. doi: 10.1155/2021/6666114
Hold, G. L., Schwiertz, A., Aminov, R. I., Blaut, M., Flint, H. J. (2003). Oligonucleotide probes that detect quantitatively significant groups of butyrate-producing bacteria in human feces. Appl. Environ. Microb. 69, 4320–4324. doi: 10.1128/AEM.69.7.4320-4324.2003
Horvath, P., Barrangou, R. (2010). CRISPR/Cas, the immune system of bacteria and archaea. Science 327, 167–170. doi: 10.1126/science.1179555
Huang, L., Zhang, H., Wu, P., Entwistle, S., Li, X., Yohe, T., et al. (2018). DbCAN-seq: A database of carbohydrate-active enzyme (CAZyme) sequence and annotation. Nucleic Acids Res. 46, D516–D521. doi: 10.1093/nar/gkx894
Janecek, S., Svensson, B. (2016). Amylolytic glycoside hydrolases. Cell. Mol. Life Sci. 73, 2601–2602. doi: 10.1007/s00018-016-2240-z
Kanehisa, M., Furumichi, M., Tanabe, M., Sato, Y., Morishima, K. (2017). KEGG: New perspectives on genomes, pathways, diseases and drugs. Nucleic Acids Res. 45, D353–D361. doi: 10.1093/nar/gkw1092
Kanehisa, M., Sato, Y., Kawashima, M., Furumichi, M., Tanabe, M. (2016a). KEGG as a reference resource for gene and protein annotation. Nucleic Acids Res. 44, D457–D462. doi: 10.1093/nar/gkv1070
Kanehisa, M., Sato, Y., Morishima, K. (2016b). BlastKOALA and GhostKOALA: KEGG tools for functional characterization of genome and metagenome sequences. J. Mol. Biol. 428, 726–731. doi: 10.1016/j.jmb.2015.11.006
Khan, M. T., van Dijl, J. M., Harmsen, H. J. (2014). Antioxidants keep the potentially probiotic but highly oxygen-sensitive human gut bacterium faecalibacterium prausnitzii alive at ambient air. PloS One 9, e96097. doi: 10.1371/journal.pone.0096097
Kindt, A., Liebisch, G., Clavel, T., Haller, D., Hormannsperger, G., Yoon, H., et al. (2018). The gut microbiota promotes hepatic fatty acid desaturation and elongation in mice. Nat. Commun. 9, 3760. doi: 10.1038/s41467-018-05767-4
Kumar, S., Stecher, G., Li, M., Knyaz, C., Tamura, K. (2018). MEGA X: Molecular evolutionary genetics analysis across computing platforms. Mol. Biol. Evol. 35, 1547–1549. doi: 10.1093/molbev/msy096
Lairson, L. L., Henrissat, B., Davies, G. J., Withers, S. G. (2008). Glycosyltransferases: Structures, functions, and mechanisms. Annu. Rev. Biochem. 77, 521–555. doi: 10.1146/annurev.biochem.76.061005.092322
Lee, L., Ser, H., Khan, T. M., Long, M., Chan, K., Goh, B., et al. (2018). IDDF2018-ABS-0239 dissecting the gut and brain: Potential links between gut microbiota in development of alzheimer’s disease? Gut 67, A18. doi: 10.1136/gutjnl-2018-IDDFabstracts.37
Lenoir, M., Martin, R., Torres-Maravilla, E., Chadi, S., Gonzalez-Davila, P., Sokol, H., et al. (2020). Butyrate mediates anti-inflammatory effects of faecalibacterium prausnitzii in intestinal epithelial cells through Dact3. Gut Microbes 12, 1–16. doi: 10.1080/19490976.2020.1826748
Li, L., Stoeckert, C. J., Roos, D. S. (2003). OrthoMCL: Identification of ortholog groups for eukaryotic genomes. Genome Res. 13, 2178–2189. doi: 10.1101/gr.1224503
Liu, L., Wang, Y., Yang, Y., Wang, D., Cheng, S. H., Zheng, C., et al. (2021). Charting the complexity of the activated sludge microbiome through a hybrid sequencing strategy. Microbiome 9, 205. doi: 10.1186/s40168-021-01155-1
Lombard, V., Golaconda, R. H., Drula, E., Coutinho, P. M., Henrissat, B. (2014). The carbohydrate-active enzymes database (CAZy) in 2013. Nucleic Acids Res. 42, D490–D495. doi: 10.1093/nar/gkt1178
Lopez-Siles, M., Duncan, S. H., Garcia-Gil, L. J., Martinez-Medina, M. (2017). Faecalibacterium prausnitzii: From microbiology to diagnostics and prognostics. ISME J. 11, 841–852. doi: 10.1038/ismej.2016.176
Lopez-Siles, M., Khan, T. M., Duncan, S. H., Harmsen, H. J. M., Garcia-Gil, L. J., Flint, H. J. (2012). Cultured representatives of two major phylogroups of human colonic faecalibacterium prausnitzii can utilize pectin, uronic acids, and host-derived substrates for growth. Appl. Environ. Microb. 78, 420–428. doi: 10.1128/AEM.06858-11
Macfarlane, G. T., Macfarlane, S. (2011). Fermentation in the human large intestine. J. Clin. Gastroenterol. 45, S120–S127. doi: 10.1097/MCG.0b013e31822fecfe
Maioli, T. U., Borras-Nogues, E., Torres, L., Barbosa, S. C., Martins, V. D., Langella, P., et al. (2021). Possible benefits of faecalibacterium prausnitzii for obesity-associated gut disorders. Front. Pharmacol. 12. doi: 10.3389/fphar.2021.740636
Makela, M. R., DiFalco, M., McDonnell, E., Nguyen, T., Wiebenga, A., Hilden, K., et al. (2018). Genomic and exoproteomic diversity in plant biomass degradation approaches among aspergilli. Stud. Mycol. 91, 79–99. doi: 10.1016/j.simyco.2018.09.001
Marissen, J., Haiss, A., Meyer, C., Van Rossum, T., Bunte, L. M., Frommhold, D., et al. (2019). Efficacy of bifidobacterium longum, b. infantis and lactobacillus acidophilus probiotics to prevent gut dysbiosis in preterm infants of 28 + 0-32+6 weeks of gestation: A randomised, placebo-controlled, double-blind, multicentre trial: The PRIMAL clinical study protocol. BMJ Open 9, e32617. doi: 10.1136/bmjopen-2019-032617
Martinez-Fleites, C., Proctor, M., Roberts, S., Bolam, D. N., Gilbert, H. J., Davies, G. J. (2006). Insights into the synthesis of lipopolysaccharide and antibiotics through the structures of two retaining glycosyltransferases from family GT4. Chem. Biol. 13, 1143–1152. doi: 10.1016/j.chembiol.2006.09.005
Martín, R., Miquel, S., Benevides, L., Bridonneau, C., Robert, V., Hudault, S., et al. (2017). Functional characterization of novel faecalibacterium prausnitzii strains isolated from healthy volunteers: A step forward in the use of f. prausnitzii as a next-generation probiotic. Front. Microbiol. 8. doi: 10.3389/fmicb.2017.01226
McArthur, A. G., Waglechner, N., Nizam, F., Yan, A., Azad, M. A., Baylay, A. J., et al. (2013). The comprehensive antibiotic resistance database. Antimicrob. Agents Chemother. 57, 3348–3357. doi: 10.1128/AAC.00419-13
Medema, M. H., Blin, K., Cimermancic, P., de Jager, V., Zakrzewski, P., Fischbach, M. A., et al. (2011). AntiSMASH: Rapid identification, annotation and analysis of secondary metabolite biosynthesis gene clusters in bacterial and fungal genome sequences. Nucleic Acids Res. 39, W339–W346. doi: 10.1093/nar/gkr466
Miquel, S., Leclerc, M., Martin, R., Chain, F., Lenoir, M., Raguideau, S., et al. (2015). Identification of metabolic signatures linked to anti-inflammatory effects of faecalibacterium prausnitzii. Mbio 6 (2), e00300-15. doi: 10.1128/mBio.00300-15
Miquel, S., Martín, R., Lashermes, A., Gillet, M., Meleine, M., Gelot, A., et al. (2016). Anti-nociceptive effect of faecalibacterium prausnitzii in non-inflammatory IBS-like models. Sci. Rep-Uk 6, 19399. doi: 10.1038/srep19399
Miquel, S., Martin, R., Rossi, O., Bermudez-Humaran, L. G., Chatel, J. M., Sokol, H., et al. (2013). Faecalibacterium prausnitzii and human intestinal health. Curr. Opin. Microbiol. 16, 255–261. doi: 10.1016/j.mib.2013.06.003
Pararajasingam, A., Uwagwu, J. (2017). Lactobacillus: The not so friendly bacteria. BMJ Case Rep. 2017, bcr2016218423 . doi: 10.1136/bcr-2016-218423. 2016.
Passera, M., Pellicioli, I., Corbellini, S., Corno, M., Vailati, F., Bonanomi, E., et al. (2016). Lactobacillus casei subsp. rhamnosus septicaemia in three patients of the paediatric intensive care unit. J. Hosp. Infect. 94, 361–362. doi: 10.1016/j.jhin.2016.09.018
Qin, J., Li, Y., Cai, Z., Li, S., Zhu, J., Zhang, F., et al. (2012). A metagenome-wide association study of gut microbiota in type 2 diabetes. Nature 490, 55–60. doi: 10.1038/nature11450
Quevrain, E., Maubert, M. A., Michon, C., Chain, F., Marquant, R., Tailhades, J., et al. (2016). Identification of an anti-inflammatory protein from faecalibacterium prausnitzii, a commensal bacterium deficient in crohn’s disease. Gut 65, 415–425. doi: 10.1136/gutjnl-2014-307649
Radhakrishnan, S. T., Alexander, J. L., Mullish, B. H., Gallagher, K. I., Powell, N., Hicks, L. C., et al. (2022). Systematic review: The association between the gut microbiota and medical therapies in inflammatory bowel disease. Aliment. Pharm. Ther. 55, 26–48. doi: 10.1111/apt.16656
Rittiphairoj, T., Pongpirul, K., Mueller, N. T., Li, T. (2019). Probiotics for glycemic control in patients with type 2 diabetes mellitus: Protocol for a systematic review. Syst. Rev. 8, 227. doi: 10.1186/s13643-019-1145-y
Saitou, N., Nei, M. (1987). The neighbor-joining method: A new method for reconstructing phylogenetic trees. Mol. Biol. Evol. 4, 406–425. doi: 10.1093/oxfordjournals.molbev.a040454
Soucy, S. M., Huang, J., Gogarten, J. P. (2015). Horizontal gene transfer: Building the web of life. Nat. Rev. Genet. 16, 472–482. doi: 10.1038/nrg3962
Ueda, A., Shinkai, S., Shiroma, H., Taniguchi, Y., Tsuchida, S., Kariya, T., et al. (2021). Identification of faecalibacterium prausnitzii strains for gut microbiome-based intervention in alzheimer’s-type dementia. Cell Rep. Med. 2, 100398. doi: 10.1016/j.xcrm.2021.100398
Urban, M., Cuzick, A., Seager, J., Wood, V., Rutherford, K., Venkatesh, S. Y., et al. (2020). PHI-base: The pathogen-host interactions database. Nucleic Acids Res. 48, D613–D620. doi: 10.1093/nar/gkz904
Varela, E., Manichanh, C., Gallart, M., Torrejón, A., Borruel, N., Casellas, F., et al. (2013). Colonisation byFaecalibacterium prausnitzii and maintenance of clinical remission in patients with ulcerative colitis. Aliment. Pharm. Ther. 38, 151–161. doi: 10.1111/apt.12365
Wu, N., Yang, X., Zhang, R., Li, J., Xiao, X., Hu, Y., et al. (2013). Dysbiosis signature of fecal microbiota in colorectal cancer patients. Microb. Ecol. 66, 462–470. doi: 10.1007/s00248-013-0245-9
Zhang, M., Qiu, X., Zhang, H., Yang, X., Hong, N., Yang, Y., et al. (2014). Faecalibacterium prausnitzii inhibits interleukin-17 to ameliorate colorectal colitis in rats. PloS One 9, e109146. doi: 10.1371/journal.pone.0109146
Zhong, C., Han, M., Yu, S., Yang, P., Li, H., Ning, K. (2018). Pan-genome analyses of 24 shewanella strains re-emphasize the diversification of their functions yet evolutionary dynamics of metal-reducing pathway. Biotechnol. Biofuels 11, 193. doi: 10.1186/s13068-018-1201-1
Keywords: Faecalibacterium prausnitzii, probiotics, fatty acid metabolism, probiotic potential risk, probiotic potential risk assessment indices
Citation: Bai Z, Zhang N, Jin Y, Chen L, Mao Y, Sun L, Fang F, Liu Y, Han M and Li G (2023) Comprehensive analysis of 84 Faecalibacterium prausnitzii strains uncovers their genetic diversity, functional characteristics, and potential risks. Front. Cell. Infect. Microbiol. 12:919701. doi: 10.3389/fcimb.2022.919701
Received: 13 April 2022; Accepted: 09 December 2022;
Published: 04 January 2023.
Edited by:
Philippe Langella, Institut National de recherche pour l’agriculture, l’alimentation et l’environnement (INRAE), FranceReviewed by:
Thomas Jové, U1092 Anti-Infectieux supports moléculaires des résistances et innovations thérapeutiques (INSERM), FranceHuan Li, Lanzhou University, China
Copyright © 2023 Bai, Zhang, Jin, Chen, Mao, Sun, Fang, Liu, Han and Li. This is an open-access article distributed under the terms of the Creative Commons Attribution License (CC BY). The use, distribution or reproduction in other forums is permitted, provided the original author(s) and the copyright owner(s) are credited and that the original publication in this journal is cited, in accordance with accepted academic practice. No use, distribution or reproduction is permitted which does not comply with these terms.
*Correspondence: Gangping Li, bGlnYW5ncGluZ0BodXN0LmVkdS5jbg==; Maozhen Han, aGFubXpAYWhtdS5lZHUuY24=
†These authors have contributed equally to this work