- 1Science and Education Office, The First Affiliated Hospital, Jinan University, Guangzhou, China
- 2Department of Stomatology, The Sixth Affiliated Hospital of Jinan University, Dongguan, China
- 3School of Stomatology, Jinan University, Guangzhou, China
- 4Medical Center of Stomatology, The First Affiliated Hospital, Jinan University, Guangzhou, China
- 5Chaoshan Hospital, The First Affiliated Hospital of Jinan University, Chaozhou City, China
Aim: There is a bidirectional association between diabetes and periodontitis. However, the effect of diabetes on the periodontitis salivary microbiota has not been elucidated. The aim of this study was to determine the effect of the presence of diabetes on the microbiota among Chinese patients with periodontitis.
Materials and Methods: Unstimulated whole saliva samples were collected from the periodontitis with diabetes group (TC), chronic periodontitis group (CP), and periodontally healthy and systemically healthy group (H) by spitting method. Bacterial genomic DNA was PCR-amplified at the V4 variable region of 16S rRNA gene. The library was constructed according to the obtained sequence results, and biological analysis and statistical analysis were carried out. Functional prediction of three groups of microbial communities was performed by the PICRUSt algorithm.
Results: There was no significant difference in bacterial diversity between the TC and CP groups. Compared with the H group, the TC group and CP group presented a higher diversity of salivary flora. Firmicutes, Streptococcus, Haemophilus, Veillonella, and Haemophilus parainfluenzae dominated the H group. Corynebacterium, Leptotrichia, Dialister, Comamonas, Capnocytophaga, Catonella, Filifactor, Campylobacter, Treponema, Campylobacter concisus, Prevotella oralis, and Porphyromonas gingivalis were significantly enriched in the TC and CP groups. Among them, Treponema and P. oralis were the most abundant in the TC group. The PICRUSt results showed that many pathways related to cell motility and functional metabolism of the salivary microbial flora changed in the TC group and the CP group.
Conclusions: Diabetes was not the main factor causing the altered diversity of salivary microbiota in patients with periodontitis; however, the presence of diabetes altered the abundance of some microbiota in saliva.
Introduction
Diabetes mellitus is a common metabolic disorder characterized by chronic hyperglycemia. The latest epidemiological studies have shown that more than 440 million people worldwide suffer from diabetes (Lovic et al., 2020), mainly in countries in the Asia-Pacific region. According to statistics, China has the largest number of diabetic patients in the world (Ma, 2018). Therefore, diabetes is an unresolved public health problem in China and throughout the world.
Periodontitis is the sixth most common complication in people with diabetes (Löe, 1993). Current epidemiological investigations have demonstrated a bidirectional relationship between diabetes and periodontitis. Type 2 diabetes mellitus (T2DM) is the most common type of diabetes, and its age of onset is more similar to periodontitis. Considering the different etiologies of type 1 diabetes (T1DM) and T2DM, and the potential differences in the pathobiology of these two diseases, it is more beneficial to explore their effects on periodontitis separately to explain their relationship with periodontitis. Systemic effects associated with T2DM and periodontitis may act synergistically to produce more severe periodontal disease progression than T1DM (Novak et al., 2008). Increased severity of periodontal disease in T2DM may reflect the altered pathogenic potential of periodontal bacteria and/or altered host inflammatory response characteristics, which may lead to a breakdown of periodontal homeostasis (Naguib et al., 2004). However, studies on the effect of T2DM on the periodontitis microbiota have yielded conflicting results (Polak and Shapira, 2018). This may be due to factors such as different regions, ethnic differences, and age (Taylor et al., 2013). Therefore, it is necessary to conduct in-depth research on the differences in the microbiota structure of periodontitis patients with or without diabetes among different populations.
In the Chinese population, the impact of diabetes on periodontitis salivary flora is relatively unknown. In the study of inflammatory mediators and microbiota in periodontitis and systemic diseases, saliva has been proven to be a viable substitute for serum and gingival crevicular fluid (Deepa and Thirrunavukkarasu, 2010), which helps to solve some problems inherent to gingival crevicular fluid sampling (Ghallab, 2018), such as time consumption, multiple sampling, and blood contamination. With the loss of periodontal attachment and the appearance of deep periodontal pockets, many serum-like fluids and microorganisms in gingival crevicular fluid infiltrate into saliva; thus, most periodontal pathogens and inflammatory mediators can be detected in saliva (Kim et al., 2018). Therefore, this study used a Chinese population as the research object to explore the impact of diabetes on periodontitis-related microorganisms in the saliva, in order to provide a theoretical basis for further research on the microbial mechanism of diabetes on periodontitis.
Methods
Study Population
The subjects of the study were patients who visited the Department of Stomatology and Endocrinology of the First Affiliated Hospital of Jinan University from January 2021 to January 2022. Thirty volunteers were recruited by convenience sampling and divided into the periodontitis with diabetes group (TC group, n = 10), chronic periodontitis group (CP group, n = 10), and healthy group (H group, n = 10).
Periodontitis was defined by the presence of alveolar bone resorption in at least 30% of sites and more than 4 sites with probing depth (PD) ≥4 mm and clinical attachment loss (CAL) ≥2 mm (Zhou et al., 2013). T2DM was defined as glycated hemoglobin (HbA1C) ≥6.5% and fasting blood glucose (FBG) ≥7.0 mmol/L (Maboudi et al., 2019). Individuals who were diagnosed with periodontitis and had no history of diabetes, self-reported systemic health, or blood tests with normal HbA1C were included in the CP group. Patients newly diagnosed with T2DM in the endocrinology department and periodontitis diagnosed in the stomatology department were included in the TC group. Individuals without periodontitis and self-reported systemic health were included in the H group.
The following patients were excluded from our study: 1) any patient diagnosed with metabolic disease other than diabetes and systemic disease that may have affected the progression of the periodontitis, such as hyperthyroidism or cancer; 2) prior to the experimental history of special medication, such as antibiotics or hormones, within 6 months; 3) received periodontal treatment, such as scaling, within 6 months; 4) pregnant or breastfeeding; 5) current smokers (Becker et al., 2014); 6) remaining tooth with root caries. All participants signed a written informed consent form and agreed to use their own saliva samples for this study. This study was approved by the Medical Ethics Committee of the First Affiliated Hospital of Jinan University in China (Approval Number Kyk-2022-014) and strictly followed the Declaration of Helsinki.
Saliva Collection and DNA Extraction
Before the periodontal assessment, unstimulated whole saliva samples were collected by spitting. All research subjects were asked to fast for at least 8 h and to avoid eating, rinsing, brushing their teeth, and performing other oral exercises for at least 2 h before sampling. Then, 2 ml of saliva was collected between 9:00 am and 11:00 am and immediately stored in a −80°C refrigerator. The genomic DNA of the samples was extracted by the conventional cetyl trimethylammonium bromide method (CTAB), and the purity and concentration of the DNA were then detected by agarose gel electrophoresis.
High-Throughput Sequencing
With the use of the diluted genomic DNA as a template and specific primers with Barcode, PCR amplification was performed on the 16S rRNA gene V4 variable region. The forward primer sequence was 515F (5′-GTGCCAGCMGCCGCGCTAA-3′), and the reverse primer sequence was 806R (5′-GGACTACHVGGGTTWTCTAAT-3′). The TruSeq® DNA PCR-Free Sample Preparation Kit (Illumina, San Diego, CA, USA) was used for library construction. The library quality was assessed on the Qubit@ 2.0 Fluorometer (Thermo Scientific, Waltham, MA, USA) and Agilent Bioanalyzer 2100 system (Agilent Technologies, Santa Clara, CA, USA). After the library was qualified, the Illumina NovaSeq 6000 platform was used for on-machine sequencing.
Biological Analysis and Statistical Analysis
The original sequence was spliced and filtered to obtain clean data (effective tags). Then, Uparse software (Uparse v7.0.1001, http://www.drive5.com/uparse/) was used to perform operational taxonomic unit (OTU) clustering on the effective tags of all samples. Species annotation taxonomic analysis was performed using the Silva database (http://www.arb-silva.de/) based on the Mothur algorithm. Differences in dominant species between groups were compared using MUSCLE software (version 3.8.31, http://www.drive5.com/muscle/). After the data were normalized, alpha diversity analysis and beta diversity analysis were performed using QIIME software (Version 1.9.1) and R software (Version 2.15.3). With the use of R software (Version 2.15.3), statistical analysis methods, such as T-test, MetaStat, and analysis of similarity (ANOSIM), were used to test the significance of differences in the species composition and community structure of the grouped samples. PICRUSt predicted microbial function, and a T-test was used to detect functional differences between groups. Statistical analysis was performed on the baseline data of the study population using ANOVA or Tukey’s test and the chi-square test in GraphPad Prism software.
Results
Sociodemographic Characteristics and Clinical Examination
As shown in Table 1, there was no significant difference in age or sex among the TC group, CP group, and H group (p > 0.05). There were significant differences in CAL and PD between the CP group, TC group, and H group (p < 0.05), but there were no significant differences in CAL or PD between the CP group and TC group (p > 0.05). The average levels of HbA1C and FBG in the TC group were 8.54% ± 1.38% and 12.03 ± 2.63 mmol/L.
Operational Taxonomic Unit and Sequencing Depth Analysis
To study the bacterial community composition of each sample, OTUs were clustered with 97% consistency for the effective tags of all samples, and species annotation was then performed on the sequences of OTUs. In total, 2,471 OTUs were identified in this study, from which 22 phylum-, 277 genus-, and 264 species-level taxa were detected. As shown in Figure 1A, 711 OTUs overlapped in the three sets of samples. The CP group had 1,094 OTUs, the TC group had 1,045 OTUs, and the H group had 1,952 OTUs. The CP group had 224 unique OTUs, the TC group had 188 unique OTUs, and the H group had 1,150 unique OTUs. The sparsity curve among the three groups was flat (Figure 1B), indicating that the sequencing sampling of the study was reasonable and that more data volumes would yield only a small number of new species.
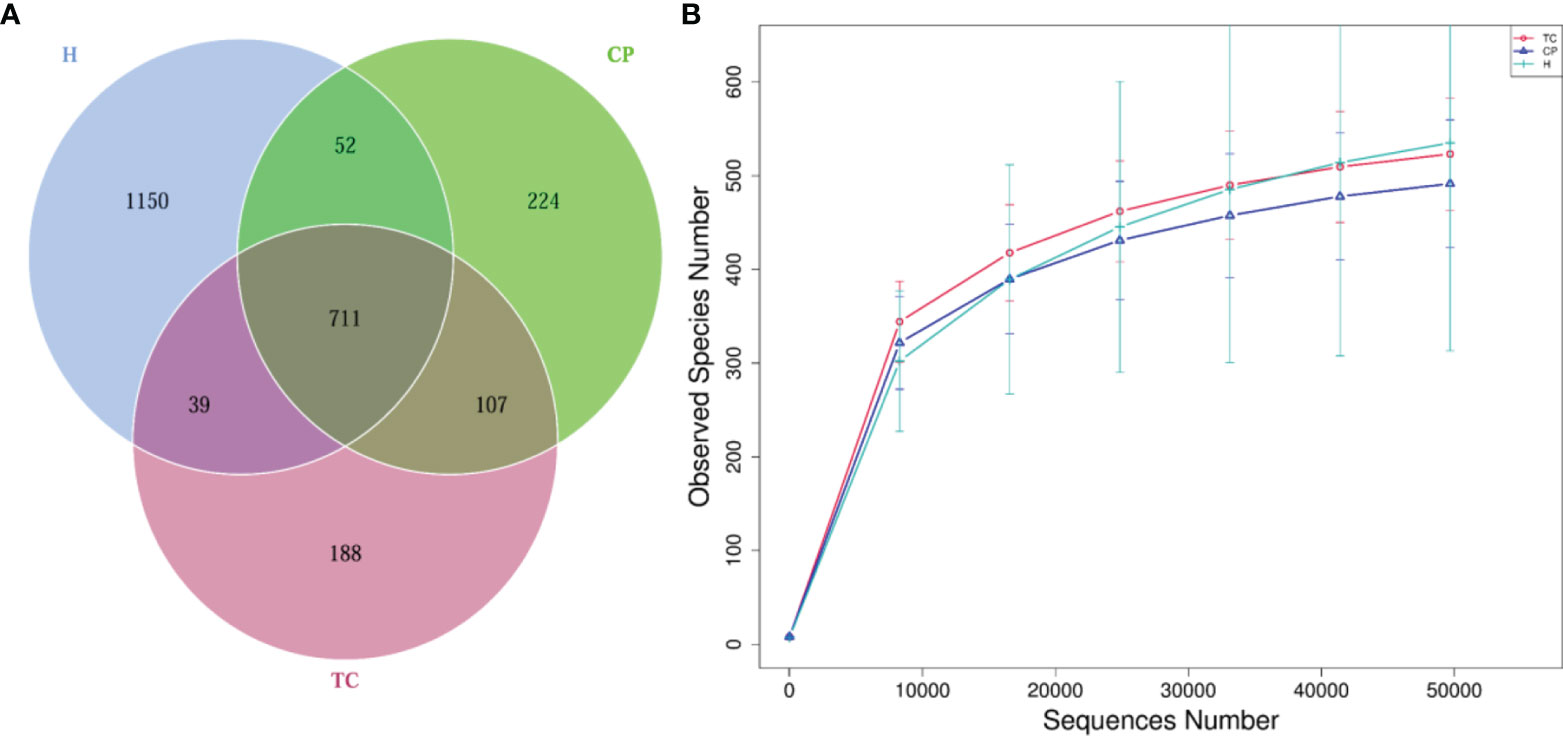
Figure 1 Venn diagram and rarefaction curve diagram. (A) Venn diagram. Each circle in the figure represents a group, the numbers in the overlapping parts of the circles and the circles represent the number of operational taxonomic units (OTUs) shared among the samples (groups), and the numbers without overlapping parts represent the unique OTUs of the groups. (B) Rarefaction curve diagram. The abscissa is the number of sequencing strips randomly selected from a sample, and the ordinate is the number of OTUs that can be constructed based on the number of sequencing strips. Different groups are represented by curves with different colors.
Microbial Diversity Analysis
The alpha diversity among the three groups is shown in Figures 2A, B. From the Beeswarm of the observed species (Figure 2A), there was no significant difference in species abundance among the three groups (p > 0.05). The Beeswarm of Shannon indicated (Figure 2B) that the diversity of the TC group and the CP group was significantly increased compared with that of the H group. However, there was no significant difference in species abundance or diversity between the TC group and the CP group.
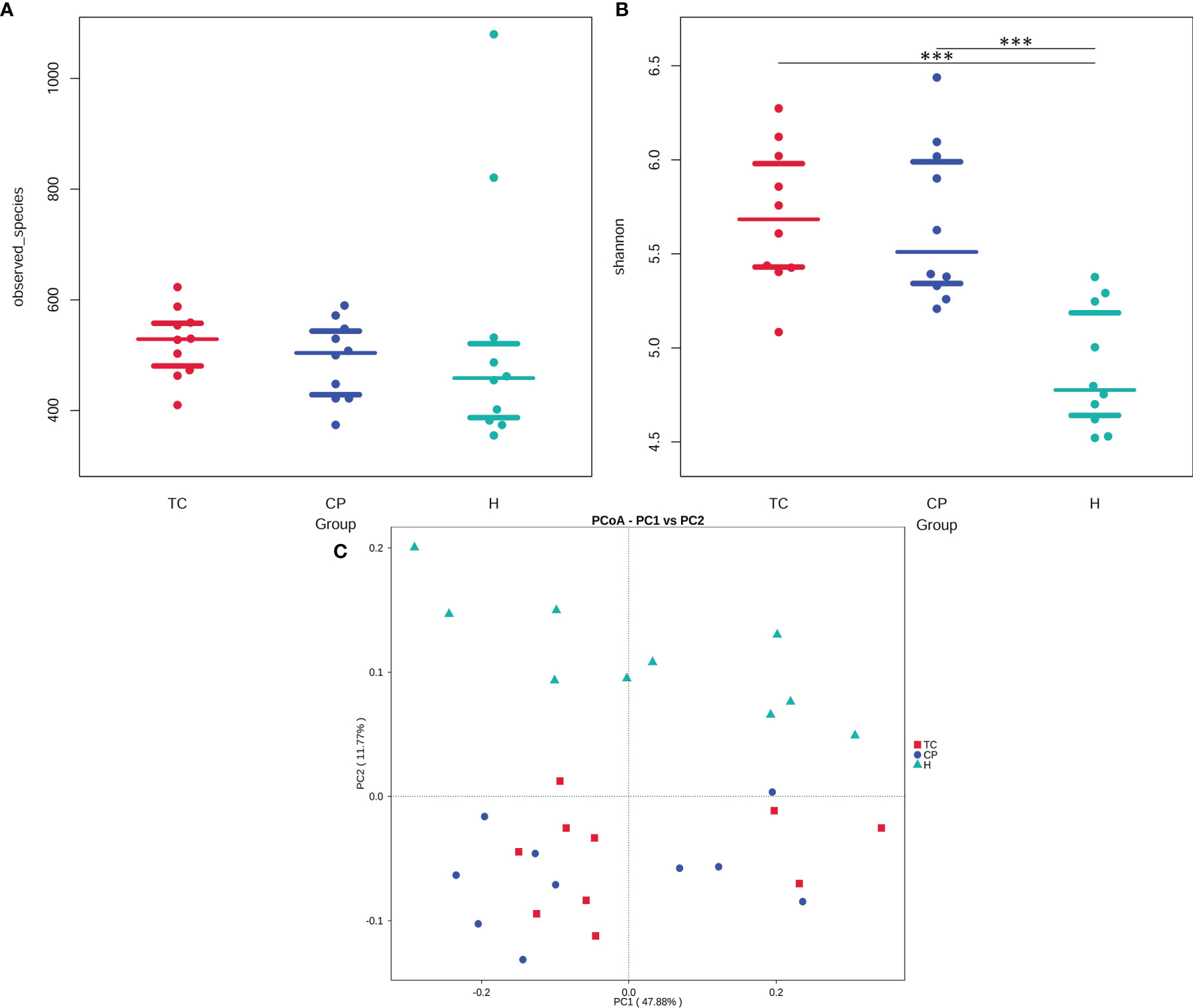
Figure 2 Diversity analysis plot. (A) Beeswarm of the observed species index. (B) Beeswarm of the Shannon index. (C) Principal coordinate analysis (PCoA) plot. The samples of the same group in the figure are represented by the same color, each point represents a sample, and the distance between the points represents the degree of difference.
The beta diversity among the three groups is shown in Figure 2C. The principal coordinate analysis (PCoA) based on the weighted UniFrac distance showed that the samples in the TC group and the CP group were closer in distance, indicating that the degree of bacterial evolution of the samples between the two groups was similar, and the bacterial community structure between the two groups was similar. The distance between the H group and the TC and CP groups was farther, indicating that the bacterial community structures of the disease study group (TC and CP groups) and the H group were significantly different. ANOSIM further showed that there was no significant difference in the flora structure between the TC group and the CP group (R2 = 0.008, p > 0.05). The bacterial community structure between the CP group and the H group (R2 = 0.162, p < 0.001) and the TC group and the H group (R2 = 0.204, p < 0.001) were statistically significant.
Differences in Bacterial Community Structure Between Groups at the Phylum Level
As shown in Figure 3A, at the phylum level, the three groups of samples were dominated by Proteobacteria, Bacteroidota, Firmicutes, Fusobacteriota, and Actinobacteria. The MetaStat and T-test results (Figure 4 and Supplementary Figure S1) showed that the phyla Fusobacteriota, Cyanobacteria, and Spirochaetota in the TC group were higher than those in the H group, and the phylum Gracilibacteria was lower than that in the H group; the phyla Fusobacteriota, Campylobacterota, Spirochaetota, and Cyanobacteria in the CP group were higher than those in the H group, while Firmicutes was lower than that in the H group; Spirochaetota was higher in the TC group than in the CP group.
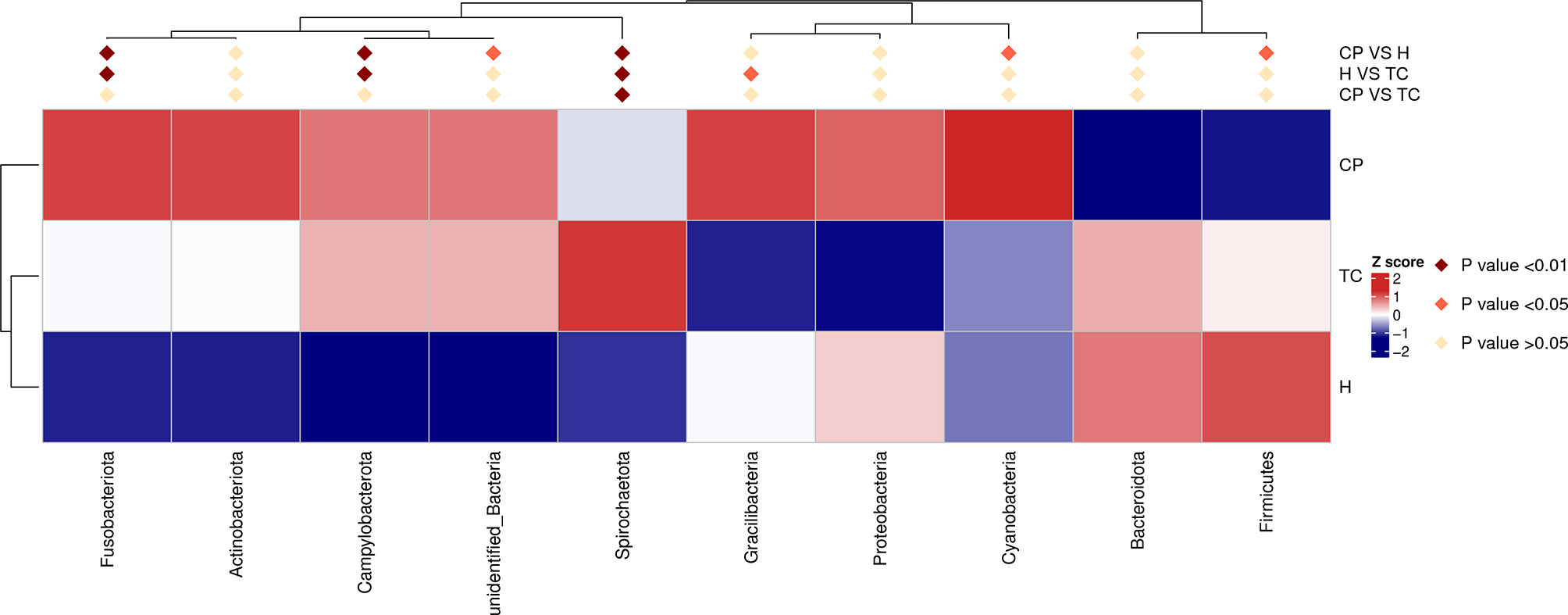
Figure 4 MetaStat analysis; phylum annotation heatmap. Different colors in the heatmap represent the values obtained after the Z score of the relative content of differential metabolites is standardized, reflecting the level of their relative content (red represents high content, and blue represents low content).
Differences in Bacterial Community Structure Between Groups at the Genus Level
As shown in Figure 3B, the genus-level distribution among the three groups of samples was in the order of Neisseria, Prevotella, Haemophilus, Streptococcus, Veillonella, and Porphyromonas. The MetaStat and T-test results (Figure 5 and Supplementary Figure S2) showed that the Leptotrichia, Selenomonas, Campylobacter, Catonella, Treponema, Corynebacterium, Capnocytophaga, Dialister, Comamonas, and Filifactor genera were higher in the TC group than in the H group; Haemophilus was lower than that in the H group; the Neisseria, Leptotrichia, Campylobacter, Catonella, Treponema, Corynebacterium, Capnocytophaga, Dialister, and Filifactor genera were higher in the CP group than in the H group, and Haemophilus, Streptococcus, and Veillonella were lower than those in the H group; the Treponema genus was higher in the TC group than the CP group.
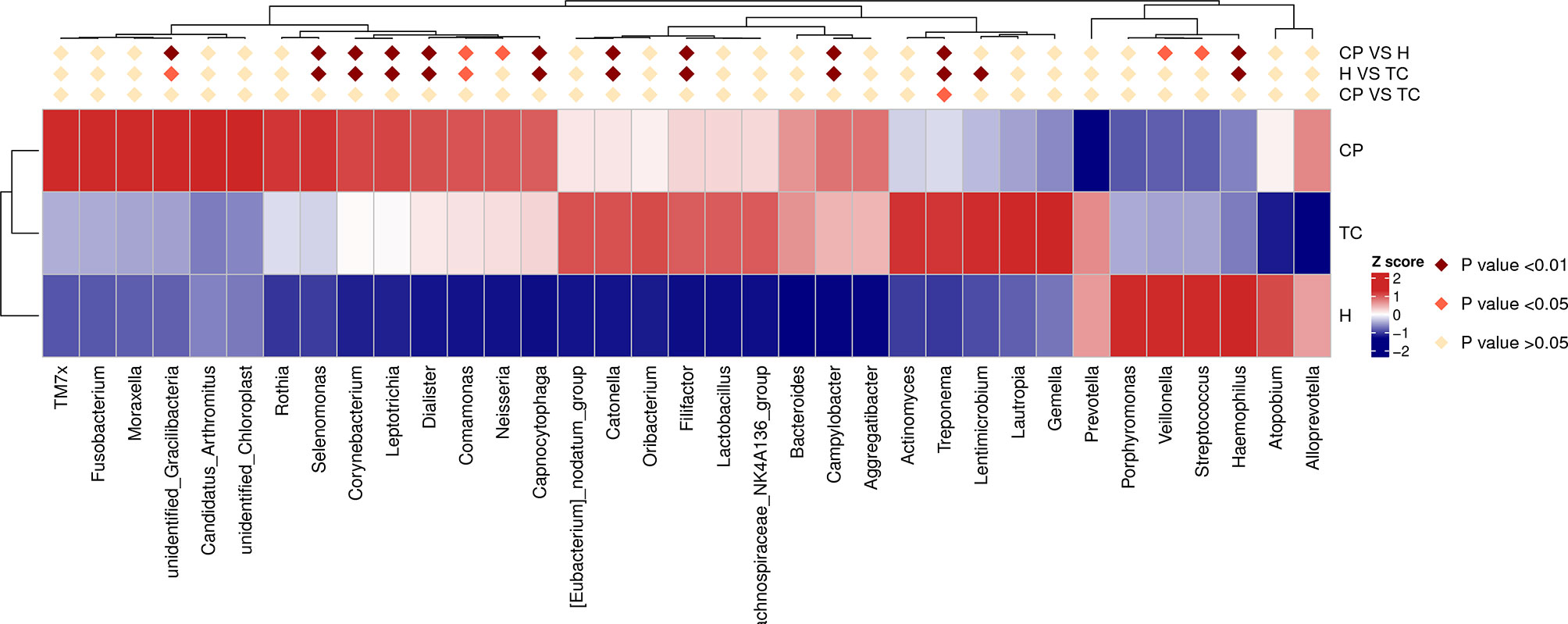
Figure 5 MetaStat analysis; genus annotation heatmap. Different colors in the heatmap represent the values obtained after the Z score of the relative content of differential metabolites is standardized, reflecting the level of their relative content (red represents high content, and blue represents low content).
Differences in Bacterial Community Structure Between Groups at the Species Level
The MetaStat and T-test results (Figure 6 and Supplementary Figure S3) showed that, at the species level, Campylobacter concisus, Prevotella oris, Porphyromonas gingivalis, and Prevotella intermedia were higher in the CP group and TC group than in the H group and that Haemophilus parainfluenzae was lower than that in the H group. The relative abundances of P. intermedia were higher in the TC group, compared with the CP group (Supplementary Figure S3).
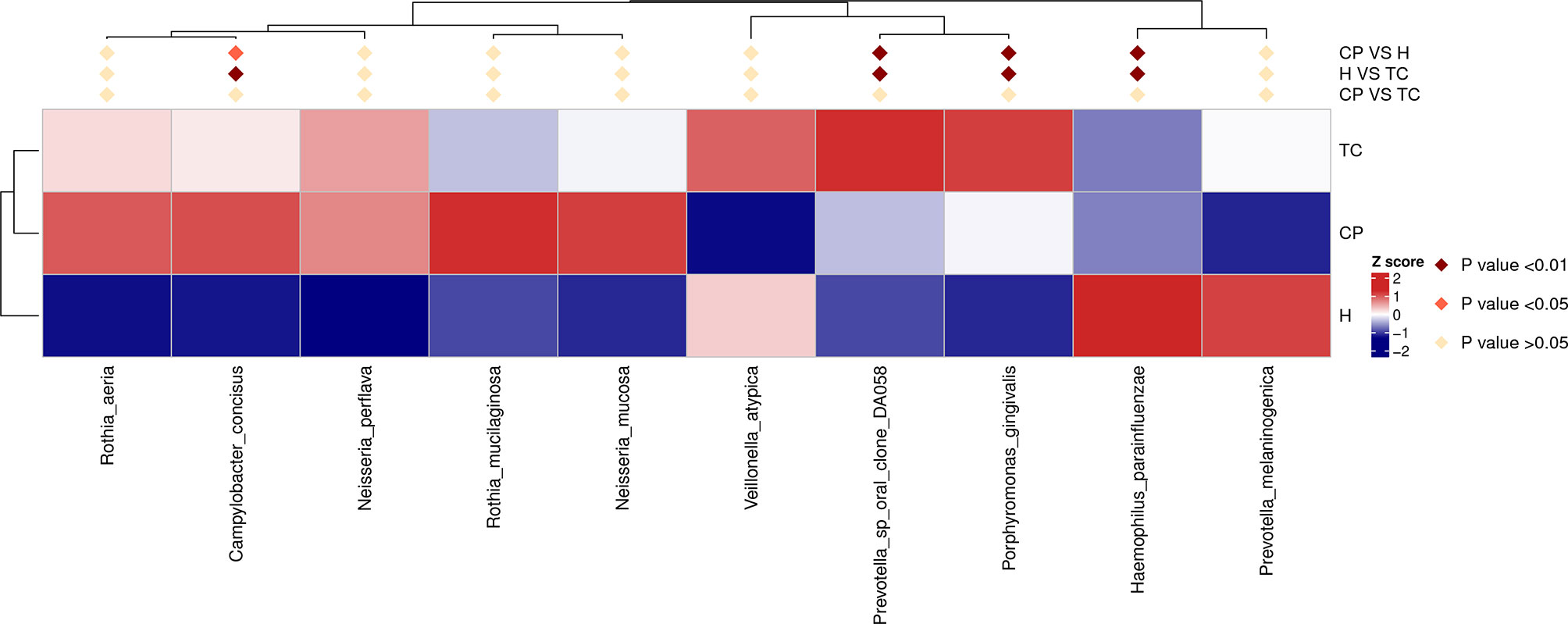
Figure 6 MetaStat analysis; species annotation heatmap. Different colors in the heatmap represent the values obtained after the Z score of the relative content of differential metabolites is standardized, reflecting the level of their relative content (red represents high content, and blue represents low content).
Predictive Analysis of Salivary Microbial Community Function
Using the PICRUSt algorithm, we performed functional predictions based on the Kyoto Encyclopedia of Genes and Genomes (KEGG) database for the three groups of microbial communities. As shown in Supplementary Figures S4A, B, at level 1, there were significant differences in the genetic information processing and the functions of cellular processes between the TC group, the CP group, and the H group (p < 0.05). As shown in Supplementary Figures S4C, D, S5, and S6, at level 2 and level 3, the TC group exhibited significantly enriched cellular processes (bacterial motility proteins, flagellar assembly, and bacterial chemotaxis), signaling (two-component system), basal energy (oxidative phosphorylation), and amino acid metabolism (aspartate metabolism, glutamate metabolism, histidine metabolism, tyrosine metabolism, tryptophan metabolism, etc.); the CP group showed significantly enriched amino acid metabolism (alanine, aspartic acid, glutamine, lysine, histidine, tyrosine, tryptophan, phenylalanine, etc.), cellular processes (cell motility, bacterial motility proteins, flagellar assembly, and bacterial chemotaxis), membrane transport (secretory system), basal energy (energy metabolism and oxidative phosphorylation), and other functions; group H showed significantly enriched replication and repair, translation, nucleotide metabolism (purine metabolism, pyrimidine metabolism) and other functions.
Discussion
Periodontitis is an inflammatory disease that is thought to be associated with a variety of microbial disturbances (Darveau, 2010). Considering the bidirectional relationship between diabetes and periodontitis, in the context of diabetes, the bacterial community structure and diversity of periodontitis may be altered, thereby exacerbating periodontal bone loss. In recent years, many countries have carried out research on the impact of diabetes on periodontitis-related flora. However, the findings of these studies are not necessarily applicable to the Chinese population. Therefore, based on the Chinese population, we explored the influence of the presence or absence of diabetes on the salivary flora of periodontitis patients.
Similar to our findings, Liu (Liu et al., 2021) and Farina (Farina et al., 2019) et al. showed that diabetes did not affect the diversity of salivary microbiota in the context of periodontitis disease. Previous studies have shown that the presence of diabetes alone does not affect the structure of the salivary flora. This is further supported by studies by Almeida-Santos (Almeida-Santos et al., 2021) and Shi et al. (2020) In the study by Sun et al., periodontitis patients with and without T2DM had an increased diversity of salivary flora compared with healthy controls, while there was no significant difference between T2DM and periodontitis with T2DM patients after active blood sugar control (Sun et al., 2020). Therefore, they believe that periodontitis-related parameters are the main factors affecting the composition of salivary microbes, and the combined effect of T2DM and periodontitis on changes in the salivary microbiome is significantly greater than that of T2DM alone.
Compared with the H group or the CP group, the TC group showed an increasing trend in the flora biodiversity. This is similar to that reported by Balmasova (Balmasova et al., 2021) and Chen (Chen et al., 2020). However, Sabharwal (Sabharwal et al., 2019) and Saeb et al. (2019) believed that the presence of diabetes reduces the diversity of periodontitis salivary flora. These studies have different viewpoints, which may be attributed to the following reasons: 1) the sample size of each study was different, and small sample sizes may lead to a limited detection rate of flora related to the background of periodontitis. 2) Salivary flora may be affected by blood sugar status and different stages of periodontal disease, but many studies have not strictly distinguished whether the differential changes in salivary flora are caused by hyperglycemia or periodontal disease. 3) Studies have shown that the microbiota of stimulated saliva samples and unstimulated saliva samples is different (Gomar-Vercher et al., 2018); thus, the different ways of collecting saliva samples in each study may have affected the microbiota analysis results.
The dominant bacterial phyla in saliva were mainly Firmicutes, Bacteroidota, Proteobacteria, Actinobacteria, and Fusobacteriota (Lazarevic et al., 2010; Wade, 2013), among which Firmicutes were mainly enriched in healthy people (Griffen et al., 2012; Esparbès et al., 2021). This is largely consistent with the findings of this study. The statistical analysis of the differences in community structure at the phylum level showed that the common dominant bacterial phyla in the TC and CP groups were Fusobacteriota, Spirochaetota, and Campylobacterota, and Spirochaetota was more significantly enriched in the TC group. The Spirochaetota phylum is mainly composed of the genus Treponema. At the genus level, differential expression of this genus was observed in the TC group. It has been reported that the Spirochaetota flora accounts for 50% of the subgingival microbial population in periodontitis and less than 1% in healthy individuals (Chan and McLaughlin, 2000). They were found to be significantly higher in relative abundance in moderate-to-severe periodontitis (Listgarten and Levin, 1981; Armitage et al., 1982). The results of Marotz et al. confirmed that the log ratio of Treponema and Corynebacterium is a novel microbial indicator of periodontitis, and the increase in the detection rate of Treponema predicts the trend of periodontitis (Marotz et al., 2022). Diabetes has been reported to significantly increase the expression levels of these genera in the oral cavity (Sabharwal et al., 2019; Sun et al., 2020; Balmasova et al., 2021). A potential reason for the selective increase in this microbiota in periodontitis with diabetes may be the altered microenvironment due to differences in blood glucose, leading to the preferential proliferation of microorganisms with different growth requirements in specific oral niches.
At the genus level, the three groups of saliva samples were dominated by Neisseria, Prevotella, and Haemophilus species. The relative relationship between Corynebacterium, Leptotrichia, Dialister, Comamonas, Capnocytophaga, Catonella, Filifactor, Campylobacter, and Treponema in the TC and CP groups was higher than that in the H group, while Haemophilus, Veillonella, and Streptococcus were more abundant in the H group. In the process of plaque formation, Streptococcus, Haemophilus, Veillonella, Actinomyces, and Fusobacterium are the main flora in the early stage of bacterial colonization (Marsh, 2010). Periodontal disease-associated flora was observed to increase in abundance within the plaque as the plaque matured (Borisy and Valm, 2021). Belstrøm et al. suggested that Streptococcus gradually decreased in the late stage of oral plaque formation, while the abundance of some periodontal disease-related genera, such as Leptotrichia and Prevotella, gradually increased (Belstrøm et al., 2018). Therefore, the differences in the composition of microflora in saliva may indirectly reflect different stages of plaque formation and different stages of periodontitis. At the genus level, oral Streptococcus was confirmed to be the most abundant genus in healthy populations. However, Haemophilus, Veillonella, Prevotella, Leptotrichia, Campylobacter, Neisseria, and Capnocytophaga were not only expressed in periodontitis but also enriched in the periodontally healthy (Cao et al., 2018; Chen et al., 2018; Abusleme et al., 2021). This may be due to differences in the pathogenic potential of bacteria of the same genus.
At the species level, the dominant floras of the TC group and CP group were C. concisus, Prevotella oralis, and P. gingivalis, while the dominant flora of the H group was H. parainfluenzae. The presence of diabetes increased the expression of P. intermedia in saliva. These two bacteria belong to a core group closely associated with periodontitis and were confirmed to be positively associated with the subgingival plaque flora (Sakamoto et al., 2000; Belstrøm et al., 2017). Therefore, we speculate that patients with T2DM are more susceptible to the dysbiosis of oral flora, which in turn increases the risk and severity of periodontitis. In fact, the role of P. gingivalis and Prevotella (e.g., P. intermedia) in periodontitis has been extensively studied, while research on C. concisus in periodontitis is still limited. Studies have reported that the abundance of C. concisus is associated with increased levels of attachment loss in subjects with periodontitis (Haffajee et al., 1984). Henne et al. suggested that periodontitis does not affect changes in the absolute abundance of periodontitis and does not affect absolute changes in the abundance of C. concisus in the oral cavity, but the proportion of different species within Campylobacter may reflect microbial community changes as periodontitis progresses (Henne et al., 2014). The role of Campylobacter in periodontitis still needs further research in the future.
This study used the PICRUSt algorithm to predict the biological functions of differential enrichment of salivary microbiota in different groups. It is worth noting that cell motility, bacterial motility proteins, flagellar assembly, and bacterial chemotaxis were significantly enriched in the TC and CP groups. These biological functions related to the microbial virulence system can promote the adhesion and invasion of pathogenic microorganisms in periodontal tissue and then play an important role in the pathological process of periodontitis (Cai et al., 2021). In addition, similar to previous studies (Ikeda et al., 2020), we observed upregulation of pathways related to the metabolism of amino acids, such as aspartate, glutamate, tyrosine, tryptophan, and phenylalanine, in the TC group and the CP group. Although the underlying mechanism of amino acids in diabetes and periodontitis is not clear, amino acid metabolism (e.g., phenylalanine, tryptophan, and tyrosine) has been associated with the risk of diabetes and periodontitis in previous studies (Chen et al., 2016; Liebsch et al., 2019). Increases in certain amino acid catabolism-derived metabolites may induce disruption of host homeostasis, which in turn promotes the progression of periodontal inflammation (Kim, 2011).
It is worth noting that the small sample size of this study may have limited the detection rate of microbiota. In addition, we did not consider the effect of different periodontitis states on the expression of salivary flora. Therefore, the findings of this study need to be interpreted with caution. Nonetheless, we detected differential expression of some microbiota in periodontitis saliva in the presence of diabetes, and these results may inform future studies of these two common diseases.
Conclusions
Diabetes was not the main factor causing the altered diversity of salivary microbiota in patients with periodontitis, but the presence of diabetes altered the expression abundance of some microbiota in saliva.
Data Availability Statement
The datasets presented in this study can be found in online repositories. The name of the repository and accession number can be found below: NCBI; PRJNA843376.
Ethics Statement
The studies involving human participants were reviewed and approved by The Ethics Committee of the First Affiliated Hospital of Jinan University, China. The patients/participants provided their written informed consent to participate in this study.
Author Contributions
ZL designed the research project. CL, QZ and JD conducted experiments and contributed significantly to analysis and manuscript preparation. KC and XJ performed the data analyses and wrote the manuscript. FM and SM helped perform the analysis with constructive discussions. All the authors discussed and analyzed the experimental data, and wrote and revised the manuscript. All authors have reviewed the manuscript.
Funding
This work was partly supported by the National Nature Science Foundation (grant No. 81804153), Guangzhou Science and Technology Plan Foundation and Application Foundation Research Project (grant No. 202102020020), Guangdong Foundation for Basic and Applied Basic Research (grant No. 2019A1515110161), Fundamental Research for the Central Universities (grant No. 21621410), Scientific Research Project of Guangdong Provincial Administration of Traditional Chinese Medicine (grant No. 20201107), and Starting fund for doctoral research of the Sixth Affiliated Hospital of Jinan University (grant No. JDLY2022001).
Conflict of Interest
The authors declare that the research was conducted in the absence of any commercial or financial relationships that could be construed as a potential conflict of interest.
Publisher’s Note
All claims expressed in this article are solely those of the authors and do not necessarily represent those of their affiliated organizations, or those of the publisher, the editors and the reviewers. Any product that may be evaluated in this article, or claim that may be made by its manufacturer, is not guaranteed or endorsed by the publisher.
Supplementary Material
The Supplementary Material for this article can be found online at: https://www.frontiersin.org/articles/10.3389/fcimb.2022.933833/full#supplementary-material
Supplementary Figure S1 | T test analysis of species differences at the phylum level between groups. (A) TC and H groups. (B) CP and H groups. (C) TC and CP groups. The left picture shows the difference in species abundance between groups, and each bar in the figure represents the mean value of species with significant differences in abundance between groups in each group.
Supplementary Figure S2 | T test analysis of species differences at the genus level between groups. (A) TC and H groups. (B) CP and H groups. (C) TC and CP groups. The left picture shows the difference in species abundance between groups, and each bar in the figure represents the mean value of species with significant differences in abundance between groups in each group.
Supplementary Figure S3 | T test analysis of species differences at the species level between groups. (A) TC and H groups. (B) CP and H groups. (C) TC and CP groups. The left picture shows the difference in species abundance between groups, and each bar in the figure represents the mean value of species with significant differences in abundance between groups in each group.
Supplementary Figure S4 | PICRUSt predictions of the functional composition of the saliva microbiome. (A) KEGG pathway at level 1 between the TC and the H groups; (B) KEGG pathway at level 1 between the CP and the H groups; (C) KEGG pathway at level 2 between the TC and the H groups; (D) KEGG pathway at level 2 between the CP and the H groups. The left picture shows the difference in species abundance between groups, and each bar in the figure represents the mean value of species with significant differences in abundance between groups in each group.
Supplementary Figure S5 | PICRUSt predictions of the functional composition of saliva microbiome at level 3 between TC and H group. The left picture shows the difference in species abundance between groups, and each bar in the figure represents the mean value of species with significant differences in abundance between groups in each group.
Supplementary Figure S6 | PICRUSt predictions of the functional composition of saliva microbiome at level 3 between CP and H group. The left picture shows the difference in species abundance between groups, and each bar in the figure represents the mean value of species with significant differences in abundance between groups in each group.
References
Abusleme, L., Hoare, A., Hong, B. Y., Diaz, P. I. (2021). Microbial Signatures of Health, Gingivitis, and Periodontitis. Periodontol. 2000 86 (1), 57–78. doi: 10.1111/prd.12362
Almeida-Santos, A., Martins-Mendes, D., Gayà-Vidal, M., Pérez-Pardal, L., Beja-Pereira, A. (2021). Characterization of the Oral Microbiome of Medicated Type-2 Diabetes Patients. Front. Microbiol. 12. doi: 10.3389/fmicb.2021.610370
Armitage, G., Dickinson, W., Jenderseck, R., Levine, S., Chambers, D. (1982). Relationship Between the Percentage of Subgingival Spirochetes and the Severity of Periodontal Disease. J. Periodontol. 53 (9), 550–556. doi: 10.1902/jop.1982.53.9.550
Balmasova, I. P., Olekhnovich, E. I., Klimina, K. M., Korenkova, A. A., Vakhitova, M. T., Babaev, E. A., et al. (2021). Drift of the Subgingival Periodontal Microbiome During Chronic Periodontitis in Type 2 Diabetes Mellitus Patients. Pathogens 10 (5), 504. doi: 10.3390/pathogens10050504
Becker, S. T., Beck-Broichsitter, B. E., Graetz, C., Dörfer, C. E., Wiltfang, J., Häsler, R. (2014). Peri-Implantitis Versus Periodontitis: Functional Differences Indicated by Transcriptome Profiling. Clin. Implant Dent. Relat. Res. 16 (3), 401–411. doi: 10.1111/cid.12001
Belstrøm, D., Sembler-Møller, M. L., Grande, M. A., et al. (2017). Microbial Profile Comparisons of Saliva, Pooled and Site-Specific Subgingival Samples in Periodontitis Patients. PloS One 12 (8), e0182992. doi: 10.1371/journal.pone.0182992
Belstrøm, D., Sembler-Møller, M., Grande, M., Kirkby, N., Cotton, S. L., Paster, B. J, et al. (2018). Impact of Oral Hygiene Discontinuation on Supragingival and Salivary Microbiomes. JDR Clin. Trans. Res. 3 (1), 57–64. doi; 10.1177/2380084417723625
Borisy, G. G., Valm, A. M. (2021). Spatial Scale in Analysis of the Dental Plaque Microbiome. Periodontol. 2000 86 (1), 97–112. doi: 10.1111/prd.12364
Cai, Z., Lin, S., Hu, S., Zhao, L. (2021). Structure and Function of Oral Microbial Community in Periodontitis Based on Integrated Data. Front. Cell. Infect. Microbiol. 11. doi: 10.3389/fcimb.2021.663756
Cao, Y., Qiao, M., Tian, Z., Yu, Y., Xu, B., Lao, W., et al. (2018). Comparative Analyses of Subgingival Microbiome in Chronic Periodontitis Patients With and Without IgA Nephropathy by High Throughput 16S rRNA Sequencing. Cell Physiol. Biochem. 47 (2), 774–783. doi: 10.1159/000490029
Chan, E., McLaughlin, R. (2000). Taxonomy and Virulence of Oral Spirochetes. Oral. Microbiol. Immunol.: Mini Rev 15 (1), 1–9. doi: 10.1034/j.1399-302x.2000.150101.x
Chen, W.-P., Chang, S.-H., Tang, C.-Y., Liou, M.-L., Tsai, S.-J. J., Lin, Y.-L. (2018). Composition Analysis and Feature Selection of the Oral Microbiota Associated With Periodontal Disease. BioMed. Res. Int. 2018, 3130607. doi: 10.1155/2018/3130607
Chen, T., Ni, Y., Ma, X., Bao, Y., Liu, J., Huang, F, et al. (2016). Branched-Chain and Aromatic Amino Acid Profiles and Diabetes Risk in Chinese Populations. Sci. Rep. 6 (1), 1–8. doi: 10.1038/srep20594
Chen, B., Wang, Z., Wang, J., Su, X., Yang, J., Zhang, Q., et al. (2020). The Oral Microbiome Profile and Biomarker in Chinese Type 2 Diabetes Mellitus Patients. Endocrine 68 (3), 564–572. doi: 10.1007/s12020-020-02269-6
Darveau, R. P. (2010). Periodontitis: A Polymicrobial Disruption of Host Homeostasis. Nat. Rev. Microbiol. 8 (7), 481–490. doi: 10.1038/nrmicro2337
Deepa, T., Thirrunavukkarasu, N. (2010). Saliva as a Potential Diagnostic Tool. Indian J. Med. Sci. 64 (7), 293–306. doi: 10.4103/0019-5359.99854
Esparbès, P., Legrand, A., Bandiaky, O. N., Carpentier, M., Martin, H., Montassier, E., et al. (2021). Subgingival Microbiota and Cytokines Profile Changes in Patients With Periodontitis: A Pilot Study Comparing Healthy and Diseased Sites in the Same Oral Cavities. Microorganisms 9 (11), 2364. doi: 10.3390/microorganisms9112364
Farina, R., Severi, M., Carrieri, A., Miotto, E., Sabbioni, S., Trombelli, L., et al. (2019). Whole Metagenomic Shotgun Sequencing of the Subgingival Microbiome of Diabetics and Non-Diabetics With Different Periodontal Conditions. Arch. Oral. Biol. 104, 13–23. doi: 10.1016/j.archoralbio.2019.05.025
Ghallab, N. A. (2018). Diagnostic Potential and Future Directions of Biomarkers in Gingival Crevicular Fluid and Saliva of Periodontal Diseases: Review of the Current Evidence. Arch. Oral. Biol. 87, 115–124. doi: 10.1016/j.archoralbio.2017.12.022
Gomar-Vercher, S., Simón-Soro, A., Montiel-Company, J. M., Almerich-Silla, J. M., Mira, A. (2018). Stimulated and Unstimulated Saliva Samples Have Significantly Different Bacterial Profiles. PloS One 13 (6), e0198021. doi: 10.1371/journal.pone.0198021
Griffen, A. L., Beall, C. J., Campbell, J. H., Firestone, N. D., Kumar, P. S., Yang, Z. K., et al. (2012). Distinct and Complex Bacterial Profiles in Human Periodontitis and Health Revealed by 16S Pyrosequencing. ISME J. 6 (6), 1176–1185. doi: 10.1038/ismej.2011.191
Haffajee, A., Socransky, S., Ebersole, J., Smith, D. (1984). Clinical, Microbiological and Immunological Features Associated With the Treatment of Active Periodontosis Lesions. J. Clin. Periodontol. 11 (9), 600–618. doi: 10.1111/j.1600-051X.1984.tb00913.x
Henne, K., Fuchs, F., Kruth, S., Horz, H.-P., Conrads, G. (2014). Shifts in Campylobacter Species Abundance may Reflect General Microbial Community Shifts in Periodontitis Progression. J. Oral. Microbiol. 6 (1), 25874. doi: 10.3402/jom.v6.25874
Ikeda, E., Shiba, T., Ikeda, Y., Suda, W., Nakasato, A., Takeuchi, Y., et al. (2020). Japanese Subgingival Microbiota in Health vs Disease and Their Roles in Predicted Functions Associated With Periodontitis. Odontology 108 (2), 280–291. doi: 10.1007/s10266-019-00452-4
Kim, H. (2011). Glutamine as an Immunonutrient. Yonsei Med. J. 52 (6), 892–897. doi: 10.3349/ymj.2011.52.6.892
Kim, E.-H., Joo, J.-Y., Lee, Y. J., Koh, J. K., Choi, J. H., Shin, Y., et al. (2018). Grading System for Periodontitis by Analyzing Levels of Periodontal Pathogens in Saliva. PloS One 13 (11), e0200900. doi: 10.1371/journal.pone.0200900
Lazarevic, V., Whiteson, K., Hernandez, D., François, P., Schrenzel, J. (2010). Study of Inter-and Intra-Individual Variations in the Salivary Microbiota. BMC Genomics 11 (1), 1–11. doi: 10.1186/1471-2164-11-523
Liebsch, C., Pitchika, V., Pink, C., Samietz, S., Kastenmüller, G., Artati, A., et al. (2019). The Saliva Metabolome in Association to Oral Health Status. J. Dent. Res. 98 (6), 642–651. doi: 10.1177/0022034519842853
Listgarten, M., Levin, S. (1981). Positive Correlation Between the Proportions of Subgingival Spirochetes and Motile Bacteria and Susceptibility of Human Subjects to Periodontal Deterioration. J. Clin. Periodontol. 8 (2), 122–138. doi: 10.1111/j.1600-051X.1981.tb02352.x
Liu, Y.-K., Chen, V., He, J.-Z., Zheng, X., Xu, X., Zhou, X.-D. (2021). A Salivary Microbiome-Based Auxiliary Diagnostic Model for Type 2 Diabetes Mellitus. Arch. Oral. Biol. 126, 105118. doi: 10.1016/j.archoralbio.2021.105118
Löe, H. (1993). Periodontal Disease: The Sixth Complication of Diabetes Mellitus. Diabetes Care 16 (1), 329–334. doi: 10.2337/diacare.16.1.329
Lovic, D., Piperidou, A., Zografou, I., Grassos, H., Pittaras, A., Manolis, A. (2020). The Growing Epidemic of Diabetes Mellitus. Curr. Vasc. Pharmacol. 18 (2), 104–109. doi: 10.2174/1570161117666190405165911
Ma, R. C. (2018). Epidemiology of Diabetes and Diabetic Complications in China. Diabetologia 61 (6), 1249–1260. doi: 10.1007/s00125-018-4557-7
Maboudi, A., Eghbalian-Nouzanizadeh, A., Seifi, H., Bahar, A., Mohadese, M., Ali Mohammadpour, R., et al. (2019). Serum Levels of Interleukin-23 and 35 in Patients With and Without Type 2 Diabetes Mellitus and Chronic Periodontitis. Caspian J. Internal Med. 10 (3), 295–302. doi: 10.22088/cjim.10.3.295
Marotz, C., Molinsky, R., Martino, C., Bohn, B., Roy, S., Rosenbaum, M, et al. (2022). Early Microbial Markers of Periodontal and Cardiometabolic Diseases in ORIGINS. NPJ Biofilms Microbiomes 8 (1), 1–10. doi: 10.1038/s41522-022-00289-w
Marsh, P. D. (2010). Microbiology of Dental Plaque Biofilms and Their Role in Oral Health and Caries. Dental Clinics 54 (3), 441–454. doi: 10.1016/j.cden.2010.03.002
Naguib, G., Al-Mashat, H., Desta, T., Graves, D. T. (2004). Diabetes Prolongs the Inflammatory Response to a Bacterial Stimulus Through Cytokine Dysregulation. J. Invest. Dermatol. 123 (1), 87–92. doi: 10.1111/j.0022-202X.2004.22711.x
Novak, M. J., Potter, R. M., Blodgett, J., Ebersole, J. L. (2008). Periodontal Disease in Hispanic Americans With Type 2 Diabetes. J. Periodontol. 79 (4), 629–636. doi: 10.1902/jop.2008.070442
Polak, D., Shapira, L. (2018). An Update on the Evidence for Pathogenic Mechanisms That may Link Periodontitis and Diabetes. J. Clin. Periodontol. 45 (2), 150–166. doi: 10.1111/jcpe.12803
Sabharwal, A., Ganley, K., Miecznikowski, J. C., Haase, E. M., Barnes, V., Scannapieco, F. A. (2019). The Salivary Microbiome of Diabetic and non-Diabetic Adults With Periodontal Disease. J. Periodontol. 90 (1), 26–34. doi: 10.1002/JPER.18-0167
Saeb, A. T., Al-Rubeaan, K. A., Aldosary, K., Udaya Raja, G. K., Mani, B., Abouelhoda, M., et al. (2019). Relative Reduction of Biological and Phylogenetic Diversity of the Oral Microbiota of Diabetes and Pre-Diabetes Patients. Microb. Pathog. 128, 215–229. doi: 10.1016/j.micpath.2019.01.009
Sakamoto, M., Umeda, M., Ishikawa, I., Benno, Y. (2000). Comparison of the Oral Bacterial Flora in Saliva From a Healthy Subject and Two Periodontitis Patients by Sequence Analysis of 16S rDNA Libraries. Microbiol. Immunol. 44 (8), 643–652. doi: 10.1111/j.1348-0421.2000.tb02545.x
Shi, B., Lux, R., Klokkevold, P., Chang, M., Barnard, E., Haake, S., et al. (2020). The Subgingival Microbiome Associated With Periodontitis in Type 2 Diabetes Mellitus. ISME J. 14 (2), 519–530. doi: 10.1038/s41396-019-0544-3
Sun, X., Li, M., Xia, L., Fang, Z., Yu, S., Gao, J., et al. (2020). Alteration of Salivary Microbiome in Periodontitis With or Without Type-2 Diabetes Mellitus and Metformin Treatment. Sci. Rep. 10 (1), 1–14. doi: 10.1038/s41598-020-72035-1
Taylor, J. J., Preshaw, P. M., Lalla, E. (2013). A Review of the Evidence for Pathogenic Mechanisms That may Link Periodontitis and Diabetes. J. Clin. Periodontol. 40, S113–S134. doi: 10.1111/jcpe.12059
Wade, W. G. (2013). The Oral Microbiome in Health and Disease. Pharmacol. Res. 69 (1), 137–143. doi: 10.1016/j.phrs.2012.11.006
Keywords: diabetes, periodontitis, saliva, 16S rDNA, microbiota
Citation: Lu C, Zhao Q, Deng J, Chen K, Jiang X, Ma F, Ma S and Li Z (2022) Salivary Microbiome Profile of Diabetes and Periodontitis in a Chinese Population. Front. Cell. Infect. Microbiol. 12:933833. doi: 10.3389/fcimb.2022.933833
Received: 01 May 2022; Accepted: 06 June 2022;
Published: 01 August 2022.
Edited by:
Zheng Zhang, Nankai University, ChinaReviewed by:
Shu Deng, Boston University, United StatesKe Deng, Shanghai Jiao Tong University, China
Ying An, Fourth Military Medical University, China
Yalin Zhan, Peking University Hospital of Stomatology, China
Copyright © 2022 Lu, Zhao, Deng, Chen, Jiang, Ma, Ma and Li. This is an open-access article distributed under the terms of the Creative Commons Attribution License (CC BY). The use, distribution or reproduction in other forums is permitted, provided the original author(s) and the copyright owner(s) are credited and that the original publication in this journal is cited, in accordance with accepted academic practice. No use, distribution or reproduction is permitted which does not comply with these terms.
*Correspondence: Zejian Li, dGxpemVqaWFuQGpudS5lZHUuY24=
†These authors have contributed equally to this work