- 1Department of Food Science, Cornell University, Ithaca, NY, United States
- 2Department of Clinical Microbiology, SciLifeLab, Umeå University, Umeå, Sweden
- 3Laboratory for Molecular Infection Medicine Sweden (MIMS), Umeå University, Umeå, Sweden
- 4Umeå Centre for Microbial Research, Umeå University, Umeå, Sweden
- 5Integrated Science Lab, Umeå University, Umeå, Sweden
Mobilized colistin resistance genes (mcr) may confer resistance to the last-resort antimicrobial colistin and can often be transmitted horizontally. mcr encode phosphoethanolamine transferases (PET), which are closely related to chromosomally encoded, intrinsic lipid modification PET (i-PET; e.g., EptA, EptB, CptA). To gain insight into the evolution of mcr within the context of i-PET, we identified 69,814 MCR-like proteins present across 256 bacterial genera (obtained by querying known MCR family representatives against the National Center for Biotechnology Information [NCBI] non-redundant protein database via protein BLAST). We subsequently identified 125 putative novel mcr-like genes, which were located on the same contig as (i) ≥1 plasmid replicon and (ii) ≥1 additional antimicrobial resistance gene (obtained by querying the PlasmidFinder database and NCBI’s National Database of Antibiotic Resistant Organisms, respectively, via nucleotide BLAST). At 80% amino acid identity, these putative novel MCR-like proteins formed 13 clusters, five of which represented putative novel MCR families. Sequence similarity and a maximum likelihood phylogeny of mcr, putative novel mcr-like, and ipet genes indicated that sequence similarity was insufficient to discriminate mcr from ipet genes. A mixed-effect model of evolution (MEME) indicated that site- and branch-specific positive selection played a role in the evolution of alleles within the mcr-2 and mcr-9 families. MEME suggested that positive selection played a role in the diversification of several residues in structurally important regions, including (i) a bridging region that connects the membrane-bound and catalytic periplasmic domains, and (ii) a periplasmic loop juxtaposing the substrate entry tunnel. Moreover, eptA and mcr were localized within different genomic contexts. Canonical eptA genes were typically chromosomally encoded in an operon with a two-component regulatory system or adjacent to a TetR-type regulator. Conversely, mcr were represented by single-gene operons or adjacent to pap2 and dgkA, which encode a PAP2 family lipid A phosphatase and diacylglycerol kinase, respectively. Our data suggest that eptA can give rise to “colistin resistance genes” through various mechanisms, including mobilization, selection, and diversification of genomic context and regulatory pathways. These mechanisms likely altered gene expression levels and enzyme activity, allowing bona fide eptA to evolve to function in colistin resistance.
1 Introduction
Colistin, a polycationic peptide, serves as a last-resort antimicrobial, which can be used to treat infections caused by multidrug-resistant (MDR), extensively drug-resistant (XDR), and pan-drug-resistant (PDR) Gram-negative bacteria (El-Sayed Ahmed et al., 2020; Binsker et al., 2022). Consequently, colistin has been designated by the World Health Organization (WHO) as a highest priority critically important antimicrobial for human medicine (World Health Organization, 2019). Due to the critical importance of colistin as an antibiotic of last resort, colistin resistance among Gram-negative pathogens represents an increasingly dire global public health threat (El-Sayed Ahmed et al., 2020; World Health Organization, 2021; Binsker et al., 2022).
The binding of colistin to bacterial cells is initiated by an electrostatic attraction between the colistin cationic head group and the anionic phosphate group on the lipid A of the bacterial lipopolysaccharide (LPS), which displaces Ca2+ and Mg2+ ions (Olaitan et al., 2014; El-Sayed Ahmed et al., 2020). Subsequently, colistin’s hydrophobic tail integration in the lipid bilayers leads to cell membrane disruption and cell death by targeting cytoplasmic membrane LPS (Trimble et al., 2016; Sabnis et al., 2021) (Figure 1A). Multiple colistin resistance mechanisms have been reported, including (i) modification of lipid A (Guo et al., 1997; Gunn et al., 1998); (ii) expression of a multi-drug efflux system in Pseudomonas aeruginosa (Abuoun et al., 2017); (iii) the complete loss of LPS in Acinetobacter baumannii (Moffatt et al., 2010); (iv) overproduction of capsular polysaccharide in Klebsiella pneumoniae (Campos et al., 2004); and (v) enzymatic degradation of colistin via colistin-degrading proteases in Stenotrophomonas maltophilia (Ito-Kagawa and Koyama, 1980; Lee et al., 2022). The main mechanism of bacterial colistin resistance is the modification of lipid A via the addition of a cationic group, such as phosphoethanolamine (pEtN), 4-amino-4-deoxy-L-arabinose, and glycine to lipid A (Trimble et al., 2016), which reduces the overall membrane negative charge and subsequently decreases colistin binding affinity to the cell (Olaitan et al., 2014; El-Sayed Ahmed et al., 2020). While it has been shown that lipid A is essential for growth in most bacterial species, lipid A modifications are dispensable for cell survival under laboratory growth conditions (Trent, 2004; Raetz et al., 2007; Parsons and Rock, 2013). However, lipid A modification plays a significant role in bacterial adaptation to different stress conditions, including mild acid stress, change in oxygen level, increase in growth temperature, pH change, osmotic stress, and the presence of cationic antimicrobial compounds (Trent, 2004; Raetz et al., 2007; Gunn, 2008; Anandan and Vrielink, 2020a; Troudi et al., 2021).
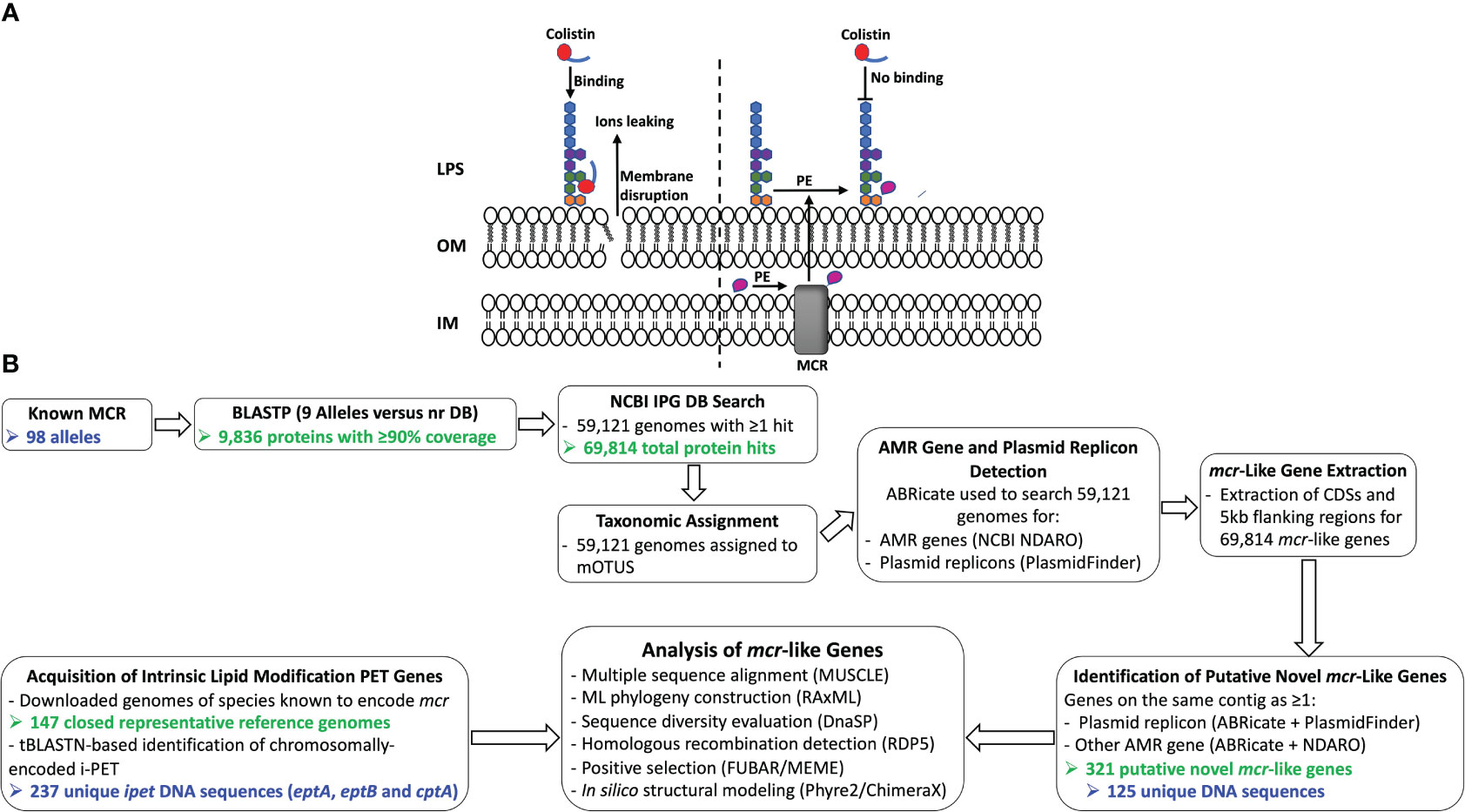
Figure 1 (A) Schematic diagram of colistin’s mode of action and resistance mechanism. Colistin, a cationic antimicrobial peptide, binds to the negatively charged lipid A, displacing membrane-bound cations and disrupting membrane integrity by inserting the hydrophobic tail into the membrane’s lipid (panel A, left). Lipid A modification neutralizes the negative membrane charge, thus reducing colistin binding and cell susceptibility to colistin (panel A, right). IM, inner membrane; LPS, lipopolysaccharide; MCR, mobilized colistin resistance protein; OM, outer membrane; PE, phosphoethanolamine. (B) Schematic outline of the main methodologies used to acquire and characterize MCR and MCR-like proteins from publicly available whole-genome sequencing (WGS) data (see the Materials and Methods section for details). The number of MCR, putative novel MCR-like, and/or intrinsic lipid modification phosphoethanolamine transferase (i-PET) proteins produced at relevant steps are shown in green text; the number of such genes/proteins used in final analyses are shown in blue text. AMR, antimicrobial resistance; BLASTP, protein basic local alignment search tool; CDSs, coding sequences; DB, database; IPG, Identical Protein Group; LPS, lipopolysaccharide; MCR, mobilized colistin resistance amino acid sequences; ML, maximum likelihood; mOTUs, marker gene-based operational taxonomic units; NCBI, National Center for Biotechnology Information; NDARO, National Database of Antibiotic Resistant Organisms; nr, non-redundant protein sequence; (i) -PET, (intrinsic) phosphoethanolamine transferases; tBLASTN, translated nucleotide basic local alignment search tool.
Some Gram-negative bacteria (e.g., Neisseria, Serratia, Brucella, Burkholderia spp.) showcase intrinsic resistance to colistin; others, including pathogenic Enterobacteriaceae, can acquire resistance via numerous mechanisms, including chromosomal mutations (e.g., those that modify the bacterial cell surface) and/or mobilized colistin resistance (mcr) genes (El-Sayed Ahmed et al., 2020; World Health Organization, 2021; Binsker et al., 2022). mcr genes represent a particularly notable and concerning mechanism of colistin resistance, as they are predominantly plasmid-borne and/or associated with transposon insertional elements and can facilitate the acquisition and rapid dissemination of colistin resistance (Kieffer et al., 2017; Trebosc et al., 2019; El-Sayed Ahmed et al., 2020). mcr encodes an inner membrane-anchored protein that belongs to a complex group of phosphoethanolamine transferase (PET) enzymes (Gao et al., 2016), which add phosphoethanolamine (pEtN) to the 4’ phosphate group of lipid A (Cox et al., 2003; Reynolds et al., 2005; Tamayo et al., 2005b; Raetz et al., 2007; Parsons and Rock, 2013; Anandan et al., 2017). Gram-negative bacterial species possess one or more chromosomal PET genes, which function in intrinsic lipid modification, including EptA, EptB, and CptA (Anandan and Vrielink, 2020a). For example, Escherichia coli str. K-12 substr. MG1655 harbors homologs of EptA, EptB, and CptA (Reynolds et al., 2005; Zhao et al., 2019; Anandan and Vrielink, 2020b). We will collectively refer to eptA, eptB, and cptA genes as ipet (intrinsic lipid modification PET) genes and their products as i-PET enzymes or proteins. Lipid A modifying i-PET enzymes phylogenetically cluster into three distinct groups that correlate with the enzyme specificity to the pEtN-acceptor substrate site on the LPS. More specifically, (i) EptA adds pEtN to the N-acetylglucosamine moiety of lipid A, (ii) EptB adds pEtN to the 3-deoxy-d-manno-octulosonic acid (KDO) of the LPS core region, and (iii) CptA/EptC adds pEtN to the second heptose of the LPS core region (Harper et al., 2017; Anandan and Vrielink, 2020a).
Interestingly, MCR proteins share a high degree of sequence and structural similarity with i-PET enzymes present in many Gram-negative bacteria (Bakovic et al., 2007; Anandan and Vrielink, 2020a; Samantha and Vrielink, 2020), including pathogenic members of Enterobacteriaceae (El-Sayed Ahmed et al., 2020; Gogry et al., 2021). PET protein structure includes two discretely folded domains connected by a bridging helix and extended loop, an N-terminal transmembrane domain, and a C-terminal periplasmic soluble catalytic domain (Anandan et al., 2017; Anandan and Vrielink, 2020a). Moreover, PET proteins are metalloenzymes that share a conserved zinc-binding site tetrahedrally coordinated by the side chains of conserved Glu246, Thr285, His466, and Asp465 residues (numbers corresponding to the MCR-1 sequence), with the Thr285 residue acting as the catalytic nucleophile for the pEtN transfer (Stojanoski et al., 2016; Anandan et al., 2017). While multiple conserved or partially conserved amino acid (AA) residues were suggested to be involved in pEtN binding (Sun et al., 2017; Dortet et al., 2018; Stogios et al., 2018; Carroll et al., 2019; Garcia-Menino et al., 2020), the lipid-binding pocket remains poorly defined (Carroll et al., 2019).
As of April 2022, ten major groups of MCR proteins have been defined based on their AA identities/similarities (termed MCR-1 to -10, which are encoded by mcr genes mcr-1 to -10, respectively) (Liu et al., 2016; Xavier et al., 2016; Abuoun et al., 2017; Borowiak et al., 2017; Carattoli et al., 2017; Yin et al., 2017; Wang et al., 2018b; Yang et al., 2018; Carroll et al., 2019; Wang et al., 2020). Here, we will refer to these major groups as “mcr families” or “MCR families” (for nucleotide sequences/genes and amino acid sequences/proteins, respectively), as has been proposed previously (Partridge et al., 2018). Within MCR families, AA sequence identities and similarities can vary. Here, we will use the term “mcr allele” or “MCR allele” to denote specific variants of genes and proteins within a given MCR family, respectively (e.g., alleles within the mcr-3 family are denoted as mcr-3.1, mcr-3.2, mcr-3.3, and so on, and their products as MCR-3.1, MCR-3.2, MCR-3.3, and so on, respectively) (Partridge et al., 2018). In total, >95 MCR alleles have been described as of April 2022.
While MCR proteins share a conserved overall structure (Stojanoski et al., 2016; Sun et al., 2017; Xu et al., 2018a; Carroll et al., 2019; Son et al., 2019), the genes that encode them vary in terms of sequence similarity (Li et al., 2018; Carroll et al., 2019; Ramaloko and Osei Sekyere, 2022). Moreover, it has been suggested that mcr alleles can vary in terms of the colistin resistance levels they confer (Nang et al., 2019; El-Sayed Ahmed et al., 2020). However, the molecular bases of mcr genetic and phenotypic heterogeneities are poorly understood. Furthermore, it has been suggested that mcr evolved from an eptA chromosomal gene copy via mobilization and that Moraxella spp. are a potential source for MCR-like colistin resistance determinants (Kieffer et al., 2017). Indeed, several lines of evidence support the notion that mcr evolved from eptA through mobilization, including: (i) MCR and EptA share a conserved overall protein structure and acceptor substrate specificity, (ii) ISApl1-dependent mobilization of mcr has been demonstrated (Abuoun et al., 2017; Kieffer et al., 2017), and (iii) insertion of ISApl1 upstream of eptA increased eptA expression and led to an increased level of colistin resistance in XDR Acinetobacter baumannii isolates (Trebosc et al., 2019). However, the exact ancestor of mcr and the molecular mechanism of evolution of intrinsic lipid modification eptA to mobilized colistin-resistant determinant remain unknown.
Here, we aim to provide further insight into the evolutionary relationships between mcr families, all within the context of ipet. We show that some ipet genes (e.g., eptA) may differ from canonical mcr in terms of their genomic context, and we identify differentially conserved residues, which may play a role in the levels of colistin resistance conferred by different mcr alleles. Finally, using a large number (>69,000) of MCR and MCR-like proteins extracted from publicly available bacterial genomes, we identify 125 putative novel mcr-like genes (representing 13 clusters at an 80% AA identity threshold), which may be co-harbored on plasmids with other antimicrobial resistance (AMR) genes. Overall, the results presented here provide insight into the evolution and diversity of mcr and ipet genes.
2 Materials and methods
2.1 Acquisition of MCR and MCR-like amino acid sequences
A representative amino acid (AA) sequence from each published MCR family (i.e., at the time of the search, MCR-1 to -9, accessed April 23, 2019; Supplementary Table S1) was queried against the National Center for Biotechnology Information (NCBI) non-redundant (nr) protein database using the protein BLAST (BLASTP) webserver (https://blast.ncbi.nlm.nih.gov/Blast.cgi?PAGE=Proteins; accessed April 23-24, 2019) (Johnson et al., 2008). BLASTP default settings were used for all parameters except max target sequences, which was raised to 5,000. BLASTP hits corresponding to proteins with high query coverage (i.e., ≥90% of the length of the original MCR query) were maintained in subsequent steps (n = 41,270 of 45,001 total hits). Because MCR families can share low degrees of AA identity (e.g., < 40%) (Partridge et al., 2018), no additional sequence identity thresholds were employed so that potentially novel, remote MCR homologs could be identified. From all 41,270 BLASTP hits, the union of all nr protein accession numbers was taken, yielding a total of 9,866 nr protein accession numbers associated with MCR and MCR-like proteins. The AA sequences of all 9,866 MCR and MCR-like proteins, as well as all associated NCBI Identical Protein Group (IPG) accession numbers, were downloaded using the rentrez package version 1.2.1 (Winter, 2017) in R version 3.5.3 (R Core Team, 2021). Finally, known MCR families are similar in terms of sequence length (e.g., the representatives of MCR-1 to -9 listed in Supplementary Table S1 ranged from 538-565 AA in length, with an average/median length of 545/541 AA). We thus removed BLASTP hits that represented extreme outliers based on sequence length as follows: the median absolute deviation of the lengths of the AA sequences of all MCR and MCR-like proteins was calculated, and AA sequences of MCR-like proteins falling outside the range of sequence lengths encompassed by 15 times the median absolute deviation were removed (with 30 proteins removed by this step).
Overall, this initial BLASTP search yielded a total of 9,836 MCR and MCR-like AA sequences, which were used in subsequent steps (Supplementary Table S2). While this search did not specifically query MCR families and alleles described after the access date (i.e., April 23, 2019), the selected BLASTP parameters allowed us to detect MCR families and alleles, which were in NCBI’s nr database but were not yet discovered and published. For example, MCR-10 was not specifically queried in the initial BLASTP search (Supplementary Table S1), as it had not yet been discovered (Wang et al., 2020). However, the approach described above identified MCR-10.1 in NCBI’s nr database prior to its discovery, and MCR-10.1 was thus included among the 9,836 MCR and MCR-like proteins used in subsequent steps (NCBI Protein Accession WP_023332837.1; Supplementary Table S2).
2.2 Acquisition and characterization of genomes harboring MCR-like proteins
NCBI RefSeq Assembly accession numbers for all genomes associated with ≥1 MCR or MCR-like protein in NCBI’s IPG database were acquired via rentrez (see section “Acquisition of MCR and MCR-like amino acid sequences” above). Latest assembly versions for all RefSeq genomes were then downloaded via NCBI’s FTP site (n = 59,129 total genomes; accessed May 19, 2020). To confirm that ≥1 MCR or MCR-like protein could be detected in each genome, the BLASTX algorithm implemented in DIAMOND version 0.9.13.114 (Buchfink et al., 2015) was used to perform a translated search of each genome against AA sequences of all 9,836 MCR and MCR-like proteins identified as described above, plus the AA sequences of all 53 known MCR alleles available in ResFinder at the time (so that proteins that most closely resembled a known MCR allele would be labeled as such; https://cge.cbs.dtu.dk/services/ResFinder/, see section “Acquisition of MCR and MCR-like amino acid sequences” above) (Zankari et al., 2012). The following DIAMOND BLASTX parameters were used to confirm the presence of MCR and/or MCR-like proteins: a maximum E-value threshold of 1e-5 (-e 0.00001), a minimum subject coverage threshold of 90% (–subject-cover 90), a minimum percent AA identity of 90% (–id 90). This resulted in a total of 69,814 confirmed hits of MCR and MCR-like proteins in 59,121 RefSeq genomes, which were used in subsequent steps (Supplementary Table S2).
To assign each genome to a species using a standardized taxonomic framework, all 59,121 genomes were assigned to a marker-gene based operational taxonomic unit (mOTU) using classify-genomes (accessed June 3, 2020; https://github.com/AlessioMilanese/classify-genomes) and version 2.5 of the mOTUs taxonomy (Milanese et al., 2019) (Supplementary Table S2). Antimicrobial resistance (AMR) genes were detected in each genome via the nucleotide BLAST-based approach implemented in ABRicate version 0.9.8 (https://github.com/tseemann/abricate), using the NCBI National Database of Antibiotic Resistant Organisms (NDARO; accessed April 19, 2020) (Feldgarden et al., 2019). ABRicate was additionally used to detect plasmid replicons present in the PlasmidFinder database (Carattoli et al., 2014) in each genome (accessed April 19, 2020). AMR genes and plasmid replicons were considered to be present in a genome using minimum nucleotide identity and coverage thresholds of 80% each (Supplementary Table S2). PlasFlow version 1.1.0 (Krawczyk et al., 2018) was additionally used to predict whether contigs were plasmid-associated or chromosomal in origin (using default settings; Supplementary Table S2).
2.3 Identification of putative novel mcr-like genes
Of the 69,814 MCR and MCR-like proteins identified in 59,121 genomes from NCBI’s RefSeq database (see section “Acquisition and characterization of genomes harboring MCR-like proteins” above), we identified 321 MCR-like proteins, which were located on the same contig as (i) ≥1 plasmid replicon (detected via ABRicate and the PlasmidFinder database) and (ii) ≥1 additional AMR gene (detected via ABRicate and the NDARO), with contigs harboring previously described mcr alleles excluded (see section “Acquisition and characterization of genomes harboring MCR-like proteins” above for details regarding plasmid replicon and AMR gene detection; Supplementary Table S2). These criteria were used to identify and prioritize putative novel MCR-like proteins, as (i) the presence of a plasmid replicon on the same contig as an MCR-like protein indicated that the MCR-like protein and plasmid replicon were likely to be in close proximity (thus predicting that the gene was on a plasmid, rather than a chromosome); and (ii) the presence of other AMR genes on the same contig as an MCR-like protein indicated that the MCR-like protein may have been harbored on a plasmid that was potentially associated with multidrug-resistance. These 321 MCR-like proteins represented 125 unique nucleotide sequences, which we will refer to hereafter as “putative novel mcr-like genes” and their products as “putative novel MCR-like proteins” (Supplementary Table S3).
2.4 Acquisition of chromosomally encoded intrinsic lipid modification PET genes
Closed representative genome sequences of 147 reference species known to harbor mcr genes were downloaded from the NCBI RefSeq Assembly database (accessed March 12, 2021). These included Enterobacteriaceae (NCBI txid543), Proteus (NCBI txid583), Aeromonas (NCBI txid642), and Moraxella (NCBI txid475) species (Supplementary Table S4). Assembled genomes were imported into Geneious version 2019.2.3 (Biomatters, Auckland, New Zealand), and sequences of complete closed chromosomes were extracted for subsequent analysis. AA sequences of E. coli str. K-12 substr. MG1655 CptA (NCBI Protein Accession WP_000556306.1), EptA (NCBI Protein Accession WP_000919792.1), and EptB (NCBI Protein Accession WP_001269197.1) were queried against the closed chromosome sequences using translated nucleotide BLAST (tBLASTN, as implemented in Geneious version 2019.2.3). A total of 237 unique hits that showed ≥90% query coverage were selected to represent chromosomally encoded intrinsic lipid modification PET genes (Supplementary Table S5). We will collectively refer to eptA, eptB, and cptA intrinsic lipid modification PET-encoding genes as ipet genes and their products as i-PET enzymes or proteins.
2.5 Construction of maximum likelihood phylogeny of mcr and mcr-like genes
Maximum likelihood (ML) phylogenies were inferred from the nucleotide sequences of (i) all mcr alleles available in NCBI’s NDARO (n = 98, accessed March 12, 2021; Supplementary Table S6), (ii) putative novel mcr-like genes identified in this study (n = 125; see section “Identification of putative novel mcr-like genes” above) and/or (iii) ipet (n = 237) coding sequences (see section “Acquisition of chromosomally encoded intrinsic lipid modification PET genes” above; Supplementary Table S7). Back-translated nucleotide multiple sequence alignments (NTbtn-MSA) were constructed using MUSCLE (Edgar, 2004) with the default settings in Geneious version 2019.2.3. The resulting NTbtn-MSAs were used to construct ML phylogenies with 100 bootstrap replicates via RAxML, using the GTRGAMMA nucleotide substitution model and default settings (RAxML GUI version 2.0.7 and RAxML version 8.2.12) (Stamatakis, 2014). The resulting trees were visualized and edited using iTOL version 6.5 (https://itol.embl.de/) (Letunic and Bork, 2007).
2.6 Clustering of PET amino acid sequences
AA sequences of the MCR, putative novel MCR-like, and i-PET proteins (n = 98, 125, and 237 proteins, respectively; Supplementary Table S7) were clustered into families using CD-HIT version 4.8.1 (Li and Godzik, 2006; Fu et al., 2012) with an 80% AA sequence identity threshold (-c 0.8) and a word length of 5 (-n 5; i.e., the value suggested by CD-HIT for the given AA sequence identity threshold). CD-HIT clusters that did not contain a known MCR allele at the 80% AA sequence identity threshold were treated as putative novel MCR families, as 80% AA identity represents a conservative threshold for MCR family delineation (e.g., at this threshold, MCR-1, MCR-2, and MCR-6 clustered together and would be considered to be members of the same MCR family) (Partridge et al., 2018).
2.7 Descriptive sequence analysis
The number of polymorphic sites, nucleotide diversity per site, average pairwise nucleotide differences per sequence, number of synonymous substitutions (S) and non-synonymous substitutions (N), and the dN/dS ratio for each NTbtn-MSA were calculated using DnaSP version 6.12.03 (Supplementary Table S7; see section “Construction of maximum likelihood phylogeny of mcr and mcr-like genes” above) (Rozas et al., 2017).
2.8 Homologous recombination detection
The Recombination Detection Program (RDP5) was used to detect homologous recombination events within the NTbtn-MSA of mcr, putative novel mcr-like, and ipet genes (n = 98, 125, and 237 genes, respectively; Supplementary Table S7) (Martin et al., 2021). Seven homologous recombination detection methods (RDP, BOOTSCAN, GENECON V, MAXCHI, CHIMAERA, SISCAN, and 3Seq) were used to perform a full exploratory recombination analysis using the default settings in RDP5. Only recombination events detected by at least three methods were selected for further analysis to reduce false positives. Recombination events with similar breakpoints were merged into a single event, and the overall significance of the recombination evidence for each event was evaluated via the Pairwise Homoplasy Index (PHI) test with default settings in RDP5.
2.9 Positive selection analysis
The Datamonkey server (https://www.datamonkey.org/) (Weaver et al., 2018) was used to identify mcr residues that evolved under positive selection. The “hyphy cln” command within HyPhy version 2.5.32(MP) (Kosakovsky Pond et al., 2020) was used to remove stop codons within the NTbtn-MSA of the 98 known mcr alleles (see section “Construction of maximum likelihood phylogeny of mcr and mcr-like genes” above; Supplementary Table S6). The resulting cleaned alignment was supplied as input to the command line implementation of GARD (Kosakovsky Pond et al., 2006; Weaver et al., 2018; Kosakovsky Pond et al., 2020), which was used to detect recombination breakpoints within the alignment using default settings. GARD partitioned the dataset into fragments of 1-1,204 bp and 1,205-1,779 bp.
The resulting partitioned dataset produced by GARD (with suffix ".best.gard") was supplied as input to the following (both accessed January 27, 2022): (i) FUBAR (Fast, Unconstrained Bayesian AppRoximation; https://www.datamonkey.org/fubar), which uses a Bayesian approach to infer non-synonymous (dN) and synonymous (dS) substitution rates on a per-site basis and assumes constant selection pressure for each site along the entire phylogeny (Murrell et al., 2013); (ii) MEME (mixed-effect model evolution-based positive selection analysis; https://www.datamonkey.org/meme), which tests the hypothesis that individual sites have been subjected to episodic positive selection (Murrell et al., 2012). For both FUBAR and MEME, the universal genetic code option was selected; for FUBAR, additional parameters under “advanced options” were set to their default values.
2.10 In silico structural modeling and construction of sequence logos
Structural modeling of MCR-1 was done using the Phyre2 server (accessed June 20, 2021) (Kelley et al., 2015) based on Neisseria meningitidis lipooligosaccharide phosphoethanolamine transferase EptA (Anandan et al., 2017). The protein structure was viewed and annotated using UCSF ChimeraX version 1.3 (Pettersen et al., 2021). Residues predicted to have evolved under positive selection were mapped on the MCR-1 protein 3D structural model using UCSF ChimeraX (Pettersen et al., 2021).
An NTbtn-MSA and encoded AA-based MSA of the 98 known mcr alleles were constructed using MUSCLE (Edgar, 2004) with default settings in Geneious version 2019.2.3. The ESPript 3 server version 3.0.8 was used to align MCR-1 secondary structure elements onto the AA MSA of the 98 known MCR alleles (Robert and Gouet, 2014). Residues under negative or positive selection were mapped onto the AA MSA of the 98 known MCR alleles in Geneious version 2019.2.3. The 19 AA residues and codons (57 bases) identified by MEME were extracted from the corresponding MSA across the 98 mcr alleles in Geneious and saved as separate MSA FASTA files. The resulting MSA FASTA files were used to construct AA and nucleotide graphical representation logos via the WebLogo3 server (accessed May 26, 2022) (Crooks et al., 2004).
An AA-based MSA of MCR, putative novel MCR-like, and i-PET proteins (n = 98, 125, and 237 proteins, respectively; Supplementary Table S7) was constructed using MUSCLE with default settings in Geneious version 2019.2.3 (Edgar, 2004). The ESPript 3 server version 3.0.8 was used to align EptA secondary structure elements onto the MSA (Robert and Gouet, 2014). The resulting MSA was used to visually identify cysteine residues involved in disulfide bond formation across the different proteins based on the experimentally identified disulfide bonds in the EptA structure (Anandan et al., 2017) and site-directed mutagenesis analyses of the corresponding sites in CptA (Zhao et al., 2019).
2.11 Characterization of the genomic context of PET-encoding genes
To characterize the genomic context surrounding different PET-encoding genes, genomic regions 5 Kb upstream and downstream of all PET-encoding genes were extracted from each PET-harboring genome (i.e., all genomes in which MCR and MCR-like proteins were detected, and all i-PET-harboring genomes used in this study; Supplementary Tables S2, S4, respectively). NCBI Prokaryotic Genome Annotation Pipeline (PGAP) annotations (Tatusova et al., 2016) were used to identify genes directly upstream and downstream of PET-encoding genes (i.e., not separated from PET-encoding genes by another ORF). Sequences were visually examined, and genes were considered to be in the same operon if they were located within approximately < 20 nucleotides and had no apparent promoter-like sequence in this intergenic region.
2.12 Collection of phenotypic colistin resistance data
Colistin minimum inhibitory concentration (MIC) data were collected from the literature (Supplementary Table S8) (Di Pilato et al., 2016; Liu et al., 2016; Xavier et al., 2016; Abuoun et al., 2017; Borowiak et al., 2017; Carattoli et al., 2017; Ling et al., 2017; Liu et al., 2017; Lu et al., 2017; Poirel et al., 2017; Tijet et al., 2017; Yang et al., 2017; Yin et al., 2017; Zhao et al., 2017; Alba et al., 2018; Carattoli et al., 2018; Chavda et al., 2018; Dortet et al., 2018; Duggett et al., 2018; Eichhorn et al., 2018; Fernandes et al., 2018; Garcia-Graells et al., 2018; Hammerl et al., 2018; Kieffer et al., 2018; Li et al., 2018; Liu et al., 2018; Poirel et al., 2018; Rebelo et al., 2018; Shen et al., 2018; Teo et al., 2018; Wang et al., 2018b; Wang et al., 2018c; Wise et al., 2018; Xiang et al., 2018; Xu et al., 2018b; Xu et al., 2018c; Yang et al., 2018; Abdul Momin et al., 2019; Bitar et al., 2019; Chavda et al., 2019; Cui et al., 2019; Deshpande et al., 2019; Hadjadj et al., 2019; Li et al., 2019; Long et al., 2019; Wang et al., 2019; Yang et al., 2019; Yuan et al., 2019; Zhang et al., 2019; Zheng et al., 2019; Bitar et al., 2020; Cha et al., 2020; Fan et al., 2020; Garcia-Menino et al., 2020; Hatrongjit et al., 2020; Lei et al., 2020; Martins-Sorenson et al., 2020; Neumann et al., 2020; Ngbede et al., 2020; Wang et al., 2020; Anyanwu et al., 2021; Hu et al., 2021; Khanawapee et al., 2021; Leangapichart et al., 2021; Snyman et al., 2021; Stosic et al., 2021; Uddin et al., 2021; Yu et al., 2021). A search of NCBI’s PubMed database (https://pubmed.ncbi.nlm.nih.gov/) was conducted on August 13, 2021, using keywords [mcr and colistin resistance]. The search results were exported to an EndNote library (EndNote 20, Clarivate, Philadelphia, USA). A similar search was performed on the Web of Science Core Collection database (Clarivate, Philadelphia, USA), and the search results were used to amend the EndNote library. Duplicate entries in the EndNote library were deleted, and full-text PDF files were downloaded using EndNote “Find Full Text”. When needed, Supplementary Materials were downloaded and incorporated into the PDF file.
The EndNote library was searched for each known mcr allele using the allele designation (e.g., mcr-3.3) with the “Any Field + PDF with Notes” search criteria. The search results were sorted by date to identify the primary publication(s) describing the allele for the first time. The full-text articles were visually scanned to identify the publication(s) describing (i) the identification of the allele and (ii) the functional characterization of colistin resistance. The “Materials and Methods” and “Results” sections that described colistin resistance phenotypic data were examined carefully, and colistin MIC data were collected. We opted to use MIC values determined by the broth microdilution method, which is recommended by the European Committee on Antimicrobial Susceptibility Testing (EUCAST) (Jayol et al., 2018). We excluded MIC data determined by agar dilution and gradient agar disk diffusion (colistin gradient strip zone of inhibition) methods, which have been suggested to be less accurate in determining colistin resistance levels when compared to the broth microdilution method (Chew et al., 2017; Garcia-Menino et al., 2020; Yusuf et al., 2020). If no data were identified in the EndNote library for specific mcr alleles, we performed an additional search using Google Scholar, using the given allele’s designation.
Colistin MIC values reported for native mcr-harboring strains (Supplementary Table S8) were base-2 logarithm-transformed using the log2 function in R and displayed in a heatmap using iTOL version 6. An ML phylogeny of species reported for the native mcr-harboring strains was additionally supplied to iTOL. Briefly, a type strain and/or NCBI species reference genome was downloaded from NCBI’s RefSeq Assembly database for each mcr-harboring native strain species (n = 18 total species, with one genome selected per species; Supplementary Table S8). The “classify_wf” workflow implemented in the Genome Taxonomy Database Toolkit (GTDB-Tk) version 2.1.0 was used to construct an AA MSA of all 18 genomes, as well as to confirm species assignments reported in NCBI (using version R207_v2 of GTDB) (Chaumeil et al., 2019; Parks et al., 2022). The AA MSA produced by GTDB-Tk (i.e., the file named “gtdbtk.bac120.user_msa.fasta”) was supplied as input to IQ-TREE version 1.5.4 (Nguyen et al., 2015), which was used to construct the ML phylogeny, using (i) the optimal AA substitution model selected via Bayesian Information Criteria values produced via ModelFinder (i.e., the “LG+F+R3” model) (Yang, 1995; Le and Gascuel, 2008; Soubrier et al., 2012; Kalyaanamoorthy et al., 2017); and (ii) one thousand replicates of the ultrafast bootstrap approximation (Minh et al., 2013).
3 Results
3.1 MCR-like proteins are distributed across a wide range of Gram-negative bacterial taxa
A protein BLAST (BLASTP)-based search for MCR and MCR-like proteins in NCBI’s nr and IPG databases showed that MCR- and MCR-like proteins were distributed across a wide range of Gram-negative bacterial taxa (Figure 1). More than 69,000 BLASTP hits against known MCR families were detected in whole-genome sequencing (WGS) data spanning 256 bacterial genera, including Escherichia, Shigella, Salmonella, Klebsiella, Aeromonas, Moraxella, Enterobacter, Xanthomonas, and Pseudomonas spp. (per the mOTUs taxonomy; Supplementary Table S2). Among the 69,814 total BLASTP hits to MCR and MCR-like proteins identified here, 15,321 (21.9%) corresponded to proteins annotated as EptA (i.e., the associated protein was annotated in NCBI with the term “EptA” or “eptA”; Supplementary Table S2). Mobilization is a hallmark of mcr, and insertion sequence (IS) elements have been found to flank some mcr alleles; thus, we also searched for transposon-related elements (e.g., IS elements or transposase genes) within the 5 Kb flanking regions of the 69,814 mcr and mcr-like genes identified here (Supplementary Table S2). Overall, we found that 1,097 of the 69,814 mcr and mcr-like genes possessed transposon-related elements within their 5 Kb flanking regions (based on NCBI Prokaryotic Genome Annotation Pipeline [PGAP] annotations within these regions, accessed May 19-21, 2020; Supplementary Table S2) (Tatusova et al., 2016). It has been reported that multiple mcr alleles have lost one or both IS elements (Snesrud et al., 2017; Snesrud et al., 2018; Wang et al., 2018a; El-Sayed Ahmed et al., 2020). Thus, we did not include the presence of IS elements as a criterium for the identification of mcr and putative novel mcr-like genes in this study.
As mcr genes are typically plasmid-borne, while intrinsic lipid modification PET-encoding genes (ipet genes) are typically chromosomally encoded, we hypothesized that mcr-like genes detected on the same contig as a plasmid replicon and other antimicrobial resistance (AMR) genes were more likely to encode PET associated with colistin resistance. Initially, we identified 321 BLASTP hits to MCR proteins, which met these criteria (defined here as “putative novel MCR-like proteins” and their encoding genes designated as “putative novel mcr-like genes”; Figure 1B and Supplementary Table S3). Sequence similarities showed that the 321 putative novel mcr-like genes represented 125 unique nucleotide sequences, which were selected for further analyses (Figure 1B and Supplementary Tables S3, S7). To further analyze these genes, we created a back-translated nucleotide multiple sequence alignment (NTbtn-MSA) of the 125 unique, putative novel mcr-like genes identified here, along with 98 known mcr alleles (Supplementary Tables S3, S6). These 223 sequences showed an average pairwise nucleotide diversity per site (p) of 0.42675, with an average number of pairwise nucleotide differences per sequence (k) of 623.052 over 1,776 sites. The relatively high p value observed here was not surprising, as it has been shown previously that mcr families encompass an extensive degree of genetic diversity (Khedher et al., 2020); this is also evident within an NTbtn-MSA constructed using the 98 known mcr alleles alone (i.e., p = 0.3614 and k = 553.669 over 1,776 sites; Supplementary Table S6).
A maximum likelihood (ML) phylogeny inferred from the NTbtn-MSA of known mcr alleles and putative novel mcr-like genes showed a robust separation of clades and subclades representing different mcr families (Figure 2 and Supplementary Figure S1). Specifically, mcr alleles clustered into two distinct phylogenetic lineages: (i) lineage A included alleles belonging to the mcr-1, mcr-2, mcr-5, and mcr-6 families, and (ii) lineage B included all remaining mcr alleles (Figure 2 and Supplementary Figure S1). Interestingly, both clades contained putative novel mcr-like genes, with 21 and 103 putative novel mcr-like genes clustering into lineage A and B, respectively (Figure 2 and Supplementary Figure S1). While some putative novel mcr-like genes clustered with known mcr families (i.e., three genes clustered with mcr-7.1 and two genes clustered with mcr-5; Supplementary Figure S1), the majority of novel mcr-like genes grouped into two large phylogenetic clades that were distinct from known mcr families (Figure 2 and Supplementary Figure S1).
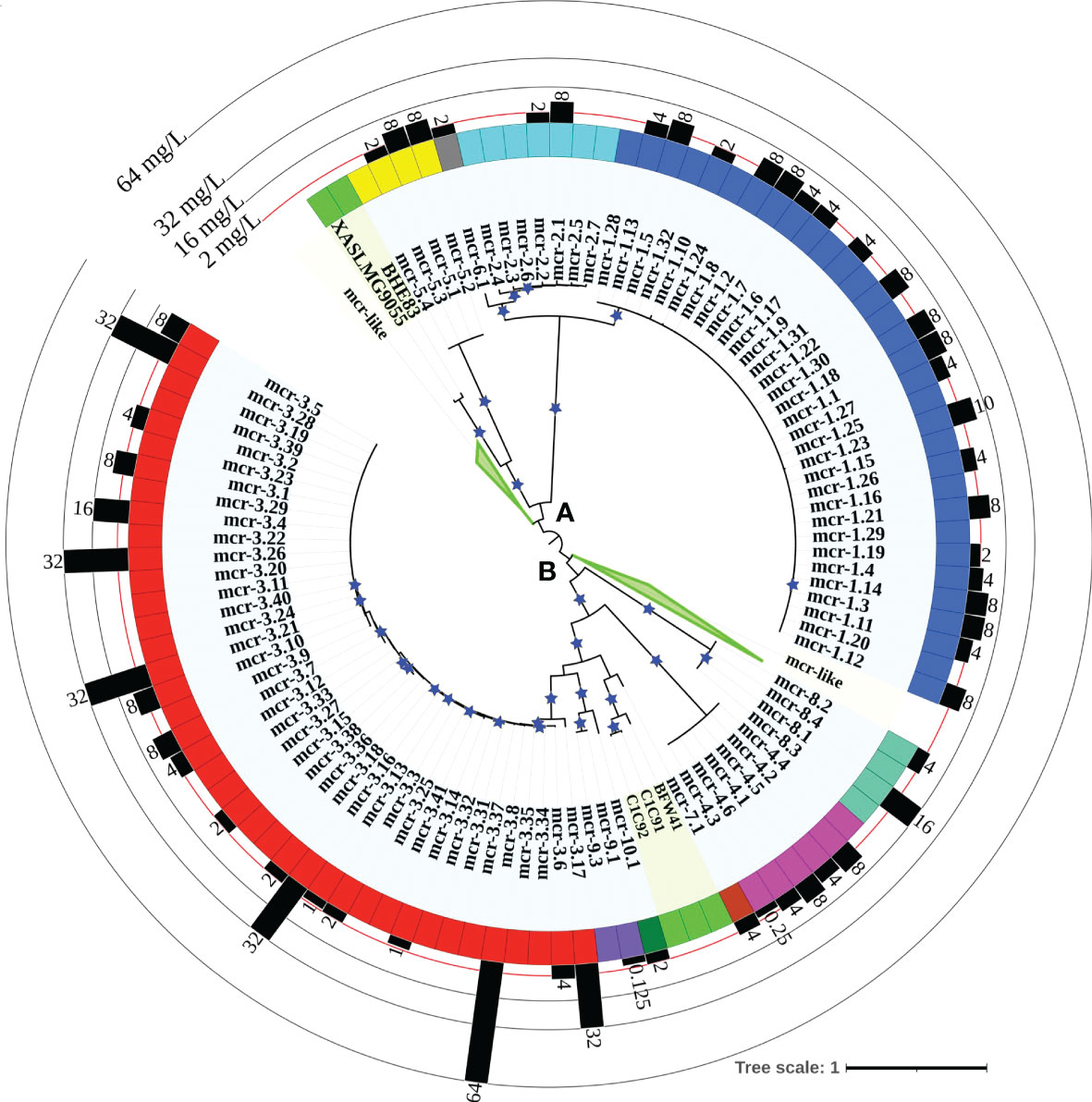
Figure 2 Maximum likelihood (ML) phylogeny inferred from a nucleotide back-translation-based multiple sequence alignment (NTbtn-MSA) of (i) 98 mcr alleles and (ii) 125 unique sequences of mcr-like genes located on the same contig as ≥1 plasmid replicon and ≥1 other antimicrobial resistance (AMR) gene (i.e., “putative novel mcr-like genes”). Sequences were aligned using MUSCLE. The ML phylogeny was constructed with RAxML, using the GTRGAMMA nucleotide substitution model and 100 bootstrap replicates. The tree was edited using the iTOL web server (https://itol.embl.de/) and rooted at the midpoint, with branch lengths reported in substitutions per site. Branches with bootstrap values ≥70% are denoted by blue stars. Clades exclusively composed of putative novel mcr-like genes were collapsed for clarity (green branches; see Supplementary Figure S1 for a fully expanded tree). Tip label shading corresponds to known mcr alleles (light blue) and putative novel mcr-like genes identified in this study (light green). The colors of the inner ring represent different mcr families (mcr-1 to -10). The outer graph represents the maximum reported colistin minimum inhibitory concentration (MIC) values of native strains harboring different mcr alleles. The colistin breakpoint established by the Clinical and Laboratory Standards Institute (CLSI) is 2 mg/L (red line). Isolates with colistin MIC ≥2 mg/L are considered colistin-resistant. Colistin MIC values were compiled from different mcr-harboring Gram-negative species as listed in Supplementary Table S8; metadata associated with each gene can be found in Supplementary Table S6.
3.2 Some mcr alleles are more closely related to eptA than to other mcr alleles
Consistent with the wide range of genetic diversity observed among nucleotide sequences of mcr families, AA sequence similarities among MCR families also varied widely, with similarities ranging from 59.3% to 100% and identities ranging from 29.7% to 99.8% (Supplementary Tables S9, S10). To gain further insight into MCR diversity in the context of intrinsic lipid modification PET (i-PET), we identified and aggregated AA and nucleotide sequences associated with 237 chromosomally encoded i-PET proteins (i.e., EptA, EptB, and CptA; Supplementary Table S5). These i-PET proteins were extracted from 147 genomes of genera, which had been reported to harbor mcr (e.g., Aeromonas, Citrobacter, Cronobacter, Enterobacter, Escherichia, Klebsiella, Moraxella, Proteus, and Salmonella spp.; Supplementary Tables S4, S5) (Nang et al., 2019). The nucleotide and AA sequences of the 237 chromosomally encoded i-PET proteins identified here were compared to those of the 98 known MCR alleles, as well as the sequences of the 125 putative novel MCR-like proteins identified here (n = 460 total PET; Supplementary Table S7). Based on nucleotide sequences, all 460 PET-encoding genes queried here displayed extensive sequence diversity (i.e., p = 0.50571 and k = 450.085; Supplementary Table S7).
Based on AA sequences, MCR alleles shared a relatively low degree of AA similarity with EptB and CptA, ranging from 46% to 58% and 42% to 49%, respectively (Supplementary Tables S9 and S10). Notably, however, MCR alleles differed in terms of the degree of AA similarity they shared with EptA: overall, MCR allele AA similarities to EptA ranged from 61% to 76%, while some MCR alleles shared a higher degree of AA similarity to EptA than to other MCR alleles (Supplementary Tables S9, S10). For example, MCR-8.1 showed average similarities of 72.8 ± 1.1% to EptA and 70 ± 7% to other MCR alleles (Supplementary Tables S9 and S10). Conversely, MCR-1.1 showed average similarities of 64.5 ± 1.5% to EptA and 76.1 ± 18.1% to other MCR alleles (Supplementary Tables S9 and S10). This suggests that sequence similarity alone cannot sufficiently discriminate canonic EptA from MCR.
3.3 Colistin resistance and intrinsic lipid modification PET proteins represent distinct clades, which show some associations with PET functionality
To further characterize the diversity and evolution of PET-encoding genes, we constructed an ML phylogeny using the nucleotide sequences of the 98 known mcr, 125 putative novel mcr-like, and 237 ipet genes identified here (representing a total of 460 PET-encoding nucleotide sequences; monophyletic groups within this phylogeny will be referred to as “lineages”, followed by “clades”, “subclades”, and “clusters”, Supplementary Table S7). The phylogeny of these 460 PET-encoding genes included one singleton gene, as well as (i) lineage I (105 taxa), which included cptA genes, and (ii) lineage II (354 taxa), which included eptA, eptB, mcr, and all 125 putative novel mcr-like genes (Figure 3 and Supplementary Figure S2). Lineage I included clades I-A and I-B, with two large I-B subclades (subclade I-B-1 and I-B-2; Figure 3 and Supplementary Figure S2). Subclade I-B-1 represented bona fide cptA, based on sequence similarity to experimentally confirmed cptA genes (Tamayo et al., 2005a). Subclade I-B-2, on the other hand, represented 71 chromosomally encoded ipet genes, but did not include any experimentally confirmed cptA genes (Figure 3 and Supplementary Figure S2). The fact that subclade I-B-2 clustered with the cptA-containing subclade I-B-1 may, however, indicate that genes in subclade I-B-2 encode enzymes with acceptor substrate specificities similar to, but not identical to, the substrate specificity of CptA. Within lineage II, eptA and eptB clustered into distinct phylogenetic groups (clade II-A and cluster II-B-2g, respectively; Figure 3 and Supplementary Figure S2). Overall, this suggests clustering of PET-encoding genes is correlated with the enzymes’ acceptor substrate specificities, consistent with previous reports (Harper et al., 2017) (Figure 3 and Supplementary Figure S2).
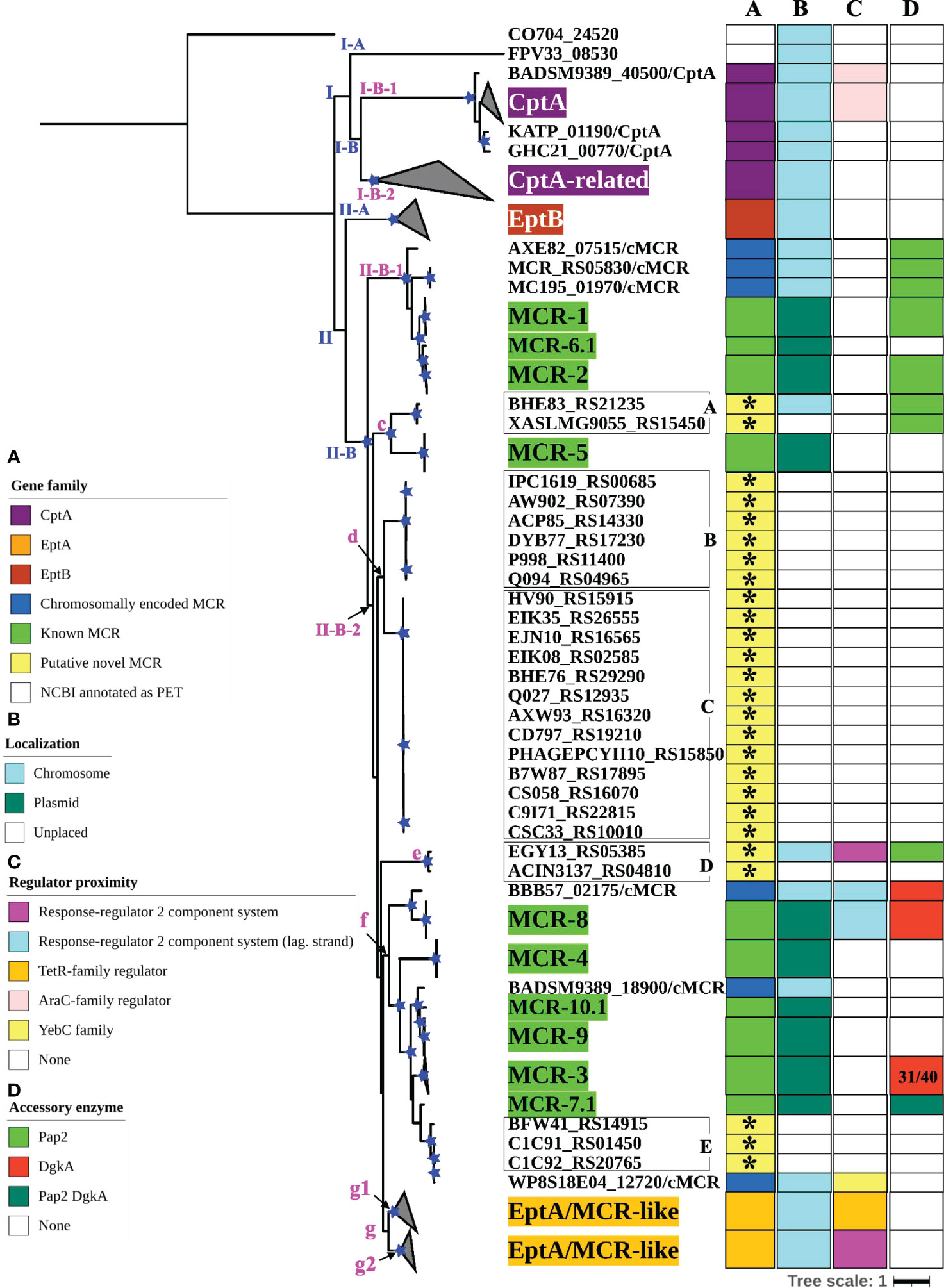
Figure 3 Maximum likelihood (ML) phylogeny inferred from a nucleotide back-translation-based multiple sequence alignment (NTbtn-MSA) of (i) 98 mcr alleles, (ii) 125 unique sequences of mcr-like genes located on the same contig as ≥1 plasmid replicon and ≥1 other antimicrobial resistance (AMR) gene (i.e., “putative novel mcr-like genes”), and (iii) 237 chromosomal phosphoethanolamine transferase (ipet) genes. Sequences were aligned using MUSCLE. The ML phylogeny was constructed with RAxML, using the GTRGAMMA nucleotide substitution model and 100 bootstrap replicates. The tree was edited using the iTOL web server (https://itol.embl.de/) and rooted at the midpoint, with branch lengths reported in substitutions per site. Branches with bootstrap values ≥70% are denoted by blue stars. Linages and clades described in the main text are numbered in blue, while subclades and clusters are numbered in magenta. Clades exclusively composed of genes from the same family were collapsed and color-coded, as shown in the left color legend key (see Supplementary Figure S2 for a fully expanded tree). Five PET families clustered based on an 80% AA identity threshold representing 26 putative novel mcr genes are identified by black boxes around the taxa names with capital letters A to E and asterisks in color strip (A). Color-coded regions in strip (A) denote mcr families, putative novel mcr-like genes, and eptA, eptB, and cptA homologues. Color-coded regions in strip (B) denote gene localization as chromosomally encoded (cyan), plasmid-encoded (green), or unplaced (white); localization was assigned based on the first gene reported in the literature (note that for some gene families, e.g., mcr-3, chromosomally encoded genes have also been reported). Color-coded regions in strip (C) represent the regulatory system juxtaposing phosphoethanolamine transferase (PET)-encoding genes: magenta regions represent genes contained within the same operon as a two-component response sensor-regulatory system; cyan regions represent genes located divergent of a two-component response sensor-regulatory system; orange regions represent genes located adjacent to a TetR-type regulator; pink regions represent genes located adjacent to an AraC-type regulator; yellow region represents gene located adjacent to a YebC-type regulator; white regions represent genes represented by single-gene operons with no upstream or downstream regulatory protein. Color-coded regions in strip (D) represent PET-encoding genes localized adjacent to accessory enzyme-encoding genes, including pap2 encoding lipid A phosphatase (light green), dgkA encoding diacylglycerol kinase (red), or both pap2 and dgkA genes (dark green); white regions represent the absence of accessory enzyme-encoding genes upstream or downstream of PET-encoding genes.
Clade II-B represented subclades II-B-1 and II-B-2; alleles belonging to the mcr-1, mcr-2, and mcr-6 families clustered into subclade II-B-1, while alleles belonging to the remaining mcr families clustered into subclade II-B-2 (along with the eptA genes, which represent cluster II-B-2g; Figure 3 and Supplementary Figure S2). Overall clustering was consistent between this phylogeny (Figure 3) and the phylogeny based only on mcr and mcr-like genes (Figure 2), expect for the placement of the mcr-5 family and two closely related genes (i.e., gene loci BHE83_RS21235 and XASLMG9055_RS15450), which were placed with mcr-1, mcr-2 and mcr-6 in Figure 2 and with mcr-3, mcr-4, mcr-7, mcr-8, mcr-9, and mcr-10 in Figure 3 (consistent with the fact that the mcr-5 branch did not receive strong bootstrap support in either phylogeny; Figures 2, 3). Notably, all 125 putative novel mcr-like genes were grouped into subclade II-B-2; 99 of these genes clustered with eptA genes (cluster II-B-2g) rather than with known mcr alleles (Figure 3), even though these 99 genes were co-localized on a contig with a plasmid replicon and additional AMR gene(s) (Supplementary Tables S2, S3). The remaining 26 putative novel mcr-like genes clustered with known mcr alleles in the ML phylogeny, including (i) two and three genes that clustered with mcr-5 and mcr-7 (clusters II-B-2c and II-B-2f; Figure 3), and (ii) 21 putative novel mcr-like genes that formed two distinct phylogenetic clusters (groups II-B-2-d and II-B-2e; Figure 3). These data suggest that these 26 putative novel mcr-like genes are likely to be previously unidentified mcr genes.
Our phylogeny also provided further evidence that some mcr families and alleles (i.e., mcr-3, mcr-4, mcr-5, mcr-8, and mcr-7.1) are more closely related to eptA than to other mcr alleles (i.e., mcr-1, mcr-2, and mcr-6.1; Figure 3 and Supplementary Figure S2) and that mcr genes and eptA share a single common ancestor. Interestingly, the phylogeny constructed here also showed that chromosomally encoded mcr genes, which have previously been reported (Ling et al., 2017; Dortet et al., 2018; Stogios et al., 2018; Wang et al., 2021), represent four distinct phylogenetic groups. The chromosomally encoded mcr genes of three Moraxella spp. were included in subclade II-B-1 (loci numbers AXE82_07515, MC195_01970, and MCR_RS05830; Figure 3 and Supplementary Figure S2). Chromosomally encoded mcr genes from Kosakonia sacchari (locus number BBB57_02175), Aeromonas caviae (locus number WP8S18E04_12720), and Buttiauxella agrestis (locus number BADSM9389_18900) grouped into clusters II-B-2e, II-B-2f, and II-B-2f, respectively (Figure 3 and Supplementary Figure S2).
3.4 The genomic contexts of mcr genes and eptA are widely diverse
To identify additional criteria that may aid in mcr gene identification, we assessed the genomic context of eptA, eptB, cptA, mcr, and the 125 putative novel mcr-like genes identified here (Figure 3; Supplementary Figure S2 and Supplementary Table S11). Genes in lineage I represented two different genomic contexts: (i) the majority of cptA genes in subclade I-B-1 (n = 26 out of 33) were located 70-200 nucleotides upstream of an AraC-type transcriptional regulator-encoding gene, while (ii) all 71 cptA-related genes in subclade I-B-2 were represented by single gene operons (Figure 3; Supplementary Figure S2 and Supplementary Table S11). In comparison, genes in clade II-A (which represented eptB; n = 66) were always represented by single-gene operons (Figure 3; Supplementary Figure S2 and Supplementary Table S11).
The 99 putative novel mcr-like genes that clustered with eptA into cluster II-B-2g, as well as the 59 chromosomally encoded eptA genes, represented two genomic contexts that partitioned into two distinct phylogenetic subgroups (g1 and g2) within cluster II-B-2g (Figure 3 and Supplementary Figure S2). All genes in the “g1” subgroup (n = 37) were found to be located adjacent to a putative TetR/AcrR family transcriptional regulator (Figure 3; Supplementary Figure S2 and Supplementary Table S11). In contrast, all genes in the “g2” group (n = 121) were located in an operon with a PmrAB-like two-component sensor histidine kinase-response regulator system (Figure 3, Supplementary Figure S2, and Supplementary Table S11). Importantly, the known mcr genes (which were grouped into subclades II-B-1 and II-B-2) showed genomic contexts that were distinct from the genomic context of the ipet genes (detailed in the preceding paragraph); genomic context also differed among the mcr families (Figure 3; Supplementary Figure S2 and Supplementary Table S11). Among the mcr genes in subclade II-B-1, mcr-1 and mcr-2 alleles were located in a putative operon upstream of PAP2 family lipid A phosphatase-encoding pap2 (with some pap2 in proximity to mcr-2 showing evidence for truncation), while mcr-6.1 represented a single gene operon. Interestingly, among the mcr genes in cluster II-B-2f, mcr-7.1 was located 125 nucleotides upstream of an operon that contained pap2, as well as dgkA (which encodes a diacylglycerol kinase), while mcr-3 alleles were located upstream of dgkA. This is of relevance, as it has been shown that pap2 and dgkA play a role in mcr-dependent colistin resistance through recycling and modification of lipid metabolism byproducts (Choi et al., 2020; Gallardo et al., 2020; Purcell et al., 2022). Among the other mcr genes in subclade II-B-2, mcr-8 alleles were located adjacent to, but divergent from, an operon containing a two-component sensor histidine kinase-response regulator, while alleles belonging to the remaining mcr families (mcr-4, mcr-5, mcr-9.1, and mcr-10.1) were single-gene operons (Figure 3; Supplementary Figure S2 and Supplementary Table S11). The genomic context of the 26 putative novel mcr-like genes in clade II-B included the following: (i) the 19 genes in cluster II-B-2d, as well as the three genes in cluster II-B-2f, and the gene ACIN3137_RS04810 in cluster II-B-2e were classified as single gene operons; (ii) the two genes in cluster II-B-2c were located within an operon with a pap2 gene; and (iii) the EGY13_RS05385 gene in cluster II-B-2e was located in an operon with a PmrAB-like two-component sensor histidine kinase-response regulator system and divergent from a pap2 gene (Figure 3; Supplementary Figure S2 and Supplementary Table S11). This suggests that the genomic contexts of the 26 putative novel mcr-like genes in clade II-B are similar to those of known mcr genes.
3.5 Structurally and functionally important disulfide bonds are differentially conserved among different MCR and i-PET families
Sequence analysis of EptA, EptB, CptA, and known MCR proteins showed that five disulfide bonds, which were identified in the EptA structure (Anandan et al., 2017), were differentially conserved among different MCR families (Supplementary Figure S2 and Supplementary Table S7). Previously, structural and mutational analyses of the E. coli EptC, a CptA homolog, revealed that the inability of EptC to confer colistin resistance was due to the lack of several disulfide bonds (Zhao et al., 2019). Zhao et al. (Zhao et al., 2019) suggested that the disulfide bonds function synergistically to restrain the flexibility of different loops, especially a loop near the active site, which forms part of a potential substrate entry tunnel. Our sequence analysis showed that CptA homologs (i.e., lineage I) did not have any predicted disulfide bonds. In contrast, EptA and EptB homologs (cluster II-B-2g and clade II-A, respectively) were predicted to contain five and three disulfide bonds, respectively (Supplementary Figure S2 and Supplementary Table S7). Interestingly, three predicted disulfide bonds were conserved in MCR-1, MCR-2, and MCR-6 alleles (which group into subclade II-B-1), while five predicted disulfide bonds were conserved in alleles belonging to the other MCR families (Supplementary Figure S2 and Supplementary Table S7). However, the role that disulfide bond number variability plays in the functional diversity of MCR alleles remains unknown. All 26 putative novel mcr genes in clade II-B were predicted to have the same five disulfide bonds as EptA (Supplementary Figure S2 and Supplementary Table S7).
3.6 Varying levels of colistin resistance have been reported for different mcr-harboring strains
Through the literature search conducted here, colistin MIC values were obtained for 70 of 98 mcr alleles in their native strains, consistent with reports that MIC data are lacking for multiple mcr alleles in the NCBI Gene Catalog (see Nang, et al. for a recent extensive literature survey of colistin MIC values associated with different bacterial isolates) (Nang et al., 2019). Using data extracted from published studies, we found that markedly diverse colistin MIC levels have been reported for native strains harboring different mcr families, as well as native strains harboring different mcr alleles within the same mcr family (Figures 2, 4 and Supplementary Table S8). For example, different colistin MIC values were reported for native strains harboring mcr-1.10 (MIC=2 mg/L), mcr-1.4 (MIC= 4 mg/L), mcr-1.2 (MIC=8 mg/L), and mcr-1.23 (MIC=10 mg/L; Figure 4 and Supplementary Table S8). Similarly, native strains harboring different mcr-3 alleles showed a wide range of reported colistin MIC values (1, 2, 4, 8, 16, 32, and 64 mg/L; Figures 2, 4 and Supplementary Table S8), despite the relatively high genetic similarity of mcr-3 alleles (i.e., MCR-3 alleles with reported MIC values share 88.7% to 99.8% AA identity and 97.6% to 100% AA similarity). This showcases that sequence similarity alone cannot explain colistin MIC values in mcr-3-harboring native strains, thus highlighting the importance of considering mcr-mediated colistin resistance holistically (e.g., considering strain background, genomic context, transcription and expression). Furthermore, low levels of colistin resistance (i.e., MIC < 2 mg/L), which would be classified as colistin susceptible under standard testing conditions, were reported for some mcr-harboring bacterial strains (e.g., a strain harboring mcr-3.14; Figure 4 and Supplementary Table S8) (Shen et al., 2018; Teo et al., 2018).
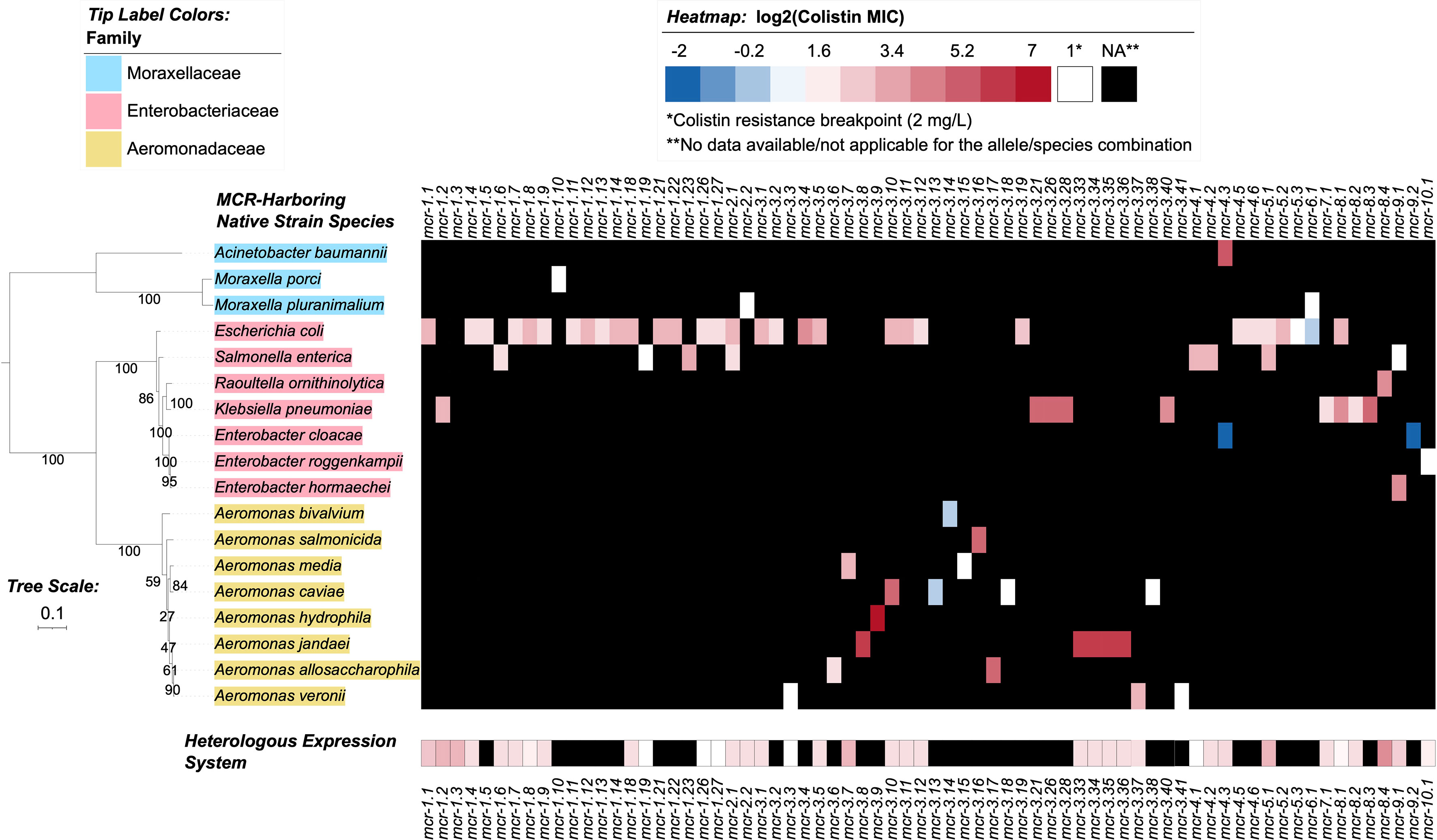
Figure 4 Heatmap showcasing colistin minimum inhibitory concentration (MIC) values reported for MCR alleles in their native strains and in heterologous expression systems. MIC values were obtained from a review of the literature (see the Materials and Methods section for details). Heatmap cell colors correspond to base-2 logarithm-transformed MIC values, where white cells denote a value of 1 (i.e., the log2-transformed colistin resistance breakpoint of 2 mg/L, established by the Clinical and Laboratory Standards Institute [CLSI]); blue shading denotes MIC values below this breakpoint, and red shading denotes MIC values above. MCR alleles with no data reported for a given species (due to the allele not being present in the species, or due to an MIC not being reported) are denoted in the heatmap by black shading. For individual species with multiple MIC values reported for a single MCR allele, the median MIC value is reported (Supplementary Table S8). For mcr-2.1, Salmonella enterica was selected to represent an unspecified “Salmonella spp.” reported in the literature for the native strain (Supplementary Table S8). The maximum likelihood (ML) phylogeny displayed to the left of the heatmap denotes type strains and/or NCBI representative genomes of reported species for the native strains (Supplementary Table S8). Phylogeny tip label colors correspond to taxonomic families assigned via the Genome Taxonomy Database Toolkit (GTDB-Tk). The phylogeny was constructed with IQ-TREE, using an alignment of amino acid sequences produced via GTDB-Tk as input. The phylogeny is rooted along the midpoint, with branch lengths reported in substitutions per site. Branch labels denote branch support percentages obtained using the ultrafast bootstrap approximation. The phylogeny was edited and displayed using iTOL.
Varying colistin MIC values were also reported among different genera expressing the same mcr allele (Figure 4 and Supplementary Table S8). For example, E. coli and Salmonella spp. harboring the mcr-2.1 allele had colistin MIC values of 8 and 4 mg/L, respectively (Xavier et al., 2016; Garcia-Graells et al., 2018; Poirel et al., 2018). Interestingly, an E. coli wildtype strain harboring the mcr-3.10 allele on a plasmid had a reported colistin MIC value of 8 mg/L, while Aeromonas caviae and Proteus mirabilis harboring a chromosomal mcr-3.10 allele had reported colistin MIC values of 32 mg/L (Wang et al., 2018c). Overall, it is apparent that colistin MIC values can vary between mcr-harboring native strains. However, any differences between mcr families and alleles should be interpreted cautiously, as additional factors may contribute to a given native strain’s colistin MIC (e.g., additional colistin resistance-conferring mutations, the presence or regulation of additional genes affecting colistin sensitivity) (El-Sayed Ahmed et al., 2020).
Notably, multiple mcr alleles have also been characterized via heterologous expression in standard laboratory strains (Figure 4 and Supplementary Table S8). MIC values extracted from the literature also indicated extensive variability in colistin MIC values among E. coli laboratory strains heterologously expressing different mcr alleles (Supplementary Table S8). In heterologous expression systems, several factors are likely to affect AMR levels, including the strains’ genetic background, plasmid copy number, level and regulation of gene expression, and cellular toxicity resulting from protein overexpression (Wagner et al., 2008; Schlegel et al., 2012; Tietgen et al., 2018).
3.7 Recombination plays a limited role in the evolution of mcr
Thirteen potential recombination events were initially identified in an NTbtn-MSA of mcr, putative novel mcr-like, and ipet genes via multiple detection methods in RDP5 (n = 460 total PET-encoding genes; Supplementary Figure S3 and Supplementary Table S7). Specifically, initial evidence for recombination among mcr-3 alleles was identified (i.e., events 1-3; Supplementary Figure S3). However, visual inspection of the recombination events indicated that recombinant fragments in these three events shared similar breakpoints, indicating that these fragments represented a single recombination event (Supplementary Figure S3). Moreover, within the mcr and ipet gene phylogeny, mcr-3 alleles identified in recombination events 1-4 clustered closely into subclades with relatively short terminal branches (Figure 3 and Supplementary Figure S3). Similar patterns were observed for alleles associated with recombination events 5-8 and 10-13 (Figure 3 and Supplementary Figure S3), indicating that the recombination signals identified in events 1-8 and 10-13 (i.e., all events except for event 9) might have been caused by evolutionary processes other than homologous recombination, such as inter-lineage and inter-site mutation-rate variation (Bertrand et al., 2016; Martin et al., 2021).
Recombination event 9, which included mcr-7.1, mcr-3.34, and a newly identified putative novel mcr-like gene from Aeromonas caviae (gene locus ID C1C91_01450 and NCBI Nucleotide accession CP025706.1), was identified by six different recombination detection methods in RDP5 (pairwise homoplasy index [PHI] raw P = 0.035; Supplementary Figure S3). The PET phylogenies (Figures 2, 3) revealed that the putative recombinant and parents were divergent and had relatively long terminal branches, suggesting that recombination event 9 may have played a role in the evolution of some mcr-3 and mcr-7 alleles (Supplementary Figure S3). Overall, these results posit a limited role for homologous recombination in the evolution and diversification of mcr alleles. However, it is feasible that some recombination events (e.g., between closely related sequences or genes not included in the analysis) were involved in the evolution of mcr.
3.8 Positive selection likely contributed to the evolution of specific amino acid residues that may induce localized structural variation among MCR alleles
The role of positive selection in the evolution of genes can be assessed by estimating the non-synonymous to synonymous substitution rate (dN/dS) ratio (w), where an overall w > 1 indicates positive selection (Anisimova and Kosiol, 2009; Delport et al., 2009). Alternatively, w = 1 or w < 1 suggests neutral or negative selection, respectively (Murrell et al., 2012). Overall, an average w value of 0.198 across the 98 known mcr alleles was observed (via DnaSP; Supplementary Table S6), suggesting that, overall, mcr evolved under negative selection. To further test for selection at specific AA sites, we used FUBAR (the Fast, Unconstrained Bayesian AppRoximation method, which infers dN/dS on a per-site basis) (Murrell et al., 2013), which predicted that 216 of 593 AA sites showed strong evidence for evolving under negative selection (FUBAR posterior probability ≥ 0.99; Supplementary Figure S4 and Supplementary Table S12). We found that AA sites that may have evolved under negative selection were distributed among the three structural components of MCR-1. Specifically, 36% of the AA residues of the membrane-anchored domain, 18% of the AA residues of the bridging region, and 44% of the AA residues of the catalytic domain were predicted to evolve under negative selection (Supplementary Figure S4). Using FUBAR, no sites were predicted to evolve under positive selection at posterior probability ≥ 0.99.
While an overall dN/dS ratio < 1 indicates that positive selection did not likely contribute to the overall evolution of mcr, positive selection may have still played a role in the evolution of allele-specific and branch-specific AA sites. Indeed, relatively few genes with dN/dS ratio > 1 are expected to exist (Murrell et al., 2012). Hence, we used a mixed-effect model of evolution (MEME) approach to identify positive selection at the level of individual alleles, a branch, or a set of branches. MEME showed that positive selection may have played a role in the diversification of 19 allele-specific AA sites within 34 branch-specific nodes distributed among two partitions in the phylogeny of 98 known mcr alleles (MEME raw P < 0.1; Figure 5 and Supplementary Table S13). Among the 19 AA sites, 11 residues were located within the membrane-anchored domain, one in the bridging helix, one in the interdomain flexible loop, and six in the catalytic domain (Figure 5). Interestingly, MEME identified multiple positive selection events in the mcr-2 and mcr-9 family phylogeny branches (Figure 5).
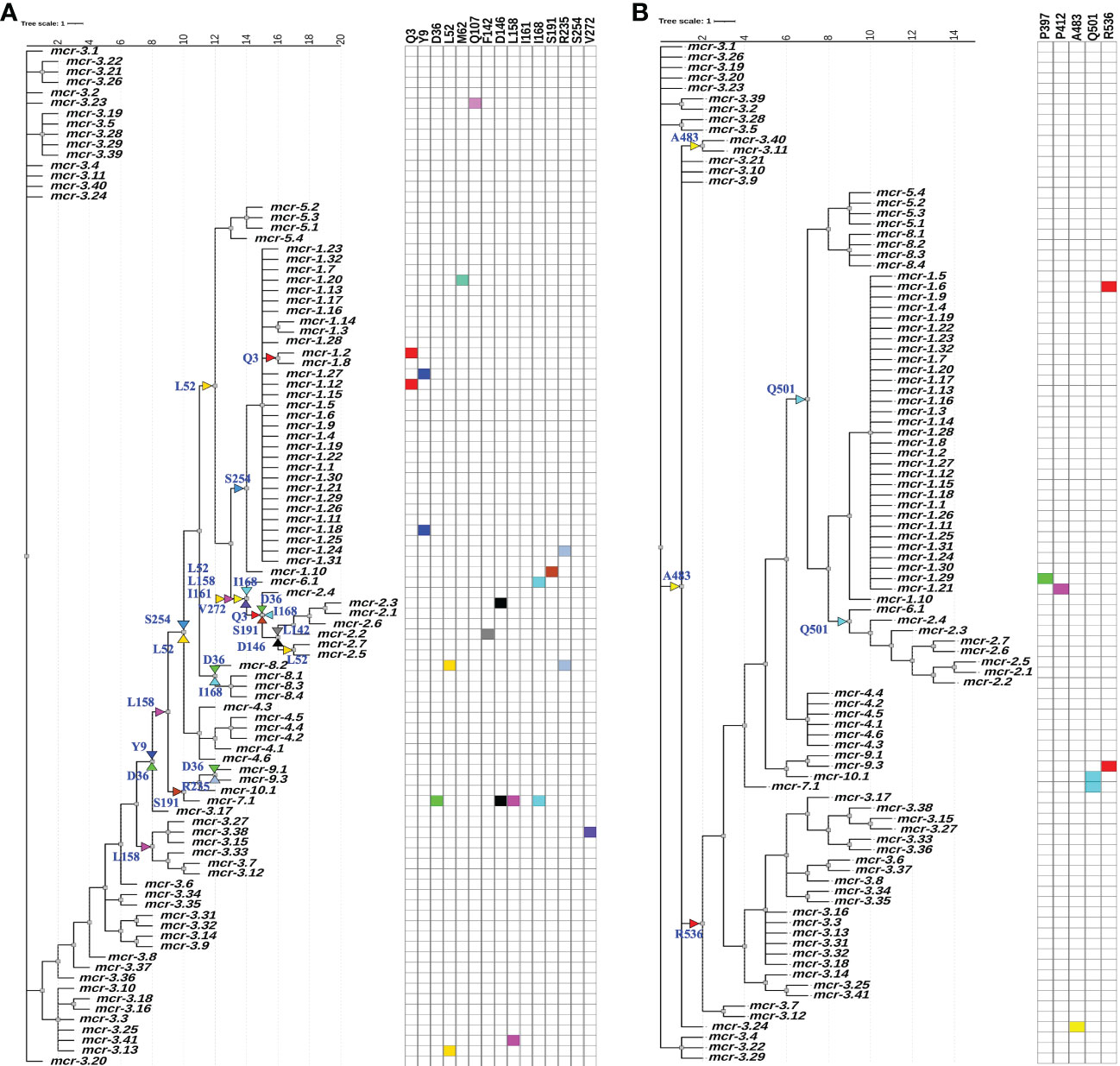
Figure 5 Identification of amino acid (AA) residues under branch-specific positive selection. A phylogeny of 98 known mcr alleles produced and partitioned by GARD (https://www.datamonkey.org/gard) shows partition 1 (A) and partition 2 (B) branch-specific AA sites evolving under positive selection. Sequences were aligned using MUSCLE, and the resulting multiple sequence alignment (MSA) was supplied as input to GARD. The partitioned dataset produced by GARD was supplied as input to MEME (mixed-effect model of evolution-based selection analysis; https://www.datamonkey.org/meme), which was used to identify AA residues under branch-specific positive selection. The tree (default output/rooting produced by GARD/MEME, with branch lengths reported in substitutions per site) was edited using the iTOL web server (https://itol.embl.de/). Color-coded regions and triangles represent AA residues under allele-specific and branch-specific positive selection, respectively.
While MEME did not identify evidence of positive selection for AA residues involved in zinc and pEtN binding, the analysis predicted that several structurally critical residues evolved under positive selection (Supplementary Table S13). Mapping of these residues on the structural model of MCR-1 (Figures 6A, B) indicated that several of the AA residues predicted to have evolved under positive selection were located in structurally important regions, including: (i) Gln107, which is located at a periplasmic loop connecting the third and fourth transmembrane segments juxtaposing the substrate entry tunnel; (ii) Ser191 and Arg235, which are located at a bridging region that connects the N-terminal membrane domain and the C-terminal catalytic domain; and (iii) Pro397and Pro412, and Ala483, which are located adjacent to the active site and substrate entry tunnel, respectively (Figures 6A, B). Subsequently, we evaluated the sequence diversity of the codons associated with these 19 AA residues among the 98 mcr alleles (Figure 6C). Overall, we found that the coding sequences of the 19 AA residues were highly conserved among alleles of the same mcr family. In contrast, these sequences were less conserved among alleles of different families. Specifically, 47 of 57 nucleotide sites were polymorphic among the 98 mcr alleles; p values of synonymous and non-synonymous substitutions were 0.472 and 0.348 among all 98 alleles. Comparatively, p values of synonymous and non-synonymous substitutions were 0.003 and 0.017 among mcr-1 alleles, and 0.028 and 0.029 among mcr-3 alleles, respectively.
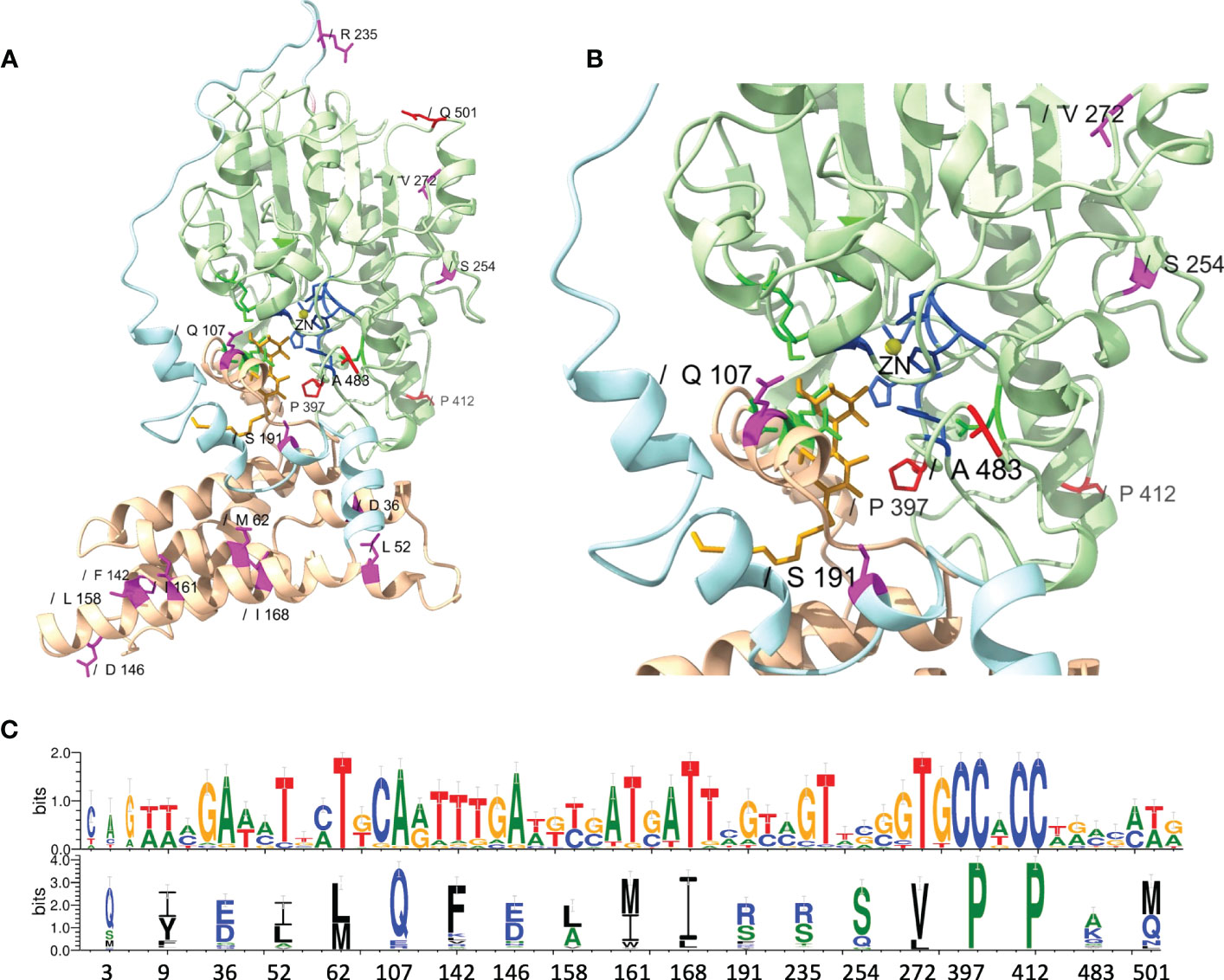
Figure 6 Structural localization and conservation of amino acid (AA) residues under branch-specific positive selection. (A) Structural model of the MCR-1 protein, constructed based on Neisseria meningitidis phosphoethanolamine transferase EptA (Anandan et al., 2017). The MCR structural model was constructed using the Phyre2 server, and the structure was viewed and edited using UCSF ChimeraX. The structural model shows the transmembrane-anchored domain (yellow) and the soluble periplasmic catalytic domain (light green) connected by a bridging helix and extended loop (light blue). AA residues involved in zinc (yellow circle) and phosphoethanolamine (pEtN) binding are colored dark blue and green, respectively. Branch-specific AA residues evolving under positive selection are shown in magenta (partition 1) and red (partition 2). (B) Close-up of the MCR active site showing the localization of branch-specific AA residues under positive selection in relation to the active site and the substrate entry tunnel. The structure shows Gln107, which is located at a periplasmic loop connecting the third and fourth transmembrane segments juxtaposing the substrate entry tunnel; Ser191, which is located at a bridging region that connects the N-terminal membrane domain and the C-terminal catalytic domain; and Pro397, which is located adjacent to the active site. (C) Web-logo of nucleotide and AA sequences showing the conservation of 19 AA residues identified by MEME (https://www.datamonkey.org/meme) to have evolved under positive selection among the 98 known MCR alleles.
3.9 Novel MCR alleles and families may be present among publicly available bacterial genomes
To further assess the novelty of the 125 putative novel mcr-like genes identified here, all MCR, putative novel MCR-like, and i-PET proteins were partitioned into clusters based on AA identity (Supplementary Table S7). At an 80% AA identity threshold, MCR, putative novel MCR-like, and i-PET AA sequences were partitioned into 64 clusters; of these 64 clusters, the AA sequences associated with the 125 putative novel mcr-like genes (Supplementary Table S3) were partitioned into 13 clusters, none of which contained known MCR proteins. Considering AA identity among the putative novel MCR-like proteins alone (i.e., ignoring genomic context, as well as AA identity/phylogenetic similarity to i-PET proteins/ipet genes), these 13 clusters can be thought of as “PET families”, as 80% AA identity is a conservative MCR family delineation threshold. For comparison, the ten known MCR families were partitioned into seven families using this approach: one family aggregated all MCR-1, MCR-2, and MCR-6 alleles, one aggregated all MCR-9 and MCR-10 alleles, and each of the five remaining known MCR families were partitioned into separate PET families at 80% AA identity. However, when (i) genomic context and (ii) AA identity/phylogenetic similarity to i-PET proteins/ipet genes were considered, eight of the 13 PET families (representing 99 putative novel MCR-like proteins) were more similar to EptA than to MCR proteins (i.e., they grouped into cluster II-B-2g; Figure 3 and Supplementary Figure S2). Thus, putative novel MCR-like proteins in cluster II-B-2g are likely to function as EptA or EptA-like proteins rather than bona fide MCR proteins.
Notably, five PET families (encompassing 26 putative novel MCR-like proteins) resembled known MCR families, not only by AA identity and phylogenetic relatedness, but also based on genomic context (Figure 3 and Supplementary Figure S2). Indeed, two putative novel mcr genes in cluster II-B-2c were located in an operon with a PAP2-encoding gene (Figure 3 and Supplementary Figure S2). Interestingly, the genomic context of a gene in cluster II-B-2e shared characteristics of both mcr and eptA genomic contexts (locus EGY13_RS05385; Figure 3 and Supplementary Figure S2). While the EGY13_RS05385 gene was located in an operon with a two-component sensor histidine kinase-response regulator system (similar to eptA genes), it was located adjacent to a PAP2-encoding gene (similar to mcr genes). Thus, a conservative 80% AA identity threshold for clustering not only further supports that we likely identified 26 novel mcr genes, but also suggests that these mcr genes represent five novel MCR families (designated novel MCR families A to E; Figure 3).
As no hard AA identity cutoff has been proposed for defining novel MCR families (Partridge et al., 2018), there may be some ambiguity as to whether the five putative novel MCR-like families identified here truly represent putative novel MCR families or alleles of existing families, despite sharing < 80% AA identity with all known MCR families. For example, the mcr-like genes grouped into mcr family A (i.e., Xanthomonas campestris locus tag BHE83_RS21235 and Xanthomonas phaseoli locus tag XASLMG9055_RS15450) were phylogenetically close to mcr-5; thus, they might be considered to be alleles of mcr-5, rather than a novel mcr family (Figure 3 and Supplementary Figure S2). However, in contrast to mcr-5 alleles, mcr family A genes were located in an operon with a PAP2 family lipid A phosphatase-encoding gene, which might suggest that these genes represent a novel mcr family, rather than novel mcr-5 alleles. Similarly, mcr-like genes grouped into mcr family E (i.e., Aeromonas hydrophila and Aeromonas caviae locus tags BFW41_RS14915, C1C91_RS01450, and C1C92_RS20765) may be considered to be novel mcr-7 alleles based on phylogenetic similarity (Supplementary Figure S2). However, while the mcr-7.1 allele was localized adjacent to an operon containing DgkA and PAP2 enzymes, mcr family E genes consist of single-gene operons. Comparatively, the mcr-like genes classified into mcr families B, C, and D (n = 6, 13, and 2 genes; Figure 3) were phylogenetically distinct from known mcr genes but resembled mcr genes rather than eptA in terms of sequence similarity, phylogenetic relatedness, and genomic context, and thus likely represent novel mcr families. Regardless, future experimental and phenotypic analyses are needed to define the potential roles of these genes in colistin resistance and assess their functional diversity.
4 Discussion
Overall, our data show that (i) PET proteins can be detected across a wide range of Gram-negative bacterial species and are genetically and functionally diverse; (ii) the evolution and diversification of mcr is likely the result of a multifaceted process, which includes positive selection, gene mobilization, and diversification of regulatory pathways and gene contexts; (iii) a holistic approach that considers genomic context, in addition to sequence similarity and genomic localization, may aid in the identification of novel mcr families and alleles.
4.1 Genetically and functionally diverse PET proteins can be detected across a wide range of Gram-negative bacterial species
Previous studies have shown that PET-encoding genes are distributed across a range of Gram-negative bacteria (Nang et al., 2019; El-Sayed Ahmed et al., 2020; Khedher et al., 2020). Here, we identified >69,000 proteins homologous to colistin resistance-conferring PET in >1,000 bacterial species (based on the mOTUs taxonomy; Supplementary Table S2). Using a subset of PET-encoding genes composed of (i) all 98 known MCR-encoding alleles, (ii) 125 genes encoding putative novel MCR-like proteins, and (iii) 237 chromosomal ipet genes, we provide further insight into the genetic diversity of colistin resistance-conferring and intrinsic PET-encoding genes alike (Figure 3). However, it is important to note the limitations of the criteria used here to identify the 125 putative novel mcr-like genes (i.e., co-localization on the same contig as a predicted plasmid replicon and at least one other AMR gene). Previous studies have shown that 10% of bacterial genomes are composed of several essential replicons, including secondary chromosomes and chromids, which use a plasmid-like origin of replication for propagation (Harrison et al., 2010; Dicenzo and Finan, 2017). Hence, the approach used here could incorrectly classify such a contig as plasmid-associated. However, by considering additional factors (e.g., genomic context), we were able to refine our predictions and identify which of the 125 putative novel mcr-like genes likely represent bona fide novel mcr genes. Thus, we believe this limitation has no major impact on our conclusions.
Consistent with previous studies (Carroll et al., 2019; Khedher et al., 2020), we have shown that mcr families are genetically diverse. The phylogenetic analyses conducted here also suggest that eptA and mcr genes share a common ancestor. While previous studies have hypothesized that mcr evolved from eptA through the mobilization of an eptA gene copy flanked by transposable insertion elements (Kieffer et al., 2017; Wang et al., 2018a), with Moraxella spp. suggested as a potential source (Kieffer et al., 2017), our data fail to provide clear evidence of a specific event or events that may have led to the emergence of PET genes that confer colistin resistance.
In addition to being genetically diverse, increasing evidence suggests that PET-encoding genes are functionally diverse (Anandan and Vrielink, 2020a). Additionally, it has been shown that different mcr alleles may confer different levels of colistin resistance (Nang et al., 2019). While this conclusion is supported by the high level of genetic diversity and the diverse genomic contexts of mcr genes (e.g., presence of regulatory genes and genes encoding accessory enzymes that support MCR function, such as PAP2 and DgkA enzymes), assessment of colistin resistance levels in mcr-harboring strains should be interpreted with extreme caution. Colistin’s primary mode of action is the displacement of LPS-stabilizing cations, followed by insertion into the membranes and cell disruption; however, it has been suggested that oxidative damage plays a role in colistin-dependent cell killing (Yu et al., 2015; El-Sayed Ahmed et al., 2020). Thus, variations among stress response systems can alter the susceptibility of bacterial cells to colistin treatment (Touati, 2000; Imlay, 2003; O'connor and Mcclean, 2017). Furthermore, additional factors may influence colistin MICs associated with mcr-harboring native bacterial strains, including additional colistin resistance-conferring mutations, the level and regulation of mcr transcription, the presence or regulation of additional genes affecting colistin sensitivity, and the growth and testing conditions used to assess colistin sensitivity (Martinez-Servat et al., 2018; El-Sayed Ahmed et al., 2020; Loose et al., 2020). Thus, a clear understanding of the extent to which mcr genetic diversity contributes to colistin resistance heterogeneity remains a major challenge.
4.2 Evolution and diversification of mcr is the result of a multifaceted process
Our data suggest that multiple factors might be involved in the evolution and functional diversification of mcr, including the diversification of the genomic context and the regulatory control of gene expression. We found that all analyzed chromosomal eptA, as well as the putative novel mcr-like genes most closely related to eptA, were localized adjacent to a TetR-type regulator or a two-component sensor histidine kinase-response regulator system. With the exception of mcr-8, no specific regulator was found to be associated with the remaining mcr families. In contrast, we found that lipid A phosphatase-encoding pap2 and diacylglycerol kinase-encoding dgkA were frequently located adjacent to many mcr alleles. Specifically, mcr-1 and mcr-2 alleles were located adjacent to pap2, while mcr-3 and mcr-8 alleles were located adjacent to dgkA. Recently, a detailed mutational analysis indicated that pap2 and dgkA play a significant role in mcr-dependent colistin resistance, possibly through recycling and modification of lipid metabolism products byproducts (Choi et al., 2020; Gallardo et al., 2020; Purcell et al., 2022).
Moreover, it was shown that a transposon insertional element has horizontally transferred the mcr-1-pap2 region into different plasmid backbones (Li et al., 2016; Abuoun et al., 2017; Zhang et al., 2019). The role of pap2 in mcr function is further corroborated by the recent identification of a Sutterella wadsworthensis gene encoding a single polypeptide with an N-terminal PAP2 domain and C-terminal MCR-like domain (Andrade et al., 2021). Hence, it is evident that the genomic contexts of eptA and mcr include differential association with the presence of regulatory proteins or accessory enzymes of lipid metabolism. eptA genes are exclusively located adjacent to transcription regulators, while mcr genes are frequently located adjacent to enzymes for the recycling and modification of lipid metabolism byproducts. These findings may not only help identify novel genes that are likely to represent MCR, but also may provide a framework for further classification of mcr genes.
Additionally, our data suggest that positive selection likely contributed to the diversification of specific mcr alleles, as we found that positive selection might have played a role in the evolution of several AA residues adjacent to functionally and structurally important protein regions. The AA residues predicted to evolve under positive selection were not part of the active site and the substrate-binding site; however, they are likely to affect enzyme function, such as possible alteration of substrate accessibility, specificity, and enzyme efficacy. For example, we predicted that branch-specific positive selection might be involved in the diversification of Ser191 and Arg235 in the interdomain-connecting region, which is relevant, as it has been shown that the conformational flexibility of EptA is essential in enzyme-substrate recognition (Anandan et al., 2021). The conformational changes of EptA are governed by a highly conserved domain structure that offers extensive flexibility between the membrane-bound and the periplasmic catalytic domains (Anandan et al., 2017; Anandan and Vrielink, 2020a). Moreover, Anandan et al. (Anandan et al., 2021) proposed that the bridging helix acts as a hinge region that enables extensive conformational changes between the two domains, “opening up” the catalytic domain to allow access to the considerably large lipid A substrate. Hence, diversification of Ser191 and Arg235 is likely to influence the overall conformational flexibility of the enzyme. For example, at position 191, the relatively small polar Ser (present in mcr-1 alleles) may offer better conformational flexibility compared to other AA residues identified by MEME at the same locus, such as the non-polar aromatic Phe (mcr-2 alleles) and the negatively charged Asp (mcr-8 alleles; Supplementary Table S13).
Similarly, the diversification of Gln107, Pro397, Pro412, and Ala483 residues, which are located in periplasmic loops juxtaposed and adjacent to the substrate entry tunnel and the active site (Anandan et al., 2017; Anandan and Vrielink, 2020a) and were also identified as being under positive selection, is likely to affect enzyme activity or specificity by influencing access to the substrate entry tunnel. While our data indicate that diversification of specific AA residues might contribute to the functional diversity of MCR alleles in colistin resistance, detailed site-directed mutational analyses will be required to define the role of positive selection in the functional diversity of MCR. Overall, our data raise the intriguing possibility that eptA can give rise to colistin resistance genes through a multifaceted process, which includes positive selection, as well as gene mobilization, diversification of genomic context and regulatory pathways, and possibly (to a more limited extent) recombination. However, the relative contribution of these factors in the evolution of mcr remains unknown.
4.3 A holistic approach that considers sequence, structure, genomic localization, and genomic context may aid in the discovery of novel mcr families and alleles
The identification of novel mcr genes is important from both a public health and clinical perspective. For example, in order to monitor and combat the spread of colistin resistance, it is necessary to identify novel mcr genes so that they can be included in AMR surveillance efforts (Yin et al., 2017; Carroll et al., 2019). Similarly, a comprehensive database of mcr genes can be utilized to screen pathogens isolated from patient clinical samples (e.g., to predict colistin treatment outcome and identify potential treatment failure events).
In addition to providing insight into the genetic and functional diversity of mcr and other PET-encoding genes, we identified 125 unique, putative novel mcr-like genes, which were located on the same contig as (i) ≥1 plasmid replicon and (ii) ≥1 additional AMR gene. These putative novel MCR-like proteins represented 13 PET families at a conservative 80% AA identity threshold (although there is no strict identity cutoff for defining novel MCR families) (Partridge et al., 2018). Notably, five of these families (representing 26 of the 125 genes) resembled MCR proteins based on AA identity, phylogenetic relatedness, and genomic context, and thus may represent novel MCR families. It may be tempting to speculate on the colistin resistance-conferring potential of these 26 putative novel mcr-like genes; however, the discrimination between colistin resistance-conferring mcr and intrinsic lipid modification eptA, as well as functional assessment of mcr alleles, remain a clear challenge, at least partially due to (i) the high genetic diversity among mcr families and alleles; (ii) the absence of functional characterization for many mcr alleles; and (iii) the extensive diversity of the genetic background of mcr-harboring species. While it thus is possible that some of the putative novel mcr-like genes identified here may encode i-PET enzymes, we believe that the robust approach used here to identify five putative novel mcr families composed of 26 putative novel mcr genes minimizes this possibility. However, future functional analyses will be essential to assign biological functions to the 26 putative novel mcr genes identified here.
As demonstrated here, comprehensive bioinformatic approaches are necessary to facilitate the initial identification of AMR genes, as inferring AMR phenotypes from bacterial WGS data is often challenging (Tyson et al., 2015; Ransom et al., 2020). In the case of mcr and eptA, sequence similarity alone is not sufficient to distinguish one from the other, as demonstrated here. However, functional analyses of colistin resistance are also challenging. For example, it has been shown that heterologous overexpression of Pseudomonas aeruginosa eptA (Cervoni et al., 2021) or dysregulation of Salmonella enterica serotype Typhimurium eptA transcription (Vaara et al., 1981; Olaitan et al., 2014) can also lead to colistin resistance. In addition, some mcr genes may be expressed under physiologically relevant conditions, but not under standard AMR testing conditions (Kananizadeh et al., 2022). Therefore, functional discrimination between eptA and mcr may require comprehensive physiological characterization, which may involve cloning in a heterologous expression system and the construction of gene-specific mutants (Gao et al., 2016; Liu et al., 2016; Carroll et al., 2019). These approaches are time-consuming and require a hard-to-achieve standardization process to ensure similar expression levels of active protein and minimal cytotoxicity (resulting from the expression of the membrane-anchored MCR proteins, which is likely to interfere with MIC determination). Identification of additional criteria that can be used to select and prioritize “high risk” mcr and mcr-like genes for future experimental validation efforts, as reported here, is thus important. Here, we used a high-throughput in silico approach to compile a list of >69,000 MCR and MCR-like proteins in publicly available genomes. From this list, we identified 125 unique putative novel mcr-like genes, which were refined to 26 bona fide putative novel mcr genes representing five putative novel mcr families, which are candidates for further characterization. Overall, in the future, we imagine that the holistic approach used here, which considers multiple criteria (e.g., sequence similarity, phylogenetic relatedness, genomic context, and structural homology), will facilitate the further identification of novel mcr families and alleles from bacterial WGS data and aid researchers in prioritizing mcr-like genes for experimental validation.
Data availability statement
The datasets presented in this study can be found in online repositories. The names of the repository/repositories and accession number(s) can be found in the article/Supplementary material.
Author contributions
AG and LC performed all computational analyses. AG, MW, and LC designed the study and co-wrote the manuscript. All authors contributed to the article and approved the submitted version.
Funding
LC was supported by the SciLifeLab & Wallenberg Data Driven Life Science Program (grant: KAW 2020.0239). AG and MW were supported by a Hatch grant under accession number 1023966 from the USDA National Institute of Food and Agriculture.
Conflict of interest
The authors declare that the research was conducted in the absence of any commercial or financial relationships that could be construed as a potential conflict of interest.
Publisher’s note
All claims expressed in this article are solely those of the authors and do not necessarily represent those of their affiliated organizations, or those of the publisher, the editors and the reviewers. Any product that may be evaluated in this article, or claim that may be made by its manufacturer, is not guaranteed or endorsed by the publisher.
Supplementary material
The Supplementary Material for this article can be found online at: https://www.frontiersin.org/articles/10.3389/fcimb.2023.1060519/full#supplementary-material
References
Abdul Momin, M. H. F., Liakopoulos, A., Bean, D. C., Phee, L. M., Wareham, D. W. (2019). A novel plasmid-mediated polymyxin resistance determinant (mcr-1.8) in Escherichia coli recovered from broiler chickens in Brunei darussalam. J. Antimicrob. Chemother. 74, 3392–3394. doi: 10.1093/jac/dkz352
Abuoun, M., Stubberfield, E. J., Duggett, N. A., Kirchner, M., Dormer, L., Nunez-Garcia, J., et al. (2017). mcr-1 and mcr-2 variant genes identified in Moraxella species isolated from pigs in great Britain from 2014 to 2015. J. Antimicrob. Chemother. 72, 2745–2749. doi: 10.1093/jac/dkx286
Alba, P., Leekitcharoenphon, P., Franco, A., Feltrin, F., Ianzano, A., Caprioli, A., et al. (2018). Molecular epidemiology of mcr-encoded colistin resistance in enterobacteriaceae from food-producing animals in Italy revealed through the EU harmonized antimicrobial resistance monitoring. Front. Microbiol. 9, 1217. doi: 10.3389/fmicb.2018.01217
Anandan, A., Dunstan, N. W., Ryan, T. M., Mertens, H. D. T., Lim, K. Y. L., Evans, G. L., et al. (2021). Conformational flexibility of EptA driven by an interdomain helix provides insights for enzyme-substrate recognition. IUCrJ 8, 2052–2525. doi: 10.1107/S2052252521005613
Anandan, A., Evans, G. L., Condic-Jurkic, K., O'mara, M. L., John, C. M., Phillips, N. J., et al. (2017). Structure of a lipid a phosphoethanolamine transferase suggests how conformational changes govern substrate binding. Proc. Natl. Acad. Sci. U.S.A. 114, 2218–2223. doi: 10.1073/pnas.1612927114
Anandan, A., Vrielink, A. (2020a). Structure and function of lipid a-modifying enzymes. Ann. N Y Acad. Sci. 1459, 19–37. doi: 10.1111/nyas.14244
Anandan, A., Vrielink, A. (2020b). Structure and function of lipid a–modifying enzymes. Ann. New York Acad. Sci. 1459, 19–37. doi: 10.1111/nyas.14244
Andrade, B. G. N., Goris, T., Afli, H., Coutinho, F. H., Davila, A. M. R., Cuadrat, R. R. C. (2021). Putative mobilized colistin resistance genes in the human gut microbiome. BMC Microbiol. 21, 220. doi: 10.1186/s12866-021-02281-4
Anisimova, M., Kosiol, C. (2009). Investigating protein-coding sequence evolution with probabilistic codon substitution models. Mol. Biol. Evol. 26, 255–271. doi: 10.1093/molbev/msn232
Anyanwu, M. U., Marrollo, R., Paolucci, M., Brovarone, F., Nardini, P., Chah, K. F., et al. (2021). Isolation and characterisation of colistin-resistant enterobacterales from chickens in southeast Nigeria. J. Glob Antimicrob. Resist. 26, 93–100. doi: 10.1016/j.jgar.2021.04.030
Bakovic, M., Fullerton, M. D., Michel, V. (2007). Metabolic and molecular aspects of ethanolamine phospholipid biosynthesis: the role of CTP:phosphoethanolamine cytidylyltransferase (Pcyt2). Biochem. Cell Biol. 85, 283–300. doi: 10.1139/O07-006
Bertrand, Y. J., Johansson, M., Norberg, P. (2016). Revisiting recombination signal in the tick-borne encephalitis virus: a simulation approach. PloS One 11, e0164435. doi: 10.1371/journal.pone.0164435
Binsker, U., Käsbohrer, A., Hammerl, J. A. (2022). Global colistin use: a review of the emergence of resistant enterobacterales and the impact on their genetic basis. FEMS Microbiol. Rev. 46, fuab049. doi: 10.1093/femsre/fuab049
Bitar, I., Medvecky, M., Gelbicova, T., Jakubu, V., Hrabak, J., Zemlickova, H., et al. (2019). Complete nucleotide sequences of mcr-4.3-Carrying plasmids in Acinetobacter baumannii sequence type 345 of human and food origin from the Czech republic, the first case in Europe. Antimicrob. Agents Chemother. 63, e01166-19. doi: 10.1128/AAC.01166-19
Bitar, I., Papagiannitsis, C. C., Kraftova, L., Chudejova, K., Mattioni Marchetti, V., Hrabak, J. (2020). Detection of five mcr-9-Carrying enterobacterales isolates in four Czech hospitals. mSphere 5, e01008-20. doi: 10.1128/mSphere.01008-20
Borowiak, M., Fischer, J., Hammerl, J. A., Hendriksen, R. S., Szabo, I., Malorny, B. (2017). Identification of a novel transposon-associated phosphoethanolamine transferase gene, mcr-5, conferring colistin resistance in d-tartrate fermenting Salmonella enterica subsp. enterica serovar paratyphi b. J. Antimicrob. Chemother. 72, 3317–3324. doi: 10.1093/jac/dkx327
Buchfink, B., Xie, C., Huson, D. H. (2015). Fast and sensitive protein alignment using DIAMOND. Nat. Methods 12, 59–60. doi: 10.1038/nmeth.3176
Campos, M. A., Vargas, M. A., Regueiro, V., Llompart, C. M., Alberti, S., Bengoechea, J. A. (2004). Capsule polysaccharide mediates bacterial resistance to antimicrobial peptides. Infect. Immun. 72, 7107–7114. doi: 10.1128/IAI.72.12.7107-7114.2004
Carattoli, A., Carretto, E., Brovarone, F., Sarti, M., Villa, L. (2018). Comparative analysis of an mcr-4 salmonella enterica subsp. enterica monophasic variant of human and animal origin. J. Antimicrob. Chemother. 73, 3332–3335. doi: 10.1093/jac/dky340
Carattoli, A., Villa, L., Feudi, C., Curcio, L., Orsini, S., Luppi, A., et al. (2017). Novel plasmid-mediated colistin resistance mcr-4 gene in Salmonella and Escherichia coli, Italy 2013, Spain and belgium 2015 to 2016. Euro Surveill 22, 30589. doi: 10.2807/1560-7917.ES.2017.22.31.30589
Carattoli, A., Zankari, E., Garcia-Fernandez, A., Voldby Larsen, M., Lund, O., Villa, L., et al. (2014). In silico detection and typing of plasmids using PlasmidFinder and plasmid multilocus sequence typing. Antimicrob. Agents Chemother. 58, 3895–3903. doi: 10.1128/AAC.02412-14
Carroll, L. M., Gaballa, A., Guldimann, C., Sullivan, G., Henderson, L. O., Wiedmann, M. (2019). Identification of novel mobilized colistin resistance gene mcr-9 in a multidrug-resistant, colistin-susceptible Salmonella enterica serotype typhimurium isolate. mBio 10, e00853-19. doi: 10.1128/mBio.00853-19
Cervoni, M., Lo Sciuto, A., Bianchini, C., Mancone, C., Imperi, F. (2021). Exogenous and endogenous phosphoethanolamine transferases differently affect colistin resistance and fitness in Pseudomonas aeruginosa. Front. Microbiol. 12, 778968. doi: 10.3389/fmicb.2021.778968
Cha, M. H., Woo, G. J., Lee, W., Kim, S. H., Woo, J. H., Kim, J., et al. (2020). Emergence of transferable mcr-9 gene-carrying colistin-resistant Salmonella enterica dessau ST14 isolated from retail chicken meat in Korea. Foodborne Pathog. Dis. 17, 720–727. doi: 10.1089/fpd.2020.2810
Chaumeil, P. A., Mussig, A. J., Hugenholtz, P., Parks, D. H. (2019). GTDB-tk: a toolkit to classify genomes with the genome taxonomy database. Bioinformatics 36 (6), 1925–1927. doi: 10.1093/bioinformatics/btz848
Chavda, B., Lv, J., Hou, M., Chavda, K. D., Kreiswirth, B. N., Feng, Y., et al. (2018). Coidentification of mcr-4.3 and blaNDM-1 in a clinical Enterobacter cloacae isolate from China. Antimicrob. Agents Chemother. 62, e00649-18. doi: 10.1128/AAC.00649-18
Chavda, K. D., Westblade, L. F., Satlin, M. J., Hemmert, A. C., Castanheira, M., Jenkins, S. G., et al. (2019). First report of bla VIM-4- and mcr-9-Coharboring Enterobacter species isolated from a pediatric patient. mSphere 4, e00629-19. doi: 10.1128/mSphere.00629-19
Chew, K. L., La, M. V., Lin, R. T. P., Teo, J. W. P. (2017). Colistin and polymyxin b susceptibility testing for carbapenem-resistant and mcr-positive enterobacteriaceae: comparison of sensititre, MicroScan, vitek 2, and etest with broth microdilution. J. Clin. Microbiol. 55, 2609–2616. doi: 10.1128/JCM.00268-17
Choi, Y., Lee, J. Y., Lim, S. K., Ko, K. S. (2020). Intact pap2 downstream of mcr-1 appears to be required for colistin resistance. Diagn. Microbiol. Infect. Dis. 97, 114997. doi: 10.1016/j.diagmicrobio.2020.114997
Cox, A. D., Wright, J. C., Li, J., Hood, D. W., Moxon, E. R., Richards, J. C. (2003). Phosphorylation of the lipid a region of meningococcal lipopolysaccharide: identification of a family of transferases that add phosphoethanolamine to lipopolysaccharide. J. Bacteriol 185, 3270–3277. doi: 10.1128/JB.185.11.3270-3277.2003
Crooks, G. E., Hon, G., Chandonia, J. M., Brenner, S. E. (2004). WebLogo: a sequence logo generator. Genome Res. 14, 1188–1190. doi: 10.1101/gr.849004
Cui, Y., Quan, J., Liao, W., Zhao, D., Jiang, Y., Du, X., et al. (2019). A new variant of mcr-1 identified from an extended-spectrum beta lactamase-producing Escherichia coli. J. Glob Antimicrob. Resist. 18, 26–27. doi: 10.1016/j.jgar.2019.04.009
Delport, W., Scheffler, K., Seoighe, C. (2009). Models of coding sequence evolution. Brief Bioinform. 10, 97–109. doi: 10.1093/bib/bbn049
Deshpande, L. M., Hubler, C., Davis, A. P., Castanheira, M. (2019). Updated prevalence of mcr-like genes among Escherichia coli and Klebsiella pneumoniae in the SENTRY program and characterization of mcr-1.11 variant. Antimicrob. Agents Chemother. 63, e02450-18. doi: 10.1128/AAC.02450-18
Dicenzo, G. C., Finan, T. M. (2017). The divided bacterial genome: structure, function, and evolution. Microbiol. Mol. Biol. Rev. 81, e00019-17. doi: 10.1128/MMBR.00019-17
Di Pilato, V., Arena, F., Tascini, C., Cannatelli, A., Henrici De Angelis, L., Fortunato, S., et al. (2016). mcr-1.2, a new mcr variant carried on a transferable plasmid from a colistin-resistant KPC carbapenemase-producing Klebsiella pneumoniae strain of sequence type 512. Antimicrob. Agents Chemother. 60, 5612–5615. doi: 10.1128/AAC.01075-16
Dortet, L., Bonnin, R. A., Pennisi, I., Gauthier, L., Jousset, A. B., Dabos, L., et al. (2018). Rapid detection and discrimination of chromosome- and MCR-plasmid-mediated resistance to polymyxins by MALDI-TOF MS in Escherichia coli: the MALDIxin test. J. Antimicrob. Chemother. 73, 3359–3367. doi: 10.1093/jac/dky330
Duggett, N. A., Randall, L. P., Horton, R. A., Lemma, F., Kirchner, M., Nunez-Garcia, J., et al. (2018). Molecular epidemiology of isolates with multiple mcr plasmids from a pig farm in great Britain: the effects of colistin withdrawal in the short and long term. J. Antimicrob. Chemother. 73, 3025–3033. doi: 10.1093/jac/dky292
Edgar, R. C. (2004). MUSCLE: a multiple sequence alignment method with reduced time and space complexity. BMC Bioinf. 5, 113. doi: 10.1186/1471-2105-5-113
Eichhorn, I., Feudi, C., Wang, Y., Kaspar, H., Fessler, A. T., Lubke-Becker, A., et al. (2018). Identification of novel variants of the colistin resistance gene mcr-3 in Aeromonas spp. from the national resistance monitoring programme GERM-vet and from diagnostic submissions. J. Antimicrob. Chemother. 73, 1217–1221. doi: 10.1093/jac/dkx538
El-Sayed Ahmed, M., Zhong, L. L., Shen, C., Yang, Y., Doi, Y., Tian, G. B. (2020). Colistin and its role in the era of antibiotic resistance: an extended review, (2000-2019). Emerg. Microbes Infect. 9, 868–885. doi: 10.1080/22221751.2020.1754133
Fan, R., Li, C., Duan, R., Qin, S., Liang, J., Xiao, M., et al. (2020). Retrospective screening and analysis of mcr-1 and bla NDM in gram-negative bacteria in china 2010-2019. Front. Microbiol. 11, 121. doi: 10.3389/fmicb.2020.00121
Feldgarden, M., Brover, V., Haft, D. H., Prasad, A. B., Slotta, D. J., Tolstoy, I., et al. (2019). Validating the AMRFinder tool and resistance gene database by using antimicrobial resistance genotype-phenotype correlations in a collection of isolates. Antimicrob. Agents Chemother. 63, e00483-19. doi: 10.1128/AAC.00483-19
Fernandes, M. R., Cerdeira, L., Silva, M. M., Sellera, F. P., Munoz, M., Junior, F. G., et al. (2018). Novel mcr-5.3 variant in a CTX-M-8-producing Escherichia coli ST711 isolated from an infected horse. J. Antimicrob. Chemother. 73, 3520–3522. doi: 10.1093/jac/dky341
Fu, L., Niu, B., Zhu, Z., Wu, S., Li, W. (2012). CD-HIT: accelerated for clustering the next-generation sequencing data. Bioinformatics 28, 3150–3152. doi: 10.1093/bioinformatics/bts565
Gallardo, A., Ugarte-Ruiz, M., Hernandez, M., Miguela-Villoldo, P., Rodriguez-Lazaro, D., Dominguez, L., et al. (2020). Involvement of hpap2 and dgkA genes in colistin resistance mediated by mcr determinants. Antibiot. (Basel) 9, 531. doi: 10.3390/antibiotics9090531
Gao, R., Hu, Y., Li, Z., Sun, J., Wang, Q., Lin, J., et al. (2016). Dissemination and mechanism for the MCR-1 colistin resistance. PloS Pathog. 12, e1005957. doi: 10.1371/journal.ppat.1005957
Garcia-Graells, C., De Keersmaecker, S. C. J., Vanneste, K., Pochet, B., Vermeersch, K., Roosens, N., et al. (2018). Detection of plasmid-mediated colistin resistance, mcr-1 and mcr-2 genes, in Salmonella spp. isolated from food at retail in Belgium from 2012 to 2015. Foodborne Pathog. Dis. 15, 114–117. doi: 10.1089/fpd.2017.2329
Garcia-Menino, I., Lumbreras, P., Valledor, P., Diaz-Jimenez, D., Leston, L., Fernandez, J., et al. (2020). Comprehensive statistical evaluation of Etest((R)), UMIC((R)), MicroScan and disc diffusion versus standard broth microdilution: workflow for an accurate detection of colistin-resistant and mcr-positive E. coli. Antibiot. (Basel) 9, 861. doi: 10.3390/antibiotics9120861
Gogry, F. A., Siddiqui, M. T., Sultan, I., Haq, Q. M. R. (2021). Current update on intrinsic and acquired colistin resistance mechanisms in bacteria. Front. Med. (Lausanne) 8, 677720. doi: 10.3389/fmed.2021.677720
Gunn, J. S. (2008). The Salmonella PmrAB regulon: lipopolysaccharide modifications, antimicrobial peptide resistance and more. Trends Microbiol. 16, 284–290. doi: 10.1016/j.tim.2008.03.007
Gunn, J. S., Lim, K. B., Krueger, J., Kim, K., Guo, L., Hackett, M., et al. (1998). PmrA-PmrB-regulated genes necessary for 4-aminoarabinose lipid a modification and polymyxin resistance. Mol. Microbiol. 27, 1171–1182. doi: 10.1046/j.1365-2958.1998.00757.x
Guo, L., Lim, K. B., Gunn, J. S., Bainbridge, B., Darveau, R. P., Hackett, M., et al. (1997). Regulation of lipid a modifications by Salmonella typhimurium virulence genes phoP-phoQ. Science 276, 250–253. doi: 10.1126/science.276.5310.250
Hadjadj, L., Baron, S. A., Olaitan, A. O., Morand, S., Rolain, J. M. (2019). Co-Occurrence of variants of mcr-3 and mcr-8 genes in a Klebsiella pneumoniae isolate from Laos. Front. Microbiol. 102720. doi: 10.3389/fmicb.2019.02720
Hammerl, J. A., Borowiak, M., Schmoger, S., Shamoun, D., Grobbel, M., Malorny, B., et al. (2018). mcr-5 and a novel mcr-5.2 variant in Escherichia coli isolates from food and food-producing animals, Germany 2010 to 2017. J. Antimicrob. Chemother. 73, 1433–1435. doi: 10.1093/jac/dky020
Harper, M., Wright, A., St Michael, F., Li, J., Deveson Lucas, D., Ford, M., et al. (2017). Characterization of two novel lipopolysaccharide phosphoethanolamine transferases in Pasteurella multocida and their role in resistance to cathelicidin-2. Infect. Immun. 85, e00557-17. doi: 10.1128/IAI.00557-17
Harrison, P. W., Lower, R. P., Kim, N. K., Young, J. P. (2010). Introducing the bacterial 'chromid': not a chromosome, not a plasmid. Trends Microbiol. 18, 141–148. doi: 10.1016/j.tim.2009.12.010
Hatrongjit, R., Kerdsin, A., Takeuchi, D., Wongsurawat, T., Jenjaroenpun, P., Chopjitt, P., et al. (2020). Genomic analysis of Aeromonas veronii C198, a novel mcr-3.41-Harboring isolate from a patient with septicemia in Thailand. Pathogens 9, 1031. doi: 10.3390/pathogens9121031
Hu, Y., Fanning, S., Nguyen, S. V., Wang, W., Liu, C., Cui, X., et al. (2021). Emergence of a Salmonella enterica serovar typhimurium ST34 isolate, CFSA629, carrying a novel mcr-1.19 variant cultured from egg in China. J. Antimicrob. Chemother. 76, 1776–1785. doi: 10.1093/jac/dkab090
Imlay, J. A. (2003). Pathways of oxidative damage. Annu. Rev. Microbiol. 57, 395–418. doi: 10.1146/annurev.micro.57.030502.090938
Ito-Kagawa, M., Koyama, Y. (1980). Selective cleavage of a peptide antibiotic, colistin by colistinase. J. Antibiot (Tokyo) 33, 1551–1555. doi: 10.7164/antibiotics.33.1551
Jayol, A., Nordmann, P., Lehours, P., Poirel, L., Dubois, V. (2018). Comparison of methods for detection of plasmid-mediated and chromosomally encoded colistin resistance in enterobacteriaceae. Clin. Microbiol. Infect. 24, 175–179. doi: 10.1016/j.cmi.2017.06.002
Johnson, M., Zaretskaya, I., Raytselis, Y., Merezhuk, Y., McGinnis, S., Madden, T. L. (2008). NCBI BLAST: a better web interface. Nucleic Acids Res. 36, W5–W9. doi: 10.1093/nar/gkn201
Kalyaanamoorthy, S., Minh, B. Q., Wong, T. K. F., Von Haeseler, A., Jermiin, L. S. (2017). ModelFinder: fast model selection for accurate phylogenetic estimates. Nat. Methods 14, 587–589. doi: 10.1038/nmeth.4285
Kananizadeh, P., Tada, T., Oshiro, S., Hishinuma, T., Tohya, M., Uehara, Y., et al. (2022). Modified drug-susceptibility testing and screening culture agar for colistin-susceptible enterobacteriaceae isolates harboring a mobilized colistin resistance gene mcr-9. J. Clin. Microbiol. 60 (12), e0139922. doi: 10.1128/jcm.01399-22
Kelley, L. A., Mezulis, S., Yates, C. M., Wass, M. N., Sternberg, M. J. (2015). The Phyre2 web portal for protein modeling, prediction and analysis. Nat. Protoc. 10, 845–858. doi: 10.1038/nprot.2015.053
Khanawapee, A., Kerdsin, A., Chopjitt, P., Boueroy, P., Hatrongjit, R., Akeda, Y., et al. (2021). Distribution and molecular characterization of Escherichia coli harboring mcr genes isolated from slaughtered pigs in Thailand. Microb. Drug Resist. 27, 971–979. doi: 10.1089/mdr.2020.0242
Khedher, M. B., Baron, S. A., Riziki, T., Ruimy, R., Raoult, D., Diene, S. M., et al. (2020). Massive analysis of 64,628 bacterial genomes to decipher water reservoir and origin of mobile colistin resistance genes: is there another role for these enzymes? Sci. Rep. 10, 5970. doi: 10.1038/s41598-020-63167-5
Kieffer, N., Nordmann, P., Moreno, A. M., Zanolli Moreno, L., Chaby, R., Breton, A., et al. (2018). Genetic and functional characterization of an MCR-3-Like enzyme-producing Escherichia coli isolate recovered from swine in Brazil. Antimicrob. Agents Chemother. 62, e00278-18. doi: 10.1128/AAC.00278-18
Kieffer, N., Nordmann, P., Poirel, L. (2017). Moraxella species as potential sources of MCR-like polymyxin resistance determinants. Antimicrob. Agents Chemother. 61, e00129-17. doi: 10.1128/AAC.00129-17
Kosakovsky Pond, S. L., Poon, A. F. Y., Velazquez, R., Weaver, S., Hepler, N. L., Murrell, B., et al. (2020). HyPhy 2.5-a customizable platform for evolutionary hypothesis testing using phylogenies. Mol. Biol. Evol. 37, 295–299. doi: 10.1093/molbev/msz197
Kosakovsky Pond, S. L., Posada, D., Gravenor, M. B., Woelk, C. H., Frost, S. D. (2006). Automated phylogenetic detection of recombination using a genetic algorithm. Mol. Biol. Evol. 23, 1891–1901. doi: 10.1093/molbev/msl051
Krawczyk, P. S., Lipinski, L., Dziembowski, A. (2018). PlasFlow: predicting plasmid sequences in metagenomic data using genome signatures. Nucleic Acids Res. 46, e35. doi: 10.1093/nar/gkx1321
Le, S. Q., Gascuel, O. (2008). An improved general amino acid replacement matrix. Mol. Biol. Evol. 25, 1307–1320. doi: 10.1093/molbev/msn067
Leangapichart, T., Lunha, K., Jiwakanon, J., Angkititrakul, S., Jarhult, J. D., Magnusson, U., et al. (2021). Characterization of Klebsiella pneumoniae complex isolates from pigs and humans in farms in Thailand: population genomic structure, antibiotic resistance and virulence genes. J. Antimicrob. Chemother 76 (8), 2012–2016. doi: 10.1093/jac/dkab118
Lee, D. H., Cha, J. H., Kim, D. W., Lee, K., Kim, Y. S., Oh, H. Y., et al. (2022). Colistin-degrading proteases confer collective resistance to microbial communities during polymicrobial infections. Microbiome 10, 129. doi: 10.1186/s40168-022-01315-x
Lei, C. W., Zhang, Y., Wang, Y. T., Wang, H. N. (2020). Detection of mobile colistin resistance gene mcr-10.1 in a conjugative plasmid from Enterobacter roggenkampii of chicken origin in China. Antimicrob. Agents Chemother. 64, e01191-20. doi: 10.1128/AAC.01191-20
Letunic, I., Bork, P. (2007). Interactive tree of life (iTOL): an online tool for phylogenetic tree display and annotation. Bioinformatics 23, 127–128. doi: 10.1093/bioinformatics/btl529
Li, W., Godzik, A. (2006). Cd-hit: a fast program for clustering and comparing large sets of protein or nucleotide sequences. Bioinformatics 22, 1658–1659. doi: 10.1093/bioinformatics/btl158
Li, H., Yang, L., Liu, Z., Yin, W., Liu, D., Shen, Y., et al. (2018). Molecular insights into functional differences between mcr-3- and mcr-1-Mediated colistin resistance. Antimicrob. Agents Chemother. 62, e00366-18. doi: 10.1128/AAC.00366-18
Li, A., Yang, Y., Miao, M., Chavda, K. D., Mediavilla, J. R., Xie, X., et al. (2016). Complete sequences of mcr-1-Harboring plasmids from extended-Spectrum-beta-Lactamase- and carbapenemase-producing enterobacteriaceae. Antimicrob. Agents Chemother. 60, 4351–4354. doi: 10.1128/AAC.00550-16
Li, R., Zhang, P., Yang, X., Wang, Z., Fanning, S., Wang, J., et al. (2019). Identification of a novel hybrid plasmid coproducing MCR-1 and MCR-3 variant from an Escherichia coli strain. J. Antimicrob. Chemother. 74, 1517–1520. doi: 10.1093/jac/dkz058
Ling, Z., Yin, W., Li, H., Zhang, Q., Wang, X., Wang, Z., et al. (2017). Chromosome-mediated mcr-3 variants in Aeromonas veronii from chicken meat. Antimicrob. Agents Chemother. 61, e01272-17. doi: 10.1128/AAC.01272-17
Liu, L., Feng, Y., Zhang, X., Mcnally, A., Zong, Z. (2017). New variant of mcr-3 in an extensively drug-resistant Escherichia coli clinical isolate carrying mcr-1 and blaNDM-5. Antimicrob. Agents Chemother. 61, e01757-17. doi: 10.1128/AAC.01757-17
Liu, Y. Y., Wang, Y., Walsh, T. R., Yi, L. X., Zhang, R., Spencer, J., et al. (2016). Emergence of plasmid-mediated colistin resistance mechanism MCR-1 in animals and human beings in China: a microbiological and molecular biological study. Lancet Infect. Dis. 16, 161–168. doi: 10.1016/S1473-3099(15)00424-7
Liu, H., Zhu, B., Liang, B., Xu, X., Qiu, S., Jia, L., et al. (2018). A novel mcr-1 variant carried by an IncI2-type plasmid identified from a multidrug resistant enterotoxigenic Escherichia coli. Front. Microbiol. 9, 815. doi: 10.3389/fmicb.2018.00815
Long, H., Feng, Y., Ma, K., Liu, L., Mcnally, A., Zong, Z. (2019). The co-transfer of plasmid-borne colistin-resistant genes mcr-1 and mcr-3.5, the carbapenemase gene blaNDM-5 and the 16S methylase gene rmtB from Escherichia coli. Sci. Rep. 9, 696. doi: 10.1038/s41598-018-37125-1
Loose, M., Naber, K. G., Coates, A., Wagenlehner, F. M. E., Hu, Y. (2020). Effect of different media on the bactericidal activity of colistin and on the synergistic combination with azidothymidine against mcr-1-Positive colistin-resistant Escherichia coli. Front. Microbiol. 11, 54. doi: 10.3389/fmicb.2020.00054
Lu, X., Hu, Y., Luo, M., Zhou, H., Wang, X., Du, Y., et al. (2017). MCR-1.6, a new MCR variant carried by an IncP plasmid in a colistin-resistant Salmonella enterica serovar typhimurium isolate from a healthy individual. Antimicrob. Agents Chemother. 61, e02632-16. doi: 10.1128/AAC.02632-16
Martin, D. P., Varsani, A., Roumagnac, P., Botha, G., Maslamoney, S., Schwab, T., et al. (2021). RDP5: a computer program for analyzing recombination in, and removing signals of recombination from, nucleotide sequence datasets. Virus Evol. 7, veaa087. doi: 10.1093/ve/veaa087
Martinez-Servat, S., Yero, D., Huedo, P., Marquez, R., Molina, G., Daura, X., et al. (2018). Heterogeneous colistin-resistance phenotypes coexisting in Stenotrophomonas maltophilia isolates influence colistin susceptibility testing. Front. Microbiol. 9, 2871. doi: 10.3389/fmicb.2018.02871
Martins-Sorenson, N., Snesrud, E., Xavier, D. E., Cacci, L. C., Iavarone, A. T., Mcgann, P., et al. (2020). A novel plasmid-encoded mcr-4.3 gene in a colistin-resistant Acinetobacter baumannii clinical strain. J. Antimicrob. Chemother. 75, 60–64. doi: 10.1093/jac/dkz413
Milanese, A., Mende, D. R., Paoli, L., Salazar, G., Ruscheweyh, H. J., Cuenca, M., et al. (2019). Microbial abundance, activity and population genomic profiling with mOTUs2. Nat. Commun. 10, 1014. doi: 10.1038/s41467-019-08844-4
Minh, B. Q., Nguyen, M. A., Von Haeseler, A. (2013). Ultrafast approximation for phylogenetic bootstrap. Mol. Biol. Evol. 30, 1188–1195. doi: 10.1093/molbev/mst024
Moffatt, J. H., Harper, M., Harrison, P., Hale, J. D., Vinogradov, E., Seemann, T., et al. (2010). Colistin resistance in Acinetobacter baumannii is mediated by complete loss of lipopolysaccharide production. Antimicrob. Agents Chemother. 54, 4971–4977. doi: 10.1128/AAC.00834-10
Murrell, B., Moola, S., Mabona, A., Weighill, T., Sheward, D., Kosakovsky Pond, S. L., et al. (2013). FUBAR: a fast, unconstrained bayesian approximation for inferring selection. Mol. Biol. Evol. 30, 1196–1205. doi: 10.1093/molbev/mst030
Murrell, B., Wertheim, J. O., Moola, S., Weighill, T., Scheffler, K., Kosakovsky Pond, S. L. (2012). Detecting individual sites subject to episodic diversifying selection. PloS Genet. 8, e1002764. doi: 10.1371/journal.pgen.1002764
Nang, S. C., Li, J., Velkov, T. (2019). The rise and spread of mcr plasmid-mediated polymyxin resistance. Crit. Rev. Microbiol. 45, 131–161. doi: 10.1080/1040841X.2018.1492902
Neumann, B., Rackwitz, W., Hunfeld, K. P., Fuchs, S., Werner, G., Pfeifer, Y. (2020). Genome sequences of two clinical Escherichia coli isolates harboring the novel colistin-resistance gene variants mcr-1.26 and mcr-1.27. Gut Pathog. 12, 40. doi: 10.1186/s13099-020-00375-4
Ngbede, E. O., Poudel, A., Kalalah, A., Yang, Y., Adekanmbi, F., Adikwu, A. A., et al. (2020). Identification of mobile colistin resistance genes (mcr-1.1, mcr-5 and mcr-8.1) in enterobacteriaceae and Alcaligenes faecalis of human and animal origin, Nigeria. Int. J. Antimicrob. Agents 56, 106108. doi: 10.1016/j.ijantimicag.2020.106108
Nguyen, L. T., Schmidt, H. A., Von Haeseler, A., Minh, B. Q. (2015). IQ-TREE: a fast and effective stochastic algorithm for estimating maximum-likelihood phylogenies. Mol. Biol. Evol. 32, 268–274. doi: 10.1093/molbev/msu300
O'connor, A., Mcclean, S. (2017). The role of universal stress proteins in bacterial infections. Curr. Med. Chem. 24, 3970–3979. doi: 10.2174/0929867324666170124145543
Olaitan, A. O., Morand, S., Rolain, J. M. (2014). Mechanisms of polymyxin resistance: acquired and intrinsic resistance in bacteria. Front. Microbiol. 5, 643. doi: 10.3389/fmicb.2014.00643
Parks, D. H., Chuvochina, M., Rinke, C., Mussig, A. J., Chaumeil, P. A., Hugenholtz, P. (2022). GTDB: an ongoing census of bacterial and archaeal diversity through a phylogenetically consistent, rank normalized and complete genome-based taxonomy. Nucleic Acids Res. 50, D785–D794. doi: 10.1093/nar/gkab776
Parsons, J. B., Rock, C. O. (2013). Bacterial lipids: metabolism and membrane homeostasis. Prog. Lipid Res. 52, 249–276. doi: 10.1016/j.plipres.2013.02.002
Partridge, S. R., Di Pilato, V., Doi, Y., Feldgarden, M., Haft, D. H., Klimke, W., et al. (2018). Proposal for assignment of allele numbers for mobile colistin resistance (mcr) genes. J. Antimicrob. Chemother. 73, 2625–2630. doi: 10.1093/jac/dky262
Pettersen, E. F., Goddard, T. D., Huang, C. C., Meng, E. C., Couch, G. S., Croll, T. I., et al. (2021). UCSF ChimeraX: structure visualization for researchers, educators, and developers. Protein Sci. 30, 70–82. doi: 10.1002/pro.3943
Poirel, L., Kieffer, N., Fernandez-Garayzabal, J. F., Vela, A. I., Larpin, Y., Nordmann, P. (2017). MCR-2-mediated plasmid-borne polymyxin resistance most likely originates from Moraxella pluranimalium. J. Antimicrob. Chemother. 72, 2947–2949. doi: 10.1093/jac/dkx225
Poirel, L., Larpin, Y., Dobias, J., Stephan, R., Decousser, J. W., Madec, J. Y., et al. (2018). Rapid polymyxin NP test for the detection of polymyxin resistance mediated by the mcr-1/mcr-2 genes. Diagn. Microbiol. Infect. Dis. 90, 7–10. doi: 10.1016/j.diagmicrobio.2017.09.012
Purcell, A. B., Voss, B. J., Trent, M. S. (2022). Diacylglycerol kinase a is essential for polymyxin resistance provided by EptA, MCR-1, and other lipid a phosphoethanolamine transferases. J. Bacteriol 204, e0049821. doi: 10.1128/jb.00498-21
Raetz, C. R., Reynolds, C. M., Trent, M. S., Bishop, R. E. (2007). Lipid a modification systems in gram-negative bacteria. Annu. Rev. Biochem. 76, 295–329. doi: 10.1146/annurev.biochem.76.010307.145803
Ramaloko, W. T., Osei Sekyere, J. (2022). Phylogenomics, epigenomics, virulome and mobilome of gram-negative bacteria co-resistant to carbapenems and polymyxins: a one health systematic review and meta-analyses. Environ. Microbiol 24 (3), 1518–1542. doi: 10.1111/1462-2920.15930
Ransom, E. M., Potter, R. F., Dantas, G., Burnham, C. D. (2020). Genomic prediction of antimicrobial resistance: ready or not, here it comes! Clin. Chem. 66, 1278–1289. doi: 10.1093/clinchem/hvaa172
R Core Team (2021). R: a language and environment for statistical computing (Vienna, Austria: R Foundation for Statistical Computing). Available at: https://www.R-project.org/.
Rebelo, A. R., Bortolaia, V., Kjeldgaard, J. S., Pedersen, S. K., Leekitcharoenphon, P., Hansen, I. M., et al. (2018). Multiplex PCR for detection of plasmid-mediated colistin resistance determinants, mcr-1, mcr-2, mcr-3, mcr-4 and mcr-5 for surveillance purposes. Euro Surveill 23, 29–39. doi: 10.2807/1560-7917.ES.2018.23.6.17-00672
Reynolds, C. M., Kalb, S. R., Cotter, R. J., Raetz, C. R. (2005). A phosphoethanolamine transferase specific for the outer 3-deoxy-D-manno-octulosonic acid residue of Escherichia coli lipopolysaccharide. identification of the eptB gene and Ca2+ hypersensitivity of an eptB deletion mutant. J. Biol. Chem. 280, 21202–21211. doi: 10.1074/jbc.M500964200
Robert, X., Gouet, P. (2014). Deciphering key features in protein structures with the new ENDscript server. Nucleic Acids Res. 42, W320–W324. doi: 10.1093/nar/gku316
Rozas, J., Ferrer-Mata, A., Sanchez-Delbarrio, J. C., Guirao-Rico, S., Librado, P., Ramos-Onsins, S. E., et al. (2017). DnaSP 6: DNA sequence polymorphism analysis of Large data sets. Mol. Biol. Evol. 34, 3299–3302. doi: 10.1093/molbev/msx248
Sabnis, A., Hagart, K. L., Klockner, A., Becce, M., Evans, L. E., Furniss, R. C. D., et al. (2021). Colistin kills bacteria by targeting lipopolysaccharide in the cytoplasmic membrane. Elife 10, e65836. doi: 10.7554/eLife.65836.sa2
Samantha, A., Vrielink, A. (2020). Lipid a phosphoethanolamine transferase: regulation, structure and immune response. J. Mol. Biol. 432, 5184–5196. doi: 10.1016/j.jmb.2020.04.022
Schlegel, S., Lofblom, J., Lee, C., Hjelm, A., Klepsch, M., Strous, M., et al. (2012). Optimizing membrane protein overexpression in the Escherichia coli strain Lemo21(DE3). J. Mol. Biol. 423, 648–659. doi: 10.1016/j.jmb.2012.07.019
Shen, Y., Xu, C., Sun, Q., Schwarz, S., Ou, Y., Yang, L., et al. (2018). Prevalence and genetic analysis of mcr-3-Positive Aeromonas species from humans, retail meat, and environmental water samples. Antimicrob. Agents Chemother. 62, e00404-18. doi: 10.1128/AAC.00404-18
Snesrud, E., Mcgann, P., Chandler, M. (2018). The birth and demise of the ISApl1-mcr-1-ISApl1 composite transposon: the vehicle for transferable colistin resistance. mBio 9, e02381-17. doi: 10.1128/mBio.02381-17
Snesrud, E., Ong, A. C., Corey, B., Kwak, Y. I., Clifford, R., Gleeson, T., et al. (2017). Analysis of serial isolates of mcr-1-Positive Escherichia coli reveals a highly active ISApl1 transposon. Antimicrob. Agents Chemother. 61, e00056-17. doi: 10.1128/AAC.00056-17
Snyman, Y., Whitelaw, A. C., Barnes, J. M., Maloba, M. R. B., Newton-Foot, M. (2021). Characterisation of mobile colistin resistance genes (mcr-3 and mcr-5) in river and storm water in regions of the Western cape of south Africa. Antimicrob. Resist. Infect. Control 10, 96. doi: 10.1186/s13756-021-00963-2
Son, S. J., Huang, R., Squire, C. J., Leung, I. K. H. (2019). MCR-1: a promising target for structure-based design of inhibitors to tackle polymyxin resistance. Drug Discov. Today 24, 206–216. doi: 10.1016/j.drudis.2018.07.004
Soubrier, J., Steel, M., Lee, M. S. Y., Der Sarkissian, C., Guindon, S., Ho, S. Y. W., et al. (2012). The influence of rate heterogeneity among sites on the time dependence of molecular rates. Mol. Biol. Evol. 29, 3345–3358. doi: 10.1093/molbev/mss140
Stamatakis, A. (2014). RAxML version 8: a tool for phylogenetic analysis and post-analysis of large phylogenies. Bioinformatics 30, 1312–1313. doi: 10.1093/bioinformatics/btu033
Stogios, P. J., Cox, G., Zubyk, H. L., Evdokimova, E., Wawrzak, Z., Wright, G. D., et al. (2018). Substrate recognition by a colistin resistance enzyme from Moraxella catarrhalis. ACS Chem. Biol. 13, 1322–1332. doi: 10.1021/acschembio.8b00116
Stojanoski, V., Sankaran, B., Prasad, B. V., Poirel, L., Nordmann, P., Palzkill, T. (2016). Structure of the catalytic domain of the colistin resistance enzyme MCR-1. BMC Biol. 14, 81. doi: 10.1186/s12915-016-0303-0
Stosic, M. S., Leangapichart, T., Lunha, K., Jiwakanon, J., Angkititrakul, S., Jarhult, J. D., et al. (2021). Novel mcr-3.40 variant co-located with mcr-2.3 and blaCTX-M-63 on an IncHI1B/IncFIB plasmid found in Klebsiella pneumoniae from a healthy carrier in Thailand. J. Antimicrob. Chemother. 76 (8), 2218–2220. doi: 10.1093/jac/dkab147
Sun, J., Xu, Y., Gao, R., Lin, J., Wei, W., Srinivas, S., et al. (2017). Deciphering MCR-2 colistin resistance. mBio 8, e00625-17. doi: 10.1128/mBio.00625-17
Tamayo, R., Choudhury, B., Septer, A., Merighi, M., Carlson, R., Gunn, J. S. (2005a). Identification of cptA, a PmrA-regulated locus required for phosphoethanolamine modification of the Salmonella enterica serovar typhimurium lipopolysaccharide core. J. Bacteriol. 187, 3391–3399. doi: 10.1128/JB.187.10.3391-3399.2005
Tamayo, R., Prouty, A. M., Gunn, J. S. (2005b). Identification and functional analysis of Salmonella enterica serovar typhimurium PmrA-regulated genes. FEMS Immunol. Med. Microbiol. 43, 249–258. doi: 10.1016/j.femsim.2004.08.007
Tatusova, T., Dicuccio, M., Badretdin, A., Chetvernin, V., Nawrocki, E. P., Zaslavsky, L., et al. (2016). NCBI prokaryotic genome annotation pipeline. Nucleic Acids Res. 44, 6614–6624. doi: 10.1093/nar/gkw569
Teo, J. W. P., Kalisvar, M., Venkatachalam, I., Ng, O. T., Lin, R. T. P., Octavia, S. (2018). mcr-3 and mcr-4 variants in carbapenemase-producing clinical enterobacteriaceae do not confer phenotypic polymyxin resistance. J. Clin. Microbiol. 56, e01562-17. doi: 10.1128/JCM.01562-17
Tietgen, M., Semmler, T., Riedel-Christ, S., Kempf, V., Molinaro, A., Ewers, C., et al. (2018). Impact of the colistin resistance gene mcr-1 on bacterial fitness. Int. J. Antimicrob. Agents 51, 554–561. doi: 10.1016/j.ijantimicag.2017.11.011
Tijet, N., Faccone, D., Rapoport, M., Seah, C., Pasteran, F., Ceriana, P., et al. (2017). Molecular characteristics of mcr-1-carrying plasmids and new mcr-1 variant recovered from polyclonal clinical Escherichia coli from Argentina and Canada. PloS One 12, e0180347. doi: 10.1371/journal.pone.0180347
Touati, D. (2000). Iron and oxidative stress in bacteria. Arch. Biochem. Biophys. 373, 1–6. doi: 10.1006/abbi.1999.1518
Trebosc, V., Gartenmann, S., Totzl, M., Lucchini, V., Schellhorn, B., Pieren, M., et al. (2019). Dissecting colistin resistance mechanisms in extensively drug-resistant Acinetobacter baumannii clinical isolates. mBio 10, e01083-19. doi: 10.1128/mBio.01083-19
Trent, M. S. (2004). Biosynthesis, transport, and modification of lipid a. Biochem. Cell Biol. 82, 71–86. doi: 10.1139/o03-070
Trimble, M. J., Mlynarcik, P., Kolar, M., Hancock, R. E. (2016). Polymyxin: alternative mechanisms of action and resistance. Cold Spring Harb. Perspect. Med. 6, 22. doi: 10.1101/cshperspect.a025288
Troudi, A., Pages, J. M., Brunel, J. M. (2021). Chemical highlights supporting the role of lipid a in efficient biological adaptation of gram-negative bacteria to external stresses. J. Med. Chem. 64, 1816–1834. doi: 10.1021/acs.jmedchem.0c02185
Tyson, G. H., Mcdermott, P. F., Li, C., Chen, Y., Tadesse, D. A., Mukherjee, S., et al. (2015). WGS accurately predicts antimicrobial resistance in Escherichia coli. J. Antimicrob. Chemother. 70, 2763–2769. doi: 10.1093/jac/dkv186
Uddin, M. B., Hossain, S. M. B., Hasan, M., Alam, M. N., Debnath, M., Begum, R., et al. (2021). Multidrug antimicrobial resistance and molecular detection of mcr-1 gene in Salmonella species isolated from chicken. Anim. (Basel) 11, 206. doi: 10.3390/ani11010206
Vaara, M., Vaara, T., Jensen, M., Helander, I., Nurminen, M., Rietschel, E. T., et al. (1981). Characterization of the lipopolysaccharide from the polymyxin-resistant pmrA mutants of Salmonella typhimurium. FEBS Lett. 129, 145–149. doi: 10.1016/0014-5793(81)80777-6
Wagner, S., Klepsch, M. M., Schlegel, S., Appel, A., Draheim, R., Tarry, M., et al. (2008). Tuning Escherichia coli for membrane protein overexpression. Proc. Natl. Acad. Sci. U.S.A. 105, 14371–14376. doi: 10.1073/pnas.0804090105
Wang, C., Feng, Y., Liu, L., Wei, L., Kang, M., Zong, Z. (2020). Identification of novel mobile colistin resistance gene mcr-10. Emerg. Microbes Infect. 9, 508–516. doi: 10.1080/22221751.2020.1732231
Wang, Y., Hou, N., Rasooly, R., Gu, Y., He, X. (2021). Prevalence and genetic analysis of chromosomal mcr-3/7 in Aeromonas from U.S. animal-derived samples. Front. Microbiol. 12, 667406. doi: 10.3389/fmicb.2021.667406
Wang, R., Van Dorp, L., Shaw, L. P., Bradley, P., Wang, Q., Wang, X., et al. (2018a). The global distribution and spread of the mobilized colistin resistance gene mcr-1. Nat. Commun. 91179. doi: 10.1101/220921
Wang, X., Wang, Y., Zhou, Y., Li, J., Yin, W., Wang, S., et al. (2018b). Emergence of a novel mobile colistin resistance gene, mcr-8, in NDM-producing Klebsiella pneumoniae. Emerg. Microbes Infect. 7, 122. doi: 10.1038/s41426-018-0124-z
Wang, X., Wang, Y., Zhou, Y., Wang, Z., Wang, Y., Zhang, S., et al. (2019). Emergence of colistin resistance gene mcr-8 and its variant in Raoultella ornithinolytica. Front. Microbiol. 10, 228. doi: 10.3389/fmicb.2019.00228
Wang, X., Zhai, W., Li, J., Liu, D., Zhang, Q., Shen, Z., et al. (2018c). Presence of an mcr-3 variant in Aeromonas caviae, Proteus mirabilis, and Escherichia coli from one domestic duck. Antimicrob. Agents Chemother. 62, 3. doi: 10.1128/AAC.02106-17
Weaver, S., Shank, S. D., Spielman, S. J., Li, M., Muse, S. V., Kosakovsky Pond, S. L. (2018). Datamonkey 2.0: a modern web application for characterizing selective and other evolutionary processes. Mol. Biol. Evol. 35, 773–777. doi: 10.1093/molbev/msx335
Wise, M. G., Estabrook, M. A., Sahm, D. F., Stone, G. G., Kazmierczak, K. M. (2018). Prevalence of mcr-type genes among colistin-resistant enterobacteriaceae collected in 2014-2016 as part of the INFORM global surveillance program. PloS One 13, e0195281. doi: 10.1371/journal.pone.0195281
Winter, D. J. (2017). rentrez: an r package for the NCBI eUtils API. R J. 9 (2), 520–526. doi: 10.32614/RJ-2017-058
World Health Organization. (2019). Critically important antimicrobials for human medicine, 6th revision (Geneva: World Health Organization).
World Health Organization. (2021). GLASS: the detection and reporting of colistin resistance, second edition (Geneva: World Health Organization).
Xavier, B. B., Lammens, C., Ruhal, R., Kumar-Singh, S., Butaye, P., Goossens, H., et al. (2016). Identification of a novel plasmid-mediated colistin-resistance gene, mcr-2, in Escherichia coli, Belgium, June 2016. Euro Surveill 21. doi: 10.2807/1560-7917.ES.2016.21.27.30280
Xiang, R., Liu, B. H., Zhang, A. Y., Lei, C. W., Ye, X. L., Yang, Y. X., et al. (2018). Colocation of the polymyxin resistance gene mcr-1 and a variant of mcr-3 on a plasmid in an Escherichia coli isolate from a chicken farm. Antimicrob. Agents Chemother. 62, e00501-18. doi: 10.1128/AAC.00501-18
Xu, Y., Lin, J., Cui, T., Srinivas, S., Feng, Y. (2018a). Mechanistic insights into transferable polymyxin resistance among gut bacteria. J. Biol. Chem. 293, 4350–4365. doi: 10.1074/jbc.RA117.000924
Xu, Y., Wei, W., Lei, S., Lin, J., Srinivas, S., Feng, Y. (2018b). An evolutionarily conserved mechanism for intrinsic and transferable polymyxin resistance. mBio 9, e02317-17. doi: 10.1128/mBio.02317-17
Xu, Y., Zhong, L. L., Srinivas, S., Sun, J., Huang, M., Paterson, D. L., et al. (2018c). Spread of MCR-3 colistin resistance in China: an epidemiological, genomic and mechanistic study. EBioMedicine 34, 139–157. doi: 10.1016/j.ebiom.2018.07.027
Yang, Z. (1995). A space-time process model for the evolution of DNA sequences. Genetics 139, 993–1005. doi: 10.1093/genetics/139.2.993
Yang, Y. Q., Li, Y. X., Lei, C. W., Zhang, A. Y., Wang, H. N. (2018). Novel plasmid-mediated colistin resistance gene mcr-7.1 in Klebsiella pneumoniae. J. Antimicrob. Chemother. 73, 1791–1795. doi: 10.1093/jac/dky111
Yang, Y. Q., Li, Y. X., Song, T., Yang, Y. X., Jiang, W., Zhang, A. Y., et al. (2017). Colistin resistance gene mcr-1 and its variant in Escherichia coli isolates from chickens in China. Antimicrob. Agents Chemother. 61, e01204-16. doi: 10.1128/AAC.01204-16
Yang, X., Liu, L., Wang, Z., Bai, L., Li, R. (2019). Emergence of mcr-8.2-bearing Klebsiella quasipneumoniae of animal origin. J. Antimicrob. Chemother. 74, 2814–2817. doi: 10.1093/jac/dkz213
Yin, W., Li, H., Shen, Y., Liu, Z., Wang, S., Shen, Z., et al. (2017). Novel plasmid-mediated colistin resistance gene mcr-3 in Escherichia coli. mBio 8, e00543-17. doi: 10.1128/mBio.00543-17
Yu, L., Kitagawa, H., Kayama, S., Hisatsune, J., Ohge, H., Sugai, M. (2021). Complete genome sequence of Aeromonas caviae strain MS6064, a mcr-3-Carrying clinical isolate from Japan. Microbiol. Resour Announc 10, e01037-20. doi: 10.1128/MRA.01037-20
Yu, Z., Qin, W., Lin, J., Fang, S., Qiu, J. (2015). Antibacterial mechanisms of polymyxin and bacterial resistance. BioMed. Res. Int. 2015, 679109. doi: 10.1155/2015/679109
Yuan, Y., Li, Y., Wang, G., Li, C., Xiang, L., She, J., et al. (2019). Coproduction of MCR-9 and NDM-1 by colistin-resistant Enterobacter hormaechei isolated from bloodstream infection. Infect. Drug Resist. 12, 2979–2985. doi: 10.2147/IDR.S217168
Yusuf, E., Van Westreenen, M., Goessens, W., Croughs, P. (2020). The accuracy of four commercial broth microdilution tests in the determination of the minimum inhibitory concentration of colistin. Ann. Clin. Microbiol. Antimicrob. 19, 42. doi: 10.1186/s12941-020-00383-x
Zankari, E., Hasman, H., Cosentino, S., Vestergaard, M., Rasmussen, S., Lund, O., et al. (2012). Identification of acquired antimicrobial resistance genes. J. Antimicrob. Chemother. 67, 2640–2644. doi: 10.1093/jac/dks261
Zhang, P., Bai, L., Li, Y., Wang, Z., Li, R. (2019). Loss of mcr genes mediated by plasmid elimination and ISApl1. Antimicrob. Agents Chemother. 63, e01002-19. doi: 10.1128/AAC.01002-19
Zhao, F., Feng, Y., Lu, X., Mcnally, A., Zong, Z. (2017). Remarkable diversity of Escherichia coli carrying mcr-1 from hospital sewage with the identification of two new mcr-1 variants. Front. Microbiol. 8, 2094. doi: 10.3389/fmicb.2017.02094
Zhao, Y., Meng, Q., Lai, Y., Wang, L., Zhou, D., Dou, C., et al. (2019). Structural and mechanistic insights into polymyxin resistance mediated by EptC originating from Escherichia coli. FEBS J. 286, 750–764. doi: 10.1111/febs.14719
Keywords: MCR, colistin, phosphoethanolamine transferase, antimicrobial resistance, horizontal gene transfer, mobile genetic element, plasmid
Citation: Gaballa A, Wiedmann M and Carroll LM (2023) More than mcr: canonical plasmid- and transposon-encoded mobilized colistin resistance genes represent a subset of phosphoethanolamine transferases. Front. Cell. Infect. Microbiol. 13:1060519. doi: 10.3389/fcimb.2023.1060519
Received: 03 October 2022; Accepted: 19 May 2023;
Published: 08 June 2023.
Edited by:
John Osei Sekyere, University of Pretoria, South AfricaReviewed by:
Nayeem Ahmad, Arabian Gulf University, BahrainYongchang Xu, Zhejiang University, China
Lujie Liang, Sun Yat-sen University, China
Jens Andre Hammerl, Bundesinstitut für Risikobewertung, Germany
Francesco Imperi, Roma Tre University, Italy
Copyright © 2023 Gaballa, Wiedmann and Carroll. This is an open-access article distributed under the terms of the Creative Commons Attribution License (CC BY). The use, distribution or reproduction in other forums is permitted, provided the original author(s) and the copyright owner(s) are credited and that the original publication in this journal is cited, in accordance with accepted academic practice. No use, distribution or reproduction is permitted which does not comply with these terms.
*Correspondence: Laura M. Carroll, bGF1cmEuY2Fycm9sbEB1bXUuc2U=