- 1Graduate School, Hunan University of Chinese Medicine, Changsha, China
- 2Department of Nursing, The First Affiliated Hospital of Hunan University of Chinese Medicine, Changsha, China
Background: Evidence of changes in the composition and function of the gut microbiota (GM) in post-stroke depression (PSD) patients is gradually accumulating. This study aimed to systematically evaluate the relationship between PSD and GM.
Methods: We searched in PubMed, Web of Science, Embase, Cochrane databases, Wangfang, VIP, CBM, and CNKI from the establishment of the database to April 17, 2024, and systematic review and meta-analysis were performed to investigate the differences of GM between patients with PSD spectrum and healthy controls (HC) or stroke spectrum.
Result: There were 14 studies consisting a total of 1,556 individuals included in the meta-analysis. The pooled results showed that PSD spectrum demonstrated significantly increased α diversity as indexed by Chao1 index, ACE indexes, Shannon index, and Simpson index as compared to HC. Additionally, stroke spectrum significantly increased α diversity as indexed by Simpson index compared to PSD. Furthermore, the pooled estimation of relative abundance showed that Bacteroidota, Fusobacteriota, and Pseudomonadota in PSD patients were significantly higher than those in the HC group, while the abundance of Bacillota was higher in the HC group. Moreover, significant differences in GM were observed between PSD patients and HC at the family and genus levels.
Conclusion: This study found that the α diversity of PSD patients was higher than that of HC. Moreover, there were also differences in the distribution of GM at the phylum, family, and genus levels, respectively. At the same time, the level of Lachnospira in PSD patients was lower than that in the stroke group.
Systematic review registration: https://www.crd.york.ac.uk/PROSPERO/, identifier CRD42024582708.
Introduction
Depression and cerebrovascular accident (stroke) are two major causes of socioeconomic burden (Medeiros et al., 2020). The latest report from the World Health Organization (WHO) states that depression is the leading cause of disability worldwide (WHO, 2018a), while stroke often ranks among the top three causes of disease burden (WHO, 2012; WHO, 2018b). The sequelae caused by tissue damage in stroke patients are not easily alleviated over time (Becker, 2016), leading to a variety of lifelong sequelae (Cha et al., 2022), including sensory impairment, motor impairment, cognitive impairment, and swallowing disorders. Post-stroke depression (PSD) is of substantial concern due to its high morbidity, high disability rate, and poor response to treatment (Robinson and Jorge, 2016; Das J, 2018; Guo et al., 2022). It is the most frequent and burdensome neuropsychiatric complication following a stroke (Shi et al., 2017; Taylor-Rowan et al., 2019). Its clinical features include a depressed mood, loss of interest, and increased fatigue (Sun et al., 2024). Many factors contribute to the occurrence of PSD, including the history of depression, stroke severity, lesion location, and so on (Guo et al., 2022). Unfortunately, we lack a unified mechanism to explain PSD, the mechanisms of which now involve dysregulation of hypothalamic–pituitary–adrenal (HPA) axis, increased inflammatory factors, decreased levels of monoamines, glutamate-mediated excitotoxicity, and abnormal neurotrophic response (Guo et al., 2022).
In recent decades, the fields of microbiology and neuroscience have become increasingly intertwined. Bidirectional communication along the gut–brain axis is a fundamental mechanism for the development of gut–brain signaling pathways that link the microbiota with the host, influencing brain function and behavior (Rhee et al., 2009; Grenham et al., 2011; Cryan and Dinan, 2012; Mayer et al., 2015; McVey Neufeld et al., 2015; Dinan and Cryan, 2017). Recent research has shifted focus from gut–brain communication related to digestive function and satiety (Taché et al., 1980; Konturek et al., 2004; Berthoud, 2008) to its impact on higher-order cognition and psychological effects (Rhee et al., 2009; Carabotti et al., 2015; Sarkar et al., 2016; Agustí et al., 2018). The link between gut microbiota (GM) and central nervous system disorders, including Parkinson’s disease, cerebrovascular diseases, Alzheimer’s disease, autism spectrum disorder, and depression, has become a significant area of research. This has led to new insights into the pathogenesis and potential treatments for these central nervous system conditions (Cryan et al., 2020; Socała et al., 2021).
A large number of studies have shown that abnormal gut–brain networks not only exacerbate gut inflammatory disorders (Mayer et al., 2015; Bernstein, 2017; Breit et al., 2018) and altered acute and chronic stress responses (Dinan and Cryan, 2017; Foster et al., 2017; Marin et al., 2017; Gao et al., 2018; Maniscalco and Rinaman, 2018; Partrick et al., 2018; Sun et al., 2018; Provensi et al., 2019) but also affect behavioral states such as emotional well-being and motivated behavior, particularly anxiety-like behavior (Dinan and Cryan, 2017; Arentsen et al., 2018; Heintz-Buschart et al., 2018; Jaglin et al., 2018; Luk et al., 2018; Maniscalco and Rinaman, 2018; Fouhy et al., 2019). Evidence indicates that the composition and function of the GM are altered in patients with PSD (Jiang et al., 2021), suggesting a link between changes in intestinal flora and PSD. Therefore, exploring the brain–gut–microbiota axis may uncover fundamental mechanisms of PSD and contribute to the development of new antidepressant therapies. However, due to small sample sizes and inconsistent detection methods, most current studies on the GM in PSD exhibit considerable heterogeneity.
In this study, we conducted a meta-analysis of research comparing the GM between PSD patients and healthy controls or stroke groups. We searched eight public databases, extracting relevant data directly or indirectly from the articles. Finally, we evaluated the pooled differences in GM composition between PSD patients and the comparison groups.
Materials and methods
Search strategy
For the collection of eligible studies, we systematically and comprehensively searched in PubMed, Web of Science, Embase, Cochrane databases, Wangfang, VIP, CBM, and CNKI from the establishment of the database to April 17, 2024, with no language restriction. A detailed search strategy was developed using Medical Subject Headings (MeSH) and free terms. The MeSH words were as follows: “stroke”, “depression”, and “gastrointestinal microbiome”. This meta-analysis was conducted on the basis of the Preferred Reporting Items for Systematic Reviews and Meta-analyses (PRISMA) criteria (Page et al., 2021).Taking PubMed as an example, the search strategy is shown in Supplementary Table S1.
Inclusion and exclusion criteria
Eligible studies were identified based on the following criteria (1): comparison of GM diversity and abundance between patients with PSD and healthy controls (HC) or stroke patients (2), GM samples were derived from stool (3), availability of adequate statistical data (e.g., mean, standard deviation, p-values, median, maximum, minimum, etc.) to estimate effect sizes, and (4) access to the full text. Case reports, systematic reviews, and animal research were excluded.
Outcome measures
The primary outcomes consisted of GM diversity (including α diversity and β diversity) and differences of GM abundance between the patients with PSD and HC or stroke.
Data extraction
For each eligible study, the following information was collected: the first authors’ name, date of publication, region, sample size, detection method, and richness and diversity indices from 16S rRNA sequencing data, including relative abundance of GM. Median, minimum, maximum, or 95% confidence intervals (CI) were estimated from histograms and box plots (Egger et al., 1997; Zuo et al., 2019; Huang et al., 2021; Kang et al., 2021; Li et al., 2021; Yao et al., 2023). Two authors (LQL and WXQ) independently extracted and assessed the data, with any disagreements resolved through consensus between the reviewers.
Quality assessment
The quality and risk of bias were assessed by two authors (LQL and WXQ) independently. For quality assessment of cross-sectional studies, the Agency for Healthcare Research and Quality (AHRQ) criteria (Zeng et al., 2015) were used (Ma et al., 2023), which include 11 items scored as “yes”, “no”, or “unclear”. The total score ranges from 0 to 11 points, with scores of 0–3 indicating low quality, scores of 4–7 indicating medium quality, and scores greater than 8 indicating high quality (Zeng et al., 2015). Cohort studies were evaluated using the Newcastle–Ottawa Scale (NOS) (Ma et al., 2023), which has a maximum score of 9. Scores of 1 to 3 are classified as low quality, 4 to 6 as moderate quality, and 7 to 9 as high quality (Stang, 2010).
Risk of bias assessment
For the risk of bias assessment, the Revised Risk of Bias Assessment Tool for Nonrandomized Studies (RoBANS 2) was used for each included study (Seo et al., 2023). This tool evaluates eight potential sources of bias: comparability of the target group, target group selection, confounders, measurement of intervention/exposure, blinding of assessors, outcome assessment, incomplete outcome data, and selective outcome reporting. Disagreements were resolved through consensus or by consulting with a third author.
Effect size calculations
The Comprehensive Meta-Analysis Version 3 software (Biostat Inc., Englewood, NJ, USA) was applied to calculate the effect sizes with a random-effects, inverse-variance weighted model. Assuming that deviations from Gaussian distributions were minimal, we applied previously reported conversion equations (Hozo et al., 2005) to estimate means and standard deviation from median, maximum, and minimum. Hedges’ g effect sizes were calculated from the mean differences between PSD and HC or stroke groups, divided by the pooled standard deviation. Heterogeneity across studies was assessed using Q-statistic and I2 statistic. Additionally, the presence of outliers could introduce bias and significantly affect the pooled effect sizes (Viechtbauer and Cheung, 2010; Noma et al., 2020). Outliers were defined by the following criteria (Medeiros et al., 2020): for which the upper boundary of the 95% CI was lower than the lower boundary of the overall effect CI (i.e., extremely small effect sizes) and (WHO, 2018a) for which the lower boundary of the 95% CI was higher than the upper boundary of the overall effect CI (i.e., extremely large effect sizes). To assess the robustness of our findings, we performed sensitivity analyses by sequentially excluding each study and recalculating the pooled effect size.
The potential publication bias of each GM abundance was quantitatively assessed by using Begg and Mazumdar rank correlation (Begg and Mazumdar, 1994) and Egger’s regression intercept tests (Egger et al., 1997). Moreover, the Duval and Tweedie’s trim and fill method was used to correct for non-normal distribution of effect sizes potentially due to the file drawer problem. The significant levels were set at p <0.05.
Results
Characteristics of eligible studies
A flow chart of the literature search and selection process is shown in Figure 1. A total of 314 relevant articles were retrieved from the eight databases, with 128 being duplicates. Based on the titles and abstracts, 169 articles were excluded for not meeting the eligibility criteria. Subsequently, two studies were excluded due to unavailable full texts and one due to the lack of relevant outcomes, leaving 17 articles. Finally, 14 eligible articles were included in the meta-analysis (Fan et al., 2016; Sun, 2019; Zuo et al., 2019; Ling et al., 2020; Han, 2021; Huang et al., 2021; Kang et al., 2021; Li et al., 2021; Guo et al., 2022; Huang et al., 2022; Li et al., 2022; Qin et al., 2022; Wang, 2023; Yao et al., 2023). In totally, 1,556 individuals were included, comprising 702 patients with PSD, 512 with stroke, and 342 HC. Detailed information on the eligible articles is presented in Table 1. The 14 included studies were all conducted in China across nine provinces, with nine published in Chinese and five in English.
The GM of 12 eligible studies was analyzed using high-throughput sequencing of the region of V3 or V3–V4 of the 16S rRNA gene. Specifically, Weicui Huang et al. detected and reported 12 components in GM using the Japanese Mitsuoka method (Huang et al., 2022). Yi Kang et al. only reported the presence of Enterococcus faecalis, Escherichia coli, and Bifidobacterium in feces using the ATB-expression semi-automatic microbial detection system (Kang et al., 2021). The methodological quality assessment revealed that seven studies (Zuo et al., 2019; Han, 2021; Guo et al., 2022; Li et al., 2022; Qin et al., 2022; Wang, 2023; Yao et al., 2023) had high quality, while the remaining seven (Fan et al., 2016; Sun, 2019; Ling et al., 2020; Huang et al., 2021; Kang et al., 2021; Li et al., 2021; Huang et al., 2022) were of fair quality. Details are provided in Supplementary Tables S2-1 and S2-2.
Primary outcomes: α diversity and β diversity
Among the indices of α diversity, Chao1 index, ACE indexes, Shannon index, and Simpson index were most frequently measured in the included studies. Heterogeneity testing was performed on these indices, with the results shown in Table 2. When comparing the α diversity between PSD spectrum and stroke groups, the heterogeneity test for the Chao1 index indicated I² = 59.287, while the Q test result showed that P >0.05. Consequently, a fixed-effects model was used for analysis. ACE index, Shannon index, and Simpson index exhibited low heterogeneity and were also analyzed using the fixed-effects model. For comparisons of α diversity between PSD spectrum and HC, the evenness index showed I2 = 54.933, with the Q test result indicating P >0.05; thus, a fixed-effects model was applied. However, Chao1 index, ACE index, Shannon index, and Simpson index all demonstrated high heterogeneity. Therefore, a random-effects model was used for these analyses. Simultaneously, moderation effect analysis was conducted to investigate the sources of heterogeneity among the research results. After excluding a study with a sample size of less than 50, Chao1 index, ACE indexes, and Shannon index between PSD spectrum and HC changes were substantial in the effect size, suggesting a large and statistically significant effect. Nevertheless, the effect sizes of other indexes did not show obvious changes (Supplementary Table S5).
In the comparison of α diversity between PSD spectrum and stroke, only the Simpson index showed a statistical difference (Supplementary Figure S1). Specifically, the stroke spectrum demonstrated significantly increased α diversity as indicated by the Simpson index (Hedges’ g = -0.365; 95% CI = -0.672 to -0.059; p = 0.020; n = 3). However, when comparing the PSD spectrum and HC and after removing several outlier results (Figure 2), the PSD spectrum demonstrated significantly increased α diversity as indicated by the Chao1 index (Hedges’ g = 1.669; 95% CI = 0.928 to 2.410; p < 0.001; n = 4), ACE indexes (Hedges’ g = 0.795; 95% CI = 0.203 to 1.386; p = 0.008; n = 5), Shannon index (Hedges’ g = 0.273; 95% CI = 0.048 to 0.498; p = 0.018; n = 4), and Simpson index (Hedges’ g = 0.664; 95% CI = 0.057 to 1.271; p = 0.032; n = 5). Additionally, the results of the three studies that compared the evenness index between the PSD spectrum and HC showed no significant differences between the two groups.
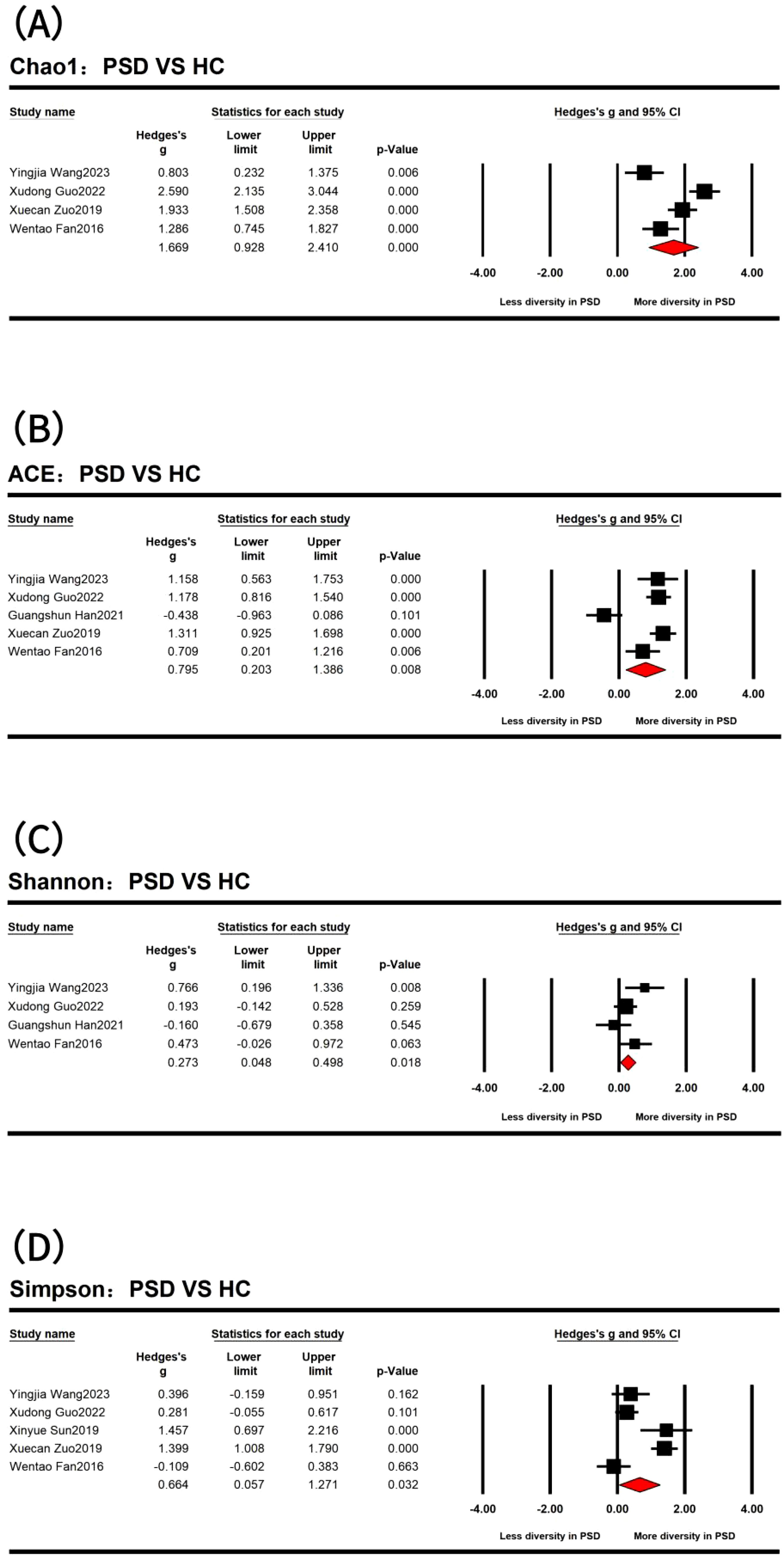
Figure 2. Forest plots of Chao1 index (A), ACE indexes (B), Shannon index (C), and Simpson index (D) in the comparisons between post-stroke depression (PSD) and healthy controls (HC) spectrum.
Only four (Ling et al., 2020; Huang et al., 2021; Li et al., 2022; Wang, 2023) of the included articles assessed β diversity (Table 3). The principal coordinate analyses were based on weighted UniFrac distance, Bray–Curtis dissimilarity, and partial least squares discriminant analysis (PLS-DA). Regarding differences between PSD and stroke patients, three studies found significant differences (Huang et al., 2021; Li et al., 2022; Wang, 2023), while one study (Ling et al., 2020) did not. Additionally, two (Li et al., 2022; Wang, 2023) studies reported significant differences between the PSD spectrum and HC. In summary, the findings across the included studies were inconsistent.
Primary outcomes: differences in microbial composition at the phylum level
At the phylum level (Figure 3), the results indicated that four dominant bacterial phyla were identified across 273 PSD spectrum and 244 HC in five studies (Fan et al., 2016; Sun, 2019; Zuo et al., 2019; Li et al., 2021; Guo et al., 2022). Specifically, the analyses of 240 PSD spectrum and 234 HC from four studies (Fan et al., 2016; Zuo et al., 2019; Li et al., 2021; Guo et al., 2022) revealed a significantly higher abundance of Bacteroidota (Bacteroidetes) (Hedges’ g = 0.405; 95% CI = 0.224 to 0.586; p < 0.001; n = 4) and Fusobacteriota (Fusobacteria) (Hedges’ g = 1.994; 95% CI = 1.317 to 2.671; p < 0.001; n = 4) in the PSD spectrum compared to HC. The remaining two dominant bacterial phyla were Pseudomonadota (Proteobacteria) and Bacillota (Firmicutes), with significant statistical differences observed in the five studies. Specifically, Pseudomonadota was more abundant in the PSD spectrum (Hedges’ g = 1.216; 95% CI = 1.027 to 1.405; p < 0.001; n = 5), while Bacillota was significantly less abundant in the PSD spectrum (Hedges’ g = -0.935; 95% CI = -1.292 to -0.579; p < 0.001; n = 5). However, no meaningful phylum-level differences were observed between PSD spectrum and stroke in the included studies. The heterogeneity test results showed that the I2 of Bacteroidota and Pseudomonadota was <75%, besides the Q test with P >0.05. The fixed effect model was used, while the random effects model was used for Bacillota and Fusobacteriota. Details are provided in Table 4.
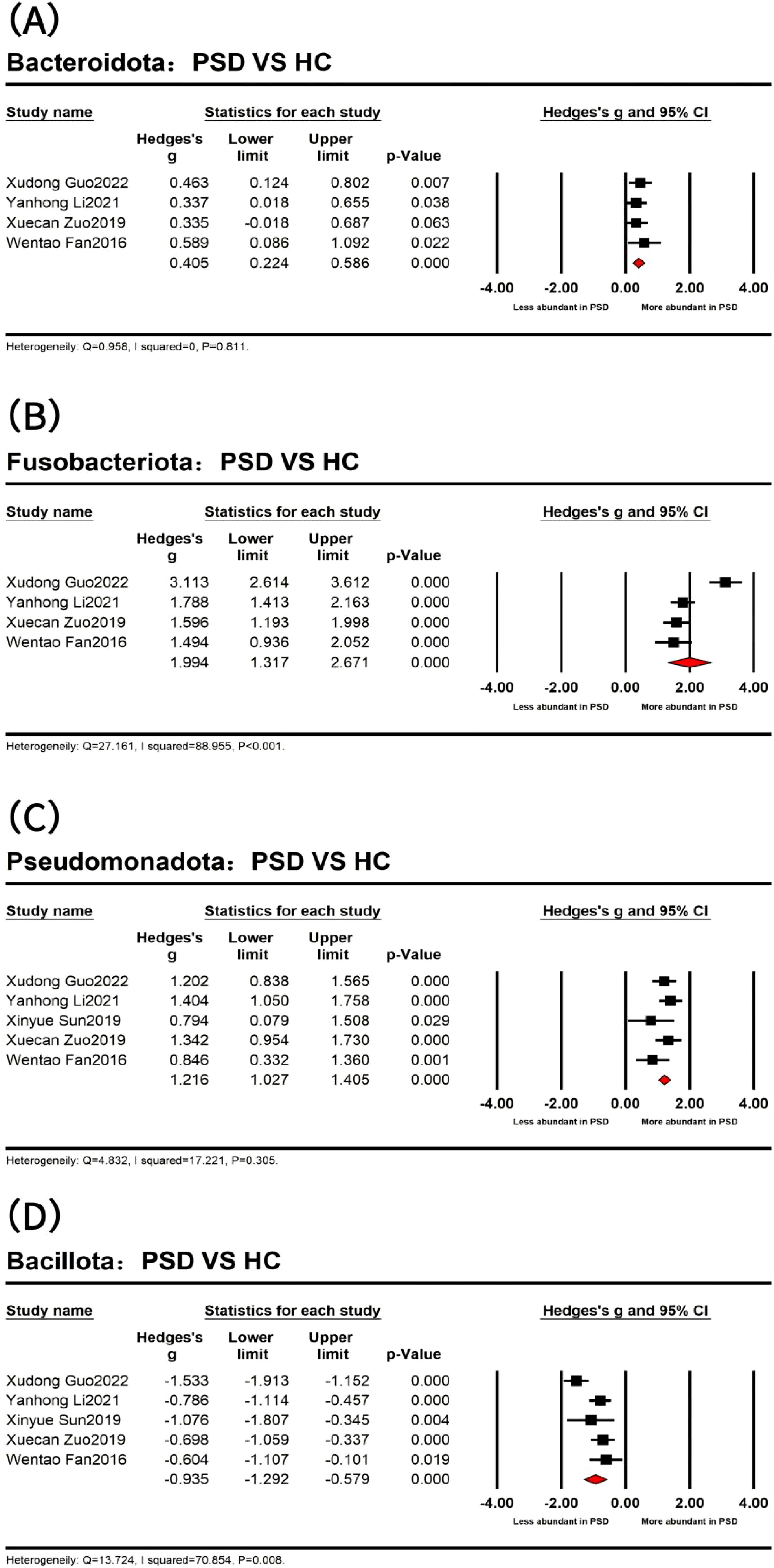
Figure 3. Forest plots of alterations of gut microbiota at the phylum level between post-stroke depression (PSD) and healthy controls (HC) spectrum, including Bacteroidota (A), Fusobacteriota (B), Pseudomonadota (C), and Bacillota (D).
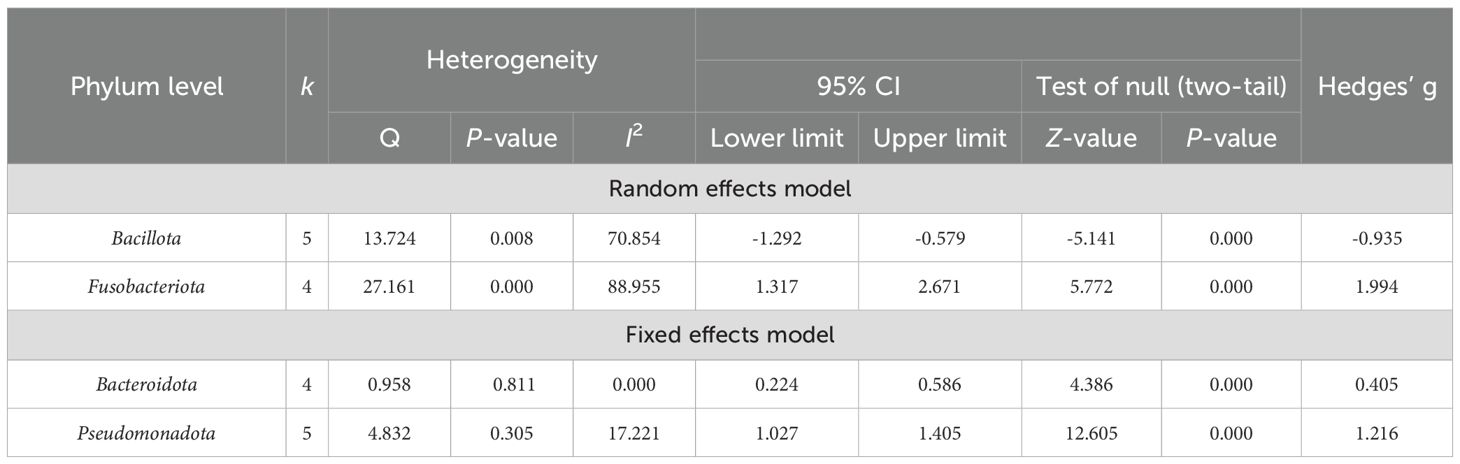
Table 4. Heterogeneity analysis and main effect analysis statistical results of gut microbiota at the phylum level.
Primary outcomes: differences in microbial composition at the family level
At the family level (Table 5), a total of 11 dominant bacterial families were identified in 240 PSD spectrum and 234 HC across four studies (Fan et al., 2016; Zuo et al., 2019; Li et al., 2021; Guo et al., 2022). The combined results of Brassicaceae were derived from three studies involving 208 PSD spectrum and 204 HC. The heterogeneity test results indicated that Prevotellaceae, Ruminococcaceae, and Lachnospiraceae had I2 values of <75%, besides Q test P-values >0.05, so these were analyzed using a fixed effects model. In contrast, the remaining eight dominant bacterial families exhibited high heterogeneity and were analyzed using random effects model. Specifically, increased abundances of Rikenellaceae, Acidaminococcaceae, Fusobacteriaceae, Brassicaceae, Enterobacteriaceae, Porphyromonadaceae, and Erysipelotrichaceae were observed in the PSD spectrum compared to HC. Conversely, Prevotellaceae, Ruminococcaceae, and
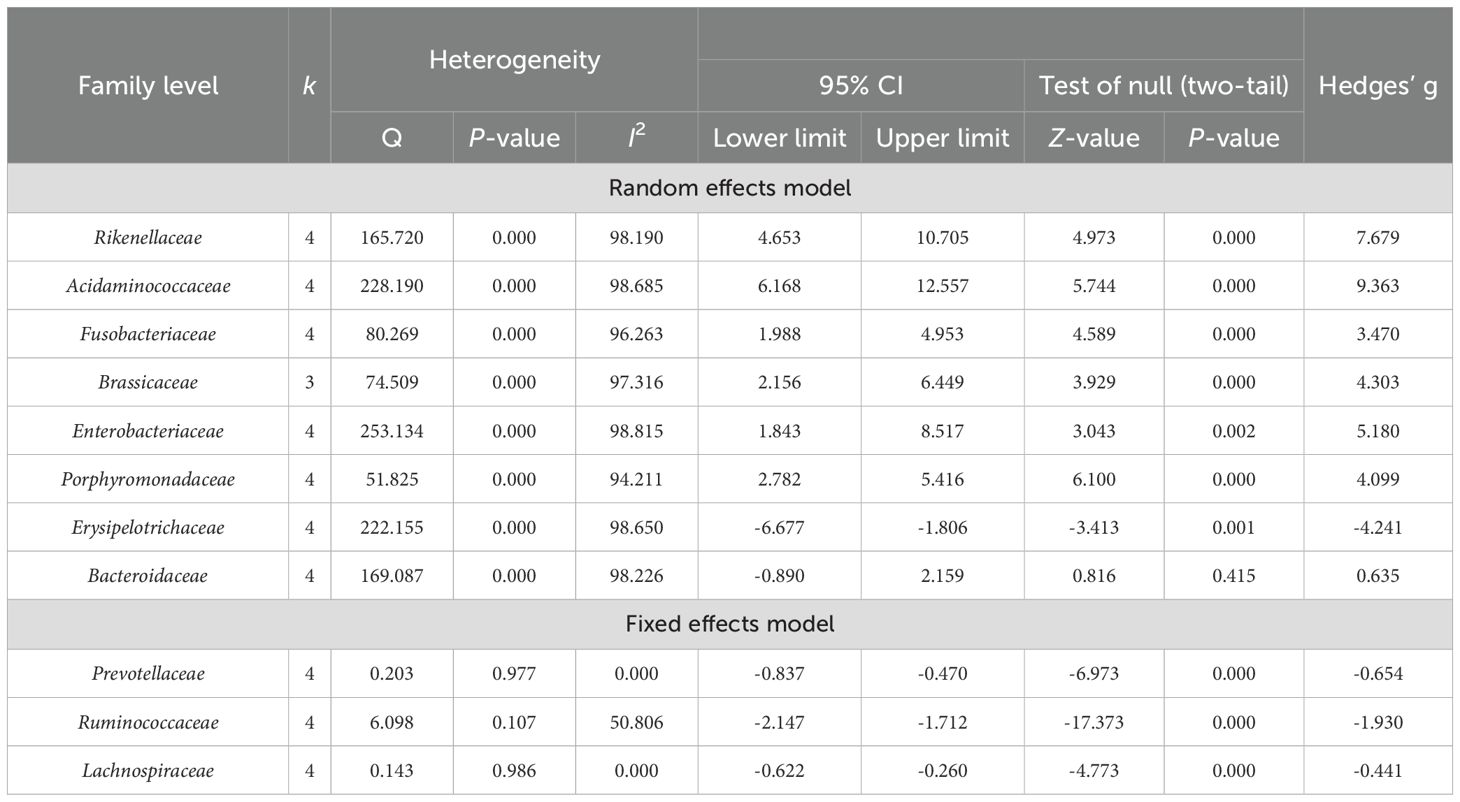
Table 5. Heterogeneity analysis and main effect analysis statistical results of gut microbiota at the family level.
Lachnospiraceae were significantly less abundant in the PSD spectrum compared to HC. After excluding one outlier for Bacteroidaceae, the heterogeneity test revealed I² = 0 and Q test p >0.05, allowing for re-analysis using a fixed effects model, which showed a reduced abundance of Bacteroidaceae (Hedges’ g = -0.240; 95% CI = -0.458 to -0.023; p = 0.031; n = 3) in the PSD spectrum compared to HC.
Primary outcomes: differences in microbial composition at the genus level
At the genus level (Table 6), a total of 21 dominant bacterial genera were identified. Of these, 19 were compared between PSD spectrum and HC, while two were compared between PSD spectrum and stroke. Firstly, a heterogeneity test was performed. For the 19 bacterial genera analyzed between PSD spectrum and HC, the results showed that the I2 values for Parasutterella, Parabasalids, Clostridium, and Alisma were <75%, while the Q test indicated P >0.05. Therefore, a fixed effects model was used for these analyses. The remaining dominant bacterial genera exhibited high heterogeneity and were analyzed using a random effects model for comparisons between PSD spectrum and stroke. The heterogeneity test results showed that Escherichia coli had an I2 value >75% and a Q test p-value <0.001, so a random effects model was employed. Conversely, for Lachnospira, the I2 value was 0 and p > 0.05, so a fixed effects model was used.
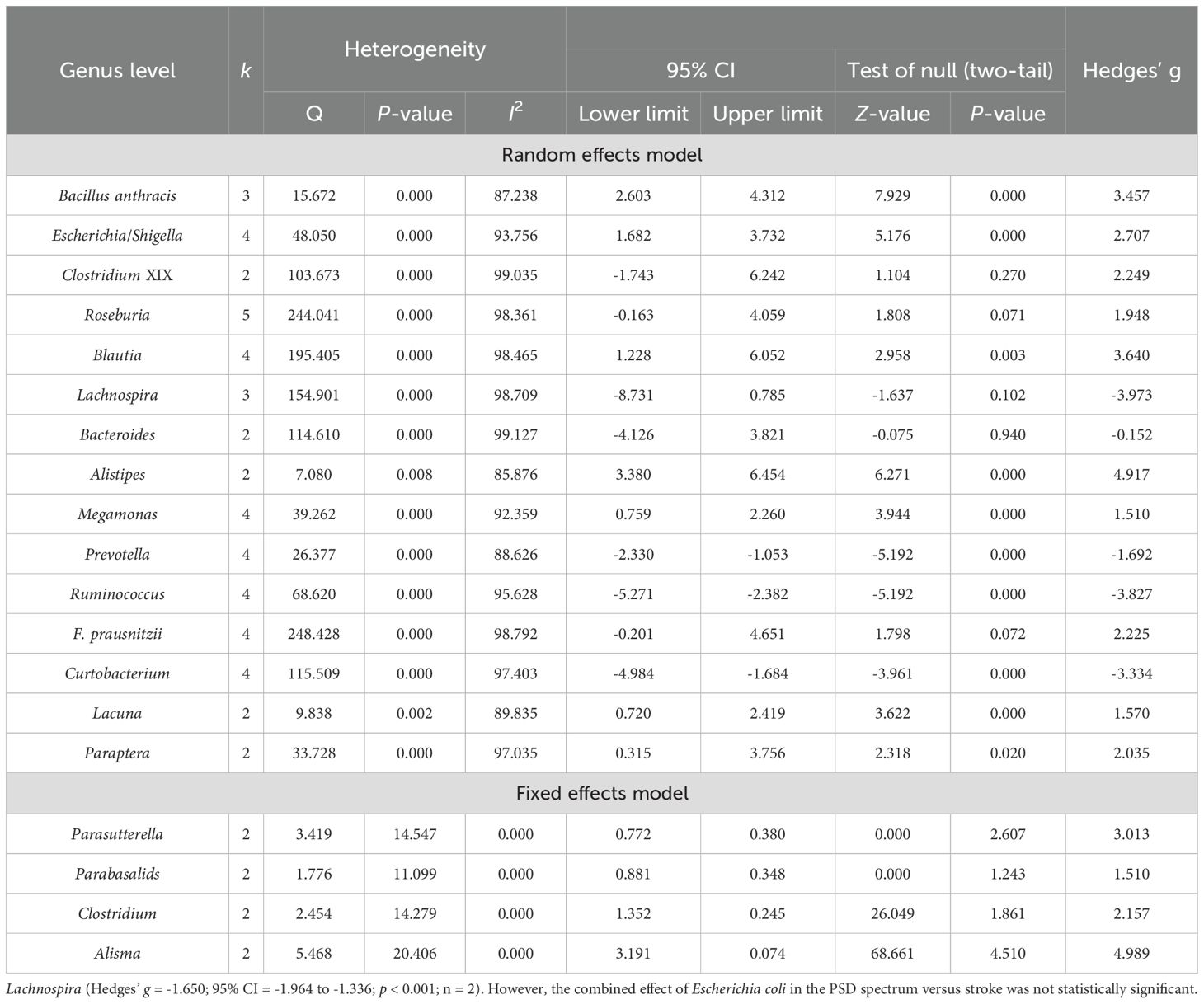
Table 6. Heterogeneity analysis and main effect analysis statistical results of gut microbiota at the genus level.
In detail, the PSD spectrum exhibited significantly higher levels of Bacillus anthracis, Escherichia/Shigella, Blautia, Alistipes, Megamonas, Parasutterella, Parabasalids, Lacuna, Clostridium, Paraptera, and Alisma compared to HC. After excluding one outlier, significantly higher abundances were also found for Roseburia (Hedges’ g = 3.211; 95% CI = 1.603 to 4.818; p < 0.001; n = 4), Lachnospira (Hedges’ g = 3.364; 95% CI = 0.222 to 6.506; p = 0.036; n = 2), and Faecalibacterium prausnitzii (F. prausnitzii) (Hedges’ g = 3.477; 95% CI = 2.128 to 4.826; p < 0.001; n = 3) in the PSD spectrum compared to HC. Conversely, the levels of Prevotella, Ruminococcus, and Curtobacterium were significantly lower in the PSD spectrum than in HC. Additionally, no significant differences were observed for Clostridium XIX and Bacteroides between the two groups. In comparisons between the PSD spectrum and stroke spectrum, the PSD spectrum had significantly lower levels of Lachnospira (Hedges’ g = -1.650; 95% CI = -1.964 to -1.336; p < 0.001; n = 2). However, the combined effect of Escherichia coli in the PSD spectrum versus stroke was not statistically significant.
Risk of bias
The quality of the included studies is summarized in Supplementary Table S3. Each study was classified as low risk for seven criteria. In addition, regarding confounding bias, only Yingjia Wang2023 controlled important confounding factors, such as BMI, diabetes, and diet, rated “low risk”. The remaining 13 studies all had unmeasured confounding factors, all rated “moderate risk”.
Publication bias
The Begg and Mazumdar rank correlations tests, along with Egger’s regression intercept tests, indicated that most of these meta-analysis results were not significantly biased by publication errors, with the exception of F-Porphyromonadaceae. The adjusted Hedges’ g was calculated for eight GM strains: F-Acidaminococcaceae, F-Fusobacteriaceae, F-Brassicaceae, F-Enterobacteriaceae, G-Blautia, G-Megamonas, G-Prevotella, and G-Faecalibacterium (Supplementary Table S4).
Discussion
This meta-analysis compared the GM abundance between patients with PSD spectrum and HC or stroke, providing five key insights into GM alterations associated with the PSD spectrum. First, α diversity was significantly higher in the PSD spectrum compared to HC but showed no significant difference when compared to the stroke spectrum. Second, the relative abundance of Pseudomonadota, Bacteroidota, and Fusobacteriota was higher in the PSD spectrum compared to HC, whereas Bacillota were less abundant. Third, at the family level, six bacterial families were more abundant and five were less abundant in the PSD spectrum compared to HC. Fourth, at the genus level, 14 genera were more abundant and five were less abundant in the PSD spectrum compared to HC. Finally, Lachnospira was found to be less abundant in the PSD spectrum compared to the stroke spectrum.
To our knowledge, previous meta-analyses have only evaluated α diversity and β diversity between the PSD spectrum and HC. Our study is the first to incorporate comparisons between the PSD spectrum and stroke spectrum. Our findings revealed that the Simpson index diversity was significantly higher in the stroke spectrum compared to the PSD spectrum, while other results showed no significant statistical differences. However, when comparing the PSD spectrum to the HC spectrum, we observed a significant increase in α diversity within the PSD spectrum. This finding contrasts with the results reported by Fang Luo et al (Luo and Fang, 2022). Regarding β diversity, the studies included in our meta-analysis yielded inconsistent results, warranting further investigation. Although increased gut microbial diversity is generally viewed as an indicator of health, it can also occur in the presence of potentially pathogenic and pro-inflammatory bacteria (Frost et al., 2019; Simpson et al., 2021). Therefore, the observed increase in gut microbial diversity within the PSD spectrum may reflect a similar underlying mechanism. The results of the sensitivity analyses showed that substantial changes in the effect size following the exclusion of a small-sample study highlight the sensitivity of our results to the inclusion of such studies. This sensitivity suggests that the original pooled effect size may underestimate the true effect. Small-sample studies are more susceptible to bias due to limited statistical power and potential methodological limitations, which could lead to either an overestimation or underestimation of the true effect. To enhance the robustness of future meta-analyses, we recommend prioritizing studies with larger sample sizes and standardized methodologies. Furthermore, sensitivity analyses should be routinely conducted to evaluate the influence of small-sample studies on pooled estimates and to ensure the reliability of the findings.
The phylum Bacillota is a significant group of bacteria in the human body, predominantly composed of gram-positive species. Most members of the Bacillota phylum are beneficial bacteria. For example, Lactobacillus species produce acetate and lactate. Moreover, antibacterial substances help prevent pathogen-related health disturbances. Butyrate, a crucial short-chain fatty acid (SCFA), has several important physiological roles, including trans-epithelial transport, reduction of mucosal inflammation, alleviation of oxidative stress, enforcement of the epithelial barrier, and further protection against colorectal cancer (CRC) (Hamer et al., 2008). Butyrate-synthesizing bacteria are predominantly found within the Bacillota phylum (Singh et al., 2023). Additionally, Bacillota is associated with anti-inflammatory effects, modulation of metabolic functions, and also SCFA production (Kumar et al., 2014; Welcome, 2019). There is also a positive correlation between Bacillota abundance and executive function performance, indicating that this phylum constitutes a beneficial component of the gut microbiota (Bhat and Kapila, 2017; Sheng et al., 2021). Our study results indicate that the abundance of Bacillota is lower in the PSD spectrum compared to the HC spectrum. Within the Bacillota phylum, Lachnospiraceae, Ruminococcaceae, Eubacteriaceae, and Clostridiaceae are the four important butyrate-synthesizing families (Singh et al., 2023). The results of our study found that members of Bacillota, including Lachnospiraceae, Ruminococcaceae, Erysipelotrichaceae, Ruminococcus, and F. prausnitzii also showed a decrease in abundance within the PSD spectrum compared with HC.
Pseudomonadota is a major phylum of gram-negative bacteria (Zhao and Lukiw, 2018) that includes a diverse range of metabolic species. Numerous studies have shown that an increase in intestinal Pseudomonadota is indicative of microbial dysbiosis or an unstable intestinal microbial community structure (Shin et al., 2015). Our study also found a significant increase in Pseudomonadota abundance within the PSD spectrum. Specifically, bacterial groups within Pseudomonadota, such as Enterobacteriaceae, Lachnospira, and Escherichia/Shigella, were more abundant in the PSD spectrum. Notably, neurotoxins derived from Escherichia coli, a member of the Pseudomonadota phylum, are known to enhance the release of proinflammatory cytokines (Cattaneo et al., 2017). Furthermore, increased Pseudomonadota abundance has been associated with worsening memory dysfunction (Hossain et al., 2019; Jeong et al., 2019). In summary, our finding of abnormally high Pseudomonadota levels in the PSD spectrum is consistent with previous literature.
Bacillota (formerly known as Firmicutes) and Bacteroidota are the two dominant phyla in the gut, comprising approximately 90% of the total gut microbiota (Human Microbiome Project Consortium. Structure, function and diversity of the healthy human microbiome, 2012). The Firmicutes/Bacteroidetes (F/B) ratio is an important indicator of gut microbiota health (Li and Ma, 2020); even deviations in this ratio are associated with various pathological conditions, including age-related disorders. An increase or decrease in the F/B ratio is often a sign of intestinal dysbiosis (Liang et al., 2018; An et al., 2023). An increased F/B ratio is commonly linked to obesity or metabolic disorders, potentially due to enhanced caloric extraction from food, fat deposition, lipogenesis, or impaired insulin sensitivity (Gibiino et al., 2018; Grigor’eva, 2020; Stojanov et al., 2020; Vaiserman et al., 2020). Conversely, a decreased F/B ratio is associated with conditions such as inflammatory bowel disease and depression as well as Alzheimer’s disease. This may result from an immune-inflammatory response triggered by reduced short-chain fatty acids (especially butyrate) production as well as the accumulation of protein metabolite like histamine and lipopolysaccharide (Gibiino et al., 2018; Schoeler and Caesar, 2019; Stojanov et al., 2020; Vaiserman et al., 2020).
Our study found that the phylum Bacteroidota was significantly more abundant in the PSD spectrum compared to HC, while the phylum Bacillota was reduced, resulting in a decreased F/B ratio indicative of intestinal dysbiosis. This finding aligns with the research by Lanxiang Liu et al., where intestinal dysbiosis was shown as a reduced F/B ratio. It is consistent with the research results of Lanxiang Liu et al (Liu et al., 2023). on major depressive disorder (MDD), which also reported a reduced F/B ratio in depressed patients. In addition, our study observed that at the family and genus levels, the abundance of Prevotellaceae, Bacteroidaceae, Bacteroides, and Prevotella was lower in the PSD spectrum compared to HC, which contrasts with the overall trends seen at the phylum level. This discrepancy be related to the intestinal barrier damage together with increased permeability, leading to bacterial translocation. Notably, previous studies have identified Alloprevotella as more dominant in healthy controls than in depressed patients (Zheng et al., 2021), consistent with our findings. Furthermore, research by Agirman et al (Agirman et al., 2021). indicated that increased intestinal permeability in depressed patients can lead to elevated plasma lipopolysaccharide (LPS) levels, which compromise the integrity of the blood–brain barrier (BBB). This breach allows inflammatory factors along with neurotoxins to enter the brain, activating immune cells and triggering inflammatory responses that contribute to the development of depression (Matsuno et al., 2022).
The phylum Fusobacteriota predominantly inhabits the oral cavity and colon, with certain species acting as opportunistic pathogens that can cause bacteremia together with various rapidly progressive infections. The virulence of Fusobacteriota is likely due to the production of lipopolysaccharide (LPS) and endotoxins as well as hemolysin (Brennan and Garrett, 2019). LPS can activate the TLR4-mediated NF-κB signaling pathway, leading to the production of pro-inflammatory cytokines such as interleukin-6 (IL-6), interleukin-8 (IL-8), and tumor necrosis factor-α (TNF-α) (Rubinstein et al., 2013; Abed et al., 2016; Bullman et al., 2017; Yang et al., 2017). A cross-sectional study investigating oral microbiota and ischemic stroke risk in elderly Chinese women revealed that specific imbalances in oral microbial composition (e.g., reduced Streptococcus while increased Porphyromonas) may elevate stroke risk through pro-inflammatory mechanisms (Wang et al., 2023). Through multi-omics analyses of early-onset cryptogenic stroke, Manzoor et al. demonstrated the elevated oral abundance of Fusobacterium nucleatum (Fusobacteria phylum), which established symbiotic interactions with pro-inflammatory Candida species, potentially promoting stroke pathogenesis via enhanced inflammatory responses along with thrombotic pathways (Manzoor et al., 2024).
Our study found that the abundance of the phylum Fusobacteriota was significantly higher in the PSD spectrum, with a corresponding increase in the Fusobacteriaceae family compared to HC. This heightened presence of Fusobacteriota may contribute to the development of post-stroke disease and could also play a role in the onset of post-stroke depression.
In addition, when comparing the gut microbiota between the PSD spectrum and stroke spectrum, no significant differences were observed in most bacterial groups, with Lachnospira being the only genus significantly less abundant in the PSD spectrum compared to the stroke spectrum (Huang et al., 2022; Li et al., 2022; Wang, 2023; Yao et al., 2023). This finding supports the earlier conclusion that Lachnospiraceae, a key butyrate-synthesizing family within the phylum Bacillota, may play a crucial role in the development of post-stroke depression due to its reduced presence in the PSD spectrum. A study investigating the gut microbiota in patients with mild cognitive impairment (MCI) and Alzheimer’s disease (AD) found that, compared to healthy controls, both AD and MCI patients exhibited a trend toward decreased Bacteroides, Lachnospira, and Ruminiclostridium_9 instead of an increase in Prevotella (Guo et al., 2021). Among these, Lachnospira was the only genus that showed a statistically significant reduction in MCI patients compared to healthy controls, highlighting its potential importance (Wang et al., 2023). Moreover, research has indicated that Lachnospira, along with Roseburia and Faecalibacterium, is negatively correlated with the severity of depressive symptoms. This underscores the significant role of Lachnospira in depression, suggesting that it could become a focal point for future research on the gut microbiota in stroke and PSD patients (Ye et al., 2021). However, given the current scarcity of studies comparing the gut microbiota between the PSD spectrum and stroke, further research is needed to draw more definitive conclusions so as to develop a more precise treatment for PSD.
It is worth noting that attributing to the variability in reporting standards across studies, as well as the lack of consistent measurement methods for certain confounders in the existing literature, the incomplete control of confounding factors exists universally. The potential impact of unmeasured confounders, such as diet, medication, and other health conditions, cannot be ruled out. These factors may have led to an overestimation/underestimation of the observed effect size. Given the comparability of baseline data, we still conducted a pooled analysis of the research results. Thus, the results of this meta-analysis should be interpreted with caution. Meanwhile, future studies are encouraged to adopt standardized reporting of confounders and employ more rigorous methods to control for these variables. To enhance the quality of future meta-analyses, we recommend that primary studies provide a more detailed reporting of confounding factors as well as their adjustment methods. Furthermore, the development of standardized guidelines for measuring and reporting confounders would significantly improve the comparability and reliability of pooled estimates.
Limitations
Despite these intriguing findings, our study has several limitations. First, we manually extracted data from the bar and box plots in several studies, which may introduce some biases. However, this process was conducted independently by two authors, reducing the likelihood that this would substantially affect the direction of statistical significance in our comparisons. Second, variations in sequencing methods could also contribute to potential biases in the results. For instance, differences in GM diversity between groups might be more pronounced when analyzing the V3–V4 region compared to the V3 region alone. However, the limited number of studies (five for the V3 region and seven for the V3–V4 region) restricted our ability to perform additional analyses. Third, all of the data were derived from studies conducted in China, involving a limited number of cities (nine provinces), which raises concerns about the generalizability of these findings to other populations.
Conclusion
In summary, our study found that the α diversity in PSD patients was higher than in HC, with notable differences in the distribution of GM at the phylum, family, and genus levels. Additionally, the abundance of Lachnospira was lower in PSD patients compared to that in the stroke group. However, due to the limited number of studies, small sample size, and geographical constraints, besides the inconsistent sequencing technologies, these findings should be interpreted with caution and may not be generalizable to a broader population. Future studies with higher quality as well as a wider scope are necessary to validate these results.
Data availability statement
The original contributions presented in the study are included in the article/Supplementary Material. Further inquiries can be directed to the corresponding author.
Author contributions
QL: Data curation, Formal Analysis, Project administration, Writing – original draft. YZ: Funding acquisition, Resources, Supervision, Writing – review & editing. XW: Data curation, Investigation, Writing – original draft. LD: Investigation, Validation, Writing – review & editing. WZ: Investigation, Validation, Writing – review & editing.
Funding
The author(s) declare that financial support was received for the research and/or publication of this article. This work was supported by the grants of Changsha Natural Science Foundation Project (NO.kp2403106).
Conflict of interest
The authors declare that the research was conducted in the absence of any commercial or financial relationships that could be construed as a potential conflict of interest.
Publisher’s note
All claims expressed in this article are solely those of the authors and do not necessarily represent those of their affiliated organizations, or those of the publisher, the editors and the reviewers. Any product that may be evaluated in this article, or claim that may be made by its manufacturer, is not guaranteed or endorsed by the publisher.
Supplementary material
The Supplementary Material for this article can be found online at: https://www.frontiersin.org/articles/10.3389/fcimb.2025.1444793/full#supplementary-material
References
Abed, J., Emgård, J. E., Zamir, G., Faroja, M., Almogy, G., Grenov, A., et al. (2016). Fap2 mediates fusobacterium nucleatum colorectal adenocarcinoma enrichment by binding to tumor-expressed gal-galNAc. Cell Host Microbe 20, 215–225. doi: 10.1016/j.chom.2016.07.006
Agirman, G., Yu, K. B., Hsiao, E. Y. (2021). Signaling inflammation across the gut-brain axis. Science. 374, 1087–1092. doi: 10.1126/science.abi6087
Agustí, A., García-Pardo, M. P., López-Almela, I., Campillo, I., Maes, M., Romaní-Pérez, M., et al. (2018). Interplay between the gut-brain axis, obesity and cognitive function. Front. Neurosci. 12. doi: 10.3389/fnins.2018.00155
An, J., Kwon, H., Kim, Y. J. (2023). The firmicutes/bacteroidetes ratio as a risk factor of breast cancer. J. Clin. Med. 12, 2216. doi: 10.3390/jcm12062216
Arentsen, T., Khalid, R., Qian, Y., Diaz Heijtz, R. (2018). Sex-dependent alterations in motor and anxiety-like behavior of aged bacterial peptidoglycan sensing molecule 2 knockout mice. Brain Behav. Immun. 67, 345–354. doi: 10.1016/j.bbi.2017.09.014
(2012). Human Microbiome Project Consortium. Structure, function and diversity of the healthy human microbiome. Nature 486, 207–214. doi: 10.1038/nature11234
Becker, K. J. (2016). Inflammation and the silent sequelae of stroke. Neurotherapeutics. 13, 801–810. doi: 10.1007/s13311-016-0451-5
Begg, C. B., Mazumdar, M. (1994). Operating characteristics of a rank correlation test for publication bias. Biometrics. 50, 1088–1101. doi: 10.2307/2533446
Bernstein, C. N. (2017). The brain-gut axis and stress in inflammatory bowel disease. Gastroenterol. Clin. North Am. 46, 839–846. doi: 10.1016/j.gtc.2017.08.006
Berthoud, H. R. (2008). Vagal and hormonal gut-brain communication: from satiation to satisfaction. Neurogastroenterol Motil. 20 Suppl 1, 64–72. doi: 10.1111/j.1365-2982.2008.01104.x
Bhat, M. I., Kapila, R. (2017). Dietary metabolites derived from gut microbiota: critical modulators of epigenetic changes in mammals. Nutr. Rev. 75, 374–389. doi: 10.1093/nutrit/nux001
Breit, S., Kupferberg, A., Rogler, G., Hasler, G. (2018). Vagus nerve as modulator of the brain-gut axis in psychiatric and inflammatory disorders. Front. Psychiatry 9. doi: 10.3389/fpsyt.2018.00044
Brennan, C. A., Garrett, W. S. (2019). Fusobacterium nucleatum - symbiont, opportunist and oncobacterium. Nat. Rev. Microbiol. 17, 156–166. doi: 10.1038/s41579-018-0129-6
Bullman, S., Pedamallu, C. S., Sicinska, E., Clancy, T. E., Zhang, X., Cai, D., et al. (2017). Analysis of Fusobacterium persistence and antibiotic response in colorectal cancer. Science. 358, 1443–1448. doi: 10.1126/science.aal5240
Carabotti, M., Scirocco, A., Maselli, M. A., Severi, C. (2015). The gut-brain axis: interactions between enteric microbiota, central and enteric nervous systems. Ann. Gastroenterol. 28, 203–209.
Cattaneo, A., Cattane, N., Galluzzi, S., Provasi, S., Lopizzo, N., Festari, C., et al. (2017). Association of brain amyloidosis with proinflammatory gut bacterial taxa and peripheral inflammation markers in cognitively impaired elderly. Neurobiol. Aging. 49, 60–68. doi: 10.1016/j.neurobiolaging.2016.08.019
Cha, J., Kim, S., Kim, P. W., Lee, H., Ko, M. M., Jang, S., et al. (2022). Development of the korean medicine core outcome set for stroke sequelae: herbal medicine treatment of elderly patients with stroke sequelae in primary clinics. Front. Pharmacol. 13. doi: 10.3389/fphar.2022.868662
Cryan, J. F., Dinan, T. G. (2012). Mind-altering microorganisms: the impact of the gut microbiota on brain and behaviour. Nat. Rev. Neurosci. 13, 701–712. doi: 10.1038/nrn3346
Cryan, J. F., O’Riordan, K. J., Sandhu, K., Peterson, V., Dinan, T. G. (2020). The gut microbiome in neurological disorders. Lancet Neurol. 19, 179–194. doi: 10.1016/S1474-4422(19)30356-4
Das J, G. K. R. (2018). Post stroke depression: The sequelae of cerebral stroke. Neurosci. Biobehav. Rev. 90, 104–114. doi: 10.1016/j.neubiorev.2018.04.005
Dinan, T. G., Cryan, J. F. (2017). Gut-brain axis in 2016: Brain-gut-microbiota axis - mood, metabolism and behaviour. Nat. Rev. Gastroenterol. Hepatol. 14, 69–70. doi: 10.1038/nrgastro.2016.200
Egger, M., Davey Smith, G., Schneider, M., Minder, C. (1997). Bias in meta-analysis detected by a simple, graphical test. BMJ. 315, 629–634. doi: 10.1136/bmj.315.7109.629
Fan, W. T., Yan, Y. M., Bie, Y. L., Wang, Q. (2016). Diversity of intestinal microflora in patients with depression after stroke. J. South. Med. University. 36, 1305–1311. doi: 10.3969/j.issn.1673-4254.2016.10.01
Foster, J. A., Rinaman, L., Cryan, J. F. (2017). Stress & the gut-brain axis: Regulation by the microbiome. Neurobiol. Stress. 7, 124–136. doi: 10.1016/j.ynstr.2017.03.001
Fouhy, F., Watkins, C., Hill, C. J., O'Shea, C. A., Nagle, B., Dempsey, E. M., et al. (2019). Perinatal factors affect the gut microbiota up to four years after birth. Nat. Commun. 10, 1517. doi: 10.1038/s41467-019-09252-4
Frost, F., Kacprowski, T., Rühlemann, M., Bang, C., Franke, A., Zimmermann, K., et al. (2019). Helicobacter pylori infection associates with fecal microbiota composition and diversity. Sci. Rep. 9, 20100. doi: 10.1038/s41598-019-56631-4
Gao, X., Cao, Q., Cheng, Y., Zhao, D., Wang, Z., Yang, H., et al. (2018). Chronic stress promotes colitis by disturbing the gut microbiota and triggering immune system response [published correction appears in Proc Natl Acad Sci U S A. 2018 Apr 30];:. Proc. Natl. Acad. Sci. U S A. 115, E2960–E2969. doi: 10.1073/pnas.1720696115
Gibiino, G., Lopetuso, L. R., Scaldaferri, F., Rizzatti, G., Binda, C., Gasbarrini, A. (2018). Exploring Bacteroidetes: Metabolic key points and immunological tricks of our gut commensals. Dig Liver Dis. 50, 635–639. doi: 10.1016/j.dld.2018.03.016
Grenham, S., Clarke, G., Cryan, J. F., Dinan, T. G. (2011). Brain-gut-microbe communication in health and disease. Front. Physiol. 2. doi: 10.3389/fphys.2011.00094
Grigor’eva, I. N. (2020). Gallstone disease, obesity and the firmicutes/bacteroidetes ratio as a possible biomarker of gut dysbiosis. J. Pers. Med. 11, 13. doi: 10.3390/jpm11010013
Guo, X. D., Li, Y. H., Zhai, Z. X. (2022). The diversity and equilibrium of microbial populations in the stool of patients with post-stroke depression. Chin. J. Microecology. 34, 685–689. doi: 10.13381/j.cnki.cjm.202206011
Guo, M., Peng, J., Huang, X., Xiao, L., Huang, F., Zuo, Z. (2021). Gut microbiome features of chinese patients newly diagnosed with alzheimer’s disease or mild cognitive impairment. J. Alzheimers Dis. 80, 299–310. doi: 10.3233/JAD-201040
Guo, J., Wang, J., Sun, W., Liu, X. (2022). The advances of post-stroke depression: 2021 update. J. Neurol. 269, 1236–1249. doi: 10.1007/s00415-021-10597-4
Hamer, H. M., Jonkers, D., Venema, K., Vanhoutvin, S., Troost, F. J., Brummer, R. J. (2008). Review article: the role of butyrate on colonic function. Aliment Pharmacol. Ther. 27, 104–119. doi: 10.1111/j.1365-2036.2007.03562.x
Han, G. S. (2021). The Intestinal Microorganisms Associated with Post-stroke Depression Based on multi-omics perspective (Guangxi: Youjiang Medical University For Nationalities). doi: 10.27908/d.cnki.gymzy.2021.000068
Heintz-Buschart, A., Pandey, U., Wicke, T., Sixel-Döring, F., Janzen, A., Sittig-Wiegand, E., et al. (2018). The nasal and gut microbiome in Parkinson’s disease and idiopathic rapid eye movement sleep behavior disorder. Mov Disord. 33, 88–98. doi: 10.1002/mds.27105
Hossain, S., Beydoun, M. A., Kuczmarski, M. F., Tajuddin, S., Evans, M. K., Zonderman, A. B. (2019). The interplay of diet quality and alzheimer’s disease genetic risk score in relation to cognitive performance among urban african americans. Nutrients. 11, 2181. doi: 10.3390/nu11092181
Hozo, S. P., Djulbegovic, B., Hozo, I. (2005). Estimating the mean and variance from the median, range, and the size of a sample. BMC Med. Res. Methodol. 5, 13. doi: 10.1186/1471-2288-5-13
Huang, W. C., Li, B. X., Liu, Z. N. (2022). Distribution of intestinal flora in patients with post-stroke depression and its relationship with serum IGF-1, BDNF, NGF and hcy levels. J. Pathogen Biol. 17, 1341–1344. doi: 10.13350/j.cjpb.221121
Huang, Y., Shen, Z., He, W. (2021). Identification of gut microbiome signatures in patients with post-stroke cognitive impairment and affective disorder. Front. Aging Neurosci. 13. doi: 10.3389/fnagi.2021.706765
Jaglin, M., Rhimi, M., Philippe, C., Pons, N., Bruneau, A., Goustard, B., et al. (2018). Indole, a signaling molecule produced by the gut microbiota, negatively impacts emotional behaviors in rats. Front. Neurosci. 12. doi: 10.3389/fnins.2018.00216
Jeong, M. Y., Jang, H. M., Kim, D. H. (2019). High-fat diet causes psychiatric disorders in mice by increasing Proteobacteria population. Neurosci. Lett. 698, 51–57. doi: 10.1016/j.neulet.2019.01.006
Jiang, W., Gong, L., Liu, F., Ren, Y., Mu, J. (2021). Alteration of gut microbiome and correlated lipid metabolism in post-stroke depression. Front. Cell Infect. Microbiol. 11. doi: 10.3389/fcimb.2021.663967
Kang, Y., Yang, Y., Wang, J., Ma, Y., Cheng, H., Wan, D. (2021). Correlation between intestinal flora and serum inflammatory factors in post-stroke depression in ischemic stroke. J. Coll. Physicians Surg. Pak. 31, 1224–1227. doi: 10.29271/jcpsp.2021.10.1224
Konturek, S. J., Konturek, J. W., Pawlik, T., Brzozowski, T. (2004). Brain-gut axis and its role in the control of food intake. J. Physiol. Pharmacol. 55, 137–154.
Kumar, H., Lund, R., Laiho, A., Lundelin, K., Ley, R. E., Isolauri, E., et al. (2014). Gut microbiota as an epigenetic regulator: pilot study based on whole-genome methylation analysis. mBio. 5, e02113–e02114. doi: 10.1128/mBio.02113-14
Li, X., Han, G., Zhao, J., Huang, X., Feng, Y., Huang, J., et al. (2022). Intestinal flora induces depression by mediating the dysregulation of cerebral cortex gene expression and regulating the metabolism of stroke patients. Front. Mol. Biosci. 9. doi: 10.3389/fmolb.2022.865788
Li, W., Ma, Z. S. (2020). FBA ecological guild: trio of firmicutes-bacteroidetes alliance against actinobacteria in human oral microbiome. Sci. Rep. 10, 287. doi: 10.1038/s41598-019-56561-1
Li, Y. H., Zhou, Q. Y., Li, P. P., Sun, S. B., Jin, Z. K., Xi, Z. M. (2021). Analysis of microbial population distribution in stool samples of patients with post-stroke depression. Henan Med. Res. 30, 2724–2727. doi: 10.3969/j.issn.1004-437X.2021.15.011
Liang, D., Leung, R. K., Guan, W., Au, W. W. (2018). Involvement of gut microbiome in human health and disease: brief overview, knowledge gaps and research opportunities. Gut Pathog. 10, 3. doi: 10.1186/s13099-018-0230-4
Ling, Y., Gu, Q., Zhang, J., Gong, T., Weng, X., Liu, J., et al. (2020). Structural change of gut microbiota in patients with post-stroke comorbid cognitive impairment and depression and its correlation with clinical features. J. Alzheimers Dis. 77, 1595–1608. doi: 10.3233/JAD-200315
Liu, L., Wang, H., Chen, X., Zhang, Y., Zhang, H., Xie, P. (2023). Gut microbiota and its metabolites in depression: from pathogenesis to treatment. EBioMedicine. 90, 104527. doi: 10.1016/j.ebiom.2023.104527
Luk, B., Veeraragavan, S., Engevik, M., Balderas, M., Major, A., Runge, J., et al. (2018). Postnatal colonization with human “infant-type” Bifidobacterium species alters behavior of adult gnotobiotic mice. PloS One 13, e0196510. doi: 10.1371/journal.pone.0196510
Luo, F., Fang, C. (2022). Association between gut microbiota and post-stroke depression in Chinese population: A meta-analysis. Heliyon. 8, e12605. doi: 10.1016/j.heliyon.2022.e12605
Ma, L. Y., Zhao, B., Ou, Y. N., Zhang, D. D., Li, Q. Y., Tan, L. (2023). Association of thyroid disease with risks of dementia and cognitive impairment: A meta-analysis and systematic review. Front. Aging Neurosci. 15. doi: 10.3389/fnagi.2023.1137584
Maniscalco, J. W., Rinaman, L. (2018). Vagal interoceptive modulation of motivated behavior. Physiol. (Bethesda). 33, 151–167. doi: 10.1152/physiol.00036.2017
Manzoor, M., Leskelä, J., Pietiäinen, M., Martinez-Majander, N., Ylikotila, P., Könönen, E., et al. (2024). Multikingdom oral microbiome interactions in early-onset cryptogenic ischemic stroke. ISME Commun. 4, ycae088. doi: 10.1093/ismeco/ycae088
Marin, I. A., Goertz, J. E., Ren, T., Rich, S. S., Onengut-Gumuscu, S., Farber, E., et al. (2017). Microbiota alteration is associated with the development of stress-induced despair behavior. Sci. Rep. 7, 43859. doi: 10.1038/srep43859
Matsuno, H., Tsuchimine, S., O’Hashi, K., Sakai, K., Hattori, K., Hidese, S., et al. (2022). Association between vascular endothelial growth factor-mediated blood-brain barrier dysfunction and stress-induced depression. Mol. Psychiatry 27, 3822–3832. doi: 10.1038/s41380-022-01618-3
Mayer, E. A., Tillisch, K., Gupta, A. (2015). Gut/brain axis and the microbiota. J. Clin. Invest. 125, 926–938. doi: 10.1172/JCI76304
McVey Neufeld, K. A., Perez-Burgos, A., Mao, Y. K., Bienenstock, J., Kunze, W. A. (2015). The gut microbiome restores intrinsic and extrinsic nerve function in germ-free mice accompanied by changes in calbindin. Neurogastroenterol Motil. 27, 627–636. doi: 10.1111/nmo.12534
Medeiros, G. C., Roy, D., Kontos, N., Beach, S. R. (2020). Post-stroke depression: A 2020 updated review. Gen. Hosp Psychiatry 66, 70–80. doi: 10.1016/j.genhosppsych.2020.06.011
Noma, H., Gosho, M., Ishii, R., Oba, K., Furukawa, T. A. (2020). Outlier detection and influence diagnostics in network meta-analysis. Res. Synth Methods 11, 891–902. doi: 10.1002/jrsm.1455
Page, M. J., McKenzie, J. E., Bossuyt, P. M., Boutron, I., Hoffmann, T. C., Mulrow, C. D., et al. (2021). The PRISMA 2020 statement: An updated guideline for reporting systematic reviews. Int. J. Surg. 88, 105906. doi: 10.1016/j.ijsu.2021.105906
Partrick, K. A., Chassaing, B., Beach, L. Q., McCann, K. E., Gewirtz, A. T., Huhman, K. L. (2018). Acute and repeated exposure to social stress reduces gut microbiota diversity in Syrian hamsters. Behav. Brain Res. 345, 39–48. doi: 10.1016/j.bbr.2018.02.005
Provensi, G., Schmidt, S. D., Boehme, M., Bastiaanssen, T. F. S., Rani, B., Costa, A., et al. (2019). Preventing adolescent stress-induced cognitive and microbiome changes by diet. Proc. Natl. Acad. Sci. U S A. 116, 9644–9651. doi: 10.1073/pnas.1820832116
Qin, L. H., Ma, J. J., Ma, L. L., Zhao, S. S., Li, Y. H. (2022). Diversity analysis of gut microbiota in patients with post-stroke depression. J. Med. Forum. 43, 64–67.
Rhee, S. H., Pothoulakis, C., Mayer, E. A. (2009). Principles and clinical implications of the brain-gut-enteric microbiota axis. Nat. Rev. Gastroenterol. Hepatol. 6, 306–314. doi: 10.1038/nrgastro.2009.35
Robinson, R. G., Jorge, R. E. (2016). Post-stroke depression: A review. Am. J. Psychiatry 173, 221–231. doi: 10.1176/appi.ajp.2015.15030363
Rubinstein, M. R., Wang, X., Liu, W., Hao, Y., Cai, G., Han, Y. W. (2013). Fusobacterium nucleatum promotes colorectal carcinogenesis by modulating E-cadherin/β-catenin signaling via its FadA adhesin. Cell Host Microbe 14, 195–206. doi: 10.1016/j.chom.2013.07.012
Sarkar, A., Lehto, S. M., Harty, S., Dinan, T. G., Cryan, J. F., Burnet, P. W. J. (2016). Psychobiotics and the manipulation of bacteria-gut-brain signals. Trends Neurosci. 39, 763–781. doi: 10.1016/j.tins.2016.09.002
Schoeler, M., Caesar, R. (2019). Dietary lipids, gut microbiota and lipid metabolism. Rev. Endocr. Metab. Disord. 20, 461–472. doi: 10.1007/s11154-019-09512-0
Seo, H. J., Kim, S. Y., Lee, Y. J., Park, J. E. (2023). RoBANS 2: A revised risk of bias assessment tool for nonrandomized studies of interventions. Korean J. Fam Med. 44, 249–260. doi: 10.4082/kjfm.23.0034
Sheng, C., Lin, L., Lin, H., Wang, X., Han, Y., Liu, S. L. (2021). Altered gut microbiota in adults with subjective cognitive decline: the SILCODE study. J. Alzheimers Dis. 82, 513–526. doi: 10.3233/JAD-210259
Shi, Y., Yang, D., Zeng, Y., Wu, W. (2017). Risk factors for post-stroke depression: A meta-analysis. Front. Aging Neurosci. 9. doi: 10.3389/fnagi.2017.00218
Shin, N. R., Whon, T. W., Bae, J. W. (2015). Proteobacteria: microbial signature of dysbiosis in gut microbiota. Trends Biotechnol. 33, 496–503. doi: 10.1016/j.tibtech.2015.06.011
Simpson, C. A., Diaz-Arteche, C., Eliby, D., Schwartz, O. S., Simmons, J. G., Cowan, C. S. M. (2021). The gut microbiota in anxiety and depression - A systematic review. Clin. Psychol. Rev. 83, 101943. doi: 10.1016/j.cpr.2020.101943
Singh, V., Lee, G., Son, H., Koh, H., Kim, E. S., Unno, T., et al. (2023). Butyrate producers, “The Sentinel of Gut”: Their intestinal significance with and beyond butyrate, and prospective use as microbial therapeutics. Front. Microbiol. 13. doi: 10.3389/fmicb.2022.1103836
Socała, K., Doboszewska, U., Szopa, A., Serefko, A., Włodarczyk, M., Zielińska, A., et al. (2021). The role of microbiota-gut-brain axis in neuropsychiatric and neurological disorders. Pharmacol. Res. 172, 105840. doi: 10.1016/j.phrs.2021.105840
Stang, A. (2010). Critical evaluation of the Newcastle-Ottawa scale for the assessment of the quality of nonrandomized studies in meta-analyses. Eur. J. Epidemiol. 25, 603–605. doi: 10.1007/s10654-010-9491-z
Stojanov, S., Berlec, A., Štrukelj, B. (2020). The influence of probiotics on the firmicutes/bacteroidetes ratio in the treatment of obesity and inflammatory bowel disease. Microorganisms. 8, 1715. doi: 10.3390/microorganisms8111715
Sun, X. Y. (2019). Observation and study of intestinal flora characteristics in patients with kidney deficiency and liver stagnation post-stroke depression (Beijing: Beijing University of Chinese Medicine).
Sun, M., Chen, H., Dong, S., Zhang, G., Zhou, X., Cheng, H. (2024). Alteration of gut microbiota in post-stroke depression patients with Helicobacter pylori infection. Neurobiol. Dis. 193, 106458. doi: 10.1016/j.nbd.2024.106458
Sun, J., Wang, F., Hu, X., et al. (2018). Clostridium butyricum Attenuates Chronic Unpredictable Mild Stress-Induced Depressive-Like Behavior in Mice via the Gut-Brain Axis. J. Agric. Food Chem. 66, 8415–8421. doi: 10.1021/acs.jafc.8b02462
Taché, Y., Vale, W., Rivier, J., Brown, M. (1980). Brain regulation of gastric secretion: influence of neuropeptides. Proc. Natl. Acad. Sci. U S A. 77, 5515–5519. doi: 10.1073/pnas.77.9.5515
Taylor-Rowan, M., Momoh, O., Ayerbe, L., Evans, J. J., Stott, D. J., Quinn, T. J. (2019). Prevalence of pre-stroke depression and its association with post-stroke depression: a systematic review and meta-analysis. Psychol. Med. 49, 685–696. doi: 10.1017/S0033291718002003
Vaiserman, A., Romanenko, M., Piven, L., Moseiko, V., Lushchak, O., Kryzhanovska, N., et al. (2020). Differences in the gut Firmicutes to Bacteroidetes ratio across age groups in healthy Ukrainian population. BMC Microbiol. 20, 221. doi: 10.1186/s12866-020-01903-7
Viechtbauer, W., Cheung, M. W. (2010). Outlier and influence diagnostics for meta-analysis. Res. Synth Methods 1, 112–125. doi: 10.1002/jrsm.11
Wang, Y. J. (2023). Characterization of intestinal flora and related influencing factors in depressed patients after ischemic stroke (Shanxi: Shanxi Medical University). doi: 10.27288/d.cnki.gsxyu.2023.000944
Wang, C., Yang, Y., Cai, Q., Gao, Y., Cai, H., Wu, J., et al. (2023). Oral microbiome and ischemic stroke risk among elderly Chinese women. J. Oral. Microbiol. 15, 2266655. doi: 10.1080/20002297.2023.2266655
Welcome, M. O. (2019). Gut microbiota disorder, gut epithelial and blood-brain barrier dysfunctions in etiopathogenesis of dementia: molecular mechanisms and signaling pathways. Neuromolecular Med. 21, 205–226. doi: 10.1007/s12017-019-08547-5
WHO (2018b). Global health estimates 2016: deaths by cause, age, sex, by country and by region, 2000-2016 (World Health Organization).
Yang, Y., Weng, W., Peng, J., Hong, L., Yang, L., Toiyama, Y., et al. (2017). Fusobacterium nucleatum increases proliferation of colorectal cancer cells and tumor development in mice by activating toll-like receptor 4 signaling to nuclear factor-κB, and up-regulating expression of microRNA-21. Gastroenterology. 152, 851–866.e24. doi: 10.1053/j.gastro.2016.11.018
Yao, S., Xie, H., Wang, Y., Shen, N., Chen, Q., Zhao, Y., et al. (2023). Predictive microbial feature analysis in patients with depression after acute ischemic stroke. Front. Aging Neurosci. 15. doi: 10.3389/fnagi.2023.1116065
Ye, X., Wang, D., Zhu, H., Wang, D., Li, J., Tang, Y., et al. (2021). Gut microbiota changes in patients with major depressive disorder treated with vortioxetine. Front. Psychiatry 12. doi: 10.3389/fpsyt.2021.641491
Zeng, X., Zhang, Y., Kwong, J. S., Zhang, C., Li, S., Sun, F., et al. (2015). The methodological quality assessment tools for preclinical and clinical studies, systematic review and meta-analysis, and clinical practice guideline: a systematic review. J. Evid Based Med. 8, 2–10. doi: 10.1111/jebm.12141
Zhao, Y., Lukiw, W. J. (2018). Bacteroidetes neurotoxins and inflammatory neurodegeneration. Mol. Neurobiol. 55, 9100–9107. doi: 10.1007/s12035-018-1015-y
Zheng, S., Zhu, Y., Wu, W., Zhang, Q., Wang, Y., Wang, Z., et al. (2021). A correlation study of intestinal microflora and first-episode depression in Chinese patients and healthy volunteers. Brain Behav. 11, e02036. doi: 10.1002/brb3.2036
Keywords: gut microbiome, dysbiosis, PSD, stroke, depression, review
Citation: Li Q, Zhang Y, Wang X, Dai L and Zhao W (2025) Gut microbiota of patients with post-stroke depression in Chinese population: a systematic review and meta-analysis. Front. Cell. Infect. Microbiol. 15:1444793. doi: 10.3389/fcimb.2025.1444793
Received: 07 June 2024; Accepted: 17 March 2025;
Published: 01 May 2025.
Edited by:
Matilde Otero-Losada, National Scientific and Technical Research Council (CONICET), ArgentinaReviewed by:
Laura Mitrea, University of Agricultural Sciences and Veterinary Medicine of Cluj-Napoca, RomaniaMuhammed Manzoor, University of Helsinki, Finland
Copyright © 2025 Li, Zhang, Wang, Dai and Zhao. This is an open-access article distributed under the terms of the Creative Commons Attribution License (CC BY). The use, distribution or reproduction in other forums is permitted, provided the original author(s) and the copyright owner(s) are credited and that the original publication in this journal is cited, in accordance with accepted academic practice. No use, distribution or reproduction is permitted which does not comply with these terms.
*Correspondence: Yuejuan Zhang, emhhbmd5dWVqdWFuaHVsaUAxNjMuY29t