- 1Laboratório de Pesquisa em Resistência Bacteriana (LABRESIS), Hospital de Clínicas de Porto Alegre (HCPA), Centro de Pesquisa Experimental, Porto Alegre, Brazil
- 2Laboratório de Microbiologia e Saúde Única do Instituto de Ciências Básicas da Saúde (ICBS) da Universidade Federal do Rio Grande do Sul, Porto Alegre, Brazil
- 3Núcleo de Bioinformática (Bioinformatics Core), Hospital de Clínicas de Porto Alegre (HCPA), Porto Alegre, Brazil
- 4Laboratório de Neuroimunologia do Instituto de Ciências Básicas da Saúde (ICBS) da Universidade Federal do Rio Grande do Sul, Porto Alegre, Brazil
It is argued that commensal bacteria in the upper respiratory tract (URT) protect against pathogen colonization and infection, including respiratory viruses. Given that the microbiome can mediate immune modulation, a link between the URT microbiome (URTM) and COVID-19 susceptibility and severity is expected. This 16S metagenomics cross-sectional study assessed URTM composition, metabolic prediction, and association with laboratory biomarkers in non-COVID-19 pneumonia (NO-CoV), moderate (M-CoV), severe (S-CoV) COVID-19 patients, as well as COVID-19-negative, asymptomatic (NC) patients. The S-CoV group exhibited reduced URTM diversity, primarily due to a decreased abundance of eubiotic taxa. Some of these taxa (e.g., Haemophilus sp., Neisseria sp.) were also associated with inflammatory biomarkers. Multiple metabolic pathways (e.g., short-chain fatty acids, vitamin B12) linked to immune response, antiviral activity, and host susceptibility showed decreased abundance in S-CoV. These pathways could suggest potential alternatives for the therapeutic arsenal against COVID-19, providing reassurance about the progress in understanding and treating this disease.
1 Introduction
The severe acute respiratory syndrome coronavirus 2 (SARS-CoV-2), the causative agent of coronavirus disease 19 (COVID-19), continues to pose a global threat due to its ability to evade the immune system through genetic mutations. Despite the pandemic slowdown, immunized individuals remain susceptible to SARS-CoV-2, which is believed to keep circulating for many years (Kissler et al., 2020; Rossman et al., 2021; Barreiro and San Román, 2022).
In COVID-19, both immune suppression and runaway inflammation have been observed and can result in more severe disease, while protective immunity consists of the induction of both humoral and cell-mediated responses (Merenstein et al., 2022). The SARS-CoV-2 inhibits Interferons I and III (IFN-I and IFN-III) and pro-inflammatory responses, promoting high viral replication in the respiratory tract (Wei et al., 2020). Paradoxically, this immune suppression leads to increased circulating cytokines in COVID-19-associated pneumonia. Severe lung damage from viral replication causes epithelial barrier breakdown, triggering a ‘cytokine storm’ with both pro- and anti-inflammatory actions (Tisoncik et al., 2012; Chen et al., 2020; Hall et al., 2022), which impair lung function promoting immunoparalysis, resulting in irreversible damage (Wong et al., 2019).
In the upper respiratory tract (URT), which comprises the nasal cavity, nasopharynx, and oropharynx, high bacterial densities are found (103–106 U−1), while in the lung, which belongs to the lower respiratory tract (LRT), bacterial densities decrease (~102 U−1). Genera characteristic of a healthy URT include Staphylococcus sp., Propionibacterium sp., Leptotrichia sp., Rothia sp., Dolosigranulum sp., Haemophilus sp., Moraxella sp., Veillonella sp., Prevotella sp., Streptococcus sp. and Corynebacterium sp (Man et al., 2017; Wypych et al., 2019). In eubiosis, these microorganisms act as ‘guardians of respiratory health’ (Wong et al., 2019; Wypych et al., 2019), avoiding colonization of the URT by pathogens, including respiratory viruses, suggesting a potential role in resisting SARS-CoV-2 infection (Bogaert et al., 2004; Wang et al., 2022).
The interactions between the microbiome and its host extend to crucial functions such as carbohydrate and protein decomposition, nutrient absorption, vitamin biosynthesis, and modulation of the immune system (Rooks and Garrett, 2016; Zheng et al., 2020). Metabolites and components from these interactions can modulate immune cells through various mechanisms, including signaling of mucosal-associated T cells (MAIT) (Smith et al., 2013; Godfrey et al., 2019; Legoux et al., 2020;, IFN-I (Flerlage et al., 2021), and dendritic cells (Schaupp et al., 2020) beyond the anti-inflammatory activity of Short-Chain Fatty Acids (SCFA) (Fukuda et al., 2011) and regulation of immunoglobulin expression (Corbett et al., 2014; Kim et al., 2016; Dalile et al., 2019; Parrot et al., 2020; Wang et al., 2022).
Recent investigations have revealed substantial dysbiosis in both intestinal and respiratory microbiomes during COVID-19, and their specific compositions are correlated with the severity of the disease and its outcomes (Ferreira et al., 2020; Lloréns-Rico et al., 2021). Additionally, SARS-CoV-2 infection has been found to heighten the susceptibility of patients to secondary pathogens, thereby playing a significant role in exacerbating morbidity and mortality associated with COVID-19 (Cox et al., 2020; Yeoh et al., 2021). Besides, the microbiome has been identified as a modulator of immune responses and diseases in the airways, increasing interest in the interaction between COVID-19 and this microbiome (Merenstein et al., 2022). This study aimed to delve into these interactions at a functional level, identifying taxa and pathways in the URT associated with COVID-19 severity.
2 Methods
2.1 Study design
This study employed a cross-sectional design focused on analyzing the upper respiratory tract microbiome in the context of COVID-19. 16S rRNA amplicon sequencing was used to assess composition and predict functionality.
A total of 88 samples of nasal and oropharyngeal combined swabs were provided by the Hospital de Clinicas de Porto Alegre (HCPA) biobank. Swabs were immersed in sterile saline solution for routine SARS-CoV-2 screening using rt-qPCR (National Center for Immunization and Respiratory Diseases (U.S.). Monteiro et al., 2023) for diagnostic purposes and stored at -80°C. The experimental groups were categorized based on the World Health Organization severity level classification (World Health Organization, 2020):
Group 1 (M-COV, n = 22): Moderate COVID-19 - Patients with signs and symptoms of pneumonia, respiratory distress syndrome (ARDS), SpO2 ≥ 90%, and a positive rt-qPCR test for SARS-CoV-2.
Group 2 (NO-COV, n = 22): Non-COVID pneumonia - Patients with signs and symptoms of pneumonia, ARDS, SpO2 ≥ 90%, and two negative rt-qPCR test for SARS-CoV-2.
Group 3 (S-COV, n = 22): Severe COVID-19 - Patients with severe signs and symptoms of pneumonia, ARDS, breathing rate > 30/min., SpO2 < 90%, and a positive rt-qPCR test for SARS-CoV-2.
Group 4 (NC, n = 22): Asymptomatic individuals - Highly exposed to SARS-CoV-2, submitted to routine screening with negative results.
In addition to the COVID-19 severity classification, inclusion criteria comprised patients who underwent rt-qPCR for SARS-CoV-2 screening and laboratory tests for biomarkers (except for the NC group), namely: alanine aminotransferase (ALT), aspartate aminotransferase (AST), c-reactive protein (CRP), creatine phosphokinase (CPK), d-dimer (DDI), hemogram, lactate dehydrogenase (LDH), serum creatinine (CRE) and urea. Demographic characteristics and clinical data were obtained from electronic medical records through a query requested by the Center of Data Science of the HCPA. The study was conducted following the Declaration of Helsinki, and the protocol was approved by the Ethics Committee of HCPA (IRB 00000921, CAAE 38972220.3.0000.5327), ensuring compliance with ethical standards and guidelines for human research. The COVID-19 vaccination was unavailable in Brazil during the study period (between July 2020 and February 2021).
2.2 Microbiome data generation
2.2.1 DNA extraction, PCR, and sequencing
The DNA extraction was performed with the DNeasy® Blood and Tissue extraction kit (QIAGEN®, Canada) following the manufacturer’s recommendations with minor adaptations. The adaptation consists of performing a bead beating procedure with silica/zirconia beads in a MP Biochemicals FastPrep24 Sample Preparation (RRID: SCR_018599) using a 30s protocol at 6.0 m/s repeated three times, after the incubation with proteinase K. The total nucleic acids were eluted in 60 μL of TE buffer (Tris-EDTA pH 8,0) and submitted to amplification of the v3v4 hypervariable region of 16S rRNA using the 16S Metagenomic Sequencing Library Preparation Illumina® (Illumina, USA, 2013; Illumina, 2013). A negative control (molecular grade water) was included for quality assurance. The libraries were sequenced on an Illumina MiSeq System (RRID: SCR_016379) platform using a v2 Reagent Kit (2x250bp; average coverage ~200.000 reads/sample) (Illumina, Inc.). Extreme care was taken to mitigate the possibility of environmental contamination during the entire sample processing (Kirstahler et al., 2018).
2.2.2 Bioinformatics analyses
The bioinformatics analyses were performed with the open-source software R Project for Statistical Computing (RRID: SCR_001905) v. 4.3.2 (Eye Holes) (R Core Team, 2021), the development interface RStudio (RRID: SCR_000432) v. 2022.12.0-353 (RStudio Team, 2020), and packages of the project Bioconductor (RRID: SCR_006442) v. 3.16 (Huber et al., 2015).
Raw reads were processed according to a previous report (Callahan et al., 2016b) using the DADA2 (RRID: SCR_023519) algorithm (Callahan et al., 2016a). Briefly, reads were quality-checked, trimmed, filtered, and truncated to position 240. Paired-end joining, determination of amplicon sequence variants (ASV), and removal of chimeric sequences were performed, followed by the taxonomic assignment using the expanded Human Oral Microbiome Database (RRID: SCR_025964) (eHOMD 16S rRNA RefSeq Version 15.22) (Escapa et al., 2020). The ASV sequences, counts, taxonomy tables, and sample metadata were merged into a phyloseq (RRID: SCR_013080) object (McMurdie and Holmes, 2013) to proceed with the analyses. All the detailed parameters are presented in the Data Sheet 2.
Taxonomic agglomeration was performed to identify the dominant taxa and their relative abundance at phyla, genera, and species levels. The Compositional Data Analysis approach (CODA) (Aitchison, 1982) was adopted to evaluate the microbiome composition using the Centered Log-Ratio (CLR) for log-ratio transformation (Pawlowsky-Glahn et al., 2015; Greenacre et al., 2021; Bars-Cortina, 2022).
The metabolic prediction was performed with the PICRUSt2 (RRID: SCR_022647) pipeline (Douglas et al., 2020). The functional prediction and the pathway inference were performed with a modified version of the MinPath (Minimal set of Pathways) (Ye and Doak, 2009) tool using the MetaCyc (RRID: SCR_007778) database (Caspi et al., 2016). The pathway annotation was performed with the ggpicrust2 (RRID: SCR_025965) package (Yang et al., 2023).
2.2.3 Statistical analysis
α-diversity was performed using the Shannon index followed by the Wilcoxon Rank-sum test (Haegeman et al., 2013). β-diversity was conducted by the Principal Component Analysis (PCA) using Aitchison distance (Aitchison, 1982; Calle, 2019). The statistical significance and the proportion of explained variance were assessed by Permutation Multivariate Analysis of Variance (PERMANOVA) (Anderson, 2017) for experimental groups and each confounding factor (age, sex, and batch).
The taxonomic differential abundance analysis was performed using the Analysis of Compositions of Microbiomes with Bias Correction 2 (ANCOM-BC2 (RRID: SCR_024901)) algorithm (Lin and Peddada, 2020) for the global test (NC as the reference group) and multiple pairwise comparisons at the genus level. The pathway’s differential abundance was evaluated using the LinDA (RRID: SCR_025966) (linear models for differential abundance analysis of microbiome compositional data) (Zhou et al., 2022) algorithm.
We accounted for multiple tests using the Benjamini-Hochberg (BH) method (Benjamini and Hochberg, 1995) for false discovery rate (FDR) and a mixed directional false discovery rate (mdFDR) control using a family-wise error controlling procedure by Holm method (Holm, 1979), with an alpha of 0.05.
We performed a pairwise log-ratio exploratory analysis with the coda4microbiome (Calle et al., 2023) package to evaluate the relationships among taxa and clinical variables. Spearman’s correlation coefficient was used to measure the association between the variables and the predictions of a generalized linear model (GLM). All the detailed parameters used for statistical analysis are presented in Data Sheet 3.
2.2.4 Code availability
The PICRUSt2 (Douglas et al., 2020) is available at https://github.com/picrust/picrust2 Custom scripts and data generated for this paper can be accessible from “Lovison_et_al_2024_COVID19_URT_microbiome”, GitHub (https://github.com/otaviolovison/Lovison_et_al_2024_COVID19_URT_microbiome).
3 Results
Among the 88 selected samples, nine were excluded due to the impossibility of extracting high-quality DNA or unsatisfactory sequencing results. The samples and their respective experimental groups that remained for the analysis were M-COV, n = 22; NO-COV, n = 19; S-COV, n = 20; and NC, n = 18. Data Sheet 1 presents a flowchart representing experimental design and the bioinformatics workflow.
The quality check as well as the error rates analysis from the preprocessing and the complete track of the reads are shown in Supplementary Material (Data Sheet 2, Table 1 - Supplementary Data 1). After the filtering step ( Data Sheet 3- 0.1 Data preparation), 11 phyla, 200 genera, 364 species, and 50,347 ASVs were identified. The complete description, representative proportions, and overall relative abundance of these taxa are presented in Supplementary Material (Table 1 - Supplementary Data 2-Table 1 - Supplementary Data 5).
3.1 α-diversity and β-diversity
The S-CoV group presented the lowest α-diversity (mean = 2.90, standard error (SD) = 0.94). In the pairwise comparison, statistically significant differences were observed (Figure 1) between M-CoV and S-CoV (p = 0.0019) and between S-CoV and NC (p = 0.039). The α-diversity summarized statistics are presented in Supplementary Material (Table 1 - Supplementary Data 6).
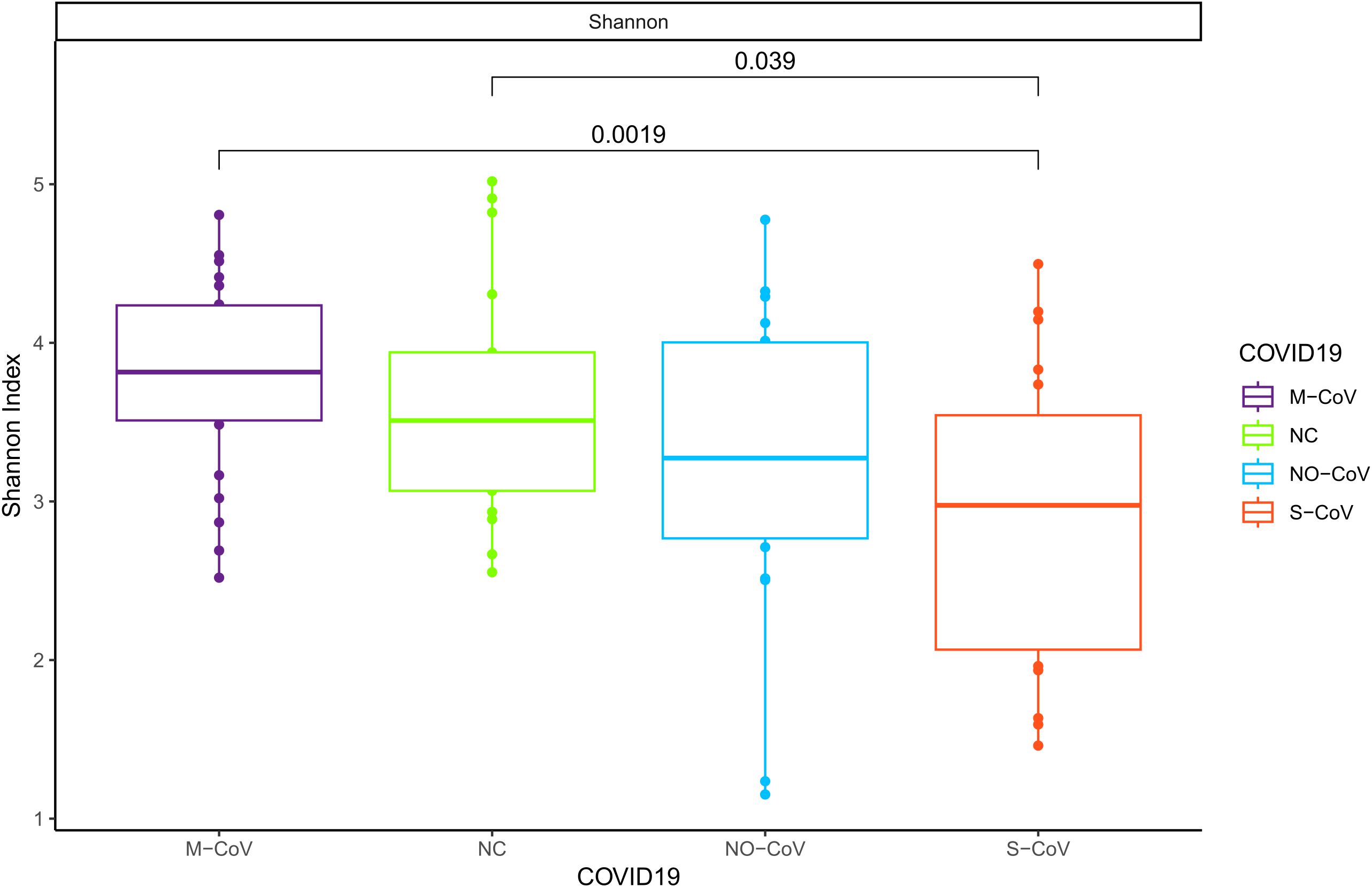
Figure 1. α-diversity analysis by Shannon index for COVID-19 groups. The Wilcoxon rank-sum test assessed statistical significance. M-CoV: moderate COVID-19; NC: Negative Control; NO-CoV: patients with pneumonia, ARDS, SpO2 ≥ 90% and a negative rt-qPCR test for SARS-CoV-2; S-CoV: severe COVID-19. The pairwise comparison shows statistical differences between M-CoV and S-CoV (p = 0.0019) and between S-CoV and NC (p = 0.039).
No statistically significant difference was detected among groups for β-diversity ( Data Sheet 3 - Exploratory Analysis, 2.0 - Beta Diversity).
3.2 Differential abundance analysis
Several taxa presented global differential abundance in comparison with the NC group. The genera Abiotrophia sp., Arthrospira sp., Enterococcus sp. and Lactobacillus sp. showed positive differential abundance for S-CoV while presenting negative for M-CoV and NO-CoV. Conversely, Acidovorax sp. showed positive differential abundance for M-CoV and NO-CoV while presenting negative for S-CoV. Gemella sp., Mesorhizobium sp., Novosphingobium sp., and Saccharibacteria_(TM7) were enriched only in M-CoV, while Dolosigranulum sp. and family Enterobacteriaceae were only increased in NO-CoV (Figure 2).
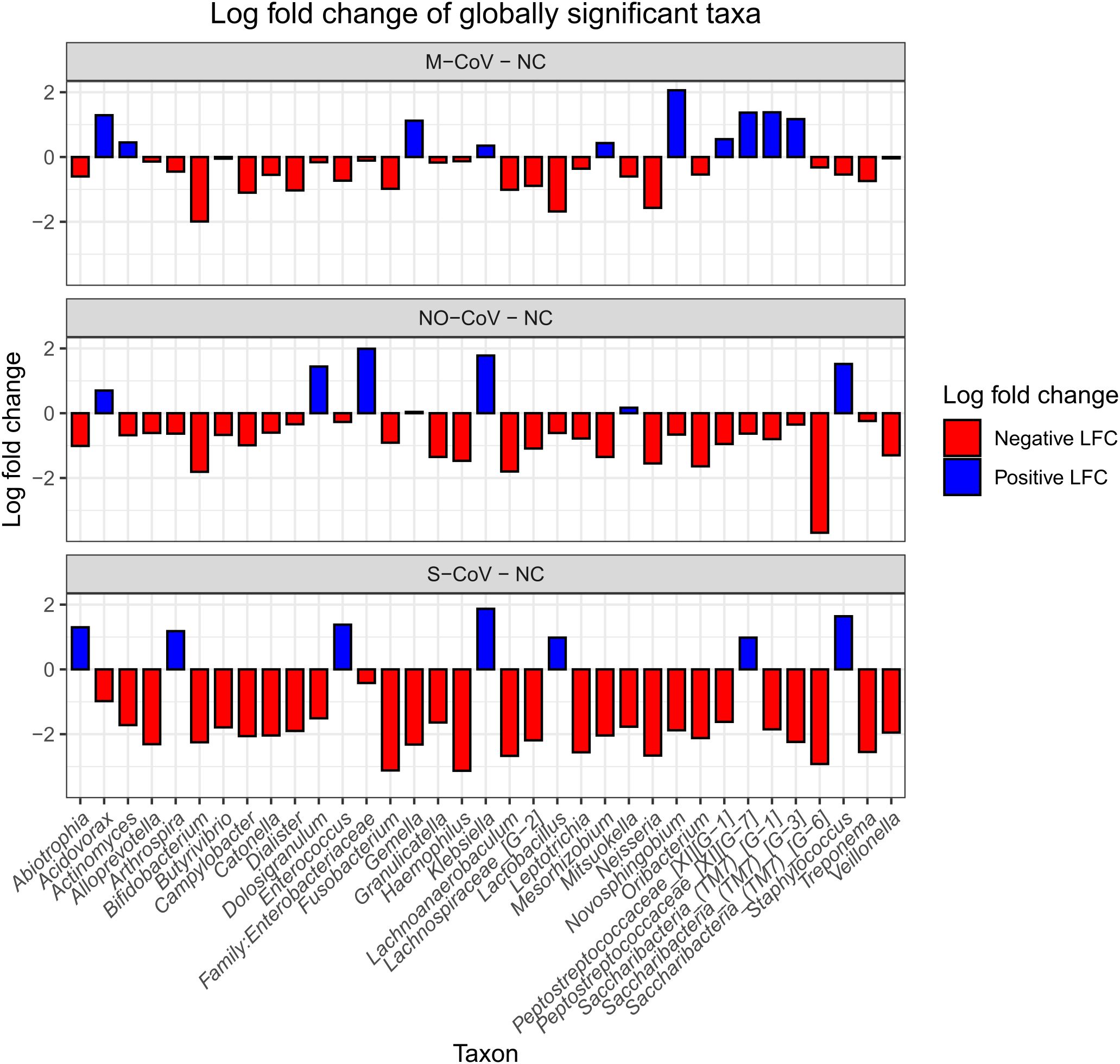
Figure 2. Log fold change (LFC) of globally significant taxa with NC as the reference group. M-CoV: moderate COVID-19; NC: Negative Control; NO-CoV: patients with pneumonia, ARDS, SpO2 ≥ 90% and a negative rt-qPCR test for SARS-CoV-2; S-CoV: severe COVID-19. Negative LFCs are colored red, and positive LFCs are colored blue.
The S-CoV group presented the most significant reduced differential abundance of eubiotic taxa and enrichment of potential pathogens such as Enterococcus sp., Klebsiella sp., and Staphylococcus sp. (Figure 2).
In the pairwise comparisons (Figure 3), differential abundance in taxa was observed among all COVID-19 groups, with the most significant differences observed between S-CoV and M-CoV (Lactobacillus sp., presented positive differential abundance for S-CoV) as well as S-CoV and NC group (no taxon presented positive LFC for S-CoV). All data generated from the differential abundance analysis are presented in Supplementary Material (Table 1 - Supplementary Data 7-Table 1 - Supplementary Data 10).
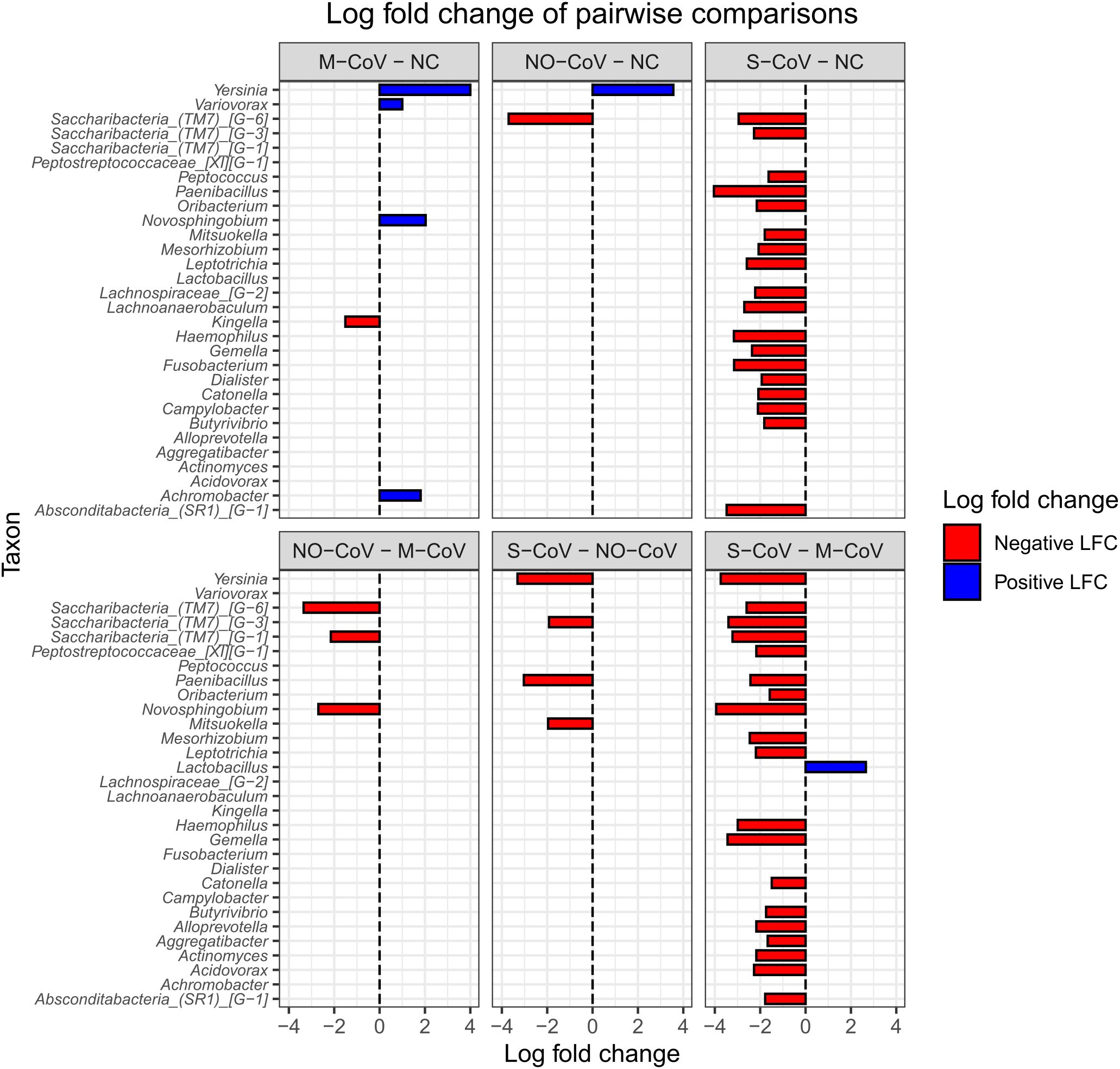
Figure 3. Log fold change for multiple pairwise comparisons between experimental groups. Only statistically significant results (p-adjusted) are demonstrated in the plot. M-CoV: moderate COVID-19; NC: Negative Control; NO-CoV: patients with pneumonia, ARDS, SpO2 ≥ 90% and a negative rt-qPCR test for SARS-CoV-2; S-CoV: severe COVID-19. Negative LFCs are colored red, and positive LFCs are colored blue.
3.3 Metabolic prediction
A total of 146 pathways presented statistically significant results in the differential abundance analysis (Table 1-Supplementary Data 11, Table 1-Supplementary Data 12), and the 30 metabolic pathways with the highest relative abundance are illustrated in Figure 4. It is possible to highlight that adenosylcobalamin (vitamin B12) and demethylmenaquinol−6 (vitamin K) biosynthesis; aromatic compounds degradation; purine nucleobases degradation; amino acid degradation; SCFA metabolism; sugar biosynthesis/lipopolysaccharide (LPS) biosynthesis/O-Antigen biosynthesis; steroid hormones degradation; amines and polyamines biosynthesis; amide, amidine, amine, and polyamine degradation; sugar degradation; tRNA processing; NAD metabolism; and protein N−glycosylation are among the most differentially abundant predicted ontologies.
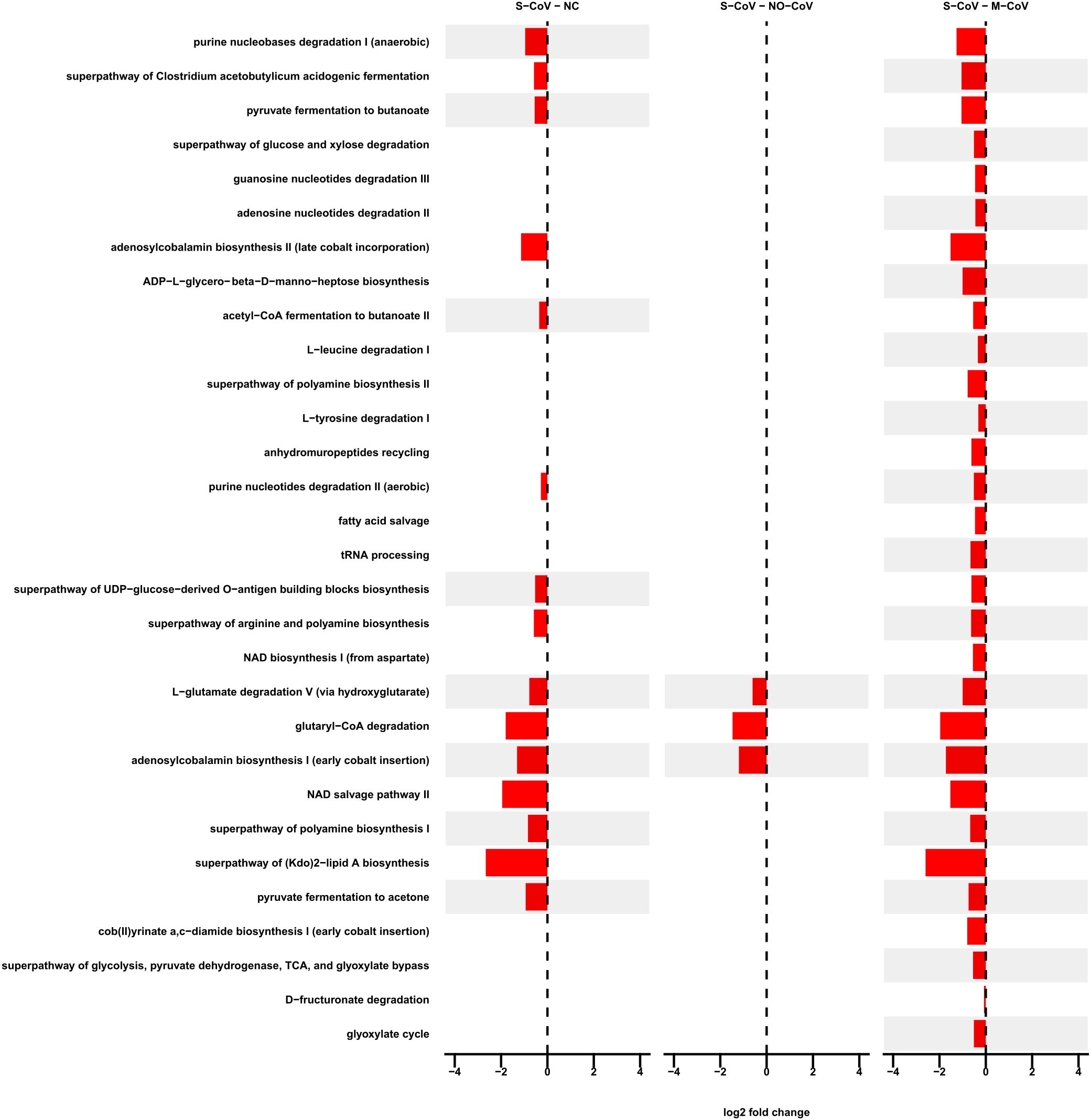
Figure 4. Differential abundance of metabolic pathways. Only statistically significant results (p-adjusted) for the top 30 pathways with the highest relative abundance are presented. NC - Negative Control; M-CoV - moderate COVID-19; NO-CoV - patients with pneumonia, ARDS, SpO2 ≥ 90% and a negative rt-qPCR test for SARS-CoV-2; S-CoV - severe COVID-19. Negative LFCs are colored red, and positive LFCs are colored blue. .
The most pronounced differences were observed between S-CoV and M-CoV, followed by S-CoV and NC. All the data generated from the pathways’ differential abundance are presented in Supplementary Material (Table 1 - Supplementary Data 11,Table 1 - Supplementary Data 12).
3.4 Pairwise log-ratio exploratory analysis
Differentially abundant taxa between experimental groups also presented significant associations with clinical variables (Supplementary Material - Table 2). The pair (s) of taxa whose log-ratio was more associated with the variables, the name of the most important taxa, and the correlation-like plot that provides the association of each pairwise log-ratio and their respective correlation values with each clinical variable are presented in the Data Sheet 3 – 5.0 Coda4Microbiome.
4 Discussion
Our study evaluated the upper respiratory tract microbiome in COVID-19 patients, revealing that severe cases (S-CoV) exhibit a statistically significant reduction in α-diversity compared to mild cases (M-CoV) and non-COVID patients (NC). This observation is consistent with previous reports linking reduced diversity to increased COVID-19 severity (Mostafa et al., 2020; Soffritti et al., 2021; Ke et al., 2022). Although β-diversity did not show significant differences - likely due to confounding factors such as antibiotic use and comorbidities (Maes et al.; Soffritti et al.; Nardelli et al., 2021) - the overall disturbance in microbial homeostasis appears to be associated with a more severe disease phenotype.
The URT microbiome was dominated by genera such as Streptococcus sp., Prevotella sp., Staphylococcus sp., Propionibacterium sp., Leptotrichia sp., Rothia sp., Dolosigranulum sp., Haemophilus sp., Moraxella sp., Veillonella sp., and Corynebacterium sp (Man et al., 2017; Wypych et al., 2019). Among these, certain opportunistic species - such as S. aureus and H. influenzae - are well-known contributors to pneumonia in critically ill COVID-19 patients (Bai et al., 2022). Notably, Abiotrophia sp. was enriched exclusively in the S-CoV group, which is positively correlated with COVID-19 severity (Hernández-Terán et al., 2021; Soffritti et al., 2021). Additionally, taxa like Saccharibacteria (specifically, Saccharibacteria_[(TM7]_(G1), (G3) and (G6))) were found in higher abundance in NC and paucisymptomatic patients, whereas ‘non-Saccharibacteria’ underrepresented taxa such as Mesorhizobium sp., Oribacterium sp., and Dolosigranulum sp. were reduced in S-CoV and associated with inflammatory biomarkers, suggesting a protective effect (Bosch et al., 2016; Brugger et al., 2016).
A group of beneficial taxa - particularly members of the Lachnospiraceae family (e.g., Catonella sp., Lachnoanaerobaculum sp., Butyrivibrio sp.) - were enriched in the NC group and inversely related to disease severity. These butyrate-producing bacteria have recognized roles in immune modulation and anti-inflammatory activity (Al Bataineh et al., 2021), suggesting that their depletion in S-CoV could impair protective mechanisms against SARS-CoV-2 infection. Similarly, Neisseria sp. - which may enhance anti-SARS-CoV-2 antibody production through interactions with T- and B-cells (Hung and Christodoulides, 2013; Santana-Mederos et al., 2022) - displayed a reduced differential abundance in S-CoV, further linking microbial composition to immune functionality.
In parallel with taxonomic alterations, our metabolic predictions revealed significant changes in key pathways that appear to reflect the functional impact of microbial dysbiosis in COVID-19. One set of interrelated findings involves the modulation of steroid hormone synthesis and vitamin metabolism. The S-CoV group showed a reduced abundance of pathways involved in steroid hormone synthesis (including androstenedione degradation), a process regulated by ACTH and the renin-angiotensin-aldosterone system (RAAS) that is linked to COVID-19 severity (Pal, 2020; Sezer et al., 2022). This observation is paralleled by the depletion of eubiotic taxa from the order Corynebacteriales (related to vitamin B12 biosynthesis) and the family Propionibacteriaceae (linked to vitamin B12, vitamin K biosynthesis, and SCFA metabolism) in S-CoV. In contrast, taxa such as Novosphingobium sp. were enriched only in M-CoV and correlated with critical inflammatory biomarkers, suggesting their possible role in mitigating severe inflammation.
Another functionally connected group of pathways relates to mucin metabolism and immune modulation. Several genera within the Peptostreptococcaceae family, known for cleaving and transporting mucin-associated monosaccharides (Flynn et al., 2016), were reduced in S-CoV and NC compared to M-CoV. This reduction aligns with observed decreases in the superpathway of fucose and rhamnose degradation—processes that influence mucin O-glycosylation and subsequent IgG Fc fucosylation (Bennett et al., 2012; Flynn et al., 2016; Wlodarska et al., 2017; Wang, 2019; Chakraborty et al., 2021). Such alterations may disrupt immune system activation and inflammatory signaling, further exacerbating disease severity.
Further, metabolic pathways involved in glycosaminoglycan (GAG) biosynthesis - evidenced by decreased superpathway of glucose and xylose degradation - may enhance the availability of xylose for GAG synthesis. This is of particular interest given that both Enterococcus sp. and Lactobacillus sp. (which carry glycosaminoglycan genetic clusters; (Kawai et al., 2018)) were enriched in S-CoV, suggesting a potential role in viral attachment via the S protein and consequent lung inflammation (Cagno et al., 2019; Kim et al., 2020).
Additional metabolic disruptions include reductions in pathways related to aromatic compound degradation, purine nucleobase metabolism, and amino acid degradation (e.g., L-tryptophan, L-glutamate, and L-lysine). These changes have been implicated in altered inflammatory responses and immune regulation (Chakraborty et al., 2021; Wu et al., 2021; Casas-Sanchez et al., 2022; Kasahara et al., 2023). The diminished capacity for polyamine and arginine metabolism, which are linked to enhanced IgA responses and vascular function (Durante, 2013, Durante, 2022; Rodriguez et al., 2017; Falck-Jones et al., 2021; Martí I Líndez and Reith, 2021; Zhao et al., 2023), further underscores the potential for metabolic impairment to contribute to severe COVID-19 outcomes.
Moreover, the decreased predicted abundance of pathways related to the biosynthesis of key microbial-derived components, such as vitamin B12 (Tamura et al., 1999; Yoshii et al., 2019) and vitamin K (Ragab et al., 2020; Kudelko et al., 2021), as well as SCFA metabolism (Dalile et al., 2019; Ashique et al., 2022; Batista et al., 2022), suggests that the loss of these beneficial metabolites may impair immune regulation and anti-inflammatory responses. This is reinforced by the observed reduction in taxa associated with SCFA production, including members of Lachnospiraceae and Propionibacteriaceae.
Lastly, the observed decreases in lipopolysaccharide (LPS) biosynthesis pathways (Maldonado et al., 2016; Bradley et al., 2022), in NAD metabolism (Zheng et al., 2022) and tRNA processing (Katanski et al., 2022) further indicate that key inflammatory and metabolic responses are disrupted in severe COVID-19.
Collectively, the close interrelation between specific taxa and their metabolic functions suggests that dysbiosis in the URT may directly impact host immune responses. The depletion of beneficial microbes such as Dolosigranulum sp. and butyrate-producing Lachnospiraceae, along with impaired vitamin and SCFA biosynthesis, could compromise mucosal immunity and facilitate an exaggerated inflammatory response. In addition, alterations in steroid hormone synthesis pathways, mucin glycosylation, and polyamine metabolism likely contribute to the cytokine dysregulation observed in severe COVID-19. This complex network of taxon - metabolic interactions underscores the potential of the URT microbiome as a modifiable factor in the progression of COVID-19.
5 Conclusions
This study demonstrates that the primary differences in URT microbiome composition and functionality are associated with COVID-19 severity rather than with SARS-CoV-2 infection. Severe COVID-19 is characterized by a significant reduction in α-diversity, a depletion of protective eubiotic taxa, and widespread alterations in metabolic pathways essential for immune modulation and host homeostasis. Despite inherent limitations (cross-sectional design, not controlling for confounding factors as age, antibiotic use, comorbidities), these findings suggest that specific microbial taxa – such as Lachnospiraceae, Propionibacteriaceae, Dolosigranulum sp. - and their metabolites - such as SCFAs, vitamins B12 and K, and key amino acids - could serve as promising targets for therapeutic intervention. Despite inherent limitations, our results provide a foundation for future studies aimed at restoring microbial homeostasis to mitigate severe respiratory outcomes.
Data availability statement
The datasets presented in this study can be found in online repositories. The names of the repository/repositories and accession number(s) can be found in the article/Supplementary Material.
Ethics statement
The studies involving humans were approved by Comitê de Ética em Pesquisa do Hospital de Clínicas de Porto Alegre. The studies were conducted in accordance with the local legislation and institutional requirements. The human samples used in this study were acquired from a by- product of routine care or industry. Written informed consent for participation was not required from the participants or the participants’ legal guardians/next of kin in accordance with the national legislation and institutional requirements.
Author contributions
OV: Conceptualization, Data curation, Formal analysis, Funding acquisition, Investigation, Methodology, Project administration, Resources, Software, Supervision, Validation, Visualization, Writing – original draft, Writing – review & editing. FZ: Conceptualization, Investigation, Methodology, Writing – review & editing. LW: Conceptualization, Data curation, Investigation, Software, Visualization, Writing – review & editing. AB: Conceptualization, Formal analysis, Funding acquisition, Investigation, Methodology, Project administration, Resources, Supervision, Writing – review & editing. AC: Writing – review & editing. AM: Conceptualization, Data curation, Formal analysis, Funding acquisition, Investigation, Methodology, Project administration, Resources, Supervision, Writing – review & editing.
Funding
The author(s) declare that financial support was received for the research and/or publication of this article. This study was financed in part by the Coordenação de Aperfeiçoamento de Pessoal de Nível Superior -Brazil (CAPES) -Finance Code 001; CHAMADA Decit/SCTIE/MS-CNPq-FAPERGS 08/2020 -PROGRAMA PESQUISA PARA O SUS: Gestão Compartilhada Em Saúde -PPSUS, grant 21/2551-0000063-5; Fundo de Incentivo à Pesquisa e Eventos (FIPE) of the Hospital de Clínicas de Porto Alegre (HCPA).
Acknowledgments
We want to thank the Hospital de Clinicas de Porto Alegre biobank for providing the samples. We want to thank Cassandro Davi Emer for the support on coding and statistical modeling. The experiments were performed in Laboratorio de Pesquisa em Resistencia Bacteriana (LABRESIS), and the bioinformatics analyses were performed using the Bioinformatics Core of HCPA.
Conflict of interest
The authors declare that the research was conducted in the absence of any commercial or financial relationships that could be construed as a potential conflict of interest.
Generative AI statement
The author(s) declare that Generative AI was used in the creation of this manuscript. For english revision.
Publisher’s note
All claims expressed in this article are solely those of the authors and do not necessarily represent those of their affiliated organizations, or those of the publisher, the editors and the reviewers. Any product that may be evaluated in this article, or claim that may be made by its manufacturer, is not guaranteed or endorsed by the publisher.
Supplementary material
The Supplementary Material for this article can be found online at: https://www.frontiersin.org/articles/10.3389/fcimb.2025.1531084/full#supplementary-material
References
Aitchison, J. (1982). The statistical analysis of compositional data. J. R. Stat. Society: Ser. B (Methodological) 44, 139–160. doi: 10.1111/j.2517-6161.1982.tb01195.x
Al Bataineh, M. T., Henschel, A., Mousa, M., Daou, M., Waasia, F., Kannout, H., et al. (2021). Gut microbiota interplay with COVID-19 reveals links to host lipid metabolism among middle eastern populations. Front. Microbiol. 12. doi: 10.3389/fmicb.2021.761067
Anderson, M. J. (2017). “Permutational multivariate analysis of variance (PERMANOVA),” in Wiley statsRef: statistics reference online. Eds. Kenett, R. S., Longford, N. T., Piegorsch, W. W., Ruggeri, F. (Massey University, Auckland, New Zealand: Wiley), 1–15. doi: 10.1002/9781118445112.stat07841
Ashique, S., De Rubis, G., Sirohi, E., Mishra, N., Rihan, M., Garg, A., et al. (2022). Short Chain Fatty Acids: Fundamental mediators of the gut-lung axis and their involvement in pulmonary diseases. Chemico-biological Interact. 368, 110231. doi: 10.1016/j.cbi.2022.110231
Bai, X., Narayanan, A., Skagerberg, M., Ceña-Diez, R., Giske, C. G., Strålin, K., et al. (2022). Characterization of the upper respiratory bacterial microbiome in critically ill COVID-19 Patients. Biomedicines 10, 982. doi: 10.3390/biomedicines10050982
Barreiro, P., San Román, J. (2022). Vulnerability to SARS-CoV-2 infection and disease: Ripping the curl after the storm. Rev. Esp Quimioter 35, 2–5. doi: 10.37201/req/s03.01.2022
Bars-Cortina, D. (2022). Alpha and beta-diversities performance comparison between different normalization methods and centered log-ratio transformation in a microbiome public dataset. Microbiology. doi: 10.1101/2022.11.07.512066
Batista, K. S., Cintra, V. M., Lucena, P. A. F., Manhães-de-Castro, R., Toscano, A. E., Costa, L. P., et al. (2022). The role of vitamin B12 in viral infections: a comprehensive review of its relationship with the muscle–gut–brain axis and implications for SARS-CoV-2 infection. Nutr. Rev. 80, 561–578. doi: 10.1093/nutrit/nuab092
Benjamini, Y., Hochberg, Y. (1995). Controlling the false discovery rate: A practical and powerful approach to multiple testing. J. R. Stat. Society: Ser. B (Methodological) 57, 289–300. doi: 10.1111/j.2517-6161.1995.tb02031.x
Bennett, E. P., Mandel, U., Clausen, H., Gerken, T. A., Fritz, T. A., Tabak, L. A. (2012). Control of mucin-type O-glycosylation: A classification of the polypeptide GalNAc-transferase gene family. Glycobiology 22, 736–756. doi: 10.1093/glycob/cwr182
Bogaert, D., de Groot, R., Hermans, P. (2004). Streptococcus pneumoniae colonization: The key to pneumococcal disease. Lancet Infect. Dis. 4, 144–154. doi: 10.1016/S1473-3099(04)00938-7
Bosch, A. A. T. M., Levin, E., Van Houten, M. A., Hasrat, R., Kalkman, G., Biesbroek, G., et al. (2016). Development of upper respiratory tract microbiota in infancy is affected by mode of delivery. EBioMedicine 9, 336–345. doi: 10.1016/j.ebiom.2016.05.031
Bradley, E. S., Zeamer, A. L., Bucci, V., Cincotta, L., Salive, M.-C., Dutta, P., et al. (2022). Oropharyngeal microbiome profiled at admission is predictive of the need for respiratory 2 support among COVID-19 Patients. Front. Microbiol. 13. doi: 10.3389/fmicb.2022.1009440
Brugger, S. D., Bomar, L., Lemon, K. P. (2016). Commensal–pathogen interactions along the human nasal passages. PloS Pathog 12, e1005633. doi: 10.1371/journal.ppat.1005633
Cagno, T., Jones, Tappare (2019). Heparan sulfate proteoglycans and viral attachment: True receptors or adaptation bias? Viruses 11, 596. doi: 10.3390/v11070596
Callahan, B. J., McMurdie, P. J., Rosen, M. J., Han, A. W., Johnson, A. J. A., Holmes, S. P. (2016a). DADA2: High-resolution sample inference from Illumina amplicon data. Nat. Methods 13, 581–583. doi: 10.1038/nmeth.3869
Callahan, B. J., Sankaran, K., Fukuyama, J. A., McMurdie, P. J., Holmes, S. P. (2016b). Bioconductor workflow for microbiome data analysis: From raw reads to community analyses. F1000Res 5, 1492. doi: 10.12688/f1000research.8986.2
Calle, M. L. (2019). Statistical analysis of metagenomics data. Genomics Inform 17, e6. doi: 10.5808/GI.2019.17.1.e6
Calle, M. L., Pujolassos, M., Susin, A. (2023). coda4microbiome: Compositional data analysis for microbiome cross-sectional and longitudinal studies. BMC Bioinf. 24, 82. doi: 10.1186/s12859-023-05205-3
Casas-Sanchez, A., Romero-Ramirez, A., Hargreaves, E., Ellis, C. C., Grajeda, B. I., Estevao, I. L., et al. (2022). Inhibition of protein N- glycosylation blocks SARS-CoV-2 infection. mBio 13, e03718–e03721. doi: 10.1128/mbio.03718-21
Caspi, R., Billington, R., Ferrer, L., Foerster, H., Fulcher, C. A., Keseler, I. M., et al. (2016). The MetaCyc database of metabolic pathways and enzymes and the BioCyc collection of pathway/genome databases. Nucleic Acids Res. 44, D471–D480. doi: 10.1093/nar/gkv1164
Chakraborty, S., Gonzalez, J., Edwards, K., Mallajosyula, V., Buzzanco, A. S., Sherwood, R., et al. (2021). Proinflammatory IgG Fc structures in patients with severe COVID-19. Nat. Immunol. 22, 67–73. doi: 10.1038/s41590-020-00828-7
Chen, N., Zhou, M., Dong, X., Qu, J., Gong, F., Han, Y., et al. (2020). Epidemiological and clinical characteristics of 99 cases of 2019 novel coronavirus pneumonia in Wuhan, China: A descriptive study. Lancet 395, 507–513. doi: 10.1016/S0140-6736(20)30211-7
Corbett, A. J., Eckle, S. B. G., Birkinshaw, R. W., Liu, L., Patel, O., Mahony, J., et al. (2014). T-cell activation by transitory neo-antigens derived from distinct microbial pathways. Nature 509, 361–365. doi: 10.1038/nature13160
Cox, M. J., Loman, N., Bogaert, D., O’Grady, J. (2020). Co-infections: Potentially lethal and unexplored in COVID-19. Lancet Microbe 1, e11. doi: 10.1016/S2666-5247(20)30009-4
Dalile, B., Van Oudenhove, L., Vervliet, B., Verbeke, K. (2019). The role of short-chain fatty acids in microbiota–gut–brain communication. Nat. Rev. Gastroenterol. Hepatol 16, 461–478. doi: 10.1038/s41575-019-0157-3
Douglas, G. M., Maffei, V. M., Zaneveld, J. R., Yurgel, S. N., Brown, J. R., Taylor, C. M., et al. (2020). PICRUSt2 for prediction of metagenome functions. Nat. Biotechnol. 38, 685–688. doi: 10.1038/s41587-020-0548-6
Durante, W. (2013). Role of arginase in vessel wall remodeling. Front. Immunol. 4. doi: 10.3389/fimmu.2013.00111
Durante, W. (2022). Targeting arginine in COVID-19-induced immunopathology and vasculopathy. Metabolites 12, 240. doi: 10.3390/metabo12030240
Escapa, I., Huang, Y., Chen, T., Lin, M., Kokaras, A., Dewhirst, F. E., et al. (2020). Construction of habitat-specific training sets to achieve species-level assignment in 16S rRNA gene datasets. Microbiome 8, 65. doi: 10.1186/s40168-020-00841-w
Falck-Jones, S., Vangeti, S., Yu, M., Falck-Jones, R., Cagigi, A., Badolati, I., et al. (2021). Functional monocytic myeloid-derived suppressor cells increase in blood but not airways and predict COVID-19 severity. J. Clin. Invest. 131, e144734. doi: 10.1172/JCI144734
Ferreira, C., Viana, S. D., Reis, F. (2020). Is gut microbiota dysbiosis a predictor of increased susceptibility to poor outcome of COVID-19 patients? An Update. Microorganisms 9, 53. doi: 10.3390/microorganisms9010053
Flerlage, T., Boyd, D. F., Meliopoulos, V., Thomas, P. G., Schultz-Cherry, S. (2021). Influenza virus and SARS-CoV-2: pathogenesis and host responses in the respiratory tract. Nat. Rev. Microbiol 19, 425–441. doi: 10.1038/s41579-021-00542-7
Flynn, J. M., Niccum, D., Dunitz, J. M., Hunter, R. C. (2016). Evidence and role for bacterial mucin degradation in cystic fibrosis airway disease. PloS Pathog 12, e1005846. doi: 10.1371/journal.ppat.1005846
Fukuda, S., Toh, H., Hase, K., Oshima, K., Nakanishi, Y., Yoshimura, K., et al. (2011). Bifidobacteria can protect from enteropathogenic infection through production of acetate. Nature 469, 543–547. doi: 10.1038/nature09646
Godfrey, D. I., Koay, H.-F., McCluskey, J., Gherardin, N. A. (2019). The biology and functional importance of MAIT cells. Nat. Immunol. 20, 1110–1128. doi: 10.1038/s41590-019-0444-8
Greenacre, M., Martínez-Álvaro, M., Blasco, A. (2021). Compositional data analysis of microbiome and any-omics datasets: A validation of the additive logratio transformation. Front. Microbiol. 12. doi: 10.3389/fmicb.2021.727398
Haegeman, B., Hamelin, J., Moriarty, J., Neal, P., Dushoff, J., Weitz, J. S. (2013). Robust estimation of microbial diversity in theory and in practice. ISME J. 7, 1092–1101. doi: 10.1038/ismej.2013.10
Hall, M. W., Joshi, I., Leal, L., Ooi, E. E. (2022). Immune immunomodulation in coronavirus disease 2019 (COVID-19): strategic considerations for personalized therapeutic intervention. Clin. Infect. Dis. 74, 144–148. doi: 10.1093/cid/ciaa904
Hernández-Terán, A., Mejía-Nepomuceno, F., Herrera, M. T., Barreto, O., García, E., Castillejos, M., et al. (2021). Dysbiosis and structural disruption of the respiratory microbiota in COVID-19 patients with severe and fatal outcomes. Sci. Rep. 11, 21297. doi: 10.1038/s41598-021-00851-0
Holm, S. (1979). A simple sequentially rejective multiple test procedure. Scandinavian J. Stat 6, 65–70.
Huber, W., Carey, V. J., Gentleman, R., Anders, S., Carlson, M., Carvalho, B. S., et al. (2015). Orchestrating high-throughput genomic analysis with Bioconductor. Nat. Methods 12, 115–121. doi: 10.1038/nmeth.3252
Hung, M.-C., Christodoulides, M. (2013). The biology of neisseria adhesins. Biology 2, 1054–1109. doi: 10.3390/biology2031054
Illumina (2013). Illumina 16s-metagenomic-library-prep-guide-15044223-b.pdf. Available online at: https://support.illumina.com/documents/documentation/chemistry_documentation/16s/16s-metagenomic-library-prep-guide-15044223-b.pdf (Accessed March, 2020).
Kasahara, K., Kerby, R. L., Zhang, Q., Pradhan, M., Mehrabian, M., Lusis, A. J., et al. (2023). Gut bacterial metabolism contributes to host global purine homeostasis. Cell Host Microbe 31, 1038–1053.e10. doi: 10.1016/j.chom.2023.05.011
Katanski, C. D., Alshammary, H., Watkins, C. P., Huang, S., Gonzales-Reiche, A., Sordillo, E. M., et al. (2022). tRNA abundance, modification and fragmentation in nasopharyngeal swabs as biomarkers for COVID-19 severity. Front. Cell Dev. Biol. 10. doi: 10.3389/fcell.2022.999351
Kawai, K., Kamochi, R., Oiki, S., Murata, K., Hashimoto, W. (2018). Probiotics in human gut microbiota can degrade host glycosaminoglycans. Sci. Rep. 8, 10674. doi: 10.1038/s41598-018-28886-w
Ke, S., Weiss, S. T., Liu, Y.-Y. (2022). Dissecting the role of the human microbiome in COVID-19 via metagenome-assembled genomes. Nat. Commun. 13, 5235. doi: 10.1038/s41467-022-32991-w
Kim, S. Y., Jin, W., Sood, A., Montgomery, D. W., Grant, O. C., Fuster, M. M., et al. (2020). Glycosaminoglycan binding motif at S1/S2 proteolytic cleavage site on spike glycoprotein may facilitate novel coronavirus (SARS-CoV-2) host cell entry. doi: 10.1101/2020.04.14.041459
Kim, M., Qie, Y., Park, J., Kim, C. H. (2016). Gut microbial metabolites fuel host antibody responses. Cell Host Microbe 20, 202–214. doi: 10.1016/j.chom.2016.07.001
Kirstahler, P., Bjerrum, S. S., Friis-Møller, A., la Cour, M., Aarestrup, F. M., Westh, H., et al. (2018). Genomics-based identification of microorganisms in human ocular body fluid. Sci. Rep. 8, 4126. doi: 10.1038/s41598-018-22416-4
Kissler, S. M., Tedijanto, C., Goldstein, E., Grad, Y. H., Lipsitch, M. (2020). Projecting the transmission dynamics of SARS-CoV-2 through the postpandemic period. Science. (2020) 368 (6493), 860–868. doi: 10.1126/science.abb5793
Kudelko, M., Yip, T. F., Hei Law, G. C., Lee, S. M. Y. (2021). Potential beneficial effects of vitamin K in SARS-CoV-2 induced vascular disease? Immuno 1, 17–29. doi: 10.3390/immuno1010003
Legoux, F., Salou, M., Lantz, O. (2020). MAIT Cell development and functions: The microbial connection. Immunity 53, 710–723. doi: 10.1016/j.immuni.2020.09.009
Lin, H., Peddada, S. D. (2020). Analysis of compositions of microbiomes with bias correction. Nat. Commun. 11, 3514. doi: 10.1038/s41467-020-17041-7
Lloréns-Rico, V., Gregory, A. C., Van Weyenbergh, J., Jansen, S., Van Buyten, T., Qian, J., et al. (2021). Clinical practices underlie COVID-19 patient respiratory microbiome composition and its interactions with the host. Nat. Commun. 12, 6243. doi: 10.1038/s41467-021-26500-8
Maldonado, R. F., Sá-Correia, I., Valvano, M. A. (2016). Lipopolysaccharide modification in Gram-negative bacteria during chronic infection. FEMS Microbiol. Rev. 40, 480–493. doi: 10.1093/femsre/fuw007
Man, W. H., de Steenhuijsen Piters, W. A. A., Bogaert, D. (2017). The microbiota of the respiratory tract: gatekeeper to respiratory health. Nat. Rev. Microbiol 15, 259–270. doi: 10.1038/nrmicro.2017.14
Martí I Líndez, A.-A., Reith, W. (2021). Arginine-dependent immune responses. Cell. Mol. Life Sci. 78, 5303–5324. doi: 10.1007/s00018-021-03828-4
McMurdie, P. J., Holmes, S. (2013). phyloseq: An R package for reproducible interactive analysis and graphics of microbiome census data. PloS One 8, e61217. doi: 10.1371/journal.pone.0061217
Merenstein, C., Bushman, F. D., Collman, R. G. (2022). Alterations in the respiratory tract microbiome in COVID-19: current observations and potential significance. Microbiome 10, 165. doi: 10.1186/s40168-022-01342-8
Monteiro, F. L., Volpato, F. C. Z., Wink, P. L., Willig, J. B., Paiva, R. M., De-Paris, F., et al. (2023). Comparison of singleplex and multiplex Real-Time Reverse Transcription – Polymerase Chain Reaction for the detection of Severe acute respiratory syndrome Coronavirus 2. Disciplinarum Scientia | Saúde. 24 (1), 49–61.
Mostafa, H. H., Fissel, J. A., Fanelli, B., Bergman, Y., Gniazdowski, V., Dadlani, M., et al. (2020). Metagenomic next-generation sequencing of nasopharyngeal specimens collected from confirmed and suspect COVID-19 patients. mBio 11, e01969–e01920. doi: 10.1128/mBio.01969-20
Pal, R. (2020). COVID-19, hypothalamo-pituitary-adrenal axis and clinical implications. Endocrine 68, 251–252. doi: 10.1007/s12020-020-02325-1
Parrot, T., Gorin, J.-B., Ponzetta, A., Maleki, K. T., Kammann, T., Emgård, J., et al. (2020). MAIT cell activation and dynamics associated with COVID-19 disease severity. Sci. Immunol. 5, eabe1670. doi: 10.1126/sciimmunol.abe1670
Pawlowsky-Glahn, V., Egozcue, J. J., Tolosana-Delgado, R. (2015). Modeling and analysis of compositional data (London: JohnWiley & Sons).
Ragab, D., Salah Eldin, H., Taeimah, M., Khattab, R., Salem, R. (2020). The COVID-19 cytokine storm; What we know so far. Front. Immunol. 11. doi: 10.3389/fimmu.2020.01446
R Core Team (2021). R: A language and environment for statistical computing. Available online at: https://www.R-project.org/ (Accessed October, 2021).
Rodriguez, P. C., Ochoa, A. C., Al-Khami, A. A. (2017). Arginine metabolism in myeloid cells shapes innate and adaptive immunity. Front. Immunol. 8. doi: 10.3389/fimmu.2017.00093
Rooks, M. G., Garrett, W. S. (2016). Gut microbiota, metabolites and host immunity. Nat. Rev. Immunol. 16, 341–352. doi: 10.1038/nri.2016.42
Rossman, H., Shilo, S., Meir, T., Gorfine, M., Shalit, U., Segal, E. (2021). COVID-19 dynamics after a national immunization program in Israel. Nat. Med. 27, 1055–1061. doi: 10.1038/s41591-021-01337-2
RStudio Team (2020). RStudio: integrated development for R. RStudio. Available online at: http://www.rstudio.com/ (Accessed October, 2021).
Santana-Mederos, D., Perez-Nicado, R., Climent, Y., Rodriguez, L., Ramirez, B. S., Perez-Rodriguez, S., et al. (2022). A COVID-19 vaccine candidate composed of the SARS-CoV-2 RBD dimer and Neisseria meningitidis outer membrane vesicles. RSC Chem. Biol. 3, 242–249. doi: 10.1039/D1CB00200G
Schaupp, L., Muth, S., Rogell, L., Kofoed-Branzk, M., Melchior, F., Lienenklaus, S., et al. (2020). Microbiota-induced type I interferons instruct a poised basal state of dendritic cells. Cell 181, 1080–1096.e19. doi: 10.1016/j.cell.2020.04.022
Sezer, S., Bal, C., Kalem, A. K., Kayaaslan, B., Eser, F., Hasanoglu, İ., et al. (2022). COVID-19 patients with altered steroid hormone levels are more likely to have higher disease severity. Endocrine 78, 373–379. doi: 10.1007/s12020-022-03140-6
Smith, P. M., Howitt, M. R., Panikov, N., Michaud, M., Gallini, C. A., Bohlooly-Y, M., et al. (2013). The microbial metabolites, short-chain fatty acids, regulate colonic T reg cell homeostasis. Science 341, 569–573. doi: 10.1126/science.1241165
Soffritti, I., D’Accolti, M., Fabbri, C., Passaro, A., Manfredini, R., Zuliani, G., et al. (2021). Oral microbiome dysbiosis is associated with symptoms severity and local immune/inflammatory response in COVID-19 patients: A cross-sectional study. Front. Microbiol. 12. doi: 10.3389/fmicb.2021.687513
Tamura, J., Kubota, K., Murakami, H., Sawamura, M., Matsushima, T., Tamura, T., et al. (1999). Immunomodulation by vitamin B12: augmentation of CD8+ T lymphocytes and natural killer (NK) cell activity in vitamin B12-deficient patients by methyl-B12 treatment. Clin. Exp. Immunol. 116, 28–32. doi: 10.1046/j.1365-2249.1999.00870.x
Tisoncik, J. R., Korth, M. J., Simmons, C. P., Farrar, J., Martin, T. R., Katze, M. G. (2012). Into the eye of the cytokine storm. Microbiol Mol. Biol. Rev. 76, 16–32. doi: 10.1128/MMBR.05015-11
Wang, T. T. (2019). “IgG fc glycosylation in human immunity,” in Fc mediated activity of antibodies. Eds. Ravetch, J. V., Nimmerjahn, F. (Springer International Publishing, Cham), 63–75. doi: 10.1007/82_2019_152
Wang, B., Zhang, L., Wang, Y., Dai, T., Qin, Z., Zhou, F., et al. (2022). Alterations in microbiota of patients with COVID-19: potential mechanisms and therapeutic interventions. Sig Transduct Target Ther. 7, 143. doi: 10.1038/s41392-022-00986-0
Wei, W. E., Li, Z., Chiew, C. J., Yong, S. E., Toh, M. P., Lee, V. J. (2020). Presymptomatic transmission of SARS-coV-2 — Singapore, january 23–march 16, 2020. MMWR Morb. Mortal. Wkly. Rep. 69, 411–415. doi: 10.15585/mmwr.mm6914e1
Wlodarska, M., Luo, C., Kolde, R., d’Hennezel, E., Annand, J. W., Heim, C. E., et al. (2017). Indoleacrylic acid produced by commensal peptostreptococcus species suppresses inflammation. Cell Host Microbe 22, 25–37.e6. doi: 10.1016/j.chom.2017.06.007
Wong, J. J. M., Leong, J. Y., Lee, J. H., Albani, S., Yeo, J. G. (2019). Insights into the immuno-pathogenesis of acute respiratory distress syndrome. Ann. Transl. Med. 7, 504–504. doi: 10.21037/atm.2019.09.28
World Health Organization (2020). Clinical management of COVID-19: interimguidance, 27 May 2020. Available online at: https://iris.who.int/handle/10665/332196 (Accessed May 8, 2020).
Wu, J., Zhao, M., Li, C., Zhang, Y., Wang, D. W. (2021). The SARS-CoV-2 induced targeted amino acid profiling in patients at hospitalized and convalescent stage. Bioscience Rep. 41, BSR20204201. doi: 10.1042/BSR20204201
Wypych, T. P., Wickramasinghe, L. C., Marsland, B. J. (2019). The influence of the microbiome on respiratory health. Nat. Immunol. 20, 1279–1290. doi: 10.1038/s41590-019-0451-9
Yang, C., Mai, J., Cao, X., Burberry, A., Cominelli, F., Zhang, L. (2023). ggpicrust2: an R package for PICRUSt2 predicted functional profile analysis and visualization. Bioinformatics 39, btad470. doi: 10.1093/bioinformatics/btad470
Ye, Y., Doak, T. G. (2009). A parsimony approach to biological pathway reconstruction/inference for genomes and metagenomes. PloS Comput. Biol. 5, e1000465. doi: 10.1371/journal.pcbi.1000465
Yeoh, Y. K., Zuo, T., Lui, G. C.-Y., Zhang, F., Liu, Q., Li, A. Y., et al. (2021). Gut microbiota composition reflects disease severity and dysfunctional immune responses in patients with COVID-19. Gut 70, 698–706. doi: 10.1136/gutjnl-2020-323020
Yoshii, K., Hosomi, K., Sawane, K., Kunisawa, J. (2019). Metabolism of dietary and microbial vitamin B family in the regulation of host immunity. Front. Nutr. 6. doi: 10.3389/fnut.2019.00048
Zhao, S., Lok, K. Y. W., Sin, Z. Y., Peng, Y., Fan, H. S. L., Nagesh, N., et al. (2023). COVID-19 mRNA vaccine-mediated antibodies in human breast milk and their association with breast milk microbiota composition. NPJ Vaccines. 8, 151. doi: 10.1038/s41541-023-00745-4
Zheng, D., Liwinski, T., Elinav, E. (2020). Interaction between microbiota and immunity in health and disease. Cell Res. 30, 492–506. doi: 10.1038/s41422-020-0332-7
Zheng, M., Schultz, M. B., Sinclair, D. A. (2022). NAD+ in COVID-19 and viral infections. Trends Immunol. 43, 283–295. doi: 10.1016/j.it.2022.02.001
Keywords: SARS-CoV-2, COVID-19, microbiome, upper respiratory tract, immune modulation
Citation: von Ameln Lovison O, Zempulski Volpato FC, Weber LG, Barth AL, Simon Coitinho A and Martins AF (2025) Unveiling the role of the upper respiratory tract microbiome in susceptibility and severity to COVID-19. Front. Cell. Infect. Microbiol. 15:1531084. doi: 10.3389/fcimb.2025.1531084
Received: 19 November 2024; Accepted: 21 April 2025;
Published: 13 May 2025.
Edited by:
Veeranoot Nissapatorn, Walailak University, ThailandReviewed by:
Sreya Ghosh, Boston Children’s Hospital and Harvard Medical School, United StatesDonald O. Natvig, University of New MexicoA, United States
Copyright © 2025 von Ameln Lovison, Zempulski Volpato, Weber, Barth, Simon Coitinho and Martins. This is an open-access article distributed under the terms of the Creative Commons Attribution License (CC BY). The use, distribution or reproduction in other forums is permitted, provided the original author(s) and the copyright owner(s) are credited and that the original publication in this journal is cited, in accordance with accepted academic practice. No use, distribution or reproduction is permitted which does not comply with these terms.
*Correspondence: Otávio von Ameln Lovison, b3RhdmlvbG92aXNvbkBnbWFpbC5jb20=