- 1INIAV—National Institute for Agrarian and Veterinary Research, Vila do Conde, Portugal
- 2LEPABE - Laboratory for Process Engineering, Environment, Biotechnology and Energy, Faculty of Engineering, University of Porto, Porto, Portugal
- 3ALiCE - Associate Laboratory in Chemical Engineering, Faculty of Engineering, University of Porto, Porto, Portugal
- 4CEB - Centre of Biological Engineering, University of Minho, Braga, Portugal
- 5CE3C—Centre for Ecology, Evolution and Environmental Changes & CHANGE, Global Change and Sustainability Institute, Faculty of Sciences, University of Lisbon, Lisboa, Portugal
- 6LABBELS – Associate Laboratory, Braga, Guimarães, Portugal
- 7Department of Medicine, University of Massachusetts Chan Medical School, Worcester, MA, United States
Foodborne outbreaks affecting millions of people worldwide are a significant and growing global health threat, exacerbated by the emergence of new and increasingly virulent foodborne pathogens. Traditional methods of detecting these outbreaks, including culture-based techniques, serotyping and molecular methods such as real-time PCR, are still widely used. However, these approaches often lack the precision and resolution required to definitively trace the source of an outbreak and distinguish between closely related strains of pathogens. Whole genome sequencing (WGS) has emerged as a revolutionary tool in outbreak investigations, providing high-resolution, comprehensive genetic data that allows accurate species identification and strain differentiation. WGS also facilitates the detection of virulence and antimicrobial resistance (AMR) genes, providing critical insight into the potential pathogenicity, treatment/control options and risks of spreading foodborne pathogens. This capability enhances outbreak surveillance, source tracing and risk assessment, making WGS an increasingly integrated component of public health surveillance systems. Despite its advantages, the widespread implementation of WGS faces several pressing challenges, including high sequencing costs, the need for specialized bioinformatics expertise, limited computational infrastructure in resource-constrained settings, and the standardization of data-sharing frameworks across regulatory and public health agencies. Addressing these barriers is crucial to maximizing the impact of WGS on foodborne disease surveillance. Even so, WGS is emerging as a vital tool in food safety and public health, and its potential to become the gold standard in outbreak detection has been recognized by public health authorities in the USA, the European Union, Australia and China, for example. This review highlights the role of WGS in foodborne outbreak investigations, its implementation challenges, and its impact on public health surveillance.
1 Introduction
Foodborne diseases cause approximately 420,000 deaths annually, with children under five accounting for 30% of deaths (Kirk et al., 2015). These diseases are more severe in low- and middle-income countries due to inadequate public health infrastructure (WHO, 2025; Hoffmann et al., 2019). Infections are usually caused by consuming food or water contaminated with bacteria, viruses, fungi, parasites, or toxins produced by them (Supplementary Table 1). The growing global food market increases the risk of outbreaks (Grace, 2015), highlighting the need for better detection systems to control foodborne diseases.
Traditional methods, including culture-based, biochemical, immunological and molecular (PCR/qPCR) techniques, are widely used to detect foodborne pathogens (Supplementary Table 2) (Priyanka et al., 2016). While effective, they lack the precision required for rapid outbreak management (Quintela et al., 2022). Whole genome sequencing (WGS) addresses these limitations by providing comprehensive genomic data to characterize virulence and antimicrobial resistance traits, distinguish closely related strains, and trace outbreak sources (Li et al., 2021).
In this way, the implementation of WGS has revolutionized the field of foodborne outbreak investigation. The ability to use high-resolution genomic data to complement epidemiological data allows health authorities to respond more quickly and accurately to outbreaks, reducing their spread and decreasing public health problems (Tang and Gardy, 2014). Additionally, WGS has been shown to establish links between distinct outbreaks and/or between geographically distant cases, a feature which traditional methods are too slow or limited to achieve due to the limited genomic information obtained (Koutsoumanis et al., 2019). Despite its benefits, WGS faces challenges such as the need for specialized equipment, bioinformatics expertise and high costs, which limit its widespread adoption (Chrystoja and Diamandis, 2014). This review highlights the critical role of WGS in foodborne outbreak investigations and public health surveillance.
2 WGS, a powerful tool in accessing foodborne outbreaks
WGS has emerged as a groundbreaking tool in the field of food safety and public health (Pightling et al., 2018). Its detailed characterization capabilities, including the identification of virulence factors and antimicrobial resistance genes, are expected to lead to its replacement of traditional methods. WGS offers improved surveillance of foodborne pathogens throughout the food supply chain (Allard et al., 2019; Collineau et al., 2019) and enables genetic comparisons to trace pathogen origins (Kovac et al., 2021). Table 1 highlights the strengths and weaknesses of traditional approaches, emphasizing WGS as a powerful modern tool while recognizing the practicality and accessibility of conventional methods.
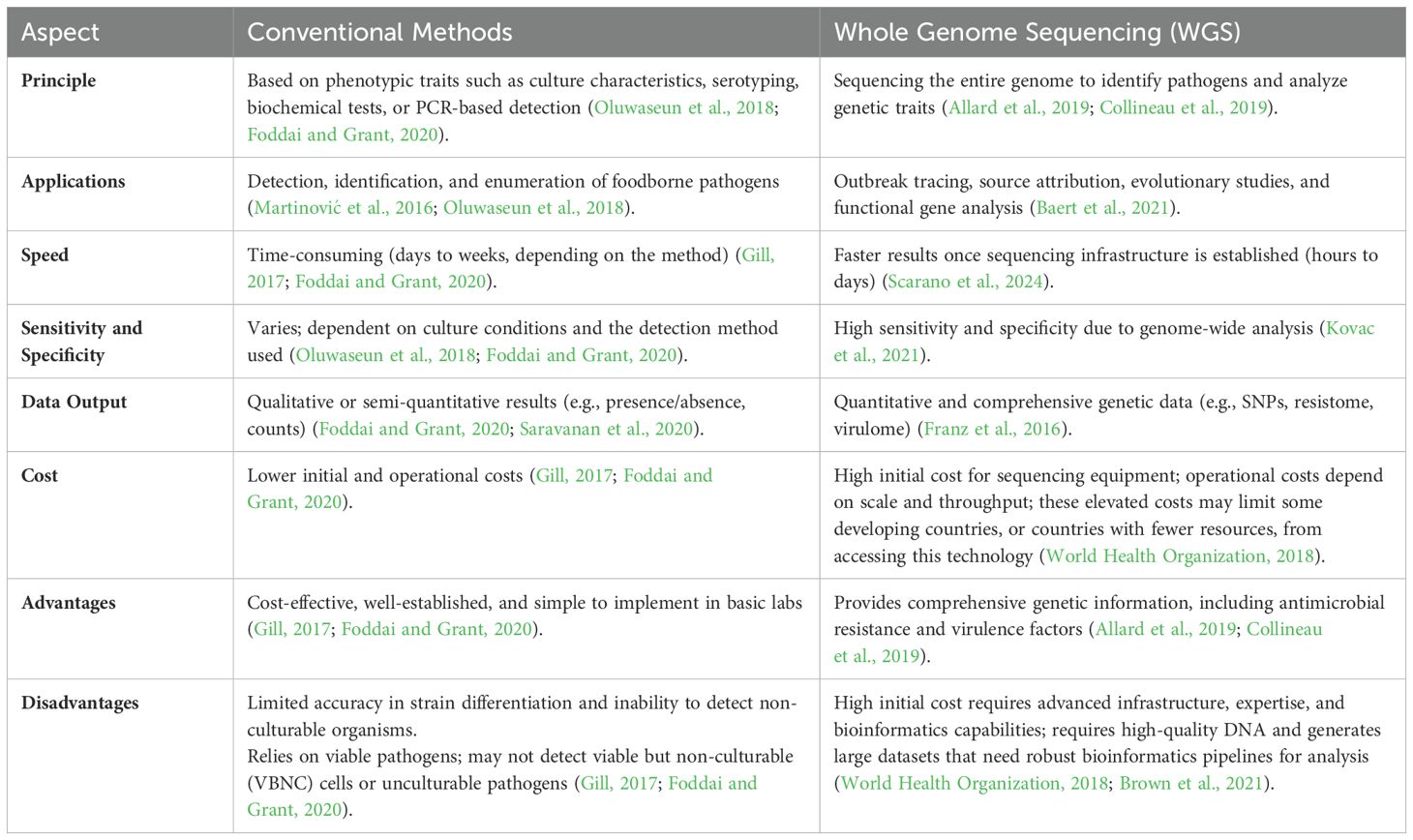
Table 1. Comparative analysis of strengths and weaknesses of conventional methods and whole genome sequencing (WGS).
2.1 WGS technologies and methodological analysis
The crucial role of WGS is to determine the complete genomic sequence of a given organism. This was only possible with the evolution of second- and third-generation sequencing technologies, which have made this technique very cost and time-effective (Stevens et al., 2022). Second-generation technologies, also known as next-generation sequencing (NGS), sequence thousands of small DNA fragments, which can be assembled to reconstruct the complete genome of the isolates. Recent third-generation sequencing (TGS), usually divided into two main technologies, Pacific Biosciences (PacBio) and Oxford Nanopore Technologies (ONT), uses innovative sequencing principles that allow direct sequencing of long genome sequences and do not require complex post-construction of the genome. TGS also provides rapid sequencing with real-time data analysis, particularly useful in time-sensitive outbreak responses, and allows for direct sequencing of native DNA or RNA, preserving epigenetic modifications (Scarano et al., 2024). Despite these advantages, TGS does have limitations. The raw error rates are generally higher than those of second-generation sequencing platforms such as Illumina, and, while costs for TGS technologies are decreasing, they remain relatively higher for high-throughput applications compared to second-generation sequencing (Ling et al., 2023). The issues associated with sequencing errors can be mitigated by combining second- and third-generation sequencing results to accurately assemble the pathogen genome (Xiao and Zhou, 2020). Supplementary Table 3 highlights the key differences between Illumina, ONT, and PacBio technologies.
In terms of analysis, the complete genome can then be compared with other known sequences deposited in public health databases, such as those available in PulseNet or The European Surveillance System (TESSy). Additionally, it can be compared with current typing databases, such as core-genome and whole-genome Multilocus Sequence Typing (MLST) (cgMLST/wgMLST), and virulence and/or AMR gene databases for rapid identification of protein-encoding alleles (Franz et al., 2016). This analysis allows the detection of subtle genetic differences that may indicate whether the pathogen comes from a common source or if it is part of a larger outbreak with multiple origins (Rantsiou et al., 2018; Stevens et al., 2022). However, WGS data analysis can be performed using multiple strategies, which introduces variability and reduces the ease of standardization, making it challenging for epidemiologists to interpret the results (Franz et al., 2016). Some of the most used methods include the k-mer approach, which uses the frequency of k-mers to create phylogenetic trees and reference-based methods that align sequenced reads to a common reference genome in order to identify single nucleotide polymorphisms (SNPs) (Franz et al., 2016). In regulatory settings, approaches such as cgMLST are often preferred over SNP-based pipelines because they provide a standardized, reproducible framework based on conserved genomic regions, making data easily comparable across laboratories and jurisdictions, facilitating faster and more reliable outbreak detection, and supporting the integration of genomic data into public health surveillance systems. There are also other alternatives, such as the nucleotide difference approach, which measures differences between reference genomes. The kSNP (k-mer-based Single Nucleotide Polymorphism) method compares the frequency of unique k-mers without prior knowledge but may be affected by mobile genetic elements (Gardner et al., 2015; Franz et al., 2016). Overall, different methodologies for WGS analysis are being developed and shared, leading to limitations in the standardization of the technique (Timme et al., 2019; Uelze et al., 2020).
2.2 Global implementation of WGS in foodborne pathogen surveillance: opportunities and challenges
As a new crucial tool for foodborne pathogen surveillance, public health agencies are beginning to implement WGS routinely to track outbreaks more precisely and accurately, enabling more effective responses. In United States of America (USA), the Centers for Disease Control and Prevention (CDC) and the Food and Drug Administration (FDA) created a special program called GenomeTrakr, which is responsible for creating a database with the sequences of pathogens from food and environmental samples (Allard et al., 2016). In the United Kingdom (UK), WGS has also been integrated into the national surveillance system and is used by the United Kingdom Health Security Agency (UKHSA) to detect important pathogens, including foodborne pathogens (Gerner-Smidt et al., 2017). Australia has also followed the UK and USA in adopting WGS as a tool to improve foodborne disease surveillance and response by creating a national program of pathogen genomics for public health, the Australian Pathogen Genomics Program (AusPathoGen) (Webb et al., 2024). Recently, the European Union (EU) has adopted a new regulation requiring Member States to conduct WGS on the isolates of five important pathogens (Salmonella enterica, Listeria monocytogenes, Escherichia coli, Campylobacter jejuni and Campylobacter coli) during the investigations of foodborne illness outbreaks, and sets data-sharing parameters (EU regulation 2025/179) to facilitate foodborne illness outbreak investigations and enable the timely detection of sources and causes. In addition, the European Food Safety Authority (EFSA) has been working with the European Centre for Disease Prevention and Control (ECDC) to develop a joint One Health system that allows the monitoring and control of foodborne diseases across borders, leading to more efficient management and control of the outbreaks (Koutsoumanis et al., 2019). Additionally, in Asia, significant advancements in the use of WGS have been made to enhance food safety and public health. In China, the National Molecular Tracing Network for Foodborne Disease Surveillance (TraNet), launched in 2013, uses WGS for real-time subtyping of foodborne pathogens, which has greatly improved outbreak investigations, source tracking, and cluster analysis across the country (Li et al., 2021). Similarly, in India, WGS has been applied to analyze antimicrobial resistance in milk and dairy-derived pathogens. A study in Anand, Gujarat, assessed the genetic diversity and resistance profiles of these pathogens, demonstrating the potential of WGS for monitoring and managing foodborne diseases in the region (Hati et al., 2024).
Although WGS offers numerous advantages, it is not without its challenges, namely in low- and middle-income countries (LMICs). LMICs are currently facing difficulties in adopting WGS for food safety and AMR surveillance, with one of the main issues being the high costs associated with the required equipment (Apruzzese et al., 2019; Vegyari et al., 2020; Price et al., 2023). In addition, for WGS to work properly, there must be a constant supply of electricity, clean water, and controlled temperatures, some of which are not always reliable in these regions (Vegyari et al., 2020). These added costs make it especially difficult for LMICs to sustain WGS over the long term. These challenges exacerbate the disparity between high-income countries and LMICs in the implementation of WGS for public health (Apruzzese et al., 2019; Vegyari et al., 2020; Price et al., 2023). While wealthier countries are using WGS for food safety and AMR surveillance, LMICs are struggling with resource limitations, widening global inequalities, and hindering compliance with evolving food safety regulations (Apruzzese et al., 2019). Addressing this gap requires urgent investment and innovative solutions such as mobile sequencing labs, the development of regional genomics hubs, specialized training, and the involvement of international aid programs to ensure collaborative and equitable access to the benefits of WGS for all countries (Apruzzese et al., 2019; Vegyari et al., 2020; Price et al., 2023). Another challenge faced in routinely implementing WGS involves having skilled personnel with bioinformatics expertise to analyze and manage the high amount of bioinformatics data generated.
3 WGS for foodborne outbreak detection and traceback investigation
The true value of WGS is measured in its real-world application (Morton et al., 2024). It provides a comprehensive analysis of the entire genome, allowing public health authorities to identify outbreak-associated pathogens that are difficult or even impossible to distinguish using traditional typing methods (Chattaway et al., 2019). This process includes the following steps, as also described in Figure 1:
i. Sample collection: Samples can be collected from patients, food, and environmental sources (Chen et al., 2020). The microorganisms are then isolated using culturomics, and the DNA/RNA is extracted and purified.
ii. Sequencing and analysis: The DNA/RNA of isolated pathogens is sequenced, and the raw data is processed and analyzed to identify/characterize the species and even determine if there are any similarities between different samples (Chen et al., 2020).
iii. Databases comparison: The outbreak strains are compared and deposited in national and international databases by the authorities — this is followed by statistical analysis to identify the patterns that can further be used to trace back to the suspected source of contamination (Chen et al., 2020).
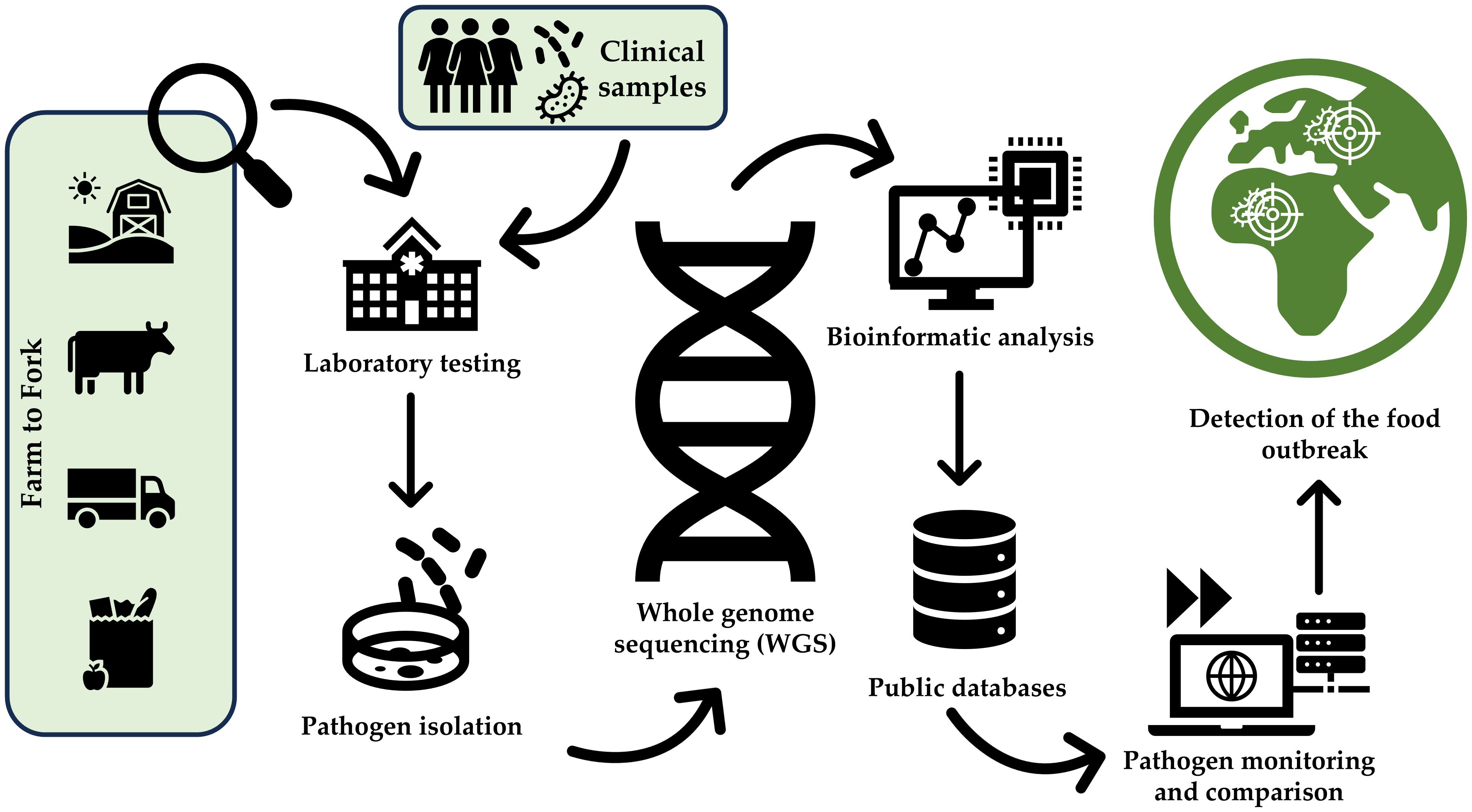
Figure 1. General workflow for foodborne outbreak detection using whole genome sequencing (WGS). The process begins with sample preparation, including the isolation and purification of DNA from food, environmental, or clinical samples. Sequencing is performed using high-throughput platforms to generate raw reads, which undergo quality control and genome assembly. The assembled genomes are annotated to identify genetic features. Integration of genomic and epidemiological data facilitates the identification of outbreak clusters and potential contamination sources, supporting effective public health responses.
This method of matching the outbreak strains more quickly, and perhaps even from across borders, allows the health authorities to take early mitigation action before the outbreak spreads further (Holmes et al., 2015).
In addition to the consequences for human health, an outbreak can have a significant impact on world trade (Tibebu et al., 2024). In some cases, the affected countries/regions may be subject to trade bans with several countries or even more stringent border controls (Jansen et al., 2019). This can have a detrimental effect on food logistics and, by extension, the global/national economy. The various consequences of an outbreak highlight the need for the rapid recognition of such events and the subsequent rapid implementation of appropriate containment measures. By enabling timely interventions, WGS significantly reduces the financial burden associated with foodborne outbreaks. Early detection and traceback minimize healthcare costs, mitigate losses from product recalls, and prevent trade disruptions. This proactive approach not only enhances public health protection but also strengthens the economic resilience of the food industry by reducing the overall impact of outbreaks.
Thus, WGS has been essential in tracing outbreak sources, controlling foodborne disease spread, and analyzing pathogen virulence. Supplementary Table 4 highlights key studies demonstrating its application in outbreak detection and virulence analysis. For instance, in 2022, there was a Salmonella Typhimurium outbreak in the USA, where WGS was crucial for the genetic characterization of Salmonella isolates, tracing the possible source of the outbreak, and linking it to a cantaloupe farm, which enabled public authorities to take the necessary actions (Seelman Federman et al., 2024). Additionally, WGS also made it possible to determine antimicrobial resistance, detect virulence factors, and perform phylogenetic analysis, providing insights into the epidemiology and genetic characteristics of Salmonella isolates from poultry meat in Pakistan (Siddique et al., 2024).
4 Use of WGS in the routine inspection process
WGS methodologies have the potential to become an essential tool in food surveillance. By determining the genetic information of harmful pathogens, health authorities can not only detect their presence, allowing early identification of potential outbreaks, but also identify genes that encode AMR and/or virulence factors (Kovac et al., 2021). The use of sequence alignment algorithms through reliable open-access databases of genes and mutations, such as ResFinder or VirulenceFinder (hosted by the Center for Genomic Epidemiology at the Technical University of Denmark), makes it possible to identify and predict antimicrobial resistance and virulence phenotypes in a simple and effective way (Stevens et al., 2022).
WGS fulfills all the requirements to become a standard procedure in food safety inspection practices, which is already practiced by some public health and regulatory agencies, such as the CDC/FDA and the ECDC/EFSA (Brown et al., 2019). During these routine inspections, food samples from various stages of production, from raw ingredients, in-process samples, and final products, can be tested for pathogens using WGS. Moreover, environmental swabs from food production facilities and processing equipment can also be analyzed (Lakicevic et al., 2023). The data obtained from WGS will allow the identification and comparison of species and strains of the pathogens, enabling the detection and comparison of minimal differences between samples and/or related bacteria (Stevens et al., 2022).
Future advances in sequencing technologies, such as the Oxford Nanopore’s MinION, which is a portable sequencing device designed for used in the field, will enable real-time, on-site WGS for public health inspection (Billington et al., 2022; Samdarshi, 2024). Additionally, AI-driven data analysis will simplify WGS interpretation and improve outbreak detection with faster and more accurate source identification. As these technologies evolve, costs will decrease, encouraging wider adoption in global food safety surveillance (Du and Guo, 2022; Qian et al., 2023).
5 WGS data sharing
5.1 Standardization of workflows to ensure the generation of comparable results
The introduction of WGS has revolutionized cross-border surveillance and outbreak investigation, establishing bioinformatic analysis of pathogen genomes as a potential gold standard. However, effective collaboration between microbiologists and informaticists and standardized methodologies are crucial for generating comparable data across laboratories (Gilchrist et al., 2015; Koutsoumanis et al., 2019).
At the European level, various initiatives have been launched to standardize life sciences data management across the EU. For example, the EFSA has provided technical guidance for implementing the WGS One Health analytical pipeline (Costa et al., 2022). However, harmonizing data across the different sectors involved in this initiative (e. g. food safety, veterinary, and public health) has presented challenges. A key issue has been ensuring data compatibility between distinct pipelines, particularly regarding the integration of pathogen sequencing data from various sources. To address this, EFSA has worked closely with the European Centre for Disease Prevention and Control (ECDC) to align analytical methods and metadata standards, although inconsistencies in data reporting remain a challenge (ECDC et al., 2019).
The One Health WGS system connects two analytical pipelines: the food and veterinary pipeline, which provides data to EFSA’s One Health WGS analytical pipeline to generate derived data for studying foodborne outbreaks; and the public health pipeline, managed by ECDC, which receives and uses public health data to its analyses (ECDC et al., 2019). While this system aims to centralize pathogen surveillance, one of the challenges has been achieving widespread participation from laboratories across different countries, each with varying capacities in terms of technical infrastructure and expertise.
In overcome these challenges, ELIXIR, the European life sciences infrastructure, has initiated the ELIXIR-CONVERGE project, funded by the European Commission, to harmonize life science data management. This project offers a toolkit to make research data publicly accessible and expands scientists’ access to diverse datasets, including food-related information (Durinx et al., 2017).
Furthermore, the European Commission also established the Inter-European Union Reference Laboratories (EURLs) Working Group on NGS. This group aims to promote NGS adoption within EURL networks, enhance NGS capacity across the EU, and facilitate collaboration among the EURLs, EFSA, and ECDC. It includes all EURLs focusing on microbiological contamination in food and feed (Michelacci et al., 2023).
Additionally, the USA has made notable progress in using NGS for pathogen surveillance, but its approach is less centralized compared to the European Union’s efforts. A key initiative in the USA is the CDC’s PulseNet, a network that facilitates the sharing of WGS data between federal and state laboratories to track foodborne outbreaks (Tolar et al., 2019). Although PulseNet significantly improves outbreak detection, it operates independently of other initiatives like the FDA’s GenomeTrakr, which is more focused on genomic surveillance of foodborne pathogens (Allard et al., 2016; Kubota et al., 2019).
Overall, while both the EU and the USA have made significant progress in integrating WGS into public health surveillance, the EU adopts a more centralized approach through EFSA, ECDC, and ELIXIR, promoting uniform data management and cross-border collaboration. The EU’s more centralized approach helps mitigate some of the standardization issues but faces challenges due to differing national capacities and regulations. In contrast, the USA’s decentralized model, exemplified by PulseNet and GenomeTrakr, allows for more flexibility but may hinder cross-state and cross-sector integration of data. As both regions move forward, overcoming these challenges will be crucial for maximizing the global impact of WGS in pathogen surveillance and food safety efforts (Allard et al., 2016; Durinx et al., 2017; Tolar et al., 2019).
5.2 Interoperable data for foodborne outbreak surveillance
Genomic sequences should follow the Findable, Accessible, Interoperable, and Reusable (FAIR) Principles, ensuring standardized, interoperable data sharing across global platforms to enhance foodborne outbreak tracking and pathogen surveillance (Hovig et al., 2021). Researchers have two primary options for managing the data they generate:
i) Direct Submission, in which they can process, store, and submit their data directly to international repositories or databases. Research results, including data and metadata for outbreak tracking, should be shared in FAIR format. Platforms like GitHub are widely used to share bioinformatics pipelines, analysis scripts and metadata, while processed genomic data can subsequently be deposited and shared in established genomic repositories such as the European Nucleotide Archive (ENA) and the National Center for Biotechnology Information (NCBI). These platforms are crucial for direct data submission, providing standardized formats that facilitate data integration into ongoing public health initiatives and global pathogen surveillance systems.
ii) Data Brokerage Model in which an intermediary can curate the raw data, analyze it according to standardized guidelines, store it, and share processed, de-identified sequence and metadata needed for outbreak tracing with public health databases and international repositories (Singh et al., 2024).
EU Member States are required to provide the ECDC and/or EFSA with scientific and technical data relevant to its mission promptly. The ECDC manages the TESSy, a platform for collecting, analyzing, and disseminating surveillance data on infectious diseases across Europe (Kramarz et al., 2014; Walle et al., 2019). Competent national authorities supply comparable and compatible data on the epidemiological surveillance of communicable diseases and related health issues to this network. EFSA is also planning to create a platform dedicated to WGS data, which could be shared with ECDC to create a single database encompassing food and human outbreak data.
The implementation of these databases, such as ECDC/TESSy platform, facilitates sequence data sharing, integrative analysis, and reporting. Data providers upload sequences, metadata, and epidemiological data to a secure storage solution with controlled, reliable, long-term access. Genomic and epidemiological data are presented together with derived data, such as allele identification, genetic markers, strain nomenclature, and genetic distances generated and visualized for comprehensive analysis (Hawkins et al., 2010; Walle et al., 2019).
6 Conclusions
WGS has revolutionized foodborne outbreak detection with high-resolution genetic data for pathogen identification, traceability and AMR/virulence profiling. Despite challenges such as cost and infrastructure, the benefits outweigh the limitations, solidifying WGS as a crucial approach in foodborne pathogen investigation. Advances in technology and data sharing will enhance accessibility and strengthen food safety and public health efforts. Looking to the future, the integration of artificial intelligence for advanced data analysis and the development of portable sequencing devices hold significant potential to expand the reach and improve the effectiveness of WGS. These innovations are poised to further revolutionize global foodborne pathogen surveillance and offer exciting opportunities for the continued development and increased accessibility of WGS technologies in diverse settings.
Author contributions
EG: Methodology, Writing – original draft, Investigation. DA: Conceptualization, Investigation, Writing – review & editing. TN: Writing – original draft, Writing – review & editing, Investigation. RO: Writing – original draft, Writing – review & editing, Investigation. SS: Investigation, Writing – review & editing. LO: Investigation, Writing – review & editing. NFA: Funding acquisition, Supervision, Writing – review & editing. CA: Investigation, Writing – review & editing, Supervision. JC: Writing – review & editing, Writing – original draft, Resources, Conceptualization, Supervision, Investigation.
Funding
The author(s) declare that financial support was received for the research and/or publication of this article. This work is financially supported by national funds through the FCT/MCTES (PIDDAC), under the project 2022.07654.PTDC. This work was also supported by national funds through FCT/MCTES (PIDDAC): LEPABE, UIDB/00511/2020 (DOI: 10.54499/UIDB/00511/2020) and UIDP/00511/2020 (DOI: 10.54499/UIDP/00511/2020) and ALiCE, LA/P/0045/2020 (DOI: 10.54499/LA/P/0045/2020). In addition, this study was supported by the Portuguese Foundation for Science and Technology (FCT) under the scope of the strategic funding of UIDB/04469/2020 unit. JC, DA, and SS also thank FCT for the CEEC Individual (DOI: 10.54499/2022.06886.CEECIND/CP1737/CT0001, 2023.06040.CEECIND, and 10.54499/CEECINST/00018/2021/CP2806/CT0003, respectively).
Conflict of interest
The authors declare that the research was conducted in the absence of any commercial or financial relationships that could be construed as a potential conflict of interest.
The author(s) declared that they were an editorial board member of Frontiers, at the time of submission. This had no impact on the peer review process and the final decision.
Generative AI statement
The author(s) declare that no Generative AI was used in the creation of this manuscript.
Publisher’s note
All claims expressed in this article are solely those of the authors and do not necessarily represent those of their affiliated organizations, or those of the publisher, the editors and the reviewers. Any product that may be evaluated in this article, or claim that may be made by its manufacturer, is not guaranteed or endorsed by the publisher.
Supplementary material
The Supplementary Material for this article can be found online at: https://www.frontiersin.org/articles/10.3389/fcimb.2025.1593219/full#supplementary-material
References
Allard, M. W., Strain, E., Melka, D., Bunning, K., Musser, S. M., Brown, E. W., et al. (2016). Practical value of food pathogen traceability through building a whole-genome sequencing network and database. J. Clin. Microbiol 54, 1975–1983. doi: 10.1128/JCM.00081-16/ASSET/36ED1836-0AD4-433A-B18D-405B73EB730A/ASSETS/GRAPHIC/ZJM9990949700003.JPEG
Allard, M. W., Strain, E., Rand, H., Melka, D., Correll, W. A., Hintz, L., et al. (2019). Whole genome sequencing uses for foodborne contamination and compliance: Discovery of an emerging contamination event in an ice cream facility using whole genome sequencing. Infection Genet. Evol. 73, 214–220. doi: 10.1016/J.MEEGID.2019.04.026
Apruzzese, I., Song, E., Bonah, E., Sanidad, V. S., Leekitcharoenphon, P., Medardus, J. J., et al. (2019). Investing in food safety for developing countries: opportunities and challenges in applying whole-genome sequencing for food safety management. Foodborne Pathog Dis. 16, 463. doi: 10.1089/FPD.2018.2599
Baert, L., McClure, P., Winkler, A., Karn, J., Bouwknegt, M., Klijn, A. (2021). Guidance document on the use of whole genome sequencing (WGS) for source tracking from a food industry perspective. Food Control 130, 108148. doi: 10.1016/J.FOODCONT.2021.108148
Billington, C., Kingsbury, J. M., Rivas, L. (2022). Metagenomics approaches for improving food safety: A review. J. Food Prot 85, 448–464. doi: 10.4315/JFP-21-301
Brown, B., Allard, M., Bazaco, M. C., Blankenship, J., Minor, T. (2021). An economic evaluation of the Whole Genome Sequencing source tracking program in the U.S. PloS One 16, e0258262. doi: 10.1371/JOURNAL.PONE.0258262
Brown, E., Dessai, U., Mcgarry, S., Gerner-Smidt, P. (2019). Use of whole-genome sequencing for food safety and public health in the United States. Foodborne Pathog Dis. 16, 441–450. doi: 10.1089/FPD.2019.2662/ASSET/IMAGES/LARGE/FIGURE2.JPEG
Chattaway, M. A., Dallman, T. J., Larkin, L., Nair, S., McCormick, J., Mikhail, A., et al. (2019). The transformation of reference microbiology methods and surveillance for salmonella with the use of whole genome sequencing in England and wales. Front. Public Health 7. doi: 10.3389/FPUBH.2019.00317/BIBTEX
Chen, J., Karanth, S., Pradhan, A. K. (2020). Quantitative microbial risk assessment for Salmonella: Inclusion of whole genome sequencing and genomic epidemiological studies, and advances in the bioinformatics pipeline. J. Agric. Food Res. 2, 100045. doi: 10.1016/J.JAFR.2020.100045
Chrystoja, C. C., Diamandis, E. P. (2014). Whole genome sequencing as a diagnostic test: challenges and opportunities. Clin. Chem. 60, 724–733. doi: 10.1373/CLINCHEM.2013.209213
Collineau, L., Boerlin, P., Carson, C. A., Chapman, B., Fazil, A., Hetman, B., et al. (2019). Integrating whole-genome sequencing data into quantitative risk assessment of foodborne antimicrobial resistance: A review of opportunities and challenges. Front. Microbiol 10. doi: 10.3389/FMICB.2019.01107
Costa, G., Di Piazza, G., Koevoets, P., Iacono, G., Liebana, E., Pasinato, L., et al. (2022). Guidelines for reporting Whole Genome Sequencing-based typing data through the EFSA One Health WGS System Vol. 19 (Parma, Italy: EFSA Supporting Publications). doi: 10.2903/SP.EFSA.2022.EN-7413
Du, Y., Guo, Y. (2022). Machine learning techniques and research framework in foodborne disease surveillance system. Food Control 131, 108448. doi: 10.1016/J.FOODCONT.2021.108448
Durinx, C., McEntyre, J., Appel, R., Apweiler, R., Barlow, M., Blomberg, N., et al. (2017). Identifying ELIXIR core data resources. F1000Research 5, 2422. doi: 10.12688/f1000research.9656.2
ECDC (European Centre for Disease Prevention and Control), EFSA (EuropeanFood Safety Authority), Van Walle, I., Guerra, B., Borges, V., Carriço, JA., et al. (2019). EFSA and ECDC technical report on the collection and analysis of whole genome sequencing data from food-bornepathogens and other relevant microorganisms isolated from human, animal, food, feed and food/feedenvironmental samples in the joint ECDC–EFSA molecular typing databas. EFSA supporting publication2019:EN-1337 92 pp. doi: 10.2903/sp.efsa.2019.EN-1337
Foddai, A. C. G., Grant, I. R. (2020). Methods for detection of viable foodborne pathogens: current state-of-art and future prospects. Appl. Microbiol Biotechnol. 104, 4281–4288. doi: 10.1007/S00253-020-10542-X
Franz, E., Gras, L. M., Dallman, T. (2016). Significance of whole genome sequencing for surveillance, source attribution and microbial risk assessment of foodborne pathogens. Curr. Opin. Food Sci. 8, 74–79. doi: 10.1016/J.COFS.2016.04.004
Gardner, S. N., Slezak, T., Hall, B. G. (2015). kSNP3.0: SNP detection and phylogenetic analysis of genomes without genome alignment or reference genome. Bioinformatics 31, 2877–2878. doi: 10.1093/BIOINFORMATICS/BTV271
Gerner-Smidt, P., Carleton, H., Trees, E. (2017). Role of whole genome sequencing in the public health surveillance of foodborne pathogens. Appl. Genomics Foodborne Pathog. 1–11. doi: 10.1007/978-3-319-43751-4_1
Gilchrist, C. A., Turner, S. D., Riley, M. F., Petri, W. A., Hewlett, E. L. (2015). Whole-genome sequencing in outbreak analysis. Clin. Microbiol Rev. 28, 541–563. doi: 10.1128/CMR.00075-13
Gill, A. (2017). The importance of bacterial culture to food microbiology in the age of genomics. Front. Microbiol 8. doi: 10.3389/FMICB.2017.00777
Grace, D. (2015). Food safety in low and middle income countries. Int. J. Environ. Res. Public Health 12, 10490. doi: 10.3390/IJERPH120910490
Hati, S., Vahora, S., Panchal, J., Patel, S., Patel, A., Chauhan, H., et al. (2024). Whole genome sequencing (WGS) analysis of antimicrobial resistance (AMR) milk and dairy-derived pathogens from Anand, Gujarat, India. Microb Pathog 197, 107076. doi: 10.1016/J.MICPATH.2024.107076
Hawkins, R. D., Hon, G. C., Ren, B. (2010). Next-generation genomics: an integrative approach. Nat. Rev. Genet. 11, 476–486. doi: 10.1038/nrg2795
Hoffmann, V., Moser, C., Saak, A. (2019). Food safety in low and middle-income countries: The evidence through an economic lens. World Dev. 123, 104611. doi: 10.1016/J.WORLDDEV.2019.104611
Holmes, A., Allison, L., Ward, M., Dallman, T. J., Clark, R., Fawkes, A., et al. (2015). Utility of whole-genome sequencing of Escherichia coli o157 for outbreak detection and epidemiological surveillance. J. Clin. Microbiol 53, 3565–3573. doi: 10.1128/JCM.01066-15/SUPPL_FILE/ZJM999094590SO1.PDF
Hovig, E., Gundersen, S., Boddu, S., Capella-Gutierrez, S., Drabløs, F., Fernández, J. M., et al. (2021). Recommendations for the FAIRification of genomic track metadata. F1000Research 10, 268. doi: 10.12688/f1000research.28449.1
Jansen, W., Müller, A., Grabowski, N. T., Kehrenberg, C., Muylkens, B., Al Dahouk, S. (2019). Foodborne diseases do not respect borders: Zoonotic pathogens and antimicrobial resistant bacteria in food products of animal origin illegally imported into the European Union. Veterinary J. 244, 75–82. doi: 10.1016/J.TVJL.2018.12.009
Kirk, M. D., Pires, S. M., Black, R. E., Caipo, M., Crump, J. A., Devleesschauwer, B., et al. (2015). World health organization estimates of the global and regional disease burden of 22 foodborne bacterial, protozoal, and viral diseases 2010: A data synthesis. PloS Med. 12, e1001921. doi: 10.1371/journal.pmed.1001921
Koutsoumanis, K., Allende, A., Alvarez-Ordóñez, A., Bolton, D., Bover-Cid, S., Chemaly, M., et al. (2019). Whole genome sequencing and metagenomics for outbreak investigation, source attribution and risk assessment of food-borne microorganisms. EFSA J. 17, e05898. doi: 10.2903/J.EFSA.2019.5898
Kovac, J., Dudley, E. G., Nawrocki, E. M., Yan, R., Chung, T. (2021). Whole genome sequencing: the impact on foodborne outbreak investigations. Compr. Foodomics, 147–159. doi: 10.1016/B978-0-08-100596-5.22697-8
Kramarz, P., Lopalco, P. L., Huitric, E., Pastore Celentano, L. (2014). Vaccine-preventable diseases: the role of the European Centre for Disease Prevention and Control. Clin. Microbiol. Infection 20, 2–6. doi: 10.1111/1469-0691.12430
Kubota, K. A., Wolfgang, W. J., Baker, D. J., Boxrud, D., Turner, L., Trees, E., et al. (2019). PulseNet and the changing paradigm of laboratory-based surveillance for foodborne diseases. Public Health Rep. 134, 22S–28S. doi: 10.1177/0033354919881650
Lakicevic, B., Jankovic, V., Pietzka, A., Ruppitsch, W. (2023). Wholegenome sequencing as the gold standard approach for control of Listeria monocytogenes in the food chain. J. Food Prot 86, 100003. doi: 10.1016/J.JFP.2022.10.002
Li, W., Cui, Q., Bai, L., Fu, P., Han, H., Liu, J., et al. (2021). Application of whole-genome sequencing in the national molecular tracing network for foodborne disease surveillance in China. Foodborne Pathogens and Disease 18, 538–546. doi: 10.1089/FPD.2020.2908
Ling, X., Wang, C., Li, L., Pan, L., Huang, C., Zhang, C., et al. (2023). Third-generation sequencing for genetic disease. Clinica Chimica Acta 551, 117624. doi: 10.1016/J.CCA.2023.117624
Martinović, T., Andjelković, U., Gajdošik, M.Š, Rešetar, D., Josić, D. (2016). Foodborne pathogens and their toxins. J. Proteomics 147, 226–235. doi: 10.1016/J.JPROT.2016.04.029
Michelacci, V., Asséré, A., Cacciò, S., Cavaiuolo, M., Mooijman, K., Morabito, S., et al. (2023). European Union Reference Laboratories support the National food, feed and veterinary Reference Laboratories with rolling out whole genome sequencing in Europe. Microb Genom 9, 1074. doi: 10.1099/MGEN.0.001074/CITE/REFWORKS
Morton, V., Kandar, R., Kearney, A., Hamel, M., Nadon, C. (2024). Transition to Whole Genome Sequencing Surveillance: The Impact on National Outbreak Detection and Response for Listeria monocytogenes, Salmonella, Shiga Toxin–Producing Escherichia coli, and Shigella Clusters in Canada 2015–2021. Foodborne Pathogens and Disease. 21, 689–697. doi: 10.1089/FPD.2024.0041
Oluwaseun, A. C., Phazang, P., Bhalla Sarin, N., Oluwaseun, A. C., Phazang, P., Bhalla Sarin, N. (2018). “Biosensors: A fast-growing technology for pathogen detection in agriculture and food sector,” in Biosensing Technologies for the Detection of Pathogens - A Prospective Way for Rapid Analysis. Rijeka, Croatia: InTech. doi: 10.5772/INTECHOPEN.74668
Pightling, A. W., Pettengill, J. B., Luo, Y., Baugher, J. D., Rand, H., Strain, E. (2018). Interpreting whole-genome sequence analyses of foodborne bacteria for regulatory applications and outbreak investigations. Front. Microbiol 9. doi: 10.3389/fmicb.2018.01482
Price, V., Ngwira, L. G., Lewis, J. M., Baker, K. S., Peacock, S. J., Jauneikaite, E., et al. (2023). A systematic review of economic evaluations of whole-genome sequencing for the surveillance of bacterial pathogens. Microb Genom 9, 947. doi: 10.1099/MGEN.0.000947/CITE/REFWORKS
Priyanka, B., Patil, R. K., Dwarakanath, S. (2016). A review on detection methods used for foodborne pathogens. Indian J. Med. Res. 144, 327–338. doi: 10.4103/0971-5916.198677
Qian, C., Murphy, S. I., Orsi, R. H., Wiedmann, M. (2023). How can AI help improve food safety? Annu. Rev. Food Sci. Technol. 14, 517–538. doi: 10.1146/ANNUREV-FOOD-060721-013815/CITE/REFWORKS
Quintela, I. A., Vasse, T., Lin, C. S., Wu, V. C. H. (2022). Advances, applications, and limitations of portable and rapid detection technologies for routinely encountered foodborne pathogens. Front. Microbiol 13. doi: 10.3389/FMICB.2022.1054782/BIBTEX
Rantsiou, K., Kathariou, S., Winkler, A., Skandamis, P., Saint-Cyr, M. J., Rouzeau-Szynalski, K., et al. (2018). Next generation microbiological risk assessment: opportunities of whole genome sequencing (WGS) for foodborne pathogen surveillance, source tracking and risk assessment. Int. J. Food Microbiol 287, 3–9. doi: 10.1016/J.IJFOODMICRO.2017.11.007
Samdarshi, S. (2024). “Genome sequencing and bioinformatics,” in Frontiers in Molecular Genetics and Genomics (Golden Leaf Publishers, Nagaland, India), 162–187.
Saravanan, A., Kumar, P. S., Hemavathy, R. V., Jeevanantham, S., Kamalesh, R., Sneha, S., et al. (2020). Methods of detection of food-borne pathogens: a review. Environ. Chem. Lett. 19, 189–207. doi: 10.1007/S10311-020-01072-Z
Scarano, C., Veneruso, I., De Simone, R. R., Di Bonito, G., Secondino, A., D’Argenio, V. (2024). The third-generation sequencing challenge: novel insights for the omic sciences. Biomolecules 14, 568. doi: 10.3390/BIOM14050568
Seelman Federman, S., Jenkins, E., Wilson, C., DeLaGarza, A., Schwensohn, C., Schneider, B., et al. (2024). An investigation of an outbreak of Salmonella Typhimurium infections linked to cantaloupe – United State. Food Control 166, 110733. doi: 10.1016/J.FOODCONT.2024.110733
Siddique, A., Tauqeer, A., Ali, A., Ahsan, A., Iqbal, S., Patel, A., et al. (2024). Whole-genome sequencing of three ciprofloxacin-resistant Salmonella Reading (ST93) strains, an emerging Salmonella serovar in the poultry sector of Pakistan. Microbiol Resour Announc 13, e0000624. doi: 10.1128/MRA.00006-24
Singh, K., Li, S., Jahnke, I., Alarcon, M. L., Mosa, A., Calyam, P. (2024). Improving big data governance in healthcare institutions: user experience research for honest broker based application to access healthcare big data. Behav. Inf. Technol. 43, 1067–1095. doi: 10.1080/0144929X.2023.2196596
Stevens, E. L., Carleton, H. A., Beal, J., Tillman, G. E., Lindsey, R. L., Lauer, A. C., et al. (2022). Use of whole genome sequencing by the federal interagency collaboration for genomics for food and feed safety in the United States. J. Food Prot 85, 755–772. doi: 10.4315/JFP-21-437
Tang, P., Gardy, J. L. (2014). Stopping outbreaks with real-time genomic epidemiology. Genome Med. 6 (104). doi: 10.1186/s13073-014-0104-4
Tibebu, A., Tamrat, H., Bahiru, A. (2024). Review: Impact of food safety on global trade. Vet Med. Sci. 10, e1585. doi: 10.1002/VMS3.1585
Timme, R. E., Strain, E., Baugher, J. D., Davis, S., Gonzalez-Escalona, N., Leon, M. S., et al. (2019). Phylogenomic pipeline validation for foodborne pathogen disease surveillance. J. Clin. Microbiol 57, e01816-18. doi: 10.1128/JCM.01816-18/FORMAT/EPUB
Tolar, B., Joseph, L. A., Schroeder, M. N., Stroika, S., Ribot, E. M., Hise, K. B., et al. (2019). An overview of pulseNet USA databases. Foodborne Pathog Dis. 16, 457–462. doi: 10.1089/FPD.2019.2637
Uelze, L., Grützke, J., Borowiak, M., Hammerl, J. A., Juraschek, K., Deneke, C., et al. (2020). Typing methods based on whole genome sequencing data. One Health Outlook 2, 1–19. doi: 10.1186/S42522-020-0010-1
Vegyari, C., Underwood, A., Kekre, M., Argimon, S., Muddyman, D., Abrudan, M., et al. (2020). Whole-genome sequencing as part of national and international surveillance programmes for antimicrobial resistance: a roadmap. BMJ Glob Health 5 (11), e002244. doi: 10.1136/BMJGH-2019-002244
Walle, V., Guerra, B., Borges, V., André Carriço, J., Cochrane, G., Dallman, T., et al. (2019). EFSA and ECDC technical report on the collection and analysis of whole genome sequencing data from food-borne pathogens and other relevant microorganisms isolated from human, animal, food, feed and food/feed environmental samples in the joint ECDC-EFSA molecular typing database. EFSA Supporting Publications 16, 1337E. doi: 10.2903/SP.EFSA.2019.EN-1337
Webb, J. R., Andersson, P., Sim, E., Zahedi, A., Donald, A., Hoang, T., et al. (2024). Implementing a national programme of pathogen genomics for public health: the Australian Pathogen Genomics Program (AusPathoGen). Lancet Microbe 6 (3), 100969. doi: 10.1016/j.lanmic.2024.100969
WHO. (2025). Estimating the burden of foodborne diseases. Available online at: https://www.who.int/activities/estimating-the-burden-of-foodborne-diseases (Accessed December 6, 2024).
World Health Organization (2018). Whole Genome Sequencing for Foodborne Disease Surveillance: Landscape Paper. (Geneva: World Health Organization). Licence: CC BY-NC-SA 3.0 IGO.
Keywords: outbreak investigation, foodborne pathogens, next-generation sequencing, molecular typing, public health
Citation: Gomes E, Araújo D, Nogueira T, Oliveira R, Silva S, Oliveira LVN, Azevedo NF, Almeida C and Castro J (2025) Advances in whole genome sequencing for foodborne pathogens: implications for clinical infectious disease surveillance and public health. Front. Cell. Infect. Microbiol. 15:1593219. doi: 10.3389/fcimb.2025.1593219
Received: 13 March 2025; Accepted: 04 April 2025;
Published: 28 April 2025.
Edited by:
António Machado, University of the Azores, PortugalReviewed by:
Evann E. Hilt, University of Minnesota Twin Cities, United StatesMuneer Yaqub, The University of Texas at Dallas, United States
Copyright © 2025 Gomes, Araújo, Nogueira, Oliveira, Silva, Oliveira, Azevedo, Almeida and Castro. This is an open-access article distributed under the terms of the Creative Commons Attribution License (CC BY). The use, distribution or reproduction in other forums is permitted, provided the original author(s) and the copyright owner(s) are credited and that the original publication in this journal is cited, in accordance with accepted academic practice. No use, distribution or reproduction is permitted which does not comply with these terms.
*Correspondence: Joana Castro, am9hbmEuY2FzdHJvQGluaWF2LnB0