- 1Centre for Languages and Literature, Lund University, Lund, Sweden
- 2Institute for Empirical Linguistics, Goethe University, Frankfurt, Germany
- 3Lund University Humanities Lab, Lund University, Lund, Sweden
Introduction: The directionality of semantic change is problematic in traditional comparative models of language reconstruction. Compared to, e.g., phonological and morphological change, the directions of meaning change over time are potentially endless and difficult to reconstruct. The current paper attempts to reconstruct the mechanisms of lexical meaning change by a quantitative model. We use a data set of 104 core concepts in 160 Eurasian languages from several families, which are coded for colexification as well as cognacy, including semantic change of lexemes in etymologies. In addition, the various meanings are coded for semantic relation to the core concept, including relations such as metaphor, metonymy, generalization, specialization, holonymy, and meronymy. Further, concepts are coded into classes and semantic properties, including factors such as animacy, count/mass, concrete/abstract, or cultural connotations, such as taboo/non-taboo.
Methodology: We use a phylogenetic comparative model to reconstruct the probability of presence at hidden nodes of different colexifying meanings inside etymological trees. We find that these reconstructions come close to meaning reconstructions based on the comparative method. By means of the phylogenetic reconstructions, we measure the evolutionary dynamics of meaning loss of co-lexifying meanings as well as concepts.
Results and discussion: These change rates are highly varying, from almost complete stability to complete unstability. Change rates vary between different semantic classes, where for instance wild animals have low change rates and domestic animals and implements have high change rates. We find a negative correlation between taboo animals and change rate, i.e., taboo animals have lower change rates than non-taboo words. Further, we find a negative correlation between animacy and change rate, indicating that animate nouns have lower change rate than inanimate nouns. A further result is a negative correlation between change rate and degree of borrowing (borrowability) of concepts, indicating that lexemes that are more likely to be borrowed are less likely to change semantically. Among semantic relations, we find that metonomy is more frequent than any other change, including metaphor, and that a change from general to more specific is in all cases more frequent than the other way round.
1. Introduction
1.1. The enigmatic domain of semantic evolution and change
The area of semantic change is a problem child in historical linguistics: compared to other domains, such as phonological and morphological change, it cannot be easily reconstructed and predicted (Urban, 2014). Over generations of speakers, the possible directions of changes of word meanings are potentially endless. Semantic change typically depends on unpredictable socio-cultural and historical changes in speech communities. Other aspects of change may be related to speakers' cognitive and communicative preferences (Meillet, 1912; Ullmann, 1962; Sweetser, 1991). Potentially endless change directions in combination with unpredictable causes for change has led several scholars to point out semantics as an area in which it is difficult—if not impossible—to establish general trends (Saussure, 1916; Jespersen, 1946; Ullmann, 1962, 1966; Anttila, 1989). This problem of defining and predicting semantic change spills over on the discipline of etymology. In etymological research, a sound methodology of reconstruction is necessary to gauge the reliability of an etymological proposal (Hoffman and Tichy, 1980). But what is a sound methodology? How can semantic changes of word meanings be assessed as more or less likely? The known linguistic history is full of examples of unpredictable changes of word meanings. However, can these be inferred to past linguistic history to assume a specific tendency or direction of change? The issue is also a problem when the comparative method is applied to infer the meaning of lexemes at reconstructed states (Malkiel, 1993; Mailhammer, 2014). The meaning of reconstructed proto-words can be specific, but they often come out as very vague (Watkins, 2000: 245–53; Durkin, 2009). This vagueness of reconstructed meanings is a problem in paleo-linguistic research, where reconstructed lexical meaning is used as a basis for connecting proto-languages to archaeological cultures (Benveniste and Lallot, 1969; Anttila, 1989; Heggarty, 2014).
Despite these obstacles, relatively much can be said about change of lexical meaning, based on an array of different methodologies (Newman, 2016). A useful and reliable method is to observe changes between language states in attested data, something that can be mechanized and done on a mass basis (Zalizniak et al., 2012). Other methods include experiments dealing with the cognitive processes of meaning change, corpus-based methods including hierarchical organization of meaning, as well as cross-linguistic lexical typology to infer patterns of semantic structuring. All these methods, individually or combined, may form a basis for assessing patterns and gauge the directionality of semantic evolution (Ullmann, 1962; Sweetser, 1991; McMahon, 1994: 174–99; Traugott and Dasher, 2002). A fundamental prerequisite for assessing semantic change, used in experiments, historical observations, corpus-based studies, and semantic typology, is the notion of polysemy. Polysemy is a synchronic variation where a lexical morpheme may refer to more than one particular meaning. This leads to semantic vagueness and a separation of meanings in one word, which is one of the initial phases of semantic change (Murphy, 2010, p. 83–47). The polysemous variation in a lexeme is not random, but rather caused by communicative and socio-cultural usages of lexemes, which make culture and cognition central aspects of semantic evolution and change (Durkin, 2009). Polysemous variation in a synchronic state can be visualized and analyzed as a semantic network organizing meanings according to various parameters, including proto-typicality, semantic nearness, and hierarchical relation (Lakoff, 1987; Geeraerts, 2010, p. 182–272; Murphy, 2010, p. 101–05). The nature of relation between various polysemous meanings, such as synonymy, antonymy, hyponomy, metaphor, and metonymy form the basis for change of lexical meaning in diachrony (Sweetser, 1991).
The concept of colexification is used when semantic networks or maps form the basis for a typological contrast, where cross-linguistic patterns can be observed (Haspelmath, 2003; François, 2008). Colexification patterns are a powerful source for assessing semantic connections on a larger scale and to infer connotations about fundamental cognitive and cultural patterns. In recent years, large-scale and empirical studies on colexification have been made possible through open databases and database conglomerates, such as CLICS (List, 2018; Rzymski et al., 2020). Recent studies have delved into global and family-related patterns of the semantic domain of emotions (Jackson et al., 2019) or universal and macro-areal patterns of perception and cognition (Georgakopoulos et al., 2021). A further topic is the connection between colexification and cognitive economy or word frequency: studies indicate that higher frequency implies higher probability to colexify (Kuiper et al., 2018), a hypothesis suggested already by Zipf (Zipf, 1949). Another application is cognitive, where studies show that global colexification trends connect to conceptual similarity of meanings preferred by speakers in cognitive experiments (Xu et al., 2020; Karjus et al., 2021).1
1.2. Outline of the current study
Our study reconstructs semantics and observes directionality of semantic change by means of a phylogenetic comparative model (Jäger, 2019; Carling et al., 2021). As a basis, we use a data set of 104 core concepts of culture vocabulary in 160 Eurasian languages of seven families, including Indo-European, Kartvelian, Northwest Caucasian, Dagestanian, Uralic, Turkic, and Semitic (some of these families are later excluded due to too few data points, see below). The data is openly available in the database DiACL (Carling, 2017) and has previously been published in the volume Mouton Atlas of Languages and Cultures (Carling, 2019). The data set includes lexemes that have been coded for cognacy as well as lexical polysemy. The total number of lexemes of the data set is 16,679. For the phylogenetic comparative model applied in this paper, we use lexemes that are coded by etymology, removing loans, which reduces the number of lexemes to 13,060. The data in the original data set from the Semitic family was not coded by etymology and is therefore not used in this paper, reducing the number of families to six.
We have selected the data partly because of its status (presence of etymology and polysemy), partly because of its cross-linguistic nature (involving several families). Due to its status of being restricted to one continent (Eurasia), we do not aim to establish any universal tendencies by our results. Nevertheless, we believe that the data is rich enough to observe general tendencies in lexical semantic evolution. The purpose of our study is three-fold. First, we wish to assess the extent to which it is possible to reconstruct meaning to unattested proto-language states by using a phylogenetic comparative model. Moreover, we aim to study the evolutionary dynamics of various meanings from the perspective of semantic relations between them. This would enable us to gauge whether some semantic relations occur more frequently, something that would add new knowledge to the topic of semantic directionality. Finally, we aim to study the evolutionary dynamics of different concepts, to figure out whether there are any inherent differences between concepts based on their meaning. This includes also in relation to their semantic classification as well as possible cultural connotations, which may affect their change rate. A data overview is given in Table 1. For a more careful description of data cleaning and coding, see Section 2.3.
2. Theory, model, data, method, and analysis
2.1. Preconditions for semantic evolution: a structuralist model of defining semantic relations
Our study aims to apply a quantitative model to semantic evolution, using a relatively large set of lexical data. This aim requires a theoretical framework that facilitates a structuring and coding of data enabling an inference corresponding to the posed research questions. For that purpose, we apply a structuralist model of lexical fields, in which lexemes of a language can have several co-occurring (i.e., polysemous) meanings (Geeraerts, 2010, p. 47–100). We assume that these meanings are in a syntagmatic relation, which can be associated to each other. These associations can be visualized as semantic fields or networks in a synchronic state, both for individual lexemes as well as for semantically related lexemes (Murphy, 2010, p. 125–32). Semantic fields and networks may overlap between lexemes, ultimately leading to a situation where most lexemes in a vocabulary can be connected to each other by their different meanings. Despite this complexity, a lexeme in a synchronic state typically has a core or prototype meaning, to which other meanings may have a relation. The semantic variability in a synchronic state, as outlined in the structuralist model, form the basis for semantic change (Geeraerts, 1997). Likewise, the typology of colexification in a larger perspective may form the basis for assessing semantic evolution and drift (François, 2008; Newman, 2016). Synchronic polysemous meanings—as well as colexified meanings cross-linguistically—can be defined by their inherent relation to each other. With a structuralist semantic model these lexical semantic relations (Murphy, 2010) are thesaurus-like references (Melčuk, 1996; Fellbaum, 1998), which can be used to observe dynamics of meanings and meaning relations in an evolutionary model. In this model, the prototype or core meaning of lexemes plays a central role. Core meanings are used as a quantitative point of reference, against which peripheral meanings can be contrasted synchronically, diachronically, and in evolution (François, 2008). Core and peripheral meanings in a synchronic state thus form a theoretical point of reference for an evolutionary inference, using a computational phylogenetic model.
As a point of reference, we use a basic matrix model for reconstructing meaning as well as semantic change, which is similar to the model outlined by Campbell (Campbell, 2013: 232–38). In an initial hypothetical state, we assume that a lexeme has just one (core) meaning. By time, this meaning expands by polysemy, and in a hypothetical second state, the lexeme carries two polysemous meanings, of which one is the core meaning. By meaning change, the initial core meaning may become lost, and in a third hypothetical state, the lexeme has only the second (originally polysemous) meaning. The third state implies that semantic change or shift has occurred (Figure 1). This transition between states is a hypothetical and simplistic paradigm and should not be seen as an established evolutionary trajectory: languages may, at any time, be in any transition between any of these states for any meaning and any lexeme.
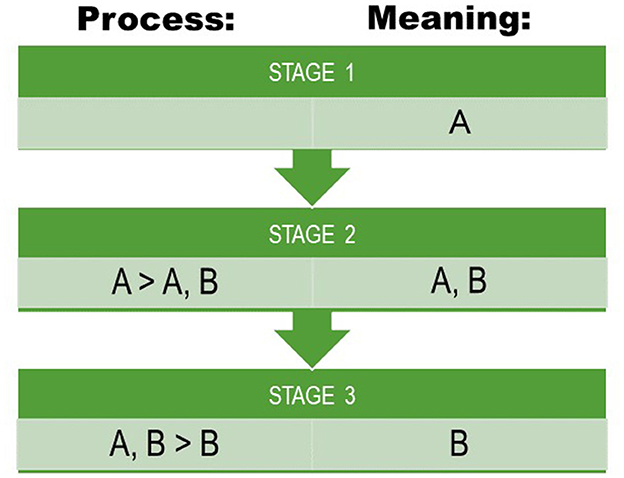
Figure 1. A schematic model for quantifying semantic evolution used in this paper. Meaning refers to the attested meaning(s) of lexemes in our data. Process refers to a hypothesized process at hidden stages giving rise to the attested meanings at attested stages. Outlined after (Campbell, 2013, p. 232–238).
In an evolutionary reconstruction model, this matrix is schematically reorganized (Figure 2). We have access to observed feature data, consisting of polysemous meaning variants of lexemes in synchronic states. The total number of meanings of lexemes in languages are potentially endless, but given the restrictions provided by the phylogenetic etymological tree (see below for the rules applied for inclusion of lexemes in etymological trees), the meaning variables in our data can be seen as categorical variables. By means of a phylogenetic comparative model, we follow a procedure where we infer a model where an etymon can retain, gain or lose meanings with a certain probability at some time interval. By this model, we can estimate meaning probabilities for unobserved internal nodes that are most likely to have preceded the values displayed by (or inferred for) their descendants, as well as to extract the gain and loss rates for meanings. This model is described in greater detail below (Section 2.4).
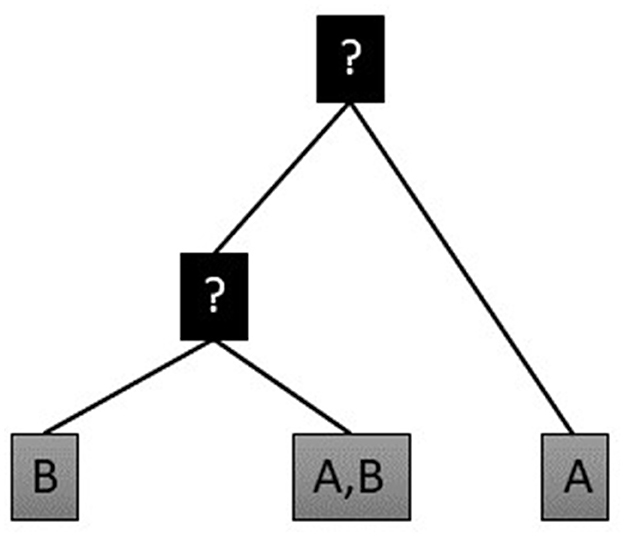
Figure 2. Toy model for reconstructing the probability of presence of meanings at hidden nodes in a phylogenetic tree, drafted after (Carling and Cathcart, 2021b).
2.2. Research questions
Our initial research question is whether we can reconstruct the probability of different meanings at hidden nodes, using a phylogenetic comparative model. Here, we are interested in whether a phylogenetic model of semantic reconstruction can be used in a similar fashion as a model for reconstructing grammar (Carling and Cathcart, 2021a). Moreover, we are interested in whether a phylogenetic comparative model for reconstructing meaning can complement or improve a traditional model of reconstruction, using the historical-comparative method (cf. the overview in Section 1.1).
In addition to that, we have several questions concerning the evolutionary dynamics of meanings, which can be retrieved from the phylogenetic comparative reconstruction.
First, we want to know whether there is variation in the evolutionary dynamics of different meanings. If this is the case, we are interested in whether these differences can be explained by any of the factors suggested in the literature to impact semantic change. We are interested in whether the factors may be cultural (e.g., taboo, related to cultural practices) or whether they refer to cognitive associations, such as prototypicality (Lakoff, 1987) or primeordiality (Goddard, 2008). We are interested in these factors not only from the perspective of individual lexical meanings or core concept meanings, but also from the perspective of semantic classes. Further, we are interested in whether there is any connection between rates of semantic evolution and effects of frequency (Pagel et al., 2007). Unfortunately, our data is reduced to culture words, and basic vocabulary would have been more appropriate to respond to these research questions. However, basic vocabulary data coded in an appropriate way has not been available to us.
Second, we want to know whether changes referring to specific semantic relations are more frequent than others. It has been suggested that increasing subjectification or rise of epistemic meanings is a more frequent type of change (Traugott, 1989), and that bidirectional metaphors are more frequent than unidirectional (Sweetser, 1991). However, we aim to expand the study of directionality of semantic relations to a higher number of possible relations, such as metonomy, metaphor, generalization or specialization (see Section 2.3).
2.3. Data: original data, recoding, and additional coding for the current study
The data set used is retrieved from five different data sets of “Culture words” from the lexical section of the DiACL database (Carling, 2017): Culture words for Indo-European, Caucasus, Turkic, Uralic, and Old Middle-Eastern non-Indo-European. All data has been published in the Mouton Atlas of Languages and Cultures (Carling, 2019) and is openly available, both in the database DiACL as well as in an online repository at Zenodo,2 which harbors the version of the data published in the atlas (Carling, 2019; Appendix 3b). The data has been recoded and prepared for the current publication. Both the data structure and the recoding will be described below.
The data consists of lexeme lists in languages, which have been compiled using a concept list (Poornima and Good, 2010; List et al., 2016; Dellert and Buch, 2018). In this specific case, the lists constitute of culture terms reflecting cultural objects and activities of high age and importance, which have been in daily use at least since the Chalcolithic 7,000–5000 years BP (Carling, 2016, 2019; Carling et al., 2019a,b).
2.3.1. Coding of cognacy
The data is coded for cognacy. The model used in the data differs slightly from cognacy coding in lexicostatistical models. First, the data allows for polymorphic coding, i.e., more than one lexeme per concept is allowed in individual languages. This coding type occurs in lexicostatistics, but there is an ongoing discussion on how it impacts chronological calculations, which means that it is not preferred (Dunn, 2014; Chang et al., 2015). Another difference of our data compared to a lexicostatistical data set is that cognacy is expanded beyond lexemes that have retained the concept meaning diachronically, to include lexemes of synchronic variation as well as completely changed meaning diachronically. This means that the cognacy coding, which we label etymological coding (and use the term etymon for cognates), is built around trees, including lexemes of various degrees of deviating semantic meaning (Carling, 2019, p. 179–89). According to the policy of the data (Carling, 2019, p. 181–82), lexemes are kept in etyma if they:
• Include derivations that do not alter the concept meaning,
• Include semantic change as long as the basic derivation (root+morpheme) is kept,
• Include also changes of word class in semantic change.
We are aware that the decision to include etymologies of changed meaning may give rise to inconsistencies and impact the results. However, we also believe that including this coding from the original data may give a more interesting result on semantic evolution. The problem and its potential impact on phylogeny, in particular tree structures and time-depth calculations, is discussed at length in the paper by Chang et al. (2015).
2.3.2. Standardization of polysemous word meanings
The original data set was manually coded and retrieved from various sources, mostly dictionaries, but also fieldwork. This resulted in an uneven status of the data, where some languages and lexemes had more detail in their semantic information, whereas other languages were less informative. An important aim for the current project was to standardize the various polysemous meanings given by different lexemes, as well as to standardize these meanings to avoid unnecessary redundancy in the data. Compared to the original meanings extracted from dictionaries and fieldwork of the database, the data of the Mouton atlas contained a substantial standardization of dictionary meanings (Carling, 2019). However, this standardization was not sufficient for the current project, and therefore, a further standardization and simplification was necessary. The first step was to harmonize all redundant forms given for various meanings, such as “round fleshy fruit of the species Malus malus” for “apple,” or “female deer,” “female of the species Capreolus capreolus” for “doe.” Other examples of redundancy included very close synonyms, such as various sub-species of animals or explanations using different terms with almost similar meaning. The standardization process was done systematically by searching the meaning fields of the data. Redundant explanations were removed, very close synonyms were conflated, and references were standardized (e.g., “young animal”, “young of animal”, “young of the animal”). In the process, external databases such as CLICS were consulted, but the process was mainly done by internal leveling of redundancy. After the process was completed, the data consisted of 21,874 meaning tokens and 6,224 meaning types, distributed as polysemous meanings of 16,679 lexemes, compiled from the original list of 104 concepts (Table 1). This data formed the basis for the coding of semantic relations, described in next paragraph.
2.3.3. Coding of semantic relations
The literature lists a number of semantic relation types, which form the basis for semantic change or shift (Lyons, 1963; McMahon, 1994; Geeraerts, 1997; Durkin, 2009; Murphy, 2010; Newman, 2016). For our coding, we wanted to include as many relations as possible, meanwhile keeping a system that was representative as well as possible to handle by the phylogenetic comparative model. We established a matrix of semantic relations, for which we defined coding motivations to work with when defining relations for the 6,224 meaning types. The meaning relations were coded between the concept meaning (e.g., APPLE, WOLF, GOLD) and each polysemous meaning for every lexeme individually (defined by their Lexeme ID) (Supplementary Table S1). In cases where the meaning was completely changed, we used the dominant concept meaning of the etymon as the basis for contrast (see above under Section 2.3.1.).
We used both linguistic and database-related sources for establishing a semantic relations matrix. For establishing our main types, we used comparative linguistic observations of frequent semantic relations occurring in semantic change, based on the sources (Bloomfield, 1933; Hock, 1991; McMahon, 1994; Campbell, 2013). Further, we used the WordNet corpus (version 3.1) to define and motivate the coding (Fellbaum, 1998). We distinguished seven semantic relation types (which were arbitrarily numerated): (0) no change/synonym, (1) metaphor, (2) distant-metonomy, (3) generalization, (4) specialization, (5) holonomy, (6) meronymy, and (7) near-metonymy. These relations were divided into two main groups, defined as whether the semantic relation was kept within the extended semantic domain (3–7), or outside of the semantic domain (1–2) of the concept meaning (Table 2, see further description below).
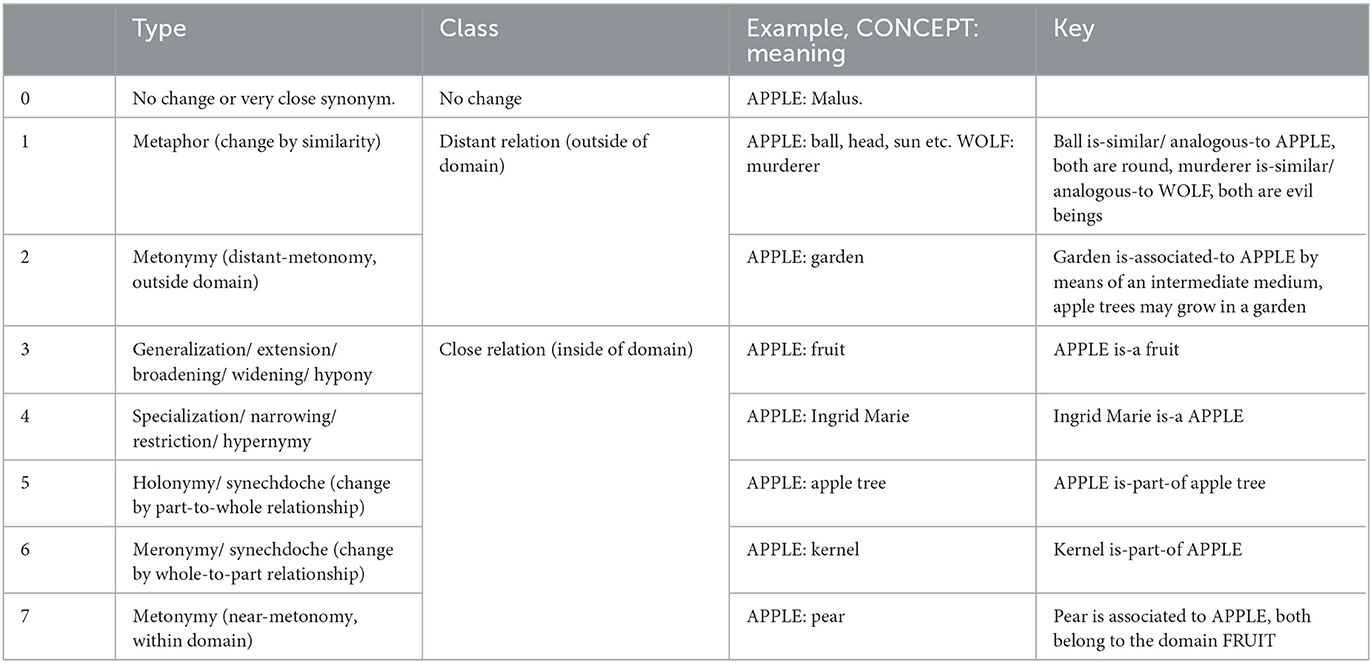
Table 2. Matrix of semantic relations coded in the data, including type, class, example and key used for coders.
Besides synonymy, which we made equal to no change (0), we followed the WordNet design and search functions (Tengi, 1998), for establishing four hierarchical relations:
• Generalization (extension/ broadening/ widening/ hyponymy) (3) was defined by an is-a or is-a-kind-of-relation. Examples from the data include, e.g., APPLE : fruit, BARLEY : grain, BISON : cattle, CATTLE : animal, LEOPARD : large cat, WOOD : material.
• Specialization (narrowing/ restriction/ hypernymy) (4) was defined by an is-a or is-a-kind-of relation, but with the order of concept and meaning reversed, compared to generalization (3). Examples from the data include, e.g., female wild boar : WILD BOAR, she-wolf : WOLF, bacon : MEAT, wooden plow : PLOW, mill's wheel : WHEEL.
• Holonymy (synechdoche) (5) was defined by a is-part-of-a-relation. Examples from the data include, e.g., APPLE : apple tree, AUTUMN : year, AXLE : wagon, HORSE : flock of horses, MEAT : body.
• Meronymy (synechdoche) (6) was defined by a is-part-of-a-relation, but with the order of concept and meaning reversed. Examples include FLAX : fiber of flax, KID : meat of kid, SUMMER : June, WHEAT : husk, TURNIP : root.
However, we did not follow the WordNet design in coding antonymy. Even though antonymy contributes to semantic change (Traugott, 2017), antonymy is relatively rare in polysemy and semantic shift (Campbell, 2013, p. 221–46). The reason for excluding antonymy was simple: we found no examples of antonymy in polysemous meanings while coding the data.
Besides these WordNet-based relations, we included metaphor and metonomy. Of these two, metaphor was easier to define, in particular since there is a rich literature on metaphor and its role in semantic shift (Lakoff, 1987: 203–13; Sweetser, 1991; Traugott and Dasher, 2002; Geeraerts, 2010). Our definition of metaphor focused on two main relational characteristics, similarity and analogy, for which we used the following formula (these two types were not differentiated in the coding):
• Similarity: “meaning 1 is similar to 2 (both are x in their appearance)”
• Analogy: “meaning 1 is similar to meaning 2 (both can be characterized as x)”.
There are numerous examples from the data of both these types, e.g., WHEEL : football (both are round), SWORD : tooth (both are sharp), SUMMER : fire (both are hot), RYE : hundred (both are numerous), HUB : compass (both are round), LION : giant (both are large). Metaphor was often coded for cases of word class shift, e.g., WHEEL : easy (both run easily). We also coded relations between agent, action, and object (with or without further metaphorical connections) as metaphor (Geeraerts, 2010, p. 210–13). This often pertained to different word classes, e.g., TO SPIN : sinew, WOLF : to strangle.
The literature on metonomy (Panther and Radden, 1999; Geeraerts, 2010, p. 213–22; Littlemore, 2015) regards this relation as closely related to or a specific type of metaphor. Different from metaphor, the literature defines metonomy by association or contiguity rather than similarity. A standard definition invokes the number of domains involved in the conceptualization process (Geeraerts, 2010, p. 215f): metaphors involve two domains, metonymies only one (Lakoff, 1987). Initially, we decided to follow a basic distinction and define metonymy by a formula “meaning 1 is associated to meaning 2, by means of the common denominator/process/connection x”. However, once the first round of coding was done using this definition, it turned out that metonomy was, compared to the other semantic relations, both much more frequent and much more heterogeneous. Metonymy relations spanned over a large number of substantially different meaning relations. We decided to implement a coding system that distinguished two types, near-metonomy and distant-metonymy. To do so, we implemented a notion of domain as a central concept in the distinction of metonomy (Handl, 2011, p. 86–97; Littlemore, 2015, p. 53–59). We defined two relations, labeled “near-metonomy” and “distant-metonomy,” based on the differentiation whether the semantic relation involved a part-for-whole/whole-for-part relation or association within a defined domain (Figure 3A). Examples for near-metonomy from the data include CATTLE : foal, COPPER : bronze, DEER : cow, FAT : butter, FUR : skin, SAW : ax, SICKLE : wood, SPADE : ditch. In a case where the relation was mediated by an intermediate associative medium, the relation was coded as “distant-metonomy” (Figure 3B). Examples for distant-metonomy include ARMY: nation, AUTUMN : fever, AX : plowshare, BEE : honey, CHICKEN : year.
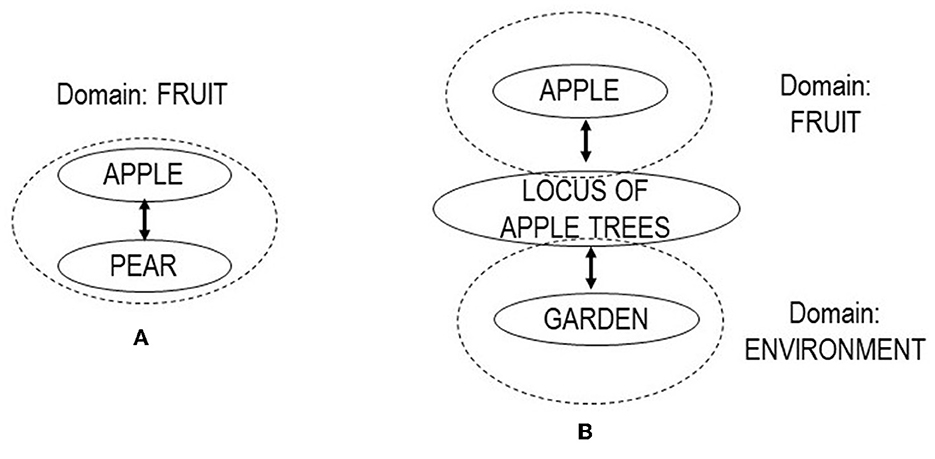
Figure 3. Schematic overview of near-metonomy (A) and distant-metonomy (B). In near-metonomy, the relation is based on contiguity or association within a defined semantic domain. In distant-metonomy, the relation is based on an intermediate associated medium (after Handl, 2011, p. 84–89).
2.3.4. Classification of concepts
To understand results and test some correlations, we grouped our list of concepts into classes. Before testing the change rates of al 6,224 meanings of our data, we classified the original 104 core concept meanings. The concept list targets culture terms, including concepts that are considered to have been in daily use in the Eurasian context since the Chalcolithic period. For our classification of concepts into semantic subgroups, we decided to reuse the classification of the original publication of the data (Carling, 2019). This groups concepts into classes according to their cultural usage and how they cluster by colexification. This classification of all core concepts is given in Table 4. In a way, it is circular to use a classification partly based on colexification for testing change rates retrieved from patterns of colexification. However, there are large discrepancies between the classes, which we believe depend on semantic properties, and the classification is clearly contributing to our understanding of these patterns, as we will see Section 3.
2.3.5. Coding of semantic properties of concepts
As we have described above, we are also interested in various factors that may affect semantic change rates, either cultural factors (e.g., taboo, cultural practices) or cognitive associations, such as prototypicality (Lakoff, 1987) or primeordiality (Goddard, 2008). To test this, we decided to code our 104 concepts according to several pre-defined semantic properties, which were of both cognitive and cultural nature. The selection of properties was partly restricted by the predefined concept list that we had at our disposal. First, we distinguished parts of speech for all concepts: nouns, verb, and adjectives. For instance, the concept list did not contain basic vocabulary or concepts referring to human beings of different sexes. Following a semantic model of basic entity properties, we coded the following distinctions (Frawley, 1992, p. 62–139): animacy/inanimacy, count/mass, concrete/abstract, as well as sharp/blunt and oblong/round. For cultural connotations and practices, we decided to code a number of different variables, which pertained to subsistence, farming practices, etc. Following the classifications and descriptions of the concepts in the original Mouton atlas (Carling, 2019), which were based on several classification codes of cultural and ethnographic studies (Lomax et al., 1977; Barker, 2006; Murdock, 2008; Kirby et al., 2016), we coded several inherent properties of concepts, e.g., culture/nature, large farming/small farming, domestication/non-domestication, industrial manufacturing (Y/N), slaughtering or vehement preparation (Y/N), light preparation (Y/N), food substance (Y/N), persistent/non-persistent, taboo/non-taboo. We will not go into further detail about the cultural property classifications here, since they (with one interesting exception) showed no significant correlation to the semantic change rates, as will be further described below. A further factor was to test causality of borrowing patterns in the data, for which we used borrowability metrics from our own data as well as from WOLD (Haspelmath and Tadmor, 2009b).
2.4. Reconstruction by a phylogenetic comparative model
The phylogenetic comparative inference (cf. also the description in Carling, 2019, p. 201) adapts a model of meaning change, which assumes that an etymon, including all lexemes present in an etymological tree, can gain or lose a given meaning at some time interval with a certain probability. We chose a single optimized probability value over a probability distribution. The applied model is based on the notion of Markov process of gain and loss probabilities, and has previously been used for typological data (Maslova, 2000). The inference uses Glottolog trees for all families (Hammarström et al., 2021), including polytomies (Supplementary Table S9, plus online material3). The choice of time interval is between a proto-language and its daughter, including language subgroupings (not using an actual time depth). These choices (optimized probability and exclusion of time intervals) were motivated by simplicity, given the complexity of the input data. The model estimates the gain/loss of each lexical meaning independently. For a meaning M, the model estimates two probabilities: (1) that of gaining the meaning M when absent, (2) that of losing the meaning M when present.
All different lexical meanings in the etyma allow an estimation of these probabilities at hidden nodes and roots of etymological trees. The dataset contains precursors (i.e., earlier states of languages), indicating that we sometimes may record an original meaning change of a lexeme in an etymon. However, the probability that an unknown node had a meaning M in an etymon is estimated from the proportion of attested languages with the meaning M. The probability of losing M is reflected in the number of changes to other meanings than M, where the expected original meaning was M, relative to the number of retentions of the meaning M.
Once the gain and loss probabilities are known, the probability of a certain meaning at hidden nodes and the root is calculated from meanings of the leaf nodes using the peeling algorithm (Felsenstein, 2004, p. 253–54). The model excluded loans and runs the model for all 1,165 etymological trees in the dataset. The resulting trees have probabilities of presence of all meanings at hidden nodes of the trees. The original data contained a coding of the semantic relation between the concept meaning and the colexified meanings of lexemes in etyma (see Section 2.3). The model has the problem that it cannot distinguish precisely which meaning diachronically changed into which meaning inside the etymological tree. Therefore, the model estimates the change type at branches between nodes based on the meanings of the attested languages. The probability is calculated individually for each lexeme and each meaning in the data. This problem is also valid for the reconstruction of the change types (semantic relations, Section 2.3), which were coded between the concept meaning in relation to different meanings of a lexeme. Therefore, the probability to change was computed based on each meaning and each lexeme individually. Since the model cannot reconstruct which meaning turned into which meaning inside an etymon, the occurrence of semantic relations is an estimation based on the assumption that the concept meaning is primordial also in earlier states of the etymological tree. Any other meaning relation cannot be estimated by the model. However, despite this shortcoming of the model, we believe that it will be possible to estimate the relative frequency of different change types pertaining to meaning relations by means of this model. The outcome of our study and the results will be further described below under Section 3.
3. Results
3.1. Reconstruction probabilities at hidden nodes
The result of phylogenetic comparative model, described in Section 2.4, consists first of reconstructed probabilities of presence (ranging from 0 to 1) of all lexemes at hidden nodes of all 1,165 etyma in our data (Supplementary Table S2). There are 70,750 reconstructed meaning probabilities (ranging from 0 to 1) at 86 ancestral nodes (Supplementary Table S3) inside etymological trees. The computation made use of Glottolog trees, and therefore the naming of ancestral nodes follows the Glottolog standard. The folder (Supplementary Table S9) gives all reconstructed etymological trees, including probabilities for each meaning at the root and at attested stages (but not at intermediate nodes, this information is given in Supplementary Table S2). Meanings with a probability larger than 0.75 are marked by green in the reconstruction (Supplementary Table S9).
Looking at the result, we notice that the reconstruction at the most ancestral hidden node (i.e., the root) reflects all meanings given in languages included in an etymon. However, only one or two meanings typically reaches over a threshold of a probability of 75%. Our first research question targets how well these reconstructions match with reconstructions by the comparative method.
As an example to begin with, we may consider two frequently discussed etyma in Indo-European linguistics, the two lexical roots for “wheel” (Carling, 2019, p. 345–54; Heggarty, 2014) (Table 3). Like in many of our reconstructions, most meanings end up at an intermediate probability (around 0.5). This is likely an artifact of the method of reconstruction, such as the model's failure to resolve polytomies and a minimization strategy favoring parsimony. This results in a model where a single language carries as much weight as all other taxa, and the choice of another model, such as a Bayesian MCMC model, could have improved the outcome.
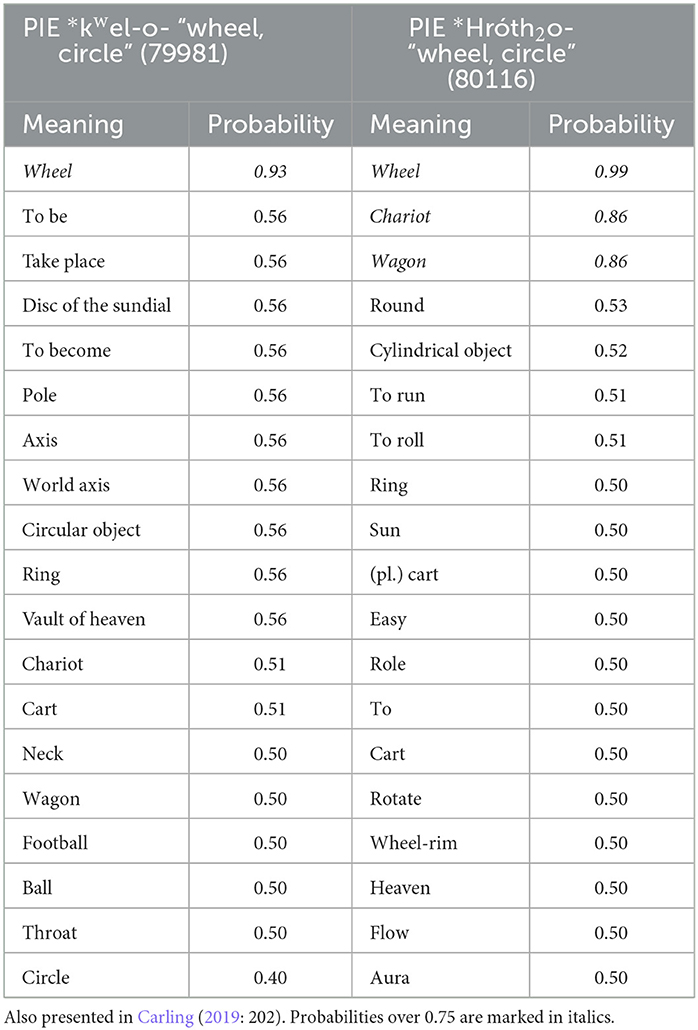
Table 3. Meaning probabilities at the ancestral node of the Proto-Indo-European language for the etyma PIE *kwel-o- “wheel, circle” (Cognate ID 79981) and PIE *Hróth2o- “wheel, circle”(Cognate ID 80116).
The examples given in Table 3 present results for the reconstruction of two roots that are relatively straightforward. Not all reconstructions are as evident as these. We may take a few other samples of more complex etymologies to see how well the method works in contrast to reconstruction by the comparative method. If we look at the Proto-Indo-European lexical root *gwolbho- “uterus” (Mayrhofer, 1986, p. 6474) (Cognate ID 55264) the most likely meaning rendered to the proto-language is “womb” (0.57), even though a substantial part of the lexemes of the etymon are from Germanic languages with the meaning “calf” (which is also the concept meaning for this etymon). In this case, the model suggests a “correct” meaning, even though the certainty is relatively low.
In the case of Middle English Garran, English garron, Scottish Gaelic gearran and Spanish garañón (Klein, 1966, p. 641), an etymon restricted to Romance, Celtic and Germanic, the model reconstruct the meaning “horse (pejorative)” as most likely (Cognate ID 56748), which is an interesting result, given that this is a Western Indo-European root for “horse,” existing besides other, more well-established roots for “horse.” It is also possible that the word is a migration term.
In many cases, the model is not capable of giving any clear result. An example is the Germanic etymon Proto-Germanic *stoda- n. “flock” (Cognate ID 56683), with the meaning “flock,” “flock of horses” “horse,” “stud,” “mare” etc. distributed in Germanic languages, and parallels in Balto-Slavic, with the meaning “herd, flock,” and “flock of horses” e.g., Old Church Slavonic stado “herd, flock” (Kroonen, 2013). All occurring meanings in the reconstruction come up with a probability around 0.5.
Etymology is a tricky and complex issue, and the results of our reconstruction will not solve this problem—the probability results will not replace or even improve the reconstructions by the comparative method. For instance, there are many examples in which the interpretation of the data coding may have affected the result. This is particular the case in which a specific stance has been taken on whether a word has been borrowed at an early state alternatively is an early migration word. An example is the word for “donkey” (Cognate ID 56767), Gothic asilus, Old English esol, eosol, Italian asino, Scottish Gaelic asal, Russian osël etc. The conglomerate is often considered to be an early migration word (Kluge, 2002, p. 174–75), which is coded as cognate in our model (Carling, 2019, p. 185–86). The model comes up with a high probability of the meanings “donkey” (1.0), “wild ass” (0.77), and “onager” (0.77) at the root (here defined as “Indo-European,” but rather a Western Indo-European proto-language). The result would have been different if another policy for rendering loans and migration words had been used in the data.
Therefore, we are not fully capable of giving a complete and adequate answer to our initial research question (see Section 2.2), whether a comparative phylogenetic method can be used to reconstruct meaning at hidden nodes. The current paper cannot come up with a solution to quantify the results, as has been done in (e.g., Carling and Cathcart, 2021a,b) for grammatical data. In evident cases, where the meaning has been preserved in most branches of the tree (e.g., PIE *kwel-o- and PIE *Hróth2o- “wheel” above), the phylogenetic comparative model confirms a reconstruction by the comparative method in giving the most preserved meaning as the most likely original one. In cases where an earlier branch has preserved a more archaic meaning, which then has changed in other branches (cf. *gwolbho- “uterus” above), the model still gives the archaic meaning as more likely, but is hesitant about the result. In complex cases with many meaning options distributed over the tree, the model cannot come up with a result (Proto-Germanic *stoda- “flock” above). However, a screening and evaluation of the 1,113 etymologies (Supplementary Table S9) of our data makes us confident about the fact that the model can be used a basis for assessing semantic evolutionary trends, as outlined in the research questions under Section 2.2.
3.2. Meaning gain and loss
Next, the model is used as a basis to infer meaning gain and loss (see Section 2.4) to assess the evolutionary dynamics of different meanings, concepts and semantic classes. The result (Supplementary Table S4) gives the transition probabilities of meanings of lexeme IDs, using attested languages and including results from transitions between states of hidden nodes (Supplementary Table S3), involving all families and excluding loans. The result distinguishes four types of probability of change:
• P(0|0) = The probability (of a lexeme) not to have a meaning after not having a meaning
• P(1|0) = The probability (of a lexeme) to gain meaning after not having meaning
• P(0|1) = The probability (of a lexeme) to lose meaning after having meaning
• P(1|1) = The probability (of a lexeme) to keep meaning after having meaning
Four additional columns in the file (Supplementary Table S4), #(0|0), #(1|0), #(0|1), #(1|1), give the number of transitions used to compute the probability for each meaning of a lexeme. Most meanings in the result set have very few data points underlying the computed probability, and therefore, these numbers are of importance to clean the results. The file contains the result for all 6,224 meaning types in the data. If all lexeme IDs with the same meaning are converged by the weighted arithmetic mean (proportional to the number of transitions), the resulting number of meanings is 3,442, given in the file (Supplementary Table S5). In this study, we are mainly interested in rates of change, which we equal to loss rates, i.e., the probability to lose a meaning after having the meaning P(0|1). The information about keeping meaning is coded by the attested semantic relation (0) as well as in the probability P(0|0).
However, a plot demonstrating the distribution of change rates for all meanings (Supplementary Figure S6) indicates a large variation by meanings for which the reconstruction is based on fewer than 15 datapoints. After removing meanings with fewer than 15 datapoints, 262 meanings remain. These meanings, including their loss rates, are given in Supplementary Table S7, with their involved core concepts in Supplementary Table S8. As we can see in these files, the meanings range from almost complete stability (0.11 probability to change) to almost complete instability (0.99 probability to change). In trying to explain and understand the result, we have to break down the list, merge by concept and class, and test possible explanations, discussed in Section 2.1.
3.3. Possible explanations and correlations of loss rates
The results concerning change rates of various meanings, as indicated before, show a large variation. We compute the results in two different ways, one that give the change rate of concept meanings only (Table 4) and one that give the rates of concept meanings, including meanings occurring in etyma with concepts (Figure 4). As we can see from this graph, the variability increases substantially when all meanings occurring in etyma are added to the concepts. However, the graph also indicates that there are substantial differences between concepts: concepts with low change rates have a low variability in the change rates also of connected meanings, whereas concepts of higher change rates have a higher variability in change also of connected meanings. The variability increases even more when concept meanings and meanings of etyma are grouped by their semantic classes (Figure 5). Some of the classes have a very low variability, such as DOMESTIC ANIMALS and TREES. However, these results are mainly to be explained by a low number of involved concepts and little data rather than low variability in change.
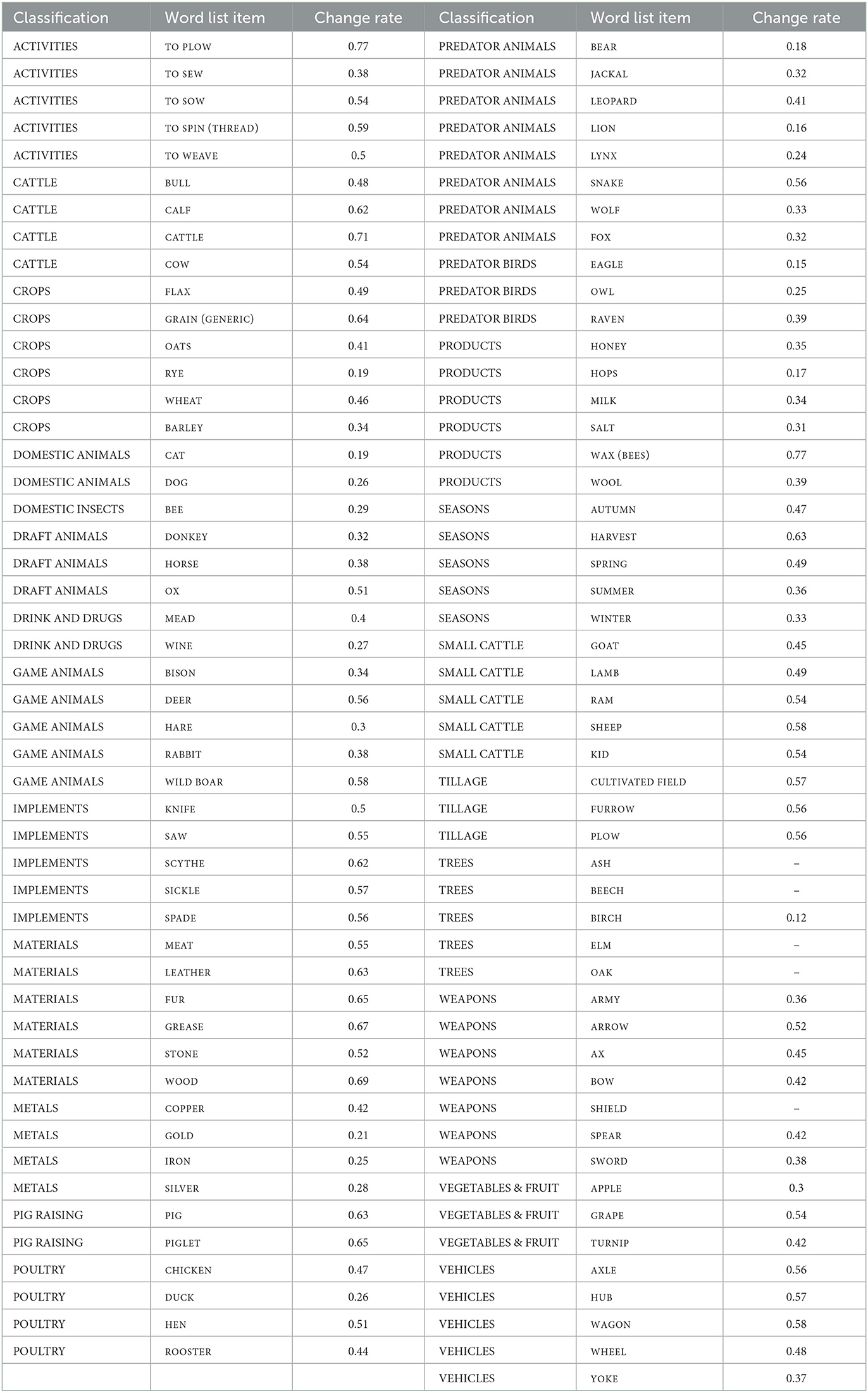
Table 4. Concepts and their classifications, based on their mutual patterns of colexification (Carling, 2019, p. 189–93), and the change rates (=loss rates), excluding the loss rates of connected polysemous meanings.
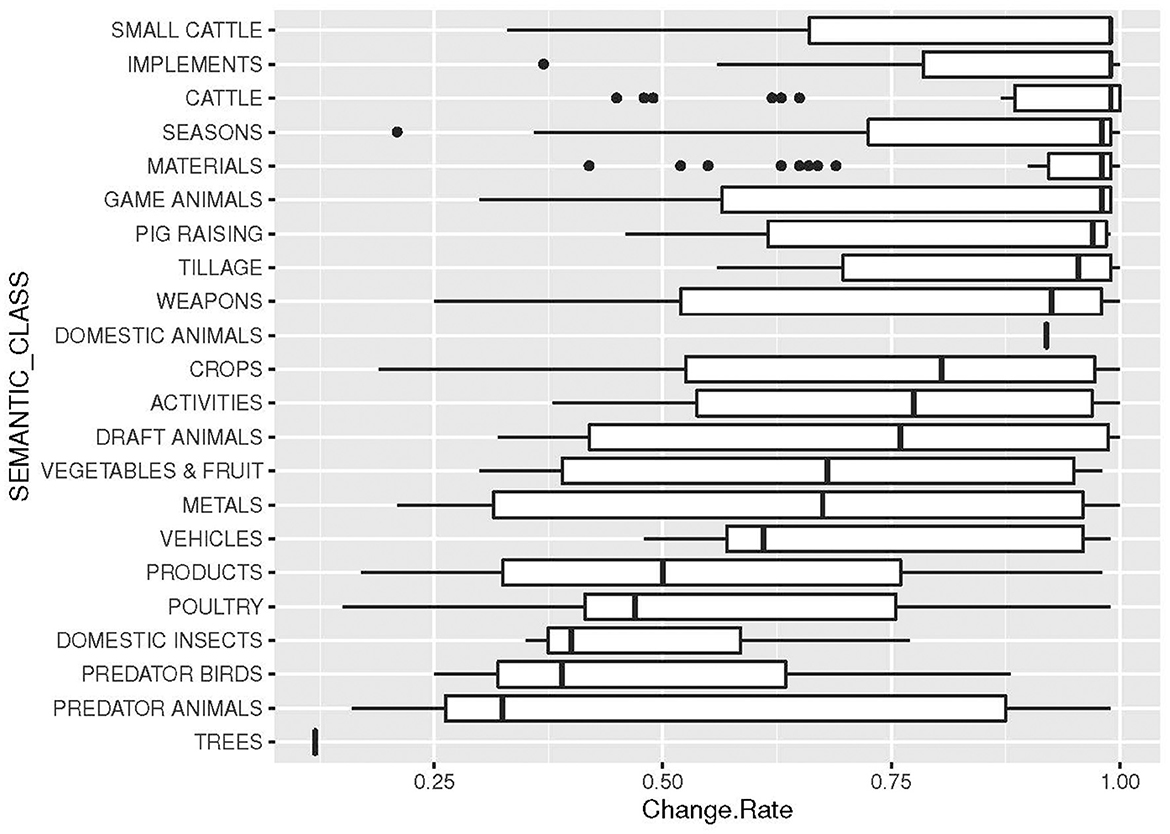
Figure 5. Change rates of concepts, organized by classes (cf. Table 4).
One of our initial aims was to look for cultural explanations of change rates (2.1–2.2). It is tempting to think of cultural explanations when one realizes that, e.g., CATTLE and SMALL CATTLE have higher change rates than wild animals (PREDATORS and GAME ANIMALS), WEAPONS and TILLAGE higher change rates than METALS, and so forth. All these cultural factors are discussed in the original publication of the data (Carling, 2019) and it is also clear that cultural factors have an impact on borrowing, as is demonstrated in another study on our data (Carling et al., 2019a). For that purpose, we initiated a coding of all concepts meanings pertaining to cultural factors. We ran correlation tests between all coded cultural semantic properties (2.1) and change rates but found no correlation between change rates and cultural factors, with one interesting exception, namely the factor TABOO (here only taboo animals). After a first testing of the change rates of concepts and their coded properties, we removed the property coding that gave no results and focused on the properties that showed a result. After this first testing round, only one cultural feature remained (taboo animal), but several of what we labeled “cognitive” properties remained. The properties that showed a significant correlation to change rates we expanded to embrace all 262 remaining meanings, not just the concept meanings (Table 4, Figure 4), and then we ran renewed tests.
To test correlations, we ran a logistic regression test by the package rms in R. We compute the pseudo R2 by a Hosmer–Lemeshow test, calculated significance by a likelihood ratio p-value, and visualized by the packages ggplot2 and dplyr.
The first factor to test was word class. We found a small negative correlation between change rate and nouns (pseudo R-square: 6.41% p-value: 0.0008) and a small positive correlation between change rates and verbs (pseudo R-square: 5.61% p-value: 0.0031). This result is interesting but should possibly be taken with a grain of salt. First, the concept list contains relatively few verbs, and they all show high change rates. Second, it was not possible to account for verbal and nominal derivation in a systematic fashion inside the data, which would potentially affect the outcome.
Moving over to cultural semantic features, we found no correlations, with an interesting exception: in the cultural list, meanings coded as TABOO (Y/N), including the concepts CROW, SNAKE, LEOPARD, WOLF, FOX, JACKAL, LYNX, BEAR, LION, and EAGLE, showed a negative correlation to change rates (pseudo R-square: 24.52% p-value: < 0.0001) (Figure 6). In fact, the taboo animals are among the meanings that show the lowest change rates of the data (Figure 4). In historical linguistic literature, taboo is listed as a key factor triggering semantic change (Hock and Joseph, 1996, p. 231–34), which is probably also correct: languages typically have a number of euphemistic terms for feared and despised animals, such as wolf and bear. However, our results indicate that the basic lexemes for these animals almost never change, which could possibly be an artifact of that they are not used; the euphemistic terms are used instead (cf. Concluding discussion).
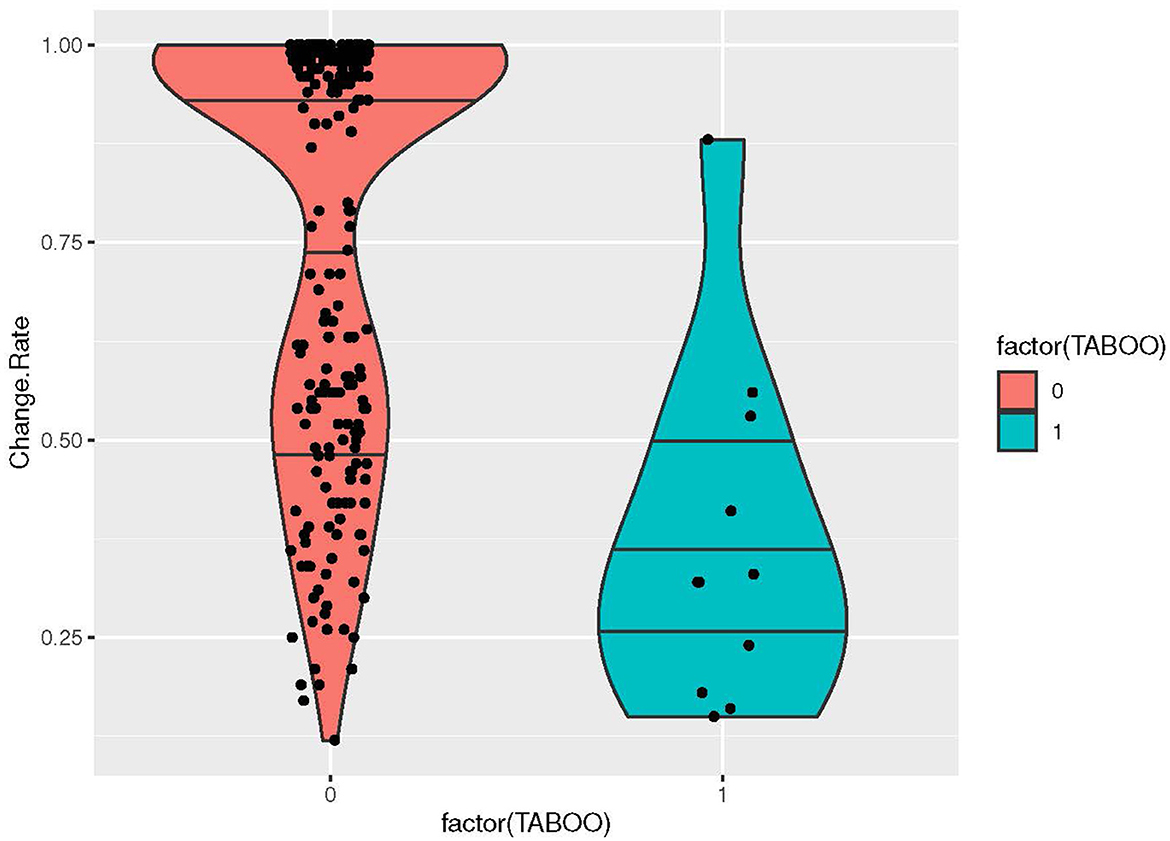
Figure 6. Violin plot visualizing the distribution of change rates for the cultural factor of TABOO ANIMAL (YES/NO), including the concepts CROW, SNAKE, LEOPARD, WOLF, FOX, JACKAL, LYNX, BEAR, LION, and EAGLE.
If we look at the properties that we label as “cognitive”, i.e., inherent properties of entities that impact how they are classified in, e.g., gender and classifier systems (Corbett, 1991, p. 7–32; Frawley, 1992, p. 62–139), we found a negative correlation between ANIMACY=Y and change rate, indicating that animate nouns have lower change rates and inanimate nouns higher change rates (pseudo R-square: 3.88% p-value: 0.0005) (Figure 7). For the properties MASS/COUNT and ABSTRACT/CONCRETE there was no significant correlation. We summarize the most important findings in Table 5.
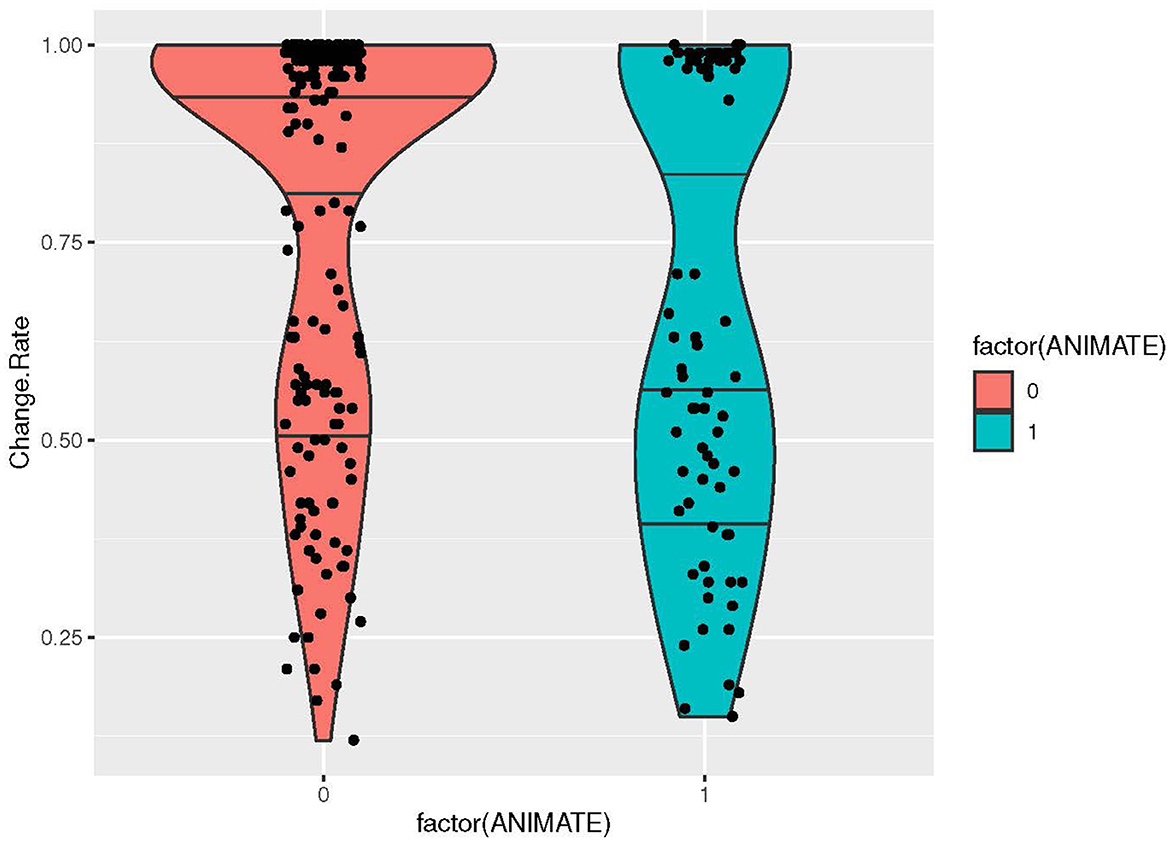
Figure 7. Violin plot visualizing the distribution of change rates for the property factor of ANIMATE (YES/NO).
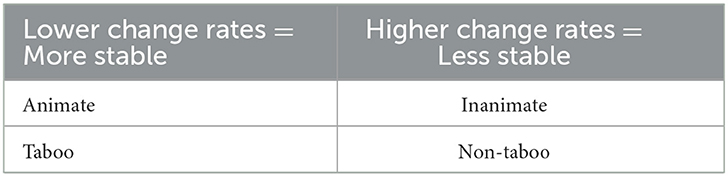
Table 5. Summary of main correlations between semantic change rates and cultural features/ semantic properties.
A further problem worth investigating is the relation between semantic change and the degree of borrowing (or borrowability) of a lexical concept. The original data contained information about loans, including the source and target language of lexemes in the data. The borrowability of concepts were established by computing the rate of certainly borrowed lexemes for each concept, a computation performed in an earlier publication of the same data (Carling et al., 2019a). Our semantic change rates are based on a version of the data set that excludes loans, i.e., loans were excluded before the model was run on the data (2.4). This was done to reduce any noise pertaining to semantic change due to borrowing. We therefore run the semantic change rates against the borrowability scores (i.e., the rates of borrowing for all concepts) of our data (Figure 8, DiACL) as well as against the borrowability scores for the same concepts in the World Loanword Database WOLD (Haspelmath and Tadmor, 2009b) (Figure 8, WOLD). For the purpose of contrasting DiACL and WOLD, we used the recoding of the WOLD data of the previous study of borrowability of lexical concepts, based on the same data as in this study (Carling et al., 2019a). We found small negative correlations between change rate and borrowability (Figure 8). The correlation was stronger against the WOLD score (R = −0.22) than against our own data (R = −0.18). These correlations were not significant at the 0.05 level, but they are significant at the 0.1 level. Although the evidence is weak, this indicates that lexemes that are more likely to be borrowed are less likely to change semantically (see Concluding discussion below).
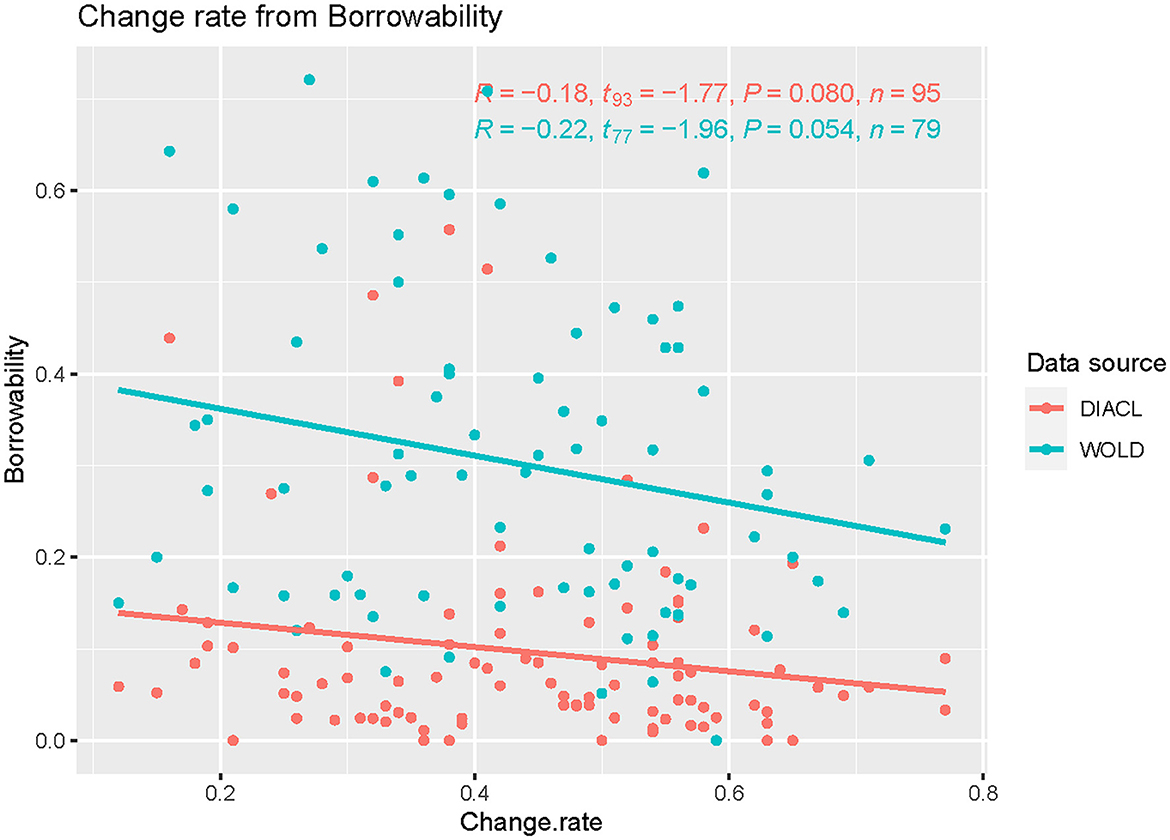
Figure 8. Semantic change rate (x) and borrowability (y) of concepts in the data set, based on the borrowability scores of our own data (DiACL) and the World Loanword Database (WOLD). The graph indicates a negative correlation between change rate and borrowability, which is stronger in the global sample (WOLD) than in our own data (DiACL).
3.4. Evolution with respect to semantic relations
The results of the reconstruction of the coded semantic relations are interesting as well (see Section 2.4 for a description of the model and Table 2 for an overview of the coding). There are 21,689 meaning transitions for which the type of change was computed (Table 6). Of these transitions, almost half (49%) represented no change. If we remove the transitions that imply no change, we are left with 10,987 change transitions. The most frequent change was distant metonymy at 16% of all transitions (32% of change transitions) and near-metonomy at 11% of all (21% of changes). Considering the difficult distinction between these two types (see Section 2.3), it is noteworthy that metonomy was by far more frequent than any other change type, together representing 27% of all transitions (53% of changes). After that, we find metaphor, 10% of all transitions (20% of changes). The is-a-type transitions are all found at lower levels: specialization at 7% of all transitions (14% of changes), generalization at 5% of all transitions (9% of changes), meronymy at 1% of all transitions (2% of changes) and holonymy at 0% of all transitions (1% of changes). These figures are interesting. Apparently, a move from more general to more special is more frequent: specialization is more frequent than generalization, meronomy is more frequent than holonymy. However, the is-a-changes outdo only 13% of all transitions (26% of changes), putting them way behind metonomy and metaphor in frequency. Concerning distant and close change (see Section 2.1), we found that 49% of transitions imply no change, 26% of transitions a distant change and 24% of transitions a close change (see Section 2.3). Among changes, the distribution of distant and close changes (in or outside of a semantic domain) were almost evenly distributed. All these numbers will be further discussed below.
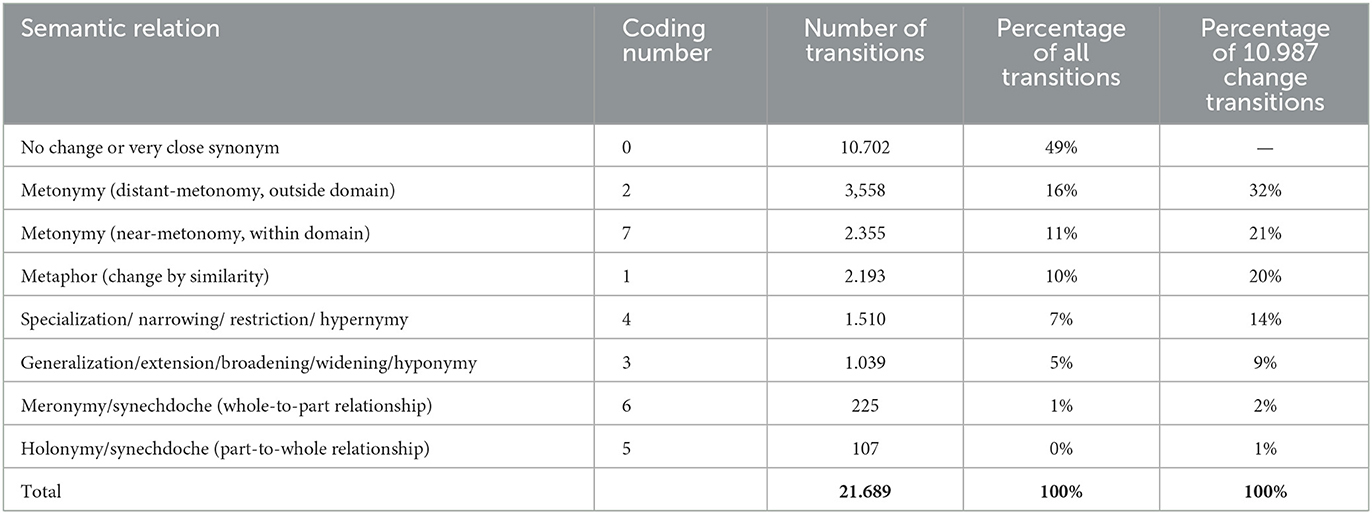
Table 6. Overview of distribution of transition types, defined by their semantic relation, including no change, distant-metonomy, near-metonomy, metaphor, specialization, generalization, meronomy, and holonomy.
4. Concluding discussion
The current study aimed at understanding the mechanisms of semantic evolution, using a quantitative approach. The study has several shortcomings and the results give rise to many questions. However, since there are relatively few computational studies on semantic evolution, we believe that our study can contribute to the field. In this section, we will analyze results and point out some strengths and weaknesses of the performed model, method, data, and result.
We selected to use a model where we take generalized concepts, or prototype words, as a basis for the investigation. The concept model is relatively well established for studying semantic typology and colexification (François, 2008; Poornima and Good, 2010; Jackson et al., 2019; Rzymski et al., 2020). In our study, we made a distinction between concepts (WOLF) and polysemous meanings of concept lexemes (e.g., she-wolf). The concept meaning is then normally one of the polysemous meanings of a lexeme (WOLF: wolf, she-wolf) (Carling, 2019). When we code the semantic relation between the concept word and its colexifying meanings, we used a model that is similar to the extension of diachronic semantic networks used in the study by Georgakopoulos and Polis (2021). This coding of semantic relations between concept words and colexifying meanings served a special purpose: to make a quantitative study of various semantic relation types.
Our first aim was the reconstruct the probability of presence of various meanings at earlier states in lexical etyma, using a phylogenetic model. This aim was inspired by similar reconstructions of comparative concepts in typology (Dunn et al., 2011; Carling and Cathcart, 2021a). Compared to similar reconstructions of typology, both the ingoing data as well as the result of semantics are much more complex and variating. In typology, we typically have a general feature (e.g., WORD ORDER) with a defined number of unique variants (SOV, SVO, VSO, OVS, OSV, SOV), of which the data consists of one of these variants in a synchronic layer. Our data for the semantic reconstruction was constituted by polysemous meanings in individual languages in etymological trees. The number of meanings in a synchronic layer ranged from 1 to 8, but even though the meanings were standardized, our 104 concept meanings colexify with 6,224 meaning types (21,874 tokens). These meanings formed the basis for the reconstruction, which has several consequences. First, many meanings were reconstructed with a medium certainty (0.50), but they did not disappear either (cf. the discussion under 3.1). Moreover, a large number of reconstructions were based on very few meanings, resulting in a high amount of noise in the data (3.1). It is likely that there is a large overlap between few meanings and a result of medium probability in reconstructions (this could possibly have been solved by using another model). For the computation of change rates, which we defined as the probability to lose a meaning after having it, this noise had to be removed (3.2), which resulted in a set of 262 meanings, reconstructed on a satisfactory number of meaning tokens. These were the meanings used to test theories and hypotheses on causes of semantic evolution (3.3).
The model has several shortcomings, most importantly that it cannot identify meaning change diachronically. If a lexeme in a synchronic layer has the polysemous meanings a,b,c,d and then in another (ancestral or descendant) synchronic layer the meanings a,c,e,f , we may assume that the meanings a and c have stayed the same, but we cannot say anything about the assumed path of change for the meanings b,e,f→b,d,e,f. Accordingly, results pertaining to the relation between a (the concept meaning) and the other meanings b,c,d,e,f are merely estimations. The result cannot be compared to a grammatical typological model based on a general feature (e.g., WORD ORDER) with a defined number of unique variants (SOV, SVO, VSO, OVS, OSV, SOV) at different states, where we may reconstruct the transitions between states of these variants (Carling and Cathcart, 2021a).
Despite these shortcomings mentioned here, we believe that the results may give indications of responses to our research questions. Many of the responses were not what we expected, and for other research questions it was not possible to get a response based on the model and the data. Our initial and most important research question was whether meanings could be reconstructed at hidden nodes, based on colexification (2.2). The result indicated that this was possible, but it must be admitted that the result was not very convincing, in particular due to the problem of the noise generated by meanings with few occurrences. Any contrast to a reconstruction of meaning in a traditional comparative-historical model would be arbitrary, for two reasons: first, the problem of dealing with meaning reconstructions based on few occurrences, and second, the problem of the comparative-historical method to give a definite meaning at a proto-language state (3.1).
We envisaged two different causes for semantic change rates: cultural and cognitive. The idea of cultural causes was inspired by the evident impact of culture on borrowing (Carling et al., 2019a), and it would have been possible—even likely—that cultural practices had a similar impact on semantic change rates. Considering the large variation between different concepts and semantic classes (Figures 4, 5) it is tempting to look for cultural explanations. However, even though some tendencies could be observed, none of them were clear enough to establish a significant correlation—with one exception: the group of taboo animals. Initially, we expected taboo animals to be among the groups of higher probability to change—taboo is frequently mentioned as an important triggering mechanism of language change (Hock, 1986, p. 296–94). In the spectrum of higher change rates, we find several classes of animals, including cattle, pigs, and other domestic animals. However, taboo animals were less likely to change than any other semantic class in our data (Figure 5). A possible explanation was to envisage a scenario where taboo animals are lexically substituted in ordinary speech, but the basic lexemes stay the same, resulting in low change. However, there may be other possible explanations, and it is also possible that this correlation becomes lower with larger amounts of data of different types. This remains to be investigated.
For cognitive causes, we hypothesized that concepts of higher frequency and salience would have lower change rates. This has been suggested by other authors, studying basic vocabulary (Pagel et al., 2007; Vejdemo and Hörberg, 2016). However, our data constituted only of culture words, and frequency turned out to be trivial on this type of vocabulary: all lexemes were about equally infrequent (measured on the largest corpora of European languages). Instead, we coded the data for some important inherent semantic properties of importance to nominal categorization, including animacy, abstractness, and countability (Seifart, 2010). Only animacy gave a significant correlation to change rate, and the results were as expected: animate concepts had lower change rates in contrast to inanimate concepts. It is possible that a larger data corpus would give a result also for the other properties (abstractness and countability), but this remains to be investigated. In addition, nouns had lower change rates than verbs, which is an expected result, but the number of verbal concepts in the data were too few to draw serious conclusions of this result.
Further, we have the negative correlation between borrowability and semantic change rates, established both through our own data (DiACL) as well as the World Loanword Database (WOLD) (Figure 8, see Section 3.3). Words that were more likely to be borrowed had generally lower change rates. It is a bit puzzling and difficult to explain. If we follow a model where more frequent and salient words are less likely to be borrowed and more stable (i.e., the basic vocabulary-model), we would expect a reverse correlation, where more unstable lexemes are more likely to be borrowed and change their meaning. It is possible that an investigation of basic vocabulary would have given a different result. A possible scenario is that lexemes that are borrowed are more likely to become distinct and frozen in their meaning. The contrast of our result with a global sample (Haspelmath and Tadmor, 2009a), indicates that the tendency of borrowability is not a restricted, areal phenomenon. For the reason, the negative correlation to semantic change rates is an interesting phenomenon that requires further investigation.
Last but not least, we have the results pertaining to the frequency of semantic relations. Given the caveat of the problem of reconstructing the exact transitions between various meanings, we notice a trend in the results. Metonomy is by far the most frequent type of change, even when metonomy inside the semantic domain (near-metonomy) and metonomy outside of the domain (distant-metonomy) are separated, as in our study. Metaphor is the third most frequent type of change. The is-a-type and is-part-of type changes are much less frequent, but the tendency is evident: a move from more general to more specific is more frequent than the other way around (3.4).
To sum up, we can conclude that a model that reconstructs the probability of lexical meaning in prehistory is possible. This model can also be used to assess the semantic change rates of lexical concepts. Apart from taboo concepts, a cultural model cannot explain the change rates of concepts, whereas a model defining semantic properties is more successful. In general, we conclude that more data and more studies are required to confirm the tendencies of semantic change observed in this study.
Data availability statement
The datasets presented in this study can be found at: https://zenodo.org/record/3817133#.Y-wpHnbP1dh.
Author contributions
GC conceived the method and model, did statistical analyses based on the results from the Markov model estimates, and wrote the text. SC, VBS, and OL compiled and checked the data and gave feedback on the text. JF did the statistical analyses, the graphs, and gave feedback on the text. All authors contributed to the article and approved the submitted version.
Funding
The project was funded by the Marcus and Amalia Wallenberg Foundation, project MAW 2017.0050 (PI GC).
Acknowledgments
Harald Hammarström has performed the probabilistic Markov model estimates of ancestral states and extracted gain and loss rates of meanings. He has no further responsibility for the content of the paper. We also thank the Lund University Humanities Lab for support.
Conflict of interest
The authors declare that the research was conducted in the absence of any commercial or financial relationships that could be construed as a potential conflict of interest.
Publisher's note
All claims expressed in this article are solely those of the authors and do not necessarily represent those of their affiliated organizations, or those of the publisher, the editors and the reviewers. Any product that may be evaluated in this article, or claim that may be made by its manufacturer, is not guaranteed or endorsed by the publisher.
Supplementary material
The Supplementary Material for this article can be found online at: https://www.frontiersin.org/articles/10.3389/fcomm.2023.1126249/full#supplementary-material
Supplementary Table S1. Complete data set, including semantic coding.
Supplementary Table S2. Reconstruction of meaning at internal nodes.
Supplementary Table S3. List of hidden nodes (languages).
Supplementary Table S4. Lexeme meaning transitions.
Supplementary Table S5. Lexeme meaning transitions after adjustment.
Supplementary FIGURE S6. Datapoints change rate.
Supplementary Table S7. Change rate summary after adjustment.
Supplementary Table S8. Change rate summary after adjustment with concepts.
Supplementary Table S9. Probability reconstructions at hidden nodes, overview (Full set in online Material, available at https://zenodo.org/record/7767455#.ZB3AmnbMKDg).
Footnotes
1. ^In this text, we use polysemy to refer to the synchronic occurence of multiple meanings, whereas colexification refers to the phenomenon of multiple meanings from a typological and synchronic perspective.
References
Anttila, R. (1989). Historical and Comparative Linguistics. Current Issues in Linguistic Theory. Amsterdam: John Benjamins Pub. Co.
Barker, G. (2006). The Agricultural Revolution in Prehistory : Why Did Foragers Become Farmers?. Oxford: Oxford University Press Inc.
Benveniste, É., and Lallot, J. (1969). Le Vocabulaire des Institutions Indo-Européennes. 1, Économie, Parenté, Société. Paris: Éditions de Minuit.
Campbell, L. (2013). Historical Linguistics. An Introduction. Edinburgh: Edinburgh University Press.
Carling, G. (2016). Language: The Role of Culture and Environment in Proto-Vocabularies in Human Lifeworlds: The Cognitive Semiotics of Cultural Evolution. Bern: Peter Lang, 83–96.
Carling, G. (2017). DiACL - Diachronic Atlas of Comparative Linguistics Online. Lund: Lund University. Available online at: https://diacl.ht.lu.se/
Carling, G. (2019). Mouton Atlas of Languages and Cultures. Europe and West, Central and South Asia. Berlin - Boston: Mouton de Gruyter.
Carling, G., and Cathcart, C. (2021a). Reconstructing the evolution of Indo-European grammar. Language 97, 561–598. doi: 10.1353/lan.2021.0047
Carling, G., and Cathcart, C. (2021b). Evolutionary dynamics of Indo-Eurpean alignment patterns. Diachronic 23, 2021. doi: 10.1075/dia.19043.car
Carling, G., Cathcart, C., and Round, E. (2021). Reconstructing the origins of language families and variation. Handb. Hum. Symb. Evol. 31, 34. doi: 10.1093/oxfordhb/9780198813781.013.34
Carling, G., Cronhamn, S., Farren, R., Aliyev, E., and Frid, J. (2019a). The causality of borrowing: lexical loans in Eurasian languages. PLOS ONE 30, 588. doi: 10.1371/journal.pone.0223588
Carling, G., van de Weijer, J., Cronhamn, S., Johansson, N., and Farren, R. (2019b). “The Cultural Lexicon of Indo-European in Europe: Quantifying Stability and Change,” in Talking Neolithic: Proceedings of the Workshop on Indo-European Origins held at the Max Planck Institute for Evolutionary Anthropology, Leipzig, eds G. Kroonen, J. P. Mallory, and B. Comrie (Washington, DC: Journal of Indo-European Studies Monograph Series), 39–68.
Chang, W., Hall, D., Cathcart, C., and Garrett, A. (2015). Ancestry-constrained phylogenetic analysis supports the Indo-European steppe hypothesis. Language 91, 194–244. doi: 10.1353/lan.2015.0005
Dellert, J., and Buch, A. (2018). A new approach to concept basicness and stability as a window to the robustness of concept list rankings. Lang. Dyn. Change 8, 157–181. doi: 10.1163/22105832-00802001
Dunn, M. (2014). “Language phylogenies,” in The Routledge Handbook of Historical Linguistics, eds C. Bowern and B. Evans (London; New York, NY: Routledge). 190–211.
Dunn, M., Greenhill, S. J., Levinson, S. C., and Gray, R. D. (2011). Evolved structure of language shows lineage-specific trends in word-order universals. Nature 473, 79–82. doi: 10.1038/nature09923
Fellbaum, C. (1998). WordNet : An Electronic Lexical Database. Language, Speech, and Communication. Cambridge, MA: MIT Press.
François, A. (2008). “Semantic maps and the typology of colexification. Intertwining polysemous networks across languages”, in From Polysemy to Semantic Change: Towards a Typology of Lexical Semantic Associations, ed M. Vanhove (Amsterdam: Benjamins), 163–215.
Geeraerts, D. (1997). Diachronic Prototype Semantics : A Contribution to Historical Lexicology. Oxford Studies in Lexicography and Lexicology. Oxford: Clarendon.
Georgakopoulos, T., Grossman, E., Nikolaev, D., and Polis, S. (2021). Universal and macro-areal patterns in the lexicon: a case-study in the perception-cognition domain. Linguistic Typology. 26, 439–487. doi: 10.1515/lingty-2021-2088
Georgakopoulos, T., and Polis, S. (2021). Lexical diachronic semantic maps: mapping the evolution of time-related lexemes. J. Hist. Ling. 11, 367–420. doi: 10.1075/jhl.19018.geo
Hammarström, H., Forkel, R., Haspelmath, M., and Bank, S. (2021). Glottolog 4, 4. Jena: Max Planck Institute for the Science of Human History.
Handl, S. (2011). The Conventionality of Figurative Language: A Usage-Based Study / Sandra Handl. Language in Performance. Tübingen: Narr.
Haspelmath, M. (2003). The Geometry of Grammatical Meaning: Semantic Maps and Cross-Linguistic Comparison. The New Psychology of language, Vol. 2. Mahwah, NJ: Erlbaum, 211–242.
Haspelmath, M., and Tadmor, U. (2009a). Loanwords in the Worlds Languages: A Comparative Handbook. Berlin: Mouton de Gruyter.
Haspelmath, M., and Tadmor, U. (2009b). World Loanword Database. (WOLD). Leipzig: Max Planck Institute for Evolutionary Anthropology.
Heggarty, P. (2014). “Prehistory through language and archaeology,” in The Routledge Handbook of Historical Linguistics, eds C. Bowern and B. Evans (London, New York: Routledge), 598–626.
Hock, H. H. (1986). Principles of Historical Linguistics Trends in Linguistics. Studies and Monographs. Berlin: Mouton de Gruyter.
Hock, H. H., and Joseph, B. D. (1996). Language History, Language Change, and Language Relationship : An Introduction to Historical and Comparative Linguistics. Trends in Linguistics. Studies and Monographs. Berlin: Mouton de Gruyter.
Hoffman, K., and Tichy, E. (1980). Checkliste zur Aufstellung bzw. Beurteilung Etymologischer Deutungen Zur Gestaltung des Etymologischen Wörterbuches einer Grosscorpus-Sprache. Wien: Österreichischen Akademie der Wissenschaften, 46–52.
Jackson, J. C., Watts, J., Henry, T. R., List, J. M., Forkel, R., Mucha, P. J., et al. (2019). Emotion semantics show both cultural variation and universal structure. Science 366, 1517–1522. doi: 10.1126/science.aaw8160
Jäger, G. (2019). Computational historical linguistics. Theor. Ling. 45, 151–182. doi: 10.1515/tl-2019-0011
Jespersen, O. (1946). Mankind, Nation and Individual: From a Linguistic Point of View. London: Allen and Unwin.
Karjus, A., Blythe, R. A., Kirby, S., Wang, T., and Smith, K. (2021). Conceptual similarity and communicative need shape colexification: an experimental study. Cognitive Sci. 45, 1–30. doi: 10.1111/cogs.13035
Kirby, K. R., Gray, R. D., Greenhill, S. J., Jordan, F. M., Gomes-Ng, S., Bibiko, H. J., et al. (2016). D-PLACE: a global database of cultural, linguistic and environmental diversity. PLoS ONE 11, 1–14. doi: 10.1371/journal.pone.0158391
Klein, E. (1966). A Comprehensive Etymological Dictionary of the English Language: Dealing With the Origin of Words and Their Sense Development Thus Illustrating the History of Civilization and Culture. Amsterdam: Elsevier.
Kuiper, K., Fromont, R., and Gerhard, D. (2018). Polysemy and word frequency: a replication. J. Res. Design Stat. Ling. Commun. Sci. 4, 144–155. doi: 10.1558/jrds.33751
Lakoff, G. (1987). Women, Fire, and Dangerous Things: What Categories Reveal About the Mind. Chicago: University of Chicago Press.
List, J. M. (2018). CLICS2. An improved database of cross-linguistic colexifications assembling lexical data with the help of cross-linguistic data formats. Ling.Typol. 22, 277–306. doi: 10.1515/lingty-2018-0010
List, J. M., Cysouw, M., and Forkel, R. (2016). “Concepticon: a resource for the linking of concept lists,” in Proceedings of the Tenth International Conference on Language Resources and Evaluation, 2393–2400.
Littlemore, J. (2015). Metonymy: Hidden Shortcuts in Language, Thought and Communication. New York, NY: Cambridge University Press.
Lomax, A., Arensberg, C. M., Berleant-Schiller, R., Dole, G. E., Hippler, A. E., Jensen, K. E., et al. (1977). A worldwide evolutionary classification of cultures by subsistence systems [and comments and reply]. Current Anthropol. 18, 659–708. doi: 10.1086/201975
Lyons, J. (1963). Structural Semantics: An Analysis of Part of the Vocabulary of Plato. Oxford: Basil Blackwell.
Mailhammer, R. (2014). Etymology. in Claire Bowern and Bethwyn Evans. (eds.). The Routledge Handbook of Historical Linguistics. (London - New York: Routledge). 423–41.
Maslova, E. (2000). A dynamic approach to the verification of distributional universals. Ling. Typol. 4, 307. doi: 10.1515/lity.2000.4.3.307
Melčuk, I. A. (1996). “Lexical functions: a tool for the description of lexical relations in the lexicon,” in Lexical Functions in Lexicography and Natural Language Processing, ed L. Wanner (Amsterdam - Philadelphia: Benjamins), 37–102.
Newman, J. (2016). “Semantic shift,” in The Routledge Handbook of Semantics, ed N. Riemer (London, NY: Routledge), 266–80.
Pagel, M., Atkinson, Q. D., and Meade, A. (2007). Frequency of word-use predicts rates of lexical evolution throughout Indo-European history. Nature 449, 717. doi: 10.1038/nature06176
Poornima, S., and Good, J. (2010). “Modeling and encoding traditional wordlists for machine applications,” in Proceedings of the 2010 Workshop on NLP and Linguistics: Finding the Common Ground, ACL 2010 (Uppsala: Association for Computational Linguistics), 1–9.
Rzymski, C., Tresoldi, T., Greenhill, S. J., Wu, M. S., Schweikhard, N. E., Koptjevskaja-Tamm, M., et al. (2020). The database of cross-linguistic colexifications, reproducible analysis of cross-linguistic polysemies. Scientific Data 7, 13. doi: 10.1038/s41597-019-0341-x
Seifart, F. (2010). Nominal classification. Lang. Ling. Compass 4, 719–736. doi: 10.1111/j.1749-818X.2010.00194.x
Sweetser, E. (1991). From Etymology to Pragmatics: Metaphorical and Cultural Aspects of Semantic Structure. Cambridge University Press.
Tengi, R. I. (1998). “Design and implementation of the WordNet lexical database and searching software,” in WordNet. An Electronic Lexical Database, ed C. Fellbaum (Cambridge, MA: The MIT Press), 105–27.
Traugott, E. C. (1989). On the rise of epistemic meanings in english: an example of subjectification in semantic change. Language 65, 31–55. doi: 10.2307/414841
Traugott, E. C., and Dasher, R. B. (2002). Regularity in Semantic Change. Cambridge: Cambridge University Press.
Ullmann, S. (1966). “Semantic universals,” in Universals of Language, ed J. H. Greenberg. (Cambridge, MA: MIT Press), 217–262.
Urban, M. (2014). Lexical Semantic Change and Semantic Reconstruction. Routledge Handbook of Historical Linguistics. London - New York: Routledge.
Vejdemo, S., and Hörberg, T. (2016). Semantic factors predict the rate of lexical replacement of content words. PLoS ONE 11, e0147924. doi: 10.1371/journal.pone.0147924
Watkins, C. (2000). The American Heritage Dictionary of Indo-European Roots. Boston: Houghton Mifflin.
Xu, Y., Duong, K., Malt, B. C., Jiang, S., and Srinivasan, M. (2020). Conceptual relations predict colexification across languages. Cognition 201, 104280. doi: 10.1016/j.cognition.2020.104280
Zalizniak, A. A., Ganenkov, B. M. D. G. I, and Maisak T, R. M. (2012). The catalogue of semantic shifts as a database for lexical semantic typology. Linguistics 50, 633–669. doi: 10.1515/ling-2012-0020
Keywords: lexical semantics, language evolution, phylogenetics, Indo-European, colexification
Citation: Carling G, Cronhamn S, Lundgren O, Bogren Svensson V and Frid J (2023) The evolution of lexical semantics dynamics, directionality, and drift. Front. Commun. 8:1126249. doi: 10.3389/fcomm.2023.1126249
Received: 17 December 2022; Accepted: 26 April 2023;
Published: 19 May 2023.
Edited by:
Eitan Grossman, Hebrew University of Jerusalem, IsraelReviewed by:
Ezequiel Koile, Max Planck Institute for Evolutionary Anthropology, GermanyTiago Tresoldi, Uppsala University, Sweden
Copyright © 2023 Carling, Cronhamn, Lundgren, Bogren Svensson and Frid. This is an open-access article distributed under the terms of the Creative Commons Attribution License (CC BY). The use, distribution or reproduction in other forums is permitted, provided the original author(s) and the copyright owner(s) are credited and that the original publication in this journal is cited, in accordance with accepted academic practice. No use, distribution or reproduction is permitted which does not comply with these terms.
*Correspondence: Gerd Carling, Z2VyZC5jYXJsaW5nQGdtYWlsLmNvbQ==; Johan Frid, am9oYW4uZnJpZEBodW1sYWIubHUuc2U=