- 1Faculty for Special Needs Education, Ludwigsburg University of Education, Ludwigsburg, Germany
- 2Institute for Educational Science, Paderborn University, Paderborn, Germany
Reading research shows that phonological decoding skills and intelligence work mostly independent from each other. However, there is a variety of results on the relationship between reading skills and IQ measures. Studies in this field mainly focus on students with reading disabilities (RD) or students with intellectual disabilities (ID) and less on pupils with Special Educational Needs in Learning (SEN-L). We performed a secondary data analysis to evaluate differences in reading skills and fluid intelligence between students with (N = 144) and without (N = 157) SEN-L and the relationship between SEN-L, reading skills, and fluid intelligence. Participants completed a standardized screening of reading skills (SLS 2-9) and a German culture fair intelligence test (CFT 20-R). Students with SEN-L had lower scores in both tests. Correlations between both scores were smaller within the two groups than in the total sample. Implications of the findings are discussed.
Introduction
Special Educational Needs in Learning (SEN-L) are the most prevalent type of SEN in Germany: Regularly, about 2.45% of all students are officially diagnosed as having SEN-L in the academic year 2017/18 (Kultusministerkonferenz, 2019b, c). Most of the federal states in Germany define SEN-L according to the guidelines provided by the conference of the state ministers for education (Kultusministerkonferenz, 2019a).1 These guidelines suggest that SEN-L can be diagnosed if a student fails to achieve the minimal standards of the regular curriculum for a longer period despite adequate individual support provided by the regular education system (Kultusministerkonferenz, 2019a, p. 8). Despite this, some of the federal states use the criterion of an IQ between 85 and 70 (borderline intellectual functioning) as the criterion to distinguish students with SEN-L from “garden variety” poor learners on the one hand and students with intellectual disabilities (ID) on the other hand. In the German school system, SEN-L is distinguished from learning disabilities (LD) as defined by the WHO, such as reading learning disability (RD), or dyslexia. More detailed information on the system of special needs education in Germany can be found in Kocaj et al. (2018) and Sansour and Bernhard (2018).
Reading research suggests that phonological decoding and intelligence mostly work independent from each other (Stanovich, 1988). It is argued that an IQ discrepancy criterion should be irrelevant for the diagnosis of RD as problems in reading skills do not differ between students with discrepant RD and non-discrepant RD (Stanovich et al., 1984; Stanovich, 1988, 1991a,b; Siegel, 1989a,b, 2016; Tønnessen, 1995; Gustafson and Samuelsson, 1999; Klicpera and Gasteiger Klicpera, 2001; Scarborough and Parker, 2003).
However, there is a huge base of research on the relationship of reading skills and intelligence. Research on the correlation of both show a big variance from close to zero up to 0.80 (Bishop and Butterworth, 1980; Schulte and Borich, 1984; Stanovich et al., 1984; Carver, 1990; Naglieri, 1996, 2001; Naglieri and Ronning, 2000; Vellutino, 2001; Cotton and Crewther, 2009). A potential explanation for this variances are differences in the assessments used as well as focusing on different aspects of the two constructs, e.g., verbal measures of intelligence are higher correlated to reading ability than nonverbal measures (Cotton and Crewther, 2009).
Furthermore, intelligence seems to predict reading abilities especially in the context of early literacy and of RD (de Jong and van der Leij, 1999; Tiu et al., 2003; Bowey, 2005; Kortteinen et al., 2009).
All these findings on the relation between intelligence and reading have been obtained with normal readers or persons with RD but not with persons with SEN-L (which is associated with a borderline intellectual functioning and severe learning difficulties in all areas of academic achievement). Despite the fact that students with SEN-L are not students with ID (IQ < 70), some evidence on students with SEN-L could carefully be drawn from research on students with ID: According to Euker (2018) most studies can’t find a relationship between IQ and reading abilities in students with ID (Cohen et al., 2001; Conners et al., 2001; Katims, 2001; Conners et al., 2006). However, intelligence influences the learning progress in reading trainings for students with ID (Allor et al., 2014). For the German context, the study by Euker (2018) suggests evidence for the efficacy of a reading training for students with ID with no significant relationship between intelligence and the training outcomes.
Taken together, there is little knowledge about the relation of (fluid) intelligence and reading skills in students with SEN-L, although IQ is used as a criterion for the diagnosis of SEN-L. The current paper aims to fill this gap using a dataset from the project “How to design educational material for inclusive classrooms” (Noll, 2020; Noll et al., 2020). The following research questions are surveyed:
1. Is there a difference between students with and without diagnosed SEN-L regarding reading skills and fluid intelligence?
2. Does diagnosed SEN-L have an impact on the correlation between reading skills and fluid intelligence?
3. Are reading skills and fluid intelligence good predictors for diagnosed SEN-L?
Methods
Dataset for Secondary Analysis
To contribute mentioned desiderata, we performed a secondary analysis of data from the project “How to design educational material for inclusive classrooms” (Noll, 2020; Noll et al., 2020).
Participants
Students from 24 classes out of 12 different schools with 4 different organizational forms [9 special needs schools for students with SEN-L; 2 inclusive schools; and one Realschule (middle-school)] in the State of Rhineland-Palatinate participated in the initial study. Due to the mathematical background of the original project the main inclusion criteria was that rational numbers had not been part of the curriculum in all the participants’ previous school biography. Also, written consents from all students and parents had to be present. Data collection procedures were approved by the Commissioner for Data Protection and the Supervision and Service Administration Body of the state. The sample size was N = 303 in total. Within the sample N = 144 students were diagnosed with SEN-L. The other 159 participants did not have any Special Educational Needs. Table 1 summarizes the main properties of the sample: On average, participants with SEN-L were about 1 year older than participants without SEN-L. Because of varying curriculums and due to different organizational forms, the age of the participants varied from 9 to 14 years (Mage = 11.1, SDage = 1.04).
Procedures
Data were collected directly in the classrooms in a 90-min sequence. In a 15-min introduction, potential participants were informed about the initial project and the procedure of data collection. The examiner (a Ph.D. student from the project “How to design educational material for inclusive classrooms”) stressed the fact that participation was voluntary and that results had no effect on their school career or grades. Also, full anonymization was guaranteed. Subsequently fluid intelligence was measured with part 1 of the “Grundintelligenztest Skala 2 – CFT 20-R” (Weiß, 2006) administered as group test with prolonged test-time (40-min) to accommodate the pupils with SEN-L as stated in the manual. After that the participants had a 10-min break bevor taking the “Salzburger Lese-Screening für die Schulstufe 2-9–SLS 2-9” (Wimmer and Mayringer, 2014) to test their basic reading ability. The SLS 2-9 was also administered as a group test.
Instruments
The CFT 20-R (Weiß, 2006) is a German culture fair intelligence test. It is based on the theory of fluid and crystallized intelligence (Cattell, 1963) and measures general fluid intelligence using four different subtests (pattern reasoning, classification, matrices, and topologies) utilizing abstract symbols and yielding the use of language. The test can be administered as a group test for children and adolescents between 8; 5; and 19 and up to 60 years for part 1. Reliability for the administered part 1 of the test is high (r = 0.92). Test-retest correlations vary from 0.69 (students with SEN after 5 months) to 0.85 (students from mainstream schools after 2 months). Medium to high correlations (r = 0.60 to r = 0.75) with other construct related intelligence tests (PSB subtests 2, 3, 4; Raven; IST and FAT) speak for the validity of the instrument. The CFT 20-R is scored on an IQ scale with age norms (M = 100; SD = 15).
The SLS 2-9 (Wimmer and Mayringer, 2014) is a time efficient (group) screening measure assessing basic reading comprehension skills in children and adolescents from grades 2 to 9. Participants are asked to give a true or false statement about simple sentences. The raw score is the number of correct answers given within a 3-min period. Reliability (parallel test) ranges from 0.87 for grade 8 to 0.95 for grade 2 students. According to the manual validity analyses show that low results in the SLS 2-9 are linked to more and longer fixations of single words in gaze-tracking studies. Convergent Validity with the more extensive ELFE II is high (rct = 0.77; Lenhard et al., 2018). The SLS 2-9 is scored on a standardized scale with class norms (M = 100; SD = 15).
Data Analysis
Data analysis was done using GNU R (R Core Team, 2019). Descriptive statistics were calculated using the psych-package (Revelle, 2018).
To answer research question (1) we compared the results of students with and without SEN-L using independent t-tests. Welch-correction was used if Levene’s test indicated that assumption of homogeneity of variances was violated. Cohen’s d was calculated using the effsize-package (Torchiano, 2019). Research question (2) was evaluated using Pearson correlations.
For research question 3, logistic regression (Long, 1997; Field, 2015) with SEN-L as dependent variable and with reading skills and fluid intelligence as predictor variables was performed using the glm-function in Gnu R. Model χ2 was computed using the lrtest-function from the lmtest-package (Zeileis and Hothorn, 2002). As a goodness-of-fit index we calculated pseudo R2 as introduced by Cox and Snell (1989) and Nagelkerke (1991) using the PseudoR2-function from the DescTools-package (Signorell et al., 2020).
Results
As displayed in Table 2 we could show that students without SEN had significantly higher fluid intelligence (d = 1.49) as well as significantly better reading skills (d = 1.15) than their peers with SEN-L. Within the total sample, reading skills, and fluid intelligence were strongly positively correlated with r(301) = 0.51, p < 0.001. Splitting the sample into the two groups (students with SEN-L vs. students without SEN) resulted in reduced correlations of r(157) = 0.33 (students without SEN; p < 0.001), and r(142) = 0.31 (students with SEN-L; p < 0.001).
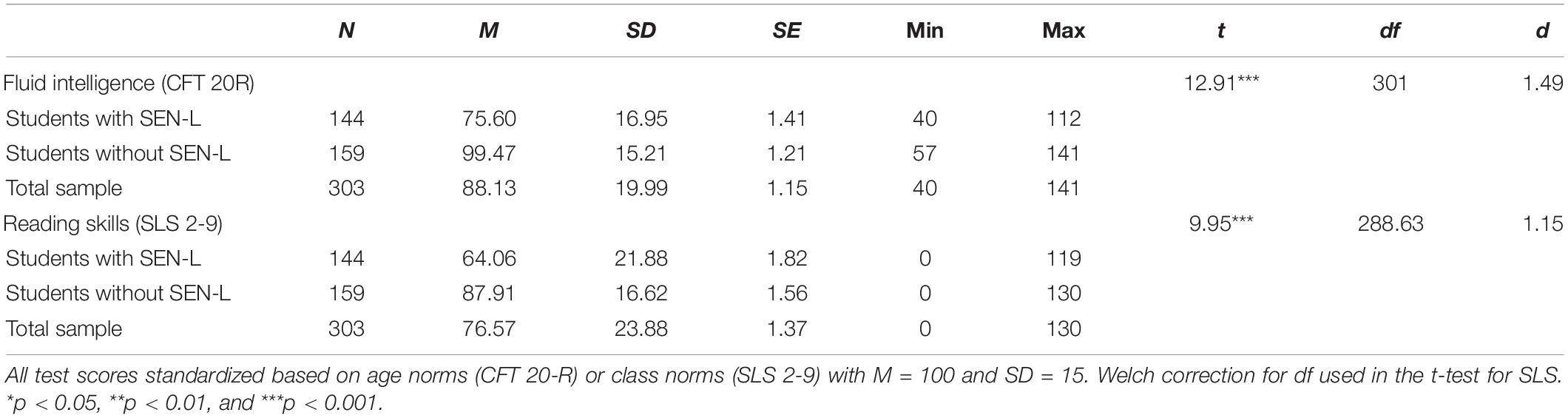
Table 2. Descriptive Statistics and independent sample t-test for group differences in fluid intelligence and reading skills.
To analyze if reading skills and fluid intelligence have an impact on the probability to be diagnosed as having a SEN-L, we used logistic regression with the grouping factor SEN-L as dependent variable and with reading skills and fluid intelligence as predictor variables (see Table 3). With pseudo R2 = 0.407 (Cox and Snell) and pseudo R2 = 0.543 (Nagelkerke) the model predicts the diagnosis of SEN-L quite well.
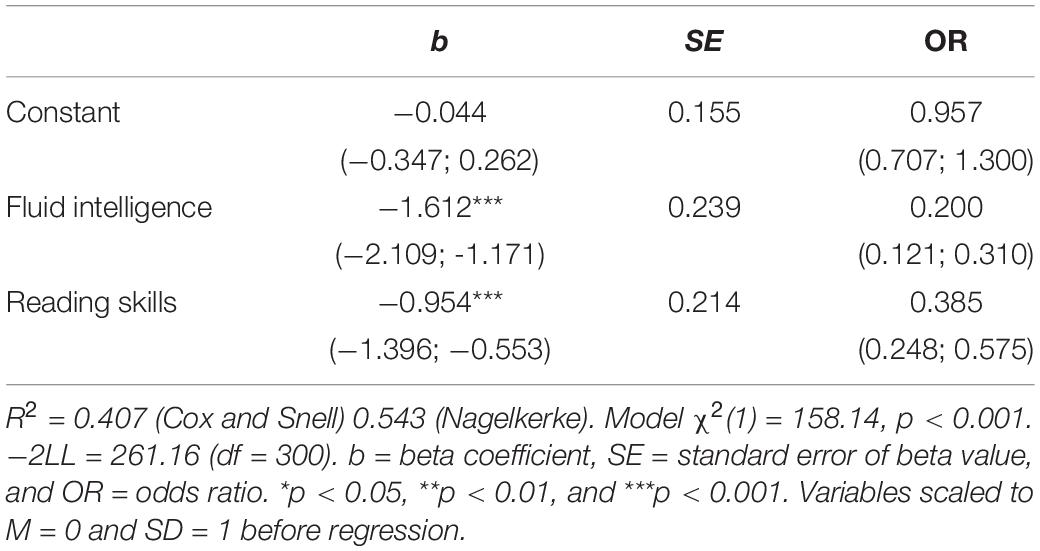
Table 3. Coefficients with 95%-CI of the model predicting whether a student has been diagnosed with SEN-L.
Discussion
The finding that the two groups perform differently in measures of reading and fluid intelligence was not surprising. However, due to the unclear definition and diagnostic criteria of SEN-L in Germany this was not self-evident. Table 2 shows an apparent amount of overlap in the scores between the groups. Therefore, it seemed necessary to check this difference before further analysis.
We could find medium correlations between reading skills and fluid intelligence in both groups. These correlations did not differ between the two groups (z = −0.19, p = 0.42).
These findings for students with SEN-L are congruent to the findings for regular students as they are reported in the introduction. This provides first evidence that findings from the general population regarding the relationship between reading and intelligence might be transferred to the group of pupils with SEN-L and that fluid intelligence does not seem to be the main factor to explain reading difficulties in pupils with SEN-L. Also, the results of our analysis question the role of IQ measures in diagnosing SEN-L. The findings suggest that reading education for SEN-L students should be like established recommendations based on RD in general. We would argue for using the actual learning progress as the best indicator for educational decision making in an evidence-based reading program instead of IQ measures. If standardized evidence-based measures of reading instruction do not lead to sufficient learning progress, individualized support can be applied.
Furthermore, according to the results for research question 3, in a logistic regression reading skills and fluid intelligence worked quite well as predictors for the diagnosis of SEN-L. With increasing level of fluid intelligence and reading skills the chance of being diagnosed with SEN-L decreases significantly. However, if taking reading skills and fluid intelligence as a hard criterion for severe learning problems, it can be assumed that a substantial numbers of actual SEN-L diagnoses are false positives. This finding, which is congruent to Kottmann (2006), is problematic, because in the German school system this diagnosis has an important impact on school career with strong restrictions for possible graduations and choices of vocation.
Altogether, our data suggests that general findings from populations without SEN concerning the relationship between fluid intelligence and reading skills might be transferred to the population of pupils with SEN-L. For the practical service for pupils our analysis strengthens evidence to not overestimate the role of (fluid) intelligence for diagnostic decisions.
Limitations
Although the number of participants to answer our questions is statistically sufficient, limitations to the generalization of our findings may arise due to the sampling process which was based on classes’ mathematical background knowledge instead of sociodemographic data. Also, for pupils with SEN-L the measurement of intelligence and reading via a group-tests might not be ideal. Test accommodation for pupils with not apparent additional special needs could not be guaranteed within the reading measure.
Data Availability Statement
The data analyzed in this study is subject to the following licenses/restrictions: We do not have permission to share the full dataset. Requests to access these datasets should be directed to Anna Noll, bm9sbEB1bmktbGFuZGF1LmRl.
Ethics Statement
Ethical review and approval was not required for the study on human participants in accordance with the local legislation and institutional requirements. Written informed consent to participate in this study was provided by the participants’ legal guardian/next of kin.
Author Contributions
MS and DS developed the research questions, planned the data analysis, did the interpretation of the results, drafted the introduction, discussion, and limitation sections, and edited the final manuscript for submission. MS provided the data set from the project “How to design educational material for inclusive classrooms” and drafted the methods section (excluding data analysis). DS did the data analysis and drafted the data analysis subsection of the methods section. Both authors contributed to the article and approved the submitted version.
Funding
The dataset was part of the project “How to Design Educational Material for Inclusive Classes” within the DFG graduate school UpGrade funded by Deutsche Forschungsgemeinschaft (DFG). Open access publication was funded by the Ludwigsburg University of Education.
Conflict of Interest
The authors declare that the research was conducted in the absence of any commercial or financial relationships that could be construed as a potential conflict of interest.
Footnotes
- ^ The Kultusministerkonferenz is the panel where the ministers for education of all German federal states discuss and develop common guidelines. These guidelines are tentative recommendations.
References
Allor, J. H., Mathes, P. G., Roberts, J. K., Cheatham, J. P., and Otaiba, S. A. (2014). Is scientifically based reading instruction effective for students with below-average IQs? Except. Child. 80, 287–306. doi: 10.1177/0014402914522208
Bishop, D. V. M., and Butterworth, G. E. (1980). Verbal-performance discrepancies: relationship to birth risk and specific reading retardation. Cortex 16, 375–389. doi: 10.1016/S0010-9452(80)80039-6
Bowey, J. A. (2005). “Predicting individual differences in learning to read,” in The Science of Reading: A Handbook, eds M. J. Snowling, and C. Hulme (Oxford: Blackwell), 155–172. doi: 10.1002/9780470757642.ch9
Carver, R. P. (1990). Intelligence and reading ability in grades 2–12. Intelligence 14, 449–455. doi: 10.1016/S0160-2896(05)80014-5
Cattell, R. B. (1963). Theory of fluid and crystallized intelligence: a critical experiment. J. Educ. Psychol. 54, 1–22. doi: 10.1037/h0046743
Cohen, D., Rivière, J. P., Plaza, M., Thompson, C., Chauvin, D., Hambourg, N., et al. (2001). Word identification in adults with mild mental retardation: does IQ influence reading achievement? Brain Cogn. 46, 69–73. doi: 10.1016/S0278-2626(01)80037-3
Conners, F. A., Atwell, J. A., Rosenquist, C. J., and Sligh, A. C. (2001). Abilities underlying decoding differences in children with intellectual disability. J. Intellect. Disabil. Res. JIDR 45(Pt 4), 292–299. doi: 10.1046/j.1365-2788.2001.00319.x
Conners, F. A., Rosenquist, C. J., Sligh, A. C., Atwell, J. A., and Kiser, T. (2006). Phonological reading skills acquisition by children with mental retardation. Res. Dev. Disabil. 27, 121–137. doi: 10.1016/j.ridd.2004.11.015
Cotton, S. M., and Crewther, S. G. (2009). The relationship between reading and intelligence in primary school aged children: implications for definitional models of dyslexia. Open Educ. J. 2, 42–50. doi: 10.2174/1874920800902010042
Cox, D. R., and Snell, E. J. (1989). “Analysis of binary data,” in Monographs on Statistics and Applied Probability, 2 Edn, ed. P. McCullagh (London: Chapman and Hall).
de Jong, P. F., and van der Leij, A. (1999). Specific contributions of phonological abilities to early reading acquisition: results from a Dutch latent variable longitudinal study. J. Educ. Psychol. 91, 450–476. doi: 10.1037/0022-0663.91.3.450
Euker, N. (2018). Entwicklung und Evaluation eines Silbenbasierten Leselehrgangs bei Schülerinnen und Schülern mit geistiger Behinderung[Development and Evaluation of a syllable-based Reading Program for Students with Intellectual Disabilities]. Ph.D. thesis, Justus-Liebig-Universität, Gießen.
Field, A. P. (2015). Discovering statistics using IBM SPSS statistics: And Sex and Drugs and Rock ‘n’ Roll, 4th Edn. Thousand Oaks, CA: SAGE.
Gustafson, S., and Samuelsson, S. (1999). Intelligence and dyslexia: implications for diagnosis and intervention. Scand. J. Psychol. 40, 127–134. doi: 10.1111/1467-9450.00109
Katims, D. S. (2001). Literacy assessment of students with mental retardation: an exploratory investigation. Educ. Train. Ment. Retardat. Dev. Disabil. 36, 363–372.
Klicpera, C., and Gasteiger Klicpera, B. (2001). Macht Intelligenz einen Unterschied? Rechtschreiben und phonologische Fertigkeiten bei diskrepanten und nichtdiskrepanten Lese/Rechtschreibschwierigkeiten [Does intelligence make a difference? Spelling and phonological skills in children with discrepant and non-discrepant reading/writing difficulties]. Z. Kinder Jugendpsychiatr. Psychother. 29, 37–49. doi: 10.1024//1422-4917.29.1.37
Kocaj, A., Kuhl, P., Jansen, M., Pant, H. A., and Stanat, P. (2018). Educational placement and achievement motivation of students with special educational needs. Contemp. Educ. Psychol. 55, 63–83. doi: 10.1016/j.cedpsych.2018.09.004
Kortteinen, H., Närhi, V., and Ahonen, T. (2009). Does IQ matter in adolescents’ reading disability? Learn. Individ. Diff. 19, 257–261. doi: 10.1016/j.lindif.2009.01.003
Kottmann, B. (2006). Selektion in die Sonderschule: Das Verfahren zur Feststellung von sonderpädagogischem Förderbedarf als Gegenstand empirischer Forschung [Selection in the Special School: The Procedure of Diagnosing Special Educational Needs as a Subject for Empirical Research]. Leipzig: Klinkhardt.
Kultusministerkonferenz (2019a). Empfehlungen zur schulischen Bildung, Beratung und Unterstützung von Kindern und Jugendlichenim sonderpädagogischen Schwerpunkt LERNEN [Recommendations for school education, councelling, and support of students with special educational needs in learning]. Available online at: https://www.kmk.org/fileadmin/Dateien/veroeffentlichungen_beschluesse/2019/2019_03_14-FS-Lernen.pdf (accessed June 29, 2020).
Kultusministerkonferenz (2019b). Sonderpädagogische Förderung in Allgemeinen Schulen (ohne Förderschulen) 2017/18 [Special needs education in regular schools (without special schools) 2017/18]. Available online at: https://www.kmk.org/fileadmin/Dateien/pdf/Statistik/Dokumentationen/Aus_SoPae_Int_2017.pdf (accessed June 29, 2020).
Kultusministerkonferenz (2019c). Sonderpädagogische Förderung in Förderschulen 2017/18 [Special needs education in Special Schools 2017/18]. Available online at: https://www.kmk.org/fileadmin/Dateien/pdf/Statistik/Dokumentationen/Aus_Sopae_2017.pdf (accessed June 29, 2020).
Lenhard, W., Lenhard, A., and Schneider, W. (2018). ELFE II. Ein Leseverständnistest für Erst- bis Siebtklässler – Version II, 3rd Edn. Boston, MA: Hogrefe.
Long, J. S. (1997). Regression Models for Categorical and Limited Dependent Variables. Abingdon: SAGE.
Nagelkerke, N. J. D. (1991). A Note on a General Definition of the Coefficient of Determination. Biometrika 78:691. doi: 10.2307/2337038
Naglieri, J. A. (1996). An examination of the relationship between intelligence and reading achievement using the MAT-SF and MAST. J. Psychoeduc. Assess. 14, 65–69. doi: 10.1177/073428299601400106
Naglieri, J. A. (2001). Do ability and reading achievement correlate? J. Learn. Disabil. 34, 304–310. doi: 10.1177/002221940103400403
Naglieri, J. A., and Ronning, M. E. (2000). The relationship between general ability using the naglieri nonverbal ability test (NNAT) and stanford achievement test (SAT) reading achievement. J. Psychoeduc. Assess. 18, 230–239. doi: 10.1177/073428290001800303
Noll, A. (2020). Lesebarrieren in einem inklusiven Mathematikunterricht überwinden: Ergebnisse einer qualitativen und einer quantitativen Studie [How to Overcome Reading Barriers in Inclusive Mathematics Instructions: Results from a Qualitative and a Quantitative Study] (1st ed. 2020). Landauer Beiträge zur mathematikdidaktischen Forschung. Cham: Springer.
Noll, A., Roth, J., and Scholz, M. (2020). Lesebarrieren im inklusiven Mathematikunterricht überwinden – visuelle und sprachliche Unterstützungsmaßnahmen im empirischen Vergleich [How to overcome reading barriers in inclusive mathematics instructions – an empirical comparison of visual and linguistic support]. J. Math. Didaktik 41, 157–190. doi: 10.1007/s13138-020-00158-z
R Core Team (2019). R: A Language and Environment for Statistical Computing. Vienna: R Foundation for Statistical Computing.
Revelle, W. (2018). psych: Procedures for Psychological, Psychometric, and Personality Research. Available online at: https://cran.r-project.org/web/packages/psych/index.html (accessed June 29, 2020).
Sansour, T., and Bernhard, D. (2018). Special needs education and inclusion in Germany and Sweden. Alter 12, 127–139. doi: 10.1016/j.alter.2017.12.002
Scarborough, H. S., and Parker, J. D. (2003). Matthew effects in children with learning disabilities: development of reading, IQ, and psychosocial problems from grade 2 to grade 8. Ann. Dyslexia 53, 47–71. doi: 10.1007/s11881-003-0004-6
Schulte, A., and Borich, G. D. (1984). Considerations in the use of difference scores to identify learning-disabled children. J. Sch. Psychol. 22, 381–390. doi: 10.1016/0022-4405(84)90026-8
Siegel, L. S. (1989a). IQ is irrelevant to the definition of learning disabilities. J. Learn. Disabil. 22, 469–478. doi: 10.1177/002221948902200803
Siegel, L. S. (1989b). Why we do not need intelligence tests scores in the definition and analyses of learning disabilities? J. Learn. Disabil. 22, 514–518. doi: 10.1177/002221948902200814
Siegel, L. S. (2016). “IQ and learning disabilities: R.I.P,” in Learning Disabilities: Theoretical and Research Issues, eds H. L. Swanson, and B. K. Keogh (Abingdon: Routledge), 111–128.
Signorell, A., Aho, K., Alfons, A., Anderegg, N., Aragon, T., Arppe, A., et al. (2020). DescTools: Tools for Descriptive Statistics. Available online at: https://cran.r-project.org/web/packages/DescTools/index.html (accessed June 29, 2020).
Stanovich, K. E. (1988). Explaining the differences between the dyslexic and the garden-variety poor reader: the phonological-core variable-difference model. J. Learn. Disabil. 21, 590–604. doi: 10.1177/002221948802101003
Stanovich, K. E. (1991a). Conceptual and empirical problems with discrepancy definitions of reading disability. Learn. Disabil. Q. 14, 269–280. doi: 10.2307/1510663
Stanovich, K. E. (1991b). Discrepancy definitions of reading disability: has intelligence led Us astray? Read. Res. Q. 26, 7–29.
Stanovich, K. E., Cunningham, A. E., and Feeman, D. J. (1984). Intelligence, cognitive skills, and early reading progress. Read. Res. Q. 19, 278–303.
Tiu, R. D., Thompson, L. A., and Lewis, B. A. (2003). The role of IQ in a component model of reading. J. Learn. Disabil. 36, 424–436. doi: 10.1177/00222194030360050401
Torchiano, M. (2019). effsize: Efficient Effect Size Computation. Available online at: https://cran.r-project.org/web/packages/effsize/index.html (accessed June 29, 2020).
Tønnessen, F. E. (1995). On defining ‘Dyslexia’. Scand. J. Educ. Res. 39, 139–156. doi: 10.1080/0031383950390205
Vellutino, F. R. (2001). Further analysis of the relationship between reading achievement and intelligence. J. Learn. Disabil. 34, 306–310. doi: 10.1177/002221940103400404
Wimmer, H., and Mayringer, H. (2014). SLS 2-9: Salzburger Lese-Screening für die Schulstufen 2-9. Boston, MA: Hogrefe.
Keywords: reading skills, fluid intelligence, Special Educational Needs, secondary data analysis, Germany
Citation: Scholz M and Scheer D (2020) The Relationship Between Reading Skills and Intelligence in Students With and Without Special Educational Needs in Learning. Front. Educ. 5:123. doi: 10.3389/feduc.2020.00123
Received: 30 March 2020; Accepted: 22 June 2020;
Published: 08 July 2020.
Edited by:
Brahm Norwich, University of Exeter, United KingdomReviewed by:
Emmanouela Terlektsi, University of Birmingham, United KingdomGeoff Anthony Lindsay, University of Warwick, United Kingdom
Copyright © 2020 Scholz and Scheer. This is an open-access article distributed under the terms of the Creative Commons Attribution License (CC BY). The use, distribution or reproduction in other forums is permitted, provided the original author(s) and the copyright owner(s) are credited and that the original publication in this journal is cited, in accordance with accepted academic practice. No use, distribution or reproduction is permitted which does not comply with these terms.
*Correspondence: Markus Scholz, bWFya3VzLnNjaG9sekBwaC1sdWR3aWdzYnVyZy5kZQ==; David Scheer, ZGF2aWQuc2NoZWVyQHVwYi5kZQ==