- 1TUM School of Education, Chair for Educational Psychology, Technical University of Munich, Munich, Germany
- 2TUM School of Education, Centre for International Student Assessment, Technical University of Munich, Munich, Germany
Teachers' ability to assess student cognitive and motivational-affective characteristics is a requirement to support individual students with adaptive teaching. However, teachers have difficulty in assessing the diversity among their students in terms of the intra-individual combinations of these characteristics in student profiles. Reasons for this challenge are assumed to lie in the behavioral and cognitive activities behind judgment processes. Particularly, the observation and utilization of diagnostic student cues, such as student engagement, might be an important factor. Hence, we investigated how student teachers with high and low judgment accuracy differ with regard to their eye movements as a behavioral and utilization of student cues as a cognitive activity. Forty-three participating student teachers observed a video vignette showing parts of a mathematics lesson to assess student characteristics of five target students, and reported which cues they used to form their judgment. Meanwhile, eye movements were tracked. Student teachers showed substantial diversity in their judgment accuracy. Those with a high judgment accuracy showed slight tendencies toward a more “experienced” pattern of eye movements with a higher number of fixations and shorter average fixation duration. Although all participants favored diagnostic student cues for their assessments, an epistemic network analysis indicated that student teachers with a high judgment accuracy utilized combinations of diagnostic student cues that clearly pointed to specific student profiles. Those with a low judgment accuracy had difficulty using distinct combinations of diagnostic cues. Findings highlight the power of behavioral and cognitive activities in judgment processes for explaining teacher performance of judgment accuracy.
Introduction
Teacher assessment skills are an essential component of professional competence (Baumert and Kunter, 2006, 2013; Binder et al., 2018). In their daily professional lives, teachers are required to continuously make educational decisions when assigning grades, planning lessons, adapting their teaching, and providing feedback. To effectively make informed decisions, teachers must constantly monitor their students' learning-relevant cognitive (e.g., cognitive abilities or knowledge) and motivational-affective characteristics (e.g., academic self-concept or interest) and the specific combination of these characteristics within individual students (Corno, 2008; Herppich et al., 2018; Heitzmann et al., 2019; Loibl et al., 2020). Some students may, for example, possess high cognitive characteristics combined with low motivational-affective characteristics, indicating underestimation of their abilities. Other students are aware of their abilities, and hold high cognitive and motivational-affective characteristics (Seidel, 2006). Students with such varying profiles differ in how they engage with, achieve in, and experience their learning environment (Seidel, 2006; Lau and Roeser, 2008; Jurik et al., 2013, 2014), and their positive educational development depends on tailored teacher instruction (Huber et al., 2015). Therefore, it is alarming to know that teachers are struggling to accurately assess the diversity of cognitive and motivational-affective characteristics among their students (Huber and Seidel, 2018; Südkamp et al., 2018). To assess student profiles accurately, teachers are required to observe their students for relevant cues, such as the intensity and content of engagement, and use combinations of such cues to infer underlying combinations of student characteristics (Cooksey et al., 1986; Nestler and Back, 2013; Thiede et al., 2015). Yet systematic linkages of judgment processes and judgment accuracy are rather rare (Karst and Bonefeld, 2020), and are still unclear in terms of how they are used to assess student profiles (Praetorius et al., 2017; Huber and Seidel, 2018). Thus, the aim of the present study is to explore such connections and contribute to existing research in the field by providing detailed insights into the process of observing and assessing student profiles. Therefore, we investigate how well teachers are able to accurately judge various student profiles. Moreover, we link this judgment accuracy to two factors: eye movements (as a measure of the behavioral activity of observing students) and utilization of student cues (as a measure of the cognitive activity) behind judgment processes.
Student Characteristic Profiles as Targets for Teacher Assessment
Since the seminal work of Snow (1989), cognitive and motivational-affective student characteristics are seen as fundamental determinants of learning and achievement. Robust empirical studies with large representative samples and meta-analyses have shown that cognitive abilities (Deary et al., 2007; Roth et al., 2015), pre-achievement (Steinmayr and Spinath, 2009), academic self-concept as students' perception of their subject-specific abilities (Shavelson et al., 1976; Valentine et al., 2004; Steinmayr and Spinath, 2009; Huang, 2011; Marsh and Martin, 2011), and subject interest (Schiefele et al., 1992; Jansen et al., 2016) are among the most decisive student characteristics for educational outcomes.
Consistent and Inconsistent Combinations of Cognitive and Motivational-Affective Characteristics
A strand of research has begun to investigate the complex and interrelated influences that cognitive and motivational-affective characteristics might have on student learning. Therefore, researchers have followed a person-centered approach to examine the intra-individual interplay of student characteristics for the purposes of identifying which combinations of cognitive and motivational-affective characteristics are predominant among students (Seidel, 2006; Lau and Roeser, 2008; Linnenbrink-Garcia et al., 2012; Seidel et al., 2016; Südkamp et al., 2018).
Seidel (2006), for example, used a latent-cluster analysis to identify homogenous subgroups of students that are distinct from one another, in that each subgroup showed a unique pattern of cognitive characteristics—cognitive abilities and pre-knowledge—combined with subject interest and academic self-concept as motivational-affective characteristics. Five so-called student profiles were identified. Two of these profiles can be seen as “consistent,” in that they are assigned to individuals who displayed either low or high levels of cognitive and motivational-affective characteristics: First, “strong” students were very likely to show high values for all characteristics. Second, students who were likely to show low values for all characteristics and were labeled as “struggling.” The remaining three profiles are considered to be “inconsistent,” since the interplay of cognitive and motivational-affective characteristics within individuals to whom these profiles are assigned are either opposing or non-uniform: “Overestimating” students showed relatively low values for cognitive characteristics but were likely to report high subject interest and positive self-concept. Hence, these students might overestimate their abilities. “Underestimating” students displayed an opposite pattern in which high cognitive abilities were combined with low interest and low self-concept. These students seemed to underestimate their abilities. Finally, “uninterested” students stood out due to their high cognitive abilities and particularly low subject interest. Altogether, 57% of the students investigated by Seidel (2006) belonged to inconsistent profiles.
Looking at student diversity from the viewpoint of student characteristic profiles is meaningful, since other studies have repeatedly found mixtures of consistent and inconsistent profiles (Lau and Roeser, 2008; Linnenbrink-Garcia et al., 2012; Seidel et al., 2016; Südkamp et al., 2018). In all of these studies, there was a significant proportion of students that shared inconsistent profiles, ranging in studies from 10% (Südkamp et al., 2018) to more than half of investigated students (Seidel, 2006; Linnenbrink-Garcia et al., 2012). This seems to be a generalizable finding, since the reviewed studies are spread across different subjects including physics, science, biology, mathematics, and language arts, and addressed different cognitive (e.g., cognitive abilities, pre-knowledge, grades) and motivational-affective characteristics (e.g., academic self-concept, learning motivation, anxiety, task-value; Seidel, 2006; Lau and Roeser, 2008; Linnenbrink-Garcia et al., 2012; Seidel et al., 2016; Südkamp et al., 2018). For teachers, these inconsistent profiles are meaningful and quite likely to be present in every classroom. One way in which specific differences between the profiles become apparent to teachers is through student engagement as a central component of student learning.
Student Engagement Reflects Student Characteristic Profiles
Relationships exist between student characteristic profiles and engagement in learning activities as a precondition for achievement. These relationships have been examined in research from two perspectives: first using students' own reports of classroom experience as an antecedent of their engagement; and second by proximal assessments of student engagement through self-reports and video observation. Students with high motivational-affective characteristics seem to perceive their learning environment as particularly supportive and experience high-quality teaching (Seidel, 2006; Lau and Roeser, 2008). Along with these positive perceptions, students with high motivational-affective characteristics are also more frequently cognitively and behaviorally engaged in learning activities independent of the level of their cognitive characteristics. They report high levels of elaborating and organizing information (Jurik et al., 2014), attention, and participation in learning activities and classroom talk (Lau and Roeser, 2008), and show especially high numbers of verbal interactions with teachers (Jurik et al., 2013). In contrast, students with low motivational-affective characteristics often perceive their learning environment in a negative way (Seidel, 2006; Lau and Roeser, 2008) and suffer from low engagement (Lau and Roeser, 2008; Jurik et al., 2013, 2014). These differences in engagement result in differential effects on student learning and achievement (Lau and Roeser, 2008; Linnenbrink-Garcia et al., 2012).
Diversity in terms of characteristic profiles shapes students' classroom experiences and engagement, and in turn, educational achievement. Therefore, it is argued that teachers need to be aware of these prototypical profiles if they want to effectively support student learning (Huber and Seidel, 2018). Moreover, to make appropriate educational decisions and take effective actions, teachers must also be able to correctly assess the complex combinations of cognitive and motivational-affective characteristics of individual students (Praetorius et al., 2017).
Teacher Judgment Accuracy of Student Characteristic Profiles
To date, research has focused mainly on how accurately teachers judge individual student characteristics, and less on how they assess the interplay of characteristics through student profiles. It is important to note, however, that the ability to achieve the former is a necessary precondition to performing the latter (Südkamp et al., 2018). According to meta-analyses, teachers make relatively accurate judgments of students' cognitive abilities (Machts et al., 2016) and achievement (Südkamp et al., 2012). The few studies that deal with judgment accuracy of motivational-affective characteristics showed that teachers are only somewhat able to accurately assess students' self-concept (Spinath, 2005; Praetorius et al., 2013; Urhahne and Zhu, 2015) and interest (Karing, 2009). Hence, teachers seem to have more difficulties assessing student motivational-affective characteristics than cognitive characteristics (Kaiser et al., 2013; Praetorius et al., 2017). Moreover, that teachers intermingle single student characteristics, for example, when prompted to assess achievement and motivation separately, indicates that they tend to perceive students holistically (Kaiser et al., 2013). As a result of this phenomenon, it is particularly important to focus on how teachers assess student profiles that combine cognitive and motivational-affective characteristics.
So far, few studies have addressed this issue. Two studies have shown that teachers tend to underestimate the extent of inconsistent profiles among their students (Huber and Seidel, 2018; Südkamp et al., 2018). Teachers seem to assume that cognitive and motivational-affective characteristics typically go hand in hand, and subsequently categorize their students simply as being average, below-average, or above-average (Huber and Seidel, 2018; Südkamp et al., 2018). However, when teachers are explicitly asked to assign students to consistent and inconsistent profiles—when the degree of inconsistency itself is not in question—it was shown that experienced teachers are more accurate in assessing inconsistent student profiles than student teachers, although a considerable amount of variance was apparent among experienced and student teachers alike (Seidel et al., 2020). These differences in judgment accuracy might originate from differences in the preceding judgment process (Loibl et al., 2020). Therefore, to understand why some teachers achieve high judgment accuracy when assessing student profiles while others fail to do so, it is necessary to investigate in more detail the processes of judgment formation. As research has focused predominantly on teacher judgment accuracy, far less is known about the cognitive and behavioral activities that drive the judgment process itself, especially when it comes to the connection between judgment process and judgment accuracy (Herppich et al., 2018; Karst and Bonefeld, 2020; Loibl et al., 2020).
Teachers' Process for Judging Student Characteristic Profiles
Judgment processes comprise behavioral and cognitive activities (Loibl et al., 2020). Since teaching is a vision-intense profession in which it is important to gain information by monitoring what is happening in the classroom (Carter et al., 1988; Gegenfurtner, 2020), the observation of students to gain information (i.e., behavioral activity) and the interpretation of this information to make decisions (i.e., cognitive activity) are likely to be relevant activities of judgment processes. The ability to succeed in these activities is recognized as a central component of a teacher's professional competence (Blömeke et al., 2015; Santagata and Yeh, 2016), and is often labeled as professional vision (Goodwin, 1994; van Es and Sherin, 2002, 2008; Sherin and van Es, 2009; Seidel and Stürmer, 2014). In psychological research, the so-called lens model, which is based on Brunswik's (1956) paradigm that humans observe and interpret information cues to make sense of their ambiguous environment, systematizes this idea. The model is regularly considered in other fields involving judgment formation such as social sciences, business science, and medicine (Cooksey et al., 1986; Funder, 1995, 2012; Kaufmann et al., 2013; Kuncel et al., 2013). It also receives attention in the educational field (Cooksey et al., 1986, 2007; Marksteiner et al., 2012; Thiede et al., 2015; Praetorius et al., 2017).
According to the lens model, teachers are required to observe and utilize—that is combine and interpret—several student behaviors (i.e., student cues) to inform themselves about student characteristics (Cooksey et al., 1986; Nestler and Back, 2013; Thiede et al., 2015). Therefore, the manifestation of student characteristics in specific observable student cues is a precondition to judgment. Such student cues are referred to as “diagnostic” (Funder, 1995; Thiede et al., 2015) or “ecologically valid” (Cooksey et al., 1986; Nestler and Back, 2013; Back and Nestler, 2016). In other words, accurate judgments depend on the observation and use of diagnostic student cues (Nestler and Back, 2013; Förster and Böhmer, 2017). To do so, teachers require a professional knowledge base, which allows them to connect student cues to underlying student characteristics (Funder, 1995, 2012; Meschede et al., 2017). In this sense, successful judgment processes represent an applied form of professional knowledge of teachers (Jacobs et al., 2010; Stürmer et al., 2013; Kersting et al., 2016; Lachner et al., 2016). Therefore, the lens model provides a suitable framework for the investigation of teachers' behavioral and cognitive activities in the process of accurately judging latent, and not directly observable, student profiles (Nestler and Back, 2013; Förster and Böhmer, 2017; Praetorius et al., 2017; Loibl et al., 2020).
Observation of Students as a Behavioral Activity in the Judgment Process
Eye movements are an indicator for teacher observation behavior (Gegenfurtner, 2020; Loibl et al., 2020), and fall into one of two categories: saccades and fixations. Saccades are fast movements in which the eye is turned for the purposes of bringing objects of interest in front of the fovea so that they can be seen sharply. Fixations are moments when the eye is relatively still and visual information is processed (Holmqvist et al., 2011; Krauzlis et al., 2017). The location of fixations, that is the object on which one fixates, as well as the number and duration of fixations on an object, are driven by top-down and bottom-up processes through declarative knowledge (e.g., knowing where to look for relevant information) and saliency of situational features that attract attention (e.g., student movements such as hand raising behavior or visual features as bright colored clothing), respectively (DeAngelus and Pelz, 2009; Schütz et al., 2011; Gegenfurtner, 2020).
Eye tracking—which measures where one is looking—is a relatively new method in educational science (Jarodzka et al., 2017). Nevertheless, some studies have already provided initial evidence concerning teachers' observation behavior. This evidence comes primarily in the form of comparisons between experienced and student teachers in the context of professional vision (Stürmer et al., 2017; Wyss et al., 2020), classroom management (van den Bogert et al., 2014; Cortina et al., 2015; Wolff et al., 2016), and teacher-student interactions (McIntyre et al., 2017, 2019; McIntyre and Foulsham, 2018; Haataja et al., 2019, 2020; Seidel et al., 2020). Overall, in comparison with student teachers, experienced teachers seem to show a more knowledge-driven pattern of eye movement, which represents selective viewing and fast information processing. Experienced teachers also focus more on areas that are rich in information and pay more attention to students than to other things in the classroom. Moreover, experienced teachers continuously monitor the classroom as a whole even if they are in the process of recognizing relevant events or interacting with individual students (van den Bogert et al., 2014; Cortina et al., 2015; Wolff et al., 2016; McIntyre et al., 2017, 2019; McIntyre and Foulsham, 2018; Wyss et al., 2020). Therefore, experienced teachers show a pattern of monitoring relevant areas with more fixations but shorter fixation durations (van den Bogert et al., 2014; Seidel et al., 2020), similar to experts in other domains (Gegenfurtner et al., 2011). So far, only one study has connected teachers' judgment accuracy with eye movements. Hörstermann et al. (2017) investigated primacy effects concerning the location of information cues in case vignettes for students' social background and performance. It was found that student teachers paid most attention to the information presented at the top left of the case vignettes. The type of information presented in this location, whether related to students' social background or performance, did not bias the accuracy of decisions concerning school track. The available research on teacher eye movement suggests that it can be an appropriate method for gaining additional information about teacher judgment processes. Therefore, it can be used to study, for example, whether teachers, who formed accurate student judgments as the result of a judgment process, also showed an “experienced” pattern of eye movement such as faster information processing, indicating a top-down driven process of advanced knowledge organization.
Utilization of Student Cues as a Cognitive Activity in the Judgment Process
In terms of accurately judging student profiles, teachers are required to assess student characteristics and their intra-individual consistency. In this case, several diagnostic student cues that point toward the level of cognitive and motivational-affective characteristics need to be used in combination. In particular, the intensity and content of engagement can be considered as relevant diagnostic student cues (see section Student engagement reflects student characteristic profiles for the connection of student profiles and student engagement). With intensity of student engagement, we refer to its level of presence. With regard to behavioral aspects, for example, rare hand-raising behavior represents lower intensity of engagement, while frequent hand-raising behavior represents higher intensity of engagement. By content of student engagement we refer to the level of knowledge and understanding that becomes apparent through student engagement. For example, when engaging verbally in teacher-student interactions, correctness of an answer or use of technical language represent the content of student engagement. To distinguish, for example, students with strong and overestimating profiles (Seidel, 2006) frequent hand-raising behavior (intensity of engagement) might be a diagnostic cue for a high level of self-concept (Böheim et al., 2020; Schnitzler et al., 2020), whereas incorrect answers (content of engagement) point toward low knowledge, and correct answers (content of engagement) indicate high knowledge (Thiede et al., 2015). Consequently, only if teachers utilize combinations of diagnostic student cues containing information about cognitive and motivational-affective characteristics, they may infer the correct student profile. Otherwise, profiles that share a similar level of cognitive (e.g., strong and underestimating) or motivational-affective characteristics (e.g., strong and overestimating) might be interchanged.
Empirical findings regarding the question of how well teachers are able to utilize diagnostic student cues are limited. In general, experienced teachers are much better able to interpret relevant classroom events than student teachers and beginning teachers (Sabers et al., 1991; Berliner, 2001; Star and Strickland, 2008; Meschede et al., 2017; Kim and Klassen, 2018; Keppens et al., 2019). This is due to an encapsulated knowledge structure along cognitive schemata which results from the integration of practical experiences with declarative knowledge (see for a current review on expertise development in domains that focus on diagnosing Boshuizen et al., 2020), allowing for fast information processing (Carter et al., 1988; Berliner, 2001; Kersting et al., 2016; Lachner et al., 2016; Kim and Klassen, 2018). However, differences in the abilities to interpret classroom events already appear among student teachers who had only limited opportunities to engage in teaching practice (Stürmer et al., 2016). When it comes to the explicit consideration of judgment processes, teachers seem to consider student background characteristics such as gender, ethnicity, immigration status, and socioeconomic status (SES) to assess students' cognitive and motivational-affective characteristics (Meissel et al., 2017; Praetorius et al., 2017; Garcia et al., 2019; Brandmiller et al., 2020). Moreover, teachers seem to rely on these rather unimportant and misleading student cues especially when they experience low accountability for their decisions (Glock et al., 2012; Krolak-Schwerdt et al., 2013). Additionally, student teachers tend to utilize as many student cues as available, irrespective of whether they are diagnostic or unimportant, while experienced teachers seem to do so only if available cues are inconsistent (Glock et al., 2013; Böhmer et al., 2015, 2017).
Only a small number of studies have investigated teachers' use of cues in connection with their judgment accuracy. For example, beginning teachers seem to be aware of diagnostic cues for detecting whether someone is telling the truth or lying when observing videos. However, cues were utilized in a way that led to inaccurate judgments (Marksteiner et al., 2012). Another study investigated the effect of the availability of different cues (only students' names; students' name and answers on practice tasks; and only students' answers on practice tasks) on the type of information used to assess students' performance on a set of mathematical tasks. Teachers were most accurate in assessing low performance if they knew only students' answers on the practice tasks because under this condition they used more answer-related, diagnostic information (Oudman et al., 2018). When analyzing which cues experienced and student teachers utilize to assess student profiles from video observation, prior findings indicate that these two groups do not differ in the number of student cues utilized. However, experts seem to use a broader range of cues, while student teachers tended to focus more on rather salient student cues, such as frequency of hand-raising (Seidel et al., 2020).
Based on the studies summarized above, it still remains quite unclear which student cues, diagnostic or unimportant, and which student cue combinations are utilized by teachers in everyday teaching to assess cognitive and motivational characteristics, not to mention their combination in student profiles (Glock et al., 2013; Praetorius et al., 2017; Huber and Seidel, 2018; Brandmiller et al., 2020). Furthermore, a link between judgment accuracy and judgment process remains to be established. For example, how do teachers, who succeed in assessing student profiles, differ from those who have difficulty doing so? Do they utilize student cues in a different way?
The Present Study
Against this background, the present study aimed to expand research on the connection between judgment accuracy and judgment process. Furthermore, we considered student diversity in terms of previously identified consistent and inconsistent student profiles as identified by Seidel (2006), and took into account observation of students and utilization of student cues as behavioral and cognitive activities, respectively, as drivers of judgment processes. In addition, we focused on student teachers and the differences previously determined to exist among this group. We addressed the following three research questions:
RQ1 concerning judgment accuracy:
a) How accurately can student teachers judge student profiles?
b) How does student teachers' judgment accuracy differ across student profiles?
c) Which student profiles do student teachers interchange predominantly?
Considering previous findings, we assumed that some student teachers would display high judgment accuracy while others would struggle to assign student characteristic profiles. We expected student teachers to assess consistent profiles with a high judgment accuracy and inconsistent profiles with a lower accuracy due to previous findings, which reported that teachers systematically underestimate the level of diversity among their students. Moreover, we assumed that they would interchange profiles that share the same level of cognitive characteristics but that differ in their motivational-affective characteristics, since teachers were previously found to be better able to assess cognitive characteristics with a higher accuracy.
To deepen our understanding on the interdependence of judgment accuracy and judgment processes we considered two process indicators—behavioral and cognitive activities.
RQ2 concerning observation of students as a behavioral activity:
Across different student profiles, what differences indicate high and low judgment accuracy in student teachers' eye movements
a) with regard to the number of fixations?
b) with regard to the average fixation duration?
We expected that student teachers with a higher judgment accuracy would show a pattern of a higher number of fixations with shorter average duration than those with low judgment accuracy.
RQ3 concerning utilization of student cues as a cognitive activity:
a) Which student cues do student teachers utilize to assess student cognitive and motivational-affective characteristics (student profiles)?
b) What combinations of student cues do student teachers with high and low judgment accuracy use to assess student cognitive and motivational-affective characteristics (student profiles)?
This research question was explorative, given its novelty. Nevertheless, we expected that student teachers with a high judgment accuracy would utilize combinations of diagnostic student cues that reflect both student cognitive and motivational-affective characteristics and point particularly to different student profiles.
Methods
Participants
Forty-three student teachers (MAge = 21. 59; SD = 1.60; 62.8% female) participated in our study during their fourth semester of a bachelor's teacher training program at the Technical University of Munich. All participants enrolled in a program to become teachers in German high-track secondary schools for science and/or mathematics. We invited student teachers to participate in the study during one of their pedagogical courses. Participants received a 20 Euro voucher.
Procedure
The present study was conducted in line with the Ethical Principles of Psychologists and Code of Conduct of the American Psychological Association from 2017 (APA American Psychological Association, 2017). Participants have been assured that their data will be used in accordance with the data protection guidelines and analyzed for scientific purposes only. They gave informed consent before participation.
Data collection took place in the university laboratory. At the study's outset, participants were familiarized with previously identified student profiles (Seidel, 2006): individual student cognitive and motivational-affective characteristics, as well as their interplay in the form of profiles (strong, struggling, overestimating, underestimating, and uninterested), were illustrated. This included descriptions of each student characteristic avoiding student cues that might be observable in classrooms. Next, to make participants familiar with the classroom environment and the lesson topic, they watched a short video trailer (2:30 min) showing the class in question.
Participants then encountered the assessment situation of this study. We instructed participants to assess the profiles of five target students shown in an 11-min video. Each target student was marked with a random letter (B, E, K, P, T), so that participants were always aware of them (Figure 1). While participants watched the video, eye tracking was conducted. Finally, participants were asked to assign a profile to each target student. In doing so, they were also asked to voluntarily indicate, in an open answer format, the student cues they had utilized for their assessment. Each student profile could only be assigned once.
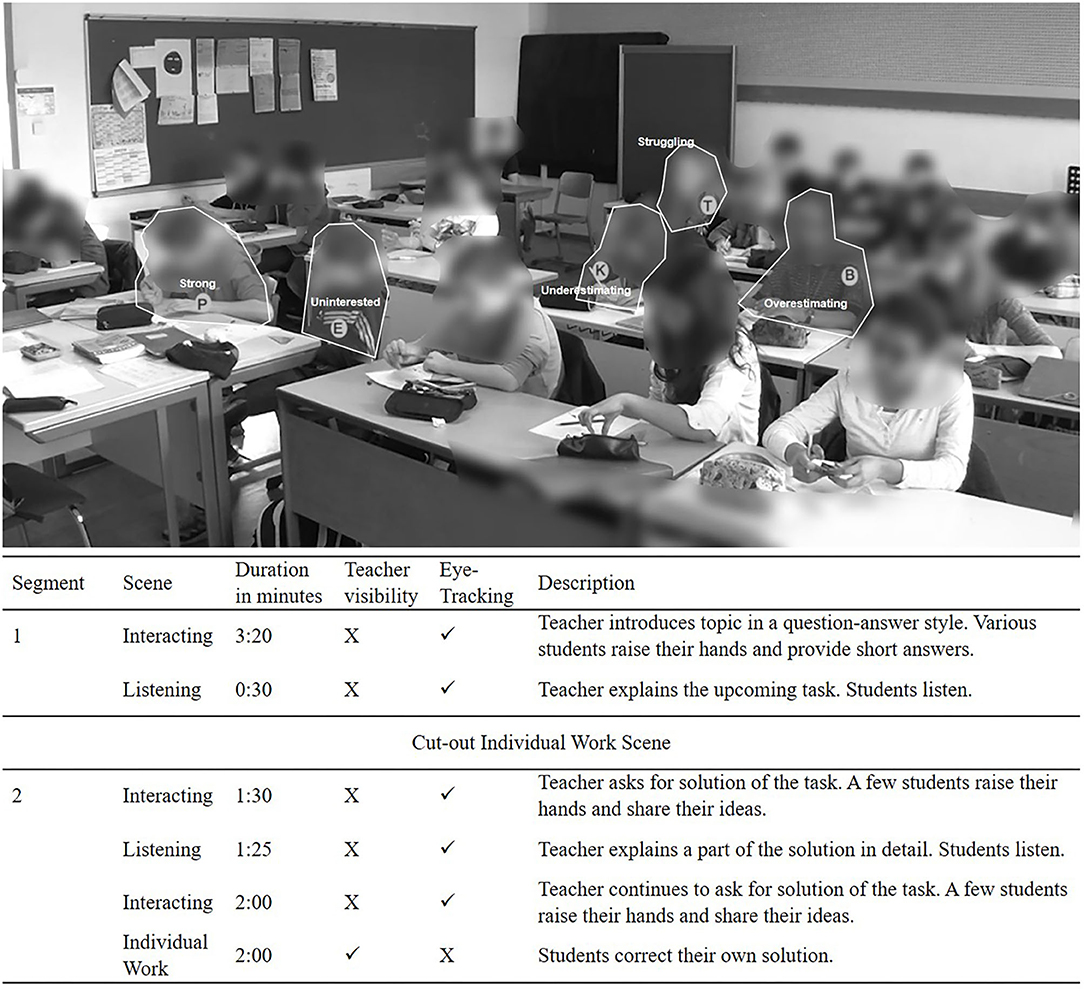
Figure 1. Video stimulus used for eye-tracking analysis. This is an exemplary screenshot of the classroom and areas of interest (AOI) used. AOIs are only marked for the purposes of illustration in this paper, and were not visible to participants. Faces are also blurred for presentation in the publication to ensure the protection of data privacy; faces were visible to participants during the study and when drawing the AOIs. Students were marked with letters that did not refer to any underlying profile: B, E, K, P, and T. This figure was previously published as “Video stimulus for eye movement analysis” by Seidel et al. (2020) and is licensed under CC BY 4.0. The original figure was changed by adding the Table in the lower part.
Video Stimulus
The video presented during the assessment stemmed from a previous study on teacher–student interactions in classrooms (Seidel et al., 2016), and showed an eighth grade mathematics lesson in a German high-track secondary school in which a new topic was being introduced. The video consisted of two segments. The first segment showed a teacher describing a task to be accomplished in a subsequent individual work phase. The second segment showed students sharing their results with the teacher after the individual work phase. The individual work itself was not included in the video stimulus; instead, a short text informed participants when students were working on the individual task. Both segments comprised several scenes of students listening to their teacher's lecture, and of students interacting with their teacher in a classroom dialogue. Details about the video segments and scenes are provided in Figure 1.
Each target student represented one student profile (strong, struggling, overestimating, underestimating, and uninterested). The students' profiles were empirically determined in a previous video study from which the video stemmed (Seidel et al., 2016). We carefully selected the five target students to best represent a particular student profile with regard to observable student cues. To achieve this, three researchers involved in the present study ranked the students independently in terms of their representation of the identified student profile.
Apparatus
We recorded participants' eye movements with the SMI RED 500 binocular remote eye tracker using Experiment Center software version 3.7 (SMI, 2017b) on a 22-inch display monitor and at a sampling frequency of 500 Hz. Eye tracking conditions were standardized for all participants. Light conditions were kept stable by closing the window blinds and using ceiling lighting. Participants were positioned 65 cm in front of the eye tracker. To increase the precision of eye tracking, a height-adjustable table was used in combination with a chin rest to ensure that the equipment was adjusted to each individual participant, and prevented them from performing strong (head) movements (Nyström et al., 2013). Moreover, before beginning eye tracking, a 9-point automatic calibration followed by a validation was implemented to ensure data quality.
Measurements
Judgment Accuracy
To measure judgment accuracy, participants received one accuracy point if the assigned profile matched the underlying data-driven profile, or no point (wrong profile assigned) for each of the five target students. Moreover, the points for each profile were added up across all profiles. The participants could therefore receive between 0 and 5 points overall. Since each profile could only be assigned once, when four correct assignments were made, the fifth profile would result from exclusion, and the overall judgment accuracy score was recoded to range from 0 (no correct assignment) to 4 (only correct assignments).
Student Observation
To assess teachers' observation behavior we used eye movement data. To ensure high quality of these data, we set two thresholds. First, the tracking ratio had to be at least 90%. Second, the deviations on the horizontal x-axis and vertical y-axis during validation of the calibration process were not allowed to exceed 1° (Holmqvist et al., 2011). Due to these quality criteria, eye movement data were processed for n = 32 (74.4%) of the participants with an average tracking ratio of 96% and average deviations on the x-axis = 0.49° and y-axis = 0.56°. We defined each of the five target students as one dynamic area of interest (AOI) using the BeGaze software version 3.7 (SMI, 2017a) to capture eye movement related to each (Figure 1). The exactness of the drawn AOIs was ensured throughout the whole video by making manual adjustments to the AOIs whenever needed, for example when a student leaned over toward their neighbor. To identify fixations in participants' eye movements, we used the default velocity-based algorithm as recommended by Holmqvist et al. (2011) and implemented in previous eye tracking studies (Wolff et al., 2016). Thus, the fixation count (i.e., number of fixations on one AOI) and the average fixation duration (i.e., the average length of one fixation within one AOI) were assessed in relation to each of the target students.
Student Cue Utilization
To assess the student cues utilized, we coded participants' open answers to the question of which cues they had observed and utilized to assess student characteristics profiles. This question was asked separately for each target student. Thus, participants could provide between zero and five answers because answering this question for every target student was voluntary. Nevertheless, the majority of participants (n = 27, 62.8%) had indicated cues for at least one student profile, resulting in 124 answers. These answers were equally distributed across the five profiles. Most answers were provided as lists of single student cues separated by semicolons or bullet points. Therefore, we chose these single student cues as units of analysis for coding, resulting in 376 units. Two researchers coded these units inductively, resulting in a final coding scheme of 26 single codes (Table 1) for which they reached a high interrater agreement (κ = 0.93 for segment comparison with a minimum code intersection rate of 95% at the segment level). Based on research on student engagement (Fredricks et al., 2004, 2016a,b; Appleton et al., 2008; Rimm-Kaufman et al., 2015; Sinatra et al., 2015; Lam et al., 2016; Chi et al., 2018; Böheim et al., 2020), we then clustered the single codes in five categories, namely (1) behavioral, (2) cognitive, and (3) emotional engagement, pointing toward the intensity of student engagement; (4) knowledge, which represents the content of student engagement; and (5) student confidence.
Data Analysis
Judgment Accuracy
To investigate student teachers' judgment accuracy, we applied a mixture of descriptive and non-parametric testing, first by visually inspecting the distribution of judgment accuracy scores. Second, a Friedman test for repeated measures with Dunn-Bonferroni post-hoc comparisons corrected for multiple comparisons was calculated to examine whether participants differed in their judgment accuracy among student profiles. This non-parametric procedure was chosen because accuracy scores on profile level could only take on the values of zero (incorrect assessment) and one (correct assessment). Thus, they deviated strongly from a normal distribution. Finally, we descriptively investigated which profiles student teachers interchanged most frequently with one another to determine which profiles were difficult to distinguish.
Observation of Students
To investigate whether student teachers with low and high judgment accuracy differed in fixation count and average fixation duration, we summed up the fixation counts and averaged the fixation durations for each target student. To investigate the effects of high vs. low judgment accuracy on these variables, we split the whole sample along the median of the overall accuracy score and identified the two subgroups accordingly (Iacobucci et al., 2015a,b). This resulted in a group of n = 21 with low judgment accuracy (Mjudgment accuracy = 1.14, SD = 0.73) and a group of n = 22 with high judgment accuracy (Mjudgment accuracy = 3.27, SD = 0.46). These two subgroups differed significantly from one another in their judgment accuracy [T(33.35) = −11.45, p < 0.001]. High-quality eye tracking data were available for n = 17 low accuracy and n = 15 high accuracy student teachers. We calculated a series of unpaired t-tests to compare the number of fixations and the average fixation duration between student teachers with low and high judgment accuracy for each student profile (see Table 2 for intercorrelations). Here, we used Bonferroni adjusted p-values for multiple testing to consider alpha error accumulation.
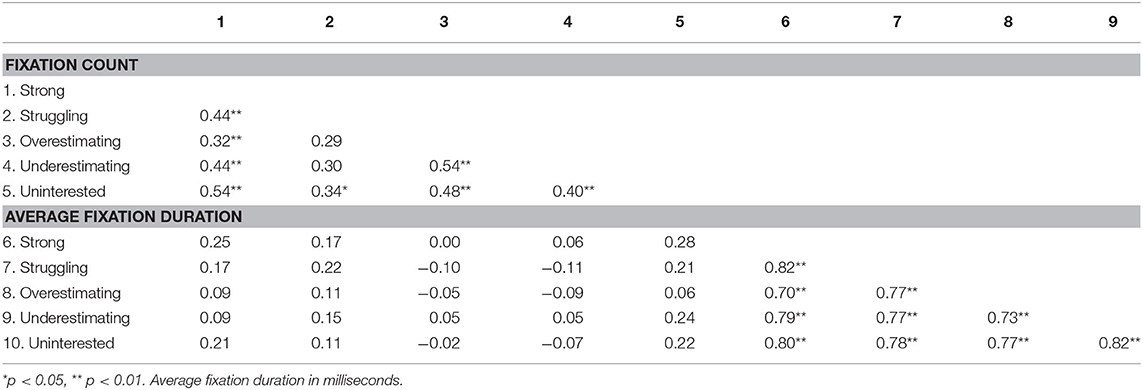
Table 2. Intercorrelations for fixation count and average fixation duration across student profiles.
Student Cue Utilization
To identify which student cues participants used to assess student profiles, we inspected the relative frequencies of the inductively derived codes.
To investigate differences in cue utilization among student teachers with either high or low judgment accuracy, we compared whether the two groups used different combinations of student cues to infer student profiles. To achieve this, we again compared the median split subgroups. Of these, n = 11 student teachers with low judgment accuracy and n = 16 student teachers with a high judgment accuracy had provided at least one answer to the question of which student cues they had used to assess a specific student profile. To investigate how both groups of student teachers used cue combinations in this context, we applied an epistemic network analysis (ENA, Shaffer et al., 2009, 2016; Shaffer and Ruis, 2017; Csanadi et al., 2018; Wooldridge et al., 2018) using ENA Web Tool version 1.7.0 (Marquart et al., 2018). ENA is a graph-based analysis that allows for modeling of the structure and strength of co-occurrences of a relatively small number of elements in a network (see for examples of this kind of analysis Andrist et al., 2015; Csanadi et al., 2018; Wooldridge et al., 2018). In general, the size of network nodes corresponds to the importance of that element in the network, and the strength of the connection between two elements represents the frequency of their combination (see Figure 2 for prototypical networks). Thus, ENA allowed us first, to investigate which single student cues co-occurred within answers to the question of which cues had been utilized, and second, whether teachers with low and high judgment accuracy differed in the way they combined student cues to assess student characteristics profiles.
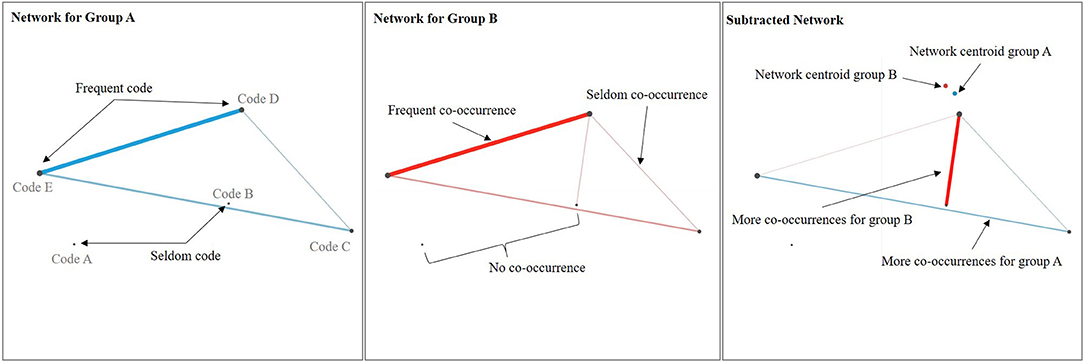
Figure 2. Prototypical networks from epistemic network analysis. These are prototypical networks for two groups, A (blue) and B (red). Each node of the network represents a code. The size of the nodes represents the occurrence of single codes. The bigger a node, the more often the respective code appeared. The thickness of the connections between the nodes represents the co-occurrences of codes. The thicker the connecting line, the more often the respective codes co-occurred. If codes did not co-occur, they remain unconnected. A subtracted network illustrates the comparison between networks A and B. The color and strength of the connecting lines indicate for which group specific codes co-occurred more often. A network centroid summarizes the structure of a network in one point.
ENA is based on three core entities. The first entity consists of the codes for which one wants to investigate co-occurrences. In this study, predominantly reported student cues were used as codes. Therefore, we included cues that accounted for more than 3% of all codings (see Table 1 for the included cues). The second entity refers to the unit of analysis, which defines the object of ENA, for which we used judgment accuracy (low vs. high) and student profile (strong, struggling, overestimating, underestimating, and uninterested). Hence, within each group of low and high judgment accuracy, one network per student profile was constructed. Third, stanza determines the proximity that codes must have to one another in order to be considered as co-occurring. In our case, each of the 124 answers to the question of which cues student teachers had utilized to assess the profile of student B/E/K/P/T were defined as stanzas. Hence, ENA took only code co-occurrence within single answers into account. For example, to create a network for the strong profile within the group with a high judgment accuracy, the only cues used were those coded for that particular group's responses when asked which cues they had used to assign a profile to student “P.”
To create networks, one adjacency matrix was created for each stanza, indicating whether each of all possible code combinations was present or absent in a particular stanza. In our case, for every answer, one matrix was constructed to indicate whether combinations of student cues were present or absent. These adjacency matrixes were then accumulated for each unit, representing the number of stanzas for which each student cue combination was present. Each cumulative adjacency matrix was then converted into an adjacency vector. Thus, a high-dimensional space is created in which each dimension represents a specific combination of student cues. These vectors may vary in their length, because for some student profiles more or fewer answers were available than for others. To account for these differences, the adjacency vectors were spherically normalized to represent relative frequencies of student cue combinations. Next, the high-dimensional space was reduced to a low-dimensional projected space via mean rotation—to maximize the differences between the two groups of student teachers with low and high judgment accuracy—and singular value decomposition (SVD), a method similar to principal component analysis that reduces the number of dimensions to those that explain most variance in the data. ENA represents each network in the low-dimensional projected space both by a single point, which locates that unit's network centroid (a summary of the structure of its connections), and a weighted network graph. To visualize the network graphs, we chose mean rotation as the x-axis and the singular value that explains most of the variance in the data as the y-axis. The network graphs were then visualized using nodes and edges. Nodes correspond to the student cues. Their position is fixed due to an optimization routine that minimizes deviations between the plotted points and the respective network centroids. A correlation was estimated for the relation between the centroids and the projected points as a measure of model fit. Edges represent the relative frequency of co-occurrences of the cues (Andrist et al., 2015; Shaffer et al., 2016; Shaffer and Ruis, 2017).
These ENA characteristics allow quantitative and qualitative comparisons of networks for single participants or groups, as is the case in our study. Specifically, we compared the location of the network centroids for the two groups of low and high judgment accuracy student teachers with t-tests along the x- and y- axes and inspected so-called subtracted networks (see Figure 2 for a prototypical subtracted network). Subtracted networks visualize the differences between two networks and compare which code co-occurrences were more frequent in which network. The edges are color-coded accordingly and indicate in which group the code combination occurred more frequently. This visualization allows a qualitative comparison of code co-occurences between our two groups but does not test whether these differences are significant.
Results
Judgment Accuracy
To investigate student teachers' judgment accuracy the distribution of student teachers' overall judgment accuracy score is depicted in Figure 3. Student teachers had an average judgment accuracy score of 2.23 (SD = 1.23) across all profiles. This distribution indicates that student teachers differ substantially in their judgment accuracy. Roughly one half of the participants assessed three or five student profiles correctly and gained an accuracy score of three or four points, while the other half showed difficulties in accurately assigning student profiles.
A significant Friedman test [χ2(4) = 25.53, p < 0.001] indicated that student teachers' judgment accuracy differed among student profiles. As shown in Figure 4, the following descending order was identified for average judgment accuracy at profile level: Uninterested, struggling, underestimating, strong, and overestimating. Results of the post-hoc tests (Table 3) suggest that student teachers tended to assign students to the uninterested (M = 0.67, SD = 0.47) and the struggling profile (M = 0.65, SD = 0.48) with a similarly high accuracy. Moreover, these profiles were clearly judged more accurately than the underestimating (M = 0.40, SD = 0.49), the strong (M = 0.35, SD = 0.48), and the overestimating (M = 0.30, SD = 0.46) ones. However, none of these differences reached significance when correcting for multiple comparisons.
Regarding student teachers' difficulties in distinguishing students with certain profiles from one another, our descriptive analysis yielded three main findings (Figure 5). First, the strong and overestimating profiles were most often “mixed up,” or interchanged. The strong profile was even more often assigned to the overestimating student (65.1%) than to the strong one (34.9%). Similarly, the overestimating profile was more often assigned to the strong student (51.2%) than to the overestimating one (30.2%). Second, the struggling and the underestimating profiles were also frequently interchanged. Of the participants, 20.9% assigned the underestimating profile to the struggling student and 32.6% assigned the struggling profile to the underestimating student. Third, the profiles of lower motivational-affective characteristics—struggling, underestimating, and uninterested—were also sometimes confused. These findings suggest that motivational-affective characteristics seem to outshine cognitive characteristics; profiles with similar motivational-affective characteristics were frequently interchanged with one another (for example strong and overestimating) while profiles with different motivational-affective characteristics were rather clearly distinguished (for example strong and struggling).
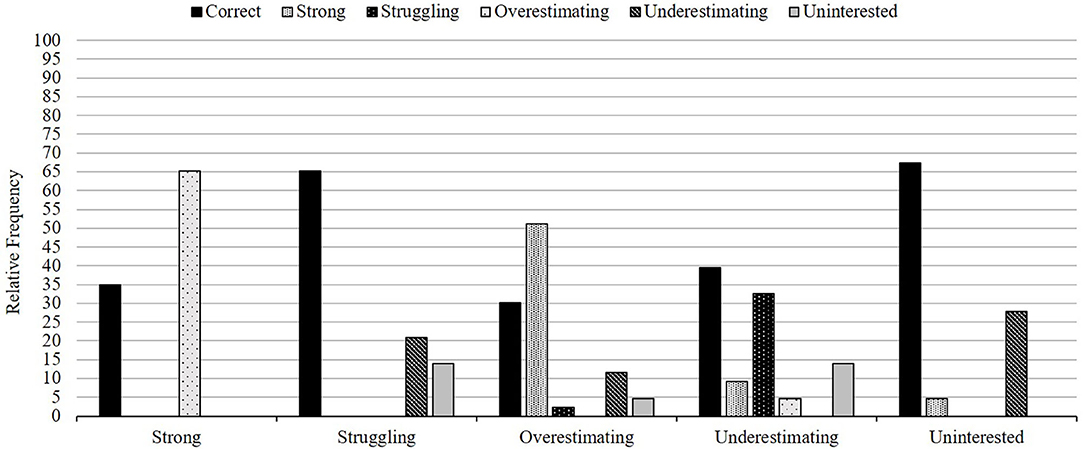
Figure 5. Relative frequencies of correct assessments (black columns) and interchanged student profiles (gray shaded columns).
Differences in Eye Movements
Descriptive statistics for student teachers' fixation counts and average fixation durations, as well as t-test results regarding differences in these variables between high and low judgment accuracy, are presented in Table 4. When comparing means for both groups descriptively, student teachers with a high judgment accuracy displayed the anticipated pattern. They had higher fixation counts on each student besides the struggling one, and showed shorter average fixation durations on each of the target students than student teachers with low judgment accuracy. T-tests indicated that student teachers with high judgment accuracy had more fixations on the overestimating [t(30) = −2.72, p = 0.011] student and showed shorter average fixation durations for the strong [t(30) = 2.21, p = 0.035] and underestimating student [t(30) = 2.52, p = 0.017]. However, when adjusting for multiple comparisons, these differences were no longer significant. According to this, there are only minimal differences in the expected direction between the two groups that exist on a purely descriptive level and inferential statistics do not support our assumptions.
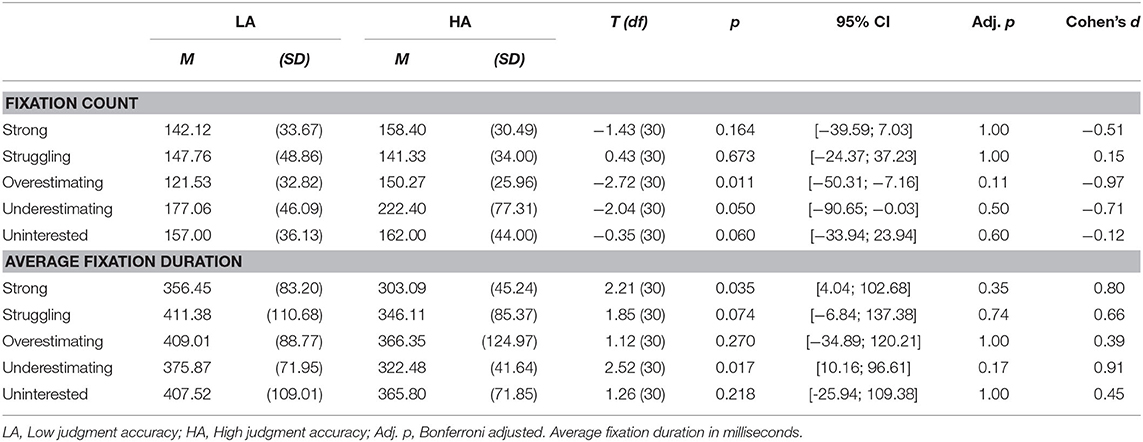
Table 4. T-test comparisons for fixation count and average fixation duration for student teachers with high and low judgment accuracy.
Differences in Student Cue Utilization
Student teachers reported a variety of cues, referring to the intensity of student behavioral, cognitive, and emotional engagement (18 cues in total), as well as to level of knowledge as the content of student engagement (6 cues in total), and to confidence (2 cues; Table 1). Thus, student teachers reported the use of more diverse cues concerning intensity of engagement than content of engagement. Relative frequencies demonstrated predominantly used cues were observations of general class participation, hand-raising behavior, preoccupation with things other than the lecture, and inattention, as well as the quality of verbal contributions, general understanding of the subject matter, and lack of confidence. Therefore, student teachers seemed to focus on diagnostic student cues when assigning student profiles.
For the epistemic network analysis, Table 5 gives a descriptive overview on the usage of the different student cues between participants with a low and high judgment accuracy across the five student profiles.
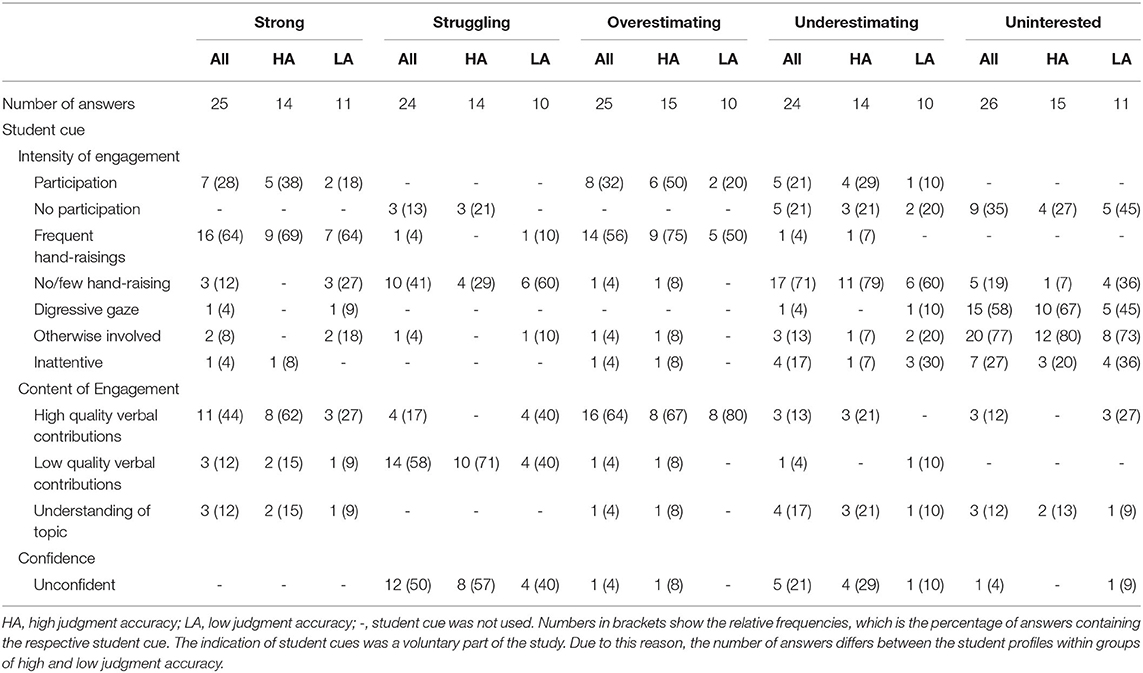
Table 5. Absolut and relative frequencies of utilized student cues for student teachers with high and low judgment accuracy across student profiles.
Our epistemic network model had a good fit with the data with Spearman and Pearson correlation being equal to 1.00 both for our x-axis and y-axis. Figure 6 presents a comparison of the networks between student teachers with high and low judgment accuracy. It depicts the network centroids for both groups as squares and for each profile as points. The closer the centroids are located to one another, the more similar the network structures. In our case, the centroids of student profiles that were often interchanged are located more closely to one another than those that were not frequently interchanged. Specifically the centroids of the struggling, underestimating, and uninterested profiles are in local proximity, as are those of the strong and overestimating profiles, indicating that interchanges between student profiles are reflected in similar network structures. For the comparison of network centroids of student teachers with low and high judgment accuracy, we found significant differences along the x-axis [t(5.21) = −3.60, p = 0.01, d = 2.28]. Deviation on the y-axis was non-significant [t(8.00) = 0.00, p = 1.00, d = 0.00]. Thus, in general, the networks for both groups differed from one another.
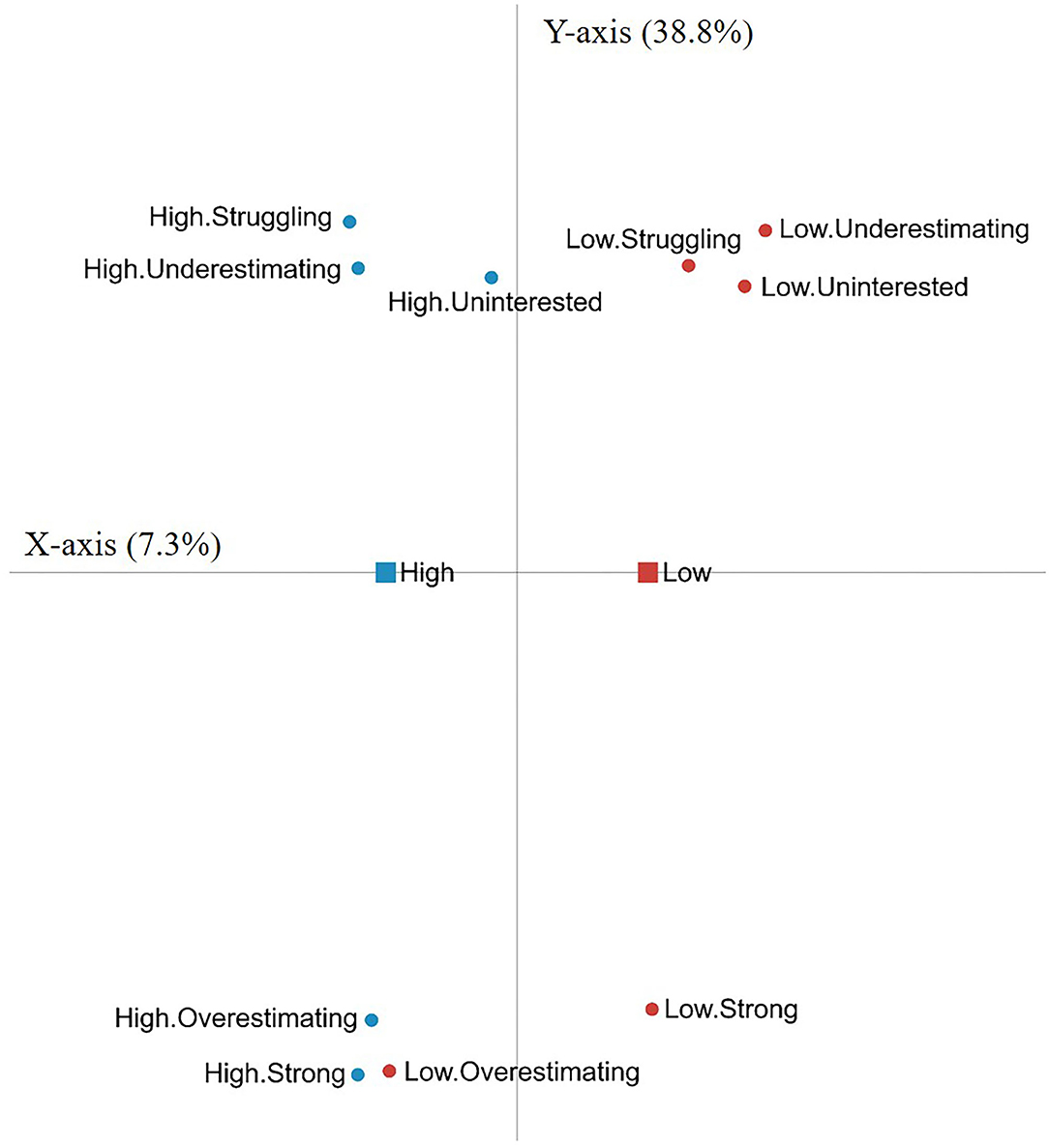
Figure 6. Comparison of mean networks between student teachers with high and low judgment accuracy. High, High judgment accuracy (blue); Low, Low judgment accuracy (red). The figure presents the location of the centroids of the mean networks for HA and LA student teachers as squares. Centroids of the networks for each student profile are presented as points. The closer the centroids are located to one another, the more similar the network structures. The X-axis represents mean rotation, and the Y-axis represents the singular value that explains most of the variance in the data. Explained variance on the X-axis and Y-axis are 7.3 and 38.8%, respectively.
The qualitative inspection of subtracted networks for each student profile, however, provided a detailed picture of how both groups differed in their use of combinations of student cues. Networks from student teachers with low and high judgment accuracy for all student profiles are shown in Figures 7–11. Each of these figures presents networks for high judgment accuracy participants in part (a), networks for low judgment accuracy participants in part (b), and subtracted networks for group comparison in part (c). For the purposes of our analyses, we first described for each student profile the dominant pattern of student cues for participants with low and high judgment accuracy and make relations of the student cues to underlying motivational and cognitive characteristics. Second, we inspected the subtracted networks to identify differences in the utilization of student cues, meaning differences in which student cues were reported in combination with one another, between student teachers with high and low judgment accuracy.
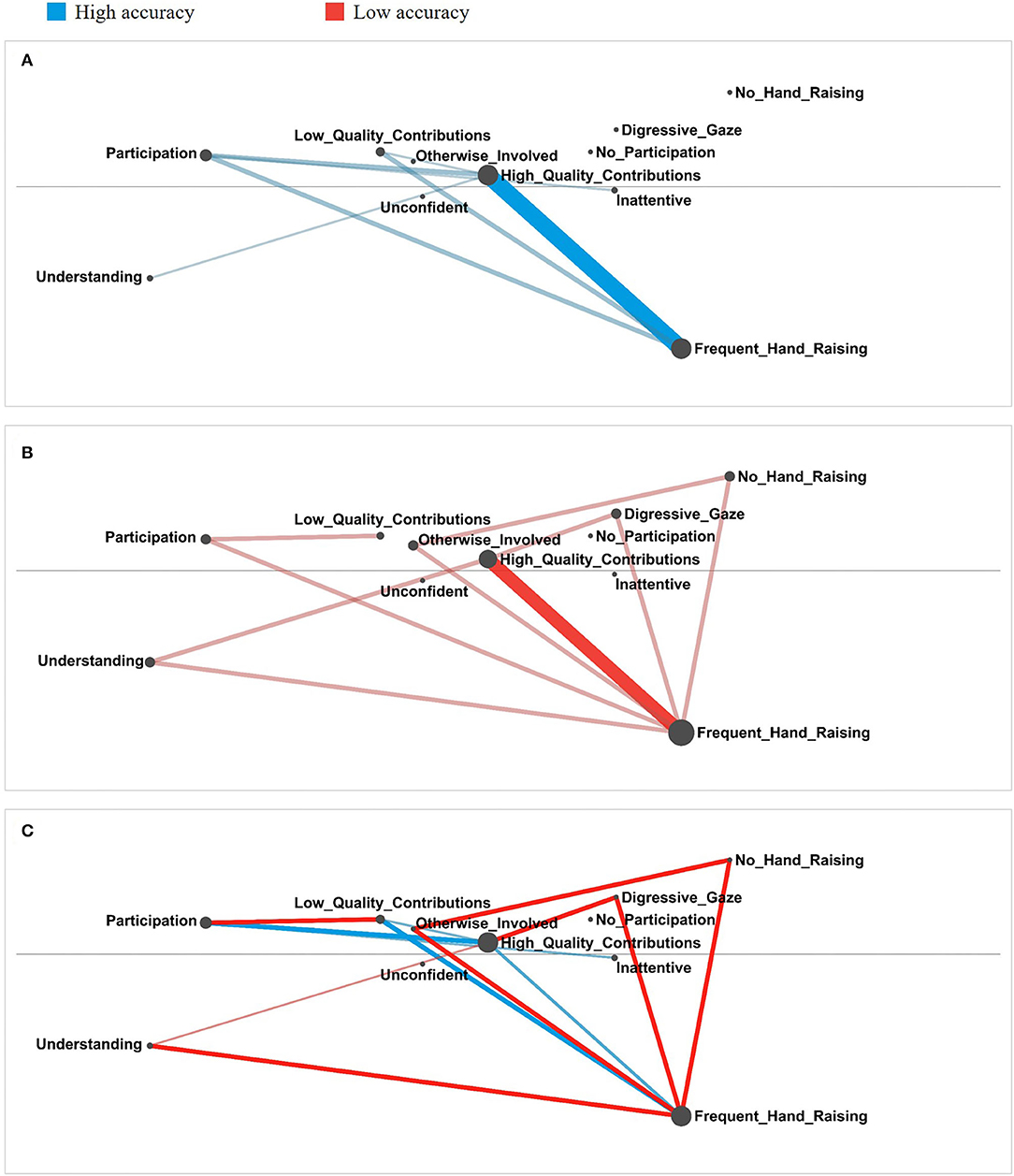
Figure 7. Comparison of networks for strong student profile between student teachers with high and low judgment accuracy. (A) Depicts the network of the strong student profile for student teachers with high accuracy. (B) Depicts the network of the strong student profile for student teachers with low accuracy. (C) Shows the comparison of both networks. Here, the color of connections indicates which group utilized the respective code combination more frequently. In order to make the characteristics of the individual networks clearly visible and to make the differences visually easier to recognize, minimum edge weight and scale for edge weight were set to 0.1 and 1.3, respectively.
Networks for the strong student profile are shown in Figure 7. Both, student teachers with high and low judgment accuracy, focused heavily on a combination of two student cues to diagnose this profile—frequent hand raisings (intensity of engagement) and high quality of answers (content of engagement). As shown in the subtracted network [part (c) in Figure 7], the group of student teachers with a low judgment accuracy differed in that they also reported many other combinations of student cues of which some contradicted the strong student profile (e.g., no hand raisings and preoccupation with things other than the lecture). Hence, high accuracy student teachers seem to use predominantly combinations of student cues which are clearly pointing to a strong profile while low accuracy student teachers indicated many different cue combinations that did not clearly refer to the strong profile.
Regarding the struggling student profile (see Figure 8), both groups used a pattern of three student cues for their judgment—an unconfident appearance combined with the avoidance of hand-raisings (intensity of engagement) and low quality of verbal contributions (content of engagement). Differences in these patterns are shown in part (c) in Figure 8. Those with a high judgment accuracy took also into account that the student showed a low level of general participation in the learning activities while those with a low judgment accuracy seem to rate the quality of the verbal contributions as high using this as a cue for their judgment. Thus, student teachers with a high judgment accuracy utilized combinations that unambiguously indicate a struggling student profile whereas those with a low judgment accuracy may have made a false assessment by perceiving the answers as being of high quality which might not be indicative of a struggling profile.
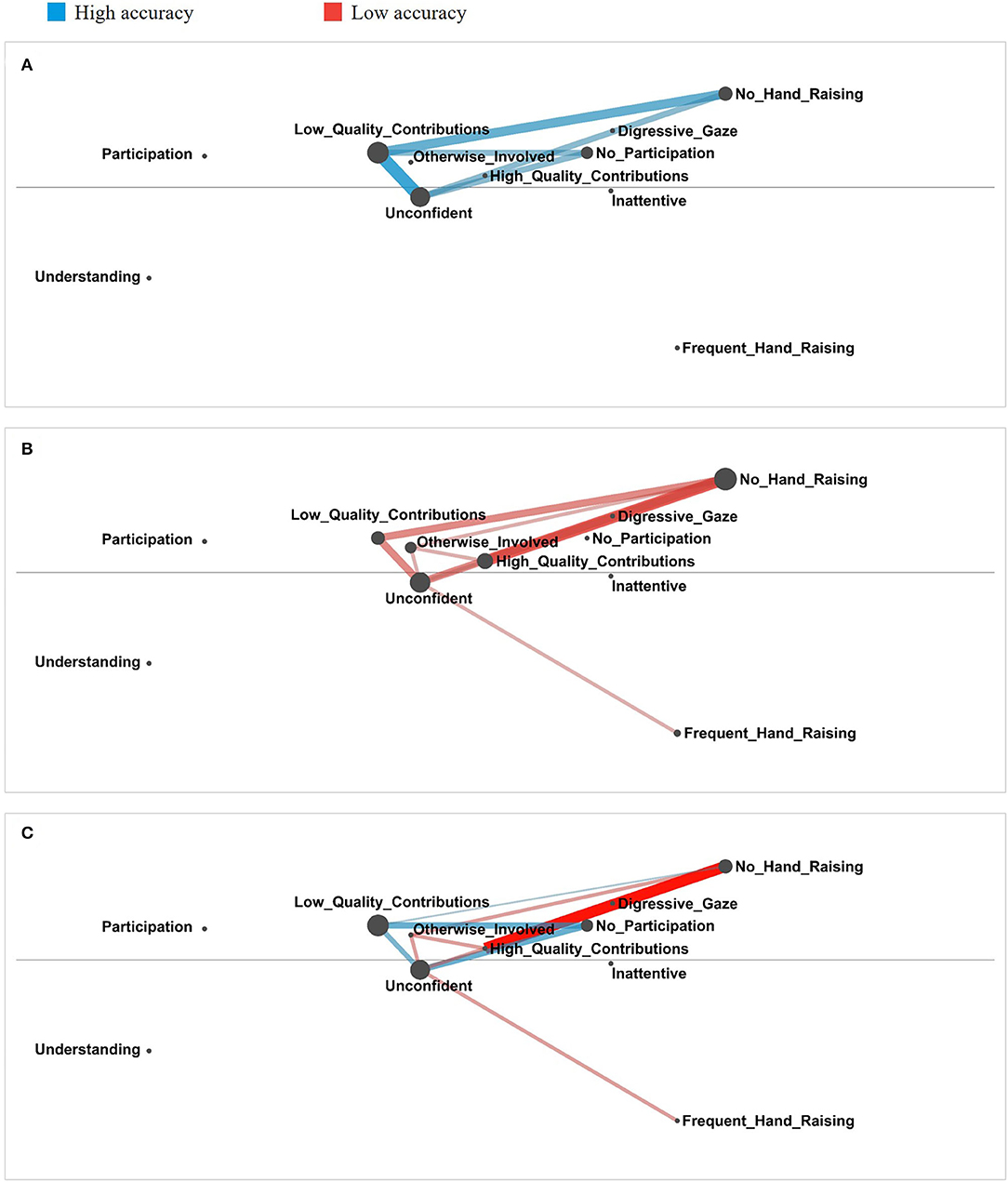
Figure 8. Comparison of networks for struggling student profile between student teachers with high and low judgment accuracy. (A) Depicts the network of the struggling student profile for student teachers with high accuracy. (B) Depicts the network of the struggling student profile for student teachers with low accuracy. (C) Shows the comparison of both networks. Here, the color of connections indicates which group utilized the respective code combination more frequently. In order to make the characteristics of the individual networks clearly visible and to make the differences visually easier to recognize, minimum edge weight and scale for edge weight were set to 0.1 and 1.3, respectively.
For the overestimating student profile (see Figure 9), student teachers with high and with low judgment accuracy relied mostly on a combination of three student cues—frequent hand-raisings, general active class participation (intensity of engagement), and high-quality of answers (content of engagement). The combination of these student cues is not a diagnostic feature of an overestimating profile, but rather of a strong one. As shown in the subtracted network [part (c) in Figure 9], high accuracy student teachers also used a variety of other cue combinations. Some of them captured aspects of an overestimating profile, such as making low quality verbal contributions. These other combinations might be important for high accuracy student teachers to assess the overestimating profile correctly.
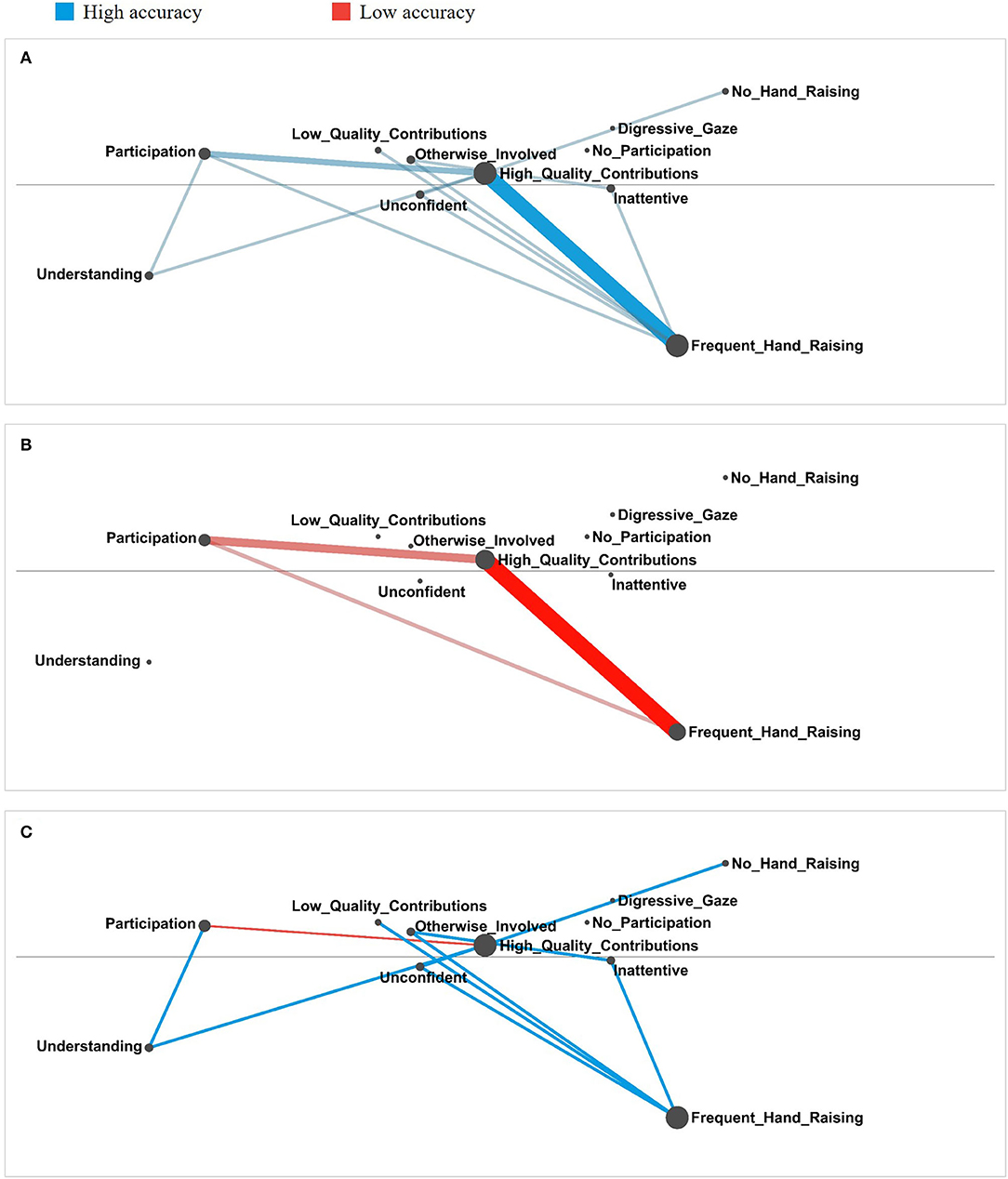
Figure 9. Comparison of networks for overestimating student profile between student teachers with high and low judgment accuracy. (A) Depicts the network of the overestimating student profile for student teachers with high accuracy. (B) Depicts the network of the overestimating student profile for student teachers with low accuracy. (C) Shows the comparison of both networks. Here, the color of connections indicates which group utilized the respective code combination more frequently. In order to make the characteristics of the individual networks clearly visible and to make the differences visually easier to recognize, minimum edge weight, and scale for edge weight were set to 0.1 and 1.3, respectively.
In terms of the underestimating student profile (see Figure 10), both groups utilized a pattern of four student cues: An unconfident appearance combined with avoidance of hand-raisings but high participation in learning activities (intensity of engagement), and understanding of the topic (content of engagement). The subtracted network [part (c) in Figure 10] illustrates that in comparison high accuracy student teachers focused more on the general high participation and high quality of contributions while those with a low judgment accuracy combined the student cue of no hand-raisings with many other cues. Again, the main pattern of high accuracy student teachers seems to be a diagnostic feature for the student profile to be diagnosed. The variety of cue combinations of the low accuracy group, on the other hand, did not allow for a conclusive profile assessment.
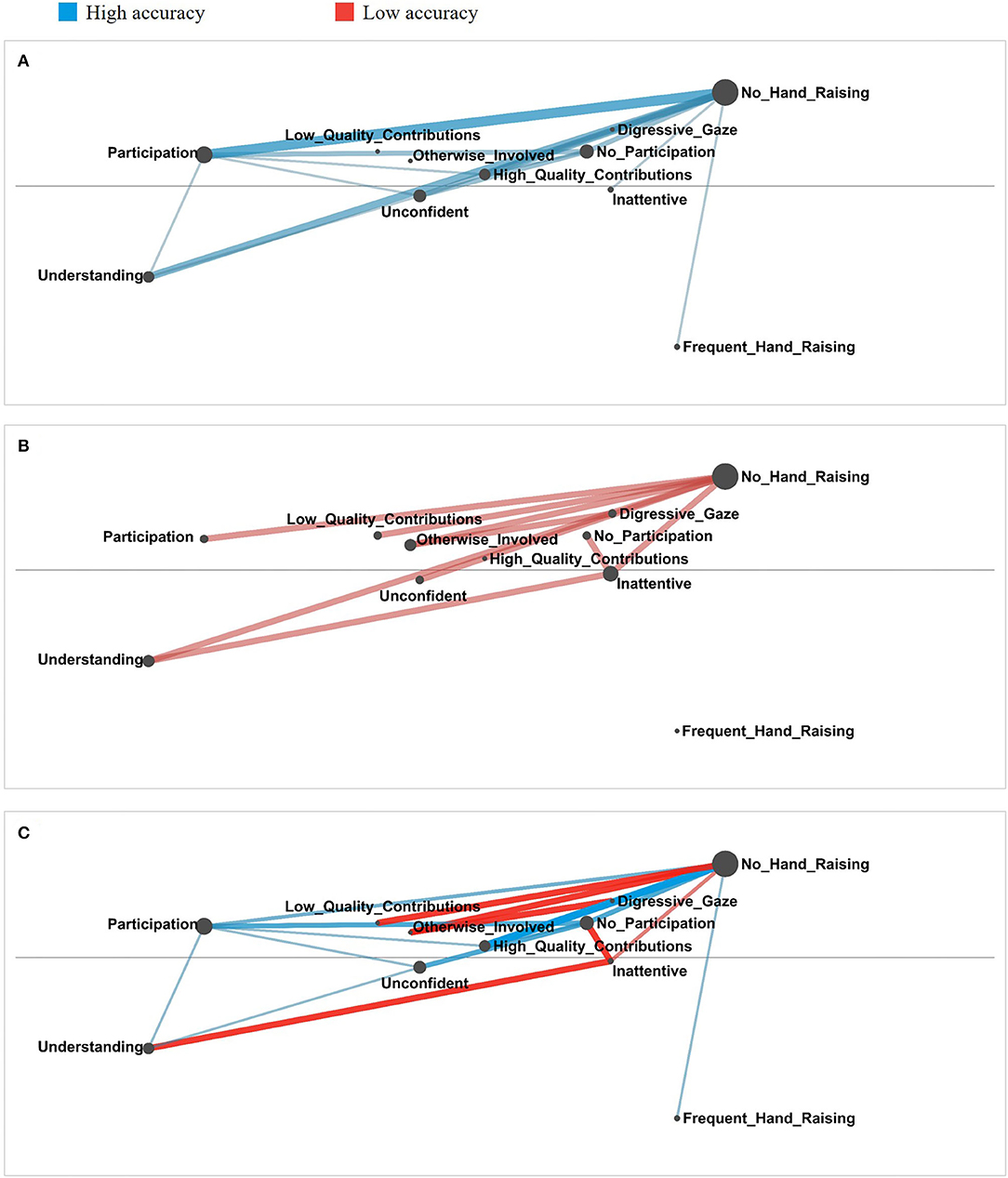
Figure 10. Comparison of networks for underestimating student profile between student teachers with high and low judgment accuracy. (A) Depicts the network of the underestimating student profile for student teachers with high accuracy. (B) Depicts the network of the underestimating student profile for student teachers with low accuracy. (C) Shows the comparison of both networks. Here, the color of connections indicates which group utilized the respective code combination more frequently. In order to make the characteristics of the individual networks clearly visible and to make the differences visually easier to recognize, minimum edge weight and scale for edge weight were set to 0.1 and 1.3, respectively.
To identify the uninterested student profile (see Figure 11), both groups relied on a combination of two student cues: preoccupation with things other than the learning activities and an absent gaze (intensity of engagement). As shown in the subtracted network [part (c) Figure 11], the low judgment accuracy group also reported combinations of other cues indicative for a low motivation (e.g., being inattentive). Hence, both groups utilized cue combinations that are diagnostic features of the uninterested profile. However, the high accuracy group focused on a combination specific to this profile, while the low accuracy group also included cues utilized to assess other profiles.
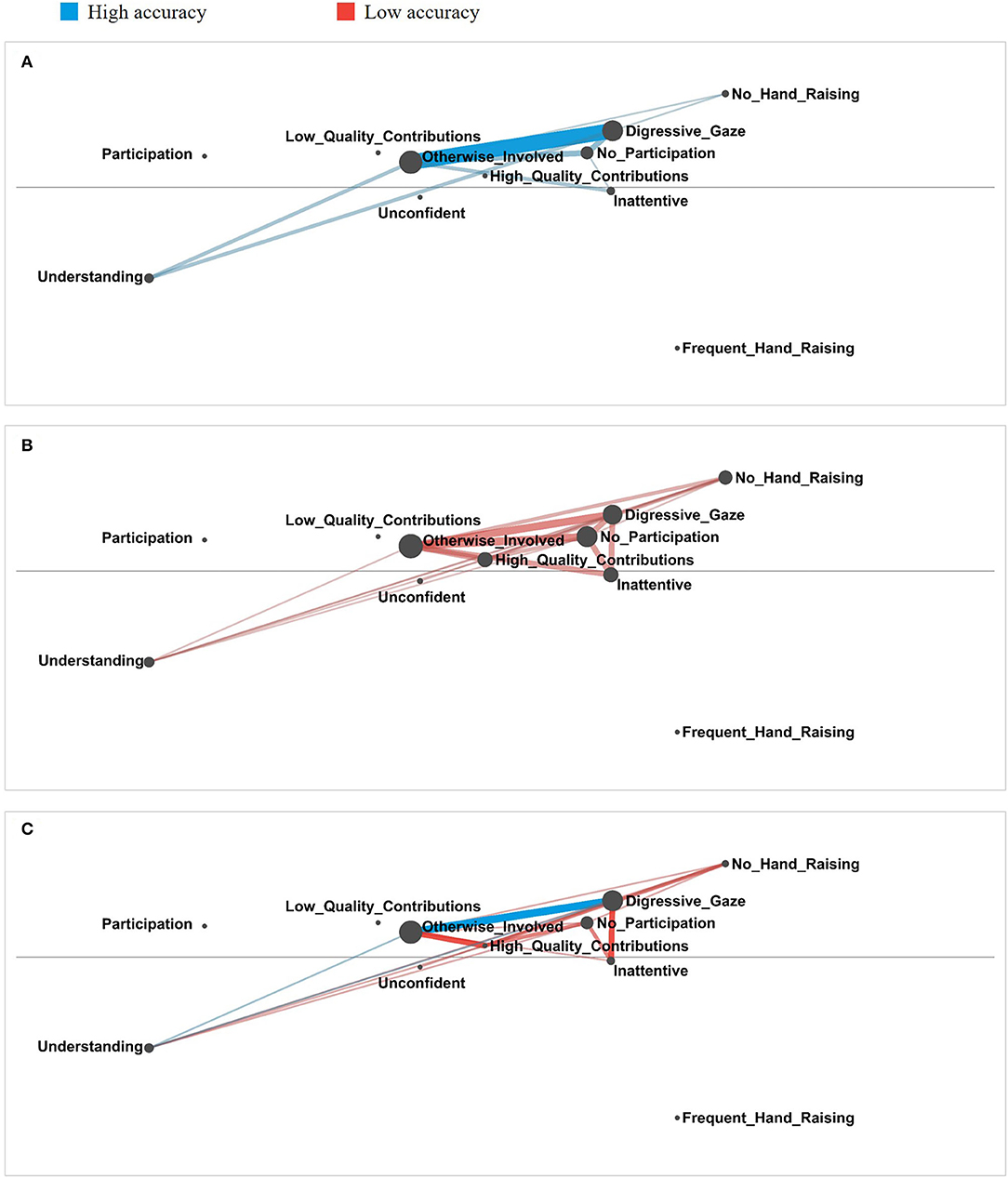
Figure 11. Comparison of networks for uninterested student profile between student teachers with high and low judgment accuracy. (A) Depicts the network of the uninterested student profile for student teachers with high accuracy. (B) Depicts the network of the uninterested student profile for student teachers with low accuracy. (C) Shows the comparison of both networks. Here, the color of connections indicates which group utilized the respective code combination more frequently. In order to make the characteristics of the individual networks clearly visible and to make the differences visually easier to recognize, minimum edge weight and scale for edge weight were set to 0.1 and 1.3, respectively.
Discussion
With the present study we aimed to connect teachers' judgment accuracy to preceding judgment processes. Therefore, we investigated student teachers' accuracy in assessing student profiles, which represent the diversity of students' cognitive and motivational-affective characteristics. Moreover, we explored the differences between student teachers with high and low judgment accuracy to shed light on the process of forming accurate judgments. Therefore, we considered eye movements when observing students as a behavioral activity associated with judgment processes and utilization of combinations of student cues as a cognitive activity.
Student Teachers' Differ in Judgment Accuracy of Student Characteristics
As part of our first research question, we investigated how accurately student teachers can judge student profiles overall, whether they vary in their accuracy across different student profiles, and which of the student profiles they interchange most frequently. In line with our assumptions and previous findings on student teachers' ability to interpret classroom events (Stürmer et al., 2016), the participating student teachers differed substantially in their judgment accuracy. One half assessed most of the student profiles correctly, while the other half struggled to do so. We had expected that student teachers would be more accurate in judging consistent profiles (strong, struggling) than inconsistent ones (overestimating, underestimating, uninterested) since teachers generally seemed to overestimate the level of consistency among their students (Huber and Seidel, 2018; Südkamp et al., 2018) and tended to intermingle cognitive and motivational-affective characteristics (Kaiser et al., 2013). Student teachers succeeded particularly well in recognizing the uninterested (inconsistent) and struggling (consistent) profiles, while they showed difficulties in identifying the strong (consistent), overestimating, and underestimating (inconsistent) student profiles. Hence, our assumption were partially disconfirmed.
This result can be explained with our exploration of the most frequent interchanges of student profiles. First, the uninterested and struggling student were less often interchanged with the other profiles resulting in a higher accuracy for these students. Regarding the uninterested profile, which was surprisingly accurately assessed despite its inconsistency, it can be assumed that this was because the student showed clear, easily observable cues that made judgment easy compared to the other profiles. Second, the strong and overestimating students were often interchanged, resulting in a low judgment accuracy for both profiles. Third, the student with the underestimating profile was often thought to be struggling or uninterested, also leading to low judgment accuracy. Hence, participants tended to interchange student profiles with similar levels of motivational-affective characteristics. This means that our student teachers were quite capable of assessing whether students were interested and feeling competent. However, assessing the level of cognitive characteristics appeared to be more challenging for them. This is somewhat surprising, as previous research findings have indicated that teachers have more difficulty in correctly assessing the motivation of their students than in assessing their achievement. So far it has been assumed that this is because student motivation is not directly observable, but must rather be inferred from the intensity of student engagement (Kaiser et al., 2013; Praetorius et al., 2017). For example, the level of student self-concept must be concluded based on hand-raising behavior (Böheim et al., 2020; Schnitzler et al., 2020). Moreover, it was previously assumed that classroom activities in general lack opportunities to observe student motivation (Kaiser et al., 2013). Thus, our results highlight student teachers' assessment competence. Although participants were unfamiliar with the students and had only limited opportunity to observe them via a relatively short video vignette as a proxy for real classroom teaching, some were already quite able to observe student engagement in a professional manner and thereby form correct judgments about student motivation. This is especially remarkable as our student teachers, only recently started to acquire declarative (pedagogical) knowledge (Shulman, 1986, 1987). Furthermore, they had only a few opportunities to gain the teaching experience necessary to the development of advanced knowledge structures that allow for the application of a professional knowledge base toward specific teaching situations (Carter et al., 1988; Berliner, 2001; Kersting et al., 2016; Lachner et al., 2016; Kim and Klassen, 2018).
However, the question remains as to why participants struggled to assess the level of cognitive characteristics. One possible reason may be that our video vignette did not provide enough opportunities to observe the content of student engagement. Another reason may be that the situations in which content of student engagement became salient were not sufficiently selective, and therefore differences in performance were not visible. Here, especially the observation of individual work that we did not show in the video could have been an important source of information containing other student cues about student cognitive characteristics. Hence, future research might systematically vary the available student cues with regard to the inclusion of information about intensity and content of engagement. Moreover, triangulation with qualitative analyses, like think-aloud protocols, could provide deeper insights into the conditions under which teachers experience either sufficient or limited availability of information about student cognitive and motivational-affective characteristics.
High Judgment Accuracy Relates Minimally to a More “Experienced” Pattern of Eye Movements When Observing Students
Our second research question investigated whether the eye movements of student teachers with a high judgment accuracy differed from those of student teachers with low judgment accuracy. To this end, we focused on the number of fixations and the average fixation duration. Here, a higher number of fixations and shorter average fixation duration represented an “experienced” pattern typical to expert teachers (Gegenfurtner et al., 2011; van den Bogert et al., 2014; Seidel et al., 2020). On a descriptive level we found the expected pattern, those student teachers with a low judgment accuracy showed slight tendencies to fixate the target students on average less often and for a longer time, a pattern typical for student teachers. In contrast, student teachers with a high judgment accuracy displayed an eye movement pattern minimally more similar to experienced teachers, with more fixations and a shorter average fixation duration. Overall, most of these differences were non-significant when correcting for multiple comparisons and therefore results must be interpreted with caution. Nevertheless, our findings make it likely that higher judgment accuracy might be associated with an “experienced” pattern of eye movements, which is an indicator for knowledge-driven observation and rapid information processing (Gegenfurtner et al., 2011; van den Bogert et al., 2014; Seidel et al., 2020). Our findings therefore show that eye movements are a relevant behavioral activity during judgment processes that allow for inferences about the accuracy of judgment formation. Thus, we expand upon the research of teachers' eye movements and emphasize its potential for investigation of issues other than those associated with classroom management. Since teaching is a vision-intense profession that requires teachers to regularly infer information from observing their classrooms (Carter et al., 1988; Gegenfurtner, 2020), the systematic investigation of eye movements might provide insights into different competencies of professional teachers. In terms of assessing teacher competence, future research might also investigate how (student) teachers distribute their gaze across different students and search for information. For example, do teachers with a higher judgment accuracy regularly check upon all of the students, or do they start to observe some students more intensively until they form a decision about their profile, and then move on to the next student for the purposes of profile assessment?
High Judgment Accuracy Relates to a Utilization of Particular Combinations of Diagnostic Student Engagement Cues
Our third research question was explorative in nature and followed the call of previous research to investigate which student cues teachers utilize to assess cognitive and motivational-affective characteristics, as well as their combination within individual students (Glock et al., 2013; Praetorius et al., 2017; Huber and Seidel, 2018; Brandmiller et al., 2020). Therefore, we investigated which student cues student teachers utilized to assess student characteristic profiles. Moreover, we aimed to identify differences in student cue utilization of student teachers with high and low judgment accuracy based on the assumptions of the lens model (Brunswik, 1956; Funder, 1995, 2012). In other words, accurate judgments of latent student characteristics depend on inference of intensity and content of student engagement as diagnostic cues for student motivation and cognitive characteristics. For the purposes of investigation, we applied the relatively new method of epistemic network analysis, which enabled us to gain detailed insights in how student teachers combined student cues to form judgments.
As outlined in our theory section (student) teachers need to observe and utilize student cues, which are diagnostic and provide information both about students' cognitive and motivational-affective characteristics, to assess student characteristic profiles accurately. According to our inductive coding of reported student cues, in general student teachers utilized diagnostic cues to assess student profiles. This means that they considered a mixture of student cues containing information about the intensity and content of student engagement, which relate back to student cognitive and motivational-affective characteristics. These were first and foremost the intensity and content of student behavioral engagement (Fredricks et al., 2004). Student teachers took into account in particular whether students showed general participation in learning activities, whether they raised their hands to contribute to classroom dialogue, and also considered the quality of students' verbal contributions frequently. That student teachers dominantly rely on such diagnostic student cues, contradicts previous research which showed that teachers also take into account misleading or unimportant information like student gender, ethnicity, immigration status, and SES in their assessment of student characteristics (Meissel et al., 2017; Praetorius et al., 2017; Garcia et al., 2019; Brandmiller et al., 2020). However, these studies used text vignettes to provide teachers with specific information about target students. Hence, our implementation of a video vignette as another proxy to everyday teaching, which contains rich information about students' engagement, might complement these prior findings, because teachers' utilization of student cues depends on availability of information, which differs between text vignettes and classroom videos (Funder, 1995, 2012). Thus, future research might systematically investigate the role of the stimulus (video or text vignette) and the amount and diversity of available information in teachers' use of diagnostic cues and ignorance of misleading ones. Additionally, our participants reported more diverse student cues with regard to the intensity of engagement than content of student engagement. This finding might explain why student teachers struggled to assess the level of student cognitive characteristics. The available student cues may have not contained diverse enough information to allow for a differentiation of student cognitive characteristics. For example, although student teachers considered the quality of student verbal contributions, they might not have provided deep insights into student knowledge because the video stemmed from an introductory lesson. The teacher's questions might have been rather easy so that most of the students were able to answer them correctly and could follow instructions. Other sources of information like students' solutions to mathematical tasks might contain more sufficient information to assess students' cognitive characteristics. Hence, upcoming studies might investigate which sources of information provide teachers with cues that allow for a differentiation between students in terms of cognitive and motivational-affective characteristics. Overall, here we provide promising findings, in that student teachers are already able to observe and utilize diagnostic student cues.
Results from our epistemic network analysis pointed toward systematic differences in how student teachers with low and high judgment accuracy combined student cues. As we had expected, student teachers with a high judgment accuracy seemed to utilize combinations of student cues of intensity and content of engagement that were diagnostic features of particular student profiles. In contrast, those student teachers with a low judgment accuracy also relied on diagnostic student cues but seemed to utilize many different cue co-occurrences for each student profile, including misleading combinations. For example, to identify the struggling profile, both groups focused on combinations of an unconfident appearance, avoidance of hand-raisings (intensity of engagement), and low quality of answers (content of engagement). However, some student teachers with a low judgment accuracy seemed to rate the quality of the verbal contributions at the same time as high probably affecting their assessment and leading to incorrect judgments due to this misleading student cue. This overlaps with previous research, which reported that student teachers try to use as much information as possible to form judgments, while experienced teachers select the most relevant information (Böhmer et al., 2017). In this sense, student teachers with a high judgment accuracy seemed to have already developed a professional skill in that they were able to observe diagnostic student cues, utilize the relevant co-occurrences of these cues, and correctly infer student profiles. In terms of the development of teachers' professional vision, it can be assumed that student teachers with a high judgment accuracy are already able to apply their acquired declarative knowledge to assess student profiles from observation (Jacobs et al., 2010; Stürmer et al., 2013; Kersting et al., 2016; Lachner et al., 2016). Conversely, those with a low judgment accuracy struggle to recognize relevant information, as is quite typical of student teachers and beginning teachers (Carter et al., 1988; Berliner, 2001; Star and Strickland, 2008; Kim and Klassen, 2018; Keppens et al., 2019).
Another interesting finding resulted from our epistemic network analysis. Networks of student profiles that were frequently interchanged showed a rather similar structure. This means that difficulties in distinguishing the struggling, underestimating, and uninterested profiles from one another, as well as the strong and overestimating profiles, can be traced back to the utilization of similar combinations of student cues. This makes sense in part, since the interchanged profiles overlapped in their motivational-affective characteristics and therefore showed a similar intensity of engagement. However, differences in students' level of cognitive characteristics could have resulted in differentiated cue combinations of intensity of engagement and content of engagement, which would have allowed to distinguish the profiles. As outlined above, at this point it remains unclear whether this was due to the student cues contained in our video vignette or whether this is a general challenge for (student) teachers.
Our findings emphasize cognitive activities of judgment processes as a key to judgment accuracy. Teachers' utilization of student cues determines whether student teachers are successful in judging student characteristic profiles accurately. In this regard, epistemic network analysis seems to be a promising approach. Based on such an analysis, which visualizes the frequency of all co-occurrences of utilized student cues, it becomes evident that accurate judgments, difficulties with assessments, and interchanges of student profiles can be traced back to reliance on particular combinations of diagnostic student cues. Hence, epistemic network analysis allowed us to consider and investigate the complexity of everyday teacher judgment processes in which teachers are required to observe and interpret several pieces of information in combination to assess their students.
Practical Implications
Although our study has shown that some of the student teachers are already quite able to successfully assess student characteristics, a large number of them still struggles with this important task, which will later become a regular part of their professional everyday life. Furthermore, our results highlight the role of observing and using diagnostic student cues for accurate judgments. From this, several recommendations for teacher education can be derived. Teacher education should promote student teachers' declarative knowledge base with respect to learning relevant student characteristics, their intra-individual combination in consistent and inconsistent student profiles, and student cues that are diagnostic for these characteristics (that is intensity and content of engagement) as a foundation for the development of a professional vision in the context of assessing student characteristics (Stürmer et al., 2013). As the judgment of student characteristics requires student teachers to apply their knowledge toward teaching practice, they should receive support and instruction in how to do so in a step-by-step approach in which practice tasks are subsequently approximated to real-life teaching (Grossman et al., 2009). In this sense, the guided observation and reflection of classroom videos could be effective, like it has already been shown for the development of other areas of professional classroom perception (Star and Strickland, 2008; van Es and Sherin, 2008; Stürmer et al., 2013). Such practical experience might lead to changes in student teachers' perceptions of student cues, they could for example become more sensitive to the variance of participation among students leading to a more refined differentiation of whether students raise their hands often or seldom. Moreover, they might learn to identify, focus, and consistently use diagnostic student cues to assess the same student characteristic across several students (Nestler and Back, 2013). Besides this guided practice in teacher education courses, simulations have recently been discussed as a way to support teacher students in the development of their assessment skills (Chernikova et al., 2020a,b; see Codreanu et al., 2020 for an example concerning students' mathematical skills). In terms of observing students, it has been shown in other disciplines that the modeling of expert eye movements can help learners to develop effective eye movement patterns (Jarodzka et al., 2012, 2013). It is assumed that this can also be applied to teacher education although (intervention) studies are still pending (Gegenfurtner, 2020). Our findings are thus a good starting point for the development of appropriate teacher education programs.
Limitation
There are some methodological issues that need to be considered when interpreting the results. First, we used only one video as a stimulus. Thus, difficulties in distinguishing student profiles, the type of frequently used student cues, and differences in student cue utilization between student teachers may stem from specific features of the video. Hence, future studies might investigate whether our findings are replicable with other classroom videos and real-life teaching. Second, we did not systematically vary the available student cues, but rather used an every-day classroom video as a proxy for real classroom teaching so that student cue availability could be considered as “natural.” In our case, we did not include a student individual work phase although this might have contained student cues that relate to student cognitive characteristics. This might have resulted in the rather weak performance of participants in judging student cognitive characteristics in comparison to other previous studies (Kaiser et al., 2013; Praetorius et al., 2017). Therefore, upcoming research might systematically investigate the influence of cue availability on judgment accuracy. Third, our video was relatively short, and student teachers were unfamiliar with the students. Although other studies showed that even very short videos are sufficient for accurate judgments (Praetorius et al., 2015), it might be interesting to systematically investigate whether familiarity with students influences judgment accuracy. Additionally, the comparisons of the eye movement patterns were based on median split of the whole sample, resulting in two relatively small subgroups. As a consequence, the tendencies in the expected direction toward differences in number of fixations and average fixation duration across student teachers with high and low judgment accuracy might have not reached significance. Therefore, differences might be underestimated within the present study and judgment accuracy might actually show a stronger connection to eye movements as a behavioral activity of judgment processes. Moreover, our participants provided the student cues on a voluntary base. This might have resulted in the presence of a bias and, above all, participants who are confident in their diagnostic accuracy might have worked on the task. Thus, it might have been likely that we included participants with a relatively higher judgment accuracy in our analysis on usage of student cues. However, we found no significant differences in the average diagnostic accuracy score across all student profiles between those participants who reported student cues (M = 2.48; SD = 1.25) and those who did not (M = 1.81; SD = 1.1); t(41) = −1.77, p = 0.085. Additionally, the judgment accuracy of those participants included in our analysis on utilization of student cues spread across the whole range of accuracy scores, ranging between 0 and 4 points. Nevertheless, our results should be interpreted with this limitation in mind and differences in the patterns of student cue usage between student teachers with high and low judgment accuracy can unfortunately be less obvious in our study than they actually are. As a related issue, our analysis of student cue utilization is based on student teachers' self-reports. This might have resulted in a social desirability bias of their answers and would be one explanation why our findings contradict previous ones in which teachers relied on unimportant student cues such as gender or SES (Meissel et al., 2017; Praetorius et al., 2017; Garcia et al., 2019; Brandmiller et al., 2020). In this context, our results might not fully reflect potentially problematic cue usage of student teachers. Finally, we argue that student teachers with a high judgment accuracy successfully applied their acquired declarative knowledge to our specific situation, although they had only limited opportunities to connect their knowledge base to teaching experiences. However, we did not measure our participants' declarative knowledge. Thus, future research might elaborate on this issue and consider student cue utilization and eye movements as a mediator between teachers' declarative knowledge and judgment accuracy.
Conclusion
The present study was one of the first that aimed to connect judgment processes to judgment accuracy. Therefore, we considered student diversity, in the form of student characteristic profiles, as an assessment target; investigated eye movements as a behavioral activity; and looked at utilization of student cues as a cognitive activity; all in keeping with the lens model. The results advanced the understanding of teachers' accurate judgments. First, we identified a level of diversity among student teachers. A significant portion of the sample group was already quite successful in the complex task of assessing student profiles. The methodology of eye tracking indicates that this success tends to go along with a more “experienced” pattern of eye movements. The epistemic network analysis demonstrated the importance of using specific diagnostic student cues for high judgment accuracy. With this study, we have brought together research on the judgment process and on judgment accuracy. This allowed us to provide detailed insights into the processes of accurate judgments and is a necessity to understanding teaching as a vision-intense profession.
Data Availability Statement
The datasets generated for this study are available from the corresponding author upon request.
Ethics Statement
Ethical review and approval was not required for the study on human participants in accordance with the local legislation and institutional requirements. The patients/participants provided their written informed consent to participate in this study.
Author Contributions
KS organized the data collection and database, performed statistical analysis, and wrote the first draft of the manuscript. All authors contributed to the conception and design of the study, manuscript revision, and read and approved the submitted version.
Funding
The development of the ENA webtool was funded by the National Science Foundation (DRL-1661036, DRL-1713110), the Wisconsin Alumni Research Foundation, and the Office of the Vice Chancellor for Research and Graduate Education at the University of Wisconsin-Madison. The present research project was funded by the Deutsche Forschungsgemeinschaft (German Research Foundation, grant no. SE1397/7-3). The funders had no role in the study's design, data collection and analysis, decision to publish, or preparation of the manuscript.
Conflict of Interest
The authors declare that the research was conducted in the absence of any commercial or financial relationships that could be construed as a potential conflict of interest.
References
Andrist, S., Collier, W., Gleicher, M., Mutlu, B., and Shaffer, D. (2015). Look together: analyzing gaze coordination with epistemic network analysis. Front. Psychol. 6:1016. doi: 10.3389/fpsyg.2015.01016
APA American Psychological Association (2017). Ethical Principles of Psychologists and Code of Conduct. Washington, DC: American Psychological Association (APA). Available online at: https://www.apa.org/ethics/code
Appleton, J. J., Christenson, S. L., and Furlong, M. J. (2008). Student engagement with school: critical conceptual and methodological issues of the construct. Psychol. Schools 45, 369–386. doi: 10.1002/pits.20303
Back, M. D., and Nestler, S. (2016). “Accuracy of judging personality,” in The Social Psychology of Perceiving Others Accurately, eds J. A. Hall, M. S. Mast, and T. V. West (Cambridge: Cambridge University Press), 98–124. doi: 10.1017/CBO9781316181959.005
Baumert, J., and Kunter, M. (2006). Stichwort: professionelle Kompetenz von Lehrkräften [Keyword: Professional competence of teachers]. Zeitschrift für Erziehungswissenschaft 9, 469–520. doi: 10.1007/s11618-006-0165-2
Baumert, J., and Kunter, M. (2013). “The COACTIV model of teachers' professional competence,” in Cognitive Activation in the Mathematics Classroom and Professional Competence of Teachers: Results From the COACTIV Project, eds M. Kunter, J. Baumert, W. Blum, U. Klusmann, S. Krauss, and M. Neubrand (New York, NY: Springer), 25–48. doi: 10.1007/978-1-4614-5149-5_2
Berliner, D. C. (2001). Learning about and learning from expert teachers. Int. J. Educat. Res. 35, 463–482. doi: 10.1016/S0883-0355(02)00004-6
Binder, K., Krauss, S., Hilbert, S., Brunner, M., Anders, Y., and Kunter, M. (2018). “Diagnostic skills of mathematics teachers in the COACTIV study,” in Diagnostic Competence of Mathematics Teachers: Unpacking a Complex Construct in Teacher Education and Teacher Practice, eds T. Leuders, K. Philipp, and J. Leuders (Cham: Springer), 33–53.
Blömeke, S., Gustafsson, J.-E., and Shavelson, R. J. (2015). Beyond dichotomies. Zeitschrift für Psychologie 223, 3–13. doi: 10.1027/2151-2604/a000194
Böheim, R., Knogler, M., Kosel, C., and Seidel, T. (2020). Exploring student hand-raising across two school subjects using mixed methods: an investigation of an everyday classroom behavior from a motivational perspective. Learn. Instruct. 65:101250. doi: 10.1016/j.learninstruc.2019.101250
Böhmer, I., Gräsel, C., Krolak-Schwerdt, S., Höstermann, T., and Glock, S. (2017). “Teachers' school tracking decisions,” in Competence Assessment in Education: Research, Models and Instruments, eds D. Leutner, J. Fleischer, J. Grünkorn, and E. Klieme (Cham: Springer International Publishing), 131–148. doi: 10.1007/978-3-319-50030-0_9
Böhmer, I., Hörstermann, T., Gräsel, C., Krolak-Schwerdt, S., and Glock, S. (2015). Eine Analyse der Informationssuche bei der Erstellung der Übergangsempfehlung: Welcher Urteilsregel folgen Lehrkräfte? [An analysis of the search for information when preparing the school tracking recommendation: What judgment rule do teachers follow?]. J. Educat. Res. Online 7, 59–81.
Boshuizen, H. P. A., Gruber, H., and Strasser, J. (2020). Knowledge restructuring through case processing: the key to generalise expertise development theory across domains? Educat. Res. Rev. 29:100310. doi: 10.1016/j.edurev.2020.100310
Brandmiller, C., Dumont, H., and Becker, M. (2020). Teacher perceptions of learning motivation and classroom behavior: the role of student characteristics. Contemporary Educat. Psychol. 51, 336–355. doi: 10.1016/j.cedpsych.2020.101893
Brunswik, E. (1956). Perception and the Representative Design of Psychological Experiments. 2nd Edn. Berkeley: University of California Press.
Carter, K., Cushing, K., Sabers, D., Stein, P., and Berliner, D. C. (1988). Expert-novice differences in perceiving and processing visual classroom information. J. Teacher Educat. 39, 25–31. doi: 10.1177/002248718803900306
Chernikova, O., Heitzmann, N., Fink, M. C., Timothy, V., Seidel, T., and Fischer, F. (2020a). Facilitating diagnostic competence in higher education – a meta-analysis in medical and teacher education. Educat. Psychol. Rev. 32, 157–196. doi: 10.1007/s10648-019-09492-2
Chernikova, O., Heitzmann, N., Stadler, M., Holzberger, D., Seidel, T., and Fischer, F. (2020b). Simulation-based learning in higher education: a meta-analysis. Rev. Educat. Res. 4, 499–541. doi: 10.3102/0034654320933544
Chi, M. T. H., Adams, J., Bogusch, E. B., Bruchok, C., Kang, S., Lancaster, M., et al. (2018). Translating the ICAP theory of cognitive engagement into practice. Cognit. Sci. 42, 1777–1832. doi: 10.1111/cogs.12626
Codreanu, E., Sommerhoff, E., Huber, S., Ufer, S., and Seidel, T. (2020). Between authenticity and cognitive demand: finding a balance in designing a video-based simulation in the context of mathematics teacher education. Teach. Teacher Educat. 95:103146. doi: 10.1016/j.tate.2020.103146
Cooksey, R. W., Freebody, P., and Wyatt-Smith, C. (2007). Assessment as judgment-in-context: analysing how teachers evaluate students' writing. Educat. Res. Evaluat. 13, 401–434. doi: 10.1080/13803610701728311
Cooksey, R. W., Freeboy, P., and Davidson, G. R. (1986). Teachers' predictions of children's early reading achievement: an application of social judgment theory. Am. Educat. Res. J. 23, 41–64. doi: 10.3102/00028312023001041
Corno, L. (2008). On teaching adaptively. Educat. Psychol. 43, 161–173. doi: 10.1080/00461520802178466
Cortina, K. S., Miller, K. F., McKenzie, R., and Epstein, A. (2015). Where low and high inference data converge: validation of CLASS assessment of mathematics instruction using mobile eye tracking with expert and novice teachers. Int. J. Sci. Math Educat. 13, 389–403. doi: 10.1007/s10763-014-9610-5
Csanadi, A., Eagan, B., Kollar, I., Shaffer, D. W., and Fischer, F. (2018). When coding-and-counting is not enough: using epistemic network analysis (ENA) to analyze verbal data in CSCL research. Int. J. Comput. Supported Collaborative Learn. 13, 419–438. doi: 10.1007/s11412-018-9292-z
DeAngelus, M., and Pelz, J. B. (2009). Top-down control of eye movements: yarbus revisited. Visual Cognition 17, 790–811. doi: 10.1080/13506280902793843
Deary, I. J., Strand, S., Smith, P., and Fernandes, C. (2007). Intelligence and educational achievement. Intelligence 35, 13–21. doi: 10.1016/j.intell.2006.02.001
Förster, N., and Böhmer, I. (2017). “Das Linsenmodell - Grundlagen und exemplarische Anwendungen in der pädagogisch-psychologischen Diagnostik [The lens-model—basics and exemplary applications in educational-psychological diagnostics],” in Diagnostische Kompetenz von Lehrkräften: Theoretische und methodische Weiterentwicklungen, eds A. Südkamp and A.-K. Praetorius (Münster, New York: Waxmann), 46–50.
Fredricks, J. A., Blumenfeld, P. C., and Paris, A. H. (2004). School engagement: potential of the concept, state of the evidence. Rev. Educat. Res. 74, 59–109. doi: 10.3102/00346543074001059
Fredricks, J. A., Filsecker, M., and Lawson, M. A. (2016a). Student engagement, context, and adjustment: addressing definitional, measurement, and methodological issues. Learn. Instruct. 43, 1–4. doi: 10.1016/j.learninstruc.2016.02.002
Fredricks, J. A., Wang, M.-T., Schall Linn, J., Hofkens, T. L., Sung, H., Parr, A., et al. (2016b). Using qualitative methods to develop a survey measure of math and science engagement. Learn. Instruct. 43, 5–15. doi: 10.1016/j.learninstruc.2016.01.009
Funder, D. C. (1995). On the accuracy of personality judgment: a realistic approach. Psychol. Rev. 102, 652–670. doi: 10.1037/0033-295X.102.4.652
Funder, D. C. (2012). Accurate personality judgment. Curricul. Direct. Psychol. Sci. 21, 177–182. doi: 10.1177/0963721412445309
Garcia, E. B., Sulik, M. J., and Obradović, J. (2019). Teachers' perceptions of students' executive functions: disparities by gender, ethnicity, and ELL status. J. Educat. Psychol. 111, 918–931. doi: 10.1037/edu0000308
Gegenfurtner, A. (2020). Professional Vision and Visual Expertise. Regensburg: University of Regensburg.
Gegenfurtner, A., Lehtinen, E., and Säljö, R. (2011). Expertise differences in the comprehension of visualizations: a meta-analysis of eye-tracking research in professional domains. Educat. Psychol. Rev. 23, 523–552. doi: 10.1007/s10648-011-9174-7
Glock, S., Krolak-Schwerdt, S., Klapproth, F., and Böhmer, M. (2012). Improving teachers' judgments: accountability affects teachers' tracking decision. Int. J. Tech. Inclusive Educat. 1, 89–98. doi: 10.20533/ijtie.2047.0533.2012.0012
Glock, S., Krolak-Schwerdt, S., Klapproth, F., and Böhmer, M. (2013). Beyond judgment bias: how students' ethnicity and academic profile consistency influence teachers' tracking judgments. Soc. Psychol. Educat. 16, 555–573. doi: 10.1007/s11218-013-9227-5
Goodwin, C. (1994). Professional vision. Am. Anthropolog. 96, 606–633. doi: 10.1525/aa.1994.96.3.02a00100
Grossman, P., Compton, C., Igra, D., Romfeldt, M., Shahan, E., and Willimanson, P. W. (2009). Teaching practice: a cross-professional perspective. Teachers College Record 111, 2055–2100.
Haataja, E., Garcia Moreno-Esteva, E., Salonen, V., Laine, A., Toivanen, M., and Hannula, M. S. (2019). Teacher's visual attention when scaffolding collaborative mathematical problem solving. Teach. Teach. Educat. 86:102877. doi: 10.1016/j.tate.2019.102877
Haataja, E., Salonen, V., Laine, A., Toivanen, M., and Hannula, M. S. (2020). The relation between teacher-student eye contact and teachers' interpersonal behavior during group work: a multiple-person gaze-tracking case study in secondary mathematics education. Educat. Psychol. Rev. 8:229. doi: 10.1007/s10648-020-09538-w
Heitzmann, N., Seidel, T., Opitz, A., Hetmanek, A., Wecker, C., Ufer, S., et al. (2019). Facilitating diagnostic competence in simulations: a conceptual framework and a research agenda for medical and teacher education. Frontline Learn. Res. 7, 1–24. doi: 10.14786/flr.v7i4.384
Herppich, S., Praetorius, A.-K., Förster, N., Glogger-Frey, I., Karst, K., Leutner, D., et al. (2018). Teachers' assessment competence: integrating knowledge-, process, and product-oriented approaches into a competence-oriented conceptual model. Teach. Teacher Educat. 76, 181–193. doi: 10.1016/j.tate.2017.12.001
Holmqvist, K., Nyström, M., Andersson, R., Dewhurst, R., Jarodzka, H., and Van de Weijer, J. (2011). Eye Tracking: A Comprehensive Guide to Methods and Measures. First edition. Oxford, New York, NY; Auckland: Oxford University Press.
Hörstermann, T., Pit-ten Cate, I. M., Krolak-Schwerdt, S., and Glock, S. (2017). “Primacy effects in attention, recall and judgment patterns of simultaneously presented student information: Evidence from an eye-tracking study,” in Student Achievement: Perspectives, Assessment and Improvement Strategies, ed G. Hughes (Hauppauge: Nova Science Publishers Incorporated), 1–28.
Huang, C. (2011). Self-concept and academic achievement: a meta-analysis of longitudinal relations. J. School Psychol. 49, 505–528. doi: 10.1016/j.jsp.2011.07.001
Huber, S. A., Häusler, J., Jurik, V., and Seidel, T. (2015). Self-underestimating students in physics instruction: development over a school year and its connection to internal learning processes. Learn. Individ. Diff. 43, 83–91. doi: 10.1016/j.lindif.2015.08.021
Huber, S. A., and Seidel, T. (2018). Comparing teacher and student perspectives on the interplay of cognitive and motivational-affective student characteristics. PLoS ONE 13:e0200609. doi: 10.1371/journal.pone.0200609
Iacobucci, D., Posavac, S. S., Kardes, F. R., Schneider, M. J., and Popovich, D. L. (2015a). The median split: robust, refined, and revived. J. Consumer Psychol. 25, 690–704. doi: 10.1016/j.jcps.2015.06.014
Iacobucci, D., Posavac, S. S., Kardes, F. R., Schneider, M. J., and Popovich, D. L. (2015b). Toward a more nuanced understanding of the statistical properties of a median split. J. Consumer Psychol. 25, 652–665. doi: 10.1016/j.jcps.2014.12.002
Jacobs, V. R., Lamb, L. L., and Philipp, R. (2010). Professional noticing of children's mathematical thinking. J. Res. Mathemat. Educat. 41, 169–202. doi: 10.5951/jresematheduc.41.2.0169
Jansen, M., Lüdtke, O., and Schroeders, U. (2016). Evidence for a positive relation between interest and achievement: examining between-person and within-person variation in five domains. Contemporary Educat. Psychol. 46, 116–127. doi: 10.1016/j.cedpsych.2016.05.004
Jarodzka, H., Balsev, T., Homqvist, K., Nyström, M., Scheiter, K., Gerjets, P., et al. (2012). Conveying clinical reasoning based on visual observation via eye-movement modelling examples. Instruct. Sci. 5, 813–827. doi: 10.1007/s11251-012-9218-5
Jarodzka, H., Holmqvist, K., and Gruber, H. (2017). Eye tracking in educational science: theoretical frameworks and research agendas. J. Eye Movement Res. 10, 1–18. doi: 10.16910/jemr.10.1.3
Jarodzka, H., van Gog, T., Dorr, M., Scheiter, K., and Gerjets, P. (2013). Learning to see: guiding students' attention via a model's eye movements fosters learning. Learn. Instruct. 25, 62–70. doi: 10.1016/j.learninstruc.2012.11.004
Jurik, V., Gröschner, A., and Seidel, T. (2013). How student characteristics affect girls' and boys' verbal engagement in physics instruction. Learn. Instruct. 23, 33–42. doi: 10.1016/j.learninstruc.2012.09.002
Jurik, V., Gröschner, A., and Seidel, T. (2014). Predicting students' cognitive learning activity and intrinsic learning motivation: how powerful are teacher statements, student profiles, and gender? Learn. Individ. Diff. 32, 132–139. doi: 10.1016/j.lindif.2014.01.005
Kaiser, J., Retelsdorf, J., Südkamp, A., and Möller, J. (2013). Achievement and engagement: how student characteristics influence teacher judgments. Learn. Instruct. 28, 73–84. doi: 10.1016/j.learninstruc.2013.06.001
Karing, C. (2009). Diagnostische Kompetenz von Grundschul- und Gymnasiallehrkräften im Leistungsbereich und im Bereich Interessen [Diagnostic competence of primary and secondary school teachers regarding achievement and interest]. Zeitschrift für Pädagogische Psychol. 23, 197–209. doi: 10.1024/1010-0652.23.34.197
Karst, K., and Bonefeld, M. (2020). Judgment accuracy of preservice teachers regarding student performance: the influence of attention allocation. Teach. Teacher Educ. 94:103099. doi: 10.1016/j.tate.2020.103099
Kaufmann, E., Reips, U.-D., and Wittmann, W. W. (2013). A critical meta-analysis of lens model studies in human judgment and decision-making. PLoS ONE 8:e83528. doi: 10.1371/journal.pone.0083528
Keppens, K., Consuegra, E., Goossens, M., Maeyer, S., and de Vanderlinde, R. (2019). Measuring pre-service teachers' professional vision of inclusive classrooms: a video-based comparative judgement instrument. Teach. Teach. Educat. 78, 1–14. doi: 10.1016/j.tate.2018.10.007
Kersting, N. B., Sutton, T., Kalinec-Craig, C., Stoehr, K. J., Heshmati, S., Lozano, G., et al. (2016). Further exploration of the classroom video analysis (CVA) instrument as a measure of usable knowledge for teaching mathematics: taking a knowledge system perspective. ZDM Mathematics Educat. 48, 97–109. doi: 10.1007/s11858-015-0733-0
Kim, L. E., and Klassen, R. M. (2018). Teachers' cognitive processing of complex school-based scenarios: differences across experience levels. Teach. Teach. Educat. 73, 215–226. doi: 10.1016/j.tate.2018.04.006
Krauzlis, R. J., Goffart, L., and Hafed, Z. M. (2017). Neuronal control of fixation and fixational eye movements. Philosophic. Transact. R. Soc. London 372:20160205. doi: 10.1098/rstb.2016.0205
Krolak-Schwerdt, S., Böhmer, M., and Gräsel, C. (2013). The impact of accountability on teachers' assessments of student performance: a social cognitive analysis. Soc. Psychol. Educat. 16, 215–239. doi: 10.1007/s11218-013-9215-9
Kuncel, N. R., Klieger, D. M., Connelly, B. S., and Ones, D. S. (2013). Mechanical versus clinical data combination in selection and admissions decisions: a meta-analysis. J. Appl. Psychol. 98, 1060–1072. doi: 10.1037/a0034156
Lachner, A., Jarodzka, H., and Nückles, M. (2016). What makes an expert teacher? Investigating teachers' professional vision and discourse abilities. Instruct. Sci. 44, 197–203. doi: 10.1007/s11251-016-9376-y
Lam, S.-,f., Jimerson, S., Shin, H., Cefai, C., Veiga, F. H., Hatzichristou, C., et al. (2016). Cultural universality and specificity of student engagement in school: the results of an international study from 12 countries. Br. J. Educat. Psychol. 86, 137–153. doi: 10.1111/bjep.12079
Lau, S., and Roeser, R. W. (2008). Cognitive abilities and motivational processes in science achievement and engagement: a person-centered analysis. Learn. Individual Diff. 18, 497–504. doi: 10.1016/j.lindif.2007.11.002
Linnenbrink-Garcia, L., Pugh, K. J., Koskey, K. L. K., and Stewart, V. C. (2012). Developing conceptual understanding of natural selection: the role of interest, efficacy, and basic prior knowledge. J. Exp. Educat. 80, 45–68. doi: 10.1080/00220973.2011.559491
Loibl, K., Leuders, T., and Dörfler, T. (2020). A framework for explaining teachers' diagnostic judgements by cognitive modeling (DiaCoM). Teach. Teach. Educat. 91:103059. doi: 10.1016/j.tate.2020.103059
Machts, N., Kaiser, J., Schmidt, F. T. C., and Möller, J. (2016). Accuracy of teachers' judgments of students' cognitive abilities: a meta-analysis. Educat. Res. Rev. 19, 85–103. doi: 10.1016/j.edurev.2016.06.003
Marksteiner, T., Reinhard, M.-A., Dickhäuser, O., and Sporer, S. L. (2012). How do teachers perceive cheating students? Beliefs about cues to deception and detection accuracy in the educational field. Eur. J. Psychol. Educat. 27, 329–350. doi: 10.1007/s10212-011-0074-5
Marquart, C. L., Hinojosa, C., Swiecki, Z., Eagan, B., and Shaffer, D. W. (2018). Epistemic Network Analysis (Version 1.7.0) [Software]. Available online at: http://app.epistemicnetwork.org
Marsh, H. W., and Martin, A. J. (2011). Academic self-concept and academic achievement: relations and causal ordering. Br. J. Educat. Psychol. 81, 59–77. doi: 10.1348/000709910X503501
McIntyre, N. A., and Foulsham, T. (2018). Scanpath analysis of expertise and culture in teacher gaze in real-world classrooms. Instruct. Sci. 46, 435–455. doi: 10.1007/s11251-017-9445-x
McIntyre, N. A., Jarodzka, H., and Klassen, R. M. (2019). Capturing teacher priorities: using real-world eye-tracking to investigate expert teacher priorities across two cultures. Learn. Instruct. 60, 215–224. doi: 10.1016/j.learninstruc.2017.12.003
McIntyre, N. A., Mainhard, M. T., and Klassen, R. M. (2017). Are you looking to teach? Cultural, temporal and dynamic insights into expert teacher gaze. Learn. Instruct. 49, 41–53. doi: 10.1016/j.learninstruc.2016.12.005
Meissel, K., Meyer, F., Yao, E. S., and Rubie-Davies, C. M. (2017). Subjectivity of teacher judgments: exploring student characteristics that influence teacher judgments of student ability. Teach. Teach. Educat. 65, 48–60. doi: 10.1016/j.tate.2017.02.021
Meschede, N., Fiebranz, A., Möller, K., and Steffensky, M. (2017). Teachers' professional vision, pedagogical content knowledge and beliefs: on its relation and differences between student and in-service teachers. Teach. Teach. Educat. 66, 158–170. doi: 10.1016/j.tate.2017.04.010
Nestler, S., and Back, M. D. (2013). Applications and extensions of the lens model to understand interpersonal judgments at zero acquaintance. Curr. Direct. Psychol. Sci. 22, 374–379. doi: 10.1177/0963721413486148
Nyström, M., Andersson, R., Holmqvist, K., and van de Weijer, J. (2013). The influence of calibration method and eye physiology on eyetracking data quality. Behav. Res. Methods 45, 272–288. doi: 10.3758/s13428-012-0247-4
Oudman, S., van de Pol, J., Bakker, A., Moerbeek, M., and van Gog, T. (2018). Effects of different cue types on the accuracy of primary school teachers' judgments of students' mathematical understanding. Teach. Teach. Educat. 76, 214–226. doi: 10.1016/j.tate.2018.02.007
Praetorius, A.-K., Berner, V.-D., Zeinz, H., Scheunpflug, A., and Dresel, M. (2013). Judgment confidence and judgment accuracy of teachers in judging self-concepts of students. J. Educat. Res. 106, 64–76. doi: 10.1080/00220671.2012.667010
Praetorius, A.-K., Drexler, K., Rösch, L., Christophel, E., Heyne, N., Scheunpflug, A., et al. (2015). Judging students' self-concepts within 30s? Investigating judgement accuracy in a zero-acquaintance situation. Learn. Individ. Diff. 37, 231–236. doi: 10.1016/j.lindif.2014.11.015
Praetorius, A.-K., Koch, T., Scheunpflug, A., Zeinz, H., and Dresel, M. (2017). Identifying determinants of teachers' judgment (in)accuracy regarding students' school-related motivations using a Bayesian cross-classified multi-level model. Learn. Instruct. 52, 148–160. doi: 10.1016/j.learninstruc.2017.06.003
Rimm-Kaufman, S. E., Baroody, A. E., Larsen, R. A. A., Curby, T. W., and Abry, T. (2015). To what extent do teacher–student interaction quality and student gender contribute to fifth graders' engagement in mathematics learning? J. Educat. Psychol. 107, 170–185. doi: 10.1037/a0037252
Roth, B., Becker, N., Romeyke, S., Schäfer, S., Domnick, F., and Spinath, F. M. (2015). Intelligence and school grades: a meta-analysis. Intelligence 53, 118–137. doi: 10.1016/j.intell.2015.09.002
Sabers, D. S., Cushing, K. S., and Berliner, D. C. (1991). Differences among teachers in a task characterized by simultaneity, multidimensional, and immediacy. Am. Educat. Res. J. 28, 63–88. doi: 10.3102/00028312028001063
Santagata, R., and Yeh, C. (2016). The role of perception, interpretation, and decision making in the development of beginning teachers' competence. ZDM Math. Educat. 48, 153–165. doi: 10.1007/s11858-015-0737-9
Schiefele, U., Krapp, A., and Winteler, A. (1992). “Interest as a predictor of academic achievement: a meta-analysis research,” in The Role of Interest in Learning and Development, eds K. A. Renninger, S. Hidi, and A. Krapp (Hillsdale: Erlbaum), 183–212.
Schnitzler, K., Holzberger, D., and Seidel, T. (2020). All better than being disengaged: student engagement patterns and their relations to academic self-concept and achievement. Eur. J. Psychol. Educat. doi: 10.1007/s10212-020-00500-6. [Epub ahead of print].
Schütz, A. C., Braun, D. I., and Gegenfurtner, K. R. (2011). Eye movements and perception: a selective review. J. Vision 11:9. doi: 10.1167/11.5.9
Seidel, T. (2006). The role of student characteristics in studying micro teaching–learning environments. Learn. Environ. Res. 9, 253–271. doi: 10.1007/s10984-006-9012-x
Seidel, T., Jurik, V., Häusler, J., and Stubben, S. (2016). “Mikro-Umwelten im Klassenverband: Wie sich kognitive und motivational-affektive Schülervoraussetzungen auf die Wahrnehmung und das Verhalten im Fachunterricht auswirken [Micro-environments in the classroom: How cognitive and motivational-affective student characteristics affect perception and behavior in class],” in Bedingungen und Effekte guten Unterrichts, eds N. McElvany, W. Bos, H. G. Holtappels, M. M. Gebauer, and F. Schwabe (Münster: Waxmann Verlag), 65–87.
Seidel, T., Schnitzler, K., Kosel, C., Stürmer, K., and Holzberger, D. (2020). Student characteristics in the eyes of teachers: differences between novice and expert teachers in judgment accuracy, observed behavioral cues, and gaze. Educat. Psychol. Rev. 15:98. doi: 10.1007/s10648-020-09532-2
Seidel, T., and Stürmer, K. (2014). Modeling and measuring the structure of professional vision in preservice teachers. Am. Educat. Res. J. 51, 739–771. doi: 10.3102/0002831214531321
Shaffer, D. W., Collier, W., and Ruis, A. R. (2016). A tutorial on epistemic network analysis: analyzing the structure of connections in cognitive, social, and interaction data. J. Learn. Analyt. 3, 9–45. doi: 10.18608/jla.2016.33.3
Shaffer, D. W., Hatfield, D., Svarovsky, G. N., Nash, P., Nulty, A., Bagley, E., et al. (2009). Epistemic network analysis: a prototype for 21st-century assessment of learning. Int. J. Learn. Media 1, 33–53. doi: 10.1162/ijlm.2009.0013
Shaffer, D. W., and Ruis, A. R. (2017). “Epistemic network analysis: A worked example of theory-based learning analytics,” in Handbook of Learning Analytics, eds C. Lang, G. Siemens, A. Wise, and D. Gašević. First edition (Solar Society for Learning Analytics Research), 175–187. doi: 10.18608/hla17.015
Shavelson, R. J., Hubner, J. J., and Stanton, G. C. (1976). Self-concept: validation of construct interpretations. Rev. Educat. Res. 46, 407–441. doi: 10.3102/00346543046003407
Sherin, M. G., and van Es, E. A. (2009). Effects of video club participation on teachers' professional vision. J. Teacher Educat. 60, 20–37. doi: 10.1177/0022487108328155
Shulman, L. S. (1986). Those who understand: knowledge growth in teaching. Educat. Res. 15, 4–14. doi: 10.3102/0013189X015002004
Shulman, L. S. (1987). Knowledge and teaching: foundations of the new reform. Harvard Educat. Rev. 57, 1–21. doi: 10.17763/haer.57.1.j463w79r56455411
Sinatra, G. M., Heddy, B. C., and Lombardi, D. (2015). The challenges of defining and measuring student engagement in science. Educat. Psychol. 50, 1–13. doi: 10.1080/00461520.2014.1002924
Snow, R. E. (1989). “Cognitive-conative aptitude interactions in learning,” in Abilities, Motivation, and Methodology: The Minnesota Symposium on Learning and Individual Differences, eds R. Kanfer, P. L. Ackerman, and R. Cudeck (New York, NY: Lawrence Erlbaum Associates), 435–474. doi: 10.4324/9780203762905
Spinath, B. (2005). Akkuratheit der Einschätzung von Schülermerkmalen durch Lehrer und das Konstrukt der diagnostischen Kompetenz [Accuracy of teachers' assessment of student characteristics and the construct of diagnostic competence]. Zeitschrift für Pädagogische Psychologie 19, 85–95. doi: 10.1024/1010-0652.19.12.85
Star, J. R., and Strickland, S. K. (2008). Learning to observe: using video to improve preservice mathematics teachers' ability to notice. J. Math. Teach. Educat. 11, 107–125. doi: 10.1007/s10857-007-9063-7
Steinmayr, R., and Spinath, B. (2009). The importance of motivation as a predictor of school achievement. Learn. Individual Diff. 19, 80–90. doi: 10.1016/j.lindif.2008.05.004
Stürmer, K., Könings, K. D., and Seidel, T. (2013). Declarative knowledge and professional vision in teacher education: effect of courses in teaching and learning. Br. J. Educat. Psychol. 83, 467–483. doi: 10.1111/j.2044-8279.2012.02075.x
Stürmer, K., Seidel, T., and Holzberger, D. (2016). Intra-individual differences in developing professional vision: preservice teachers' changes in the course of an innovative teacher education program. Instruct. Sci. 44, 293–309. doi: 10.1007/s11251-016-9373-1
Stürmer, K., Seidel, T., Müller, K., Häusler, J., and Cortina, K. (2017). What is in the eye of preservice teachers while instructing? An eye-tracking study about attention processes in different teaching situations. Zeitschrift für Erziehungswissenschaft 20, 75–92. doi: 10.1007/s11618-017-0731-9
Südkamp, A., Kaiser, J., and Möller, J. (2012). Accuracy of teachers' judgments of students' academic achievement: a meta-analysis. J. Educat. Psychol. 104, 743–762. doi: 10.1037/a0027627
Südkamp, A., Praetorius, A.-K., and Spinath, B. (2018). Teachers' judgment accuracy concerning consistent and inconsistent student profiles. Teach. Teach. Educat. 76, 201–213. doi: 10.1016/j.tate.2017.09.016
Thiede, K. W., Brendefur, J. L., Osguthorpe, R. D., Carney, M. B., Bremner, A., Strother, S., et al. (2015). Can teachers accurately predict student performance? Teach. Teach. Educat. 49, 36–44. doi: 10.1016/j.tate.2015.01.012
Urhahne, D., and Zhu, M. (2015). Accuracy of teachers' judgments of students' subjective well-being. Learn. Individ. Diff. 43, 226–232. doi: 10.1016/j.lindif.2015.08.007
Valentine, J. C., DuBois, D. L., and Cooper, H. (2004). The relation between self-beliefs and academic achievement: a meta-analytic review. Educat. Psychol. 39, 111–133. doi: 10.1207/s15326985ep3902_3
van den Bogert, N., van Bruggen, J., Kostons, D., and Jochems, W. (2014). First steps into understanding teachers' visual perception of classroom events. Teach. Teach. Educat. 37, 208–216. doi: 10.1016/j.tate.2013.09.001
van Es, E. A., and Sherin, M. G. (2002). Learning to notice: scaffolding new teachers' interpretations of classroom interactions. J. Tech. Teach. Educat. 10, 571–596.
van Es, E. A., and Sherin, M. G. (2008). Mathematics teachers' “learning to notice” in the context of a video club. Teach. Teach. Educat. 24, 244–276. doi: 10.1016/j.tate.2006.11.005
Wolff, C. E., Jarodzka, H., van den Bogert, N., and Boshuizen, H. P. A. (2016). Teacher vision: expert and novice teachers' perception of problematic classroom management scenes. Instruct. Sci. 44, 243–265. doi: 10.1007/s11251-016-9367-z
Wooldridge, A. R., Carayon, P., Shaffer, D. W., and Eagan, B. (2018). Quantifying the qualitative with epistemic network analysis: a human factors case study of task-allocation communication in a primary care team. IISE Transact. Healthcare Syst. Eng. 8, 72–82. doi: 10.1080/24725579.2017.1418769
Keywords: judgment accuracy, judgment process, lens model, student cue utilization, student engagement, student profiles, epistemic network analysis, eye tracking
Citation: Schnitzler K, Holzberger D and Seidel T (2020) Connecting Judgment Process and Accuracy of Student Teachers: Differences in Observation and Student Engagement Cues to Assess Student Characteristics. Front. Educ. 5:602470. doi: 10.3389/feduc.2020.602470
Received: 03 September 2020; Accepted: 13 November 2020;
Published: 10 December 2020.
Edited by:
Chris Davison, University of New South Wales, AustraliaReviewed by:
Peter Nyström, University of Gothenburg, SwedenHui Yong Tay, Nanyang Technological University, Singapore
Copyright © 2020 Schnitzler, Holzberger and Seidel. This is an open-access article distributed under the terms of the Creative Commons Attribution License (CC BY). The use, distribution or reproduction in other forums is permitted, provided the original author(s) and the copyright owner(s) are credited and that the original publication in this journal is cited, in accordance with accepted academic practice. No use, distribution or reproduction is permitted which does not comply with these terms.
*Correspondence: Katharina Schnitzler, S2F0aGFyaW5hLlNjaG5pdHpsZXJAdHVtLmRl