- Centre for Research in Applied Measurement and Evaluation, University of Alberta, Edmonton, AB, Canada
The objective of the present paper is to propose a refined conception of critical thinking in data-rich environments. The rationale for refining critical thinking stems from the need to identify specific information processes that direct the suspension of prior beliefs and activate broader interpretations of data. Established definitions of critical thinking, many of them originating in philosophy, do not include such processes. A refinement of critical thinking in the digital age is developed by integrating two of the most relevant areas of research for this purpose: First, the tripartite model of critical thinking is used to outline proactive and reactive information processes in data-rich environments. Second, a new assessment framework is used to illustrate how educational interventions and assessments can be used to incorporate processes outlined in the tripartite model, thus providing a defensible conceptual foundation for inferences about higher-level thinking in data-rich environments. Third, recommendations are provided for how a performance-based teaching and assessment module of critical thinking can be designed.
Introduction
In response to the question, how much data are on the internet, Gareth Mitchell from Science Focus Magazine answers the question by considering the overall data held by just four companies - Amazon, Facebook, Google, and Microsoft (https://www.sciencefocus.com/future-technology/how-much-data-is-on-the-internet/). These four companies are estimated to hold a sum total of at least 1,200 petabytes (PB) of online data, which equals 1.2 million terabytes (TB) or 1.2 trillion gigabytes (GB). Neuroscientists propose that the average human brain holds 2.5 PB or 2.5 million GB of information in memory (Reber, 2010), or just over 7 billion 60,000-word books. However, information stored in memory is often subject to error not only from the way it is encoded but also retrieved (Mullet and Marsh, 2016).
Critical thinking requires people to minimize bias and error in information processing. Students entering post-secondary education today may be “digital natives” (Prensky, 2001) but they are still surprisingly naïve about how to critically think about the wealth of digital information available. According to Ridsdale et al. (2015), youth may be quite adept at using digital hardware such as smart phones and apps but they often lack the mindware to think and act critically with the information they access with their devices (Stanovich, 2012). Although this lack of mindware can be observed in the mundane activities of how some first-year undergraduates might tackle their research assignments, it is dramatically illustrated in the political narratives of radicalized young adults (Alava et al., 2017). Young adults are particularly vulnerable to misinformation because they are in the process of developing their cognitive abilities and identities (Boyd, 2014). The objective or rationale for this paper is to propose a refined conception (Ennis, 2016) of critical thinking in data-rich environments. It is the authors’ view that a refined conception is required because data-rich environments have ushered in many cognitive traps and the potential for personal biases to derail critical thinking as traditional understood. The research questions addressed in this conceptual paper are as follows: What can traditional definitions of critical thinking gain by considering explicit inclusion of cognitive biases? How can refined definitions of critical thinking be incorporated into theoretical frameworks for the design of performance assessments?
One of the most recommended strategies for helping young adults analyze and navigate online information is to directly and explicitly teach and assess critical thinking (Alava et al., 2017; Shavelson et al., 2019). However, teaching and assessing critical thinking is fraught with difficulties, including a multitude of definitions, improper evaluation, and studies that incorporate small samples and controls (Behar-Horenstein and Niu, 2011; El Soufi and Huat See, 2019). Aside from these predictable difficulties, new challenges have emerged. For example, the informational landscape has changed over the course of the last 30 years. The rapid increase in quantity coupled with the decrease in quality of much online information challenges the limits of human information processing.
Critical thinking today is primarily conducted in data-rich online environments, meaning that postsecondary students are searching, navigating, and thinking about a virtually limitless number of sources. Oxford University’s Change Data Lab (Roser et al., 2020) writes: “adults aged 18–29 in the US are more likely to get news indirectly via social media than directly from print newspapers on news sites; and they also report being online ‘almost constantly.’” As shown in Figure 1, not only is the total time spent online increasing but the increase is mostly the time spent on mobile phones. As mobile phones are smaller devices, compared to desktops, laptops, and tablets, they can be expected to force even faster navigation and processing of information, which would be expected to increase the odds of error-prone thinking.
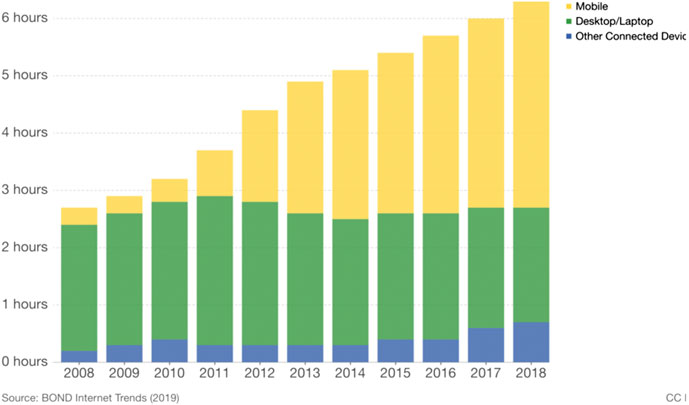
FIGURE 1. Max Roser, Hannah Ritchie, and Esteban Ortiz-Ospina (2020) - Internet. Published online at OurWorldInData.org. Retrieved from: https://ourworldindata.org/internet [Online Resource]; Data source accessed https://www.bondcap.com/report/itr19/. Permission granted under the common creative license.
Cognitive traps are ubiquitous in online data-rich environments. For example, information can be presented as serious and credible when it is not. However, traditional critical thinking definitions have not tended to focus on avoiding cognitive traps; namely, how processing errors can be avoided. This creates a problem not only for teaching but also assessing critical thinking among postsecondary students in today’s classrooms. Thus, there are at least two research opportunities in addressing this problem: 1) provide a refinement of what critical thinking entails specifically for the teaching and assessment of critical thinking in data-rich environments and 2) illustrate a framework for the design of teaching and assessment modules that can lead to stronger inferences about students’ critical thinking skills in today’s information world.
The present paper contributes to the literature on critical thinking in data-rich environments by providing a refinement of what critical thinking entails for teaching and assessment in data-rich environments. The refinement is rooted in cognitive scientific advancements, both theoretical and empirical, of higher-level thinking, and essentially attempts to offer test designers an update on the construct of critical thinking. In other words, this conceptual analysis does the work of translating key psychological aspects of the critical thinking construct for pragmatic purposes–student assessment. Building on the refinement of this construct, the paper also includes recommendations for the type of framework that should guide the design of teaching and assessment modules so that key aspects of students’ critical thinking skills are not missed. Toward this end, this refinement can enhance the construct representation of assessments of critical thinking in data-rich environments. Educational assessments are only as good as their representation of the construct intended for measurement. Without the ongoing refinement of test constructs such as critical thinking, assessments will not provide the most accurate information in the generation of inferences of student thought; refinements of test constructs are especially vital in complex informational landscapes (Leighton and Gierl, 2007). Thus, a refinement of critical thinking among young adults in data-rich environments is developed by integrating two of the most topical and relevant areas of research for this purpose: First, Stanovich and Stanovich’s (2010)tripartite model of critical thinking is used to outline the limitations of human information processing systems in data-rich environments. Second, Shavelson et al.’s (2019) assessment framework is used to illustrate how specific educational assessment designs can be built on the tripartite model and can provide a more defensible evidentiary base for teaching and drawing inferences about critical thinking in data-rich environments. The paper concludes with an illustration of how mindware can be better integrated into teaching and performance-based assessments of critical thinking. The present paper contributes directly to the special issue on Assessing Information Processing and Online Reasoning as a Prerequisite for Learning in Higher Education by refining the conceptualization of critical thinking in data-rich environments among postsecondary students. This refinement provides an opportunity to guide instructive and performance-based assessment programs in the digital age.
Theoretical Frameworks Underlying Mindware for Critical Thinking
In the 1999 science fiction movie MatrixWachowski et al. (1999), human beings download computer “programs” to allow them to think and function in a world that has been overtaken by intelligent machines. Not only do these programs allow human beings to live in a dream world, which normalizes a dystopian reality, but also to effortlessly disregard their colonization. Cognitive scientists propose something analogous to these “programs” for human information processing. For example, Perkins (1995) coined the term mindware to refer to information processes, knowledge structures, and attitudes that can be acquired through instruction to foster good thinking. Rizeq et al. (2020, p. 2) indicate contaminated mindware as “beliefs that may be unhelpful and that may inhibit reasoning processes … (Stanovich, 2009; Stanovich et al., 2008; Stanovich, 2016).”
Treating human information processing as analogous to computer programs, which can be contaminated, is useful and powerful because it highlights the presence of errors or bugs in thinking that can invariably distort the way in which data are perceived and understood, and instantaneously “infect” the thinking of both self and others. However, the predictability of such programs also permits anticipating when these thinking errors are likely to occur. Educational interventions and assessments can be designed to capitalize on the predictability of thinking errors to provide a more comprehensive level of thinking instruction and evaluation. Specifying what critical thinking entails in data-rich environments requires explicit attention not only to the information processes, knowledge structures, and attitudes that instantiate good critical thinking but also to the thinking bugs that derail it. Hyytinen et al. (2019, p. 76) indicate that a critical thinker needs to have knowledge of what is reasonable, the thinking skills to evaluate and use that knowledge, as well as dispositions to do so (Facione, 1990; Halpern, 2014; Hyytinen et al., 2015).” We agree but we would go further in so far as critical thinkers also need to know what their own biases are and how to avoid cognitive traps (Toplak and Flora, 2020).
Traditional Definitions of Critical Thinking
Established or traditional definitions of critical thinking have typically focused on the proactive processes that comprise critical thinking (Leighton, 2011). Proactive processes are positive in action. Proactive processes, such as analyzing and evaluating, are often the focus of educational objectives (e.g., Bloom’s taxonomy; Bloom, 1956). Proactive processes help to identify the actions and goals of good thinking in ideal or optimal conditions. However, they are not particularly useful for creating interventions or assessments intended to diagnose faulty thinking (Leighton and Gierl, 2007). The problem is that these processes reflect only aspects of good thinking and do not reflect other processes that should be avoided for good thinking to occur. For example, reactive thinking processes such as neglecting and confirming must be resisted in order for proactive processes do their good work. Reactive processes are not bad in many circumstances, especially those where thinking has to be quick to avoid imminent danger (Kahneman, 2011). However, in circumstances where imminent danger is not present and actions can be enhanced by careful processing of information, it can be useful to learn about reactive processes; this is especially relevant for designing teaching interventions and assessments of critical thinking (Leighton, 2011).
The omission of reactive processes in traditional definitions of critical thinking is perhaps not surprising since many of these definitions grew out of philosophy and not out of empirical disciplines such as experimental psychology (Ennis, 2015, Ennis, 2016). Nonetheless, this section addresses established definitions in order to provide a conceptual foundation on which to build more, targeted definitions of critical thinking for specific purposes.
Proactive Processes in Critical Thinking
Ennis (2016) provides a justification for distinguishing the basic concept of critical thinking from a particular conception of it; that is, a particular definitional instance of it in specific situations. In an analysis of the many theoretically inspired definitions of critical thinking, Ennis (2016, p. 8) explains that many established definitions share a conceptual core. To illustrate this core, consider three definitions of critical thinking outlined in Ennis (2016, p.8-9):
1. “Active, persistent, and careful consideration of any belief or supposed form of knowledge in the light of the grounds that support it and the further conclusions to which it tends” (Dewey, 1933, p. 9 [first edition 1910]).
2. “Purposeful, self-regulatory judgment which results in interpretation, analysis, evaluation, and inference, as well as explanation of the evidential, conceptual, methodological, criteriological, or contextual considerations upon which that judgment is based” (Facione 1990; Table 1).
3. “Critical thinking is skilled, active interpretation and evaluation of observations, communications, information, and argumentation as a guide to thought and action” (Fisher and Scriven 1997, p. 20).
These three examples illustrate what Ennis (2016, p. 11) considers to be the defining processes of critical thinking, namely, “the abilities to analyze, criticize, and advocate ideas” and “reach well-supported … conclusions.” These proactive processes represent the conceptual core.
Aside from the conceptual core, Ennis (2016) suggests that variations or distinct conceptions of critical thinking can be proposed without endangering the core concept. These variations arise from particular teaching and assessment situations to which the core concept is applied and operationalized. For example, in reviewing four different examples of particular teaching and assessment cases [i.e., Ennis’s (1996) Alpha Conception, Clemson’s (2016) Brief Conception, California State University (2011), and Edward Glaser’s (1941) Brief Conception of Critical Thinking], Ennis (2016) explains that in each case the concept of critical thinking is operationalized to have a particular meaning in a given context. Ennis (2016) concludes:
In sum, differences in the mainstream concept [of critical thinking] do not really exist, and differences in conceptions that are based on the mainstream concept of critical thinking are usually to a great extent attributable to and appropriate for the differences in the situations of the people promoting the conception. (p. 13)
Building on Ennis’ (2016) proposal, then, a conception of critical thinking is offered herein to serve a specific purpose: to teach and assess critical thinking skills in data-rich environments. To do this, the core concept of critical thinking must include those information processes that guard against manipulability in data-rich environments.
Reactive Processes in Critical Thinking
Educational interventions and assessments must address reactive processes if they are to bolster critical thinking in non-idealized conditions. This is especially important in data-rich environments where information is likely to be novel, abundant (almost limitless), and quickly accessible. The tendency for people to simplify their information processing is amplified in data-rich environments compared to data-poor environments where information is routine and can be comfortably processed serially (e.g., writing a term paper on a familiar topic with ample time allowance). The simplification of data is necessary as the human brain only processes about 5–7 pieces of information in working memory at any one time (Miller, 1956; see also; Cowan, 2001). This limitation exists atop the more basic limitation of what can be consciously perceived in the visual field (Kroll et al., 2010). Thus, human beings instinctively simplify the signals they receive in order to create a manageable information processing experience (Kroll et al., 2010).
Most of the information simplified and perceived will be forgotten unless it is actively processed via rehearsal and transfer into long-term memory. However, rehearsed information is not stored without error. Storage contains errors because another limitation of information processing is that memory is a constructive process (Schacter, 2012). What is encoded is imbued with the schemata already in memory, and what is then retrieved depends on how the information was encoded. Thus, aside from the error-prone simplification process that permits the human information process to perceive successful navigation of the environment, there is the error-prone storage-and-retrieval process that characterizes memory. Data-rich environments accentuate these significant limitations of human information processing. Consequently, identifying both proactive and reactive information processes is necessary to generate realistic educational interventions and assessments that can help 1) ameliorate thinking bugs in today’s data-rich environments while at the same time 2) cultivating better mindware for critical thinking.
The Tripartite Model of Critical Thinking
One of the largest problems with modern initiatives to teach and assess critical thinking in data-rich environments is the neglect of empirically based theoretical frameworks to guide efforts (Leighton, 2011). Without such frameworks, the information processes taught and measured are primarily informed by philosophical instead of psychological considerations. The former emphasizes proactive over reactive processes but both are needed. The emphases on proactive processes does not actually help educators identify and rectify the existing bugs in students’ mindware.
The conception of critical thinking that is advanced here is based on Stanovich and Stanovich’s (2010; see also Stanovich, 2021) Tripartite Model. The model focuses on both proactive and reactive processes. Unlike philosophical treatments of critical thinking, the tripartite model devotes significant attention to biased and error-prone information processing. According to Stanovich and Stanovich (2010, p. 219; italics added): “the tendency to process information incompletely has been a major theme throughout the past 30 years of research in psychology and cognitive science (Dawes, 1976; Taylor, 1981; Tversky and Kahneman, 1974).” The tripartite model does not provide a simple definition of what critical thinking entails given the complexity of the processes involved. Instead, it provides an outline of three levels of mindware that have been found to be constantly interacting in the process of critical thinking.
Three Levels of the Mind
The tripartite model integrates decades of cognitive and neuroscientific research, ranging from Tversky and Kahneman’s (1974) early work on biases and heuristics to the later work on dual process models of thinking (Evans, 2003). The model shown in Figure 2 illustrates the relations between three distinct levels of information processing–the reflective mind (RM), the algorithmic mind (AM), and the autonomous mind (AUM). In Figure 2, the level of information processing that functions to manipulate data in working memory, store, retrieve, and generate responses is the AM. This is the level that is directly on display and observed when human beings process and respond to questions, for example, on educational assessments and tests of intelligence. The AM can be defined by its processing speed, pattern recognition and retrieval from long-term memory, and manipulation of data in working memory.
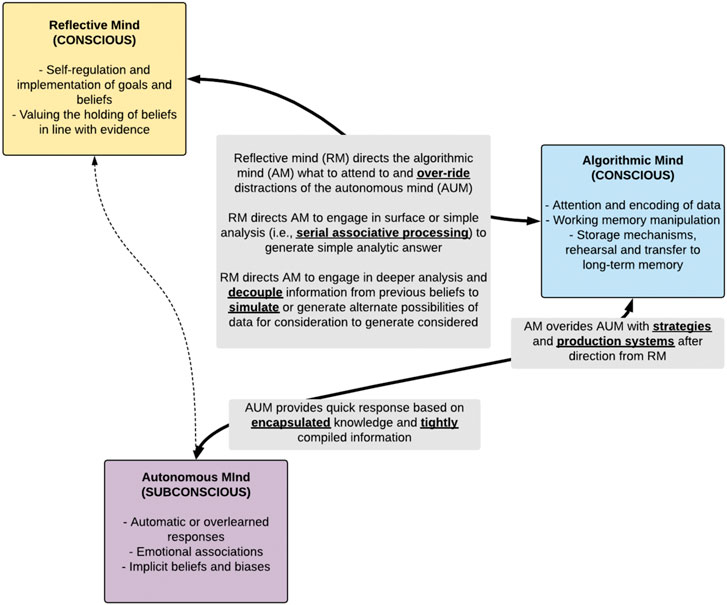
FIGURE 2. Adapted tripartite model (Stanovich and Stanovich, 2010) to illustrate the connections among three different aspects or minds integral to human cognition.
The AM takes direction from two sources–the reflective mind or RM and the autonomous mind or AUM. The AUM is the subconscious part of human information processing that retains data acquired by means of imprinting, tacit and procedural learning, and emotionally laden events, resulting in many forms of automatic responses and implicit biases. The AUM is the level at which encapsulated or modularized knowledge can be retrieved to generate a quick and simplified response, which exerts minimal load on working memory. Depending on the influence of the AUM, the AM is capable of biased or unbiased responses. For example, in view of what appears to be a large insect, the AUM signals the AM to focus on getting out of the way. This is a biased response but it is an expedient response that is often observed in logical tasks (see Leighton and Dawson, 2001).
Unlike the AUM, the RM is a conscious and deliberative aspect of human information processing. The RM is the part of information processing that involves goals, beliefs, and values. It is the part of the mind that provides intentionality to human behavior (Dennett, 1987). It directs the AM to suspend simple processing and expend the cognitive effort to deeply process information. The RM also functions to direct the AM to resist or override signals from the AUM to respond too quickly. Thus, it is the information processing directed by the RM–to engage and suspend certain processes - that needs to be the focus of most educational interventions and assessments of critical thinking.
Decoupling and Simulation Processes
According to Stanovich and Stanovich (2010), the RM directs the AM to engage in two forms of proactive information processes. Both require cognitive effort. First, decoupling involves the process of suspending prior beliefs and attending to information in the context in which it is provided. For example, decoupling processes have been examined in belief bias studies (Leighton and Sternberg, 2004). In these studies, participants are typically asked to evaluate arguments that have been created to differ along two dimensions–logical soundness and believability of conclusion. For example, a logically flawed argument is paired with a believable conclusion, for example, All politicians are liars; All crooks are liars; Therefore, all politicians are crooks. In response to these types of arguments, participants have been found to accept conclusions that are believable rather than logically sound. However, performance can be improved by instructing participants to explicitly consider the structure of the argument. In other words, the instructions are clearly designed to engage the RM. When explicit instructions are included, participants will show improved performance in correctly rejecting conclusions from flawed arguments.
Second, for decoupling to work, simulation is often activated in tandem. Simulation involves the process of actively considering distinct ways of interpreting information. For example, shown in Figure 3 are two panels showing distinct interpretations of the premises of the argument provided earlier about politicians and crooks. The one on the left shows the easiest interpretation or mental model of the argument about politicians (conclusion - All politicians are crooks). The interpretation shown on the left is one which often may correspond to prior beliefs. On the right, an additional interpretation can be created to indicate that no politicians are crooks. The interpretation shown on the right may be less common but equally plausible given the premises of the argument. The effort to create additional interpretations or simulate information that contradicts prior beliefs has been found to correlate positively with working memory capacity (Johnson-Laird and Bara, 1984). In fact, both decoupling and simulation have been found to require significant working memory resources and, thus, cognitive effort for participants to willingly adopt (Johnson-Laird and Bara, 1984; Leighton and Sternberg, 2004; Stanovich, 2011; Leighton and Sternberg, 2012).
Most classroom assessments and achievement tests, even those that are purportedly designed to be cognitively complex, are not developed to evaluate whether students can decouple or simulate thinking (Leighton, 2011). Instead most tests are developed to measure whether students can reproduce what they have learned in the classroom, namely, a form of optimal performance given instruction (Leighton and Gierl, 2007; Stanovich and Stanovich, 2010). Often, then, there is little incentive for students to begin to suspend beliefs and imagine situations where what they have been told does not hold. Not surprisingly, most students try to avoid “overthinking” their responses on multiple-choice or even short-answer tests precisely because such simulated thinking could lead to choosing an unexpected or non-keyed response.
Suspending Serial Associative Processing
Unlike the thinking evoked by most classroom and achievement tests, information processing in data-rich environments calls for a different standard of evaluation. Data-rich environments typically offer students the possibility to navigate freely through multiple sites, unrestricted by time limits and/or instructions about how their performance will be evaluated. In such open, data-rich environments, individuals set their own standard of performance. According to the tripartite model, serial associative processing is likely to be the standard most often set by individuals. Serial associative processing is directed by the RM but it is simple processing nonetheless. It means that information is accepted as it is presented or rejected if it fails to conform with what is already known (prior beliefs). There is no decoupling or simulation. Johnson-Laird and Bara (1984; Johnson-Laird, 2004) called this simple type of processing single-model reasoning because information is attended and processed but goes unchallenged. Serial associative processing is different from the automatic responses originating in the AUM. Serial associative processing does involve analysis and evaluation but it does not consider multiple perspectives and so it is biased in its implementation.
Critical Thinking as Coordinated Suspension and Engagement of Information Processes
Consider again the defining processes Ennis (2016, p. 11) proposes for critical thinking: “the abilities to analyze, criticize, and advocate ideas” and “reach well-supported … conclusions.” In light of Stanovich and Stanovich’s (2010) model, the processes mentioned by Ennis only reflect the AM and do not reflect the coordinated effort of the RM and AM to suspend serial associative processing and engage in decoupling and simulation. In other words, what is missing in most traditional conceptions of critical thinking are reactive processes, namely, processes that lead thinking astray such as serial associative processing, which must be suspended for better thinking to emerge.
In data-rich environments, actively resisting serial associative processing is a necessary component of critical thinking. This form of information processing must be actively resisted because the incentive is for individuals to do the opposite in the wake of massive amounts of information. Although applying this resistance will be cognitively effortful, it can be learned by teaching students to become more meta-cognitively aware of their information processing. However, even meta-cognitive awareness training is unlikely to help students resist serial associative processing, if critical thinking is under-valued by the RM. Thus, the design of teaching interventions and assessments must consider the construct of critical thinking not as a universally accepted and desired form of thinking but as a skill that students choose to apply or ignore (Leighton et al., 2013). Consequently, interventions must persuade students of the benefits associated with critical thinking and assessments need to measure the processes that are most relevant for critical thought (e.g., decoupling and simulation). In the next section, Shavelson et al.’s (2019) assessment framework is used to illustrate how specific educational assessment designs can build on the tripartite model of critical thinking, and provide a more defensible conceptual foundation for inferences about critical thinking in data-rich environments.
Measuring Decoupling and Simulation: Shavelson et al.’s (2019) Assessment Framework
Shavelson et al.’s (2019) assessment framework is premised on three objectives. First, performance assessments are appropriate for measuring higher-level thinking constructs; second, assessments of higher-level thinking constructs should be developed in ways that clearly link scores to claims about postsecondary students’ capabilities; and third, higher-level thinking constructs, such as critical thinking, should require postsecondary students to make sense of complex information outside typical classroom environments. Each of these objectives is elaborated and connected to measuring key information processes for critical thinking.
Performance Assessments
Performance assessments typically contain tasks (i.e., selected and constructed) that require test-takers to attend to multiple types of materials (e.g., articles, testimonials, videos) and generate responses that involve an evaluation of those materials for the purpose of providing a reasoned answer on a topic. The topic is often novel and the tasks are complex such as evaluating a claim about whether a privately funded health-care clinic should be adopted by a community. The goal of a performance assessment is to approximate the informational demands of a real-world situation, calling on individuals to have to weigh different perspectives in the process of analyzing and evaluating materials.
The motivation to approximate real-world situations is a requirement in performance assessments. The constructs measured need to be assessed in the types of situations that justify making claims about what the test-taker can do in a context that approximates real-life. For example, performance assessments would not be the tool to use if the objective was to measure criterion or optimal performance (Stanovich and Stanovich, 2010), that is, whether someone has learned the normative timeline for the Second World War or to factor polynomials. Both of these objectives do not reflect the types of skills required in complex environments, where typical performance is sought in determining whether the test-taker can invoke and manage specific information processes in providing a response.
Measuring the Mindware
In Shavelson et al.’s (2019, p. 4) framework, the environments or contexts in which to measure critical thinking are broadly conceived:
a. contexts in which thought processes are needed for solving problems and making decisions in everyday life, and
b. contexts in which mental processes can be applied that must be developed by formal instruction, including processes such as comparing, evaluating, and justifying.
In considering both these measurement contexts, data-rich environments satisfy both. For example, the real-life contexts in which people must solve problems and make decisions nowadays typically involve seeking, analyzing, and evaluating a lot of information. Most of this information may be online where there is almost no oversight on quantity or quality control.
However, people do not solve problems and make decisions in a cognitive vacuum. This is where Stanovich and Stanovich’s (2010) tripartite model provides the necessary conceptual foundation to Shavelson et al.’s (2019) assessment framework for measuring critical thinking. The beliefs and values of the RM direct the type of information that is sought and how that information should be analyzed. Heretofore, the idea of values has not been elaborated. The valuing of critical thinking or stated differently, holding the value that beliefs should line up with evidence provides an impetus for engaging in effortful thinking. Churchland (2011) indicates that such values–what we consider good, bad, worthwhile or not–are rooted in the brain and have evolved as mechanisms to help human beings adapt and survive. Thus, the question for the reflective mind is one of why is critical thinking beneficial for me? Consequently, the design of performance assessments must include opportunities for measuring two fundamental catalytic processes for critical thinking: (a) whether the RM values critical thinking and for what reasons and (b) how the RM then directs the AM to engage or suspend serial associative processing for analyzing and evaluating the resources provided so that critical thinking can be achieved. The reason for measuring whether the RM values critical thinking is to establishing that a student is indeed motivated to engage in the effort it requires. A student may value critical thinking but not know how to do it, but it is also necessary to determine whether a student does not value it and therefore, irrespective of having the skills to do it, chooses not to do it. The educational intervention for each of these scenarios will be different depending on the cognitive and affective state of the student (Leighton et al., 2013).
The question of how this engagement or suspension is measured is not trivial as it would involve finding a way to measure test-takers’ epistemic values, prior beliefs, and biases about the topic. Moreover, it would involve providing confirming or disconfirming sources of data in the assessment at different levels of quality. As test-takers select data sources to analyze and evaluate, evidence of the active suspension of prior belief (i.e., decoupling) and rejection of information at face value (i.e., simulation) needs to be collected to warrant the claim that the information processes inherent to critical thinking were applied.
Creating the Performance Assessment
According to Evidence Centered Design (ECD; Mislevy et al., 2003), an assessment is most defensibly designed by paying careful attention to the claim that is expected to be made from the assessment performance. In the case of Shavelson et al.’s (2019) assessment framework, the following high-level claim is desired:
[T]he assessment task presented here taps critical thinking on everyday complex issues, events, problems, and the like. The evidence comes from evaluating test-takers’ responses to the assessment tasks and potential accompanying analyses of response processes such as think-aloud interviews or log file analyses. (p. 9)
Because the claim includes ‘critical thinking on everyday complex issues, events, problems, and the like’ it becomes necessary to situate this claim within the specific data-rich environment that is of most interest to the developer but also the environment that is of most interest to the test-taker. In data-rich environments, thinking will not be general but specifically guided by the relevance of topics. In particular, what is essential to consider in such environments is that individuals are unconstrained by how they search and attend to information given the vast quantity and quality of sources. Thus, test takers’ value proposition of thinking critically for a given topic needs to be considered in their performance. If respondents do not value it, they are unlikely to engage in the effort required to suspend serial processing. And claims about what they can or cannot do will be less defensible.
At the outset of a performance assessment, a test-taker who does not value critical thinking for a given topic is unlikely to engage the critical information processes expected on the assessment. The following four facets of the data that Shavelson et al. (2019) indicate must be attended are unlikely to be invoked in depth:
1. Trustworthiness of the information or data—is it reliable, unreliable, or uncertain?
2. Relevance of the information or data—is it relevant or irrelevant to the problem under consideration?
3. Manipulability of the information to judgmental/decision/bias—is the information subject to judgmental errors and well-known biases?
4. Solution to the story problem—is the problem one where a judgment can be reached, a decision recommended, or a course of action suggested?
Each of these facets forms the basis of a question that is designed to direct the algorithmic mind (AM) to process the data in a particular way. However, the AM is an information processor that does not direct itself; it is directed by the RM. Consequently, for each of these facets, it is important to consider that both the RM and the AM are being induced and measured. For example, if critical thinking is to be demonstrated, all facets–trustworthiness, relevance, manipulability, and solution generation–require the RM to direct the AM to (a) override the autonomous mind (AU) in its reactionary response, (b) suspend serial associative processing, (c) decouple from pre-existing beliefs, and (d) simulate alternative worlds where the information is considered in the context in which it is presented. Although it is beyond the scope of the paper to illustrate the interplay of the RM and AM for each of these four facets, an example may suffice. Consider a critical thinking task that begins with a story about the delivery of a new vaccine for inoculating people against the COVID19 virus. After presentation of the story, the first item needs to probes the RM - whether the test-taker indicates importance in comprehending a story about vaccine safety. If the test-taker responds “yes,” the self-report can be validated against eye tracking reaction time data to check its validity (assuming greater importance would lead to more time spent reading). The second set of items can then probe the test-taker’s analysis of the trustworthiness of the information, for example, is the story reliable and how do you know? What information was irrelevant (e.g., the color of the viles) and was it decoupled from relevant information (e.g., the temperature at which the vaccine must be stored)? What variables in the story were re-imagined or simulated (e.g., transportation of a vaccine across multiple freezers might erode its integrity), leading to a different conclusion than the one stated in the story. The response to these second set of items must be evaluated, in aggregate, against the response for the first item in order to determine the rigor of AM thinking devoted to analyzing the veracity of the story and it elements. If the second response is weak, in light of a motivated RM, then one might generate the inference that the test-takers lacks the essential skills to think critically.
The induction of the RM to engage the AM in a specific manner in a performance assessment becomes an integral part of the critical thinking construct that is being measured in data-rich environments. In fact, one of the most important questions to be presented to test-takers before they engage with a performance measure of critical thinking might be a question that directly probes the RM to reveal the goals that drive its performance–does the RM value holding beliefs that are in line with evidence? In the absence of inducing the RM to accept the objective of the performance assessment, the RM’s direction of the AM will simply reflect the least effortful course of thinking.
Shown in Figure 4 are examples of preliminary questions to ask the respondent at the initiation of the performance assessment. These would be required to measure the meta-cognitive approach adopted by the test-taker in the specific data-rich environment in which the performance assessment is embedded. By incorporating preliminary questions into the design of the assessment such as how do you define critical thinking and do you value it, the assessment yields two sources of evidentiary data about the test-taker: First, what do they believe critically thinking entails? And second, are they motivated to demonstrate this type of thinking, namely, the construct of interest? Both these sources of data about the test-taker would help in the interpretation of their assessments results. If test-takers can define critical thinking but do not value it or are not willing to suspend associative serial processing, low scores may only reveal their lack of interest or motivation. The latter of which becomes a key challenge for educational interventions unless the reasons for its benefits can be shown.
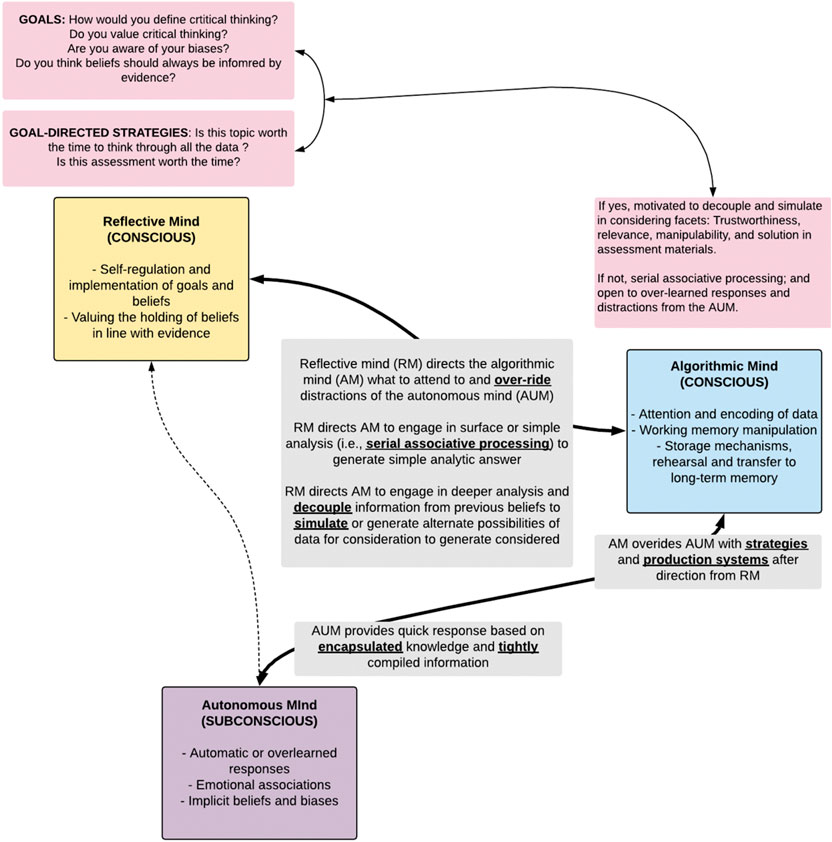
FIGURE 4. Refining the connections among three different aspects or minds (Stanovich and Stanovich, 2010) integral to engaging facets of critical thinking on performance assessments (Shavelson et al., 2019) in data-rich environments.
Moving Beyond Just Teaching Critical Thinking Skills
How well educators are poised to teach and assess critical thinking in data-rich environments might depend less on a specific instructional formula and more on how it is incentivized for students. In other words, there needs to be a clear message to students about what it is that they gain by suspending personal biases and engaging analytical strategies; for example, “Did you know that by becoming aware that you are reacting positively to the flashiest site of health information you may not be getting the best information? Or “Did you know that in searching for information about a political issue you will typically be drawn to information that confirms your prior beliefs? If you want to be fully prepared for debates, try searching for information that challenges what you believe so you can be prepared for both sides of the argument.”
A shortcoming with almost all assessments of critical thinking as of the writing of this paper is that they are designed from traditional definitions of critical thinking; meaning that these assessments do not test for cognitive biases explicitly. For example, the Halpern Critical Thinking Assessments (Butler, 2012) measure five dimension of critical thinking premised on traditional conceptions of critical thinking (i.e., verbal reasoning, argument analysis, thinking as hypothesis testing, likelihood/uncertainty, and decision making and problem solving) but not cognitive biases. Another popular critical thinking test is the Cornell Critical Thinking Level Test Z (Ennis and Millman, 2005) which measures induction, deduction, credibility, identification of assumptions, semantics, definitions, and prediction in planning experiments. However, all these attributes are proactive and not reactive. Only measuring proactive attributes can almost be viewed, ironically, as yet another instance of our tendency to confirm biases. What is needed is actively falsifying what we believe–testing the limits of what we want to think is true. There are at least two notable exceptions to the typical critical thinking tests. One is the Cognitive Reflection Test (Frederick, 2005), which measures a person’s skill at reflecting on a question and resisting answering with the first response that comes to mind. In essence, this test measures reactive processes. The other is the Comprehensive Assessment of Rational Thinking (CART) by Stanovich (2016). The CART is focused on measuring the preponderance and avoidance of thinking errors or contaminated mindware. For example, the CART contains 20 subtests that assess tendencies toward overconfidence, showing inconsistent preferences and being swayed by irrelevant information. Critical thinking tests designed to measure avoidance of reactive processes are relatively new and perhaps not surprisingly there are no large-scale studies of whether it can be effectively taught. It is for this reason that the work we present here is necessary and we believe presents a contribution to the literature.
Proactive critical thinking can be taught so there is no reason to think that awareness of reactive critical thinking cannot also be taught. To be sure, most of the research on teaching critical thinking skills has been in the area of proactive skills. A meta-analysis of strategic approaches to teaching critical thinking uncovered that various forms of critical thinking can be taught with measurable positive effects (Abrami et al., 2015). However, the average effect size of educational interventions was 0.30 (Cohen’s d); thus, weak to moderate at best (Cohen, 1977; Abrami et al., 2015). Part of the challenge is that critical thinking, like any other disposition and/or skill, takes time to cultivate and uptake is determined by how well the audience (students) buys into what is being taught.
One would expect different approaches for teaching critical thinking depend not only on the specific goal of instruction but also how well students believe in the benefits articulated. For example, Lorencová et al. (2019) conducted a systematic review of 39 studies of critical thinking instruction in teacher education programs. The most often cited targeted skills for instruction were analysis and evaluation. A majority of the educational interventions had the following characteristics: (a) took place during a course in one semester with an average number of 66 students, (b) were face-to-face, (c) used infusion (i.e., critical thinking added as a separate module to existing curriculum), or immersion (i.e., critical thinking integrated into the full curriculum) as the primary context for instruction with (d) discussion and self-learning as tools for pedagogy. The most frequently used standardized assessment tool for measuring learning gains was the CCTDI or California Critical Thinking Disposition Inventory, which is a measure of thinking dispositions instead of actual critical thinking performance.
In addition to the CCTDI, most instructors also developed their own assessments, including assessment of typical case studies, essays, and portfolios. Most of the 39 studies reviewed showed fully positive or some positive results; only 3 studies reported null results. Not surprisingly, however, larger effects between pre- and post-intervention were observed for studies employing instructor-created, non-standardized tools compared to standardized assessment tools.
One of the biggest challenges identified by Lorencová et al. (2019) is not with the interventions of critical thinking but with assessments to measure gains. Instructor-developed assessments suffer from a variety of problems such as demand characteristics, low reliability, and potentially biased grading. Thus, little can be concluded about what reliably works among the many strategies for critical thinking without good measures. A related problem is that many of these interventions do not indicate how long the effects last; good measures are also required to gauge the temporal effects of interventions. Additional problems that often plague intervention studies involve relatively small sample sizes. These challenges may be overcome in a variety of ways. For example, moving away from idiosyncratic instructor-developed critical thinking assessments and moving toward the establishment of a consortia of researchers that can pool their items for review, field-testing, refinement and ultimately leverage large enough samples to establish reliable norms for inferences. Toward this end, Shavelson et al. (2019) exemplify this work in their International Performance Assessment of Learning (iPAL) consortium.
In another recent review of critical thinking interventions in professional programs in the social sciences and STEM fields, Puig et al. (2019) noted the prevalence of unstandardized forms of assessments for measuring critical thinking, most of which were qualitative. For example, Puig et al. (2019, p. 867) indicate that most of the studies they reviewed based their results largely on “the opinions of students and/or teachers, as well as on other factors such as students’ motivation, or their level of engagement to the task... students’ perceptions, learning reflections and their participation in the task, and others even did not assess CT.” These measures may begin to probe the values and beliefs of the RM but they ignore the information processes of the AM in instantiating critical thinking.
Schmaltz et al. (2017) indicate that part of the reason educators at all levels of instruction, including postsecondary institutions, find it so challenging to teach critical thinking is that it is not well defined and there are not enough empirical studies to show what works. Although the deficits raised by Schmaltz et al. (2017) are justified, the problem of showing what works requires measuring human behavior with minimal bias. Thus, the deficits identified by Schmaltz et al. (2017) may actually reside more with the assessments used to evaluate interventions than with the interventions themselves. Just as there many ways to teach algebra or essay composition successfully depending on the students involved, so must teaching critical thinking take on different methods as shown in the literature (e.g., Abrami et al., 2015; Lorencová et al., 2019). However, focusing so intently on the specific characteristics of educational interventions may hurt more than it helps if it distracts from the assessments that need to be designed to measure changes in thinking. In whatever form critical thinking is taught, what is certainly needed are assessments that reliably measure the construct of critical thinking, however it has been conceptualized and operationalized (Ennis, 2016).
Teaching and Assessing Critical Thinking in Data-Rich Environments
Teaching and assessing critical thinking in data-rich environments requires not only a conception of what critical thinking entails in such environments but also an adequate assessment of the information processes associated with this type of thinking. Building first on Stanovich and Stanovich’s (2010) tripartite model, the instructional goals must include (a) students becoming self-aware of what types of thinking they value and in what circumstances and (b) students learning to apply strategies they believe are valuable in thinking critically in identified circumstances. The premise is this: If critical thinking is valued for a given topic, strategies such as decoupling and simulation can be explicitly taught, taken up by students, practiced and assessed using online information sources and tasks. This is also where Shavelson et al.’s (2019) framework provides an excellent assessment foundation to structure teaching and assessment modules. Prompts and performance tasks can be embedded throughout digital modules to assess students’ goals for information processing, strategies for searching and analyzing data tables, reports, and graphs for the stated goals, time spent on different informational resources, and evaluation of conclusions.
Teaching and assessment modules for critical thinking must motivate students to expend the cognitive resources to suspend certain information processes (e.g., serial associative processing). As previous reviews have found (e.g., Lorencová et al., 2019), motivation is a pre-requisite to decouple and simulate as these are cognitively taxing forms of processing. In the pre-development stage of any teaching or assessment form, one of the most important tasks is to survey the population of students about interests warranting critical thinking. Then, digital teaching modules and assessments can be designed around topics that would motivate students to expend the resources needed to engage with tasks; for example, the effects of social media on mental health, the cost and value of postsecondary education or even a learning disability can be used as topics to spark the interest of students. Starting from a position of awareness about the topics that warrant attention, students can be invited to learn about resisting serial associative processing in the collection of data (e.g., finding high-quality data that are relevant but opposed to what is believed about a topic), decoupling in the analysis of conclusions (e.g., looking at statistics that do not misrepresent the data), and simulation in evaluations of conclusions (e.g., weighing the evidence in line with its quality).
However, incentivizing students to pay attention to what they are processing does not mean it will be processed critically. Especially when topics are of interest, individuals are likely to hold strong opinions and seek to actively confirm what they already believe. Thus, teaching modules must begin with a process of having students become aware of a bias to confirm, and invoking reminders to students that this bias can surface unless it is constantly under check in their self-awareness. For example, a prompt for students to become aware of their biases can be integrated into the introductory sections of a teaching and assessment module. Prompts can also be designed to remind them of the critical thinking they have indicated they value. Previously presented information processes (e.g., suspending prior beliefs or decoupling) can be flashed as reminders in searching, assessing task information, and evaluating conclusions. Another option might be to have students choose to assume the perspective of a professional such as a journalist, a lawyer, or a counselor and to challenge them to process information as that professional would be expected to do.
Consider the following screenshot in Figure 5 from a storyboard associated with the design of a teaching and assessment module on autism. Following an introductory screen, participating students are advised in the second screen that they are going to be learning about ways to collect, manage, evaluate, and apply data on autism. The third screen introduces them to the research project and poses initial questions they are unlikely to be able to answer critically. The fourth screen introduces them to potential data sources, such as an online report from a mainstream news channel and a report from a national statistics agency. At this point, students can be prompted to rate the trustworthiness of the sources, which reflects the first facet of Shavelson et al.‘s framework. In the fifth screen, students navigate to the data source(s) selected. Irrespective of the data source selected, students are probed on the manipulability and relevance of the data source, and how it advances the investigation. At each point during the module, students are scaffolded in evidence-based learning about autism and asked to provide responses designed to reveal their chosen information processing. For example, in the fourth screen where students are asked to list the data sources for autism, the sources students indicate can be categorized according to at least two dimensions. First, is each source trustworthy? Relevant? Second, how much time and effort did students spend analyzing the sources (using reaction time data). If students appear to carefully choose what they think are trustworthy and relevant sources but do not ascribe the trustworthiness or relevance to substantive criteria, then students may value critical thinking (RM) but do not have the knowledge or skills to properly direct this value (RM) in their information processing. In this case, the scaffolding comes in the form of an instructional part of the module that explains the criteria that should be used for judging reliability, and relevancy in the case of neurodevelopmental disorders such as Autism.
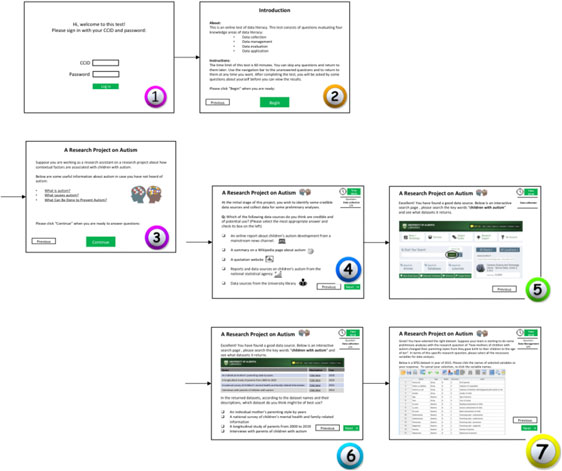
FIGURE 5. Example of story board to illustrate the design of a digital teaching and assessment module of critical thinking in the area of autism.
The storyboard shown in Figure 5 does not show how students’ potential bias may be assessed at the beginning of the module. However, opportunities to bring bias into students’ awareness can be inserted as is shown in the fourth screen in Figure 6. Following this fourth screen, another screen (not shown) could be inserted to teach students what it means to decouple and simulate in the process of information processing. For example, the instructional module can show why an uncontrolled variable (e.g., a diet supplement) should be decoupled from another variable that was controlled (e.g., age of the mother). In this way, students are reminded of their biases (e.g., diet supplements are bad for you), instructed on what it means to think critically in an information-rich environment and also prompted to decide whether such strategies should be applied in considering data during the assessment.
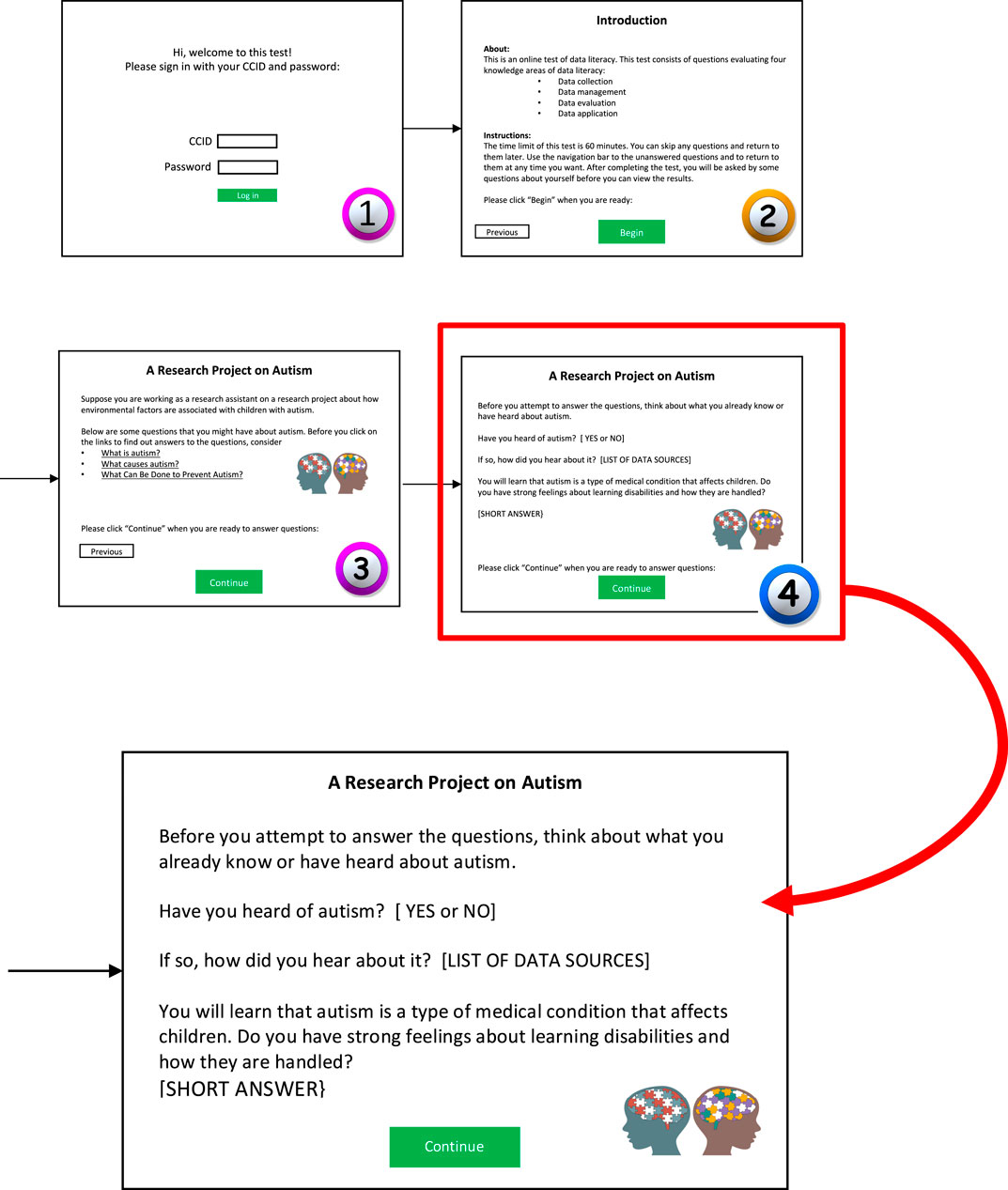
FIGURE 6. Example of how to insert a probe for students to consider their own biases about the topic of autism.
Discussion and Conclusion
New ways of teaching and assessing critical thinking in data-rich environments are needed, given the explosion of online information. This means employing definitions of critical thinking that explicitly outline the contaminated mindware that should be avoided in data-rich environments. The democratization of information in the digital age means that anyone, regardless of qualifications or motivation, can share stories, ideas, and facts with anyone who is willing to read, watch, and be convinced. Although misinformation has always existed, never before has it been as ubiquitous as it is today and cloaked in the pretense of trustworthiness as found on the world-wide web. Errors in reasoning and bias in information processing are, therefore, central to the study of critical thinking (Leighton and Sternberg, 2004). Consequently, three lines of thinking were presented for why a refined conception of critical thinking in data-rich environments is warranted. First, traditional definitions of critical thinking typically lack connections to the information processes that are required to overcome bias. Second, data-rich environments pose cognitive traps in critical thinking that require more attention to bias. Third, personal dispositions such as motivation are more important than previously thought in the teaching and measurement of critical information processes. Because the present paper is not empirical but rather conceptual, we end not with main findings but with essential take home ideas. The first essential idea is that explicitly articulating a refined conception of critical thinking, one that includes reactive processes and/or mindware, must become part of how good thinking is described and taught. The second essential idea is that teaching and assessing proactive and reactive processes of critical thinking must be empirically examined.
The contemporary teaching and assessment of critical thinking must be situated within environments that are rich in data and evoke more than proactive but mechanistic information processes of analysis and evaluation. Teaching and assessment of critical thinking in data-rich environments must become more sophisticated to consider students’ 1) interest in the topics that merit critical thinking, 2) self-awareness of human bias, and 3) how both interest and self-awareness are used by students’ reflective minds (RM) to guide strategic application of critical-thinking processes in the AM. A conceptual refinement of critical thinking in data-rich environments, then, must be based on a strong theoretical foundation that presents a coordination of the reflective, algorithmic, and autonomous minds (Stanovich and Stanovich, 2010). This is provided by Stanovich and Stanovich’s (2010) tripartite model and supported by decades of empirical research into human thinking processes (Leighton and Sternberg, 2004; Kahneman, 2011; Stanovich, 2012; Shavelson et al., 2019).
A theoretical foundation for operationalizing a new conception of critical thinking, however, is useless for practice unless there is a framework that permits the principled design of teaching and assessment modules. Shavelson et al. (2019) provides such a framework. Shavelson et al. (2019) assessment framework provide the structure for generating performance-based tasks that evoke the reflective and algorithmic information processes required of critical thinking in data-rich environments.
The mindware that students download in performance assessments of critical thinking must reflect the sophistication of this form of information processing. Most students do not acquire these skills in secondary school or even post-secondary education (Stanovich, 2012; Ridsdale et al., 2015; Shavelson et al., 2019). Stanovich (2012, p. 356) states: “Explicit teaching of this mindware is not uniform in the school curriculum at any level. That such principles are taught very inconsistently means that some intelligent people may fail to learn these important aspects of critical thinking.” He indicates that although cognitive biases are often learned implicitly, without conscious awareness, critical-thinking skills must be taught explicitly to help individuals come to know when and how to apply higher-level skills. Instruction in critical thinking thus requires domain-specific knowledge and transferable skills that allow individuals to 1) coordinate the RM and AM, 2) recognize bias, and 3) regulate the application of higher-level thinking strategies. A more sophisticated conception of critical thinking provides an opportunity to guide instructive and performance-based assessment programs in the digital age.
Author Contributions
The authors confirm being the sole contributors of this work and have approved it for publication.
Acknowledgements
Preparation of this paper was supported by a grant to the first author from the Social Sciences and Humanities Research Council of Canada (SSHRC Grant No. 435-2016-0114).
Conflict of Interest
The authors declare that the research was conducted in the absence of any commercial or financial relationships that could be construed as a potential conflict of interest.
References
Abrami, P. C., Bernard, R. M., Borokhovski, E., Waddington, D. I., Wade, C. A., and Persson, T. (2015). Strategies for teaching students to think critically a meta-analysis. Rev. Educ. Res. 85 (2), 275–314. doi:10.3102/0034654308326084
Alava, S., Frau-Meigs, D., and Hassan, G. (2017). Youth and violent extremism on social media. Mapping the research. Paris, France: UNESCO.
Behar-Horenstein, L. S., and Niu, L. (2011). Teaching critical thinking skills in higher education: a review of the literature. J. Coll. Teach. Learn. 8 (2), 25–42. doi:10.19030/tlc.v8i2.3554
Bloom, B. S. (1956). Taxonomy of educational objectives. Vol. 1: Cognitive domain. New York, NY: McKay, 20–24.
Boyd, D. (2014). It’s complicated: the social lives of networked teens. London: Yale University Press.
Butler, H. A. (2012). Halpern Critical Thinking Assessment predicts real-world outcomes of critical thinking. Appl. Cognit. Psychol. 26 (5), 721–729. doi:10.1002/acp.2851
California State University (2011). Executive order 1065. Available at: https://calstate.policystat.com/policy/6741976/latest/ (Accessed April 25, 2020).
Churchland, P. S. (2011). Braintrust: what neuroscience tells us about morality. Princeton, NJ: Princeton University Press.
Clemson University (2016). Clemson Think2. Available at: https://www.clemson.edu/academics/programs/thinks2/documents/QEP-report.pdf (Accessed April 25, 2020).
Cohen, J. (1977). Statistical power analysis for the behavioral sciences. Revised edn. New York: Lawrence Erlbaum Associates, 490.
Cowan, N. (2001). The magical number 4 in short-term memory: a reconsideration of mental storage capacity. Behav. Brain Sci. 24 (1), 87–85. doi:10.1017/s0140525x01003922
Dawes, R. M. (1976). Shallow psychology. In Cognition and social behavior. Editors J. S. Carroll, and J. W. Payne (Hillsdale, NJ: Erlbaum), pp. 3–11.
El Soufi, N., and Huat See, B. (2019). Does explicit teaching of critical thinking improve critical thinking skills of English language learners in higher education? A critical review of causal evidence. Stud. Educ. Eval. 60, 140–162. doi:10.1016/j.stueduc.2018.12.006
Ennis, R. H. (2015). “Critical thinking: a streamlined conception,” in The Palgrave handbook of critical thinking in higher education. Editors M. Davies, and R. Barnett (New York: Palgrave), 31–47.
Ennis, R. H. (2016). “Definition: a three-dimensional analysis with bearing on key concepts,”in Ontario society for the study of argumentation (OSSA) conference archive, University of Windsor, May 18–21, 2016. 105. Available at: https://scholar.uwindsor.ca/ossaarchive/OSSA11/papersandcommentaries/105 (Accessed April 26, 2016).
Ennis, R. H., and Millman, J. (2005). Cornell critical thinking test, level X. 5th Edn. Seaside, CA: The Critical Thinking Company.
Evans, J. St. B. T. (2003). In two minds: dual-process accounts of reasoning. Trends Cognit. Sci. 7 (10), 454–459. doi:10.1016/j.tics.2003.08.012
Facione, P. (1990). Consensus statement regarding critical thinking and the ideal critical thinker. Millbrae, CA: California Academic Press.
Fisher, A., and Scriven, M. (1997). Critical thinking: its definition and assessment. Point Reyes, CA: Edgepress.
Frederick, S. (2005). Cognitive reflection and decision making. J. Econ. Perspect. 19 (4), 25–42. doi:10.1257/089533005775196732
Glaser, E. (1941). An experiment in the development of critical thinking. New York: Teacher’s College, Columbia University.
Hyytinen, H., Nissinen, K., Ursin, J., Toom, A., and Lindblom‐Ylänne, S. (2015). Problematising the equivalence of the test results of performance-based critical thinking tests for undergraduate students. Stud. Educ. Evaluation 44, 1–8. doi:10.1016/j.stueduc.2014.11.001
Hyytinen, H., Toom, A., and Shavelson, R. J. (2019). “Enhancing scientific thinking through the development of critical thinking in higher education,” in Redefining scientific thinking for higher education: higher-order thinking, evidence-based reasoning and research skills. Editors M. Murtonen, and K. Balloo (London: Palgrave Macmillan), 59–78.
Johnson-Laird, P. N. (2004). “Mental models and reasoning,” in The nature of reasoning. Editors J. P. Leighton, and R. J. Sternberg (New York, NY: Cambridge University Press), 169–204.
Johnson-Laird, P. N., and Bara, B. G. (1984). Syllogistic inference. Cognition 16 (1), 1–61. doi:10.1016/0010-0277(84)90035-0
Kroll, E. B., Rieger, J., and Vogt, B. (2010). “How does repetition of signals increase precision of numerical judgment?,” in Brain informatics. BI 2010. Lecture notes in computer science. Editors Y. Yao, R. Sun, T. Poggio, J. Liu, N. Zhong, and J. Huang (Berlin, Heidelberg: Springer), Vol. 6334. doi:10.1007/978-3-642-15314-3_19
Leighton, J. P. (2011). “A cognitive model of higher order thinking skills: implications for assessment,” in Current perspectives on cognition, learning, and instruction: assessment of higher order thinking skills. Editors G. Schraw, and D.H. Robinson (Charlotte, NC: Information Age Publishing), 151–181.
Leighton, J. P., Chu, M-W., and Seitz, P. (2013). “Cognitive Diagnostic assessment and the learning errors and formative feedback (LEAFF) model,” in Informing the practice of teaching using formative and interim assessment: a systems approach. Editor R. Lissitz (Charlotte, NC: Information Age Publishing), 183–207.
Leighton, J. P., and Dawson, M. R. W. (2001). A parallel processing model of Wason’s card selection task. Cognit. Syst. Res. 2 (3), 207–231. doi:10.1016/s1389-0417(01)00035-3
Leighton, J. P., and Gierl, M. J. (2007). Defining and evaluating models of cognition used in educational measurement to make inferences about examinees’ thinking processes. Educ. Meas. Issues Pract. 26 (2), 3–16. doi:10.1111/j.1745-3992.2007.00090.x
Leighton, J. P., and Sternberg, R. J. (2004). The nature of reasoning (Cambridge, MA: Cambridge University Press).
Leighton, J. P., and Sternberg, R. J. (2012). “Reasoning and problem solving,” in Handbook of psychology. Experimental psychology. Editors A. Healy, and R. Proctor 2nd Edn (New York: Wiley), Vol. 4, 631–659.
Lorencová, H., Jarošova, E., Avgitidou, S., and Dimitriadou, C. (2019). Critical thinking practices in teacher education programmes: a systematic review. Stud. Higher Educ. 44 (5), 844–859. doi:10.1080/03075079.2019.1586331
Miller, G. A. (1956). The magical number seven plus or minus two: some limits on our capacity for processing information. Psychol. Rev. 63 (2), 81–97. doi:10.1037/h0043158
Mislevy, R. J., Almond, R. G., and Lukas, J. F. (2003). A brief introduction to evidence-centered design. ETS Res. Rep. Ser. 2003 (1), i–29. doi:10.1002/j.2333-8504.2003.tb01908.x
Mullet, H. G., and Marsh, E. J. (2016). Correcting false memories: errors must be noticed and replaced. Mem. Cognit. 44 (3), 403–412. doi:10.3758/s13421-015-0571-x
Perkins, D. N. (1995). Outsmarting IQ: the emerging science of learnable intelligence. New York, NY: Free Press.
Prensky, M. (2001). Digital natives, digital immigrants. Horizon 9 (5), 1–6. doi:10.1108/10748120110424816
Puig, B., Blanco-Anaya, P., Bargiela, I. M., and Crujeiras-Pérez, B. (2019). A systematic review on critical thinking intervention studies in higher education across professional fields. Stud. High Educ. 44 (5), 860–869. doi:10.1080/03075079.2019.1586333
Reber, P. (2010). What is the memory capacity of the human brain? Sci. Am. Mind, 21(2), 70. doi:10.1038/scientificamericanmind0510-70
Ridsdale, C., Rothwell, J., Smit, M., Hossam, A.-H., Bliemel, M., Irvine, D., et al. (2015). Strategies and best practices for data literacy education: Knowledge synthesis report. Halifax, NS: Dalhousie University. Available at: https://dalspace.library.dal.ca/handle/10222/64578 (Accessed July 1, 2018).
Rizeq, J., Flora, D. B., and Toplak, M. E. (2020). An examination of the underlying dimensional structure of three domains of contaminated mindware: paranormal beliefs, conspiracy beliefs, and anti-science attitudes. Thinking Reasoning 1–25. doi:10.1080/13546783.2020.1759688
Roser, M., Ritchie, H., and Ortiz-Ospina, E. (2020). Internet. Published online atOurWorldInData.orgAvailable at: https://ourworldindata.org/internet (Accessed May 1, 2020).
Schacter, D. L. (2012). Adaptive constructive processes and the future of memory. Am. Psychol. 67 (8), 603–613. doi:10.1037/a0029869
Schmaltz, R. M., Jansen, E., and Wenckowski, N. (2017). Redefining critical thinking: teaching students to think like scientists. Front. Psychol. 8, 459–464. doi:10.3389/fpsyg.2017.00459
Shavelson, R. J., Zlatkin-Troitschanskaia, O., Beck, K., Schmidt, S., and Marino, J. P. (2019). Assessment of university students’ critical thinking: next generation performance assessment. Int. J. Test. 19 (4), 337–362. doi:10.1080/15305058.2018.1543309
Stanovich, K. E. (2009). What intelligence tests miss: the psychology of rational thought. New Have, CT: Yale University Press.
Stanovich, K. E. (2012). “On the distinction between rationality and intelligence: implications for understanding individual differences in reasoning,” in The Oxford handbook of thinking and reasoning. Editors K. Holyoak, and R. Morrison (New York: Oxford University Press), 343–365.
Stanovich, K. E. (2016). The comprehensive assessment of rational thinking. Educ. Psychol. 51 (1), 23–34. doi:10.1080/00461520.2015.1125787
Stanovich, K. E. (2021). “Why humans are cognitive misers and what it means for the great rationality debate,” in Routledge handbook of bounded rationality. 1st Edn, Editor R. Viale (London: Routledge), 11.
Stanovich, K. E., and Stanovich, P. J. (2010). “A framework for critical thinking, rational thinking, and intelligence,” in Innovations in educational psychology: perspectives on learning, teaching, and human development. Editors D. D. Preiss, and R. J. Sternberg (New York: Springer Publishing Company), 195–237.
Stanovich, K. E., Toplak, M. E., and West, R. F. (2008). The development of rational thought: a taxonomy of heuristics and biases. Adv. Child. Dev. Behav. 36, 251–285. doi:10.1016/s0065-2407(08)00006-2
Taylor, S. E. (1981). The interface of cognitive and social psychology. In Cognition, social behavior, and the environment. Editor J. H. Harvey (Hillsdale, NJ: Erlbaum), pp. 189–211.
Toplak, M. E., and Flora, D. B. (2020). Resistance to cognitive biases: longitudinal trajectories and associations with cognitive abilities and academic achievement across development. J. Behav. Decis. Making, 1–15. doi:10.1002/bdm.2214
Tversky, A., and Kahneman, D. (1974). Judgment under uncertainty: heuristics and biases. Science 185 (4157), 1124–1131. doi:10.1126/science.185.4157.1124
Keywords: post-secondary education, critical thinking, data-rich environments, cognitive biases, performance assessments
Citation: Leighton JP, Cui Y and Cutumisu M (2021) Key Information Processes for Thinking Critically in Data-Rich Environments. Front. Educ. 6:561847. doi: 10.3389/feduc.2021.561847
Received: 13 May 2020; Accepted: 21 January 2021;
Published: 24 February 2021.
Edited by:
Patricia A. Alexander, University of Maryland, United StatesReviewed by:
Sheng-Yi Wu, National Pingtung University, TaiwanHongyang Zhao, University of Maryland, College Park, United States
Copyright © 2021 Leighton, Cui and Cutumisu. This is an open-access article distributed under the terms of the Creative Commons Attribution License (CC BY). The use, distribution or reproduction in other forums is permitted, provided the original author(s) and the copyright owner(s) are credited and that the original publication in this journal is cited, in accordance with accepted academic practice. No use, distribution or reproduction is permitted which does not comply with these terms.
*Correspondence: Jacqueline P. Leighton, amFjcXVlbGluZS5sZWlnaHRvbkB1YWxiZXJ0YS5jYQ==