- Academy of Psychology and Educational Sciences, Southern Federal University, Rostov-on-Don, Russia
Background: During the COVID-19 pandemic, universities all over the world have had to reorganize their work for remote education to ensure continuity of learning. This situation has forced both teachers and students into an atypical, very specific situation, in which they need to cope with a number of psychological factors. Meanwhile, there is a research gap in academic knowledge of the social and psychological factors that determine attitudes toward forced remote learning.
Objective: To analyze the psychological traits of students in relation to their attitudes toward forced remote learning.
Methods: The study assessed self-regulation and communication skills, as well thinking and learning styles of 280 students in the situation of forced remote learning. We used the methods of surveying and psychological testing for data collection. The data were analyzed in R statistical software using the regression modeling methods.
Results: We found that the number of students with positive (46%) and negative (54%) attitudes toward forced remote learning during the COVID-19 pandemic was approximately equal. Using regression analysis, we identified several statistically significant associations between the interpersonal communicative skills of students (self-regulation, shyness, alienation, manipulative and cooperative communication styles) and their thinking styles (right-hemispheric and integrated), on the one hand, and their attitude to remote learning, on the other. It was also illustrated that depending on the attitude to the forced remote learning, students differ by the percentage of assimilated learning materials while studying under the lockdown.
Conclusion: The results identify that success of remote learning in many ways depends on the extent to which it accommodates psychological traits of students who are forced to acquire new knowledge without traditional classroom instruction.
Introduction
During the COVID-19 pandemic, educational institutions in more than 150 countries have faced unprecedented circumstances: All the campuses were closed, and learning was continued only remotely, mostly—with the use of digital platforms. Simultaneously, together with the risks, new opportunities have emerged. The forced transition to remote learning has prompted teachers and students to accelerate their mastery of digital technologies.
The coronavirus pandemic has had a substantial number of indirect effects, including those related to education and learning. As schools all over the world were compelled to shift to remote learning due to the closure of educational institutions, some 1.5 billion students have been affected by these necessary policy measures. Despite a lot of discussion, when it comes to the macro side of the situation, such as the effectiveness of the educational policy responses, not much is known about the psychological aspects of remote learning. It should be noted that this is the first time that the mode of learning has changed so substantially: learning based on physical person-to-person interaction between a teacher and a student in a classroom is not possible in a situation of school closures on a global scale. In these circumstances, both teachers and students need to cope with a number of psychological factors (Seymour-Walsh et al., 2020). It is critical to study the topic of remote education not only as a learning system, but also as a psychological phenomenon that includes such components as personal thinking styles and learning motivation, mental processes (e.g., creative thinking and spatial cognition), and psychological mechanisms (Yan et al., 2003; Singh and Thurman, 2019).
The current situation has a negative effect on people’s well-being. The indeterminacy of the situation causes increased anxiety in students who are in self-isolation during the lockdown. At the same time, the efficacy of coping strategies mostly depends on the psychological characteristics of the people involved, particularly their level of life-satisfaction (Rasskazova et al., 2020).
Analysis of academic research in Russia and abroad has made it possible to identify risks, difficulties, and advantages of remote learning. Several groups of difficulties exist with regards to students’ experiences during the transition to the remote learning mode: psychological, socio-psychological, social, operational, and difficulties related to risks for mental and physical health (Grigoryev, 2020). Psychological difficulties include a loss of basic cognitive skills, greater demands on the psychological qualities of teachers, difficulties in motivation and self-discipline. Socio-psychological difficulties are closely related to the loss of direct contact both between teacher and student and among students, with a loss of real support that can lead to loneliness and anxiety. An increase in social autonomy, alienation between students and teachers, cyberbullying, and cyber trolling are problems students face when it comes to the social aspect of remote learning. In the operational field, the issue lies in the administration of the learning process, staff qualifications and university resources to deliver remote learning, including the schedule and the process of examination (Henderson et al., 2017). The last group of difficulties identifies the risks related to physical and mental health, which includes difficulty in understanding the subject matter, visual impairment, physical inactivity, depressive states, as well as existential and ethical risks (e.g., virtualization of life, digital addiction; Jewitt, 2017).
The obvious advantages of remote learning for students include, first of all, freedom to choose the time and place of study, the possibility of repeatedly referring to the recordings of lessons, viewing training materials and assignments, and access to the experience of leading teachers all over the world (Mullagaliev and Urazlina, 2017; Sorokova, 2020). Moreover, the use of animations and multimedia tools increases the amount of acquired material, compared with students’ ordinary activities pre-pandemic (Kochetkova and Terskaia, 2017).
As Prudnikova and Poskakalova (2019) suggest, the use of such materials increases educational motivation, makes it possible to study on individual educational paths according to personal inclinations and the current level of a student’s development, expands the intensity of training through the increase of the channels to receive information, and also combines a variety of teaching practices and technologies (Prudnikova and Poskakalova, 2019). However, independent studies that compared online and face-to-face education showed the latter to be more effective: students who took the online course scored lower for academic performance, satisfaction of basic psychological needs, motivation, demonstration of knowledge gained, and as a result, lower final grades (Bettinger et al., 2017; Hurlbut, 2018; Wang et al., 2019).
At the same time, another body of work showed that online learning helped highly motivated students with high intellectual abilities to expand their knowledge when online learning was used in addition to traditional methods (Heppen et al., 2017). Those students who learned using a blended approach, in which online resources complement, but do not replace, traditional education, showed almost the same high results as those who learned in a traditional format (Escueta et al., 2017; Darling-Aduana, 2019).
In the case of remote learning, classes require that students remain highly motivated, self-regulated, and able to organize their own activities. Difficulties in online interaction are caused by the inability to establish and maintain psychological contact through non-verbal communication between teacher and student (Muilenburg and Berge, 2005). The lack of eye contact, facial expressions, and gestures violates the logic of the learning process, can lead to a decrease in students’ interest, and, as a consequence, reduce learning effectiveness (Phirangee et al., 2016).
Some authors emphasize that the use of digital technologies for remote learning should be based on the participants’ age and psychological characteristics (Muilenburg and Berge, 2005; Verkhovskaia et al., 2016; Rubtsova et al., 2018). Meanwhile, there has been little research describing the relationship between the attitude of university students to remote learning, their adaptation to this learning mode, and their psychological characteristics. One of the few existing studies based on the analysis of focus group interviews suggest that students express a desire for a more personalized learning experience, but one that is still highly social within learning communities (Shearer et al., 2020). The circumstances of the spread of COVID-19 have created high uncertainty regarding future formats of study at universities. All this makes empirical measurement of attitudes to forced remote learning among students a highly relevant problem.
Moreover, a research gap exists in academic knowledge on the psychological factors that determine attitudes toward remote learning, as well as psychological features that draw a portrait of students according to their ability to adapt to the new learning mode. This study aims to shed light on how psychological factors of students are linked to their attitudes toward remote learning during the COVID-19 outbreak.
Data and Methods
Research Questions and Hypotheses
The major objective of this study is to identify the psychological characteristics of students in relation to their attitudes toward forced remote learning. As such, the study is guided by the following research question: What are the main personality traits of students with a positive attitude to remote learning, and of those who have difficulties in adapting to the rapidly changed learning practices? The cognitive interest of our investigation lies in understanding these patterns in the light of the special education needs of those students who demonstrate higher motivation and efficiency in learning.
A number of other research questions complements that objective, constituting the research agenda of the present article:
• Is there any shift in perceived academic performance of those students who could adapt to remote learning and those who couldn’t?
• Is there any statistically significant effect of interpersonal communication traits on adaptivity to remote learning?
• What is the relation between attitudes to forced remote learning and personal style of thinking?
Research Design
The empirical part of the study was conducted in May 2020, employing the methods of survey and psychological testing for data collection. The sample consisted of 280 students (with 2–5 years of university education) from the Southern Federal University (SFedU) in Rostov-on-Don, Russia, of whom 23.1% were males and 76.9% were females; the average age of the sample was 21.5 years. They took part in the voluntary intensive online project “SfeduNet 2.0: Solutions for the Future.” Students with the high cognitive orientation and a focus on intensive teamwork to achieve efficient results in the research affiliate were main participants of the project. They were selected on the voluntary basis, but in the process of psychological testing that estimated their self-efficacy, result-orientation, and teamwork skills. The students worked in the teams comprised of 10 members where they had to engage in various research projects in accordance with their cognitive interests. Choosing this group for research, we wanted to look at the forced remote learning through the eyes of students with diverse learning needs, namely with high internal learning motivation, maximally focused on the development of their professional competencies.
Psychological Tests
For addressing the outlined research questions, we developed a short questionnaire. The students were asked about their attitude toward forced remote learning and were given two options to respond: either positive or negative. The second question dealt with the assimilated information and the students were asked the following: “How do you think, what share of information taught could you assimilate while studying in the forced remote learning situation?” The answers were given on the scale from 0 to 1 and thus reflected self-perceived efficiency of studying under the conditions of the forced remote learning. Finally, another important question of the questionnaire referred to the perceived change in the academic performance. As such, the students were asked if their academic performance while studying under the conditions of the forced remote learning changed, having three answer options: improved, worsened, or did not change.
For studying personality traits, we adopted the methods of psychological testing. As such, we used two psychological tests. The first was Kunitsina’s (1991) scale on self-regulation and the success of interpersonal communication. Her approach allowed us to determine the communicative and personal characteristics associated with informal interpersonal confidential communication. It is particularly useful to identify the degree of communication skills and the presence of communication difficulties, the nature of these difficulties, and personal awareness about them. The test is comprised of 102 statement that characterize intensity of contacts with people. The statements are summarized in 26 subdimensions that outline different communication styles, such as: self-regulation, shyness, communicative skills, manipulativeness, cooperativeness, etc. For each dimension, the students were assessed on the scale between 0 and 12.
Torrance’s (1990) classification of students by their thinking and learning styles on three groups, those who have a dominant left hemisphere (emphasizing logic and analysis), a dominant right hemisphere (emphasizing emotions, intuition, images), or an integrated type (when both hemispheres are equally active), was the second psychological test used in the study. The test includes 40 statements (that result in 40 max score points) regarding behavior of a person in various hypothetical situations. The statements are accompanied by three answer options, each corresponding to a specific thinking style—left, right. Hemispheric, or integrated one. By the end of assessment, respondent gets a specific score in each of the three domains, where higher values indicate the dominance of the respective thinking style. As a result, each participant gets a score by distributing 40 points across three dimensions depending on the answers.
Data Analysis
The data analysis of data started with descriptive statistics to provide an overview of the sample parameters and frequency distributions. Attitude toward forced remote learning was a dependent variable of the study, with binary responses: positive and negative. Predictors included the following variables on the continuous scale: percentage of assimilated learning information that came from the questionnaire and set of scores obtained through the psychological testing via Torrance’s (1990) and Kunitsina’s (1991) scales. As such, a set of continuous variables that described interpersonal communication styles referred to quantitative assessment of students on their exposure to manipulative, alienated, shy communication styles, as well as their skills in interpersonal communication. Test of Torrance’s test of thinking and learning styles allowed us to assign to each student a score on the scale from on their exposure to three aspects: left-hemispheric thinking, right-hemispheric thinking, or integrated thinking. Finally, another categorical variable employed in the analysis referred to the perceived change in academic performance after the shift to the forced remote learning, a nominal variable with three outcomes: did not change (reference), improved, worsened. The data in Table 1 present descriptive statistics of the key variables of the study that were derived in result of psychological testing. As can be seen by the close values of mean and median, as well as mostly low deviation of skewness from 0, most of the variables follow approximately normal distribution.
For assessing the significance of the differences between the means of the different subgroups we adopted the Wilcoxon rank sum test. We chose this test since the data did not follow a normal distribution and therefore a conventional t-test could give a misleading result. In the last stage, we employed regression modeling to understand how the relation of students to remote learning is associated with their personality traits and psychological characteristics. Since, as was mentioned earlier, the data do not always follow a normal distribution, we were seeking a model that allows non-linear functions for the predictors. In this context, the Generalized Additive Model (hereafter GAM), became an efficient solution. This statistical approach introduced by Hastie and Tibshirani (1990) and developed by Hastie et al. (2009, 2015) as advanced regression for prediction and classification. In comparison to regression modeling based on linear functions, GAMs have the advantage of capturing non-linearities and variation in data (Hill and Lewicki, 2006) through the application of non-parametric smoothing splines. Given that it is quite problematic to achieve linearity in associations between variables that measure attitudes and personality traits, GAMs have great explanatory power in modeling human behavior and the potential of being effectively applied in the psychometrics and behavioral research.
All the data analysis was carried out in R, lingua franca of statistical computing.
Results
Perceived Academic Performance of Students With Regard to Their Attitude Toward Remote Learning
The study aims to shed light on how psychological traits of students are linked to their attitudes toward forced remote learning. Our study shows that the students were divided into relatively equal groups by their attitude toward forced remote education: while 54% have a positive attitude to the forced remote learning, 46% have a negative one. The confidence intervals for the two groups overlap, which allows us to conclude that the associated levels of uncertainty for both student cohorts do not produce statistically significant differences. In other words, we cannot say that one group clearly dominates over the other, despite the difference observed in the frequency values.
Our first objective was to understand how the students differ in their perceived change in academic performance with regard to their attitude toward forced remote learning. While filling out the questionnaire, the students were asked about the percentage of information that they find easy to assimilate while learning remotely. Not surprisingly, those who have a positive attitude toward remote learning understand the material better (M = 46.3, SD = 22.2) than the students who are uncomfortable with remote learning (M = 37.1, SD = 20.7). Since the distribution of scores on the amount of successfully assimilated information did not follow the normal one (which is explicitly seen of Figure 1), we ran the Wilcoxon rank sum test to assess whether the difference was statistically significant. We found that the learning outcomes of students who are comfortable with studying remotely are significantly higher than those of students who did not adapt to the current situation, W = 488.5, p < 0.05.
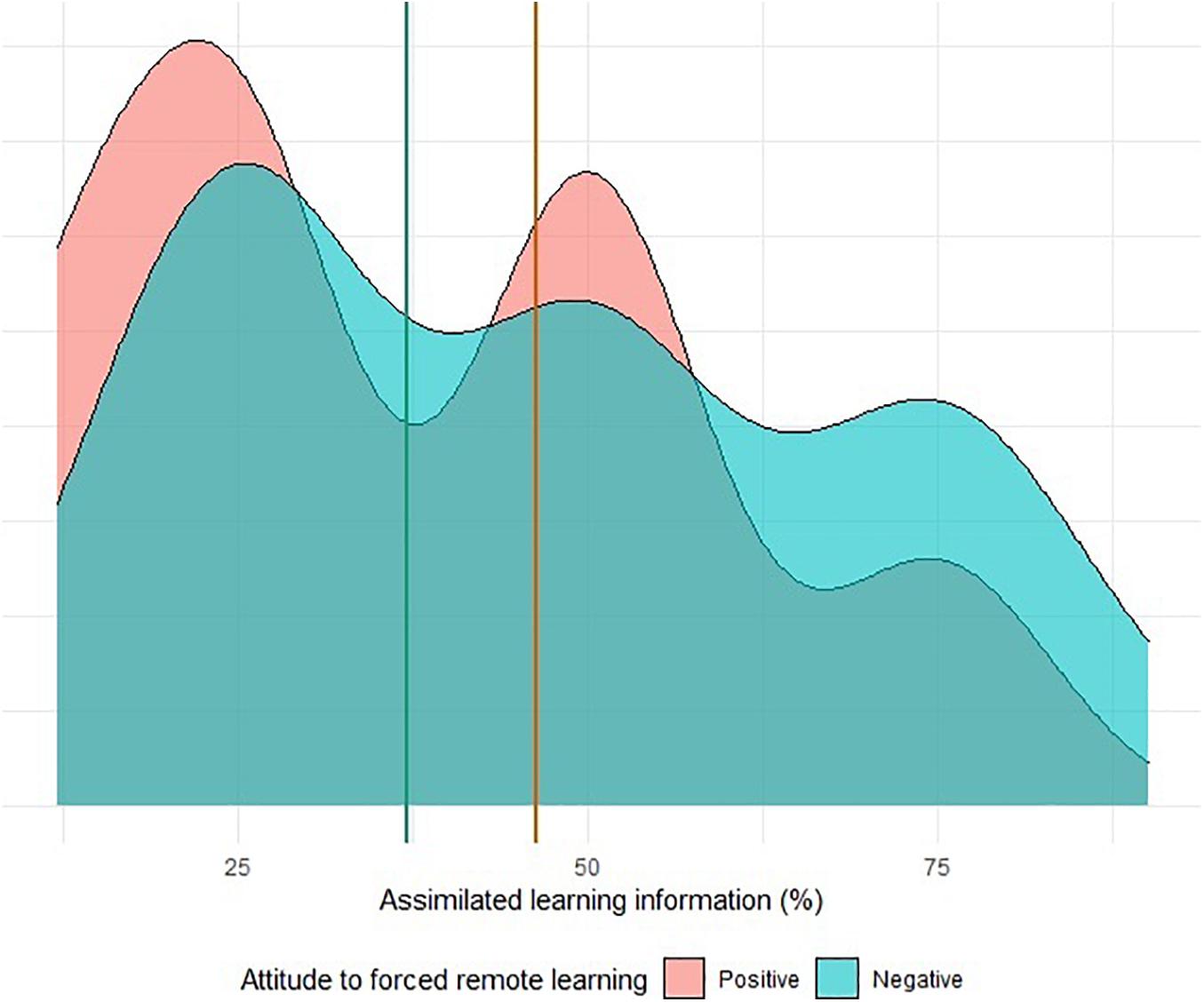
Figure 1. Probability density function of the percentage of assimilated information with respect to attitude toward remote learning. The vertical lines indicate group means.
In order to understand the magnitude of these differences, it is useful to examine the overlap between the two distributions (Figure 1). It turns out that both groups obtain multimodal distribution as several peaks are explicitly visible for both groups of students. It allows for hypothesizing that additional grouping or clustering exists with regards to assimilation of the learning material, however, it is impossible to predict the factors behind it based on descriptive analysis only. The horizontal lines indicate that amongst students with positive attitude toward forced remote learning, the mean percentage of assimilated information is higher than amongst those who have a negative one. On the other hand, given that two groups show a high overlap in distribution, the substantial nature of these differences in practice is questionable.
Regression Models
Another important conclusion produced from the probability density plot pertains to the multimodal character observed in the distribution of scores on the percentage of information assimilated by the groups with positive and negative attitudes toward the forced remote learning. The graph allows us to hypothesize that there are multiple subgroups within the two plotted groups, and there are different psychological factors that affect the relationship between attitude toward forced remote learning and percentage of the information that is assimilated. It can be assumed that, for example, the distribution is affected by the presence of people with a different set of communicative skills or perceived change in academic performance or psychological type. To test these hypotheses in line with our research questions, the analysis needs to go beyond descriptive statistics. Therefore, we employed regression analysis using a GAM.
We built two regression models, both having “attitude to remote learning” as the dependent variable. The variable is a binary one, with the values indicating a positive relation (coded as 1) or a negative relation (coded as 0) of students to the learning mode adopted due to the school closures. The first model was intended to assess the relation between the dependent variable and the students’ communicative skills. It had the following equation:
where s is a non-parametric smoothing spline, i.e., non-linear function used to describe a relation between a predictor and outcome variable. To run the analysis, we used mgcv package in R (Wood and Wood, 2015). The details on GAMs could be found in Wood (2017).
The second model was intended to estimate the effects of personal thinking and learning styles on attitudes to forced remote learning. It included the predictors in accordance with the equation below:
The results of the models are summarized in Table 2. Since the models calculated are semi-parametric, i.e., they employ both linear and non-linear terms, for non-linear non-parametric smoothing splines no regression coefficient is produced. For meaningful interpretation of the non-parametric associations between independent and dependent variable, one needs to examine the figures to understand what kind of statistical trend is produced in outcome due to an increase in the predictor. Therefore, the full picture could be obtained only after exploring both Table 2, as well as Figures 2, 3 together.
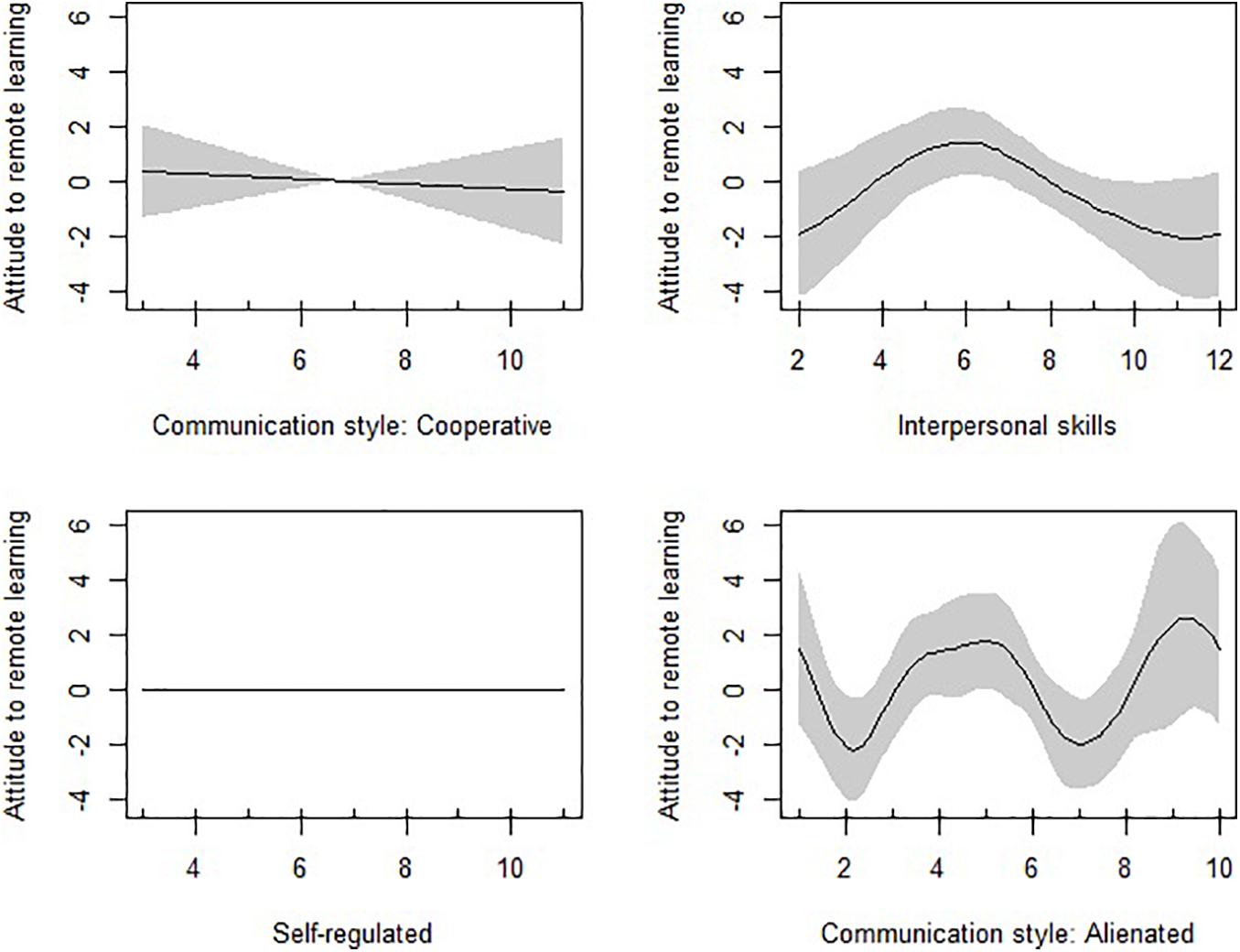
Figure 2. GAM components (partial prediction), plots with 95% confidence intervals. Interpersonal communicative skills model.
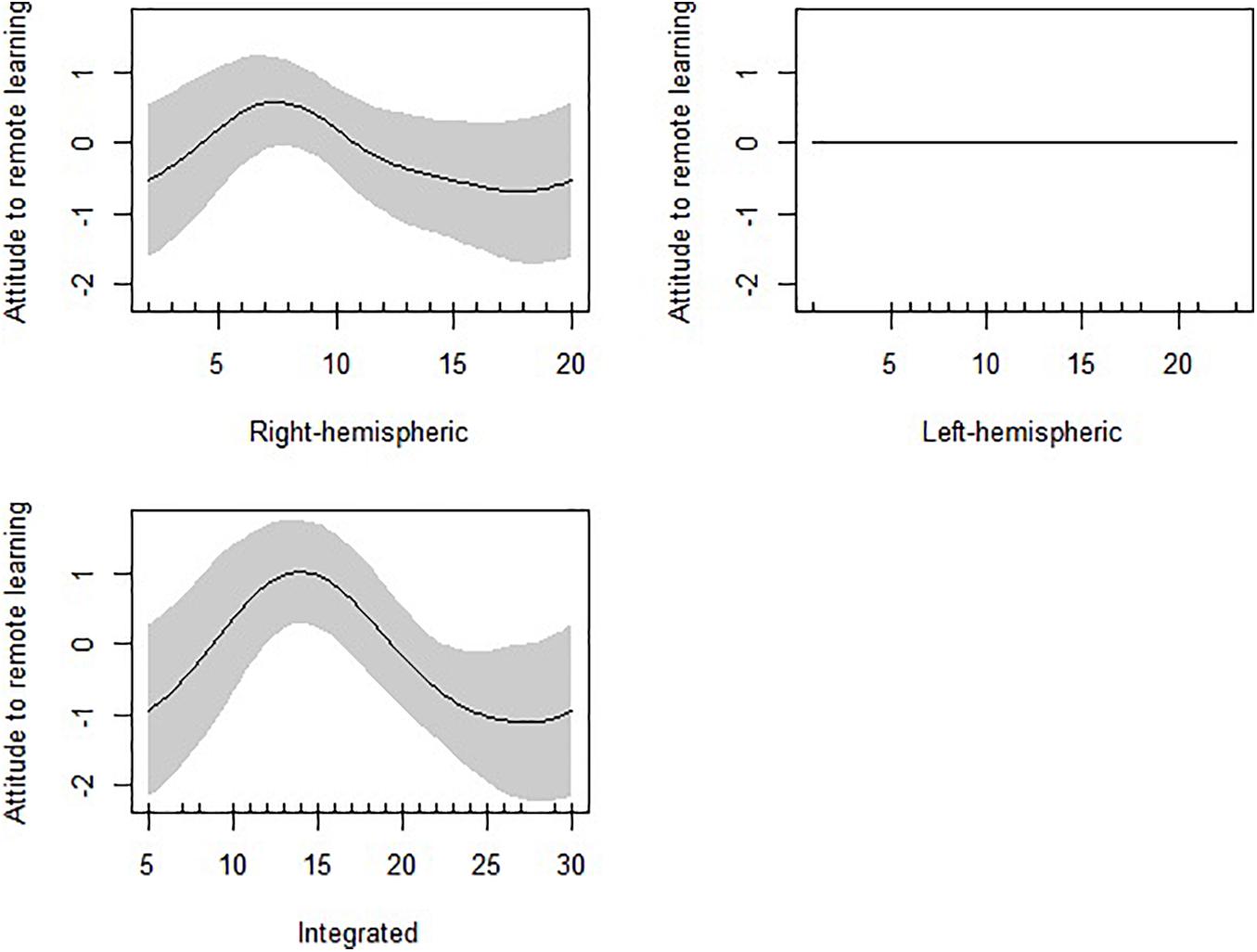
Figure 3. GAM components (partial prediction), plots with 95% confidence intervals. Thinking and learning styles model.
The model of interpersonal communication skills revealed statistically significant effects of manipulative style, shyness, interpersonal skills, alienation, and perceived change in academic performance. The effects of manipulative style and shyness were linear. It can be said that the more manipulative is the student’s style of interpersonal communication, the less likely that person is to have a positive attitude to forced remote learning. Based on the logit values in Table 2, it can be calculated that an increase of 1 unit on the manipulative style scale leads to a 5% decrease of the odds of having a positive attitude to remote learning. On the other hand, shy people have a higher probability of a positive attitude to remote learning: An increase of 1 unit on the shyness scale increases the odds of a positive attitude by 18%. Since the linear effects of interpersonal skills and alienation did not produce meaningful results, we employed non-parametric smoothing splines to understand the effect of these predictors. Interesting effects were found among alienated people and those who have high interpersonal skills. The trend lines on Figure 2 clearly indicate the relationship between these predictors and the output variables.
In the case of interpersonal skills, the line goes up but reaches a peak in the middle, then rapidly shows a negative trend. This means that those with low and intermediate interpersonal skills tend to have a positive attitude to remote technology, whereas those with highly developed interpersonal skills tend not to enjoy remote learning. A more complicated trend can be found when it comes to alienation. The curve, with its multiple peaks, demonstrates that different levels of personal alienation are associated either positively or negatively with remote learning. It can, however, be said that students who have the highest alienation scores tend to have a positive attitude to remote learning. Finally, those students who reported a positive change in their perceived academic performance have a higher probability of a positive attitude to remote learning than those who did not report any changes.
The second model was intended to assess the impact of personal thinking and learning style on the attitude to remote learning (Figure 3). The model identified statistically significant effects of students with the right hemisphere active and those with integrated thinking. None of the models identified a statistically significant effect of sex, which was a controlling variable.
Research Limitations
The major limitation of the study refers to the fact that two core questions of the questionnaire—those related to the percentage of assimilated information and change in academic performance after the shift to the forced remote learning, are not the actual learning assessment or psychological measurement of how well students assimilate learning materials and what is the change in their academic performance. Oppositely, the collected data are based on students’ self-perceived reporting, i.e., it could present some positivity bias.
Discussion
The results of our survey showed that the attitudes of SFedU students toward forced remote learning were divided approximately equally: 54% of students do not like this kind of learning and 46% have a positive attitude to it. Due to the overlapping confidence intervals of these two student groups, we cannot conclude that either of these groups is clearly dominant. The survey was conducted in May 2020, when students to some extent had already been adapted to remote learning and were able to analyze its strengths and weaknesses. Therefore, in the survey results, their attitude is expressed as a trend, rather than a personal position.
It should be noted that the participants were students at SFedU with diverse learning needs and high internal cognitive motivation. Thus, we can note that the students’ answers regarding remote learning concerned the format itself, and their attitude to educational and academic activities was initially positive.
A number of other factors related to learning in conditions of self-isolation could influence the negative attitude of some students to remote learning. First, students were forced to stay in the same room and could not leave the house, which can be regarded as a sharp change in their usual lifestyle; there was a dramatic increase in the time spent working at the computer, as well as stress caused by the need to transition abruptly to a new mode of education. Moreover, the reduced physical and intellectual load may have led to non-compliance with the remote learning process, adding a dimension to potential factors determining a negative attitude.
In addition to examining the attitudes of students, we were interested in the effectiveness of learning in the new format, so we examined the impact of students’ attitudes toward the remote learning on their idea of the amount of information being acquired. Answers to the question about the percentage of information assimilated during remote learning confirmed that SFedU students who are positive about the new format learn more than students who have a negative attitude. We found that, on average, the information mastered by students with a positive attitude toward remote learning is 44% higher than among students with a negative attitude.
Such a result is quite expected and confirms the results of other researchers. Students who stated that they like to study remotely showed better academic results than those who do not like this form of study (Hurlbut, 2018). Also, in a large-scale study, both the effectiveness of remote learning and the pleasure of it (Muilenburg and Berge, 2005) were highlighted as variables that significantly affect the barriers of remote learning. At the same time, students in face-to-face learning tend to get slightly higher grades than those who study remotely (Hurlbut, 2018).
The results of the first regression model detected several patterns in the association between the students’ communicative and personal characteristics and their attitude to forced remote learning. There was an inverse linear relationship between manipulative communication styles and a positive attitude toward the remote learning format. The more a student is inclined to be manipulative, the lower the probability of a positive attitude to remote learning. Most often, such students talk about technical difficulties that supposedly do not allow them to turn on their computer’s camera or even the microphone. But in doing so, they limit the channels of information to the interlocutor, prompting him or her to concentrate on one thing (for example, the auditory channel).
A linear relationship was also found between shyness and a positive attitude toward the forced remote learning. Forced remote learning creates favorable conditions for its behavioral manifestations—silence, stiffness, a quiet voice. Shy students are comfortable in the solitude in which they find themselves because of self-isolation.
A complex non-linear relationship was found between the attitude to remote learning, on the one hand, and communication skills, as well as alienation, on the other. The highest probability of a positive attitude toward remote learning is among students whose communication skills are developed to a moderate degree. For them, communication is a personal resource for improving academic performance. The level of development of their communication skills allows them to establish psychological contact with teachers and classmates, if necessary, to remove emotional stress, which generally positively affects the content of communication. Students with either highly or poorly developed communication skills have a lower probability of a positive attitude toward remote learning. For students with highly developed communication skills, the technical limitations of the online environment constrain them, making non-verbal communication difficult for themselves and other interlocutors. They perceive online communication as inadequate. Students with poorly developed communication skills have difficulty initiating and maintaining communication.
Regarding alienation, we revealed a complex relationship. With a different degree of alienation among students, their attitude to remote learning can be either positive or negative. Stepanova and Kalacheva (2018) note that remote learning refers to alienating technology. It minimizes the personal interaction of students and teachers. Instead of reasoning, arguing, and constructing an answer, the student chooses the answers presented in advance in the tests, which leads to the simplification of thinking. All this alienates from the freedom of creative designing of a cognitive situation and leads to its limited choice. At the same time, the authors acknowledge that online technology is a way to get rid of alienation in education, because it contributes to increasing the speed and effectiveness of educational activities carried out with their help, continuous updating of its content (Stepanova and Kalacheva, 2018).
It is likely that the relationship between alienation and students’ attitudes toward forced remote learning is ambiguous and is mediated by the specific technologies that the teacher uses. If it stimulates the exchange of information and joint work of students in the Internet environment, then this contributes to the development of their cognitive activity and formation as a person, not alienating themselves from society. It can be said that alienation in education and the role of Internet technologies need to be further developed.
Using the second model, a non-linear relationship of students’ right-hemispheric and integrated thinking styles in relation to forced remote learning was revealed. The study identified the associations between students‘ thinking styles and their attitude to remote learning. The probability of a positive attitude will be higher with a weak degree of dominance of the right-hemispheric style of thinking and with a slightly more prominent, but not clearly dominant, integrated style. The relationship between the attitude to remote learning and left- hemispheric style, as well as differences in males or females were not identified. This means that the probability of a positive attitude toward remote learning is higher for those students who are quick to navigate the situation, can flexibly change their thinking strategies, choosing the most suitable for solving the task. Probably, such students should have their own thinking strategy, which allows them to save energy and time. For many countries in the world, remote learning is no longer a temporary emergency situation but has started to become something more long-term. Highly likely, a lot of countries or educational institutions all over the world will shift to blended learning in the near future. The experience gained through the organization of forced remote learning could become essential in incorporating good practices that facilitate psychologically comfortable environment for all learners. However, the results of our study explicitly outline that the attitude toward remote learning, as well as assimilation of the learning material while studying under the conditions of forced remote learning, are in many ways associated with personality traits. Though further research is needed to shed light on how effective is remote learning in comparison to the traditional modes of delivery, one needs to bear in mind that adapting to this learning modality might be challenging for the key actors.
Conclusion
The study suggests that when it comes to the students with high learning motivation, they differ in terms of relation to forced remote learning. Moreover, shift to forced remote learning resulted in the assimilation of learning material. In general, association between the communication and learning styles on the one hand, and attitude to remote learning on the other hand, provides a convincing evidence that personality traits have their say in the ways how students adapt to the new learning modalities. These conclusions have implications on the ways remote learning should be organized at higher education institutions.
As the study evidence that not all highly motivated students are comfortable with remote learning, the solutions adopted by the universities should account for the potential impact on the motivation and learning needs of those students who before the pandemic demonstrated increased interest in pursuing education. As coming “back to normal” does not seem to be realistic even after 1 year of the pandemic in many countries of the world, education policy makers are challenged by the requirement to not only ensure connection of the enrolled young people with learning, but also to fully satisfy the diverse learning needs of students, accounting somehow for their personal traits. New learning approaches, based on blended modalities, can be put in place as a potential solution if the epidemiological situation at place allows for that. However, given the unique educational context and pandemic rates in all the countries, no universal solution can be proposed. Education stakeholders should monitor the psychological well-being of students on a regular basis, which could allow for data-driven and efficient policy solutions which are specific to a context.
The results can be useful for summarizing the experience of higher education at the emergency conditions of the COVID-19 pandemic, in order to adapt and develop optimal educational formats at universities. As our analysis confirms that the attitude to remote learning is in many ways associated with personality traits, the results highlight both challenges and potential areas for improvement in terms of establishing remote learning. While some students could be prone of adopting manipulative communication styles trying to camouflage their engagement in learning, others do their best to benefit from this situation by setting comfortable conditions for studying. As such, future psychological research should inform better decisions on involving alienated and shy students into learning more. Other possibilities for further analyses include studying the links between psychological characteristics of university students and their attitude to other challenges and perspectives of various forms of online (synchronous, asynchronous), offline, and blended learning.
Data Availability Statement
The raw data supporting the conclusions of this article are not included in the article/supplementary material to preserve participants’ confidentiality, further inquiries can be directed to the corresponding author.
Ethics Statement
The studies involving human participants were reviewed and approved by the Ethics Committee of the Academy of Psychology and Educational Science of Southern Federal University, Rostov-on-Don, Russia. The patients/participants provided their written informed consent to participate in this study.
Author Contributions
LD demonstrated research relevance, selected and analyzed the material, and analyzed, interpretated, and processed the results. GA completed data processing, statistical data analysis, and results analysis. ID collected empirical data, worked with respondents, conducted psychological testing, and selected research methods and techniques. VK demonstrated relevance of the research, analyzed available studies, and summarized results and prepared conclusions. VE adapted and translated the text, edited the material recorded, and selected and analyzed both the material and references. All authors contributed to the article and approved the submitted version.
Funding
This research was financially supported by the Southern Federal University, 2021, 07/2020-02-AP.
Conflict of Interest
The authors declare that the research was conducted in the absence of any commercial or financial relationships that could be construed as a potential conflict of interest.
References
Bettinger, E. P., Fox, L., Loeb, S., and Taylor, E. S. (2017). Virtual classrooms: how online college courses affect student success. Am. Econ. Rev. 107, 2855–2875. doi: 10.1257/aer.20151193
Darling-Aduana, J. (2019). Behavioral engagement shifts among at-risk high school students enrolled in online courses. AERA Open 5, 1–19. doi: 10.1177/2332858419887736
Escueta, M., Quan, V., Nickow, A. J., and Oreopoulos, P. (2017). Education Technology: An Evidence-Based Review. NBER Working Paper No. 23744. Cambridge, MA: NBER, doi: 10.3386/w23744
Grigoryev, A. V. (2020). The risks of digitalization of school education (based on an Astrakhan teachers survey). Soc. Sociol. Psychol. Pedagogy 6,, 31–38.
Hastie, T., and Tibshirani, R. (1990). Exploring the nature of covariate effects in the proportional hazards model. Biometrics 46, 1005–1016. doi: 10.2307/2532444
Hastie, T., Tibshirani, R., and Friedman, J. (2009). The Elements of Statistical Learning: Data Mining, Inference, and Prediction. Berlin: Springer Science & Business Media.
Hastie, T., Tibshirani, R., and Wainwright, M. (2015). Statistical Learning With Sparsity: The Lasso and Generalizations. Boca Raton, FL: CRC press, doi: 10.1201/b18401
Henderson, M., Selwyn, N., and Aston, R. (2017). What works and why? Student perceptions of ‘useful’ digital technology in university teaching and learning. Stud. in Higher Educ. 42, 1567–1579. doi: 10.1080/03075079.2015.1007946
Heppen, J. B., Sorensen, N., Allensworth, E., Walters, K., Rickles, J., Taylor, S. S., et al. (2017). The struggle to pass algebra: online vs. face-to-face credit recovery for at-risk urban students. J. Res. Educ. Effect. 10, 272–296. doi: 10.1080/19345747.2016.1168500
Hill, T., and Lewicki, P. (2006). Statistics: Methods and Applications. A Comprehensive Reference for Science, Industry, and Data Mining. Tulsa, OK: StatSoft.
Hurlbut, A. R. (2018). Online vs. traditional learning in teacher education: a comparison of student progress. Am. J. Distance Educ. 32, 248–266. doi: 10.1080/08923647.2018.1509265
Jewitt, K. (2017). The MOOC revolution—massive open online courses: the answer to problems facing education or an experiment that could destroy centuries of tradition. Compass J. Learn. Teach. 10, 1–14. doi: 10.21100/compass.v10i1.371
Kochetkova, I. S., and Terskaia, L. A. (2017). Experience in using the e-learning system (Moodle) in general scientific and special disciplines. Azimuth Sci. Res. Pedagogy Psychol. 6, 93–97.
Kunitsina, V. N. (1991). Dissertatsiia Doktora Psikhologicheskikh Nauk. Saint Petersburg: Saint Petersburg State University, 358. Doctoral dissertation.
Muilenburg, L. Y., and Berge, Z. L. (2005). Student barriers to online learning: a factor analytic study. Distance Educ. 26, 29–48. doi: 10.1080/01587910500081269
Mullagaliev, N. A., and Urazlina, N. V. (2017). On the attitude of students to the introduction of distance learning elements in a university. Innov. Sci. 1, 188–191.
Phirangee, K., Epp, C. D., and Hewitt, J. (2016). Exploring the relationships between facilitation methods, students’ sense of community, and their online behaviors. Online Learn. 20, 134–154. doi: 10.24059/olj.v20i2.775
Prudnikova, T. A., and Poskakalova, T. A. (2019). The experience of application of information and communication technologies (ICTs) as a tool to enhance learning motivation. J. Modern Foreign Psychol. 8, 67–82. doi: 10.17759/jmfp.2019080207
Rasskazova, E. I., Leont’ev, D. A., and Lebedeva, A. A. (2020). Pandemic as a challenge to subjective well-being: anxiety and coping. Couns. Psychol. Psychother. 28, 90–108. doi: 10.17759/cpp.2020280205
Rubtsova, O. V., Panfilova, A. S., and Smirnova, V. K. (2018). Study of the relationship between adolescents’ personality traits and their behavior in virtual space (using the example of the social network ‘VKontakte’). Psychol. Sci. Educ. 23, 54–66. doi: 10.17759/pse.2018230305
Seymour-Walsh, A. E., Bell, A., Weber, A., and Smith, T. (2020). Adapting to a new reality: COVID-19 coronavirus and online education in the health professions. Rural Remote Health 20:6000. doi: 10.22605/RRH6000
Shearer, R. L., Aldemir, T., Hitchcock, J., Resig, J., Driver, J., and Kohler, M. (2020). What students want: a vision of a future online learning experience grounded in distance education theory. Am. J. Distance Educ. 34, 36–52. doi: 10.1080/08923647.2019.1706019
Singh, V., and Thurman, A. (2019). How many ways can we define online learning? A systematic literature review of definitions of online learning (1988–2018). Am. J. Distance Educ. 33, 289–306. doi: 10.1080/08923647.2019.1663082
Sorokova, M. G. (2020). The digital educational environment of the university: who is more comfortable studying in it? Psychol. Sci. Educ. 25, 44–58. doi: 10.17759/pse.2020250204
Stepanova, I. N., and Kalacheva, E. V. (2018). Alienation in education. Bull. Kurgan State Univ. 1, 85–87.
Verkhovskaia, E. K., Rubtsova, O. V., and Panfilova, A. S. (2016). “Methodology for the study of self-presentation and interaction of Russian adolescents in modern social networks,” in Neurocomputers and Their Application, eds A. I. Galushkin, A. V. Chechkin, L. S. Kuravskoi, S. L. Artemenkov, G. A. Ur’ev, P. A. Marmaliuk, et al. (Moscow: MSUPE), 69–70.
Wang, C., Hsu, H. C. K., Bonem, E. M., Moss, J. D., Yu, S., Nelson, D. B., et al. (2019). Need satisfaction and need dissatisfaction: a comparative study of online and face-to-face learning contexts. Comput. Hum. Behav. 95, 114–125. doi: 10.1016/j.chb.2019.01.034
Wood, S. N. (2017). Generalized Additive Models: An Introduction with R, 2nd Edn. Boca Raton, FL: CRC Press, doi: 10.1201/9781315370279
Keywords: COVID-19 pandemic, forced remote learning, diverse educational needs, attitudes toward remote learning, communicative and personal traits, thinking and learning styles, university students
Citation: Dikaya LA, Avanesian G, Dikiy IS, Kirik VA and Egorova VA (2021) How Personality Traits Are Related to the Attitudes Toward Forced Remote Learning During COVID-19: Predictive Analysis Using Generalized Additive Modeling. Front. Educ. 6:629213. doi: 10.3389/feduc.2021.629213
Received: 13 November 2020; Accepted: 19 March 2021;
Published: 09 April 2021.
Edited by:
Rhoda Scherman, Auckland University of Technology, New ZealandReviewed by:
Yen-Hsin Chen, National Taichung University of Education, TaiwanChris Krägeloh, Auckland University of Technology, New Zealand
Copyright © 2021 Dikaya, Avanesian, Dikiy, Kirik and Egorova. This is an open-access article distributed under the terms of the Creative Commons Attribution License (CC BY). The use, distribution or reproduction in other forums is permitted, provided the original author(s) and the copyright owner(s) are credited and that the original publication in this journal is cited, in accordance with accepted academic practice. No use, distribution or reproduction is permitted which does not comply with these terms.
*Correspondence: Liudmila A. Dikaya, ZGlrYXlhQHNmZWR1LnJ1