- Auckland University of Technology, School of Sport and Recreation, Auckland, New Zealand
In New Zealand, similar to the rest of the world, the COVID-19 pandemic brought unprecedented disruption to higher education, with a rapid transition to mass online teaching. The 1st year (and 1st semester in particular) of any University degree presents unique challenges for students. Literature suggests these students have significant learning concerns as they adjust to University teaching and assessment requirements. These challenges may be exacerbated with the rapid introduction of online learning environments as they are increasingly disconnected from their peers, and, at a greater risk of struggling with web-based learning technologies.
This study investigated online learning strategies employed by 1st year students and examined the association between these strategies and student achievement. The University’s learning management system (LMS; Blackboard) was used to collect deidentified data related to students’ engagement with online content. The number of times content was clicked was recorded each day for the student’s three courses. These data were collected over a nine-week period for all students (N = 170) enrolled in the 1st semester of their degree. This nine-week period spanned from the commencement of COVID-19 online learning to the week of final assessments. The relationship between assessment date and online engagement was investigated and linear mixed models were used to determine if engagement with online learning was associated with final course grades.
The results suggested that students adopted a learning strategy that coordinated their online LMS engagement with course assessment due date. Students had a 388% (SD 58%) greater specific engagement with the LMS on the assessment due date and the day prior, than throughout the remainder of their course. A further trend was observed whereby when an assessment was due in one course the students used an ‘online bundle learning’ strategy of increased engagement with the two other courses which has positive practical implications for the timing of uploading new teaching material. Finally, a clear relationship between the level of student LMS engagement and student course grade existed. For every additional week of zero LMS engagement, the odds of a student achieving. a grade lower than B were 1.67 times higher (95% CI 1.24, 2.26; p < 0.001), regardless of the course.
The rapid transition to online learning, as a consequence of COVID-19, has highlighted the risks of student disengagement, and the subsequent impact on lower student achievement across multiple courses. In addition, the authors investigated an ‘online learning bundling’ strategy that emerged; where students engaged more with a course when they were online submitting an assessment in a different course. These results emphasize the need for a university to implement greater cross-faculty coordination with reference to course design, uploading of information to LMS and timing of assessments. Improved coordination would provide a more effective online learning environment that maximizes student engagement and therefore achievement.
Introduction
The transition to higher education (HE) is often a complicated and difficult time for students (Kember, 2001). Many new HE students have moved directly from secondary education to HE and are not used to the typical HE environment. This is characterized by less structured class time per week, less direct contact with peers and teachers, and a greater expectation for independent learning. New HE students need to adjust quickly to these different styles of teaching and assessments, while adapting to the demands of a self-directed and independent approach to their academic work. Successfully adjusting to this increased level of independence in the first year is important, as it has a strong influence on total student effort and level of achievement, as well as increasing the likelihood of the student completing the whole course (Krause, 2001, 2005). Ultimately, it is each students’ ability to adjust and engage in the HE environment that becomes a strong determinant of their level of engagement and achievement.
The HE environment has several non-academic factors that are related to student's success, time management, engagement and participation. Students must learn to cope with the new and often competing demands of the HE environment. For example, the juggle between work-life balance, and the peaks and troughs of workload. Research by Scherer et al. (2017) found that effective time management was a significant predictor of tertiary academic outcomes, as those with poor time management found it hard to plan and were often rushed at the end of a course or at assessment time. Literature highlights that in HE, there is a significantly positive relationship between students with who do manage their time effectively and academic performance (Khan et al. (2020)).
Snyder (1971) often referred to the concept of students understanding the ‘hidden curriculum’ (i.e., students knowing which key assessment points they need to attend and when, in order to achieve). This concept is important when trying to understand how students best strategize or allocate their attention and their time and has been discussed as a potential time-management issue (Miller and Parlett, 1974). However, the concept that is under-researched is the balance between strategic use of time and potentially a miss-management of time, especially for 1st year HE students.
The second non-academic factor associated with academic success is student engagement, which is defined as ‘the quality of effort devoted to educationally purposeful activities that contribute directly to desired outcomes’ (Chickering and Gamson, 1987). One way to consider engagement is that it is a gauge of the strength of the relationship between students and their HE institution. The HE institutions aim is to create an environment that affords learning to happen, but ultimately the final act of engagement lies with the student actions. Understanding and measuring student engagement in HE is a challenge, as it has multi-dimensional mechanisms, such as educational challenge, active learning, student-staff interaction, and support on campus, to name a few.
One weakness of traditionally measuring engagement in HE has been the lack of tools to objectively understand student engagement. The most commonly used tool is the National Survey of Student Engagement (NSSE) which relies on self-reporting survey data. However, ‘active learning’ (i.e., frequency of class participation; Carini et al., 2006) has been used in previous research to provide an understanding of HE engagement level. Traditionally, this has been recorded during face-to-face HE program delivered on-campus that typically feature content taught in a classroom at a prescribed time, and supplemented with prescribed readings and assessment (Broadbent, 2017). One of the more recent advancements in trying understanding student's interaction with the virtual environment in is the evolving area of HE is learning analytics (LA). In particular the use of large scale educational data about learners and their contexts. In this area, researchers have presented information about learners and their environment, with an attempt to provide models for future behavior (Ranjeeth et al., 2020). However, it appears that with advances in LA there is still little recorded improvement to student learning, or learning support for students (e.g., Viberg et al., 2018). This raises the question about how insights from LA can help facilitate the transfer into learning and teaching practices.
Understanding engagement in online HE learning environments has shown mixed results when compared to face-to-face measures. Research has shown that students that have chosen their University course specifically because it is online are likely to be have been attracted by the high level of flexibility and independence it offers (Bernard et al., 2004). They are confident they have the skills to excel, they enjoy the learning style and have the time management skills required to succeed in the online environment. Indeed, HE students have reported that time management and regular interaction with content and other students were the top skills needed to be successful with online learning (Roper, 2007).
The impact of COVID-19 led to a rapid transition for most HE institutions from face-to-face teaching to online learning environments. While a few HE institutions had online courses or blended courses in place, the majority were not prepared for this rapid change to online delivery and therefore had minimal time to re-design course delivery for this new environment. Unfortunately, there is a paucity of research examining engagement with online learning tools, particularly for those who, due to COVID-19, are suddenly forced to transition from a face-to-face to online environment which was not their initial learning style choice. Many HE institutions use Learning Management Systems (LMS) and this provides an opportunity to explore student engagement via their online learning behaviors. While there are many inter-related factors that influence student engagement, the authors have attempted to respond to the call from Viberg et al. (2018) of combing the science of learning analytics with pedagogical knowledge. Therefore, in order to better support student achievement and enhance the understanding of student engagement behaviors the aims of this study are to; (1) to understand the online learning strategy of 1st year HE students (forced) into an online environment, and (2) to examine how the strategy adopted influences student achievement.
Materials and Methods
Participants
One hundred and seventy students who were enrolled in three courses as part of the first semester of their undergraduate degree participated in this study. As a response to COVID-19 these students, that were originally enrolled in face-to-face courses, were transferred to online delivery from week three.
Two courses had two assessment points across the semester; one mid-term assessment, and one assessment at the end of semester. While the third course had three assessments. For each course the structure included live online lectures, pre-recorded video content, and weekly online tutorials. The online delivery for the three courses was completed over nine-week period.
Online Engagement
Online engagement and activity was defined as the log data collected by the LMS, e.g., time spent or number of interactions students had with the LMS (Henrie et al., 2018). In this study online engagement was defined as the number of clicks per student recorded on the LMS. For each course online engagement data were extracted from the Blackboard Learning Management System using the in-built reporting feature. For each student, every time content was clicked (e.g., announcements, course materials, assessments) this information was recorded and stored within the LMS. While some engagement research uses log data of time spent logged into a page (e.g., Henrie et al., 2018), the authors found that this measure can give a false reading if a page was left open and not attended; thus giving the impression of a very long ‘engagement’ time with the LMS. Retrospective data covering the nine-week period were exported to an Excel spreadsheet, for each of the three courses separately. These data contained a daily breakdown of engagement information for each student (total number of clicks each day), for each of the three courses, across the nine-week period.
Student Achievement
Student achievement was measured using the final course grades that students received at the end of semester. The grading system ranged from 0 to 9, where 9 represented an ‘A+’ grade, 8 represented an ‘A’ grade, and 7 represented an ‘A−’. The lowest passing grade is 1 which represented a ‘C−’, while a 0 was a failure to pass. The final course grade was calculated by averaging the mid-term and final assessment grades.
Analysis
In the first instance, student online engagement with each of the three courses were summarized using descriptive statistics (mean ± SD). The descriptive analysis was stratified by assessment days, non-assessment days, and the day prior to assessment day. The relationship between an assessment due date and change in online engagement in other courses was examined by calculating the difference between engagement on the due date and the days prior. These differences were presented as Cohen’s D effect sizes with the following thresholds: 0.2 = small effect, 0.5 = medium effect, 0.8 = large effect (Cohen, 1988). All achievement and engagement data was de-identified in order that appropriate ethical standards were maintained.
Lastly, generalized linear mixed models were used to examine if the level of engagement with online content was associated with final course grades. The final grades were dichotomized into ‘B grade or higher’ and ‘Lower than a B grade’ (B grade = 5), as this was the middle grade. This was treated as the outcome variable. Student engagement data was summarized for each student as the number of weeks throughout the nine-week period where students recorded no engagement with the online LMS. This variable, along with the course (three levels) were added as fixed effects, while each student was added as a random effect to account for the repeated measures. These models were specified with a binomial distribution and logit link function and were fit in R software (v 4.0.0) using the lme4 package.
Results
The results section present data to answer the two research aims; (1) To understand the 1st year student’s online learning strategy and engagement and, (2) to examine how the strategy adopted influences student achievement.
The mean number of online interactions per day, along with the assessment dates for each course, is shown below in Figure 1. The spikes in student online engagement generally coincide with either the actual course assessment date (Figure 1, black vertical lines) or the uploading of key information related to an assessment onto the LMS (Figure 1, course 1(red) early June and course 2 (green) mid-May).
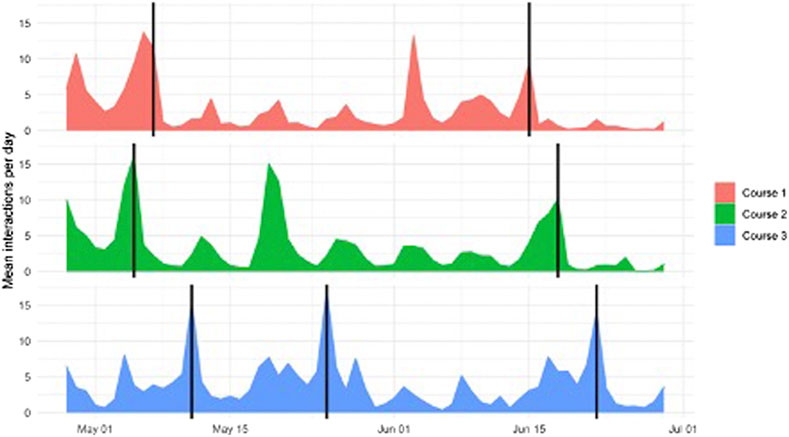
FIGURE 1. The distribution of online engagement across the semester, for each course. The black vertical bars represent the assessment dates for each course.
The values in Table 1 represent student engagement strategy through the mean number of interactions with the LMS per student per day per course and course grades.
The strategy showed the use of a low level of mean daily engagement during the semester (i.e., 3.11–3.94) with relatively high levels of engagement when an assessment was due (i.e., 10.5–15.6). There was a large difference between engagement levels on assessment due dates and ‘day-proceeding assessment due date’ compared to non-assessment days. Student strategy led to 312 and 453% more online interactions when assessments were due. Interactions with the LMS were higher around assessment due dates, however, it is also worth noting that a small part of this increase was caused by students submitting assessment; i.e., on average 3–4 interactions per course to submit an assessment. It is worth noting that each week included online lectures, workshops, discussion boards and readings, so to have a daily use of only 2–3 interactions per day would be considered quite low in relation to the staff expectations of the course demands.
A key part of this study was to understand the learning curves of students in a COVID-19 environment and the link to achievement. It is important to consider the potential achievement implications for the students that adopted a ‘low or no online engagement’ strategy, as across the nine weeks of the three courses, approximately 34% (n = 53) of all students had two weeks of zero engagement with all of their three courses.
The relationship between final course grades and the number of weeks with no online engagement is presented in Figure 2 below. All three courses displayed a similar trend; as the number of weeks with no online engagement increased, the probability of achieving a B grade or higher significantly decreased. On average, for every additional week of no online engagement, the odds of achieving a B grade or better were 0.60 (95% CI 0.44, 0.81; p < 0.001), regardless of course. The inverse of this ratio can be interpreted as: the odds of achieving a grade lower than B are 1.67 times higher for every additional week of no online engagement.
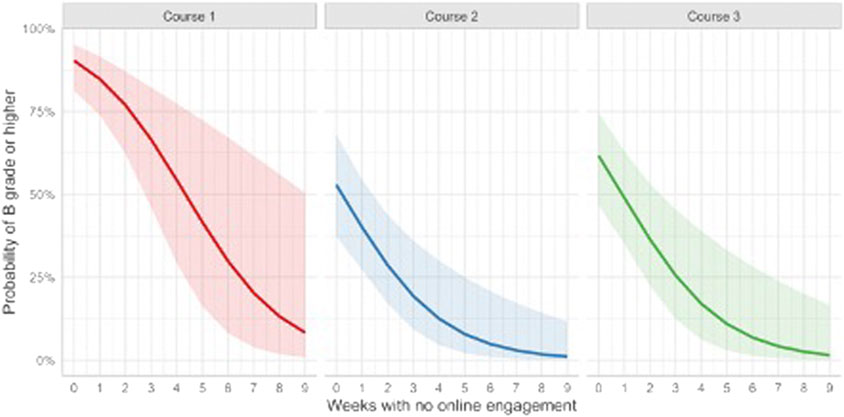
FIGURE 2. Relationship between student achievement and the number of weeks with no online engagement. Estimates obtained from a generalized linear mixed model (binomial distribution, logit link). The shaded regions represent 95% confidence intervals.
The final data presented in this study explored learning curves of students online engagement during COVID-19 when an assessment was due in one of the three courses. Table 2 below demonstrates the effect size differences between the online LMS engagement level in one course, coinciding with an assessment due in another course. This measure was determined by comparing the LMS values (mean and SD) on the day of assessment in one course to LMS values of the day before in another course. Findings showed that when an assessment was due in one course, for 80% of the time the students subsequent online engagement increased in one or both of the other two courses, despite those other two courses not having assessments due at that time. For the majority of the cases there were small to moderate effect size differences between an assessment due date and an increase in online engagement in the other courses. This strategy could be described as a ‘bundling effect’ of cross-course online engagement occurring due to assessment deadlines. The two exceptions to the ‘bundling effect’ were, (1) at the start of the semester, when online use was high across all courses as students were adjusting to a new online environment and (2) when an assessment in another course had occurred two days earlier.
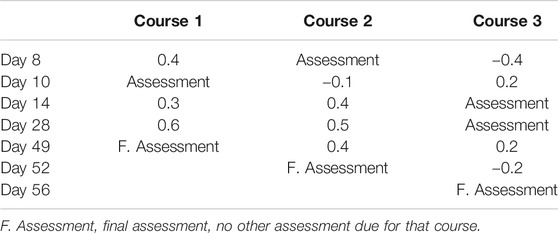
TABLE 2. Effect sizes difference of online engagement in one course, when an assessment is due in another.
Discussion
This study aimed to understand the student learning curves of 1st semester, 1st year HE students in a COVID-19 enforced online environment, and the relationship with achievement. In order to explore these topical questions, a mixed method modeling was used of daily engagement data from the University LMS and end of semester grades. The clear result from this study has been gaining an understanding of the student engagement strategy and it’s significant connection with the timing of assessments. Specifically, student online engagement displayed large peaks and troughs that correlated with assessment due dates. For many students, they had prolonged periods of little or no engagement with an online course, until close to an assessment due date. The ‘heart-beat’ graphic of Figure 1 that represented the level of online engagement with the LMS during the 56 days of the course, and the assessment due dates for the 3 analyzed courses demonstrated a clear interrelatedness between student online engagement and assessment due dates. This strategy of selective interrelated behavior of ‘when to engage’ online can be in part explained by Snyder (1971) and Miller and Parlett (1974) research of the ‘hidden curriculum’. ‘Hidden curriculum’ research demonstrated that students can be strategic about their use of time and energy in relation to course work and to assessment, and the study approaches in this paper supports this i.e., students spent more effort on tasks relating to assessment. What is uniquely demonstrated in Figure 1, is just how selective and strong the student behavior is toward assessment timing, but also worryingly the low levels of engagement between assessment dates, in particular the 53 students who had two weeks of no online engagement with their three courses.
Most HE literature links sustained effort and engagement to students’ success. However, this is strategy has not been demonstrated by the students in this study, where students were forced (quickly) to move to the online learning style. Figure 1, highlighted student engagement was low between assessment due dates, and thus not sustained evenly over the course. Table 1 also showed that the level of daily engagement on the day of and including the day before an assessment was due, was on average 388% (SD 58%) higher than the average of all the other days during the semester. These numbers clearly represent a learning curve strategy where students have focused their engagement with the LMS predominately toward assessment dates; consequently, creating a peaks and troughs approach. This strategy appears to be contrary to HE literature that demonstrates higher engagement, i.e., sustained, and more dedicate time to a subject, the more success a student has (Carini et al., 2006). Having high levels of engagement in learning, but also sustained effort has strong links to building the foundation of skills needed not only for success in HE, but also post HE (Kuh, 2003). In an online learning environment, where a lack of face-face interaction occurs, exceptional online engagement is needed in order to be successful (Bryson, 2014).
While one view of the results in Table 1 and Figure 1, might support a selective approach to the use of time engaged with the LMS in relation to assessments: a contrasting view of potential concern, for these students in these trough periods. In this study, the authors investigated the peaks and troughs approach, to see if low levels of LMS engagement was a disadvantage for students. The results shown in Figure 2 demonstrated that it was a disadvantage, and that for every additional week of no LMS engagement, the odds of achieving a grade lower than B were almost twice as high. This result unfortunately illustrated that students who implemented a strategy of no LMS engagement for a period, such as a week or more, had a strong negative impact on their final grade. This finding is in line with literature, which links sustained effort and engagement, to a student's success (Chickering, and Gamson, 1987), instead of a peaks and troughs engagement approach as highlighted in this study.
An unexpected result to arise from the analysis of LMS interactions with this research was presented in Table 2. Here the authors identified that the act of working on one course for a student assessment coincided with increasing engagement in one or both other courses. That is, when a student was online working on one course assessment, they also appeared to use that opportunity to bundle their LMS time and log on and to another course. This could be considered an ‘online bundle learning’ strategy. This strategy has been evidenced in other online environments, for example when the viewing or the sale of one product is bundled to that of another, in order to get greater sales and/or views (Jiang et al., 2018). The results in Table 2 showed effect size differences and ‘online bundle learning’ occurred 80% of the time a student was online for a course with an assessment due, they also had increased levels of LMS engagement in one or both of their other courses. The implications for the HE course leaders is to recognize the positive engagement ‘bundle’ effect when they plan the time to upload new material to their online course so that the engagement of the students is maximised.
A concluding point from Figure 1 is the impact on engagement of the timing of the final course assessments in relation to each other. While the timing of assessments is a challenge in HE, with multiple courses all needing to schedule assessments, having a short space between assessments due dates, may put substantiable pressure on students to complete these assessments. The timing of assessments is a key topic that students in HE cite as a major source of stress (Divaris, et al., 2008). The timing of assessments is an area where there needs to be greater cross faculty integration, to assist with student stress management and well-being (Divaris et al., 2008). Especially with 1st year students, where most courses are the same for students, there is the opportunity for faculty staff to work together and space the assessments more.
Conclusion
In summary, this research aimed to firstly understand the student learning strategy in the enforced COVID-19 environment and the learning curves used by 1st year students. This large cohort of students was a particularly important group to understand, as the strategies developed in the 1st semester of a degree can have an impact on overall HE achievement (Khan et al.. (2020). This study revealed that during COVID-19 student online learning engagement followed a strong pattern of peaks and troughs, where their engagement was almost 400% greater when an assessment was due, compared to other times during the semester.
The second research question considered the influence of learning strategy on achievement. The results indicated that if students implemented a compounding none-engagement strategy with a course, then their grades significantly decrease. Success in HE is traditionally linked to sustained engagement, in a course of study, but the learning curves observed did not support this traditional strategy. An alternative ‘online bundle learning’ strategy emerged that occurred across multiple courses. Recognition of this ‘bundle strategy’ in cross faculty communication is an area that needs future investigation. Not only to improve the timing of assessments for students, but to also upload material online to all courses at a time when a student is likely to be submitting an assessment in another course as the student is likely to engage more with the uploaded material at this time.
Data Availability Statement
The raw data supporting the conclusions of this article will be made available by the authors, without undue reservation.
Author Contributions
This article has been written by the four authors listed. The first two authors have contributed equally to this work and share the first authorship. The third and fourth authors were primarily focused on the data collection and analysis and editing, while the first two authors wrote the 1st draft and final edits.
Conflict of Interest
The authors declare that the research was conducted in the absence of any commercial or financial relationships that could be construed as a potential conflict of interest.
References
Bernard, R., Abrami, P., Lou, Y., Borokhovski, E., Wade, A., Wozney, L., et al. (2004). How does distance education compare with classroom instruction? A meta-analysis of the empirical literature. Rev. Educ. Res. 74, 112. doi:10.3102/00346543074003379
Broadbent, J. (2017). Comparing online and blended learner’s self-regulated learning strategies and academic performance. Internet Higher Educ. 33, 24–32. doi:10.1016/j.iheduc.2017.01.004
Carini, R. M., Kuh, G. D., and Klein, S. P. (2006). Student engagement and student learning: testing the linkages*. Res. Higher Educ. 47 (1), 1–32. doi:10.1007/s11162-005-8150-9
C. Bryson. (2014). Understanding and developing student engagement. 1st ed. Abingdon: Routledge. doi:10.4324/9781315813691
Chickering, A., and Gamson, Z. (1987). Seven principles for good practice in undergraduate education. Am. Assoc. Higher Educ. Bull. 39 (7), 3–7.
Cohen, J. (1988). Statistical power analysis for the behavioral sciences. New York, NY: Routledge Academic.
Divaris, K., Barlow, P. J., Chendea, S. A., Cheong, W. S., Dounis, A., Dragan, I. F., et al. (2008). The academic environment: the students’ perspective. Eur. J. Dental Educ. 12 (s1), 120–130. doi:10.1111/j.1600-0579.2007.00494.x
Henrie, C. R., Bodily, R., Larsen, R., and Graham, C. R. (2018). Exploring the potential of LMS log data as a proxy measure of student engagement. J. Comput. Higher Educ. 30 (2), 344–362. doi:10.1007/s12528-017-9161-1
Jiang, Y., Liu, Y., Wang, H., Shang, J., and Ding, S. (2018). Online pricing with bundling and coupon discounts. Int. J. Prod. Res. 56 (5), 1773–1788. doi:10.1080/00207543.2015.1112443
Kember, D. (2001). Beliefs about knowledge and the process of teaching and learning as a factor in adjusting to study in higher education. Stud. Higher Educ. 26 (2), 205–221. doi:10.1080/03075070120052116
Khan, I. A., Zeb, A., Ahmad, S., and Ullah, R. (2020). Relationship between university students time management skills and their academic performance. Rev. Econ. Dev. Stud. 5 (4), 853–858. doi:10.26710/reads.v5i4.900
Krause, D. (2005). Serious thoughts about dropping out in first year: trends, patterns and implications for higher education. Stud. Learn. Eval. Innovation Dev. 2 (3), 55–67. doi:10.5430/ijhe.v10n3p246
Krause, K. (2001). The university essay writing experience: a pathway for academic integration during transition. Higher Educ. Res. Dev. 20 (2), 147–168. doi:10.1080/07294360123586
Kuh, G. D. (2003). What we're learning about student engagement from NSSE: benchmarks for effective educational practices. Change Mag. Higher Learn. 35 (2), 24–32.
Miller, C. M. I., and Parlett, M. (1974). Up to the Mark: a study of the examination game. Guildford: Society for Research into Higher Education.
Ranjeeth, S., Latchoumi, T. P., and Paul, P. V. (2020). A survey on predictive models of learning analytics. Proced. Comput. Sci. 167, 37–46. doi:10.1016/j.procs.2020.03.180
Roper, A. (2007). How students develop online learning skills. Educause Q. 14, 72–81. doi:10.4324/9780203415986-21
Scherer, S., Talley, C. P., and Fife, J. E. (2017). How personal factors influence academic behavior and GPA in African American STEM students. SAGE Open 7 (2), 133–139. doi:10.1177/2158244017704686
Keywords: online bundle learning, engagment, higher education, COVID-19, Learning Management Systems
Citation: Millar S-K, Spencer K, Stewart T and Dong M (2021) Learning Curves in COVID-19: Student Strategies in the ‘new normal’?. Front. Educ. 6:641262. doi: 10.3389/feduc.2021.641262
Received: 13 December 2020; Accepted: 16 February 2021;
Published: 19 March 2021.
Edited by:
Geneviève Pagé, University of Quebec in Outaouais, CanadaReviewed by:
Tracy XP Zou, The University of Hong Kong, Hong KongSally Hamouda, Cairo University, Egypt
Copyright © 2021 Millar, Spencer, Stewart and Dong. This is an open-access article distributed under the terms of the Creative Commons Attribution License (CC BY). The use, distribution or reproduction in other forums is permitted, provided the original author(s) and the copyright owner(s) are credited and that the original publication in this journal is cited, in accordance with accepted academic practice. No use, distribution or reproduction is permitted which does not comply with these terms.
*Correspondence: Sarah-Kate Millar, c2ttaWxsYXJAYXV0LmFjLm56