- 1Department of Psychology and the Rutgers Implicit Social Cognition Laboratory, Rutgers University, Newark, NJ, United States
- 2Department of Earth and Environmental Sciences, Rutgers University, Newark, NJ, United States
Increasing academic participation among students from ethnic-racial underrepresented groups in STEM yields societal benefits including ameliorating economic ramifications of the labor shortages in STEM, improving scientific innovation, and providing opportunity, access, and participation in high-status STEM fields. Two longitudinal studies with students from underrepresented groups investigated the role of active learning interventions in the development of STEM self-efficacy and intentions to pursue STEM in the future. Study 1 longitudinally tracked high school students participating in a 4-week geoscience program that applied active learning techniques ranging from hands on experiments to peer discussion. High school student participants displayed increases in self-efficacy and STEM intentions from the start to completion of the program, an effect that was observed exclusively among those who reported strong program quality. Study 2 examined the role of mentorship effectiveness with a sample of community college STEM students interested in transferring to a 4-year college. Students’ relatively strong self-efficacy and STEM intentions at the start of the semester remained stable through the end of the semester. Altogether, the present research highlights the role of positive, inclusive educational climates in promoting STEM success among students from underrepresented group members.
Introduction
Among all students who enter college with intent to pursue STEM, only 43% of Latinx and 34% of Black students earn a STEM degree, compared to 58% of White students (Riegle-Crumb et al., 2019). Indeed, ethnic-racial identification is the strongest predictor of who leaves STEM during college, above and beyond other relevant characteristics such as gender and socio-economic status (Shaw and Barbuti, 2010). The relatively high STEM attrition among students who identify with underrepresented groups (URGs; Black, Latinx, Native American, and women) is caused in part by relatively unwelcoming atmospheres that impair URG confidence and undermine STEM intentions (Seymour and Hewitt, 1997). Similarly, the numeric underrepresentation of individuals from URGs in STEM creates cultural stereotypes that link STEM competence to White and Asian men (Miller et al., 2015; Eaton et al., 2020), which make stigmatized identities salient in STEM classrooms (Cheryan et al., 2015), especially under testing circumstances (Steele, 1997). Finally, stereotype activation impairs performance (Spencer et al., 2016), diminishes personal belief in the ability to succeed (self-efficacy) in a stereotyped domain (Dasgupta, 2011), and drives academic isolation (Swarat et al., 2004). To address the barriers that affect STEM attrition among URGs, researchers are seeking to identify which STEM education settings are conducive for URG success and why.
Active learning strategies counter challenges associated with URG numeric underrepresentation in STEM education settings by facilitating collaborative, inclusive, and self-efficacious learning environments (Theobald et al., 2020). Furthermore, active learning strategies prepare students and trainees for the flexible thinking required among most contemporary careers (Ahmad, 2019; Hesketh, 1997). Indeed, implementing more active learning strategies in STEM education increases URG participation in STEM careers, which in turn promotes United States economic competitiveness by improving innovation through increased diversity of thought (Richard, 2000; Lee and Buxton, 2010). The present research couches active learning within Bandura (1978) triadic reciprocality among the environment, personal factors, and behavior. As displayed in Figure 1, and discussed below, positive educational climates, self-efficacy, and intentions to persist in STEM are all inextricably linked (Nauta et al., 1998; Nauta et al., 2002; Vogt, 2008; Zeldin et al., 2008; Byars-Winston et al., 2010). Positive educational climates adopt active learning and are supportive and inclusive, thus promoting student dignity and feelings of agency over their learning (Thapa et al., 2012). These factors are theorized to disproportionately benefit URG students’ self-efficacy and intentions to persist in STEM (Seymour and Hewitt, 1997). We test these components and their interrelations across two longitudinal STEM intervention studies with URG high school and community college students.
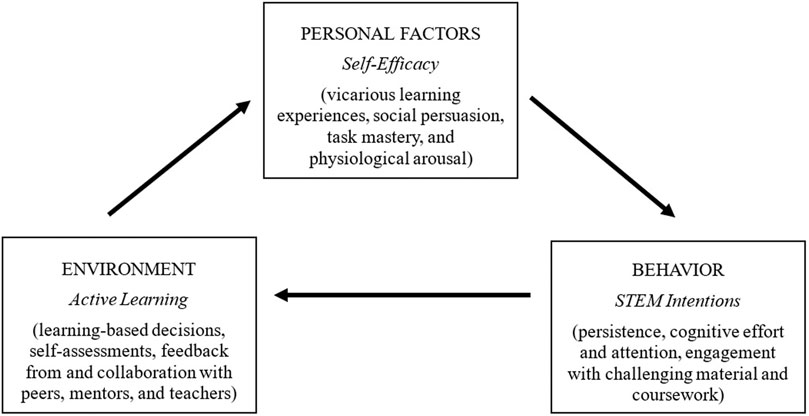
FIGURE 1. Bandura (1978) model of triadic reciprocality applied to STEM environments, personal factors, and STEM intentions.
Active Learning
Learning STEM material via traditional, passive classroom settings is historically popular in the United States (Wise, 1996), but active learning and its processes and benefits are unparalleled, including in STEM (for a review, see Ishiyama, 2013; for a meta-analyses, see Freeman et al., 2014; Schroeder et al., 2007). Active learning strategies are rooted in constructivist theories of learning that position the learner in control of their own knowledge acquisition, compared to the traditional teacher-student transmission of knowledge referred to as “teaching by telling” (Ivancic and Hesketh, 1995; Smith et al., 1997). Instead of placing the responsibility for learning-based decisions on an external source, typically the teacher, the learner oversees choosing information to process while monitoring physiological arousal including regulating one’s stress response during the learning process (Iran-Nejad, 1990). Active learning requires exploration and experimentation with a goal to develop domain specific skills (Ishiyama, 2013). Though active learning entails a wide breadth of potential activities that can be incorporated inside or outside of traditional classrooms, the central goal is for the learner to be in control of the learning process to create flexible and adaptive thinking.
Research on educational climates and applied STEM coursework also support the benefits of active over passive learning environments (McNeil, 2000; Thapa et al., 2012; Sublett, and Plasman, 2017). Educational climate research emphasizes that students experience superior short-term and long-term outcomes when embedded in supportive, cooperative, and respectful educational environments, where students are encouraged to actively participate in the learning process (Thapa et al., 2012). Research on applied STEM courses highlight how hands-on learning opportunities help contextualize more abstract STEM concepts in real-world settings, which promote academic engagement and reduces anxiety (Bozick et al., 2014; Sublett, and Plasman, 2017). Finally, a meta-analysis of 225 studies that directly compared the performance of students participating in active versus passive (traditional) STEM courses showed that not only does active learning significantly improve student performance, students in active learning settings were 1.5 times more likely to pass STEM courses (Freeman et al., 2014).
Another factor central to active learning environments is an inclusive and respectful community of peers, mentors, and teachers (Theobald et al., 2020). Active learning environments characterized by a “culture of inclusion” close achievement gaps between URG and non-URG students in STEM, because they provide dignity, collaboration, and communication of confidence in student ability (Theobald et al., 2020). Engaging with peers on relevant material improves performance, retention, and critical thinking (Stefanou, and Salisbury-Glennon, 2002; Kudish et al., 2016). For example, small group interactions in a traditional lecture hall gateway course increased performance and retention of Black students in STEM, compared to Black students who participated in a control group (Treisman, 1992). Discussing science promotes expert-like thinking (Hammer, 1994; Otero and Gray, 2008) and reduces academic isolation, which promotes performance and persistence among URG students (Swarat et al., 2004). Mentors and teachers guide learners through active learning by teaching techniques to regulate physiological arousal, attention, and effort, while encouraging students to construct their own conceptual understanding of tasks and constructs (Bell, and Kozlowski, 2008). Even though active learning emphasizes the learners’ role in knowledge acquisition, learners still need guidance from mentors to learn effective strategies while receiving feedback on their progress. Mentoring programs even present the capacity to close self-efficacy gaps between URG and non-URG college students (MacPhee, Farro, and Canetto, 2013).
Given the evidence for active learning as an effective mode of learning, research questions now center on the efficacy of different types of strategies (Bell and Kozlowski, 2008; Dou et al., 2018), who they benefit (Ballen et al., 2017), and the mechanisms that underlie them (Ballen et al., 2017; Cleveland et al., 2017). We contributed to this research by testing the role of active learning in promoting self-efficacy in STEM and in strengthening intentions to pursue STEM.
Self-Efficacy
Performance in STEM gateway courses is repeatedly found to be a primary predictor of STEM persistence during college, and URG students typically underachieve in these notoriously rigorous courses relative to non-URG students (Chen and Soldner, 2013; Aulck et al., 2017), even after controlling for prior performance and preparation, suggesting that performance gaps are attributed to psychological processes instead of ability (Haak et al., 2011). One such psychological process linked to STEM performance is self-efficacy, which refers to an individual’s belief in their capabilities in a certain domain, as well as being able to exercise control over their success in said domain (Bandura, 1977, 1982). Self-efficacy predicts future performance above and beyond past performance (Bandura and Locke, 2003). Among students who intend to major in STEM during college, those who leave STEM demonstrate lower self-efficacy than those who persist in STEM (Shaw and Barbuti, 2010).
Self-efficacy is a malleable source of domain-specific motivation and it covaries with appraisals of personal experiences, such as past performance, and situational factors, such as salient stereotypes (Fogliati, and Bussey, 2013; Schuck, 1989). Weak self-efficacy results in impaired academic performances (Pajares, 2005; Vogt, 2008), thereby creating a feedback loop such that personal interpretations of past performances alter self-beliefs and interactions with their environments, which then influence future performances (Pajares, 1996). As depicted in Figure 1, Bandura (1978) originally conceptualized this reinforcing cycle as triadic reciprocality in which environmental, behavioral, and personal factors continuously interact to either bolster or diminish performance. Active learning can increase self-efficacy via Bandura (1978) sources of self-efficacy–mastery experiences, psychological arousal, vicarious learning, and social persuasion. Mastery experiences such as hands-on guided exercises can increase self-efficacy over time, because the learner first decides how to approach the task, then continuously observes themself developing the skill, while simultaneously receiving positive feedback on their progress from teachers and mentors. For URG students pursuing STEM, mentorship enhances self-efficacy and intentions to persist because supportive mentors represent a powerful source of social persuasion by providing encouragement and recognition as a STEM group member (Betz and Schifano, 2000; Carlone and Johnson, 2007; Stout, Dasgupta, Hunsinger, and McManus, 2011). Mentors can also engage in intellectual discussions with the learners, which is a simple, yet effective active learning strategy that boosts self-efficacy and performance (Hammer, 1994; Otero and Gray, 2008).
Once a sufficient level of self-efficacy is achieved, it serves as a source of domain specific motivation where people put forth more mental effort (Rittmayer and Beier, 2008) and persist longer (Bandura, 1977). Low self-efficacy results in avoidance of tasks and relevant domains, whereas high self-efficacy promotes engagement, active participation, and sustained pursuit of challenging tasks (Pajares, 1996; Komarraju and Nadler, 2013). Self-efficacy is the mechanism underlying the relation between engaging in active learning strategies and superior performance among URG students in STEM (Ballen et al., 2017). Furthermore, the theorized link between self-efficacy and improved performance is due to increased self-regulation during performance such that one becomes more cognitively engaged, experiences lower physiological arousal, and involves continual self-evaluations during and after the task (Bandura, 1991; Zimmerman, 2000). Put another way, self-efficacious students are more likely to display the positive affect, attitudes, and self-directed behaviors needed for active learning (Pajares, 1996; Pajares and Schunk, 2001).
Intentions to Pursue and Persist in STEM
STEM intentions reflect students’ short- and long-term goals to pursue a STEM major, attend and complete graduate school in STEM, and establish a career in STEM, which are consistently associated with STEM persistence (Shaw and Barbuti, 2010; Maltese and Tai, 2011). Self-efficacy is intrinsically linked to career intentions, because students must first believe in their ability to produce a desired outcome in a given domain before they become motivated to pursue a career pathway (Bandura, 1991; Bandura, Barbaranelli, Caprara, and Pastorelli, 2001). Unsurprisingly, self-efficacy is a consistent predictor of STEM intentions among all students (Brown et al., 2016; Lent et al., 2016), and long-term engagement among URG students (Estrada, Woodcock, Hernandez, and Schultz, 2011). Some research even finds that STEM self-efficacy explains why participation in an academic support program is related to long-term intentions to pursue STEM careers (Syed et al., 2011). STEM self-efficacy appears to be a stronger predictor of career choice among URGs compared to non-URGs, at least among female students (Larose, Ratelle, Guay, Senécal, and Harvey, 2006).
STEM Educational Interventions: High School and Community College
STEM interventions with URG students in secondary and higher education settings often focus on developing self-efficacy because of its relation to performance and persistence (Betz and Schifano, 2000; Rittmayer, and Beier, 2008; Ballen et al., 2017; Liu, 2018; Kuchynka, Gates, and Rivera, 2020). In high school, STEM participation is pivotal for long-term STEM engagement (Alkhasawneh and Hargraves, 2014; Chang, et al., 2014; Lee and Luykx, 2006; Mendez, Buskirk, Lohr, and Haag, 2008; Shaw, and Barbuti, 2010; Terenzini and Pascarella, 1980; Wang, 2013), but most students in the United States report a relative dislike and avoidance of STEM by high school (Chen and Soldner, 2013). Low STEM engagement during high school is believed to be caused by inadequate exposure to varied STEM materials (Kuchynka, Gates, and Rivera, 2020) and a lack of applied STEM courses that bridge abstract concepts to real-world applications (Bozick et al., 2014; Sublett, and Plasman, 2017). Furthermore, because they are more likely to attend high schools with inadequate resources (Duncombe and Cassidy, 2016) and encounter cultural stereotypes that undermine their STEM competence (Dasgupta, 2011), URG students are at an increased risk for avoiding versus approaching STEM during high school. Fortunately, active learning environments can counteract each of these barriers and in turn promote STEM self-efficacy and intentions to pursue STEM. Study 1 examines these hypotheses with URG high school students in a science education program.
Study 2 investigates these same hypotheses with URG community college students in a STEM mentoring program. More than half of URG students who received a STEM degree from a 4-year university transferred from community colleges (National Research Council and National Academy of Engineering, 2012). Consistent with the above literature review, self-efficacy is associated with future career decisions among community college students (Collins and Bissell, 2004; Amelink, Artis, and King Liu, 2015). Qualitative studies have identified socio-cultural issues (e.g., first generation status) and inadequate advising as two of the largest barriers to a successful transfer to a 4-year university (Gard, Paton, and Gosselin, 2012). Guidance from mentors, however, can improve community college students’ self-efficacy over time, because mentors teach mentees strategies for a successful transfer (e.g., frequently asking clarification questions) and coping mechanisms, and they provide social support (Amelink, Artis, and King Liu, 2015).
Overview of the Present Research
Two longitudinal studies adopted active learning strategies to increase STEM engagement among URG students. The interventions targeted two different phases of students’ educational development, high school students participating in a 4-week intensive geoscience summer program (Study 1) and community college students participating in a mentoring program (Study 2). Both studies investigate changes in STEM self-efficacy and future intentions to pursue STEM goals among URG students, and the reciprocal relation between these variables.
Study 1
Study 1’s longitudinal design tested if a 4-week geoscience summer program yields positive changes in STEM self-efficacy and intentions to pursue STEM with a sample of URG high school students. Past educational interventions that focus on developing self-efficacy include one or more of the following three components - social persuasion (positive and constructive feedback from important others), vicarious experience (learning STEM from observing peers or mentors), or mastery experience (hands-on exercises)—and all demonstrate varying degrees of success in cultivating self-efficacy (for a review, see Rittmayer and Beier, 2008). Study 1’s geoscience program combines these intervention components, so we hypothesized that student participants will exhibit stronger STEM self-efficacy (Hypothesis 1) and its correlate stronger intentions to pursue STEM in the future (Hypothesis 2) from start to end of the program. Further, we explored whether the hypothesized changes in STEM self-efficacy explained increases in STEM intentions or vice versa. Finally, we explored if any observed changes in both criteria were qualified by perceptions of program quality. Previous research indicates that satisfaction with one’s learning environment moderates psychological benefits and performance (Tinto, 1993; Allen and Robbins, 2008). A test of program quality also allowed us to demonstrate that any changes in STEM psychological outcomes as function of completing the program are not simply due to a longitudinal aging effect, which is when variables change as a result of a cohort aging as opposed to participation in the intervention itself (Blanchard, Bunker, and Wachs, 1977).
Method
Participants and Design
The study adopted a one-factor three-level (Time: 1/Day 1, 2/Day 9, 3/Day 19) within-participants repeated-measures design. We invited all high school students enrolled in a 4-week geoscience program at a northeastern university during the summers of 2018 (n = 53) and 2019 (n = 45) to participate in the study. Students participated in the program during each weekday for the duration of the 4-weeks from 8am to 2pm. Due to attrition, the total sample size of students who completed all measurements varied across the three time points (Times 1–3 Ns = 97, 95, 88). According to G Power, a repeated measures MANCOVA with one group and three time-points yields a sample size of 86 to detect a small to medium effect size. Thus, our sample presents sufficient statistical power. Table 1 lists all participants’ demographics. Participation was voluntary, but students received a stipend for completing the program. We obtained both parental consent and child assent. This research was approved by the Rutgers University Institutional Review Board and was part of a larger preregistered study (see Open Science Foundation #32267; https://osf.io/a63m5/).
Program and Procedure
The summer geoscience program was a 4-week intervention that educated high school students from a major urban city about earth resources, energy, and the environment (Gates, 2019). Student participants received mentorship from teachers and undergraduate college students and were immersed in a community of mostly Latinx and Black peers.1 To the extent possible, the undergraduate student mentees were purposefully selected to match the demographics of the student participants (e.g., most were URG and from the same urban area) and were recruited from the Garden State Louis Stokes Alliance for Minority Participation (GSLSAMP; http://gslsamp.rutgers.edu/). The high school students actively participated in applied science exercises, such as taking water and soil samples, analyzing them with professional equipment in the laboratory, and observing geoscience in its natural environment during field trips. More specifically, active learning components included rock and mineral identification as applied to everyday and industrial use, seismic refraction profiling, radioactivity of rocks and radon in soil, assaying magnetite ore, gauging stations and flooding on a stream table, and geothermal energy. Instead of learning about abstract theoretical concepts, the geoscience program provided real-world applications for earth science material.
The measured variables listed below were completed online through Inquisit Web 5.0 (Millisecond software, 2018) in a computer classroom, three times across the 4-week program, unless otherwise noted in parentheses. Time 1 was the very first activity on day 1 of the program, Time 2 occurred around day 9, which was around the mid-point, and Time 3 was measured on day 19, the final day of the program. Participants completed the measures in the order listed below. Finally, at the end of Time 3, participants were provided with a full description of the study goals and the researchers’ contact information.
Measured Variables
STEM Self-Efficacy
Adapted from Stout, Dasgupta, Hunsinger, and McManus (2011), participants responded to two items that assessed their appraisals of their talent and confidence in science—1) “Do you think you have a talent for science?” and 2) “How confident do you feel about your science ability?”—on 7-point scales ranging from 0 (not at all) to 6 (very much so). Higher scores indicate stronger STEM self-efficacy (Times 1–3 rs = 0.86, 0.78, 0.79).
STEM Intentions
Adapted from research by Dasgupta and colleagues (Dasgupta, 2011; Stout et al., 2011), participants responded to two items that assessed their future intentions and aspirations in science—1) “If given the opportunity, how likely are you to pursue classes and courses in science in high school or college in the future?” and 2) “If given the opportunity, how likely are you to pursue a future job or career in science?” on 7-point scales ranging from 0 (not at all likely) to 6 (very likely). Higher scores indicate stronger future STEM intentions (Times 1–3 rs = 0.91, 0.92, 0.88).
Program Evaluation (Time 3 only)
Three items evaluated participants’ perceived quality of the program - 1) “Overall, how satisfied were you with the science summer program?,” 2) “Overall, how would you rate the quality of the science summer program?,” and 3) “Overall, how would you rate your learning in the science summer program?” on a 5-point scale ranging from 0 (very poor) to 4 (excellent), with 2 (average) as the midpoint. Higher scores indicate more favorable program evaluations (α = 0.86).
Demographics
Participants completed a demographics and background questionnaire at all three time points, which included gender, grade point average (GPA), age, parents’ education level, and ethnic-racial identification.
Results
Table 2 reports the descriptive statistics for all measured variables as a function of Time and the zero-order correlations among these variables. In the analyses below, we sought to understand the role of the intervention over time in STEM self-efficacy and future STEM intentions above and beyond any explained variance of year in high school and parents’ level of education. More advanced students may start the program with stronger STEM self-efficacy and future STEM intentions due to their advanced experiences with high school science courses. Similarly, students from higher socio-economic status (SES) backgrounds may also start the program with stronger STEM self-efficacy and future intentions because of their access to greater academic and extracurricular resources in STEM.
Effect of Time on STEM Self-Efficacy and STEM Intentions
To test our two main hypotheses, we ran a repeated measures MANCOVA in which Time was the within-subject three-level factor (Times 1–3), with the covariates discussed above included. Table 4 lists means and standard errors of the outcome variables as a function of Time. The multivariate effect of Time was marginally significant [F (4, 67) = 2.16, p = 0.083, ηp2 = 0.114]. Next, we ran pairwise comparisons to decompose the effect of Time on each of the two outcome variables.
First, STEM self-efficacy increased significantly from Time 1 to Time 3 [Mdiff = 0.37 SE = 0.15, p = 0.013, 95% CI (0.08, 0.66)], marginally increased from Time 2 to Time 3 [Mdiff = 0.21 SE = 0.11, p = 0.07, 95% CI (−0.02, 0.43)], and the change from Time 1 to Time 2 was not significant (p > 0.20). Second, and similar to STEM self-efficacy, future STEM intentions increased significantly from Time 1 to Time 3 [Mdiff = 0.41 SE = 0.18, p = 0.021, 95% CI (0.07, 0.76)], marginally increased from Time 2 to Time 3 [Mdiff = 0.29, SE = 0.17, p = 0.089, 95% CI (−0.05, 0.63)], and the change from Time 1 to Time 2 was not significant (p > 0.35). Thus, Hypotheses 1 and 2 were supported when we tested changes in the STEM psychological constructs from start to end of the program.
Exploratory Tests
Relation Between STEM Self-Efficacy and STEM Intentions
Next, we explored if changes in STEM self-efficacy mediates changes in future STEM intentions or vice versa. To this end, we used Montoya and Hayes’ (2017) MEMORE macro for repeated measures mediation, using Time as the predictor in both analyses. In the first analysis, STEM self-efficacy was the repeated measures mediator and future STEM intentions was the outcome variable, then this order was reversed in the second analysis.
Results showed that STEM self-efficacy indirectly predicted future STEM intentions, b = 0.15, SE = 0.10, 95% CI (0.01, 0.39). As per Figure 2, strengthening STEM self-efficacy from Time 1 to Time 3 appears to explain changes in student participants’ intentions to pursue STEM in the future. Further, the direct effect of the duration of the intervention on future STEM intentions was not significant, b = 0.21, SE = 0.16, p = 0.21, 95% CI [-0.12, 0.53], and the total effect reached significance, b = 0.36, SE = 0.17, p = 0.039, 95% CI [0.02, 0.70]. Similarly, results also showed that future STEM intentions indirectly predicted STEM self-efficacy, b = 0.11, SE = 0.07, 95% CI (0.002, 0.28). As per Figure 3, strengthening future STEM intentions bolstered their STEM self-efficacy from Time 1 to Time 3. Further the direct effect of the duration of the intervention on STEM self-efficacy was non-significant, b = 0.22, SE = 0.13, p = 0.09, 95% CI (−0.04, 0.48), and the total effect reached significance b = 0.33, SE = 0.14, p = 0.017, 95% CI (0.06, 0.60).
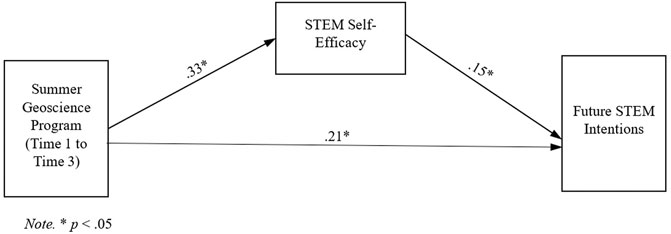
FIGURE 2. Statistical model depicting effects of the summer geoscience program on future STEM intentions, mediated by STEM self-efficacy.
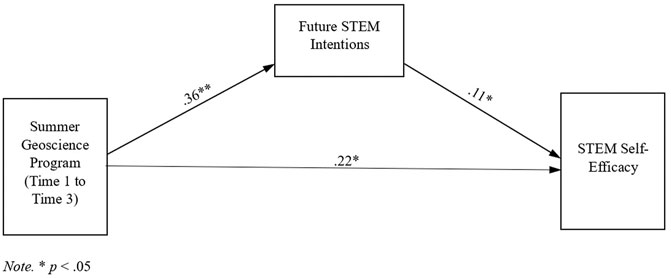
FIGURE 3. Statistical model depicting effects of the summer geoscience program on STEM self-efficacy, mediated by future STEM intentions.
Moderating Role of Program Evaluation in STEM Self-Efficacy and STEM Intentions Changes
Finally, we submitted program evaluation as the moderator, and STEM self-efficacy and STEM intentions (Times 1 and 3) as the repeated measures outcome variables in two separate models, using Montoya and Hayes’ (2017) MEMORE (macro Model 2). First, program evaluation significantly moderated changes in STEM self-efficacy from Time 1 to Time 3, b = 0.91, SE = 0.22, p < 0.001, 95% CI (0.47, 1.35). STEM self-efficacy increased from start to end of program among participants who reported high program quality, b = 1.01, SE = 0.22, p < 0.001, 95% CI (0.57, 1.45), but this was not the case among participants who reported low program quality, b = 0.20, SE = 0.19, p = 0.29. Although the omnibus model did not exhibit a statistically significant interaction on STEM intentions, b = 0.17, SE = 0.19, p = 0.35, 95% CI (−0.55, 0.20), the conditional effect was significant–STEM intentions strengthened from start to end of program among participants who reported high program quality, b = 0.46, SE = 0.19, p < 0.05, 95% CI (0.07, 0.89), but this was not the case among participants who reported low program quality, b = 0.29, SE = 0.22, p = 0.18.
Discussion
Over the course of the 4-week science program, URG high school student participants’ STEM self-efficacy and future STEM intentions increased from the program’s start to its culmination. Furthermore, program evaluations moderated these effects–increases in STEM self-efficacy and STEM intentions emerged only among participants who reported high program quality. These findings suggest that only those who were satisfied with their participation in the active learning environment benefitted psychologically, which is consistent with past educational climate research (Tinto, 1993; Allen and Robbins, 2008). Finally, changes in self-efficacy mediated increases in future intentions to pursue STEM and vice versa. These findings shed light on the interconnected relation between STEM self-efficacy and the desire to pursue STEM goals - they seem to continuously reinforce each other over time.
Study 2
Study 2 sought to examine the role of an isolated active learning component–mentoring–in URG community college students’ STEM self-efficacy and future STEM intentions. Because mentors provide vicarious learning experiences and encouragement through verbal social persuasion, mentor-mentee relationships offer the potential to boost confidence in one’s abilities, motivation, and academic goals. More than half of URG students who received a STEM degree from a 4-year university transfer from community colleges (National Science Foundation, 2012). Transferring from a community college to a 4-year university poses many obstacles, particularly ineffective advising and lack of information regarding the policies and expectations of 4-year universities (Packard, Gagnon, LaBelle, Jeffers, and Lynn, 2011). Mentoring programs are one effective method for promoting transfer rates from community colleges to 4-year degree programs, because mentors ease anxiety and increase confidence (Townsend and Wilson, 2006). Study 2’s semester-long mentoring program paired community college students interested in majoring in STEM (mentees) with undergraduate students majoring in STEM at a 4-year university.
Method
Participants and Design
The study adopted a one-factor two-level (Time: 1/beginning of program, 2/end of program) within-participants repeated-measures design. All URG students participating in the semester-long peer mentoring program in the spring 2019 (N = 77) and spring 2020 (N = 59) enrolled in the study. Due to attrition, the total final sample size of those who completed all measures at both time points was N = 87. Similar to Study 1, G Power indicates that a repeated measures MANCOVA with one group and two time-points yields a sample size of 92 to detect a small to medium effect size at 95% power. Table 1 lists all participants’ demographics. Participants received a small stipend for completing the mentoring program and participation in this study was a requirement of the mentoring program, but the informed consent notified them that their participation was voluntary and that they could withdraw at any time. We obtained informed consent from all participants. This research was approved by the Rutgers University Institutional Review Board and was part of a larger preregistered study (see As Predicted, https://aspredicted.org/q77vd.pdf, #38543).
Program and Procedure
The mentorship program occurred over the course of one semester (Smart and Gates, 2018). Similar to Study 1, the mentors were recruited from GSLSAMP and selected to mirror the demographics of the mentees such that they were mostly Black and Latinx students pursuing 4-year STEM degrees who transferred from a community college. Mentees and mentors were expected to communicate for at least 30 min per week through emails, phone calls, video calls, or text messages. The mentors were trained prior to the program on how to be a role model, friend, guide, coach, and advocate for community college students. A program administrator (none of the authors served this role) provided initial guidance to address topics such as challenges and important lessons for college, mentoring and networking experiences, tips to prepare and apply to 4-year programs, and management of course schedule. Importantly, mentees were trained prior to the start of the intervention on how to actively communicate, problem solve, and set goals with their mentor. Mentees were instructed to take an active role in the relationship and to work jointly with mentors to address academic challenges.
Mentees completed the below measured variables online through Inquisit Web 5.0 (Millisecond software, 2018) at two time points, at the beginning and the end of the program. All students received an email from the research team that included information and instructions about the study. The email included a link to the study, a unique participant ID, and a date by which the study should be completed. The research team monitored the completion rate of the study and sent reminder emails when necessary. Participants were instructed to complete each time point in one session without interruptions. Participants first provided their online consent and then completed the measures of STEM self-efficacy and STEM intentions, and the demographics questionnaire, all in this order.
Measured Variables
STEM Self-Efficacy
We administered the same measure from Study 1, except that the items referred to “STEM” rather than “science” abilities and talents (Times 1–2 rs = 0.80, 0.88).
STEM Intentions
Adapted from research by Dasgupta and colleagues (Stout et al., 2011; Dasgupta et al., 2015), participants responded to three items that assessed their intentions and aspirations to pursue STEM in the future—1) “At this time, how likely are you to transfer to a 4-years college or university in the future?” 2) “At this time, how likely are you to pursue a bachelor’s degree in the future?” and 3) “At this time, how likely are you to pursue a STEM degree at a 4-years college or university in the future?”—on 7-point scales ranging from 0 (not at all likely) to 6 (very likely). Higher scores indicate stronger STEM intentions (Times 1-2 αs = 0.66, 83).
Demographics
We administered the same measure from Study 1, in addition to items requesting information about participants’ annual family income, employment, and marital status.
Results
Table 3 reports the descriptive statistics for all measured variables as a function of Time and the zero-order correlations among these variables. In the analyses below, we sought to understand the role of the intervention over time in STEM self-efficacy and future STEM intentions above and beyond any explained variance of students’ SES. Students from higher SES backgrounds may start the program with stronger STEM self-efficacy and future intentions because of their access to greater academic and extracurricular resources.
Changes in STEM Self-Efficacy and Future STEM Intentions
To test the same two main hypotheses in Study 1, we ran a repeated measures MANCOVA in which Time was the repeated measures two-level factor (Times 1–2), with the covariates discussed above included. Table 4 lists means and standard errors of the outcome variables as a function of Time. The multivariate effect of Time was not significant (F(2, 81) = 0.22, p = 0.803, ηp2 = 0.005)—that is, student participants’ STEM self-efficacy and future intentions to pursue STEM remained stable over time.
Discussion
In retrospect, Study 2’s data are consistent with past STEM intervention research indicating that URG students do not consistently show changes in cognitive, motivational, and attitudinal changes throughout college (Dennehy and Dasgupta, 2017; Estrada et al., 2019). First, students who enter college in pursuit of STEM typically demonstrate high STEM self-efficacy, but often experience a decrease in self-efficacy when they are exposed to the rigors of STEM coursework and expectations (Liu, 2018; Kuchynka et al., 2019). It appears that self-efficacy drops are explained in part by the anxiety and self-doubt experienced during the transition from high school to college (Rosenthal et al., 2011), and the stress experienced when transferring from a 2-years to 4-years institutions (Laanan, 2001). Moreover, URG students may be at an increased risk of fluctuating self-efficacy during college, because they are more likely to experience academic isolation (Malone and Barbino, 2009; Grossman and Porche, 2014), bias (Swim, Hyers, Cohen, Fitzgerald, and Bylsma, 2003; Rankin and Reason, 2005), and a lack of support and recognition (Carlone, and Johnson, 2007).
It should be noted, however, that the community college student participants reported relatively strong STEM self-efficacy and future intentions to pursue STEM at the beginning of the program, and that the strength of these psychological constructs were maintained through the end of the program (from Table 4, means were 4.65 and 4.71, and 5.75 and 5.75, both on 0 to 6 scales, respectively). The future STEM intention scores indicate that the students who joined the program already intended to transfer to a 4-year university. Thus, these participants represent students committed to STEM goals as measured by their strong STEM intentions from the start to completion of the program even though these students are going through a potentially stressful transition period. Mentorship during this time may have helped to buffer URG students’ STEM self-efficacy and intentions to pursue STEM in the future.
General Discussion
Two longitudinal studies with URG students examined the role of two STEM interventions with active learning components in increasing STEM self-efficacy and intentions to pursue STEM in the future. In Study 1, high school URG students exhibited stronger STEM self-efficacy and stronger future STEM intentions over a 4-week geoscience program, and these two constructs were mutually related. In Study 2, community college URG students showed stable STEM self-efficacy and future STEM intentions across a semester-long mentorship program.
High School
High school represents the first time most students are exposed to advanced STEM content and given the opportunity to select or “opt-out” of a STEM pathway by avoiding advanced STEM classes. To facilitate a positive STEM self-concept and approach orientation toward STEM, high school students need low-stakes STEM exposure, where they can actively explore the material under the guidance of peers, mentors, and teachers without the pressure of testing, picking a major or a career (Kuchynka, Gates, and Rivera, 2020). Accordingly, Study 1 immersed high school URG students in a collaborative community of like-minded peers as well as mentors and teachers, who guided them through various active learning exercises including hands-on applications, group projects, and field trips. This out-of-school intervention provided students with opportunities to repeatedly experience three sources of self-efficacy - task mastery, vicarious learning experiences, social persuasion - in an inclusive environment for 4 weeks under the guidance of mentors and teachers. Students witnessed their peers and undergraduate student mentors engage with hands-on material (vicarious learning experiences), and received immediate feedback including encouragement and validation (social persuasion) as they worked independently and collaboratively on STEM tasks (task mastery) in a low stress environment without the pressures of testing. In sum, this engaging and inclusive environment played a positive role in STEM self-efficacy and intentions to pursue STEM in the future STEM.
Consistent with past research, the mutual relation between self-efficacy and future intentions observed in Study 1 suggests that these constructs are self-reinforcing (Nauta, Epperson, and Kahn, 1998; Nauta, Kahn, Angell, and Cantarelli, 2002; Zeldin, Britner, and Pajares, 2008; Byars-Winston et al., 2010). Before someone commits to pursuing a career goal, they must first believe in their ability to achieve success (Bandura, 1991; Bandura, Barbaranelli, Caprara, and Pastorelli, 2001), which, in turn, motivates them to pursue and achieve short-term and long-term goals related to that career (Pajares, 1996). Our data suggest that the development of future career intentions and self-efficacy are intertwined and continuously reinforce each other across time, enhancing approach motivation toward relevant tasks. Highly self-efficacious STEM students might be more likely to actively seek support from teachers or professors by attending office hours, as well as seeking out research opportunities in which they also collaborate with more advanced STEM students (Pajares, 1996; Pajares and Schunk, 2001). Moreover, they might join clubs or study groups that provide opportunities for extending their network of STEM peers, trainees, and researchers. Under the wrong conditions, students can be caught in a negatively reinforcing cycle, such that encountering one or more negative experiences with STEM promote early feelings of avoidance and self-doubt that reduce the likelihood of reengaging with STEM material. However, providing high school students with positive and satisfying educational climates to learn STEM material can foster an early approach orientation that promotes reengagement with STEM material over time.
Notably, only high school student participants who reported high program quality yielded the expected psychological gains, highlighting the importance of being satisfied with one’s academic environment. It appears that greater exposure to STEM material does not automatically result in enhanced self-efficacy over time if the learning environment does not fulfill students’ perceived needs for competence, autonomy, and belonging (Liu et al., 2014). This is doubly important for URG students because they are more likely to attend schools with inadequate resources (Duncombe and Cassidy, 2016), encounter cultural stereotypes that diminish STEM competence (Dasgupta, 2011), and be exposed to STEM educational climates harmful to the development of self-efficacy (Betz and Schifano, 2000).
Community College
Study 2 found that self-efficacy and STEM intentions remained stable across the semester-long mentoring program. The brevity of 2-year degree programs at community colleges presents a unique life transition, such that (traditional) students are simultaneously learning new norms and expectations, while preparing to transition to a 4-year university with its own set of norms and expectations (Terenzini, et al., 1994). Most students participating in Study 2 recently transitioned to community college from high school, and they are already planning their transition to a 4-year institution. Transitioning from high school to community college and community college to a 4-year institution is characterized by stress and self-doubt about “fitting in” and whether one will be successful, especially among first-generation college students (Terenzini, et al., 1994). Guidance from mentors can ease transitions, because they are trustworthy confidants and they teach mentees how to handle unforeseen challenges while providing emotional support and validation. Mentorship can maintain students’ confidence in their abilities and goals during stressful transition periods.
Study 2’s community college students were trained at the start of the program to take an active role in the mentee-mentor relationship. Mentees were taught what questions to ask, how to communicate with their mentor, and how to work jointly to solve their academic problems. In other words, the mentees were taught active learning strategies at the start of the intervention to maximize its benefits. Past research has demonstrated that mentorship improves community college students’ self-efficacy over time because mentors teach mentees strategies to regulate physiological arousal, provide a social support system, and offer positive validation (social persuasion) of skills and future success (Amelink, Artis, and King Liu, 2015; Terenzini, et al., 1994). Mentors also serve as a role model for mentees to emulate behaviors and align goals (Morgenroth, Ryan, and Peters, 2015). More specifically, mentees observe (vicarious experiences) mentors pursue a 4-year STEM degree, which teaches the mentees about the norms and expectations of 4-year STEM programs. Lastly, because mentors are typically selected to mirror the demographics of the mentees, mentors represent an inclusive exemplar, which counters cultural notions about who belongs and succeeds in STEM (Dasgupta, 2011; Dennehy and Dasgupta, 2017).
Instead of exclusively focusing on how to increase self-efficacy and future intentions, researchers should also address research questions about how to protect these psychological constructs during critical periods of development. Past research highlights the instability of self-efficacy during transition periods and college (Estrada et al., 2019; Kuchynka et al., 2019; Liu, 2018; Rosenthal et al., 2011). The community college students participating in Study 2’s mentorship program sought to transfer to a 4-year university, and started the intervention with relatively strong intentions to pursue STEM and high self-efficacy levels. Even though these students had set goals and felt confident about their STEM abilities, they needed continued guidance and validation from mentors to persist in reaching their academic and career goals.
Implications
Together with research showing that self-efficacy is linked to STEM persistence and performance across all educational phases (Collins and Bissell, 2004; Estrada, Woodcock, Hernandez, and Schultz, 2011; Amelink, Artis, and King Liu, 2015; Lent et al., 2016), our data have implications for closing performance and persistence gaps between URG and non-URG students. Ethnic-racial group differences in STEM participation are observed starting in high school with URG students taking less advanced STEM courses (Tyson, Lee, and Borman, 2007). Low STEM engagement during high school can be effectively ameliorated by immersing students in inclusive and collaborative communities, while providing students with repeated opportunities to learn varied STEM content that applies STEM concepts to real-world applications (Bozick et al., 2014; Plasman et al., 2017; Sublett, and Plasman, 2017). Active learning environments that encourage students to construct their own understanding of STEM material via hands-on exercises under the guidance of mentors and teachers promote self-efficacy and the pursuit of STEM goals. The relative brevity of Study 1’s summer science program (4 weeks) demonstrates the ease of developing self-efficacy among URG high school students if placed in the right educational climate.
Limitations
Study 1 and Study 2 did not include comparison groups, so we cannot account for possible self-selection (i.e., students who decided to participate in these programs may be more self-motivated than the average high school or community college students) or longitudinal time effects (i.e., the sheer passage of time can influence participants’ psychological constructs). To address this limitation, Study 1 tested if program quality moderated increases in STEM self-efficacy and future intentions; because program quality was a significant moderator, increases in self-efficacy and STEM intentions can be attributed to participation in the STEM program. Study 2 did not include a parallel measure of program quality, so we were unable to consider longitudinal time effects. Future studies should experimentally control for the possibility of selection effects by randomly assigning URG students to either a STEM intervention or a control group. Another future direction is to isolate specific aspects of active-learning programs that yield the most benefits for students.
Conclusion
Research spanning the past 3 decades repeatedly identify the following barriers for URG students in STEM: academic isolation (Malone and Barbino, 2009; Grossman and Porche, 2014), bias (Swim, Hyers, Cohen, Fitzgerald, and Bylsma, 2003; Rankin and Reason, 2005; Brown et al., 2016; Kuchynka et al., 2018), lack of mentorship (Pfund, Byars-Winston et al., 2015), lack of role models (Dasgupta, 2011, 2014), and a general lack of support (Swarat et al., 2004). All of these findings coalesce to suggest that URG students need immersion in welcoming educational environments that fulfill their needs for belongingness and validate their abilities and goals. Active learning environments work to satisfy belongingness, autonomy, and competence needs, which are all required for adaptive and healthy academic motivation (Gagné and Deci, 2005). Consistent with this, our research demonstrates that STEM self-efficacy and the pursuit of future STEM goals are dependent on providing a supportive and inclusive educational environment.
Data Availability Statement
The datasets presented in this study can be found in online repositories. The names of the repository/repositories and accession number(s) can be found below: SK and LR (2021, January 26). Geoscience Summer Intervention. Retrieved from osf. io/a63m5.
Ethics Statement
The studies involving human participants were reviewed and approved by Institutional Review Board, Rutgers University, Newark. Written informed consent to participate in this study was provided by the participants’ legal guardian/next of kin.
Author Contributions
SK wrote the manuscript and analyzed the data with the support of LR. LR conceptualized the studies with the help of SK and TR. TR helped with programming the studies, collecting the data, and preliminary analyses, with the support of LR. AG and LR are the principal and co-principal investigators, respectively, on the NSF GSLSAMP grant.
Funding
Support for the development of the programs in this research was provided by the National Science Foundation (grant OEDG-0703673 for Study 1’s high school program and grants HRD-1400780 and HRD-1909824 for Study 2’s community college program).
Conflict of Interest
The authors declare that the research was conducted in the absence of any commercial or financial relationships that could be construed as a potential conflict of interest.
Publisher’s Note
All claims expressed in this article are solely those of the authors and do not necessarily represent those of their affiliated organizations, or those of the publisher, the editors and the reviewers. Any product that may be evaluated in this article, or claim that may be made by its manufacturer, is not guaranteed or endorsed by the publisher.
Acknowledgments
The authors thank Drs. Cristiane San Miguel and Thomas Van Aken for organizing and administering Study 2’s mentorship program.
Footnotes
1The third author (Gates) was one of the teachers, but he did not participate in the design and administration of, and was blind to students’ performance on, the measured variables
References
Ahmad, T. (2019). Scenario Based Approach to Re-imagining Future of Higher Education Which Prepares Students for the Future of Work. Heswbl 10 (1), 217–238. doi:10.1108/heswbl-12-2018-0136
Alkhasawneh, R., and Hargraves, R. H. (2014). Developing a Hybrid Model to Predict Student First Year Retention in STEM Disciplines Using Machine Learning Techniques. J. STEM Educ. Innov. Res. 15 (3), 35.
Allen, J., and Robbins, S. B. (2008). Prediction of College Major Persistence Based on Vocational Interests, Academic Preparation, and First-Year Academic Performance. Res. High Educ. 49 (1), 62–79. doi:10.1007/s11162-007-9064-5
Amelink, C. T., Artis, S., and King Liu, T.-J. (2015). Examining the Self-Efficacy of Community College STEM Majors: Factors Related to Four-Year Degree Attainment. Community Coll. J. Res. Pract. 39 (12), 1111–1124. doi:10.1080/10668926.2014.941514
Aulck, L., Aras, R., Li, L., L'Heureux, C., Lu, P., and West, J. (2017). “STEM-ming the Tide: Predicting STEM Attrition Using Student Transcript Data,” in Proceedings of Machine Learning for Education (ml4ed) Workshop at ACM Knowledge Discovery and Data Mining Conference. Halifax, Nova Scotia: arXiv preprint arXiv:1708.09344.
Ballen, C. J., Wieman, C., Salehi, S., Searle, J. B., and Zamudio, K. R. (2017). Enhancing Diversity in Undergraduate Science: Self-Efficacy Drives Performance Gains with Active Learning. CBE Life Sci. Educ. 16 (4), ar56. doi:10.1187/cbe.16-12-0344
Bandura, A., Barbaranelli, C., Caprara, G. V., and Pastorelli, C. (2001). Self-efficacy Beliefs as Shapers of Children's Aspirations and Career Trajectories. Child. Dev. 72 (1), 187–206. doi:10.1111/1467-8624.00273
Bandura, A., and Locke, E. A. (2003). Negative Self-Efficacy and Goal Effects Revisited. J. Appl. Psychol. 88 (1), 87–99. doi:10.1037/0021-9010.88.1.87
Bandura, A. (1977). Self-efficacy: toward a Unifying Theory of Behavioral Change. Psychol. Rev. 84 (2), 191–215. doi:10.1037//0033-295x.84.2.191
Bandura, A. (1982). Self-efficacy Mechanism in Human agency. Am. Psychol. 37 (2), 122–147. doi:10.1037/0003-066x.37.2.122
Bandura, A. (1991). Social Cognitive Theory of Self-Regulation. Organizational Behav. Hum. Decis. Process. 50 (2), 248–287. doi:10.1016/0749-5978(91)90022-l
Bandura, A. (1978). The Self System in Reciprocal Determinism. Am. Psychol. 33 (4), 344–358. doi:10.1037/0003-066x.33.4.344
Bell, B. S., and Kozlowski, S. W. (2008). Active Learning: Effects of Core Training Design Elements on Self-Regulatory Processes, Learning, and Adaptability. J. Appl. Psychol. 93 (2), 296–316. doi:10.1037/0021-9010.93.2.296
Betz, N. E., and Schifano, R. S. (2000). Evaluation of an Intervention to Increase Realistic Self-Efficacy and Interests in College Women. J. Vocational Behav. 56 (1), 35–52. doi:10.1006/jvbe.1999.1690
Blanchard, R. D., Bunker, J. B., and Wachs, M. (1977). Distinguishing Aging, Period and Cohort Effects in Longitudinal Studies of Elderly Populations. Socio-Economic Plann. Sci. 11 (3), 137–146. doi:10.1016/0038-0121(77)90032-5
Bozick, R., Srinivasan, S., and Gottfried, M. (2014). Beyond Academic Math: The Role of Applied STEM Course Taking in High School. Teach. Coll. Rec. 116 (7), 1–35.
Brown, B. A., Henderson, J. B., Gray, S., Donovan, B., Sullivan, S., Patterson, A., et al. (2016). From Description to Explanation: An Empirical Exploration of the African-American Pipeline Problem in STEM. J. Res. Sci. Teach. 53 (1), 146–177. doi:10.1002/tea.21249
Byars-Winston, A., Estrada, Y., Howard, C., Davis, D., and Zalapa, J. (2010). Influence of Social Cognitive and Ethnic Variables on Academic Goals of Underrepresented Students in Science and Engineering: A Multiple-Groups Analysis. J. Couns. Psychol. 57 (2), 205–218. doi:10.1037/a0018608
Byars-Winston, A. M., Branchaw, J., Pfund, C., Leverett, P., and Newton, J. (2015). Culturally Diverse Undergraduate Researchers' Academic Outcomes and Perceptions of Their Research Mentoring Relationships. Int. J. Sci. Educ. 37 (15), 2533–2554. doi:10.1080/09500693.2015.1085133
Carlone, H. B., and Johnson, A. (2007). Understanding the Science Experiences of Successful Women of Color: Science Identity as an Analytic Lens. J. Res. Sci. Teach. 44 (8), 1187–1218. doi:10.1002/tea.20237
Chang, M. J., Sharkness, J., Hurtado, S., and Newman, C. B. (2014). What Matters in College for Retaining Aspiring Scientists and Engineers from Underrepresented Racial Groups. J. Res. Sci. Teach. 51 (5), 555–580. doi:10.1002/tea.21146
Chen, X., and Soldner, M. (2013). STEM Attrition: College Students’ Paths into and Out of STEM fields. Washington, DC: IES National Center for Education Studies, U.S Department of Education.
Cheryan, S., Master, A., and Meltzoff, A. N. (2015). Cultural Stereotypes as Gatekeepers: Increasing Girls' Interest in Computer Science and Engineering by Diversifying Stereotypes. Front. Psychol. 6, 49. doi:10.3389/fpsyg.2015.00049
Cleveland, L. M., Olimpo, J. T., and DeChenne-Peters, S. E. (2017). Investigating the Relationship between Instructors' Use of Active-Learning Strategies and Students' Conceptual Understanding and Affective Changes in Introductory Biology: A Comparison of Two Active-Learning Environments. Lse 16 (2), ar19. doi:10.1187/cbe.16-06-0181
Collins, S. J., and Bissell, K. L. (2004). Confidence and Competence Among Community College Students: Self-Efficacy and Performance in Grammar. Community Coll. J. Res. Pract. 28 (8), 663–675. doi:10.1080/10668920390254663
Dasgupta, N. (2011). Ingroup Experts and Peers as Social Vaccines Who Inoculate the Self-Concept: The Stereotype Inoculation Model. Psychol. Inq. 22 (4), 231–246. doi:10.1080/1047840x.2011.607313
Dasgupta, N., Scircle, M. M., and Hunsinger, M. (2015). Female Peers in Small Work Groups Enhance Women's Motivation, Verbal Participation, and Career Aspirations in Engineering. PNAS. 112 (16), 4988–4993.
Dennehy, T. C., and Dasgupta, N. (2017). Female Peer Mentors Early in College Increase Women's Positive Academic Experiences and Retention in Engineering. Proc. Natl. Acad. Sci. U S A. 114 (23), 5964–5969. doi:10.1073/pnas.1613117114
Dou, R., Brewe, E., Potvin, G., Zwolak, J. P., and Hazari, Z. (2018). Understanding the Development of Interest and Self-Efficacy in Active-Learning Undergraduate Physics Courses. Int. J. Sci. Edu. 40 (13), 1587–1605. doi:10.1080/09500693.2018.1488088
Duncombe, C., and Cassidy, M. (2016). Increasingly Separate but Unequal Schools in U.S. And Virginia Schools. Richmond, VA: The Commonwealth Institute, 1–3.
Eaton, A. A., Saunders, J. F., Jacobson, R. K., and West, K. (2020). How Gender and Race Stereotypes Impact the Advancement of Scholars in STEM: Professors’ Biased Evaluations of Physics and Biology post-doctoral Candidates. Sex Roles 82 (3-4), 127–141. doi:10.1007/s11199-019-01052-w
Estrada, M., Woodcock, A., Hernandez, P. R., and Schultz, P. W. (2011). Toward a Model of Social Influence that Explains Minority Student Integration into the Scientific Community. J. Educ. Psychol. 103 (1), 206–222. doi:10.1037/a0020743
Estrada, M., Eppig, A., Flores, L., and Matsui, J. (2019). A Longitudinal Study of the Biology Scholars Program: Maintaining Student Integration and Intention to Persist in Science Career Pathways. Understanding Interventions 10 (1), 1–26.
Fogliati, V. J., and Bussey, K. (2013). Stereotype Threat Reduces Motivation to Improve. Psychol. Women Q. 37 (3), 310–324. doi:10.1177/0361684313480045
Freeman, S., Eddy, S. L., McDonough, M., Smith, M. K., Okoroafor, N., Jordt, H., et al. (2014). Active Learning Increases Student Performance in Science, Engineering, and Mathematics. Proc. Natl. Acad. Sci. U S A. 111 (23), 8410–8415. doi:10.1073/pnas.1319030111
Gagné, M., and Deci, E. L. (2005). Self Determination Theory and Work Motivation. J. Organ. Behav. 26 (4), 331–362.
Gard, D. R., Paton, V., and Gosselin, K. (2012). Student Perceptions of Factors Contributing to Community-College-To-university Transfer success. Community Coll. J. Res. Pract. 36 (11), 833–848. doi:10.1080/10668920903182666
Gates, A. E. (2019). The Protégé Effect in the Retention of Underrepresented Minority Undergraduate Teaching Assistants in Geoscience: Preliminary Indications from Newark, New Jersey. J. Geosci. Educ. 67 (4), 417–426.
Grossman, J. M., and Porche, M. V. (2014). Perceived Gender and Racial/ethnic Barriers to STEM success. Urban Edu. 49 (6), 698–727. doi:10.1177/0042085913481364
Haak, D. C., HilleRisLambers, J., Pitre, E., and Freeman, S. (2011). Increased Structure and Active Learning Reduce the Achievement Gap in Introductory Biology. Science. 332 (6034), 1213–1216.
Hammer, D. (1994). Epistemological Beliefs in Introductory Physics. Cogn. Instruction 12 (2), 151–183. doi:10.1207/s1532690xci1202_4
Hesketh, B. (1997). Dilemmas in Training for Transfer and Retention. Appl. Psychol. 46 (4), 317–339. doi:10.1111/j.1464-0597.1997.tb01234.x
Iran-Nejad, A. (1990). Active and Dynamic Self-Regulation of Learning Processes. Rev. Educ. Res. 60 (4), 573–602. doi:10.3102/00346543060004573
Ishiyama, J. (2013). Frequently Used Active Learning Techniques and Their Impact: A Critical Review of Existing Journal Literature in the United States. Eur. Polit. Sci. 12 (1), 116–126. doi:10.1057/eps.2012.3
Ivancic, K., and Hesketh, B. (2011). Making the Best of Errors During Training. Int. J. Train. Res. 1, 103–125.
Komarraju, M., and Nadler, D. (2013). Self-efficacy and Academic Achievement: Why Do Implicit Beliefs, Goals, and Effort Regulation Matter? Learn. individual differences 25, 67–72. doi:10.1016/j.lindif.2013.01.005
Kuchynka, S., Findley-Van Nostrand, D., and Pollenz, R. S. (2019). Evaluating Psychosocial Mechanisms Underlying STEM Persistence in Undergraduates: Scalability and Longitudinal Analysis of Three Cohorts from a Six-Day Pre-college Engagement STEM Academy Program. CBE Life Sci. Educ. 18 (3), ar41. doi:10.1187/cbe.19-01-0028
Kuchynka, S., Gates, A., and Rivera, L. (2020). Identity Development during STEM Integration for Underrepresented Minority Students (Elements in Applied Social Psychology). Cambridge: Cambridge University Press.
Kuchynka, S. L., Salomon, K., Bosson, J. K., El-Hout, M., Kiebel, E., Cooperman, C., et al. (2018). Hostile and Benevolent Sexism and College Women's STEM Outcomes. Psychol. Women Q. 42 (1), 72–87. doi:10.1177/0361684317741889
Kudish, P., Shores, R., McClung, A., Smulyan, L., Vallen, E. A., and Siwicki, K. K. (2016). Active Learning outside the Classroom: Implementation and Outcomes of Peer-Led Team-Learning Workshops in Introductory Biology. CBE Life Sci. Educ. 15 (3), ar31. doi:10.1187/cbe.16-01-0051
Laanan, F. S. (2001). “Transfer Student Adjustment,” in Transfer Students: Trends and Issues. No. 114. New Directions for Community Colleges. Editor F. S. Laanan (San Francisco, CA: Jossey-Bass). doi:10.1002/cc.16
Larose, S., Ratelle, C. F., Guay, F., Senécal, C., and Harvey, M. (2006). Trajectories of Science Self-Efficacy Beliefs during the College Transition and Academic and Vocational Adjustment in Science and Technology Programs. Educ. Res. Eval. 12 (4), 373–393. doi:10.1080/13803610600765836
Lee, O., and Buxton, C. A. (2010). Diversity and Equity in Science Education: Research, Policy, and Practice. Multicultural Education Series. New York, NY: Teachers College Press.
Lee, O., and Luykx, A. (2006). Science Education and Student Diversity: Synthesis and Research Agenda. Cambridge: Cambridge University Press.
Lent, R. W., Miller, M. J., Smith, P. E., Watford, B. A., Lim, R. H., and Hui, K. (2016). Social Cognitive Predictors of Academic Persistence and Performance in Engineering: Applicability across Gender and Race/ethnicity. J. Vocational Behav. 94, 79–88. doi:10.1016/j.jvb.2016.02.012
Liu, S. (2018). Entering the STEM Pipeline: Exploring the Impacts of a Summer Bridge Program on Students' Readiness. J. Coll. Student Dev. 59 (5), 635–640. doi:10.1353/csd.2018.0060
Liu, W. C., Wang, C. K. J., Kee, Y. H., Koh, C., Lim, B. S. C., and Chua, L. (2014). College Students' Motivation and Learning Strategies Profiles and Academic Achievement: a Self-Determination Theory Approach. Educ. Psychol. 34 (3), 338–353. doi:10.1080/01443410.2013.785067
MacPhee, D., Farro, S., and Canetto, S. S. (2013). Academic Self-Efficacy and Performance of Underrepresented STEM Majors: Gender, Ethnic, and Social Class Patterns. Analyses Soc. Issues Public Pol. 13 (1), 347–369. doi:10.1111/asap.12033
Malone, K. R., and Barabino, G. (2009). Narrations of Race in STEM Research Settings: Identity Formation and its Discontents. Sci. Ed. 93 (3), 485–510. doi:10.1002/sce.20307
Maltese, A. V., and Tai, R. H. (2011). Pipeline Persistence: Examining the Association of Educational Experiences with Earned Degrees in STEM Among U.S. Students. Sci. Ed. 95 (5), 877–907. doi:10.1002/sce.20441
McNeil, L. M. (2000). Contradictions of Reform: Educational Costs of Standardized Testing. New York: Routledge.
Mendez, G., Buskirk, T. D., Lohr, S., and Haag, S. (2008). Factors Associated with Persistence in Science and Engineering Majors: An Exploratory Study Using Classification Trees and Random Forests. J. Eng. Edu. 97 (1), 57–70. doi:10.1002/j.2168-9830.2008.tb00954.x
Miller, D. I., Eagly, A. H., and Linn, M. C. (2015). Women's Representation in Science Predicts National Gender-Science Stereotypes: Evidence from 66 Nations. J. Educ. Psychol. 107 (3), 631–644. doi:10.1037/edu0000005
Montoya, A. K., and Hayes, A. F. (2017). Two-condition Within-Participant Statistical Mediation Analysis: A Path-Analytic Framework. Psychol. Methods 22 (1), 6–27. doi:10.1037/met0000086
Morgenroth, T., Ryan, M. K., and Peters, K. (2015). The Motivational Theory of Role Modeling: How Role Models Influence Role Aspirants' Goals. Rev. Gen. Psychol. 19 (4), 465–483. doi:10.1037/gpr0000059
Nauta, M. M., Epperson, D. L., and Kahn, J. H. (1998). A Multiple-Groups Analysis of Predictors of Higher Level Career Aspirations Among Women in Mathematics, Science, and Engineering Majors. J. Couns. Psychol. 45 (4), 483–496. doi:10.1037/0022-0167.45.4.483
National Research Council and National Academy of Engineering (2012). Community Colleges in the Evolving STEM Education Landscape: Summary of a Summit. Washington, DC: National Academies Press
Nauta, M. M., Kahn, J. H., Angell, J. W., and Cantarelli, E. A. (2002). Identifying the Antecedent in the Relation between Career Interests and Self-Efficacy: Is it One, the Other, or Both? J. Couns. Psychol. 49 (3), 290–301. doi:10.1037/0022-0167.49.3.290
Otero, V. K., and Gray, K. E. (2008). Attitudinal Gains across Multiple Universities Using the Physics and Everyday Thinking Curriculum. Phys. Rev. Spec. Topics-Physics Edu. Res. 4 (2), 020104. doi:10.1103/physrevstper.4.020104
Packard, B. W. L., Gagnon, J. L., LaBelle, O., Jeffers, K., and Lynn, E. (2011). Women's Experiences in the STEM Community College Transfer Pathway. J. Women Minorities Sci. Eng. 17 (2), 120–147. doi:10.1615/jwomenminorscieneng.2011002470
Pajares, F. (2005). Gender Differences in Mathematics Self-Efficacy Beliefs. Cambridge University Press.
Pajares, F., and Schunk, D. H. (2001). Self-beliefs and School success: Self-Efficacy, Self-Concept, and School Achievement. Perception 11 (2), 239–266.
Pajares, F. (1996). Self-efficacy Beliefs in Academic Settings. Rev. Educ. Res. 66 (4), 543–578. doi:10.3102/00346543066004543
Plasman, J. S., Gottfried, M., and Sublett, C. (2017). Are there Academic CTE Cluster Pipelines? Linking High School CTE Coursetaking and Postsecondary Credentials. CTE 42 (3), 219–242.
Rankin, S. R., and Reason, R. D. (2005). Differing Perceptions: How Students of Color and white Students Perceive Campus Climate for Underrepresented Groups. J. Coll. Student Dev. 46 (1), 43–61. doi:10.1353/csd.2005.0008
Richard, O. C. (2000). Racial Diversity, Business Strategy, and Firm Performance: A Resource-Based View. Amj 43 (2), 164–177. doi:10.5465/1556374
Riegle-Crumb, C., King, B., and Irizarry, Y. (2019). Does STEM Stand Out? Examining Racial/ethnic Gaps in Persistence across Postsecondary fields. Educ. Res. 48 (3), 133–144. doi:10.3102/0013189x19831006
Rittmayer, A. D., and Beier, M. E. (2008). Overview: Self-Efficacy in STEM. SWE-AWE CASEE Overviews, 1, 12.Salomon, GTelevision Is "easy" and Print Is "tough": The Differential Investment of Mental Effort in Learning as a Function of Perceptions and Attributions. J. Educ. Psychol. 76 (4), 647–658.
Rosenthal, L., London, B., Levy, S. R., and Lobel, M. (2011). The roles of Perceived Identity Compatibility and Social Support for Women in a Single-Sex STEM Program at a Co-educational University. Sex Roles 65 (9–10), 725–736.
Schroeder, C. M., Scott, T. P., Tolson, H., Huang, T.-Y., and Lee, Y.-H. (2007). A Meta-Analysis of National Research: Effects of Teaching Strategies on Student Achievement in Science in the United States. J. Res. Sci. Teach. 44 (10), 1436–1460. doi:10.1002/tea.20212
Schunk, D. H. (1989). Self-efficacy and Achievement Behaviors. Educ. Psychol. Rev. 1, 173–208. doi:10.1007/bf01320134
Seymour, E., and Hewitt, N. M. (1997). Talking about Leaving: Why Undergraduates Leave the Sciences. Boulder, CO: Westview.
Shaw, E. J., and Barbuti, S. (2010). Patterns of Persistence in Intended College Major with a Focus on STEM Majors. NACADA J. 30 (2), 19–34. doi:10.12930/0271-9517-30.2.19
Smart, D., and Gates, A. E. (2018). “Improving the Transfer Rate from 2-year to 4-year College through an Innovative Cross Campus Peer Mentoring Program,” in Proceedings of the 2018 American Educational Research Association Annual Conference (IEEE).
Smith, E. M., Ford, J. K., and Kozlowski, S. W. (1997). Building Adaptive Expertise: Implications for Training Design Strategies. Springer.
Spencer, S. J., Logel, C., and Davies, P. G. (2016). Stereotype Threat. Annu. Rev. Psychol. 67, 415–437. doi:10.1146/annurev-psych-073115-103235
Steele, C. M. (1997). A Threat in the Air. How Stereotypes Shape Intellectual Identity and Performance. Am. Psychol. 52 (6), 613–629. doi:10.1037//0003-066x.52.6.613
Stefanou, C. R., and Salisbury‐Glennon, J. D. (2002). Developing Motivation and Cognitive Learning Strategies through an Undergraduate Learning Community. Learn. Environments Res. 5 (1), 77–97. doi:10.1023/a:1015610606945
Stout, J. G., Dasgupta, N., Hunsinger, M., and McManus, M. A. (2011). STEMing the Tide: Using Ingroup Experts to Inoculate Women's Self-Concept in Science, Technology, Engineering, and Mathematics (STEM). J. Pers Soc. Psychol. 100 (2), 255–270. doi:10.1037/a0021385
Sublett, C., and Plasman, J. S. (2017). How Does Applied STEM Coursework Relate to Mathematics and Science Self-Efficacy Among High School Students? Evidence from a National Sample. J. Career Tech. Edu. 32 (1), 29–50.
Swarat, S., Drane, D., Smith, H. D., Light, G., and Pinto, L. (2004). Opening the Gateway. J. Coll. Sci. Teach. 34 (1), 18.
Swim, J. K., Hyers, L. L., Cohen, L. L., Fitzgerald, D. C., and Bylsma, W. H. (2003). African American College Students' Experiences with Everyday Racism: Characteristics of and Responses to These Incidents. J. Black Psychol. 29 (1), 38–67. doi:10.1177/0095798402239228
Syed, M., Azmitia, M., and Cooper, C. R. (2011). Identity and Academic success Among Underrepresented Ethnic Minorities: An Interdisciplinary Review and Integration. J. Soc. Issues 67 (3), 442–468. doi:10.1111/j.1540-4560.2011.01709.x
Terenzini, P. T., and Pascarella, E. T. (1980). Toward the Validation of Tinto's Model of College Student Attrition: A Review of Recent Studies. Res. High Educ. 12 (3), 271–282. doi:10.1007/bf00976097
Terenzini, P. T., Rendon, L. I., Lee Upcraft, M., Millar, S. B., Allison, K. W., Gregg, P. L., et al. (1994). The Transition to College: Diverse Students, Diverse Stories. Res. High Educ. 35 (1), 57–73. doi:10.1007/bf02496662
Thapa, A., Cohen, J., Higgins-D'Alessandro, A., and Guffey, S. (2012). School Climate Research Summary: August 2012. School Climate Brief, Number 3. New York, NY: National School Climate Center.
Theobald, E. J., Hill, M. J., Tran, E., Agrawal, S., Arroyo, E. N., Behling, S., et al. (2020). Active Learning narrows Achievement Gaps for Underrepresented Students in Undergraduate Science, Technology, Engineering, and Math. Proc. Natl. Acad. Sci. U S A. 117 (12), 6476–6483. doi:10.1073/pnas.1916903117
Townsend, B. K., and Wilson, K. (2006). "A Hand Hold for A Little Bit": Factors Facilitating the Success of Community College Transfer Students to a Large Research University. J. Coll. Student Dev. 47 (4), 439–456. doi:10.1353/csd.2006.0052
Treisman, U. (1992). Studying Students Studying Calculus: A Look at the Lives of Minority Mathematics Students in College. Coll. Math. J. 23 (5), 362–372. doi:10.1080/07468342.1992.11973486
Tyson, W., Lee, R., Borman, K. M., and Hanson, M. A. (2007). Science, Technology, Engineering, and Mathematics (STEM) Pathways: High School Science and Math Coursework and Postsecondary Degree Attainment. J. Edu. Students Placed Risk (Jespar) 12 (3), 243–270. doi:10.1080/10824660701601266
Vogt, C. M. (2008). Faculty as a Critical Juncture in Student Retention and Performance in Engineering Programs. J. Eng. Edu. 97 (1), 27–36. doi:10.1002/j.2168-9830.2008.tb00951.x
Wang, X. (2013). Why Students Choose STEM Majors. Am. Educ. Res. J. 50 (5), 1081–1121. doi:10.3102/0002831213488622
Wise, K. C. (1996). Strategies for Teaching Science: What Works? The Clearing House: A J. Educ. Strateg. Issues Ideas 69, 337–338. doi:10.1080/00098655.1996.10114334
Zeldin, A. L., Britner, S. L., and Pajares, F. (2008). A Comparative Study of the Self-Efficacy Beliefs of Successful Men and Women in Mathematics, Science, and Technology Careers. J. Res. Sci. Teach. 45 (9), 1036–1058. doi:10.1002/tea.20195
Keywords: STEM interventions, minorities, mentorship, educational climate, STEM education, high school, community college (Min5-Max 8)
Citation: Kuchynka S, Reifsteck TV, Gates AE and Rivera LM (2021) Developing Self-Efficacy and Behavioral Intentions Among Underrepresented Students in STEM: The Role of Active Learning. Front. Educ. 6:668239. doi: 10.3389/feduc.2021.668239
Received: 15 February 2021; Accepted: 08 November 2021;
Published: 09 December 2021.
Edited by:
Gary Wong, The University of Hong Kong, Hong Kong, SAR ChinaReviewed by:
Julio Ciro Benegas, National University of San Luis, ArgentinaShan Jiang, Chongqing University of Education, China
Copyright © 2021 Kuchynka, Reifsteck, Gates and Rivera. This is an open-access article distributed under the terms of the Creative Commons Attribution License (CC BY). The use, distribution or reproduction in other forums is permitted, provided the original author(s) and the copyright owner(s) are credited and that the original publication in this journal is cited, in accordance with accepted academic practice. No use, distribution or reproduction is permitted which does not comply with these terms.
*Correspondence: Sophie Kuchynka, c2syMjI0QHBzeWNob2xvZ3kucnV0Z2Vycy5lZHU=; Luis M. Rivera, bHVpc0Bwc3ljaG9sb2d5LnJ1dGdlcnMuZWR1