- 1Department of Medical Bioscience, University of the Western Cape, Bellville, South Africa
- 2Department of Mathematics and Applied Mathematics, University of the Western Cape, Bellville, South Africa
Introduction: There is much debate regarding the impact of COVID-induced lockdown on the standard of assessments, mainly since students were assessed at home via an online assessment platform. Regular orthodox lectures and assessments were carried out during the first term, while the strictly enforced South African COVID lockdown warranted that 2nd term lectures and assessments were based online. This created the fortunate control conditions to statistically compare orthodox face-to-face with online-home-based assessments.
Methods: We compared the assessments of a cohort of second-year students studying physiology and anatomy during 2019 and 2020: Orthodox face-to-face teaching and assessments (2019) were compared to online teaching and their analogous online assessments (2020) during the COVID-19-induced lockdown.
Results: Although class pass rates and marks for online assessments (2020) were significantly higher than for traditional assessments (2019), an essential finding of the study was that the Gaussian distribution of the marks across the class for both modes of assessment was statistically identical. This indicated that although students performed better with home-based online assessments, poor-performing students populated the lower spread of marks, modal students occupied the central distribution, while good students occupied the higher mark distribution of the curve.
Discussion: The students were found to be resilient in adapting to things and learning, online presentations, and computer-based assessments. No gender-based difference or advantage to adjusting to newly introduced blended learning and concomitant changes in learning assessment strategies was found. The online-home-based assessments proved to incentivize prior learning and preparation for assessments by implementing strict time limits or assessments and randomizing the selections of questions and respective (MCQ) answer choices. We conclude that although home-based online assessments significantly improve the overall mark distribution, there was no distinction in the spread of the distribution of marks, which was indicative that the home-based online assessment process was able to provide an identical measure of course proficiency as in the orthodox sit-down assessment. Therefore, our statistical analysis of the performance of students under student assessment performances indicates that there is no rationale for thinking that the home-based online mode of assessment is equivalent to or better than the orthodox modes of assessment.
Introduction
The COVID-19 pandemic has induced landmark changes to the way society, business, and education have normally operated (Donthu and Gustafsson, 2020). In a world under the constant threat of virus infections with high mortality, our mode of social interaction has altered dramatically. The tertiary landscape is not spared from the onslaught of the COVID-19 pandemic (Aristovnik et al., 2020; Hedding et al., 2020). Universities across the world closed their doors to students, and during the most severe infection trajectories, also to the research project, as labs were closed and postgraduate students were sent home (Marinoni et al., 2020; Motala and Menon, 2020).
COVID-19 has ushered in and accelerated the dawn of 4IR on the back-bone of high-speed internet connectivity (Mhlanga and Moloi, 2020), where offices and university classrooms have primarily become obsolete and rapidly replaced by home-based offices and classrooms (Mishra et al., 2020). This meant computer skills, technological infrastructure, learning resources, student and staff communication, assessments, and new (blended) modes of learning and teaching had become necessities (see, for example, the Complex Adaptive Blended Learning System (CABLS) framework suggested by Wang et al., 2015, and the recent article Jumaa et al., 2022).
In response, the tertiary sector rapidly mobilized staff to teach remotely, using the efficiency of virtual conferencing platforms (Microsoft Teams, iKamva, Zoom, Google Meet, Skype, Mentimeter, LT platform for physiology, etc.) while using established paper-based testing (PBT) moving to computer-based testing (CBT) to assess students. Rapanta et al. (2020) found that there is a need to design learning activities with specific social, cognitive, and facilitator characteristics, as well as the need to adapt the assessment to new learning requirements. Blended learning impacts the role and relationship of instructors and students and, consequently, learning assessment strategies. The COVID-19 pandemic, despite its myriad of setbacks, offers opportunities to modernize anatomy education approaches (Khasawneh, 2021). Although cadaver and laboratory education was disrupted (Özen et al., 2022), this challenge has highlighted the demand for blended learning and teaching environments that consist of some online offerings and some face-to-face classes. In Egypt (Mahdy and Sayed, 2021) early, two-thirds of veterinary anatomy students felt enthusiastic about online mode during the lockdown. Numerous studies (Mahdy and Sayed, 2021; Mahdy and Ewaida, 2022; Zarcone and Saverino, 2022) also recommend innovative measures, three-dimensional virtual tools such as Visual Body, and electronic devices such as Leica Acquire, to mitigate common problems associated with distance learning. Lima et al. (2020) proposed both synchronous and asynchronous activities using various online tools for essential topics in human physiology.
We attribute the overall performance of a cohort of second-year physiology and anatomy students in the second-year Medical Bioscience module MBS231 at our tertiary institution to this; i.e., students and teaching staff seem to benefit from online access to relevant course material. In addition, one could speculate that quality-assured learning environments have positive spin-offs over and above traditional face-to-face classes. In a study done by Paechter and Maier (2010) that involved 2,196 students from 29 Austrian universities, it was found that they appreciate online learning for its potential to provide a clear and coherent structure to learning material and that it supports self-regulated learning and distributing of information. They found that for understanding or establishing interpersonal relationships, students prefer face-to-face learning. Furthermore, students also appeared to prefer online learning for acquiring skills in self-regulated learning, but when it came to conceptual knowledge or learning skills to apply knowledge, students preferred face-to-face learning (Paechter and Maier, 2010). Online learning incentivizes students to become self-disciplined, study independently, engage in course materials independently, and prepare for exams, all of which provide a foundation for life-long learning.
Ideally, assessment security should remain uncompromised, so it is not surprising that e-proctoring has surged since the start of the COVID-19 pandemic (Flaherty, 2020). Mean scores and throughput rates remain broadly comparable across assessments, whether conducted with online proctoring at testing centers in the presence of proctors (García-Peñalvo et al., 2021). Institutions are adopting flexible multi-modal solutions to CBT, providing access to CBT via a network of secure centers or their own homes, with options such as live online proctoring and record-and-review proctoring.
The University provided students with laptops and data for network connection to allow students to access the online material. Many students suffer from unconducive living conditions away from campus. Besides implementing COVID-19 regulations, a new policy on flexible learning and teaching provisioning had to be developed. In addition, a new learning assessment policy was drafted, all designed to regulate the academic project and to maintain outcome proficiencies while maintaining academic standards.
The initial set-up cost of CBT is offset by savings on item or production costs (Boevé et al., 2015), and fortunately, for a large cohort of students such as MBS231, there is an extensive database of assessment content available that can be randomized and supplemented at low additional costs. Immediate assessment score reporting has a largely positive impact on students’ achievement emotions, too (Daniels and Gierl, 2017). A multi-modal CBT carries built-in flexibility that accommodates year-on-year changes in student cohorts, guaranteed safety, and instant adaptability to changes in COVID-19 protocols, and it has unlimited reach with less intrusion on test-takers time, as well as much greater accessibility than traditional assessment platforms. Such digital scoring saves time and resources (Seale, 2002).
This move from PBT to CBT is a means of providing greater access and less expediency, but there is still a need for understanding its impact, shortcomings, and ultimately how its design and delivery can improve, as was suggested by Guimarães (2017). This study on the impact of moving from face-to-face to digital assessment informs the validity and reliability of assessment scores in high-impact modules. We use statistical analysis to compare assessments of two cohorts of students (2019 to 2021) studying physiology and anatomy in a Medical Bioscience undergraduate program.
Theoretical framework
Developing an institutional online approach requires a skilful approach and full support to compensate for the face-to-face approach (Gregory and Lodge, 2015). Both systems favor the learner-centered approach philosophy: learners as active agents that bring knowledge, past experiences, education, ideas, and impactful new information to the classroom environment. A priority for a thriving online environment is that the instructor needs to transition into an effective online communicator, manage technology, and deliver and assess online content (Roddy et al., 2017). Monitoring students’ progress, engaging students via online platforms, doing follow-up consultations, and flipping the class is crucial for a vibrant online environment. Some instructors are new to blended environments and have to adapt rapidly to new teaching modalities. They now provide constant support via the online environment to monitor student progress, resolve learning queries, and provide access to collective competencies of the online learning experience.
Therefore, to support the blended learning approach, it is vital to consider Complex Adaptive Blended Learning System (CABLS) framework (Wang et al., 2015), illustrated in Figure 1. Within this framework, the student is located at the center of the model, with the relevant satellite components all impacting one another (Figure 1).
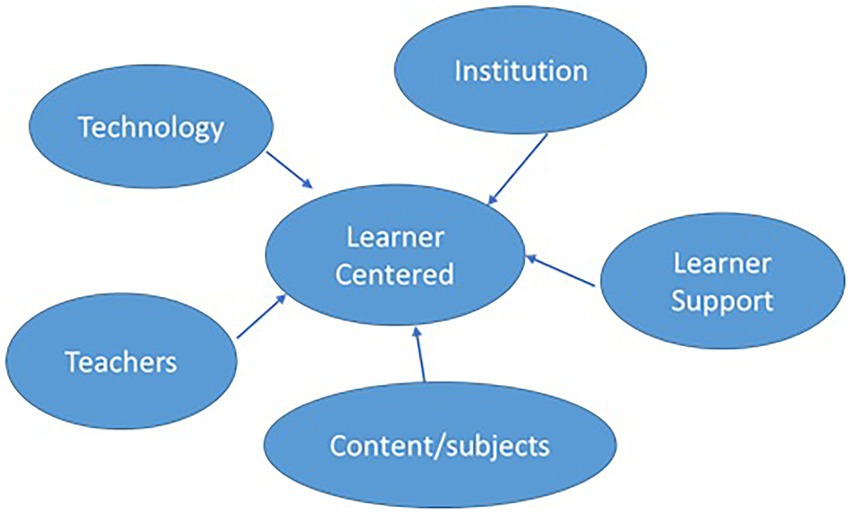
Figure 1. The conceptual framework of the Complex Adaptive Blended Learning System approach, which points to the learner-centered philosophy (Wang et al., 2015).
This philosophy is based on the learner being the central role-player, as illustrated in Figure 1. Thus, the blended learning strategy is geared toward supporting and training students for lifelong learning and identifying essential pedagogical needs of students pertaining to the 21st-century society. The role of the student is to adapt as they interact with system elements for the first time or in new ways.
As blended teaching and learning environments require significant investment and capital layout in technology (see Figure 1), equipment, and sophisticated networks to sustain the tertiary capacity to maintain academic standards and student performance, tertiary institutions could not rely on governments to bail them out. Fortunately, our institution has the iKamva platform, which is user-friendly to both staff and students and is integral for online learning, teaching, and CBT.
The transition from face-to-face to online learning technology played a crucial role for lecturers to provide much more effective communication between staff and students and students in the online learning environment. Communication modalities such as email, chat, live class questions, online assessment, and feedback provision have turned the MBS231 classroom environment from a passive into an enriching active one for students and lecturers. This is in line with what Wang et al. (2015) refer to as learner support.
The module MBS231 is a core module for the BSc Medical Bioscience degree. Online assessment has come under increasing scrutiny due to emerging perceptions of quality assurance and the ability to reflect on performance outcomes (Newman, 2015). There still needs to be more clarity between quality assurance indicators and the quality of online and blended learning approaches (Openo, 2017). The perception that online learning is not as effective requires an urgent response since it and blended learning approaches have become a critical 21st-century skill (López-Pérez et al., 2011; Vaughan, 2015; Conrad and Openo, 2018), so tracking student performance in MBS231 should be viewed in this light. In line with Wall, Hursh, and Bond (2020, p.6), assessment tasks are a “set of online activities that seek to gather systematic evidence to determine the worth and value of things in higher education.” Students’ submission of assessment tasks in MBS231 is the most telling indicator of the quality of educational outcomes (Gibbs, 2010, p. 7). It provides evidence of the learning outcomes for accountability and quality assurance (Conrad and Openo, 2018, p. 5).
The study aims to compare and analyzes the assessment data of second-year Physiology and Anatomy students (MBS231) between traditional face-to-face classroom lessons in 2019 to full online modes of learning and assessment, the latter enforced by the advent of the COVID-19 pandemic in March 2020. Presented with an opportunity to fully engage with online learning and teaching pedagogy, together with a full suite of online assessment modalities, we challenge the premise that there is a discernible distinction between assessment standards in the second-year Medical Bioscience module with home-based online assessment by comparing results to the traditional sit-down form of assessment. We describe an online learning environment that is much more transparent and responsive to students’ learning needs, and when conducted effectively, it incentivizes learning, thereby addressing quality assurance. Assessment strategies are strongly aligned with pedagogical practices in MBS231 through blended learning approaches catered for by in-person and online spaces for learning and teaching. This point is demonstrated in the methods of assessment described below.
Methods
Participants
Participants were second-year undergraduate students (N = 398) in the Department of Medical Biosciences (MBS) at the University of the Western Cape in South Africa. They were drawn from two cohorts of module MBS231 in the year 2019 (n = 197), which was a traditional assessment and 2020 (n = 201), which was an online assessment. Both cohorts were multidisciplinary and ethnically diverse. Assessment marks of the 2019 sit-down (SD) examination and the 2020 online examination are taken into account. Both cohorts consist of male and female students. The 2019 data set consisted of (n = 68) males and (n = 129) females. The 2020 data set consisted of (n = 58) males and (n = 143) females. Age ranges from 18 years to 30 years old. Data were analyzed using Graphpad Prism (version 5, San Diego, California, USA).
Sakai (iKamva) platform
The institutional e-learning content management system Sakai (iKamva) is built using responsive web design principles that allow users to access the platform from multiple devices (with internet connectivity) at any geographical location. Table 1, obtained from UWC CIECT (2020), reflects the total number of unique users logged in from mobile devices (not using the app), computers/laptops, and the iKamva Android application.
The UWC Faculty of Natural Sciences cooperates closely with CIECT in arranging blended learning approaches and plans for students and staff during the national lockdown in South Africa (UWC NSCI, 2021).
CIECT offers training to lecturers and students using iKamva to supplement traditional learning (face-to-face lecturing) and for online teaching and assessments. iKamva has various e-Tools used to deliver the program, such as announcements, course resources, tests, quizzes, assignments, discussion forums, and structured lesson tools. Sakai (iKamva Platform) has been ranked the top Open-LMS since 2019 to date.
Traditional assessment format
During 2019 both practical evaluations were conducted in the traditional sit-down manner, while in 2020, practical evaluations were both conducted as home-based online assessments due to the COVID-19 lockdown. Practicals were carried out as conventional teaching laboratory hands-on practicals in the first term of 2020, while practicals in the second term were based on narrated PowerPoint presentations augmented with mostly YouTube-based videos which replaced the laboratory hands-on component of the practicals. Practicals were then assessed via the home-based online assessment platform every second week, and these assessments formed the basis for the final practical evaluation mark of Term 2. In both practical evaluations 1 and 2, the distribution of the practical arks, and consequently throughput significantly improved for the online assessments in 2020 when compared to 2019.
Traditional assessments and examinations were based on face-to-face lessons. Module lecturers prepared a set of exam questions according to Bloom Taxonomy guidelines (Anderson et al., 2001). The question paper consisted of 13 marks multiple-choice, 12 marks for true/false, and 25 marks for short essay questions. A set percentage of questions were knowledge-based questions, and a smaller number of questions were based on an application, integration, and analytical basis. Sit-down assessments written under traditional assessment conditions were hosted in large examination venues. A question paper counted 50 marks within a 90-min time frame and was written on answer sheets collected for marking afterward.
A multiple-choice type question of no more than 5 answer choices carries a penalty of-0.25 for a wrong answer, with no reason or justification required for an answer. A True/False type question counts 1 mark with a penalty of-0.5 for a wrong answer (no reason or justification required for the answer). Only one test or exam submission was allowed. In the event of electrical load-shedding, or due to challenges involving a shortage of data and internet connectivity, the student was required to obtain an affidavit from the police station. A completely new assessment randomly generated from pools of questions would then be issued to the student. Question types (MCQ, T/F, short/long answer) were identical for traditional assessments in terms 1 of 2019 and 2020/2021. The assessment format was orthodox in Term 2 of 2019 (MCQ, T/F, and short/long answer) and online via iKamva in Term 2 of 2020/2021 (MCQ and T/F types only). Of the online iKamva assessments in Term 2 of 2020, 20% are difficult questions, while 40% are intermediate questions, and the remaining 40% are easy.
Home-based online assessments
The home-based online assessment started at the beginning of Term 2 in 2020/2021, as South Africa went into lockdown (Carlitz and Makhura, 2021) due to the COVID-19 pandemic. Although the 2020 cohort wrote their first assessments during Term 1 in an orthodox manner, and Term 2 was an online assessment. The continuous assessment (CE) mark counts 50% toward the final mark (FM), and the final exam counts 50%. The Jue 2020/2021 Final Examination papers, Paper 1 and Paper 2, were based on work covered in Term 1 and Term 2, respectively. Assessment standards were maintained and all assessments were moderated internally. Assessment questions covered all content completed in lectures and textbook-based coursework. Most questions were application and integration of knowledge and understanding, and a few were purely knowledge-based.
Pools of questions were designed using the iKamva assessment platform. Each assessment and examination contained 30 multiple-choice and 20 true/false questions randomly selected from a database of 200 multiple-choice questions and 200 true/false questions designed for each event. The iKamva platform randomizes answers per question, and it randomizes questions. A randomized set of questions is thus generated for each student to minimize peer-to-peer consultation and copying (Olt, 2002). Every assessment had a time limit that was strictly implemented, providing on average 1.5 min per question. This further incentivizes preparation, as searching for answers would take up an inordinate amount of time. Each answer counted one mark. An incorrect true/false answer accrued a-0.5 penalty and every incorrect MCQ answer accrued a-0.25 penalty.
Statistics
The data was analyzed using the software program GraphPad Prism (version 5, San Diego, California, USA). Box and whisker box plots were used to present the distribution of assessment marks (%) the box represents the lower and upper quartile, the center-line in the box represents the median (or quartile 2) and the whiskers represent the minimum and maximum values of the range of assessments.
Before statistically comparing two sets of data, a Kolmogorov–Smirnov test (Kolmogorov–Smirnov test in GraphPad Prism; Massey, 1951; Corder and Foreman, 2014: p. 26) was carried out to assess if the data were normally distributed (p > 0.05). If both sets of data were normally distributed then an unpaired t-Test (Graphpad Prism: unpaired t-Test; Ekstrøm and Sørensen, 2019: p. 153; Corder and Foreman, 2014: p. 57) was used to determine if the means were significantly different, otherwise for non-normally distributed data (p < 0.05), a Mann–Whitney statistical test was used (Graphpad Prism: the Mann–Whitney test; Corder and Foreman, 2014: p. 57). Differences were considered significant when p-value <0.05.
Results
Controls
Any study involving comparisons is only as good as the control set of data to which it will be compared. In this study, we compare 2019, 2020, and 2021 assessment data using a cohort of students registered in the course MBS231. In first comparing 2019 data with 2020, we ask two fundamental questions: are the data sets equivalent statistically and demographically, and if they are, how do the two modes of assessment (traditional versus online assessment) compare regarding the mark distribution. We then compared traditional assessments (2019) with selected home-based online assessment results between 2020 and 2021 to evaluate if the home-based assessments were different from the orthodox 2019 assessments, and if different, could statistical analysis provide insights into the differences. It must be pointed out that the essential difference between the 2019 and the 2020 cohorts of students, was that 2020 students were assessed during a very strict lockdown in South Africa, regulated by the South African armed forces which limited the movement of individuals to their homes. In contrast, in 2021, the lockdown was abandoned and individuals were free to liaise with each other, although a 10 pm national curfew was still enforced strictly.
The implicit assumption is that the control samples (student groups in 2019 and 2020) we are comparing are statistically similar. If they are statistically dissimilar it would nullify further comparison. We, therefore, interrogated these assumptions made before we compare the data from these two cohorts of students: firstly, the groups of students are second-year students, all familiar with academic processes, and have settled into the rigors of academic discipline, having overcome most of the challenges typically presented a student’s first academic year. Thus, we are presented with a more mature, homogenous group of students at the second-year level than at first-year levels, whereas educational, urban versus rural, financial, and cultural backgrounds play a crucial role in the variance of success of first-year students. The demographics of class size and the difference in the size of gender groups between the 2 years was less than 5% (see Table 1).
The demographics of our cohort of students for Physiology and Anatomy (MBS231) were also similar to the global demographics for veterinary Anatomy students surveyed during the COVID-19 pandemic (Mahdy and Ewaida, 2022). In this study, there were also more female students than male students and 80% of the student’s ages ranged between 18 and 25 years of age. Although the demographics between the two classes were very similar (see Table 1), we wanted to establish whether, under the same assessment conditions, the distribution of assessment marks between the two groups was indeed statistically equivalent. Establishing statistical normality between MBS231 student groups in 2019 and 2020 would be essential to compare traditional and home-based online assessment data between the two groups, in other words, should the statistical distribution of marks differ significantly in term test 1 (same assessment conditions for both 2019 and 2020), it would nullify further comparisons between the 2019 and 2020 groups of students.
In brief, both sets of students were exposed to traditional face-to-face (F2F) lectures, followed by an end-of term sit-down (SD) assessment. We statistically compared the 2019/2020 assessment data for the first theory tests using box-and-whisker plots and Gaussian distribution curves. The box-and-whisker plots in Figure 2A show the distribution between the two groups of students was not statistically different (p < 0.1). This is confirmed by Gaussian distribution curves superimposed on the overlaid histograms of both sets of data (p < 0.1). This endorsed the postulate that both sets of students were statistically identical from an assessment perspective. Thus, there was no reason to believe that these students would respond differently in other assessments of the module. We, therefore, established that the 2019 group of students was statistically similar to the 2020 cohort of MBS231 students by comparing their theory test 1 assessment data (p < 0.1). There was no difference in both tuition or assessment conditions for theory test 1 for these two groups of students, as COVID lockdowns in 2020 were introduced after this assessment. We, therefore, concluded that the 2019 assessment data would provide an acceptable set of control data to which we could compare the 2020 home base online assessment data.
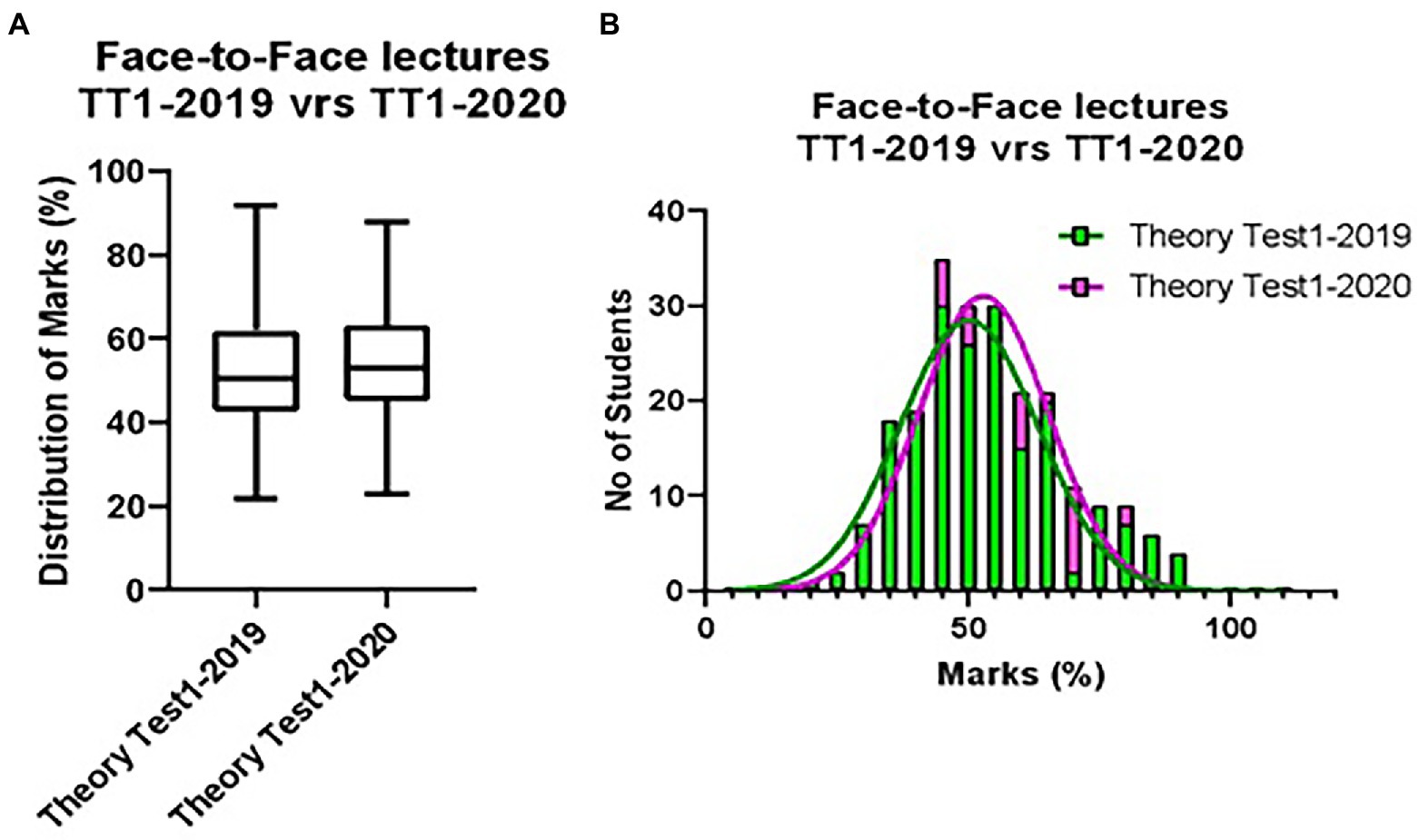
Figure 2. Graph A depicts the distribution of marks for theory test 1 (Term 1) for both 2019 and 2020. Both sets of students were presented with face-to-face lectures, and both groups were assessed using the traditional “sit-down” assessment format. Theory test 1 was not normally distributed, and therefore, the nonparametric Mann–Whitney statistical test (Graphpad Prism: the Mann–Whitney test; Corder and Foreman, 2014: p. 57) showed that there were no significant differences between the means of these sets of data (p < 0.1). Graph B presents the same sets of data using Gaussian distribution curves superimposed over the frequency distribution of the two sets of data. The data shows that both the 2019 and 2020 groups of student assessment data were equally distributed (p < 0.1). The abbreviation refers to the Theory Test.
Traditional assessments versus home-based online assessments
Given the control assessment data showed that the first theory test was statistically identical (p < 0.1; Figures 2A,B), we evaluated the impact of COVID-induced online teaching and online home-based assessments (in 2020) on the distribution of marks, and compare them to the analogous periods of traditional teaching and assessments in 2019.
Theory Test 1/2 (2019): In Figure 2A, we firstly compare Theory Test 1 (2019) to Theory Test 2 (2019). Both these assessments were carried out under the same traditional teaching and assessment procedures. Statistical analysis showed that Theory Test 2 presented a distribution of marks that was slightly, but significantly lower, than the first theory test (p < 0.0001).
Theory Test 1/2 (2020): In Figure 2B, Theory Test 1 (term 1: 2020) was carried out under orthodox teaching and assessment methods, while Theory Test 2 (term 2: 2020) was carried out under COVID-19 lockdown conditions, and thus all narrated lectures were given online and assessed using the iKamva home-based-online assessment platform. The distribution of marks in Theory Test 2 (2020) was significantly higher (p < 0.0001) than in the orthodox SD assessment in the first term.
In Figures 3C,D, we used superimposed Gaussian distribution curves over the histogram distribution of the data to confirm the statistical differences seen in Figures 3A,B (p < 0.0001).
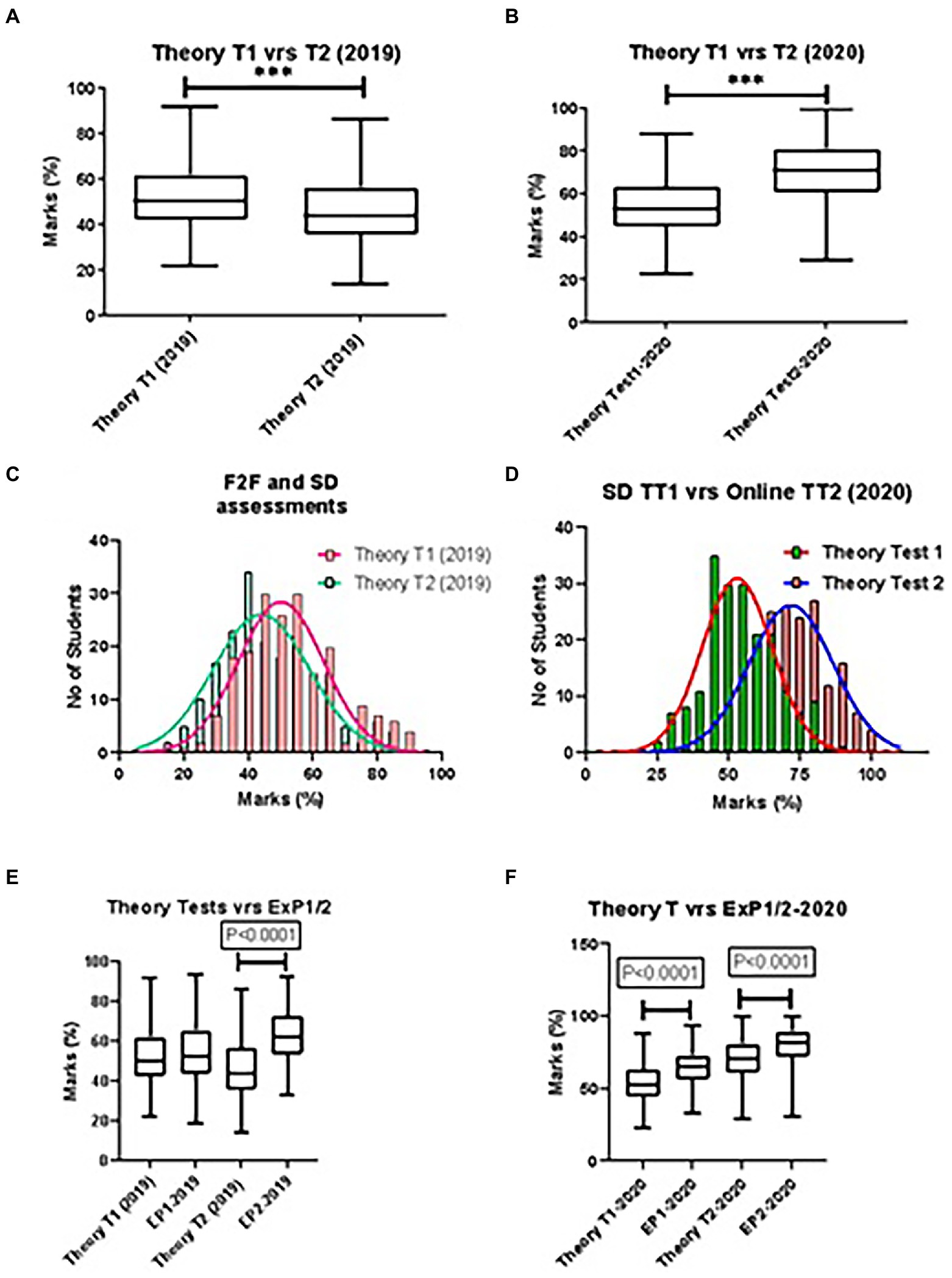
Figure 3. (A) The assessment data (2019) represents normal F2F lectures and orthodox sit-down (SD) assessments. Theory Test 2 had lower Quartile groups 2 and 3, including a slightly lower median; statistical analysis showed that these sets of data were statistically different from each other (p < 0.0001). (B) Here, we compare the analogous data sets in 2020, where Theory Test 1 was an SD assessment compared to an online home-based assessment. Statistically, the online assessment had a significantly higher distribution of marks (%; p < 0.0001). (C,D) Here we show the analogous sets of data (to A and B, respectively) using Gaussian distribution curves superimposed on frequency distributions of the two sets of data. (E,F) “B and W” plots are used to statistically compare Theory Test 1 (T1) with the analogous Exam Paper 1 (EP1) and Theory Test 2 (T2) with the analogous Exam Paper 2 (EP2). In 2019, there was no significant difference between Theory Test 1 and Exam Pape; however, the distribution of exam assessment percentages was significantly higher in Exam Paper 2 (EP2) compared to Theory Test (T2). In 2020, in both comparisons, the distribution shifted to the right in exam assessment percentages was significantly higher than in the term tests.
The theoretical work in term 1 is firstly examined during Theory Test 1, and then it was comprehensively assessed in Exam Paper 1. This is the same for term 2, where the coursework was firstly examined in Theory Test 2, and then comprehensively assessed in Exam Paper 2. The final exam mark (%) is the average of these two exam papers.
Theory Test 1/2 verses Exam Paper 1/2 (2019): The distribution of the marks (%) in Theory Test 1 was not significantly different from Exam Paper 1 (Figure 3E). However, although the distribution of the marks in Theory Test 2 was lower than in Theory Test 1, in the corresponding Exam Paper 2, the mark distribution was significantly higher (p < 0.0001).
Theory Test 1/2 verses Exam Paper 1/2 (2020): During 2020, only term one was carried out it traditionally with regard to teaching and assessments. From term 2, all lecturing was based online as well as assessments (this also took place during COVID-19 lockdown). The distribution of marks for both exam papers was significantly higher than for the theory tests (p < 0.0001; Figure 3F).
Distribution of theory test 2 assessment marks
We wanted to investigate whether students were adapting to the online assessments from 2020 to 2021. Gaussian curve analysis showed that the distribution of assessment marks for Theory Test 2 in 2020 and 2021 was not statistically different (Figure 4). It must be noted that these two successive cohorts of students were almost identical in terms of demographics (See Table 1). Given the statistical equivalence in the kurtosis of the mark distribution (p < 0.1), the difference in the heights of the curve peaks of the distributions is determined mostly by the variance in student numbers (see Table 1). However, the mean and mode of the 2020/21 distribution curves are statistically equivalent (p < 0.4).
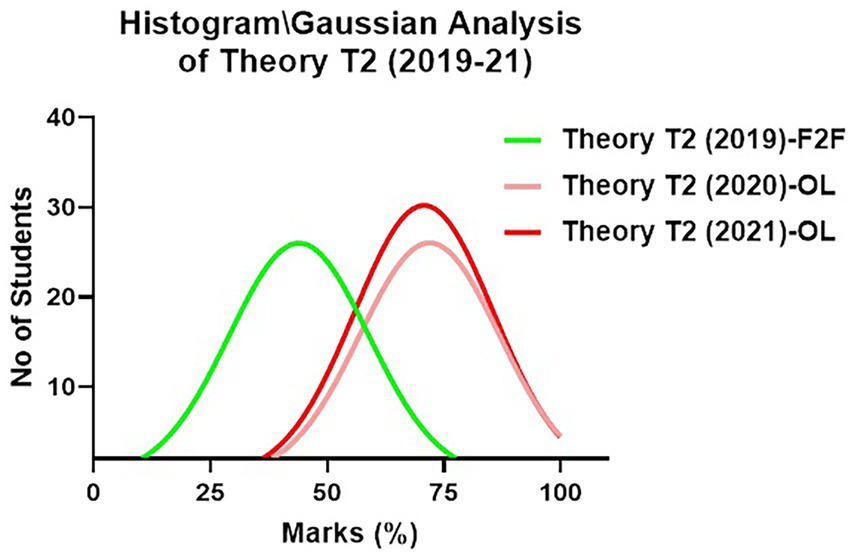
Figure 4. Gaussian statistical analysis shows that the distribution of marks for the online assessment of Theory Test 2 remained statistically equivalent (p < 0.2) for two different sets of students (2020 and, 2021). This indicated that in successive years (2020 and, 2021), standard, home-based online assessments were able to successfully grade different cohorts of MBS231 students according to their proficiency in the theoretical components of the course.
Throughput of MBS231
The throughput rate (TP%) of a module refers to the percentage of registered students that pass the module (obtaining 50% or more in an assessment). TP% is one of the most used performance indicators in Higher Education (Scott et al., 2007; Yeld, 2010; Bozalek and Boughey, 2012). The distribution of these data sets normally skews to the rightgiven that under traditional assessment conditions more students tend to pass than fail, where the mode/medians are normally between 55 and 75%.
During 2019 both practical evaluations were conducted in the traditional sit-down manner, while in 2020 practical evaluations were both conducted as home-based online assessments due to the COVID-lockdown. Practicals were carried out as conventional teaching laboratory hands-on practicals in the first term of 2020, while practicals in the second term were based on narrated PowerPoint presentations augmented with mostly YouTube-based videos which replaced the laboratory hands-on component of the practicals. Practicals were then assessed via the home-based online assessment platform every second week, and these assessments formed the basis for the final practical evaluation mark of Term 2. In both practical evaluations 1 and 2, the distribution of the practical marks, and consequently, TP significantly improved for the online assessments in 2020 (Figures 5B,D) when compared to 2019 (Figures 5A,C). The TP for Theory Test 1 and 2 for 2020 was significantly higher when comparing analogous TP in 2019, with the TP of 93% for Theory Test1 (traditional sit-down assessment) and 92% for Theory Test 2 (online lectures and online assessments), did not differ statistically. Gender differences?
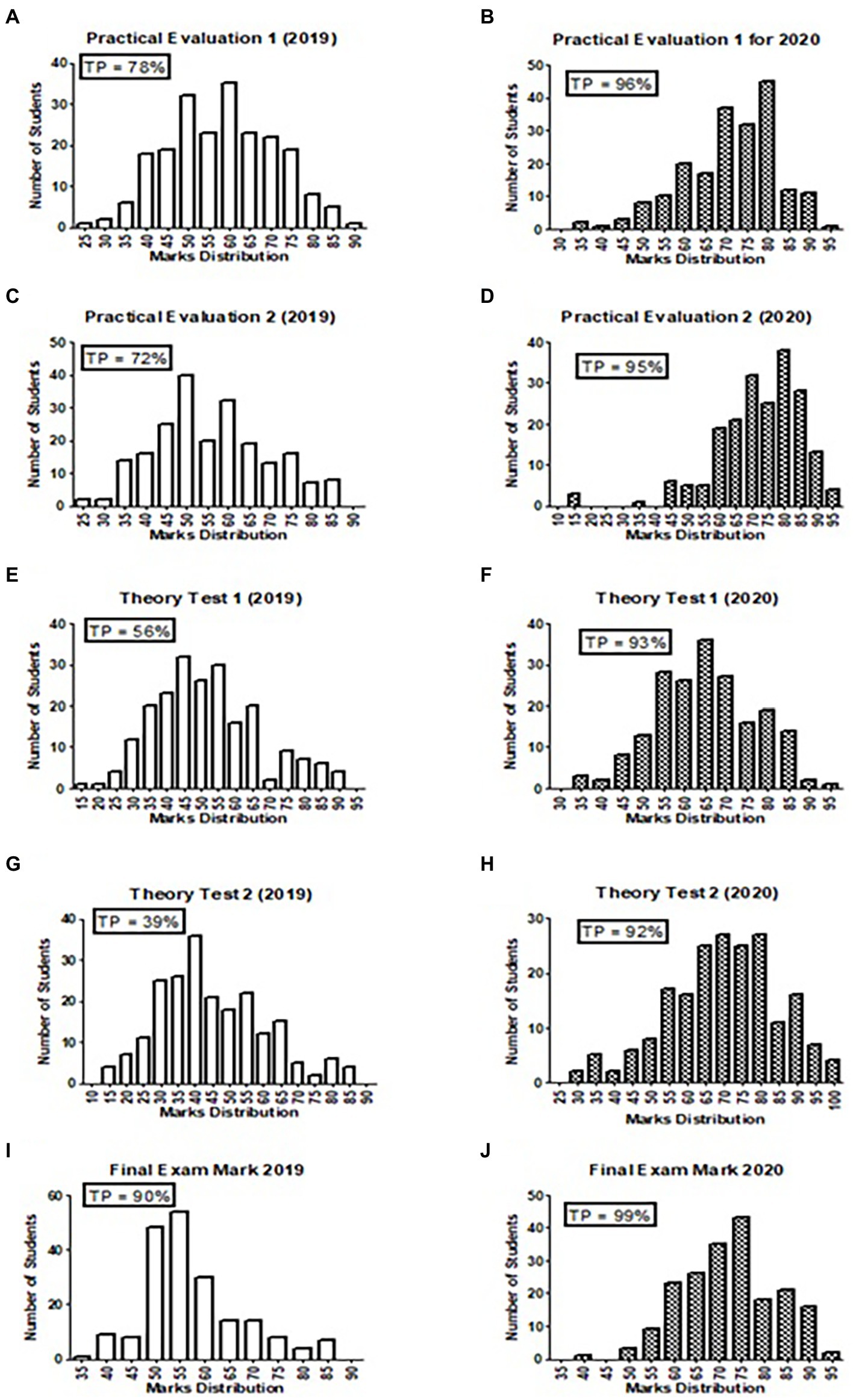
Figure 5. The following graphs depict the marks (%) distributions for all practical (A-D), theory (E-H), and exam assessments (I,J). Each assessment event depicts the percentage of students in the MBS231 course that achieved more than 50% (TP).
We wanted to investigate if there were any gender differences between the assessment distributions. This is an important consideration in light of studies showing that computer self-efficacy and anxiety do impact computer-based test performance, which was found to be more pronounced in female undergraduate students (Balogun and Olanrewaju, 2016). We found that both genders’ data subsets correlated well with the distribution for the combined assessments, viz., that if the total assessment improved or decreased, both male and female data subgroups contributed equally to that change (Figures 6A–F). We analyzed the assessment data to investigate whether male students adapted faster to the online experience compared to female students or vice versa. Based on the assessment distribution, we found no statistically significant differences in how the different gender groups adapted based on gender subgroups (Figures 6E,F).
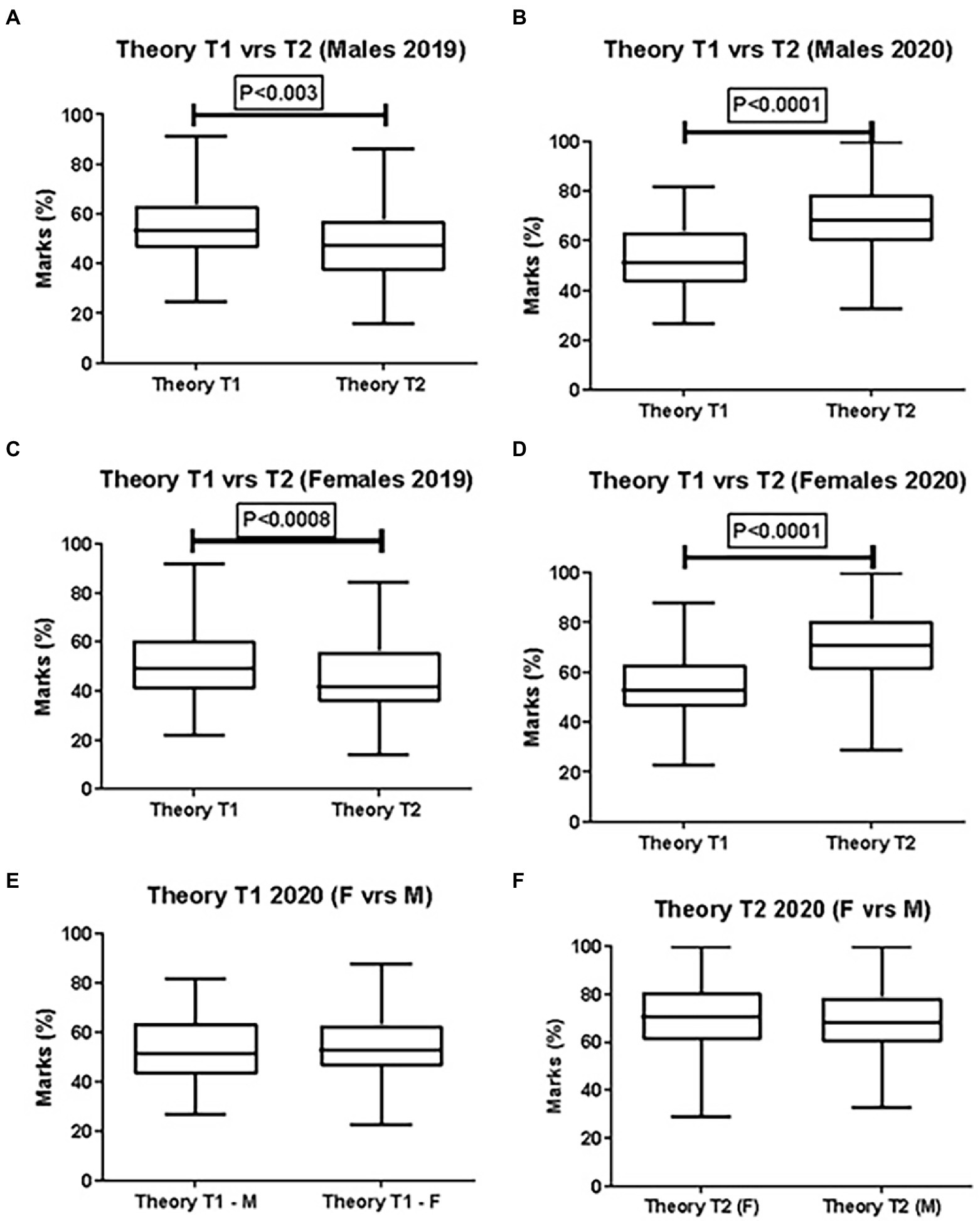
Figure 6. The graphs above present comparisons between the data gender subsets, and we compared the distribution of marks (%) for these subgroups of males (A,B) and females (C,D). The distribution comparison between males and females is presented in graphs E, and F. Theory Tests (T) for 2019 and Theory Test 1 in 2020 was assessed in the normal sit-down method, while Theory Test 2 was a home-based online assessment of online lectures (COVID-19 lockdown period).
Discussion
The choice of controls
There is a saying in experimental science “that any experiment is only as good as the control.” Fundamentally, this truism can be extended to any set of data that you would like to compare. We wanted to analyze assessment data sets in which traditional face-to-face (F2F) teaching in 2019 and compare it with online teaching and assessment data. Coincidentally, we wanted to assess the impact of the COVID-19 induced-lockdowns on assessments, using a group of students in the Life Sciences (studying Physiology and Anatomy).
To compare the data in 2019 with the data in 2020, we had to evaluate how closely the two sets of students performed in the first-term assessment under traditional teaching and assessment conditions. These two groups of students were both second-year students and had similar demographics relating to class size, gender distribution, and age (see Table 1). Second-year students are fairly computer literate and display proficiency in a wide range of skills required for online learning, acquired in their first year of study using the same assessment platform (iKamva). While there have been many reports of challenges in the second-year experience (Graunke and Sherry, 2005; Fisher et al., 2011; Conana et al., 2022), these students are better oriented to strive toward establishing identity, competence, deeper learning, and goal setting compared to first-year students. Thus, from a demographic background as well as a preparatory point of view, these two sets of students were virtually identical.
We wanted to evaluate, from a traditional assessment perspective, whether this similarity between the two groups of students would still exist. Our statistical analysis of the assessment data for 2019 and 2020 (Figure 2), showed that these two sets of data were not significantly different from each other (p < 0.1). The Gaussian distribution curves in Figure 2B further endorses this evaluation, showing symmetrical distribution between the two data sets. This statistical analysis strongly suggests that both sets of students, as per the evaluation of their assessment scores, are statistically identical. This would therefore provide a solid foundation or baseline to compare assessments between both orthodox and online teaching and assessment methods. Zarcone and Saverino (2022) assigned students from the same cohort in the same term randomly to either a computer-based or a paper-based test consisting of the same questions to measure test-mode effects and found that the group assigned the computer-based test outperformed the group who attempted the paper-based test. Although our design is different from Zarcone and Saverino (2022) in that during pre-COVID conditions, all assessments were evaluated using paper-based tests, while COVID-induced lockdowns precipitated the online assessments, the distribution of marks from online assessments were increased by an average of 16%.
The impact of online teaching and assessment
Given that both the 2019 and 2020 groups of students were statistically identical for Theory Test 1 (Term 1), we would expect that assessment data profiles should also be similar for Theory Test 2. Thus, differences between the statistical assessment profiles of 2019 and 2020 would be indicative of online teaching and assessment. We show that in 2019 there was a slight but significant decrease between the Theory Test 1 and Theory Test 2 assessments (Figures 3A,C; p < 0.0001). As the course content did not change and students were exposed to the same coursework, the assessment outcome in 2020 would be expected to be similar. However, we see that the assessment profile for Theory Test 2 (2020) significantly increased (Figures 3B,D; p < 0.0001). These observations are contrary to the findings of Prisacari et al. (2017) who found no significant difference in performance between paper-based and computer-based chemistry practice. This could potentially be a phenomenon similar to that observed by Omar et al. (2021), which revealed the effects of test score inflation in unproctored conditions.
An obvious question is what component of the increase in the assessment profile is due to the online teaching, and what component is due to the home-based online assessment? During the first term, the practical component of the course was taught using the traditional method. However, due to the COVID-lockdown, this component of the module was assessed using the home-based online assessment method. The 2019 assessment profile showed that Practical Evaluation 1 (PE1) was not statistically different from theory Test 1 TT1 (Figure 3E; p > 0.05). In 2020, PE1 was significantly increased compared to TT1 (Figure 3E). As practical coursework for both 2019 and 2020 was done using the identical traditional method the higher marks for the 2020 assessment suggest it was because of being a home-based online assessment and therefore may be weakly indicative of the differential impact of home-based online assessments. On the other hand, being assessed in an environment in which anxiety is decreased, is well known to improve assessment scores. We would also like to indicate that given the time constraints for each online MCQ question (<1 min), students who would choose to search Google/PDFs/PowerPoint presentations for the correct answers, would find that they have compromised their chances to complete the assessment in the allocated time, by inadvertently losing assessment marks in the process. This in itself would incentivize students to prepare for assessments.
However, analysis of the assessment between TT2 and PE2 suggests that to discriminate between the effects of online teaching and online assessment, more data samples are needed to be conclusive: In 2019 (term 2), students performed significantly better in their PE2 than in their TT2 (Figure 3E). This same trend was observed between PE2 and TT2 in 2020 (Figure 3F). This made it difficult to discriminate what proportion of the increase in the marks profile was due to online teaching and/or online assessment. Nevertheless, it was clear that the marks profile had substantially and statistically improved for all online components of the course as compared to the traditional program in 2019.
Interpretation of the Gaussian curves
A key feature of the Gaussian distribution curves was that the shape of the curves was not affected by online assessments (Figure 7). In fact, the curve was slightly flatter (not statistically significant), which indicated a broader distribution of the assessment data. This indicated very clearly that students that were at the lower end of the distribution curve (as per traditional assessment marks) remained at the lower end of the curve for home-based online assessments, and students that were above-average performers remained at the high end of the curve, with the majority of students centrally distributed on the bell-shaped curve. In other words, the distribution curve for online learning simply moved to the right. This further indicates that should the standard of the assessment been implicated, in other words, had the assessment had been made so easy, with most of the students easily passing the assessment, the shape of the curve would have changed distinctly by being skewed sharply to the right, and the shape of the curve would have also been very much steeper, all of which would indicate that any student in the class would have little trouble obtaining a high mark. The distribution curves of the home-base online assessments show that students were normally distributed (‘bell-shaped curve’), clearly separating weaker, moderate and good students in all online assessments according to their proficiency in the theory component of the course. This unexpected outcome is both novel and crucial to future comparative evaluations of traditional SD and home-based online assessments.
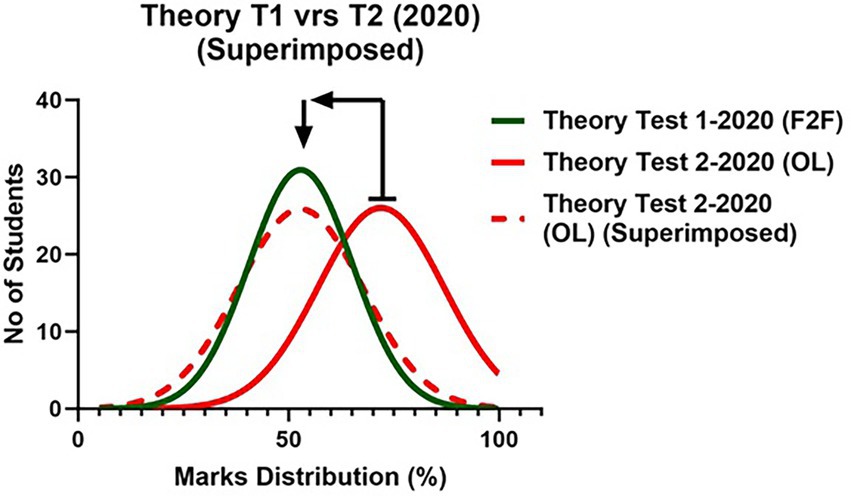
Figure 7. The statistical comparison shows that the shape (kurtosis) of the online marks distribution of Theory Test 2 was statistically not significantly different (p < 0.1) from the shape of distribution of marks in theory test 1 (Face-to Face): We superimposed the two sets of data: Theory Test 1 (F2F) and theory Test 2 (OL). F2F: orthodox assessment; OL: home-based online assessment.
We wanted to evaluate if the home-based online assessment trends during 2021 would be impacted by the evolving socio-political COVID pandemic environment, given that students could now freely move about, meet up at campus, access the library, etc., which was distinctly different to the 2020 socio-political lockdown environment. Furthermore, we wanted to evaluate whether students would adapt to the assessment methodology, by improving their marks, as would be demonstrated by a shift of the marks-distribution curve to the right, or a skewing of the distribution curve to the right. However, this was not the case, and it was surprising to see that the 2020/21 distribution curves were statistically identical (Figure 4). The significance of this is that our assessment process seems to be sustainable in terms of its accuracy in grading students according to their theoretical proficiency. This is borne out by the statistically equivalent distribution curves for 2020/21, that the spread (range) of marks (2020: 29%–100%; 2021: 31%–100%), graded students according to their theoretical proficiency. It infers that the procedural measures we have imposed on home-based online assessments, such as a limited time to answer MCQ questions, randomized questioning from large pools of question banks, and randomizing the answer choices for MCQ questions, provide a limited time for students to query MCQs via Google, PDF notes, video-based lectures, as these are time-consuming, and would eventuate in the student forfeiting completing the assessment, as the assessment automatically submits at the end of its allocated time. Although there are few recent studies that compared the distribution of student assessment marks before and during the COVID-pandemic, Saverino et al. (2021), used a similar cohort of students (anatomy) and compared their assessment distribution before and during the COVID pandemic (Saverino et al., 2021). Their assessment data supported our finding in also showing that the distribution of marks for online teaching and assessment improved relative to traditional teaching and assessments. Their distribution of online assessment data further endorsed our postulate that although assessment data for online assessment was improved relative to traditional assessments, the distribution of low to high marks remained very similar to the distribution of marks obtained during pre-pandemic traditional assessments.
The effects of online teaching/assessment on through-put (TP)
Administrators of tertiary institutions are fond of the variable TP, which indicates the percentage of the class which achieved 50% in assessments and therefore passed as opposed to that percentage of the class that failed. However, when used on its own it represents a very “blunt instrument” to analyze the assessment performance of a cohort of students. It says little about the distribution of data especially in terms of mode, median, and means, or the kurtosis of the distribution curve, etc. Nevertheless, it was interesting to consider TP relative to the effects of online assessed courses compared to traditional assessment methodologies (Omar et al., 2021). For a more informative discussion, we combined TP with distribution histograms to describe the distribution of marks for the class (see Figure 4). The TP range for orthodox teaching and the various assessments (2019: Figures 5A,C,E,G) ranged between 39% and 90% (for the Final Mark for the module), while in 2020 all TPs for online models ranged between 92% and 99% (Final Module TP Percentage). Also, the kurtosis of the distribution of 2019 Theory test marks was on average more negatively skewed (skewed to the left), while for the 2020 Theory Test online assessments the kurtosis of the distribution was bell-shaped and shifted to the right, indicative of the higher TP values for online offering and assessment. Two anomalies appear in this data, firstly, the TP for Theory Test 1 (2019) was much lower than Theory Test 1 (2020), although both were taught and assessed in the traditional manner (this improvement may be due to the introduction of an experienced professor to teach the neurology lectures in the first term). Secondly, although the continual evaluation (CE) components (Theory and Practical assessments) of the course in 2019 had much lower TPs than their associated CE assessments in 2020, the final TP for the module differed by 9% (2019: 90% versus 2020: 99%). Essentially, the number of students who had achieved proficiency within the module improved by 9% under COVID-19 lockdown conditions.
TP of CE assessment events are much more critical to academic planning compared to the TP of final marks (%) of a course module. We compared the final throughput rates between 2019 (90%) and 2020 (99%) and were surprised that final throughputs differed by only 9%. Although this difference was small, it was statistically significant and translated to 18 more students passing in 2020 (Figures 5I,J). Finally, in 2019 TP for traditional CE assessments (Figure 5A: 78%, 5C: 72%, 5E: 56%, and 5D: 39%) did not reflect the final exam TP of 90% (Figure 4I), but consistently underpredicted the final TP of the class. Whereas in 2020, TP consistently predicted pass rates of over 90% (Figures 5B: 96%, 5D: 95%, 5F: 93% and 5H: 92%), which endorsed the 99% final TP (Figure 5J). The variation and the magnitude of the TP of the various orthodox assessments during 2019 gave no predictive indication or extrapolation as to the final TP for the module. This is concerning, as low TP in pre-exam CA assessments is often used to flag modules for high failure and poor student performance. However, the TP across the CA online events were closely correlated to the final TP level of the module. Thus, TP from online assessment events appears to be a better proxy for predicting the final TP for the module.
Does gender provide an advantage in adapting to the digital teaching and assessment landscape?
Reports on the under-representation of the female gender in STEM fields have suggested that one gender may have an inherent advantage over the other or perhaps it is just an inherent preference that produces this statistical “elephant in the room”? This has been particularly evident in the computer sciences when comparing the number of female graduates to male graduates. As early as 2001 Cohoon reported that although access for women had improved dramatically over the years, this did not naturally translate into an improvement in the percentage of female graduates. This trend was similar in the engineering fields (Camp, 1997). This trend has persisted into recent times and Stoet and Geary (2018) reported on the “Gender-Equality Paradox” in STEM education. Their study suggests that in countries with more gender equality, there are fewer women in STEM. In America, just 17 percent of American computer science college degrees are awarded to women. Mahdy and Ewaida (2022) used a survey to investigate the perceptions of male and female students to transition from traditional studies to online studies. Here, they reported a trend that female students perceived that they were less comfortable with their technological skills during online learning of anatomy compared to male students, although this differential was not statistically significant.
We, therefore, wanted to use this opportunity to investigate whether a gender-based disparity existed in the medical sciences, especially when the presentation and assessment of the module were compelled to transition rapidly from the traditional (analog) teaching and assessment style to a digital format. Given the reported aversion of females to STEM, we hypothesized that the technological computer-based transition would pose more of a struggle for our female cohort of students.
We compared the performance of male students in Theory Test 1 and Theory Test 2 in 2019 (orthodox methods) with the comparative assessments in 2020. It is important to note that in the first term of 2020 orthodox methods were used to teach and assess, whereas in the second term the COVID-induced lockdown necessitated the migration to online systems (Figures 6A,B). In 2019, the distribution of male students’ assessment marks reflected the distribution of the class, with the data showing a significant decrease (Figure 6A; p < 0.003) in the assessment performance in Theory Test 2. In 2020, the distribution once again reflected the performance of the whole class, where the distribution of the home-based online assessment was significantly (Figure 6B; p < 0.0001) increased. The female cohort of students also reflected the assessment distribution of the whole class both in 2019 (Figure 6C) and 2020 (Figure 6D). However, the 2019 decrease in the traditional assessment performance in Theory Test 2 was more pronounced in the female cohort of students, as indicated by the higher level of statistical significance (Figure 6C; Female TT2 (2019); p < 0.0008) compared to males (Figure 6A; TT2 (2019); p < 0.003). This implied that the female cohort of students in 2019 appeared to be slightly more challenged than the males in Theory Test 2. However, this differential did not occur in 2020, where the statistical significance between Theory Test 1 and Theory Test 2 both generated the same statistical p-value (p < 0.0001: Figures 6B,D).
This indicated that there was no gender differential in the way males and females responded to the challenge of online learning and assessment. This was confirmed by comparing the 2020 assessment data for male versus female cohorts of students for both Theory Test 1 and 2 (Figures 6E,F). Statistically, there was no assessment difference between the male and female student cohorts that reflects a differential in the way both genders adapted to the transition of an exclusive online platform. Our data was supported by the work of Zarcone and Saverino (2022) who also compared the gender scores obtained both pre-and during the COVID pandemic. Their study also showed that both males and females responded equally to the challenge of transitioning to an online teaching format. Furthermore, collaborating our study, they showed that the average marks of both males and females improve with online teaching.
Summary remarks
Due to the continuing lockdown of countries and borders in an attempt to restrict the spreading of the COVID-19 virus, universities closed their doors, research laboratories, and students were sent home (Hedding et al., 2020). This led to a radical move away from face-to-face learning and teaching to investment in existing online platforms and exploration into the viability of the e-learning online environment as an alternative (Mishra et al., 2020; Rapanta et al., 2020). This most likely has implications for the long-term rendition of university courses, where we envision that online-based lectures and assessments will entrench themselves in the course offerings of most tertiary institutions.
Our in-depth investigation of the impact that the switch from face-to-face learning and sit-down assessments to online learning and assessments via the Sakai (iKamva) platform on the performance of a second-year Biomedical Science students indicates that online computer-based assessment is a promising alternative to traditional paper-based testing (Hosseini, 2017; Prisacari et al., 2017; Öz and Özturan, 2018). This transitional assessment ideology is supported by several reports of optimism for conducting assessments using online platforms (Wibowo et al., 2016; Martinavicius et al., 2017; Armoed, 2021). Reviews on grading and proctoring assessments during lockdown by Flaherty (2020) and García-Peñalvo et al. (2021) have noted the importance of e-proctoring in institutions that are adopting flexible multimodal solutions to computer-based assessment. Although digital scoring saves resources (Seale, 2002), some academics maintain that there is still a need to understand the impact, shortcomings, and learner proficiency (Guimarães, 2017).
Our TP data, gender assessment data and marks distribution data endorsed the view that second-year tertiary students are indeed ready to embrace online learning and teaching, together with online assessments.
The sudden transition to online studies amidst the trauma of the COVID-pandemic led to a concern as to whether students would be able to cope with the pressures of isolation and self-discipline on learning outcomes and assessments. However, in retrospect, students appeared resilient and appeared to cope well with the transition to online learning and home-based online assessments. We found that the gender data subsets correlate well with each other, where statistically there are no significant differences between the marks of gender subsets in terms of moving from paper-based to computer-based online testing platforms (Figures 5E,F). This indicated that the male and female cohorts of students were able to adjust to the challenges of the digital format of learning equally well. The data puts to rest the ideology that there is a gender differential in adjusting to learning and assessments in the online digital landscape.
Lastly, it was difficult to assign precise reasons for the improved online assessment performance. This could indeed have culminated from a combination of factors: Firstly, traditional lectures are not recorded and if students missed comprehending some components, or were just not as attentive during the lecture, they could easily miss an important part of sequential learning and would subsequently find it difficult to be able to make progress in comprehending foundational theoretical components of the theory, as opposed to online video-based lectures which were available on-demand, and in which you could easily backtrack over a section that you did not understand at the first opportunity. Secondly, the level of anxiety that is generated by orthodox assessments may indeed be counterproductive, compared to having a home-based online assessment. Further, the ability to have access to your notes during an assessment are not necessarily averse to facilitating in-depth learning, as the student has to engage the question and then intellectually engage with the course work. Our assessments were allotted a time allocation specifically to diminish the incentive to have to search Google or lecture PowerPoints to find answers and essentially reproduces an eproctoring phenomenon without the anxiety of literal proctoring (traditional assessment invigilation/supervision). As these assessments took place during strict lock-down conditions, during which no one was allowed to leave their homes, we can eliminate peer consultation during the assessments. Furthermore, we have confidence that the implementation of randomized questions and answers, in conjunction with the shortened timeframe to answer questions (< 1 min per MCQ), the limitation of online questions to only MCQs, played an important role in limiting the use of notes, or online resources during an assessment.
Limitations and strengths of the study
One of the limitations of this study is that the conditions of the COVID-induced lockdown, with its associated anxiety, fears, and physical constraints, would be difficult to repeat. Furthermore, the ethical constraints on proposing a similar study during “normal” tertiary conditions, would largely prevent the duplication of these assessment conditions. However, the data supports the postulate that students are resilient and have the necessary resolve to respond to these challenges, however ad hoc and difficult. One of the strengths of the paper is the statistical analysis and comparisons of the assessment data. Here we used Gaussian distribution curves to show that the traditional assessments were statistically identical to the home-based-online assessments in terms of the shape of the curve. This showed that the home-based-online assessments were able to categorize students in the same way as traditional supervised assessments, which provided much confidence that the standards of our assessments were maintained throughout the COVID-19 lockdown. This suggests that home-based-online assessments are comparable to traditional assessments, provided that the assessment time constraints and randomization of questions and MCQ choices are implemented.
Conclusion
• Given the traumatic nature of the COVID-19 pandemic, students proved to be resilient in adapting to both online teaching and presentations, as well as to online assessments (Figures 3, 4).
• Online assessments move the assessment distribution curve to the right but did not affect the shape of the distribution curve: what this indicated was that weaker students still occupied the lower end of the distribution curve, while the majority of students were distributed centrally, leaving the above-average students occupying the right side of the distribution curve. This fundamentally suggested that assessment standards were maintained for the online assessments.
• TP for online CE assessment events is a better predictor for the final TP of the MBS231 module than traditional assessment TP (see Figure 4).
• Given that the kurtosis (shape) of the marks-distribution curve did not statistically differ between F2F and home-based online assessments, the higher marks for online assessments than for orthodox assessments (Figure 2) could be attributed to the online presentation of lectures and supporting materials and the decrease in anxiety being assessed at home.
• In the final analysis online exam TP was only marginally better than TP for orthodox lecture presentations and assessments (Online assessment TP: 99%; orthodox assessments: 90%).
• We found no gender-based difference or advantage between males and females in adjusting to online presentations and online assessments even though computer/technical skills are a definite advantage when navigating in an online paradigm (Figure 5).
• Our analysis was unable to conclusively differentiate whether the increase in online performance was due to students having ad libitum access to online video-based lectures or if it was due to the decrease in anxiety during the assessment, or indeed a combination of the two, and if access to learning material during the assessment contributed to the increase in assessment performance.
• Reflection: Better e-proctoring tools need to be further developed for home-based online assessments. However, the randomized generation of questions for every individual student, in parallel with time constraints to complete an assessment, and the strict government lockdown (South Africa: level 5), all compelled the students to engage the course content thoroughly before an assessment. We reasoned that there would be limited time to engage in online searches during the online assessment to ensure correct answers and still complete the assessment in the allotted time.
Data availability statement
The original contributions presented in the study are included in the article/supplementary material, further inquiries can be directed to the corresponding author.
Ethics statement
Ethical review and approval were not required for the study on anonymized secondary data sets of human participants by the local legislation and institutional requirements. Written informed consent for participation was not required for this study in accordance with the national legislation and the institutional requirements for anonymized secondary data sets.
Author contributions
DF compiled the manuscripts and wrote the results and discussion sections. DS editing the manuscripts and making sure we use correct educational terms, as DS is an educational specialist in Mathematics Department. KM cleaning the data, analyzing, and writing the methods section. All authors contributed to the article and approved the submitted version.
Acknowledgments
The authors wish to thank the Department of Biomedical Sciences at University of the Western Cape for using assessment data.
Conflict of interest
The authors declare that the research was conducted in the absence of any commercial or financial relationships that could be construed as a potential conflict of interest.
Publisher’s note
All claims expressed in this article are solely those of the authors and do not necessarily represent those of their affiliated organizations, or those of the publisher, the editors and the reviewers. Any product that may be evaluated in this article, or claim that may be made by its manufacturer, is not guaranteed or endorsed by the publisher.
References
Anderson, L.W., Krathwohl, D.R., Airasian, P.W., Cruikshank, K.A., Mayer, R.E., Pintrich, P.R., et al. (2001). A Taxonomy for Learning, Teaching, and Assessing: A Revision of Bloom’s Taxonomy of Educational Objectives. New York: Longman, ISBN 0-321-08405-5.
Armoed, Z. (2021). The COVID-19 pandemic: online teaching and learning at higher education institutes. IOP Conf. Ser. Earth Environ. Sci. 654:012026. doi: 10.1088/1755-1315/654/1/012026
Aristovnik, A., Keržič, D., Ravšelj, D., Tomaževič, N., and Umek, L. (2020). Impacts of the COVID-19 pandemic on life of higher education students: a global perspective. Sustainability (Switzerland) 12, 1–34. doi: 10.3390/su12208438
Balogun, A. G., and Olanrewaju, A. S. (2016). Role of computer self-efficacy and gender in computer-based test anxiety among undergraduates in Nigeria. Psychol. Thought 9, 58–66. doi: 10.5964/psyct.v9i1.160
Boevé, A. J., Meijer, R. R., Albers, C. J., Beetsma, Y., and Bosker, R. J. (2015). Introducing computer-based testing in high-stakes exams in higher education: results of a field experiment. PLoS One 10, 1–13. doi: 10.1371/journal.pone.0143616
Bond, M. (2020). “Mapping research in student engagement and Educational Technology in higher education: A systematic evidence map,”. International Journal of Educational Technology in Higher Education 17. doi: 10.1186/s41239-019-0176-8
Bozalek, V., and Boughey, C. (2012). (Mis) framing higher education in South Africa. Soc. Policy Adm. 46, 688–703. doi: 10.1111/j.1467-9515.2012.00863.x
Carlitz, R. D., and Makhura, M. N. (2021). Life under lockdown: illustrating trade-offs in South Africa’s response to COVID-19. World Dev. 137:105168. doi: 10.1016/j.worlddev.2020.105168
Conana, H., Solomons, D., and Marshall, D. (2022). Supporting the transition from first to second-year mathematics using legitimation code theory. Enhancing Sci. Educ., 206–223. doi: 10.4324/9781003055549-15
Conrad, D., and Openo, J. (2018). Assessment Strategies for Online Learning: Engagement and Authenticity. Edmonton, AB: Athabasca University Press.
Corder, G. W., and Foreman, D. I. (2014). Nonparametric Statistics: A Step-by-Step Approach. New York: Wiley. ISBN 978-1118840313.
Donthu, N., and Gustafsson, A. (2020). “Effects of COVID-19 on business and research,”. Journal of Business Research. 117, 287–289. doi: 10.1016/j.jbusres.2020.06.008
Daniels, L. M., and Gierl, M. J. (2017). The impact of immediate test score reporting on university students’ achievement emotions in the context of computer-based multiple-choice exams. Learn. Instr. 52, 27–35. doi: 10.1016/j.learninstruc.2017.04.001
Ekstrøm, C. T., and Sørensen, H. (2019). Introduction to Statistical Data Analysis for Life Sciences, Boca Raton: CRC Press Taylor & Francis Group. ISBN 978-1-4398-2555-6.
Fisher, R., Raines, P., and Burns, T. (2011). The sophomore-year experience. Nashville, TN: Belmont University, 1–57.
Flaherty, C. (2020). Online proctoring is surging during COVID-19. Available at: https://www.insidehighered.com/news/2020/05/11/online-proctoring-surging-during-covid-19 (Accessed November 27, 2022).
García-Peñalvo, F. J., Corell, A., Abella-García, V., and Grande-de-Prado, M. (2021). Recommendations for mandatory online assessment in higher education during the COVID-19 pandemic. in Lecture Notes in Educational Technology. Springer Singapore.
Gibbs, G. (2010). Dimensions of Quality. New York: The Higher Education Academy. Available at: https://www.heacademy.ac.uk/sites/default/files/dimensions_of_quality.pdf (Accessed November 27, 2022).
Graunke, S. S., and Sherry, A. W. (2005). An exploration of the factors that affect the academic success of college sophomores. Coll. Stud. J. 39:367.
Gregory, M. S.-J., and Lodge, J. M. (2015). Academic workload: the silent barrier to the implementation of technology-enhanced learning strategies in higher education. Distance Educ. 36, 210–230. doi: 10.1080/01587919.2015.1055056
Guimarães, B. (2017). “Performance equivalency between computer-based and traditional pen-and-paper assessment: A case study in clinical anatomy,”. Anatomical Sciences Education, 11, 124–136. doi: 10.1002/ase.1720
Hedding, D. W., Greve, M., Breetzke, G. D., Nel, W., and van Vuuren, B. J. (2020). COVID-19 and the academe in South Africa: not business as usual. S. Afr. J. Sci. 116, 8–10. doi: 10.17159/sajs.2020/8298
Hosseini, M. (2017). “Replacing paper-based testing with an alternative for the assessment of Iranian undergraduate students: Administration mode effect on testing performance,” International Journal of Language and Linguistics, 5, p. 78. doi: 10.11648/j.ijll.20170503.13
Jumaa, M. I., Hanafy, S. M., and Arafa, M. A. (2022). With the new challenges of COVID-19, can we depend entirely on online learning for higher education in the medical schools? Adv. Med. Educ. Pract. 13, 829–837. doi: 10.2147/AMEP.S368438
Khasawneh, R. R. (2021). Anatomy education of medical students during the COVID-19 pandemic. Int. J. Morphol. 39, 1264–1269. doi: 10.4067/S0717-95022021000501264
Lima, K. R., das Neves, B.-H. S., Ramires, C. C., dos Santos Soares, M., Vila Martini, V. A., Lopes, L. F., et al. (2020). Student assessment of online tools to foster engagement during the COVID-19 quarantine. Adv. Physiol. Educ. 44:2020. doi: 10.1152/advan.00131.2020
López-Pérez, M. V., Pérez-López, M. C., and Rodríguez-Ariza, L.. (2011). “Blended learning in higher education: Students’ perceptions and their relation to outcomes,”. Computers & Education, 56:818–826. doi: 10.1016/j.compedu.2010.10.023
Mahdy, M. A. A., and Ewaida, Z. M. (2022). Evaluation of the emergency remote learning of veterinary anatomy during the COVID-19 pandemic: global students’ perspectives. Front. Educ. 6:728365. doi: 10.3389/feduc.2021.728365
Mahdy, M. A. A., and Sayed, R. K. A. (2021). Evaluation of the online learning of veterinary anatomy education during the Covid-19 pandemic lockdown in Egypt: students’ perceptions. Anat. Sci. Educ. 15. doi: 10.1002/ase.2149
Marinoni, G., Land, H.Van, and Jensen, T. (2020). The impact of COVID-19 on higher education around the world IAU global survey report. Available at: https://www.iau-aiu.net/IMG/pdf/iau_covid19_and_he_survey_report_final_may_2020.pdf (Accessed November 27, 2022).
Martinavicius, J., Miskinis, A., and Druteikiene, G. (2017). Factors affecting students’ satisfaction and learning outcomes. in INTED proceedings [preprint]. Valencia, Spain: Vilnius University.
Massey, F. J. (1951). The Kolmogorov–Smirnov test for goodness of fit. J. Am. Stat. Assoc. 46, 68–78. doi: 10.1080/01621459.1951.10500769
Mhlanga, D., and Moloi, T. (2020). COVID-19 and the digital transformation of education: what are we learning on 4IR in South Africa? Educ. Sci. 10, 1–11. doi: 10.3390/educsci10070180
Mishra, L., Gupta, T., and Shree, A. (2020). Online teaching-learning in higher education during lockdown period of COVID-19 pandemic. Int. J. Educ. Res. Open 1:100012. doi: 10.1016/j.ijedro.2020.100012
Motala, S., and Menon, K. (2020). In search of the “new normal”: reflections on teaching and learning during Covid-19 in a south African university. S. Afr. Rev. Educ. 26, 80–99.
Olt, M. R. (2002). Ethics and Distance Education: Strategies for Minimizing Academic Dishonesty in Online Assessment. Carrollton, GA: State University of West Georgia, Distance Education Center.
Omar, H. A., Ali, E. M., and Belbase, S. (2021). Graduate students’ experience and academic achievements with online learning during COVID-19 pandemic. Sustainability 13:13055. doi: 10.3390/su132313055
Openo, J. (2017). You can fast forward your instructor: an interpretive phenomenological analysis of blended learning. in Paper presented at Society for Teaching in learning in higher education, Halifax, NS.
Öz, H., and Özturan, T. (2018). Computer-based and paper-based testing: does the test administration mode influence the reliability and validity of achievement tests? J. Lang. Ling. Stud. 14, 67–85.
Özen, K. E., Erdoğan, K., and Malas, M. A. (2022). Evaluation of views and perceptions of the medical faculty students about distance anatomy education during the COVID-19 pandemic. Surg. Radiol. Anat. 44, 61–71. doi: 10.1007/s00276-021-02867-7
Paechter, M., and Maier, B. (2010). Online of face-to-face? Students’ experiences and preferences in e-learning. Internet High. Educ. 13, 292–297. doi: 10.1016/j.iheduc.2010.09.004
Prisacari, A. A., Holme, T. A., and Danielson, J. (2017). Comparing student performance using computer and paper-based tests: results from two studies in general chemistry. J. Chem. Educ. 94, 1822–1830. doi: 10.1021/acs.jchemed.7b00274
Rapanta, C., Botturi, L., Goodyear, P., Guàrdia, L., and Koole, M. (2020). Online university teaching during and after the Covid-19 crisis: refocusing teacher presence and learning activity. Postdigit. Sci. Educ. 2, 923–945. doi: 10.1007/s42438-020-00155-y
Roddy, C., Amiet, D. L., Chung, J., Holt, C., Shaw, L., McKenzie, S., et al. (2017). Applying best practice online learning, teaching, and support to intensive online environments: an integrative overview. Front. Educ. 2:10. doi: 10.3389/feduc.2017.00059
Saverino, D., Marcenaro, E., and Zarcone, D. (2021). Teaching histology and anatomy online during the COVID-19 pandemic. Clin. Anat. 35, 129–134. doi: 10.1002/ca.23806
Scott, I. R., Yeld, N., and Hendry, J. (2007). “A case for improving teaching and learning in south African higher education” in In Higher Education Monitor 6 (Pretoria: Council on Higher Education). isbn:978-1-919586-62-9
Seale, J. (2002). Using CAA to support student learning. LTSN Generic Centre. Available at: www.ltsn.ac.uk/application.asp?app=resources.asp&process=full_record§ion=generic&id=38 (Accessed November 27, 2022).
Stoet, G., and Geary, D. C. (2018). The gender-equality paradox in science, technology, engineering, and mathematics education. Erratum in: Psychol Sci. 31, 110–111. doi: 10.1177/0956797617741719
UWC CIECT (2020). Content owner: Juliet Stoltenkamp, Main contributors: Instructional design team [e-learning marketing campaign since 2009]. Courtesy UWC Centre for Innovative, Education and Communication Technologies (CIECT). Available at: https://ciect.wordpress.com/ (Accessed November 27, 2022).
UWC NSCI (2021). UWC Faculty of Natural Sciences: Blended Learning Approaches & Arrangements during national lockdown by CIECT, Vol. 15 https://ciect.wordpress.com/2021/02/15/science-faculty-blended-learning-approaches-arrangements-during-national-lockdown/ (Accessed November 27, 2022).
Vaughan, N. (2015). “Student assessment in a blended learning environment: a triad approach” in Assessment in online and blended learning environments. eds. S. Koc, X. Liu, and P. Wachira (IAP: Charlotte, NC)
Wang, Y., Han, X., and Yang, J. (2015). Revisiting the blended learning literature: using a complex adaptive systems framework. J. Educ. Technol. Soc. 18, 380–393.
Wibowo, S., Grandhi, S., Chugh, R., and Sawir, E. (2016). A pilot study of an electronic exam system at an Australian university. J. Educ. Technol. Syst. 45, 5–33. doi: 10.1177/0047239516646746
Yeld, N. (2010). “Some challenges and responses: higher education in South Africa” in Discourse – Transforming higher Education in South Africa: Finding New Direction. ed. G. Bloch (Johannesburg: University of Johannesburg)
Keywords: blended learning, e-learning, assessment strategies, iKamva, home-based online assessments
Citation: Fisher D, Solomons D and Makhathini KB (2022) Face-to-face versus online-based lectures: A COVID-19 induced study on assessments. Front. Educ. 7:1045311. 10.3389/feduc.2022.1045311
Edited by:
Antonio Palacios-Rodríguez, Sevilla University, SpainReviewed by:
Mohamed A. A. Mahdy, South Valley University, EgyptPietro Picerno, University of eCampus, Italy
Copyright © 2022 Fisher, Solomons and Makhathini. This is an open-access article distributed under the terms of the Creative Commons Attribution License (CC BY). The use, distribution or reproduction in other forums is permitted, provided the original author(s) and the copyright owner(s) are credited and that the original publication in this journal is cited, in accordance with accepted academic practice. No use, distribution or reproduction is permitted which does not comply with these terms.
*Correspondence: Khayelihle Brian Makhathini, a21ha2hhdGhpbmlAdXdjLmFjLnph
†ORCID: David Fisher https://orcid.org/0000-0001-6070-0234
Deon Solomons https://orcid.org/0000-0003-0659-9942
Khayelihle Brian Makhathini https://orcid.org/0000-0001-7153-8201