- Department of Chemistry and Chemistry Education, Faculty of Education, Charles University, Prague, Czechia
Only teachers who possess problem-solving skills can develop them in their students. These skills therefore need to be accentuated during teachers’ pre-service training. In this study, attention was given to pre-service chemistry teachers’ (students) problem-solving skills measured with the use of two sets of problem tasks–chemistry and general science tasks. Based on a pre-test consisting of both types of tasks, one successful, one partially successful and one unsuccessful solver was selected from a group of first-year bachelor chemistry teacher students. To compare, the tasks were also given to three experts (post-docs in the field of chemistry education). All the participants solved two tasks on a computer with their eye movements recorded. After the procedure, retrospective think-aloud and interviews were conducted to provide data about the problem-solving process. The results showed several trends. (1) Students–novices considered the chemistry task more difficult than the science task, which correlated with their task results. (2) Experts considered the science task more complex, therefore more difficult, however scored better than the students. (3) Even the successful student only solved the chemistry task using memorized facts without the support provided. (4) Experts’ direct focus on relevant parts was confirmed, whereas unsuccessful (novice) students distributed their focus toward other task parts too. (5) When students faced a problem during task solving, they used limiting strategies. This behavior was not identified in the expert group. The results thus showed a need to support students’ problem-solving strategies in several areas, especially careful reading, and identifying the main problem and supporting information. Moreover, the results showed a need to present chemistry tasks to students with more variability and explain their reasoning rather than testing field-specific, separated, memorized information.
Introduction
Problem-solving ability has been seen as crucial (Bellanca, 2010; OECD, 2016; De Wever et al., 2021) and is predicted to be one of the most important skills for the future (OECD, 2018). It is also strongly linked with the Program of International Student Assessment (PISA) research results which are widely discussed topic in the field of education as PISA is considered the most important international research in the field of measuring educational outcomes (Potužníková et al., 2014). However, it is often the target of criticism for possible errors or imperfections that may be caused by the measurement load or incorrect interpretation and uncritical approach to their results (Straková, 2016; Zhao, 2020). The general public, as well as the politicians of given countries, react to the test results. They are used to respond to current changes in educational policy (Gorur, 2016; Vega Gil et al., 2016). However, the indicator they are guided by is often only the ranking of the state’s pupils (Kreiner and Christensen, 2014; Štech, 2018). Test scores alone may not be sufficient in the future, given that tests fail to capture subtle but important differences between students. New methods are required for teachers to determine whether students have truly understood a given topic (Tai et al., 2006).
Program of international student assessment (PISA) results consistently indicate that Czech students’ ability in the area of problem-solving has been declining (OECD, 2016, 2019). The reasons behind these results remain hidden. The possible cause may be lack of its development in schools. Teachers play a crucial role in these skills’ development see e.g., Tóthová and Rusek (2021b). Apart from including problem-tasks in their lessons (Lee et al., 2000), the teachers’ own ability to solve problems is seen as necessary (Krulik and Rudnick, 1982). Only quantitative research and mere tests are not sufficient enough to get more information (e.g., Barba and Rubba, 1992). Correct answers in these tests may not refer to conceptual understanding of the problem (Phelps, 1996; Tai et al., 2006; Rusek et al., 2019). Some research aimed at this area used qualitative research (e.g., Cheung, 2009; Barham, 2020). More studies are needed to understand the state of these abilities in pre-service teachers and design university courses.
To elucidate the process of problem-solving, think-aloud (Rusek et al., 2019) and eye-tracking (Tsai et al., 2012) proved to be sufficient methods. With a combination of these methods, students’ procedures in solving tasks can be described in more detail see e.g., Tóthová et al. (2021). A more in-depth analysis and investigation into the reasons for a student’s choice of a given answer can be an indicator to further develop the monitored competences.
The present study therefore included all the above-mentioned aspects: used a combination of qualitative methods to evaluate pre-service teachers’ problem-solving skills and processes. The results can bring the information needed to develop university courses as well as improving pre-service teacher training practice.
Theoretical background
This study was based on eye-tracking as with its expansion in education research see e.g., Lai et al. (2013), its use in analyzing science problem-solving has been increasing. Despite the possibilities such as eye-tracking goggles [used e.g., in research in laboratory (van der Graaf et al., 2020)], the use of eye-tracking methods in science education research still remains mainly in laboratory conditions with the use of screen-based or stationary eye-trackers (Tóthová and Rusek, 2021c).
When analyzing problem-solving with the use of eye-tracking, attention was paid to the use of scientific representations (Lindner et al., 2017; Klein et al., 2018; Wu and Liu, 2021). To analyze those, the specific metrics, such as number of fixations (NOF) or total fixation duration (TFD), proved to be useful. The results showed that the use of representations helped students understand the text and solve given problems (Lindner et al., 2017). In the same research area, Rodemer et al. (2020) explained the influence of previous knowledge and skills when using representations. More experienced students were able to make more transitions between the provided representations, therefore using the support they were given by the task itself. The use of these representations may be influenced by the strategies students apply (Klein et al., 2018) and also vice versa, the use of text and visualizations can influence the strategies used (Schnotz et al., 2014).
It is, therefore, not surprising that the strategies used during problem-solving are often the subject of research. The differences between novices and experts in the used strategies were shown in the study by Topczewski et al. (2017). With the use of order of fixations, they identified that novices approach the same problem using different gaze patterns, i.e., different strategies. The used strategies may be influenced by the type of instructions given to the students.
As the used strategies seem to be the dealbreaker in the problem-solving process, further understanding their influence is needed. Tsai et al. (2012) analyzed the difference between successful and unsuccessful solvers in multiple-choice science problem-solving. Their results showed that the differences lied in the successful solvers’ focus on relevant factors, whereas the unsuccessful showed a higher frequency of observing irrelevant factors. In a similarly targeted study, (Tóthová et al., 2021) identified the reasons for unsuccessful problem-solving in chemistry on an example of their periodic table use. Apart from focusing on irrelevant factors, the use of limiting strategies and problems with reading was identified as the main problems. This rationale stood as the basis for the presented study, whose aim was to investigate the validity of some of the aforementioned findings in another context with the use of eye-tracking-based methods.
Aims and materials and methods
Aims
The aim of this study was to map pre-service chemistry teachers’ procedures when solving problem tasks and to compare it with experts’ procedures. The study followed these research questions:
I. What, if any, is the difference between the pre-service teachers’ performance on chemistry and general science problem tasks?
II. What, if any, is the difference between pre-service teachers’ and expert procedure when solving problem tasks?
Research procedure and participants’ selection
The study was based on mixed methods. It used a pre-experimental design (Figure 1). At the beginning of the academic year 2021/2022, first-year bachelor university students who chose chemistry teaching as one of their majors at Charles University, Faculty of Education were given two sets of tasks focusing on chemistry and general science (see above). Based on their results, one successful, one partially successful, and one unsuccessful student were chosen for the follow-up eye-tracking study.
All the study participants were explained the purpose of the study and were ensured their results as well as any other data (eye-tracking and audio recordings) will remain available only to the researchers and will not be shared with a third party. For the purpose of the data presentation, the students and experts were anonymized. All the data were saved on one computer protected by a password.
It consisted of them solving another set of problem tasks of comparable difficulty (see below) with their eye-behavior being recorded by an eye-tracking camera. After calibration, participants were asked to sit still and watch the screen. The time for solving the task was not limited. Another task occurred to the solvers after a random key click. After they finished the last task, the cued retrospective think-aloud method (Van Gog et al., 2005) was used to understand the participants’ mental processes. Based on the students’ explanations of their problem-solving processes, interviews were conducted.
As the think-aloud method is based on the solvers’ explanation of their solving process and a researcher should intervene only when a respondent stops talking (Van Someren et al., 1994), the semi-structured interviews conducted after RTA provide even more information. The follow-up interview topics aimed at: task difficulty (perceived difficulty, most difficult part, more difficult task); solving confidence; type of task (similarity and differences between given tasks, similarity and differences between given task and tasks they were used to). Students attended the experiment voluntarily. During the eye-tracking measurements, they sat approximately 70 cm from the computer screen. Respondents’ think-alouds and interviews were recorded. The entire eye-tracking phase took approximately 45 min.
To compare the task-solving process, three experts on chemistry education (all owning a Ph.D. in the field) were chosen from the researchers’ department’s academic staff and participated in the eye-tracking, RTA, and interviews.
Research tool
Two sets of complex tasks1 were prepared: one for the pre-test and one for the post-test. The pre-test tasks consisted of three complex chemistry problem tasks and three complex general scientific literacy problem tasks. The test used in the eye-tracking research phase consisted only of one complex task from each group “a chemistry task” and “a PISA task,” both originally designed for 15-year-old students. The tasks focused on working with available information and visualized data. Both chemistry tasks were taken from the Czech national chemistry curriculum standards (Holec and Rusek, 2016) from which tasks focused on general chemistry were chosen. Both the PISA-like scientific literacy tasks were represented by selected items released from PISA (Program of International Student Assessment) task pilots (Mandíková and Houfková, 2012). These tasks focused on students’ ability to plan and evaluate scientific research as well as their ability to gather information from diagrams, tables, etc.
To ensure the pre- and eye-tracking test tasks were on the same level of difficulty, “optimum level” chemistry tasks and the same scientific literacy level tasks were chosen. Also, the authors’ research group members evaluated the tasks to prevent one set from being more difficult than the other.
In the chemistry task, the solvers were given three sub-tasks (referred to as Task 1–3). The first dealt with the trends in the periodic table (halogens) and their solution required working with the periodic table and using information from a text. The second subtask targeted the reaction rate of alkali metals with water. A description of lithium and sodium’s reaction with water was given and the solvers were asked to select correct descriptions of potassium’s reaction with water. In the last subtask, a general description of the trends in the periodic table regarding atomic diameter was given and the solvers were required to order the given elements based on it.
The PISA task was set into a classroom problem–students want to design a nearby river’s pollution measurements from physical, chemical and biological points of view. There were four subtasks (also referred to as Task 1–4) to this complex task. The first asked about important and unimportant equipment, in the second, the students were supposed to describe a process of water-flow measurement. The third was about an appropriate sampling site selection on a map based on concrete criteria and the last about pollution data evaluation based on data in a table.
Apparatus
Tobii Fusion Pro with a 250 Hz sampling rate and GazePoint eye-camera GP3 (60 Hz) were used in this study. Prior to all recordings, nine-point calibrations were performed. Both instruments allowed respondents’ free head movements.
Data analysis
To evaluate the PISA-like tasks, the scoring used in the original research was taken over (Mandíková and Houfková, 2012; OECD, 2016), i.e., 2 points for a completely correct result, 1 point for partially correct and 0 points for an incorrect result. The chemistry tasks were evaluated in the same way. To ensure objectivity, two researchers evaluated the tests independently and compared. The scores were reported as a percentage for later comparison.
To analyze participants’ task-solving procedure, time fixation duration (TFD) see e.g., Lai et al. (2013) in pre-selected areas of interest (AOIs) was measured. The data are reported as proportion of total fixation duration and fixation duration in particular AOI, i.e., proportion of time fixation duration see e.g., Jian (2018). The AOIs included the task itself, answer choices area, visual parts and contexts or information. The data were analyzed with the use of IMB SPSS completed with the retrospective-think aloud recordings, as well as interviews. Both were recorded, transcribed verbatim and coded using the set of codes for students’ strategies taken from previous studies (Rusek et al., 2019; Tóthová et al., 2021). They were then divided according to Ogilvie (2009)–expansive and limiting. The software QDA Miner Lite was used for transcription analysis.
The reported confidence values allowed to relate students’ and experts’ confidence with their answers (score). These data were analyzed with respect to Caleon and Subramaniam (2010). Standard metrics as CAQ (mean confidence accuracy quotient) and CB (confidence bias) were analyzed.
The Shapiro–Wilk normality test (p > 0.05) showed the data were normally distributed with an exception of two observed areas of interest (p = 0.06 resp. 0.08). For this reason, differences between the students and experts’ TFD and t-test were used to analyze the majority of TFDs, Mann–Whitney test for the non-normally distributed data. Cohen’s d resp. r were used as effect-size tests and were interpreted according to Richardson (2011).
Results
Overall results
The overall students and experts’ results (Figure 2) reveal several findings surprising in the light of the chosen tasks’ nature. The data in Figure 1 need to be read with discretion as the total number of points was low and a loss of one point reflects dramatically on the test score.
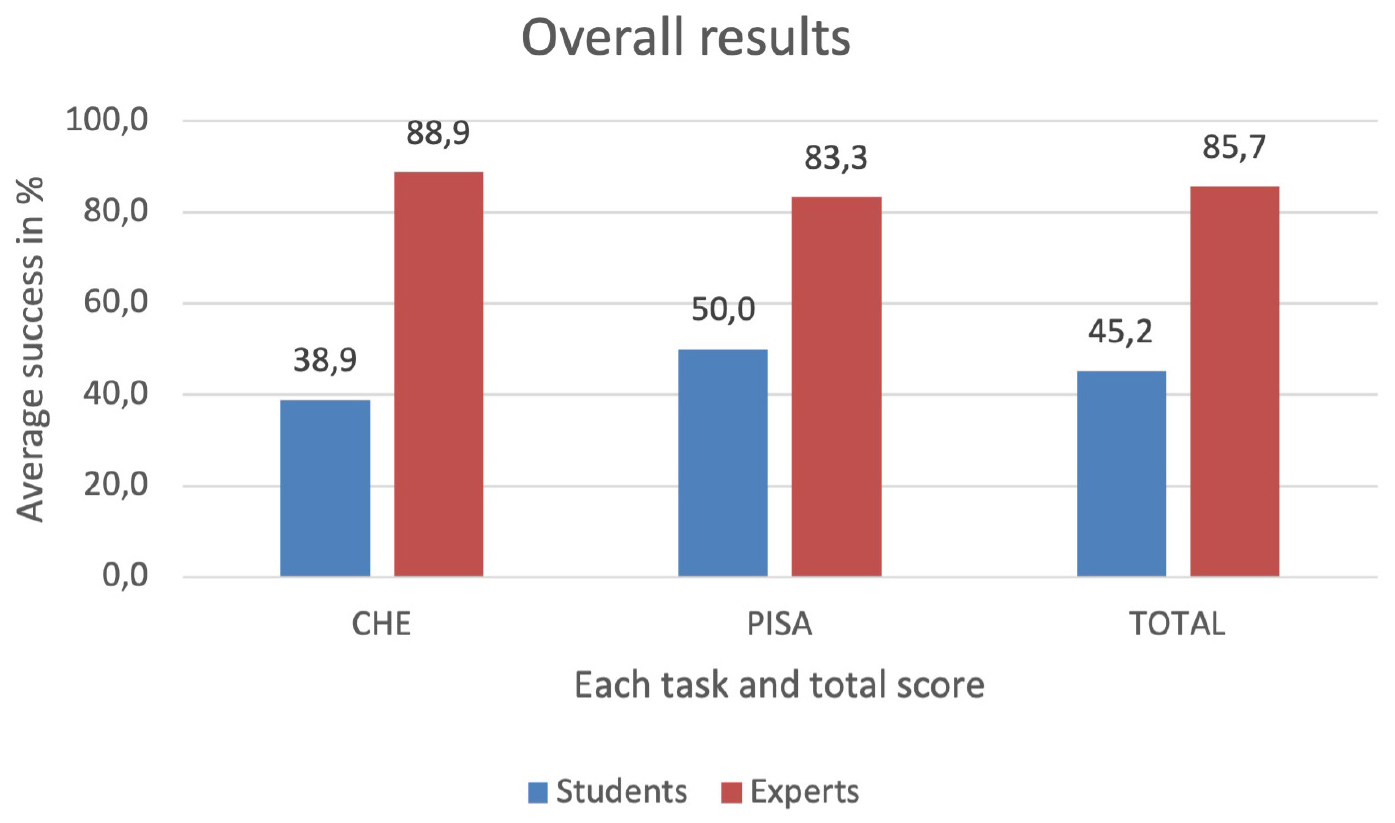
Figure 2. Participants’ performance in program of international student assessment (PISA) and chemistry (CHE) problem tasks.
To answer the first research question, the students’ and experts’ results did not statistically significantly differ (p = 0.149) as expected in such a small sample. However, the effect size (d = 1.104) showed a large effect which points to the experts’ significantly better performance. More information to answer the second research question is shown with the use of more tools: tasks, eye-tracking, retrospective think-aloud, and interviews.
The interviews showed the students overestimated their solution results. This was also reflected in their less accurate confidence scores (see Table 1 showing confidence related metrics).
The experts’ mean confidence accuracy quotient (CAQ) was positive compared to the students’. The differences were statistically significant with a large effect (p = 0.025; r = 0.598). The experts showed a higher ability to discern between what they know and do not know. On the contrary, the students’ CAQ values suggest they tend to overestimate themselves and report higher confidence despite their task solution being incorrect. The confidence bias (CB) score confirmed students’ tendency to overestimate themselves, whereas in four tasks, experts showed almost perfect to perfect calibration see Liampa et al. (2019). There was no statistically significant difference between students’ and experts’ CB (p = 0.123); however, the effect size (r = 0.413) showed a medium effect.
The eye-tracking results
The eye-tracking data served not only as a cue for the respondents’ retrospective think aloud but also as a marker of the attention both the students and experts dedicated to particular parts of the tasks. As far as the chemistry task was concerned, the students solved it on average in 5 min as compared to the experts’ 3 min 48 s. However, the unsuccessful student spent as much time on the chemistry task as the experts’ average, which was not enough. On the contrary, the successful one spent as much time on the task as one of the experts (over 5 min), which paid off. As for the PISA task, the students spent over 8 min solving in comparison with the experts’ 6 min 46 s. Again, the successful student took the longest to solve this task and the unsuccessful student used as much time as one of the experts.
This result suggests that the experts are efficient and need less time, the successful student worked their way to the solution and the unsuccessful student turned to a quick solution which did not lead to the desirable result. Naturally, this finding needs to be explained in more detail with the use of qualitative methods.
The program of international student assessment task
As far as this set of subtasks (Task 1–4) was concerned, no statistically significant differences were found on a 5% level of significance. This was expected due to the low sample size. However, when effect size was considered, five items showed a considerable power of effect-size. The experts spent more time on the task’s context (see attention map on Figure 3). The effect size had a huge power (d = 1,96), which shows they put an effort into understanding what was requested from them.
The color gradient (from red through yellow and green to white) relates to the participants’ attention and shows what part of the screen/task/area a solver focused on.
In the subtasks (Task 1–3), the students spent more time on Task 1–selecting useful equipment, and Task 4–choosing data from a table. The power of the effect was huge in both cases (dTask1 = 1.314, dTask4 = 1.645) suggesting the experts’ greater efficiency as not only did they need less time but their answers were also correct.
On the contrary, on Tasks 2 and 3, experts used more time fixating the tasks with a huge (dTask2 = 1.651) and medium (dTask3 = 0.62) effect size, which shows they paid more attention to the tasks. In the case of Task 2, it was their careful reading in order not to miss anything from the task. In Task 3, they spent the same time on the given map of a river as the students, but more time on the text.
This action was then reflected not only in their correct results but also in the amount of time spent on Task 3’s multiple-choice answer part (again the power of the effect was huge d = 1.293) which shows they just picked the correct response, in contrast to the students who spent more time on this part searching for the correct one (see Figure 4).
The chemistry task
As far as the chemistry task was concerned, surprisingly, neither the students nor the experts worked with the provided periodic table as much as was expected (see Figures 5, 6).
One obvious difference appeared when their time fixation duration was observed on the table’s legend (explaining the element groups). The students spent significantly more time on the legend. The difference was also statistically significant (p = 0.046), with a large effect size (r = 0.813) which shows the experts’ expected familiarity. Another proof of their expertise was shown in Task 3’s context. They needed significantly less time, although with just a medium effect size (d = 0.696), which showed their familiarity with the trends. On this very task, however, the large effect size (d = 0.849) showed experts spent significantly more time fixating the task. This proved to be necessary for the correct results, however, more reasoning was shown in the retrospective-think-aloud and interview (see below). A similar difference with a medium effect-size (r = 0.445) was found in Task 1, on which, again, experts spent more time than the students. Considering the task was not about simple recollection from memory, the eye-tracking data helped to reveal the reason for the experts’ success. Nonetheless, it was the think-aloud and interviews which fully explained the reasons.
Retrospective think-aloud results: Applied strategies
The data above showed a difference between the students and experts’ time fixation durations on different tasks. When the eye-behavior was replayed to each of the task solvers, their verbal description of the process helped identify the reasons behind their performance, i.e., use of strategies and facing problems. Although not all experts reached the maximum score in both (PISA and chemistry) tasks, their solving strategies differed from the students’ considerably. The number of coded strategies was similar in both analyzed groups (69, resp. 70). For the sake of more accurate interpretation, absolute numbers and relative representations are reported in Table 2.
Both students and experts applied mostly expensive strategies such as e.g., working with available information (tables, map, and information in assignment). An example is given for chemistry Task 2 in which the solvers were supposed to infer on the course of reaction between alkali metals with water: “Then I looked at the table, where I actually assessed that the order of the elements is lithium, sodium, potassium, according to which the reactivity due to the assignment should increase.” Surprisingly, the eye-tracking data did not show a difference in the time fixation duration, despite the experts being familiar with these reactions and not being expected to use inductive thought processes with the use of the periodic table.
Another example of an expansive strategy in use is reflection. In PISA Task 2, in which the solvers were supposed to choose appropriate parts of a river for water sampling, one of them mentioned “…then I actually found out…, I thought that it would be appropriate to measure all three points < on the river > , and as soon as the answer wasn’t there, I found out that the question is probably different than I initially understood….”
One type of strategy was found only in experts’ progress–mentioning alternative solutions. The example is from PISA Task 3: “When they go to map it < the area in the task > , they have to write it down somewhere. But here I mentally came across the fact that when I want to record something, I usually take my phone. Or I turn on the navigation and don’t need a map at all.” This strategy explains the same time spent on the map and also the longer time fixation duration on this task’s text.
The difference between students and experts in using reading strategies was only slightly shown by eye-tracking–shorter time spent on certain texts–was skipping unnecessary information. This behavior was not identified during the think-aloud with the students: “I skipped the picture because I was looking at what to do first, if I had needed it, I would have come back to it later.”
The possible key to the expertise in problem-solving is to avoid limiting strategies. In some cases, they may lead to the correct answer, but they are not applicable in any other cases and therefore represent unwanted behavior (Ogilvie, 2009). Students applied these strategies in almost 22% of the problem-solving process. Students, for example, used a guessing strategy when they faced a problem with a lack of knowledge. An example from chemistry Task 2: “…the second task, I had no idea, so I read the options and guessed…” This was possible to observe in the students’ eye-movements (see Figure 7) compared with an expert (see Figure 8), who read the task and answer choices. The students focused mainly on the answer choices, which reflected their guessing strategy.
This answer offers an explanation for the experts and students’ similar time fixation duration on this task. Whereas the experts’ was shorter due to their familiarity with the reaction, the students skipped this task once they did not know the correct response without trying to figure it out. This behavior, which also appeared in other tasks, was later explained in the interviews–students are not used to solving complex problem tasks in chemistry. Therefore, whenever there is not an immediately obvious solution, they turn to a limiting strategy and/or give up.
On the contrary, the experts did not use limiting strategies even when they faced problems (see Tables 2, 3). Here is a possible connection between their reported confidence. Students aware of guessing admitted it in the confidence scales; however, the experts did not have to. In order to promote students’ problem-solving skills, it seems necessary to concentrate on the processes following the problem’s identification.
Faced problems
As well as in the case of strategies, the problems students and experts faced differed. The results are shown in Table 3.
Students had problems with reasoning, e.g., in the case of PISA Task 2 concerning measuring the speed of the river: “…I mentioned that it could be the bottle. At what speed the water will flow to the bottle…” The problem with the lack of knowledge was surprising as the task aimed at basic knowledge in the respondents’ study field and working with given information seemed natural. Nevertheless, the problem with applying knowledge also occurred in one of the experts’ think-aloud comments. In chemistry Task 1, they were supposed to induce the color of an element based on a trend in the periodic table. The expert mentioned: “…I used the table with the trends, but I did not know the color.” This points to a finding also observed with the students–in spite of the correct mental process, this expert did not respond just because they did not recall the correct answer.
Another common problem was misunderstanding the assignment. This was related to the type of task (complex tasks with context). Students’ answers were often contradictory to the assignment. The following example regarding chemistry Task 3 on atomic diameter: “…I usually read it twice, because the elements are smaller when they have a smaller number of electrons, protons, neutrons, so I found the elements and sorted them according to the proton number,” in contrast to the information in the task itself (abbreviated): “The trend is the increase of the atomic radius with increasing proton number in groups. Conversely, between elements in periods, the atomic radius decreases with increasing proton number…”
Participations’ evaluation of the tasks
The interviews served to help the researchers understand the observed thought processes manifested by eye-behavior and later verbalized during the think-aloud phase. They also provided information about the participants’ evaluation of the tasks. The main answers from the interviews are shown in Table 4.
The interviews revealed a different attitude to the tasks. The students perceived the chemistry tasks as more difficult, due to the knowledge needed and the presence of the periodic table: “Chemistry was more difficult–it is more theoretical and also, there was a table that confuses me….” On the contrary, the experts assumed the PISA task would be more difficult for students because it included more data analysis, more logical reasoning and less information was given: “Basically, I think PISA will be harder for them–it’s completely up to the student. It doesn’t have any supportive info, whereas there’s always a hint in the chemistry one. PISA also depends more on reading–like table data….”
Both students and experts mentioned orientation in the tables and text as the difficult part in solving the tasks. The above-mentioned attitude toward chemistry task solving was therewith discovered. Students, in accordance with their predecessors (Tóthová et al., 2021), reported the periodic table as something that confuses them: “…if the table wasn’t available here, I’d be more confident. The presence of the table is a distracting element.” As already pointed out in the cited research, the original idea of an inductive tool, which the periodic table undoubtedly, seems hidden even to pre-service teachers.
However, the students and experts differed in the perceived tasks’ demands. The students saw the difference between the chemistry and PISA task mainly in the perceived needed knowledge in the chemistry task. They did not notice the purpose of the chemistry task (they were supposed to work with available information and periodic table). Only basic knowledge required in lower elementary school chemistry was needed. Another discovered difference between the students’ and experts’ opinion of the tasks resided in the theoretical basis of the chemistry task: “The chemical one is more theoretical and the other practical.” However, this student opinion was in accordance with one expert: “For the PISA task, they need to have experience with being outside in the scientific context. They will probably have one from school for the chemistry task.”
The results seem to reflect student-perceived nature of tasks and thus confirm the presumption formulated by Vojíø and Rusek (2022)–presenting students with a limited variation of tasks affects their abilities.
The interviews revealed the students were not used to solving these types of tasks. They agreed that tasks at school (of the “textbook genre”) did not require logical reasoning and thinking or working with information, but rather memorized knowledge: “The difficult thing here is that one has to think about what and how to answer, what makes sense and what doesn’t. For example, in the tests we were given (at school), we would have to say what we memorized. So I would specifically answer the questions and I was sure of the answer there too.” Experts in the field of chemistry education who regularly visit lower and upper secondary schools answered similarly and added: “Such tasks appear rather rarely.” One expert referred to one research’s finding (Son and Kim, 2015) that “when a more difficult task appears, they tend to divide it. At the same time, according to my experience, they do not work with tables and diagrams much. They are not even used to reading text.”
Discussion
Overall results
This study brought several results which inform not only the Czech education system but also the international science education community. First, the finding that pre-service teachers struggle with tasks developed for 15-year-olds suggests that upper-secondary school teachers consider success quite differently to the OECD (producing PISA tasks) and even national curriculum standards. In fact, this study confirmed previous studies’ results showing deficiencies in students’ achievement of curricular objectives (cf. e.g., Medková, 2013; Rusek and Tóthová, 2021).
The students with lower expertise (compared to experts) achieved worse results, which confirmed previous research results. For example, in a study from physics education (Milbourne and Wiebe, 2018), the importance of content knowledge was shown as one of the key factors affecting students’ results. This was highlighted by the areas they paid attention to. Also, Harsh et al. (2019) found a significant difference in students’ and experts’ ability to read graphical data representations associated with the latter group’s direct search patterns resulting in better results.
The worse student achievement in comparison with the experts was associated with their poorer ability to estimate the correctness of their own solution. This phenomenon is consistent with other research (e.g., Talsma et al., 2019; Osterhage, 2021).
The differences between chemistry and program of international student assessment tasks (RQ1)
Student statements during the RTA, as well as interviews, helped understand this contradiction in more detail. The tasks presented to the students tested their literacy, science thinking and required a certain level of competency.
Despite the fact that respondents were chosen from pre-service chemistry teachers, students were less successful in the chemistry tasks than in general scientific tasks. This was proven even in a previous study in freshmen and students in the last year of their studies (Tóthová and Rusek, 2021a). The fact students saw chemistry tasks as more difficult than the PISA tasks and also the students’ statements about the expected nature of chemistry tasks suggests students are used to a certain type of chemistry task. These are usually based on knowledge and do not require any higher order thinking such as analysis or synthesis of information from several sources. Even successful solvers confirmed they preferred to use memorized facts than the periodic table, which “confuses them.” This was proven by the solvers’ attention (reflected in TFD) paid to the periodic table, which was unexpectedly low. Thereby, a trend was confirmed pointing to a certain task culture for lower-secondary science textbooks (Bakken and Andersson-Bakken, 2021; Vojíø and Rusek, 2022). Also, the statements regarding the difference between the chemistry and PISA tasks which referred to the “need” of knowledge and memorized information when solving chemistry content tasks confirm the misunderstanding of chemistry’s nature, resp. the periodic table (cf. Ben-Zvi and Genut, 1998). The interesting thing is that in the scientific (PISA) task, students did not mention needed knowledge; however, there were some scientific concepts, e.g., speed calculations.
On the other hand, experts considered the science task more complex, requiring more steps to solve them. Therefore, the PISA task was, according to them, more difficult.
The differences between students and experts (RQ2)
Differences were identified in the groups’ eye-tracking records. TFD spent on defined AOIs alone cannot explain the solving process. Combining it with other methods was therefore necessary. Differences between students and experts were evident in the strategies used. Experts used only expansive strategies, whereas students tended to also use limiting strategies, e.g., guessing. The use of this strategy was found in previous research and was related more likely to the group of low achievers in the case of test-taking strategies (Stenlund et al., 2017). The use of limiting strategies also seemed to lead to incorrect solutions in this study. The reasons for using these strategies were the identified problems: lack of knowledge, non-logical reasoning or misunderstanding the assignment. This is consistent with previous research (Tóthová et al., 2021). However, when experts faced a problem, they did not use the limiting strategy and continued using expansive strategies. At the same time, this finding further stresses the importance of a more cautious approach to complex tasks such as those used in the PISA framework. Its robust task piloting as well as large samples on one hand limit external factors, nevertheless in light of this study’s (and other) results, a number of false-positive results is very likely and merely dividing students according to literacy levels hardly provides sufficient information to suggest concrete changes in classroom instruction (cf. Tóthová and Rusek, 2021b).
Also, the problems faced by students and experts differed. The problem with misunderstanding the task leading to the incorrect solution occurred in students. Eye-tracking enabled these problems to be connected to the attention paid to concrete areas in the tasks. The difference between attention paid to concrete AOIs in students and experts occurred in the context part. Whereas in the PISA task, which dealt with general scientific knowledge, experts spent more time reading the task context, in the field of their study (chemistry) their spent significantly less time on the task context. This may reflect experts’ ability to choose relevant information for their solution (c.f. Tsai et al., 2012), whereas students still have to learn this. Also, the attention paid to the task parts differed between students and experts. Students focused more on the answer choices and less on the text needed. This finding is in accordance with previous research which showed significant inadequacies in students’ reading comprehension with regards to their task-solving results (Imam et al., 2014; Akbasli et al., 2016; Tóthová et al., 2021; Tóthová and Rusek, 2021a). It further stresses the aforementioned need to understand students’ performance on PISA (and PISA like) tasks better as they have immense impact on education systems, despite the reasons not seeming to be very clear. The most problematic part seen by students was working with tables and maps. This is surprising when visualizations play a crucial role in science as well as in science education (Gilbert, 2005). This phenomenon was not connected only to the general scientific task, but also to the field-specific periodic table. Students spent significantly more time on its legend which described the groups’ names, despite it not being relevant for solving the task.
Another reason for the different solving process may be the fact that a student’s brain cannot solve problems in this manner without memorizing it (Hartman and Nelson, 2015). Research (Tóthová and Rusek, 2021b) showed that supporting problem-solving strategies in several problematic areas, especially careful reading, identifying the main problem and supporting information led to better results and problem-solving skills’ development.
Students in this study mentioned memorized information to be a determinant of successful chemistry task solution. This is in contrast with the fact they could find the information in the tasks. This was later confirmed in the interviews, where reading and working with information was named as the most difficult. The results therefore showed a need to present chemistry tasks in more variable ways to pre-service teacher students and explain their reasoning beside testing field-specific, separated, memorized information.
The results of this study are affected by two major factors which limit the extent to which the findings can be generalized. First, it is the small sample. On the one hand, it provides information only about a small group of participants, on the other, it enables the use of a vast palette of interconnected methods which enable a thorough description of the studied phenomenon. Based on these data, it is later possible to focus on a smaller, more concrete aspect on a larger research sample. Second, it is the sample selection. Though the students were pre-selected based on their pre-test result, the performance especially of partially-successful and unsuccessful students was quite similar which did not result in as many findings as expected. Again, with a larger sample, more differences could be found. Also, as the experts sample was convenient, further research could include also experts from science disciplines.
Conclusion
The comparison of students and experts’ general science and chemistry oriented complex problem tasks showed several trends in the participants’ problem-solving processes. Combining tasks, eye-tracking, cued retrospective think-aloud and interviews, though time consuming, brought several important findings which deepen contemporary understanding of the problem-solving process. Despite the results not being generalized due to a small sample, they have the potential to inform the (science) education community in its endeavor to more effective instruction.
The pre-service teacher students considered chemistry tasks more difficult than science tasks, which was reflected in their results. On the other hand, the experts considered science tasks more complex and more difficult. The reasons behind the differences in the groups’ performance revealed possible areas the students need to improve but also raised more questions to be answered in future research.
The experts spent more time reading the task context in the PISA task requiring general scientific knowledge. However, their time-fixation duration was shorter in most parts of the chemistry-related task that proved their expertise. On the contrary, students’ longer time spent on the unnecessary information was one of the indicators for their lower success. Their task-solving processes revealed their lower ability to use information provided in the text, which was identified through the lack of attention paid to the periodic table and confirmed in their spoken description of the problem-solving process (think-aloud and interviews). In the interviews, even successful students tended to mention the importance of memorized information, mainly in chemistry tasks (the field of their study), and, that provided information confused them “you simply have to know that.”
The differences between the students and experts were also shown in the strategies they used. Both groups used mainly expansive strategies. However, students used limiting strategies 22% of the time, unlike experts who did not apply limiting strategies at all. The expertness consisted in the participants’ variability of applied expansive strategies even in cases where the originally chosen strategy did not work. This is another issue teacher training needs to focus on.
Limiting strategies were connected to the problems the solvers faced. Logical reasoning, knowledge, and understanding an assignment were proved to be crucial. When reading a task, differences between reading behavior in students and experts appeared to be a possible reason for incorrect solutions. Therefore field-specific reading needs to be focused on pre-service teacher training.
The results also showed a need to support pre-service teachers’ ability to identify the main problem and supporting information. Moreover, the results showed a need to present chemistry tasks in more variable ways to PCTs and explain their reasoning other than testing field-specific, separated, memorized information. As the pool of identified novice vs. expert differences is already quite full, future research should focus on specific means for effective procedure transfer to students. As it includes many hidden processes, the combination of methods used in this study (eye-tracking, think-aloud, and interviews) are methods which will surely find their use.
Data availability statement
The raw data supporting the conclusions of this article will be made available by the authors, without undue reservation.
Ethics statement
Ethical review and approval was not required for the study on human participants in accordance with the local legislation and institutional requirements. Written informed consent for participation was not required for this study in accordance with the national legislation and the institutional requirements.
Author contributions
MR was responsible for the task selection and evaluation. MT was responsible for the eye-tracking data gathering and analysis. The results’ discussion, their implications as well as article writing was done in cooperation. Both authors contributed to the publication equally. Together, they shaped the study design.
Funding
This study was supported by the Charles University Grant Agency grant no. 492122. Analysis of the pre-service chemistry teachers’ process of solving tasks with problematic elements and the project Cooperation.
Conflict of interest
The authors declare that the research was conducted in the absence of any commercial or financial relationships that could be construed as a potential conflict of interest.
Publisher’s note
All claims expressed in this article are solely those of the authors and do not necessarily represent those of their affiliated organizations, or those of the publisher, the editors and the reviewers. Any product that may be evaluated in this article, or claim that may be made by its manufacturer, is not guaranteed or endorsed by the publisher.
Footnotes
- ^ Complex tasks are tasks consisting of several subtasks with a different focus connected by a unifying topic.
References
Akbasli, S., Sahin, M., and Yaykiran, Z. (2016). The Effect of Reading Comprehension on the Performance in Science and Mathematics. J. Educ. Pract. 7, 108–121.
Bakken, J., and Andersson-Bakken, E. (2021). The textbook task as a genre. J. Curric. Stud. 53, 729–748. doi: 10.1080/00220272.2021.1929499
Barba, R. H., and Rubba, P. A. (1992). A comparison of preservice and in-service earth and space science teachers’ general mental abilities, content knowledge, and problem-solving skills. J. Res. Sci. Teach. 29, 1021–1035. doi: 10.1002/tea.3660291003
Barham, A. I. (2020). Investigating the Development of Pre-Service Teachers’ Problem-Solving Strategies via Problem-Solving Mathematics Classes. Eur. J. Educ. Res. 9, 129–141.
Bellanca, J. A. (2010). 21st century skills: Rethinking how students learn. Bloomington: Solution Tree Press.
Ben-Zvi, N., and Genut, S. (1998). Uses and limitations of scientific models: The Periodic Table as an inductive tool. Int. J. Sci. Educ. 20, 351–360. doi: 10.1080/0950069980200307
Caleon, I., and Subramaniam, R. (2010). Development and application of a three-tier diagnostic test to assess secondary students’ understanding of waves. Int. J. Sci. Educ. 32, 939–961. doi: 10.1080/09500690902890130
Cheung, D. (2009). Using think-aloud protocols to investigate secondary school chemistry teachers’ misconceptions about chemical equilibrium. Chem. Educ. Res. Pract. 10, 97–108. doi: 10.1039/B908247F
De Wever, B., Hämäläinen, R., Nissinen, K., Mannonen, J., and Van Nieuwenhove, L. (2021). Teachers’ problem-solving skills in technology-rich environments: A call for workplace learning and opportunities to develop professionally, Teachers’ problem-solving skills in technology-rich environments: A call for workplace learning and opportunities to develop professionally*. Stud. Cont. Educ. 1–27. doi: 10.1080/0158037X.2021.2003769
Gilbert, J. K. (2005). “Visualization: A metacognitive skill in science and science education,” in Visualization in science education. Models and Modeling in Science Education, ed. J. K. Gilbert (Dordrecht: Springer), 9–27.
Gorur, R. (2016). Seeing like PISA: A cautionary tale about the performativity of international assessments. Eur. Educ. Res. J. 15, 598–616. doi: 10.1177/1474904116658299
Harsh, J. A., Campillo, M., Murray, C., Myers, C., Nguyen, J., and Maltese, A. V. (2019). “Seeing” Data Like an Expert: An Eye-Tracking Study Using Graphical Data Representations. CBE Life Sci. Educ. 18:ar32. doi: 10.1187/cbe.18-06-0102
Hartman, J. R., and Nelson, E. A. (2015). “Do we need to memorize that?” or cognitive science for chemists. Found. Chem. 17, 263–274. doi: 10.1007/s10698-015-9226-z
Holec, J., and Rusek, M. (2016). Metodické komentáøe a úlohy ke Standardùm pro základní vzdìlávání - chemie. Praha: NÚV.
Imam, O. A., Mastura, M. A., Jamil, H., and Ismail, Z. (2014). Reading comprehension skills and performance in science among high school students in the Philippines. Asia Pac. J. Educ. Educ. 29, 81–94.
Jian, Y.-C. (2018). Reading instructions influence cognitive processes of illustrated text reading not subject perception: An eye-tracking study. Front. Psychol. 9:2263. doi: 10.3389/fpsyg.2018.02263
Klein, P., Viiri, J., Mozaffari, S., Dengel, A., and Kuhn, J. (2018). Instruction-based clinical eye-tracking study on the visual interpretation of divergence: How do students look at vector field plots?. Phys. Rev. Phys. Educ. Res. 14:010116. doi: 10.1103/PhysRevPhysEducRes.14.010116
Kreiner, S., and Christensen, K. B. (2014). Analyses of model fit and robustness. A new look at the PISA scaling model underlying ranking of countries according to reading literacy. Psychometrika 79, 210–231. doi: 10.1007/s11336-013-9347-z
Krulik, S., and Rudnick, J. A. (1982). Teaching problem solving to preservice teachers. Arithmetic Teach. 29, 42–45. doi: 10.5951/AT.29.6.0042
Lai, M. L., Tsai, M. J., Yang, F. Y., Hsu, C. Y., Liu, T. C., Lee, S. W. Y., et al. (2013). A review of using eye-tracking technology in exploring learning from 2000 to 2012. Educ. Res. Rev. 10, 90–115. doi: 10.1016/j.edurev.2013.10.001
Lee, K. W. L., Tan, L. L., Goh, N. K., Chia, L. S., and Chin, C. (2000). Science teachers and problem solving in elementary schools in Singapore. Res. Sci. Technol. Educ. 18, 113–126. doi: 10.1080/713694953
Liampa, V., Malandrakis, G. N., Papadopoulou, P., and Pnevmatikos, D. (2019). Development and Evaluation of a Three-Tier Diagnostic Test to Assess Undergraduate Primary Teachers’ Understanding of Ecological Footprint. Res. Sci. Educ. 49, 711–736. doi: 10.1007/s11165-017-9643-1
Lindner, M. A., Eitel, A., Strobel, B., and Köller, O. (2017). Identifying processes underlying the multimedia effect in testing: An eye-movement analysis. Learn. Instruct. 47, 91–102. doi: 10.1016/j.learninstruc.2016.10.007
Medková, I. (2013). Dovednosti žákù ve výuce fyziky na základní škole [The Pupil’s Skills in the Physics Education at the Lower-secondary School]. Brno: Masarykova univerzita.
Milbourne, J., and Wiebe, E. (2018). The Role of Content Knowledge in Ill-Structured Problem Solving for High School Physics Students. Res. Sci. Educ. 48, 165–179. doi: 10.1007/s11165-016-9564-4
OECD (2016). Pisa 2015 Results (Volume 1): Excellence and Equity in Education. Paris: OECD Publishing.
Ogilvie, C. A. (2009). Changes in students’ problem-solving strategies in a course that includes context-rich, multifaceted problems. Phys. Rev. Special Top. Phys. Educ. Res. 5:020102. doi: 10.1103/PhysRevSTPER.5.020102
Osterhage, J. L. (2021). Persistent miscalibration for low and high achievers despite practice test feedback in an introductory biology course. J. Microbiol. Biol. Educ. 22, e00139–21. doi: 10.1128/jmbe.00139-21
Phelps, A. J. (1996). Teaching to enhance problem solving: It’s more than the numbers. J. Chem. Educ. 73:301. doi: 10.1021/ed073p301
Potužníková, E. Lokajíèková, V., and Janík, T. (2014). Mezinárodní srovnávací výzkumy školního vzdìlávání v Èeské republice: Zjištìní a výzvy. Pedagogická Orientace 24, 185–221. doi: 10.5817/PedOr2014-2-185
Richardson, J. T. (2011). Eta squared and partial eta squared as measures of effect size in educational research. Educ. Res. Rev. 6, 135–147.
Rodemer, M., Eckhard, J., Graulich, N., and Bernholt, S. (2020). Decoding Case Comparisons in Organic Chemistry: Eye-Tracking Students’. Visual Behavior. J. Chem. Educ. 97, 3530–3539. doi: 10.1021/acs.jchemed.0c00418
Rusek, M., Koreneková, K., and Tóthová, M. (2019). “How Much Do We Know about the Way Students Solve Problem-tasks,” in Project-based Education and Other Activating Strategies in Science Education XVI, eds M. Rusek and K. Vojíø (Prague: Charles University, Faculty of Education).
Rusek, M., and Tóthová, M. (2021). “Did students reach the periodic table related curricular objectives after leaving from lower-secondary education?,” in Project-Based Education and Other Activating Strategies in Science Education XVIII, eds M. Rusek, M. Tóthová, and K. Vojíø (Prague: Charles University, Faculty of Education).
Schnotz, W., Ludewig, U., Ullrich, M., Horz, H., McElvany, N., and Baumert, J. (2014). Strategy shifts during learning from texts and pictures. J. Educ. Psychol. 106, 974–989. doi: 10.1037/a0037054
Son, J. W., and Kim, O. K. (2015). Teachers’ selection and enactment of mathematical problems from textbooks. Math. Educ. Res. J. 27, 491–518. doi: 10.1007/s13394-015-0148-9
Štech, S. (2018). PISA–nástroj vzdìlávací politiky nebo výzkumná metoda? [PISA – An Instrument of Educational Policy or A research Method?]. Orbis Sch. 5, 123–133. doi: 10.14712/23363177.2018.81
Stenlund, T., Eklöf, H., and Lyrén, P. E. (2017). Group differences in test-taking behaviour: An example from a high-stakes testing program. Assess. Educ. 24, 4–20. doi: 10.1080/0969594X.2016.1142935
Straková, J. (2016). Mezinárodní výzkumy výsledkù vzdìlávání: Metodologie, pøínosy, rizika a pøíležitosti [International research on educational outcomes: Methodology, benefits, risks and opportunities]. Prague: Univerzita Karlova.
Tai, R. H., Loehr, J. F., and Brigham, F. J. (2006). An exploration of the use of eye-gaze tracking to study problem-solving on standardized science assessments. Int. J. Res. Method Educ. 29, 185–208. doi: 10.1080/17437270600891614
Talsma, K., Schüz, B., and Norris, K. (2019). Miscalibration of self-efficacy and academic performance: Self-efficacy? self-fulfilling prophecy. Learn. Individ. Differ. 69, 182–195. doi: 10.1016/j.lindif.2018.11.002
Topczewski, J. J., Topczewski, A. M., Tang, H., Kendhammer, L. K., and Pienta, N. J. (2017). NMR spectra through the eyes of a student: Eye tracking applied to NMR items. J. Chem. Educ. 94, 29–37. doi: 10.1021/acs.jchemed.6b00528
Tóthová, M., and Rusek, M. (2021b). Developing Students’ Problem-solving Skills Using Learning Tasks: An Action Research Project in Secondary School. Acta Chim. Slov. 68, 1016–1026. doi: 10.17344/acsi.2021.7082
Tóthová, M., and Rusek, M. (2021c). The Use of Eye-tracking in Science Textbook Analysis: A Literature Review. Sci. Educ. 12, 63–74. doi: 10.14712/18047106.1932
Tóthová, M., and Rusek, M. (2021a). “An analysis of pre-service chemistry teachers’ progress when solving multicomponent tasks,” in Project-Based Education and Other Activating Strategies in Science Education XVIII, eds M. Rusek, M. Tóthová, and K. Vojíø (Prague: Charles University, Faculty of Education).
Tóthová, M., Rusek, M., and Chytrý, V. (2021). Students’ Procedure When Solving Problem Tasks Based on the Periodic Table: An Eye-Tracking Study. J. Chem. Educ. 98, 1831–1840. doi: 10.1021/acs.jchemed.1c00167
Tsai, M. J., Hou, H. T., Lai, M. L., Liu, W. Y., and Yang, F. Y. (2012). Visual attention for solving multiple-choice science problem: An eye-tracking analysis. Comput. Educ. 58, 375–385. doi: 10.1016/j.compedu.2011.07.012
van der Graaf, J., Segers, E., and de Jong, T. (2020). Fostering integration of informational texts and virtual labs during inquiry-based learning. Contemp. Educ. Psychol. 62:101890. doi: 10.1016/j.cedpsych.2020.101890
Van Gog, T., Paas, F., Van Merriënboer, J. J., and Witte, P. (2005). Uncovering the problem-solving process: Cued retrospective reporting versus concurrent and retrospective reporting. J. Exp. Psychol. Appl. 11:237. doi: 10.1037/1076-898X.11.4.237
Van Someren, M., Barnard, Y., and Sandberg, J. (1994). The think aloud method: A practical approach to modelling cognitive. London: London Academic Press.
Vega Gil, L., Beltrán, J. C. H., and Redondo, E. G. (2016). PISA as a political tool in Spain: Assessment instrument, academic discourse and political reform. Eur. Educ. 48, 89–103. doi: 10.1080/10564934.2016.1185685
Vojíø, K., and Rusek, M. (2022). Opportunities for learning: Analysis of Czech lower-secondary chemistry textbook tasks. Acta Chim. Slov. 69, 359–370. doi: 10.17344/acsi.2021.7245
Wu, C. J., and Liu, C. Y. (2021). Eye-movement study of high-and low-prior-knowledge students’ scientific argumentations with multiple representations. Phys. Rev. Phys. Educ. Res. 17:010125. doi: 10.1103/PhysRevPhysEducRes.17.010125
Keywords: problem-solving skills, chemistry education, science education, eye-tracking, pre-service teachers
Citation: Tóthová M and Rusek M (2022) “Do you just have to know that?” Novice and experts’ procedure when solving science problem tasks. Front. Educ. 7:1051098. doi: 10.3389/feduc.2022.1051098
Received: 22 September 2022; Accepted: 25 October 2022;
Published: 16 November 2022.
Edited by:
Muhammet Usak, Kazan Federal University, RussiaReviewed by:
Milan Kubiatko, J. E. Purkyne University, CzechiaVincentas Lamanauskas, Vilnius University, Lithuania
Copyright © 2022 Tóthová and Rusek. This is an open-access article distributed under the terms of the Creative Commons Attribution License (CC BY). The use, distribution or reproduction in other forums is permitted, provided the original author(s) and the copyright owner(s) are credited and that the original publication in this journal is cited, in accordance with accepted academic practice. No use, distribution or reproduction is permitted which does not comply with these terms.
*Correspondence: Martin Rusek, bWFydGluLnJ1c2VrQHBlZGYuY3VuaS5jeg==