Positioning responsible learning analytics in the context of STEM identities of under-served students
- IPN-Leibniz Institute for Science and Mathematics Education, Kiel, Germany
Addressing 21st century challenges, professionals competent in science, technology, engineering, and mathematics (STEM) will be indispensable. A stronger individualisation of STEM learning environments is commonly considered a means to help more students develop the envisioned level of competence. However, research suggests that career aspirations are not only dependent on competence but also on STEM identity development. STEM identity development is relevant for all students, but particularly relevant for already under-served students. Focusing solely on the development of competence in the individualisation of STEM learning environments is not only harming the goal of educating enough professionals competent in STEM, but may also create further discrimination against those students already under-served in STEM education. One contemporary approach for individualisation of learning environments is learning analytics. Learning analytics are known to come with the threat of the reproduction of historically grown inequalities. In the research field, responsible learning analytics were introduced to navigate between potentials and threats. In this paper, we propose a theoretical framework that expands responsible learning analytics by the context of STEM identity development with a focus on under-served students. We discuss two major issues and deduce six suppositions aimed at guiding the use of as well as future research on the use of learning analytics in STEM education. Our work can inform political decision making on how to regulate learning analytics in STEM education to help providing a fair chance for the development of STEM identities for all students.
1. Introduction
Addressing the challenges of the 21st century, professionals competent in science, technology, engineering, and mathematics (STEM) will be indispensable (FEANI, 2021, pp. 7–8). A stronger individualisation of STEM learning environments is commonly considered a means to help more students develop the envisioned level of competence (National Academies of Sciences, Engineering, and Medicine, 2019). However, research suggests that even the most competent students may not aspire to a STEM career (Taskinen et al., 2013). One reason is that STEM career aspirations do not only depend on students’ STEM competence but also on students’ developing a STEM identity (Dou et al., 2019, p. 623). Carlone and Johnson (2007) accordingly identify, in addition to the dimension of competence, two more dimensions relevant to the development of a STEM identity: recognition and performance. As we will show, these additional dimensions are specifically relevant to under-served students who face historically grown inequalities due to two mechanisms of discrimination, vulnerability and iterability (Butler, 1990, 2005; Hartmann and Schriever, 2022). Vulnerability is the mechanism that describes that the same situation can have different effects for diverse students. Iterability describes the setting of norms through repetition. The development of a STEM identity is not just a question of preparing enough professionals sufficiently competent in STEM but also of justice and power (see also UN-SDG-Goal 4, 2015). STEM education researchers Waight et al. (2022, p. 19) even advocate for centering equitable perspectives in science education overall. We therefore argue that focusing solely on the development of competence in the individualisation of STEM learning environments is not only harming the goal of educating enough professionals competent in STEM, but may also create further discrimination against those students already under-served in STEM education.
One contemporary approach for individualisation of learning environments is learning analytics. Learning analytics is referring to the collection, analysis and reporting of data about learning and the environment in which learning occurs with the purpose to understand and optimise learning and the learning environment (Society for Learning Analytics Research (SoLAR), 2022). One example for the use of learning analytics is the provision of feedbacks for teachers on the competence development of students. Learning analytics often draw on machine learning techniques; that is, algorithms trained on existing data to monitor students’ competence development. However, existing data often reflect historically grown inequalities. Training algorithms with existing data will then lead to the reproduction and, worse, reinforcement of these inequalities. That is, learning analytics would under-serve precisely those students again who already face historically grown inequalities. In order to navigate between the potentials and threats in the field of learning analytics, the concept “responsible learning analytics” has been introduced (Prinsloo and Slade, 2018; Cerratto Pargman et al., 2021). To date, responsible learning analytics are focused on competence development in learning environments, neglecting the relevance of the other dimensions of STEM identity development. However, STEM learning environments often are opportunities for the development of STEM identity as well and multiple historically grown equalities exist with regard to identity development in STEM (Mujtaba and Reiss, 2013; Rosa and Moore Mensah, 2016; Avraamidou, 2019). As a result, it seems imperative that the concept of responsible learning analytics must be expanded to include STEM identity development as one important aim of individualising STEM learning environments.
In this paper, we propose a theoretical framework that positions responsible learning analytics in the context of STEM identity development, especially of under-served students. We identify two major issues of responsible learning analytics in the context of under-served students’ STEM identity development and derive suppositions to guide future work in this area. Our suppositions are meant to highlight the need for making normative decisions. In doing so, we intend to make normative decisions visible and debatable. The suppositions can guide the use of learning analytics and future research on the use of learning analytics. Our work can inform political decision making on how to regulate learning analytics in STEM education to help providing a fair chance for the development of STEM identities for all students, particularly students from under-served groups. In summary, our paper adds:
• A theoretical framework that positions responsible learning analytics in the context of STEM identities of under-served students,
• A discussion of two major issues that come with learning analytics in the context of STEM identity development, and
• Six suppositions aimed at guiding the use of as well as future research on the use of learning analytics in STEM education.
2. STEM identities of under-served students
The concept of identity has been introduced to the field of STEM research with the purpose to help understand why students engage in STEM, how some students are promoted whereas others are marginalized by current STEM education practice and hence a means to work towards more equitable STEM education (Carlone and Johnson, 2007).
2.1. STEM identities
Identities are complex constructs through which we bring our experiences and our reflective projections together, define who we are and what influences our learning (Brickhouse, 2001). Identities are shaped in social interaction and new identities need to be negotiated with regard to existing identities which can lead to conflicts (Brown, 2004, p. 811). STEM identities lead to higher career aspirations through “goal setting and behavior” (Dou et al., 2019, p. 632). For STEM identity, various frameworks exist. For our work, we were looking for an understanding of STEM identity that includes clearly operationalized dimensions and decided for the framework proposed by Carlone and Johnson (2007). According to Carlone and Johnson (2007), STEM identity can be understood by its three dimensions: recognition, performance, and competence. Comparable frameworks propose interest as another dimension of STEM identity (Godwin, 2016; Hazari et al., 2020; Mahadeo et al., 2020). While we will argue in the following that learning analytics impact recognition, performance, and competence, we see interest rather impacted by the design of learning opportunities and not by learning analytics.
STEM identity development can create conflicts that emerge from identity negotiations. At the same time, STEM identity development for all students is important. Identity negotiations as well as the role of identities for learning make STEM identity development a relevant context for learning analytics in STEM learning environments. These three dimensions recognition, performance, and competence are a relevant context for learning analytics.
Competence is understood as the empirically testable knowledge, skills, and abilities in a particular domain as well as what an individual says about oneself with regard to the own competence (Carlone and Johnson, 2007, p. 1992).
Performance is what an individual does through concrete actions. The performance definition differs from what often is understood as performance in science education. Performance as understood by Carlone and Johnson can be based on competence but does not have to be. For example, a person might perform STEM identity by communicating with adequate scientific language in a specific task without a profound understanding of the concepts and thus competence. The repetitive performance of STEM identity can “become patterned and habitual” (Carlone and Johnson, 2007, pp. 1190–1,192). A development opportunity for STEM identity can include or exclude students with different performances of their existing identities, for example their gender or social class performance.
Recognition is considered particularly relevant for the development of STEM identity. While competence and performance are components of STEM identity, STEM identity development is dependent on the recognition of “meaningful others” (Carlone and Johnson, 2007, p. 1992). Meaningful others are persons whose acceptance matters in the context of STEM, for example STEM teachers. In order for STEM identity to become habitual, recognition is a key. This holds particularly true for students who face historically grown inequalities (Carlone and Johnson, 2007, pp. 1887–1992).
2.2. Under-served students
STEM identity development is particularly relevant for under-served students who face historically grown inequalities. In order to define who we refer to as under-served students and which inequalities we focus on, we take orientation but do not limit ourselves to so-called protected categories, for example gender or race. Protected categories are identity markers based on which a person should not be discriminated against. There are various words to describe students who face inequalities due to an identity in a protected category but we specifically choose the term “under-served.” From our perspective, the term under-served offers two important features: the normative direction and the allocation of responsibility.
The normative direction points at the students not being served enough, there should be more opportunity and offer. As an example, the OECD (2018) states “that differences in students’ outcomes are unrelated to their background” (p. 13). In this example, the OECD explicitly names the categories socio-economic status, gender, or immigrant and family background. In addition, the OECD offers an analysis of the reality: “There is no country in the world that can yet claim to have entirely eliminated socio-economic inequalities in education” (OECD, 2018, p. 13). The above mentioned categories make up the analysis lens through which reality is analysed. The analysis results in a normative demand: For the named categories, eliminate the relation to students’ outcomes. Under-served students need to be provided with opportunities to reach better outcomes in the future.
Under-served allocates the responsibility for the change of reality not with the students but with the society and its institutions. It is not the students’ fault or responsibility that reality (does not) change. The society with its institutions are responsible for not providing enough or adequate opportunities for change.
Many students are under-served in terms of these opportunities. As an example for gender in Germany, women are currently opting less for a STEM career (Düchs and Ingold, 2018). As STEM identity is important for career aspirations, female students need to get more and better fitting opportunities for their STEM identity development. Another example is racism that has been found to limit STEM identity development (Avraamidou, 2019): As long as students’ racial identities are related to their STEM identities, STEM identity development opportunities for students facing racism need to be strengthened. Under-servements and historically grown inequalities are well-documented, see for example (Brown, 2004; Carlone and Johnson, 2007; Rosa and Moore Mensah, 2016; OECD, 2018; Bachsleitner et al., 2022).
2.3. Intersectionality and individual needs of students
Students can be under-served from the perspective of diverse protected categories, for example gender and race. What if students are under-served from multiple perspectives at the same time, for example gender and race? For students who are under-served from multiple category perspectives, the under-serving is not only the sum of the under-servings for each category. Multiple categories at the same time can lead to additional, distinct under-servings (Costanza-Chock, 2020, p. 17). A Black female student can experience under-servings that go beyond what a white female and a Black male student experience. Black feminist scholar Crenshaw (1989) gave a name to this phenomenon, intersectionality. Intersectionality is not limited to the categories gender and race but holds true for more combinations of categories as well. In order to provide opportunities for the STEM identity development for all students, diverse needs have to be considered. All students have individual needs. However, the needs of under-served students are particularly relevant due to two mechanisms: vulnerability and iterability.
The first mechanism is based on the dependency of humans on recognition of others (Butler, 2005). This dependency is a core need of every human being, a need for recognition (Hartmann and Schriever, 2022, pp. 95–96). Additionally, being recognised is important for students’ STEM identity development (Carlone and Johnson, 2007). However, Hartmann and Schriever emphasize that the recognition humans receive differs heavily. For students, receiving limited recognition can hinder their STEM identity development. There is no certain, quantifiable amount of recognition that students need in order to develop a STEM identity. Nonetheless, having received few recognition in the past makes students more dependent on future recognition. This particular dependency on future recognition of under-served students is called vulnerability.
The second mechanism is based on the norms that are established through repetition (Butler, 1990; Hartmann and Schriever, 2022, p. 95). For example, by 2021 214 out of 218 nobel prices in physics were given to men. If in physics learning environments successful physicists are men again and again, the combination of physicist and man is established as a norm. The iteration of the combined performance of a STEM identity and a male gender identity leads to the norm: Physicists are men. What does this mean for STEM identity development of under-served students? Whether under-served students start performing their STEM identity depends on whether they perceive this new identity performance as fitting to their performances or not (Taconis and Kessels, 2009). Whether the performances fit or not is a norm. This norm can be exclusive by re-iterating existing inequalities. The norm can also be inclusive by serving currently under-served students with STEM identity performances that fit to their performances. The establishment of norms through iteration of performances over and over again is called iterability.
2.4. Summary: STEM identities of under-served students
STEM identities of under-served students need a special focus when analysing development opportunities for STEM identity. We summarise our model of STEM identities of under-served students in Figure 1.
STEM identities consist of the three dimensions of recognition, performance, and competence. The needs of under-served students in terms of their development opportunities for STEM identities are very individual and diverse. The vulnerability of under-served students as well as the iterability of their identity performances and whether they (do not) fit in the context of STEM identity moderate the effect of STEM identity development opportunities on the dimensions recognition and performance. In terms of STEM identity development, under-served students have individual needs that often are failed to be addressed.
3. Responsible learning analytics
To improve students’ competence development, learning environments are individualised (Zhai et al., 2019, p. 1451). One approach to answer individualisation demands are learning analytics. Next to students’ needs for individualisation for competence development, students also have individual needs in terms of STEM identity development. We start by discussing learning analytics in the context of competence development. Building on this discussion, we later position learning analytics in the context of STEM identity development.
3.1. Learning analytics
Learning analytics are “the measurement, collection, analysis and reporting of data about learners and their contexts, for purposes of understanding and optimising learning and the environments in which it occurs” (Society for Learning Analytics Research (SoLAR), 2022). For example with regard to competence development, students’ results on tests can be used for competence diagnosis. Applications of learning analytics are, for example, the provision of individualised and real-time feedback or the empirical analysis of the quality of learning and teaching practices. Learning analytics allow tracking learning trajectories on a task-level and not only through tests before and after learning opportunities. In the Handbook of Learning Analytics, computational analysis techniques and digital data sources are described as “analytics of (1) network structures including actor–actor (social) networks but also actor–artefact networks, (2) processes using methods of sequence analysis, and (3) content using text mining or other techniques of computational artefact analysis” (Hoppe, 2017, p. 23). Learning analytics are most often combined with a focus on institutional strategies and systems perspectives. The learning analytics community has managed to build a huge corpus on equitable perspectives, for example asking questions of power (Wise et al., 2021), questions of geographical coverage around the world (Prinsloo and Kaliisa, 2022), and explicitly demanding for equity-focused research and praxis (Cerratto Pargman and McGrath, 2021).
3.2. Learning analytics and responsibility
While learning analytics have great potential for many educational disciplines, they also bring threats that cannot be ignored. These threats are categorized into the under-, over- or miss-use of learning analytics (Floridi et al., 2018, p. 690). Under-use relates to the failure to fully utilise the potential of learning analytics. Over-use is the application of learning analytics in cases where the outcomes do not justify the effort to put learning analytics in place. Miss-use is the application of learning analytics systems in cases that the system were not made and tested for. The miss-use can, for example, lead to feedbacks that miss-guide decision making. Over- and miss-use are more critical threats than under-use, as they can lead to undesirable outcomes (Kitto and Knight, 2019). In application of learning analytics, the potentials as well as the threats need to be addressed.
In the field of responsible learning analytics, potentials and threats are addressed by a combination of rules and principles to guide the use of learning analytics (Cerratto Pargman et al., 2021, p. 2). Possible potentials are found in a principle that is called the obligation to act − the responsibility to unfold the potentials of learning analytics. The obligation to act addresses the threat of under-use as well as it demands for learning analytics wherever learning analytics may be beneficial. In the context of competence development, learning analytics that lead to higher student outcomes should be used.
Possible threats are addressed by the principle of accountability (Prinsloo and Slade, 2018, p. 3). Staying with the example of competence development, the principle of accountability allocates the responsibility for the threats with the same persons that are responsible for improving the students learning outcomes through the use of learning analytics. Threats in responsible learning analytics in our understanding are also rooted in critical theory which explicitly addresses questions of power and justice (Prinsloo and Slade, 2018, p. 4). This is well in line with the subversive stance on learning analytics as proposed by Wise et al. (2021) as a way of engaging with issues of equity and their interaction with data on learning processes. One example of a threat where issues of equity are relevant is proxy-discrimination (Erden, 2020, p. 85). Proxy-discrimination is the discrimination of a person due to a category that itself is not protected, but related to a protected category. In the example of Erden from the US, an algorithm predicted the duration of staff membership with the category travelling distance to work. Travelling distance itself is not a protected category. However, travelling distance was strongly correlated with race which is a protected category. A responsible implementation of algorithms does not only need to ensure that protected categories are not used for prediction. An analysis of correlations of predictions with protected categories is important as well.
In order to address the potentials and threats, the responsible learning analytics has come up with various general recommendations as well as concrete methods. As general recommendations, there exist for example checklists to address privacy issues (Drachsler and Greller, 2016), policies (Slade, 2016), and principles (Floridi et al., 2018; Phillips et al., 2020). For concrete methods, there exist pre-processing, post-hoc, and direct methods with regard to equity issues (Lohaus et al., 2020). However, the impact of both general recommendations as well as concrete methods for learning analytics practice has been found to be small so far (Kitto and Knight, 2019). Equity issues have been particularly highlighted as a research need in the scientific discourse responsible learning analytics (Cerratto Pargman and McGrath, 2021).
3.3. Summary: Responsible learning analytics
In summary, responsible learning analytics acknowledge that learning analytics come with (1) potentials that lead to an obligation to act, and (2) threats that are addressed through accountability. We summarise our understanding of responsible learning analytics in Figure 2.
Responsible learning analytics can be understood as navigating between the obligation to act in terms of potentials and accountability for the threats.
4. Responsible learning analytics in the context of STEM identities of under-served students
4.1. Proposal of a theoretical framework
Learning analytics are implemented in learning environments to improve competence development. The learning environments are not only competence development opportunities, but also STEM identity development opportunities. Today, historically grown inequalities in STEM identity development exist and some students are under-served in terms of development opportunities in learning environments. Introducing learning analytics in precisely these learning environments adds the known threat of reproducing inequalities through learning analytics systems. In order to be able to address the threat of strengthening inequalities instead of countering them, we propose a theoretical framework that positions responsible learning analytics from Figure 2 in the context of STEM identity development of under-served students from Figure 1.
Responsible learning analytics’ potentials and threats effect development opportunities for STEM identity. We do not see learning analytics as development opportunities themselves because we acknowledge STEM identity as a fairly stable construct. Learning analytics can also be directed at supporting learning on small time scales such as within an instructional task or across a sequence of tasks. STEM development opportunities, however, unfold their effects on the larger scale such as lessons or lesson sets. Learning analytics can support or hinder STEM identity development through a given development opportunity. One example of a development opportunity is a classroom setting and recognition of students through the teacher. Learning analytics can provide competence diagnosis that can trigger teachers to recognise the student in front of other students or even the full class. If learning analytics come with a bias against under-served students, under-served students receive less recognition.
Defining bias in this context is to a huge degree normative. For example, Suresh and Guttag (2021) define an aggregation bias. An aggregation bias is found when a model is trained on full data sets while sub-groups would have needed separate models in order to obtain accurate results. Aggregation bias opens up questions like these: For which sub-groups would the algorithm need to function accurately and be tested? For students from all gender groups? For students from all socio-economic backgrounds? Also intersections of these sub-groups? In order to answer these questions, normative decisions need to be made for all bias analyses. In order to make these normative decisions visible and debatable, we highlight two issues in detail before we deduce suppositions from the theoretical framework.
4.2. Issues with normativity: Bias and equity
For bias, many differing definitions exist. Each definition comes with its normative implications. For example, in all papers it is agreed upon that biases should be avoided. We highlight two issues with normativity, bias and equity. We start with our understanding of bias by discussing existing definitions in the research field and positioning ourselves relative to these definitions.
Bias addresses the threats of learning analytics. Traag and Schmeling (2022) understand bias as a “direct causal effect that is unjustified” (p. 1). Our understanding of bias differs from this understanding, as for us the question of direct causality is not in focus. A correlation or an indirect causal effect are biases. This argumentation is also in line with the argumentation of the OECD that students’ outcomes should be unrelated to their backgrounds (OECD, 2018, p. 13).
Suresh and Guttag (2021) define one form of bias that is particularly relevant in the context of STEM identity development, historical bias: Historical bias is the result of the perfect reproduction of a world with existing inequalities and thereby the reproduction of the inequalities (p. 4). In the world as it is, not all persons with diverse gender, race, and class are equally represented. Training algorithms with data from the world as it is without adjusting for equity can result in a historical bias. Mitchell et al. (2021) highlight the fact that this understanding of what they call a societal bias is non-statistical (p. 146). We follow this argumentation and stress that the statistically accurate representation of the world as it is in the context of STEM identity is a bias. At the same time, statistically non-accurate representations of the world can be bias-free – if the non-accuracy is due to adjustments in order to strengthen equity.
Baker and Hawn (2021) identify representation bias, aggregation bias, and testing bias (Baker and Hawn, 2021, p. 9). To give testing bias as an example, missing evaluation for sub-group accuracy can lead to good accuracy for the whole group while the accuracy is very good for men but only partly good for women and non-binary persons. From our point of view, it is usually not feasible to address all potential sources of bias. What matters is to be explicit about which sources are considered and which are not. This leads to an understanding of bias as a limited construct bound to the particular analysis focus. Algorithms that are analysed for the most relevant biases can come with other biases, but have been subject to extensive analyses to reduce bias to the absolute minimum. Which analyses have been done and need to be done is a normative question. Being explicit on the analyses (not) made can help to inform the normative decision making processes.
Equity, in contrast to bias, addresses next to the threats also the potentials of learning analytics. Equity allows to address the demand formulated by the OECD (2018) to strive towards no relation between students’ learning outcomes and protected categories. Equity allows as well to address the idea formulated in the United Nations (2015) goal to provide education for all students.
The normative issue of equity leads us to ask: How do we want career aspirations to be distributed if not in the way they are distributed today? (Costanza-Chock, 2020, p. 63). We need to specify the protected categories we analyse for and select a distribution that we want. On a macro level, these specifications are the normative direction that the OECD or the United Nations provide. On a micro level, these specifications need to be translated in concrete thresholds in algorithmic training. For example, does an algorithm need work for all female and non-binary students at least as good as for male students? Is it possibly okay to violate this strict rule if the overall accuracy gets a lot better while the accuracy for female and non-binary students only lowers a little bit? (Lohaus et al., 2020). If a small violation of the strict rule is fine, do the designers of learning environments need to make up for this violation at another point in the learning environments through counter-measures?
Our point about the normative issue of equity is not to provide an answer to the aforementioned questions. Our point is to make the decisions on these questions visible and debatable. Without clear normative guidance, the learning analytics can reproduce existing inequalities in terms of STEM identity development. The reproduction of inequalities for STEM identity development can lead to different career aspirations in students. However, these different career aspirations in students are undesired by the OECD and the United Nations and should be prevented. At the same time, learning analytics can be designed with a clear normative direction and intervene in a world with existing inequalities towards more equity. Addressing equity in learning analytics allows to consider potentials and to address cultural change as well as to understand diversity as a value.
4.3. Suppositions
Based on our theoretical framework on responsible learning analytics in the context of STEM identity development of under-served students from Figure 3 and the two issues with normativity, we deduce suppositions for all potentials and threats.
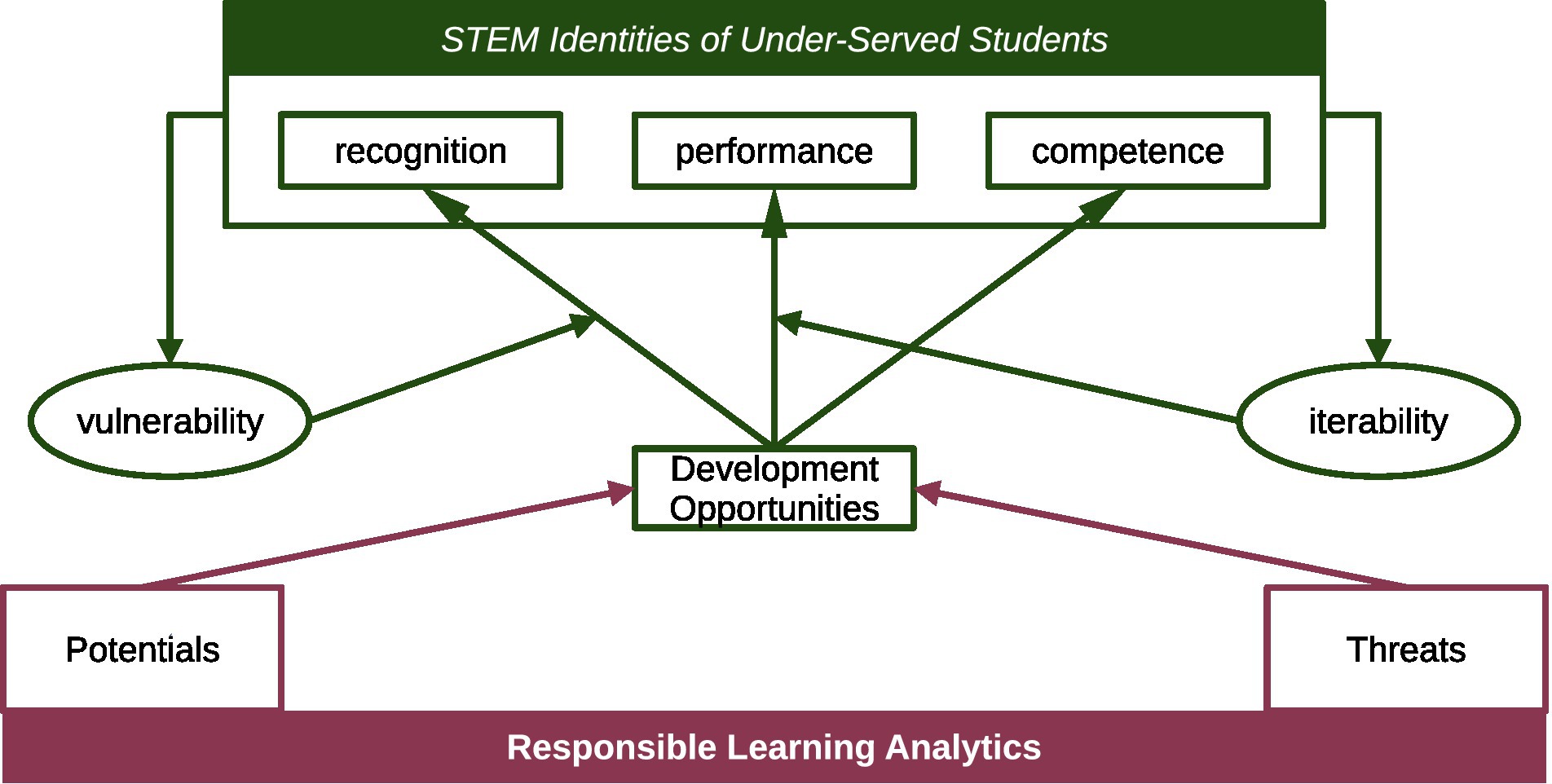
Figure 3. Responsible learning analytics in the context of STEM identities of under-served students.
4.3.1. Bias and learning analytics
Without explicit analyses and careful choice of training data sets, algorithms reproduce existing inequalities as biased algorithms. We analyse the threats of biased algorithms in learning analytics for STEM identity development in its dimensions competence, performance, and recognition.
Bias and competence: Biased algorithms can hinder STEM identity development for under-served students by under-serving them again in terms of competence feedback. Any competence feedback contributes to students’ perception of their own competence. An algorithm that provides individualised feedback to students based on what the students write in a task can be trained, for example, with one data set of many student answers to the specific task. If that data set does not represent all students equally well, the choice of this particular data set can lead to the algorithm being biased against those students that are not represented well. When biased algorithms feedback low competence development, this can lead to the student perceiving the own competence in STEM as low. Students’ perception of their own competence in STEM is an important piece in building their STEM identity. Therefore, biased competence feedbacks in learning analytics are a threat in terms of STEM identity development.
Bias and performance: Biased algorithms can hinder STEM identity development for under-served students by under-serving them again in terms of performance fitting. This under-serving can further strengthen exclusive norms by iterating them again. For example, STEM can be conceived as a male field in a class already due to many famous male scientists like Isaac Newton or Albert Einstein. If in that situation biased algorithms lead to performance feedback on class level with the male students being more competent than non-binary and female students, STEM as a male field can be further strengthened. In the next STEM identity development opportunity, non-binary and female students with interest in STEM might be questioned in their gender identities. The students would then need to negotiate between their gender and STEM identity which ultimately is another hindrance on the way toward strong STEM identities for these students. This is an example for algorithms that are biased in terms of gender. Other categories as well as their intersections need to be taken into account as well.
Bias and recognition: Biased algorithms can hinder STEM identity development for under-served students by under-serving them again in terms of recognition. This under-serving can further disadvantage precisely the students that are most vulnerable. If, for example, a biased algorithm positions a student with a high competence development through miss-classification under low competence development and feeds this classification back to the class as a ranking, the student is not recognised for the high competence development by other students. This effect can also be mediated through a teacher. A learning analytics system that provides feedback to the teacher can trigger the teacher to confront a student in a group with the low competence development classification and thus fail to appreciate a high competence development in a group setting.
4.3.2. Equity and learning analytics
Instead of focusing on not introducing new biases, equity allows countering historically grown inequalities. Algorithms that lead to stronger recognition for Black students can strengthen equity if this is done in order to make up for missing recognition of precisely these students in other settings. For this redistributive action, an analysis of the reality and the current under-servings in terms of STEM identity development is necessary. To stay with the example from biases, data sets for training of algorithms can have minimum shares for female and non-binary students if female and non-binary students face historically grown inequalities in the application field. We do not provide an answer to which redistributive action is adequate for which form of discrimination. Nevertheless, we analyse the potentials of equity in learning analytics for STEM identity development in terms of competence, performance, and recognition. Through this analysis, we aim at making the decisions on distributions visible and debatable.
Equity and competence: Responsible learning analytics can support STEM identity development for under-served students by serving them in terms of competence feedback. With the perception of the own competence being important for STEM identity development, this competence feedback can be key to STEM identity development. This holds especially true for contexts in which teachers themselves are biased and stereotyping a lot. Algorithms designed with equity in mind can be an impactful counter-measure here.
Equity and performance: Responsible learning analytics can support STEM identity development for under-served students by serving them in terms of performance fitting. This serving can counter existing disadvantages for under-served students by iterating performances that challenge exclusive norms. Staying with the example from the threats, iterating over and over non-binary and female STEM performances can counter the iteration of male STEM performances through famous male scientists. Finally, this can lead to STEM identity being perceived as inclusive and compatible with all gender identities.
Equity and recognition: Responsible learning analytics can support STEM identity development for under-served students by serving them in terms of recognition. This serving can counter existing disadvantages for under-served students by educating all students to be sensitive to varying levels of vulnerability. With the example of recognition, we propose how equity in learning analytics be implemented: Providing recognition to under-served students can be used by comparing it to historically grown inequalities in terms of recognition, for example the strong gender bias in noble price winners in physics. Making multiple levels of vulnerability visible and explicit in a moment of strong perceived recognition of precisely this vulnerable group of students can lead to them building resilience. In future recognition settings that might be biased, students’ resilience can lead to stronger self-confidence of these students as they are aware of different forms of historically grown inequalities and biases.
We do not understand the two issues as the only ones or the most adequate. The decision of which issues are the most important is a political one that cannot be answered by researchers. Instead, we find these issues impactful in terms of STEM identities of under-served students and aim at making normativity explicit and thereby debatable. There are more issues that can be thought of and that would need to be explicitly formulated in order to derive suppositions for future research from them.
4.3.3. Summary: Suppositions
Responsible learning analytics come with both, potentials and threats, for STEM identity development of under-served students. We position responsible learning analytics in the context of STEM identity development and the dimensions recognition, performance, and competence. In Table 1, we present a summary of the suppositions that we identified in the previous sections. The suppositions can give direction to future research.
5. Discussion
We proposed a theoretical framework, two issues with normativity, and six suppositions. What do these results mean for STEM identity development of under-served students? How can the results inform a process of transformation of the reality in which the OECD (2018) conclude that no country “can yet claim to have entirely eliminated […] inequalities in education” (p. 13)? In our discussion, we focus on implications for the research field of responsible learning analytics and its researchers on the one hand. On the other hand, we discuss the role of teachers when interacting with responsible learning analytics systems.
5.1. Implications for responsible learning analytics researchers
In learning analytics, many researchers demand for more equity perspectives. For example, Wise et al. (2021) propose a subversive stance on learning analytics. With subversive stance they mean to engage in questions of power and equity. Prinsloo and Slade (2018) advocate for rooting responsible learning analytics in critical theory. So far, there exist various principles on how to enact equity in practice that have been thoroughly reviewed (Sclater, 2014; Prinsloo and Slade, 2018; Cerratto Pargman and McGrath, 2021). However, Kitto and Knight (2019) find that the existing principles often have little impact in practice as they are under-specified. These experiences with principles can support further work on responsible learning analytics in the context of STEM identity development.
In order to make decisions on how to regulate learning analytics in the context of STEM identity development, concrete examples in STEM disciplines are necessary. These concrete examples can then inform political decision making on how to guide and regulate learning analytics practice. Kitto and Knight (2019) propose to create a database with concrete examples on how to navigate between threats and potentials and where guidance is missing. The report on this navigation can be informed by explicit normative decisions as formulated here and point to where these decisions fail to provide clear guidance so far.
For bias analyses, the context of STEM identity development can inform which values to look at and which analyses to make. For example, a purely competence-oriented perspective can lead to other conclusions than a perspective that includes STEM identity development. From a competence perspective, it makes more sense to ensure that learning analytics algorithms identify all students that have not yet understood a concept. A false positive is dangerous because a teacher would assume the student has understood the concept and the teacher would not intervene. A false negative would make the teacher approach the student, notice that the student actually understood the concept and continue with the classes. A STEM identity perspective also makes us ask: How is the sub-group accuracy for different categories of the positive scores? The positive scores can lead to teachers recognising students or not. Recognition is an important dimension of STEM identity, particularly for under-served students. A STEM identity perspective demands for sub-group accuracy in the positive scores and puts a new perspective on which bias analyses need to be made.
Responsible learning analytics researchers should consider the context of STEM identity development when deciding on which bias analyses to make. According to Avraamidou (2020), a special focus on recognition and the emotions linked to recognition processes seems advisable since those two dimensions seem especially important for under-served students.
5.2. Connections to critical consciousness of teachers
Next, to learning analytics systems themselves, we want to point at the importance of teachers and their interactions with learning analytics systems. From our point of view, learning analytics can support but do not replace teachers in schools. In reaching equity, teachers play a key role.
One human-centered approach to transform societies with existing inequalities in more equitable societies is critical consciousness. Critical consciousness can be understood as “reflection and action upon the world in order to transform it” (Freire, 1970, p. 51). In the context of education and for teachers, Baggett (2020) understands critical consciousness as a person’s understanding of relevance of and responsibility for categories, for example gender and race, in combination with acting accordingly.
It is crucial to understand how teachers make use of learning analytics in schools in a way that strengthens equity. We need to know what makes teachers critically reflect on the feedback learning analytics systems provide. What do teachers need in order to enact equity in learning analytics for STEM identity development of all students, particularly under-served students? Teachers are key actors in many STEM identity development opportunities and therefore need to be considered in STEM identity development contexts.
5.3. Conclusions for STEM identity development of under-served students
In order to reach the Sustainable Development Goal 4 on quality education and upward socio-economic mobility, the issues of bias and equity in learning analytics need to be addressed in the context of STEM identity development. Normative decisions need to be made explicit and visible by researchers and designers in order to make their implications debatable. Based on the suppositions we deduced from the theoretical framework, hypotheses need to be formulated as well as empirically tested. These hypotheses need to be as concrete as possible in order to lead to principles for practice that are not under-specified but concrete enough to guide practice. These principles can ultimately lead to STEM identity development opportunities for all students and equally distributed STEM career aspirations.
In addition, under-served students themselves play a crucial role in strengthening equity in practice. To transform societies and to truly change existing inequalities, we need to centre the voices and needs of under-served students and to empower them to be part of this transformation. If we fail to do so, we run into the danger of reproducing exclusion and inequalities. We need to enable students to understand how learning analytics in schools work to create equity through learning analytics. Identifying potential biases in learning analytics might help under-served students to re-evaluate their own scores and to realise that deficits might be rooted in the learning analytics tools and not in their own competencies. This could be a powerful way to empower them to claim potential biases. By doing so, under-served students can be enabled to recognise their own potentials and to construct their own STEM identity based on those potentials. This is what Shanahan (2009) calls agency in terms of STEM identity development.
In this paper, we proposed a theoretical framework that positions responsible learning analytics in the context of STEM identities of under-served students. We discussed two major issues and deduced suppositions that aim at guiding the use of as well as future research on the use of learning analytics in STEM education. With our conclusions for researchers, teachers, and under-served students as well as with our work as a whole we aim at informing political decision making in order to provide STEM identity development opportunities for all students.
Data availability statement
The original contributions presented in the study are included in the article/supplementary material, further inquiries can be directed to the corresponding author.
Author contributions
AG, AS, JÇ, MK, and KN contributed to the conception and design of the study and the theoretical framework and main results were created in collective work and discussions with all authors. AG contributed the main work in drafting the sections one to five. AG and JÇ contributed the main work in drafting the section six. All authors contributed to the article and approved the submitted version.
Funding
This work was supported by the Federal Ministry of Education and Research (BMBF). BMBF project is: 01JD2008.
Acknowledgments
Our work lays on the shoulders of various great thinkers who do not yet receive the visibility they deserve in the context of STEM education from our perspective. We want to highlight especially the work from Black feminist author Kimberlé Crenshaw on intersectionality (1989), the work from nonbinary trans* femme author Sasha Costanza-Chock on design justice (2020), the author from the Global South Paulo Freire on critical consciousness (1970), and Judith Butler with contributions to queer theory (Butler, 1990, 2005).
Conflict of interest
The authors declare that the research was conducted in the absence of any commercial or financial relationships that could be construed as a potential conflict of interest.
Publisher’s note
All claims expressed in this article are solely those of the authors and do not necessarily represent those of their affiliated organizations, or those of the publisher, the editors and the reviewers. Any product that may be evaluated in this article, or claim that may be made by its manufacturer, is not guaranteed or endorsed by the publisher.
References
Avraamidou, L. (2019). “I am a young immigrant woman doing physics and on top of that I am Muslim”: identities, intersections, and negotiations. J. Res. Sci. Teach. 57, 311–341. doi: 10.1002/tea.21593
Avraamidou, L. (2020). Science identity as a landscape of becoming: rethinking recognition and emotions through an intersectionality lens. Cult. Stud. Sci. Educ. 15, 323–345. doi: 10.1007/s11422-019-09954-7
Bachsleitner, A., Lämmchen, R., and Maaz, K. (Eds.) (2022). Soziale Ungleichheit des Bildungserwerbs von der Vorschule bis zur Hochschule: Eine Forschungssynthese zwei Jahrzehnte nach PISA. Münster: Waxmann.
Baggett, H. C. (2020). Relevance, representation, and responsibility: exploring world language teachers’ critical consciousness and pedagogies. L2 J. 12, 34–54. doi: 10.5070/L212246037
Baker, R., and Hawn, A. (2021). Algorithmic bias in education. Int. J. Artif. Intell. Educ. 32, 1052–1092. doi: 10.1007/s40593-021-00285-9
Brickhouse, N. W. (2001). Embodying science: A feminist perspective on learning. J. Res. Sci. Teach. 38, 282–295. doi: 10.1002/1098-2736(200103)38:3%3C282::AID-TEA1006%3E3.0.CO;2-0
Brown, B. A. (2004). Discursive identity: assimilation into the culture of Scienceand its implications for minority students. J. Res. Sci. Teach. 41, 810–834. doi: 10.1002/tea.20228
Carlone, H. B., and Johnson, A. (2007). Understanding the science experiences of successful women of color: science identity as an analytic lens. J. Res. Sci. Teach. 44, 1187–1218. doi: 10.1002/tea.20237
Cerratto Pargman, T., and McGrath, C. (2021). Mapping the ethics of learning analytics in higher education: A systematic literature review of empirical research. J. Learn. Anal. 8, 123–139. doi: 10.18608/jla.2021.1
Cerratto Pargman, T., McGrath, C., Viberg, O., Kitto, K., Knight, S., and Ferguson, R. (2021). Responsible Learning Analytics: Creating Just, Ethical, and Caring LA Systems Companion Proceedings LAK21.
Costanza-Chock, S. (2020). Design Justice: Community-Led Practices to Build the Worlds We Need. Cambridge: The MIT Press.
Crenshaw, K. (1989). Demarginalizing the intersection of race and sex: A black feminist critique of antidiscrimination doctrine, feminist theory and antiracist politics. Univ. Chic. Leg. Forum 1989.
Dou, R., Hazari, Z., Dabney, K., Sonnert, G., and Sadler, P. (2019). Early informal STEM experiences and STEMidentity: the importance of talking science. Sci. Educ. 103, 623–637. doi: 10.1002/sce.21499
Drachsler, H., and Greller, W. (2016). Privacy and Analytics – It’s a DELICATE Issue. LAK 16, Edinburgh.
Erden, D. (2020). “KI und Beschäftigung: Das Ende menschlicher Vorurteile oder der Beginn von Diskriminierung 2.0?” in Dann Feministisch. ed. K. I. Wenn (Berlin: Netzforma* eV), 77–90.
FEANI (2021). The UN Sustainability Goals: The Role of FEANI/ENGINEERS EUROPE and the European Engineering Community. FEANI (EU STEM Coalition Member). Available at: https://www.stemcoalition.eu/publications/un-sustainability-goals-role-feani-engineers-europe-and-european-engineering-community (Accessed October 11, 2022).
Floridi, L., Cowls, J., Beltrametti, M., Chatila, R., Chazerand, P., Dignum, V., et al. (2018). AI4People—an ethical framework for a good AI society: opportunities, risks, principles, and recommendations. Minds Mach. 28, 689–707. doi: 10.1007/s11023-018-9482-5
Godwin, A. (2016). The Development of a Measure of Engineering Identity. 2016 ASEE Annual Conference & Exposition, New Orleans, Louisiana.
Hartmann, B., and Schriever, C. (2022). Vordenkerinnen—Physikerinnen und Philosophinnen durch die Jahrhunderte. Münster: UNRAST Verlag.
Hazari, Z., Chari, D., Potvin, G., and Brewe, E. (2020). The context dependence of physics identity: examining the role of performance/competence, recognition, interest, and sense of belonging for lower and upper female physics undergraduates. J. Res. Sci. Teach. 57, 1583–1607. doi: 10.1002/tea.21644
Hoppe, H. U. (2017). “Chapter 2: computational methods for the analysis of learning and knowledge building communities,” in Handbook of Learning Analytics. 1st Edn. eds. C. Lang, G. Siemens, A. Wise and D. Gašević (SoLAR), 23–34.
Kitto, K., and Knight, S. (2019). Practical ethics for building learning analytics. Br. J. Educ. Technol. 50, 2855–2870. doi: 10.1111/bjet.12868
Lohaus, M., Perrot, M., and von Luxburg, U. (2020). Too relaxed to be fair. Proc. Mach. Learn. Res. 119, 6360–6369.
Mahadeo, J., Hazari, Z., and Potvin, G. (2020). Developing a computing identity framework: understanding computer science and information technology career choice. ACM Trans. Comput. Educ. 20, 1–14. doi: 10.1145/3365571
Mitchell, S., Potash, E., D’Amour, A., and Lum, K. (2021). Algorithmic fairness: choices, assumptions, and definitions. Ann. Rev. Stat. Appl. 8, 141–163. doi: 10.1146/annurev-statistics-042720-125902
Mujtaba, T., and Reiss, M. J. (2013). Inequality in experiences of physics education: secondary school girls’ and boys’ perceptions of their physics education and intentions to continue with physics after the age of 16. Int. J. Sci. Educ. 35, 1824–1845. doi: 10.1080/09500693.2012.762699
National Academies of Sciences, Engineering, and Medicine. (2019). Science and Engineering for Grades 6-12: Investigation and Design at the Center. Washington, DC: The National Academies Press.
Phillips, P. J., Hahn, C. A., Fontana, P. C., Broniatowski, D. A., and Przybocki, M. A. (2020). Four Principles of Explainable Artificial Intelligence. Gaithersburg: National Institute of Standards and Technology.
Prinsloo, P., and Kaliisa, R. (2022). Learning analytics on the African continent: an emerging research focus and practice. J. Learn. Anal. 9, 1–18. doi: 10.18608/jla.2022.7539
Prinsloo, P., and Slade, S. (2018). “Mapping responsible learning analytics: a critical proposal,” in Responsible Analytics & Data Mining in Education: Global Perspectives on Quality, Support, and Decision-Making. (Routledge).
Rosa, K., and Moore Mensah, F. (2016). Educational pathways of black women physicists: stories of experiencing and overcoming obstacles in life. Phys. Rev. Phys. Educ. Res. 12:020113. doi: 10.1103/PhysRevPhysEducRes.12.020113
Shanahan, M.-C. (2009). Identity in science learning: exploring the attention given to agency and structure in studies of identity. Stud. Sci. Educ. 45, 43–64. doi: 10.1080/03057260802681847
Slade, S. (2016). The Open University Ethical Use of Student Data for Learning Analytics Policy. The Open University.
Society for Learning Analytics Research (SoLAR) (2022). What is Learning Analytics? Available at: https://www.solaresearch.org/about/what-is-learning-analytics/ (Accessed August 31, 2022).
Suresh, H., and Guttag, J. (2021). A framework for understanding sources of harm throughout the machine learning life cycle. EAAMO ‘21: Equity and Access in Algorithms, Mechanisms, and Optimization. ACM, New York, NY, USA, 1–9.
Taconis, R., and Kessels, U. (2009). How choosing science depends on students’ individual fit to ‘science culture’. Int. J. Sci. Educ. 31, 1115–1132. doi: 10.1080/09500690802050876
Taskinen, P. H., Schütte, K., and Prenzel, M. (2013). Adolescents’ motivation to select an academic science-related career: the role of school factors, individual interest, and science self-concept. Educ. Res. Eval. 19, 717–733. doi: 10.1080/13803611.2013.853620
Traag, V. A., and Schmeling, L. (2022). Causal foundations of bias, disparity and fairness. ArXiv. doi: 10.48550/arXiv.2207.13665
UN-SDG-Goal 4. (2015). UN. Available at: https://sdgs.un.org/goals/goal4 (Accessed October 11, 2022).
Waight, N., Kayumova, S., Tripp, J., and Achilova, F. (2022). Towards equitable, social justice criticality: re-constructing the “black” box and making it transparent for the future of science and Technology in Science Education. Sci. & Educ. 31, 1–23. doi: 10.1007/s11191-022-00328-0
Wise, A. F., Sarmiento, J. P., and Boothe, M. (2021). Subversive Learning Analytics. Proceedings of the 11th International Conference on Learning Analytics and Knowledge (LAK’ 21), 12–16 April 2021, Irvine, CA, USA, 639–645.
Keywords: STEM identity, under-served students, responsible learning analytics, equity, bias, intersectionality
Citation: Grimm A, Steegh A, Çolakoğlu J, Kubsch M and Neumann K (2023) Positioning responsible learning analytics in the context of STEM identities of under-served students. Front. Educ. 7:1082748. doi: 10.3389/feduc.2022.1082748
Edited by:
Christoph Kulgemeyer, University of Bremen, GermanyReviewed by:
Valerie Harlow Shinas, Lesley University, United StatesAmanda Hovious, Emporia State University, United States
Copyright © 2023 Grimm, Steegh, Çolakoğlu, Kubsch and Neumann. This is an open-access article distributed under the terms of the Creative Commons Attribution License (CC BY). The use, distribution or reproduction in other forums is permitted, provided the original author(s) and the copyright owner(s) are credited and that the original publication in this journal is cited, in accordance with accepted academic practice. No use, distribution or reproduction is permitted which does not comply with these terms.
*Correspondence: Adrian Grimm, ✉ grimm@leibniz-ipn.de