- 1School of Educational Sciences, Tallinn University, Tallinn, Estonia
- 2Department for Continuing Education Research and Educational Technologies, Danube University Krems, Krems an der Donau, Austria
- 3School of Digital Technologies, Tallinn University, Tallinn, Estonia
Orchestrating collaborative learning (CL) is difficult for teachers as it involves being aware of multiple simultaneous classroom events and intervening when needed. Artificial intelligence (AI) technology might support the teachers’ pedagogical actions during CL by helping detect students in need and providing suggestions for intervention. This would be resulting in AI and teacher co-orchestrating CL; the effectiveness of which, however, is still in question. This study explores whether having an AI assistant helping the teacher in orchestrating a CL classroom is understandable for the teacher and if it affects the teachers’ pedagogical actions, understanding and strategies of coregulation. Twenty in-service teachers were interviewed using a Wizard-of-Oz protocol. Teachers were asked to identify problems during the CL of groups of students (shown as videos), proposed how they would intervene, and later received (and evaluated) the pedagogical actions suggested by an AI assistant. Our mixed-methods analysis showed that the teachers found the AI assistant useful. Moreover, in multiple cases the teachers started employing the pedagogical actions the AI assistant had introduced to them. Furthermore, an increased number of coregulation methods were employed. Our analysis also explores the extent to which teachers’ expertise is associated with their understanding of coregulation, e.g., less experienced teachers did not see coregulation as part of a teacher’s responsibility, while more experienced teachers did.
Introduction
Simultaneously monitoring several groups of students, analyzing the necessity of pedagogical action and predicting future collaboration is a formidable task. Yet, this is ubiquitously expected from teachers orchestrating their everyday collaborative learning (CL) activities. It is known that teachers are often not able to monitor and acknowledge the intricacy of group and individual operation concurrently (Schwarz and Asterhan, 2011). Hence, this is where learning analytics (LA) could be of use by helping detect students and groups who might be in need of assistance.
The gap between teachers finding LA systems “interesting” to teachers using them in their everyday work, needs to be overcome (Wise and Jung, 2019). The reasons for teachers not using LA systems are varied: over-complex tools, the uncertainty of their actual benefit (Geiger et al., 2017), or data not being presented in an actionable way (Dazo et al., 2017), so that the teachers may not know how to respond or intervene. In addition to this, the teachers are faced with some ethical concerns regarding data privacy, e.g., asking for permission to collect audio and video data of the students from the students and in case of underaged students, also their parents. In other words, if the teachers are only offered mirroring dashboards, which do not provide any warnings nor recommendations, they often seem complicated. In addition to this, teachers do not see the direct benefit deriving from the use of mirroring dashboards. However, research comparing mirroring and guiding dashboards has shown that guiding systems, which indicate problems and possible avenues of intervention, increase the teacher’s confidence in their decisions and decrease the level of effort to arrive at the decision (van Leeuwen and Rummel, 2020).
Additionally, current research showcases an urgency for guiding systems: Molenaar and Knoop-Van Campen (2019) and Amarasinghe et al. (2020) suggest that a recommendation service should be developed to existing LA systems. Correspondingly, Worsley et al. (2021) specifically draw attention to the need for systems identifying problems and at the same time offering possible avenues for solving the issues. Ultimately, the focus of collaboration analytics should be on really having an impact on teaching and learning about how to collaborate better (Wise and Jung, 2019; Worsley et al., 2021). Until now, what kind of pedagogical action to choose for a particular group of students has remained an untouched avenue (van Leeuwen and Rummel, 2020).
Coregulation, where a teacher and a student are regulating learning together (Hadwin and Oshige, 2011), is a possible pathway toward learning and teaching how to collaborate better. During coregulation, the teacher could help the students by sharing the demands of monitoring, evaluating, and regulating the task processes, more explicitly by asking information, mirroring the students’ ideas, requesting the students to reflect on their learning, modeling thinking, and offering prompts for thinking and reflecting (Hadwin and Oshige, 2011). Needless to say, the teacher being able to make sure each student (or every single group) is coregulated in each CL session, is difficult (Allal, 2020). For this reason, using an AI assistant might help to decrease the workload and at the same time offering ideas for intervention to the teacher. In this paper, we explore how AI and teacher co-orchestration would affect the understanding and use of coregulation strategies, which is a means of teaching and learning how to collaborate.
This paper is part of a larger design-based research (DBR), where empirical educational research is combined with the theory-driven design of learning environments (Baumgartner et al., 2003) with the purpose of finding solutions to support teachers during CL using LA. In prior work, we have completed three DBR iterations (Kasepalu et al., 2021), this being the fourth iteration process, where the utility of an AI assistant was explored using a paper prototype. The purpose of this study was to investigate the perceived usefulness and pedagogical actions of teachers by conducting a Wizard-of-Oz (WoZ) protocol with twenty in-service teachers from thirteen different upper-secondary and vocational schools in Estonia. One of the main contributions of this paper is a model for coregulating collaboration during a CL activity. This model is at the core of the AI assistant’s logic. The study investigates how teachers would use an AI assistant in synchronous face-to-face CL activities (pedagogical action) and whether they perceived the suggestions proposed by the assistant as something helpful (usefulness).
The paper is separated into six sections. First, we present the “Related Work” already conducted in the field of LA for CL and its coregulation, then we introduce the theoretical underpinnings of “The Collaboration Intervention Model (CIM)” that was used to inform the AI assistant in the WoZ protocol, after which we present the CIM. Next, we guide the reader through the “Methodology” section of our study followed by the “Results” section showing how useful the teachers found the AI assistant, and how much their understanding of coregulation changed. Finally, the reader can get acquainted with the “Discussion” section as well as the “Limitations and Future Work,” where we present practical, technology design and methodological implications.
Related Work
Learning Analytics Adoption in a Synchronous Face-To-Face Classroom
The LA tools available today most often offer post hoc analysis with no option for the students nor the teacher to get feedback that might help develop collaboration skills (Worsley et al., 2021). If teachers are not provided with data about the students’ process, it is difficult to identify groups who are in danger of failing or not performing well (Tarmazdi et al., 2015). As a solution, dashboards give teachers supplementary insights for them to adequately respond to the needs of the students (Molenaar and Knoop-Van Campen, 2019) by collecting multimodal data from the students (Chejara et al., 2021). We will inquire about the perceived usefulness of the AI assistant, as it is very closely associated with technology acceptance and adoption by the target user (Liu and Nesbit, 2020), which remains one of the main challenges in LA. Work-integrated learning suggests that the best practice for learning is to experience authentic work practices and practice using skills and knowledge in a real-world context, as opposed to a training course remote from the teachers’ everyday work and classroom (Jackson, 2015). Situated models of learning assume that learning that is connected to the place of work has advantages for developing meaningful knowledge and transferring learning into practice (Smith, 2003; Ley, 2019). As some studies have found that teachers’ behavior was influenced by the LA tool, e.g., in terms of providing more support for the collaborating groups (van Leeuwen, 2015) or by adjusting the runtime of activities in the classroom (Martinez-Maldonado et al., 2015), we will be inquiring whether the AI assistant affects the pedagogical actions of a teacher as only understanding the tool is not enough for technology adoption.
Coregulation in a Collaborative Learning Classroom
Teacher guidance positively influences students’ learning (Seidel and Stürmer, 2014) and can potentially play an important part in the process of students attaining collaboration skills (Worsley et al., 2021) through coregulating learning. We use coregulation in this study as Hadwin and Oshige (2011) have defined it – a teacher (or a more capable peer) and a student regulating learning together. Coregulation has been divided into three subcategories: the structure of the teaching situation; the teacher’s interventions and interactions with students; and the interactions between peers (Allal, 2020). Within the context of this study, we focus on the teacher’s interactions and interventions with students and how these skills are influenced using an AI assistant. Hadwin and Oshige (2011) see coregulation as a tool on the way toward self-regulated (a person regulating her/his own learning path) or socially shared regulation (several people regulating a collective activity) of learning. However, Allal (2020) posits that within the constraints of a classroom, students are not becoming self-regulating learners, but instead, they are adapting to learning in ever-increasingly complex forms of coregulation. In either case, the teacher cannot assume the students will become autonomous self-regulating learners without offering some guidance on the way. The teacher can coregulate by (1) offering feedback or (2) directly adapting the activities of the students. Scaffolding of metacognitive processes, not content is expected in a coregulating process, meaning that teachers should not provide tips on how to answer the next question or do the next task, but to help think about the process of learning (Hadwin and Oshige, 2011). What is more, the teachers are required to walk a fine line between giving students space to figure things out themselves and showing that they are present, and ready to support the learners. Concurrently, the teachers should be transferring control over to students. The teachers need to manage their own teaching process while paying attention to strategies students employ for solving the task and strategies they employ for collaboration (van Leeuwen and Janssen, 2019). Needless to say, in normal classroom conditions, it is not really possible for one teacher to make sure each student is individually coregulated (Allal, 2020), which is where an AI assistant might be of help. We will study if and how an AI assistant affects the understanding and methods of coregulation of the participating teachers.
Pedagogical Actions Coregulating Collaborative Learning Using Artificial Intelligence
Pedagogical actions are interventions with the purpose of supporting students to learn better (Molenaar and Knoop-Van Campen, 2019). In our study we are focusing on pedagogical actions that help coregulate CL. When students collaborate and are in the process of learning how to do so, problems arise. Teachers can implement pedagogical actions to resolve the issues, but students do not only want the problems and issues to be solved, they want to learn how these types of challenges can be addressed successfully in order to be able to later solve them themselves (Worsley et al., 2021). We have discussed the teachers not being able to diligently monitor collaboration going on in all groups, which has also been called the teacher’s professional vision – the knowledge to notice and make sense of situations in the classroom (Seidel and Stürmer, 2014). The teacher’s professional vision has been separated into three dimensions: describe, explain, and predict. However, the abundance of activities happening in a CL classroom can cause teachers to experience cognitive overload (Gegenfurtner et al., 2020), which is why they might not be able to move on beyond describing. Knowing that human capabilities are somewhat limited, we collect data in order to generate patterns not identifiable by humans (Jørnø and Gynther, 2018). However, it is said that AI systems complemented with the strengths of the teacher provide even more effective results in helping students and enhancing their learning (Baker, 2016; Holstein et al., 2019b) and thus AI and human co-orchestration systems have been proposed (Holstein et al., 2019b). The now evermore prominent idea of intelligence amplification encompasses AI leveraging teacher intelligence (Baker, 2016). The AI could possibly aid the teacher in describing the actions happening in the classroom and predicting whether the collaboration process is developing into a successful one. A mirroring LA tool only provides the teacher with some information about the collaboration without comparing it to a desired model (alerting) or offering suggestions for intervention (guiding). Notwithstanding, a systematic review of teacher guidance during collaborative learning (van Leeuwen and Rummel, 2019) revealed that the majority of the reported LA tools had only a mirroring function (as opposed to alerting or guiding). This meaning that some information was made accessible to the teacher, but the interpretation (explaining, predicting) and potential intervention thereof was left to the teacher. In the pursuit of understanding the process of teachers choosing their pedagogical actions, the Situated Model of Instructional Decision-Making (Wise and Jung, 2019) was created. The model was created with the purpose of trying to bridge the gap from teachers finding LA interesting to them using it to inform interventions in class. Wise and Jung (2019) studied the sense-making and responses of five teachers using LA tools. The model differentiates three main kinds of pedagogical action: targeted action, wait-and-see and reflection. When teachers adopt a wait-and-see strategy, they feel the need to collect more data or are just uncertain about how to act, under targeted action we can separate whole-class scaffolding, targeted scaffolding and revising course elements. The third kind of pedagogical action can spark a deep reflection within the teachers (Wise and Jung, 2019). We will study if and how the AI assistant affects the pedagogical actions undertaken by the teachers.
The following section will present the model that was drafted as the core of the AI assistant used in the WoZ protocol, where different problems occurring during collaboration are mapped with pedagogical actions the teacher could use to coregulate CL.
The Collaboration Intervention Model
This study is part of a DBR, where three iterations have been completed. We have completed a needs analysis and paper prototype in the first iteration (Kasepalu et al., 2019), a research prototype multimodal learning analytics (MMLA) tool named CoTrack in the second iteration (Chejara et al., 2020). The third iteration, a vignette study with twenty-one in-service teachers, showed a discrepancy between the evaluation of the quality of collaboration among teachers, i.e., interviewed teachers were unable to agree whether the perceived collaboration had been effective or not (Kasepalu et al., 2021). With the purpose of assessing the quality of the collaboration process, Rummel et al. (2011) have proposed the Adaptable Collaboration Analysis Rating Scheme (ACARS). The rating scheme entails seven dimensions measuring different aspects of collaboration quality: sustaining mutual understanding, collaboration flow, knowledge exchange, structure/time, argumentation, cooperative and individual task orientation. ACARS served as the basis for the CIM, our rules-based kernel for the AI assistant, where we model potential collaboration problems and adequate pedagogical interventions.
In the first iteration of our DBR process we looked into the problems teachers had with CL (see Kasepalu et al., 2021 for more details). After this, we mapped these problems with the seven dimensions proposed by Rummel et al. (2011) (see Appendix 1 for the whole model, Table 1 for an excerpt). After this, another literature review was carried out to find possible solutions for the problems that had been indicated as prominent during previous iterations. This synthesis resulted in the CIM (see Appendix 1 for the whole model), where the aforementioned seven dimensions are mapped with indicators on two levels. The two levels are group-level and class-level for the teacher to know whether the problem seems ubiquitous in the classroom or only one group needs more attention. In addition to mapping potential problems, also high indicators have been listed to possibly notify the teacher of very effective collaboration. This indicator has been added as this emerged as a subject of interest for teachers in previous DBR iterations (Kasepalu et al., 2019), providing them with positive feedback, instead of only receiving alerts of (potential) problems. The model informed the AI that was used in the WoZ protocol.
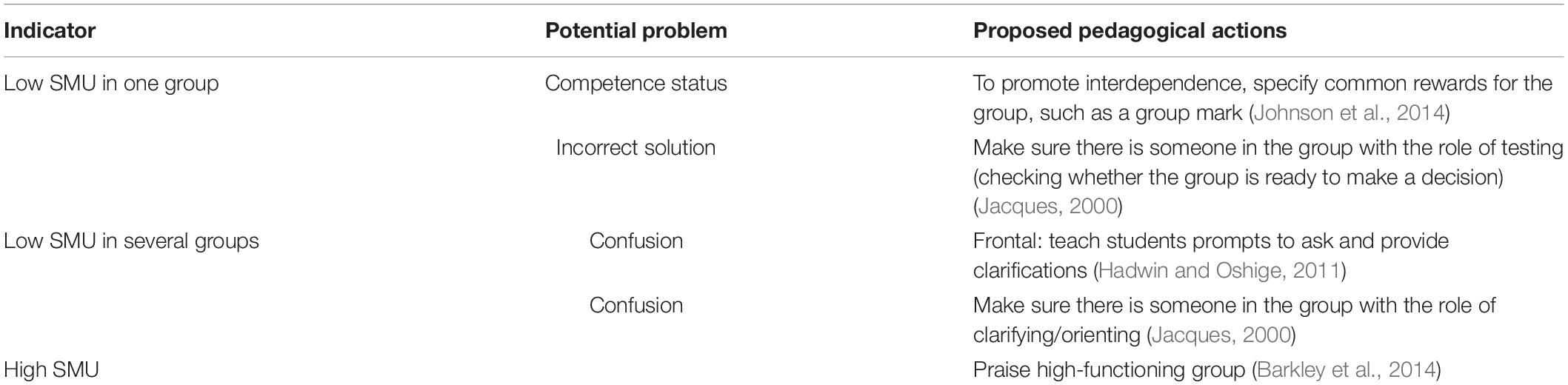
Table 1. The Collaboration Intervention Model (CIM) for Sustaining Mutual Understanding (SMU) in group and classroom level.
Study Aim and Research Questions
The study investigates how teachers would use an AI assistant based on the Collaboration Intervention Model (CIM) in synchronous face-to-face CL activities, whether teachers would find it useful and if it would initiate pedagogical action. Our goal is to see whether such an AI assistant affects the teachers’ understanding of coregulation and for this we use a vignette and WoZ method.
To study the usefulness of the AI assistant, the following two questions were asked:
RQ1 How comprehensible and meaningful for teachers are the suggestions proposed in the Collaboration Intervention Model (as the core of the AI assistant)?
RQ2 Would the teachers find an assistant providing suggestions for coregulation during collaboration helpful for supporting the students?
To study the teachers’ pedagogical actions, the following two questions were posed:
RQ3 How likely is the teacher to carry out the proposed interventions?
RQ4 How do teachers’ pedagogical actions change when an AI assistant is introduced?
Based on the idea of intelligence amplification where the AI assistant takes over some responsibilities leveraging human intelligence (Baker, 2016), we ask:
RQ5 How is the teachers’ understanding and methods of coregulation affected by the introduction of an AI assistant?
Methodology
The study followed a vignette study design similar to van Leeuwen et al. (2014) where each teacher went through eight vignettes. The vignettes were from five different groups taken from a real CL classroom recorded in a prior study and lasted 10 min altogether. The teachers responded to a pre-and post-test where the teachers’ knowledge of coregulation methods, understanding of coregulation, and confidence levels were dependent variables and getting suggestions from the AI assistant an independent variable. An overview of the instruments used can be found in Table 2. Following the vignette, teachers interacted with a paper prototype of an AI assistant using WoZ method (Kelley, 1984), where the participating teachers were given the impression that they were interacting with an AI assistant. Wizard of Oz studies have been used on intelligent human interfaces for decades with the purpose of designing user-friendly systems (Dahlbäck et al., 1993) by exploring a potential system with end-users without extensive engineering needed (Schlögl et al., 2015). The WoZ method combined with authentic data is claimed to be a fruitful direction for future work (Holstein et al., 2020).
We used quantitative analysis methods to show trends, qualitative analysis to triangulate and illustrate, we are working in a post-positivist paradigm. To answer RQ1, the teachers were asked to rate the understandability of the proposed problems and suggestions on a scale from 1 to 10. The scale was labeled as follows: 1 Very Strongly Disagree, 2 Strongly Disagree, 3 Disagree Mostly, 4 Disagree, 5 Slightly Disagree, 6 Slightly Agree, 7 Mostly Agree, 8 Agree, 9 Strongly Agree, 10 Very Strongly Agree. To study the helpfulness of an AI assistant (RQ2), we conducted a pre- and post-test. As the teachers’ coregulation is divided into two separate segments: providing feedback and adaptation (Hadwin and Oshige, 2011), the instrument was constructed accordingly asking about the importance of monitoring the students, providing feedback and offering strategies to the students as well as the confidence in the knowledge of these strategies and the overall usefulness of an AI assistant aiding the teacher during CL.
To study RQ3, we asked the teachers to rate the likelihood of them carrying out the pedagogical actions proposed by the AI assistant in their own classrooms on a Likert scale from 1 (very unlikely) to 5 (very likely). With the purpose of answering RQ4, we compared the answers the teachers gave before being introduced with the suggestions by the AI assistant and after. To answer RQ5, we compared the items from the pre- and post-test (observation, providing feedback, confidence in coregulation strategies) and the methods of coregulation suggested by the teachers.
Vignette
A vignette consisting of eight different situations from authentic CL activities were designed that had been coded with two independent coders (Cronbach’s alpha between 0.7 and 0.95 in different dimensions) using the Adaptable Collaboration Analysis Rating Scheme (Rummel et al., 2011). The learning activities in the video vignettes took place during two upper-secondary biology lessons with five different groups of mostly four students. The task was to fill out a worksheet together or compile an ethical guide for raising genetically mutated crops using Etherpad (a live multi-user text editor which runs in the browser).
The eight situations were chosen to depict the following problems: a passive student, a student off-task, isolated pair work (students are not working as a team, but instead in isolated dyads), confusion, dominance, a student doing nothing (see Table 3).
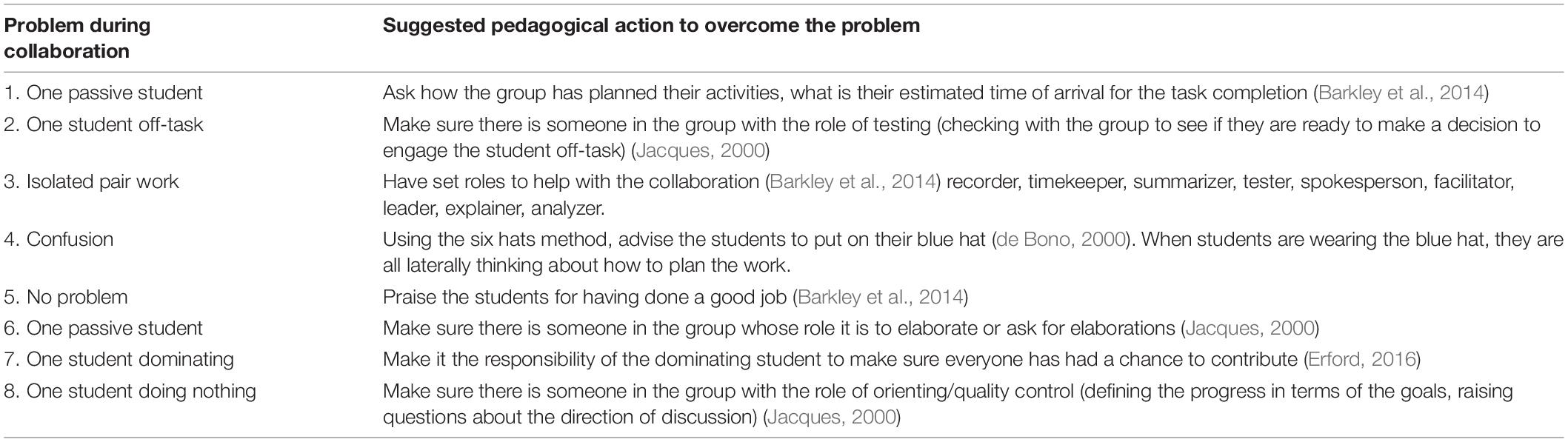
Table 3. The eight vignettes used in the Wizard-of-Oz (WoZ) protocol, and proposed suggestions for pedagogical actions, according to the CIM model.
There were eight different situations altogether, the whole duration of the vignette was 10 min. Each individual vignette lasted about 1 min after which there was some time for the teacher to comment and answer if there had been a problem during the witnessed collaboration. The teacher answered if they would intervene and elaborated on how they would do it when the answer was yes. For each situation, we also had a suggestion for a pedagogical action taken from the CIM to solve the problem (see Table 3). For instance, if a group seems confused, the teacher might suggest opting to use the blue hat from the six hats method (de Bono, 2000) where everyone needs to think about the ways the group will be working together.
Data Collection
Prior to data collection, the aims of the study were introduced to the participants, and all teachers signed a consent form, which had been approved by the CEITER ethics board. Invitations to participate in the study were sent to twenty upper secondary schools, 20 teachers (17 female and 3 male) from thirteen schools participated in the study. Before being introduced to the vignette, the teachers filled out a pre-test questionnaire which inquired about their understanding of coregulation, knowledge of coregulation and its methods and levels of confidence (see Table 4).
The vignette study was conducted in face-to-face settings with 20 teachers interviewed in Estonian, one teacher was interviewed in English. During the vignette, teachers took part in a WoZ protocol, which allows a user to interact with an AI assistant prototype that does not exist yet (Kelley, 1984).
This is how the WoZ protocol was carried out for all teachers. After the pre-test, the teachers were shown the first vignette, after which the teachers were asked to decide if the perceived collaboration seemed problematic to them or not. When identifying a problem, the teachers were then asked whether they would intervene in any way and if the answer was positive, then they had to specify how they would intervene. After this, a problem was presented (in Estonian, except one case in English) on a slip of paper and introduced this as a problem provided by the AI assistant. The teachers were asked to rate the understandability of the problem (as stated by the AI assistant) on a scale of 1–10: 1 indicating that the teacher does not understand the problem or suggestion at all, it does not seem meaningful nor comprehensible for the respondent; 10 indicates a complete understanding of the problem or suggestion, conveying that the teacher thinks that the problem/suggest is both comprehensible as well as meaningful. The scale was labeled as follows (Taherdoost, 2019): 1 Very Strongly Disagree, 2 Strongly Disagree, 3 Disagree Mostly, 4 Disagree, 5 Slightly Disagree, 6 Slightly Agree, 7 Mostly Agree, 8 Agree, 9 Strongly Agree, 10 Very Strongly Agree. As the next step, the first author introduced a suggestion on a slip of paper telling the teacher this was a suggestion offered by the AI assistant. After this, the teachers were asked to again rate the understandability of the suggestion as well as the likelihood of carrying out the intervention in their own classroom. A similar procedure was repeated for the following seven vignettes. As the last step in the WoZ protocol, all teachers filled out a post-test questionnaire.
Data Analysis
Qualitative Data Analysis
The interviews were transcribed with the help of an automated transcription tool available at http://bark.phon.ioc.ee/webtrans/ the Estonian language (Alumäe and Tilk, 2018), then further corrected by the first author. The qualitative software program QCAmap was used to manage and code the transcripts, the interviews were coded inductively by three independent coders. When looking at the verbal reactions toward the suggestions, we divided the comments into two: positively received and rejected by the teachers, and brought out examples for each. To answer RQ4, the answers the teachers provided before seeing the problems and suggestions offered by the AI assistant and afterward were coded. After this, the codes were mapped using the Situated Model of Instructional Decision-Making (Wise and Jung, 2019). We also coded the interviews according to the coregulation methods proposed by Hadwin and Oshige (2011): adapting behavior, asking for information, helping monitor, mirroring students’ ideas, providing feedback, reflecting on thinking and noted the timepoint when the teacher suggested this type of intervention.
Quantitative Data Analysis
For the quantitative analysis, RStudio was used. To study the reaction of the teachers toward the model (the understandability of the problems as well as suggestions), we used descriptive statistics methods. A boxplot was created to visualize the results. Descriptive statistics methods and a paired t-test were used to describe the perceived usefulness (RQ1) of the AI assistant and a boxplot was created to envisage the change before and after being introduced to the AI assistant. With the purpose of studying the likelihood of teachers carrying out the suggestions, we used descriptive statistics methods. To study RQ5, paired t-tests were carried out and boxplots were once more used for visualization purposes. Additionally, we carried out a correlation test (answers to pre- and post-test) using experience as a variable.
Results
RQ1 How Comprehensible and Meaningful for Teachers Are the Suggestions and Problems Proposed in the Collaboration Intervention Model as the Core of the Used Artificial Intelligence Assistant?
The problems detected by AI assistant seemed rather understandable for the teachers participating in the study (mean 7.975 on a scale 1–10, see Figure 1 for the boxplot). However, the solutions offered by the AI assistant were rated even more understandable (mean 8.1875 on a scale 1–10, see Figure 1 for the boxplot).
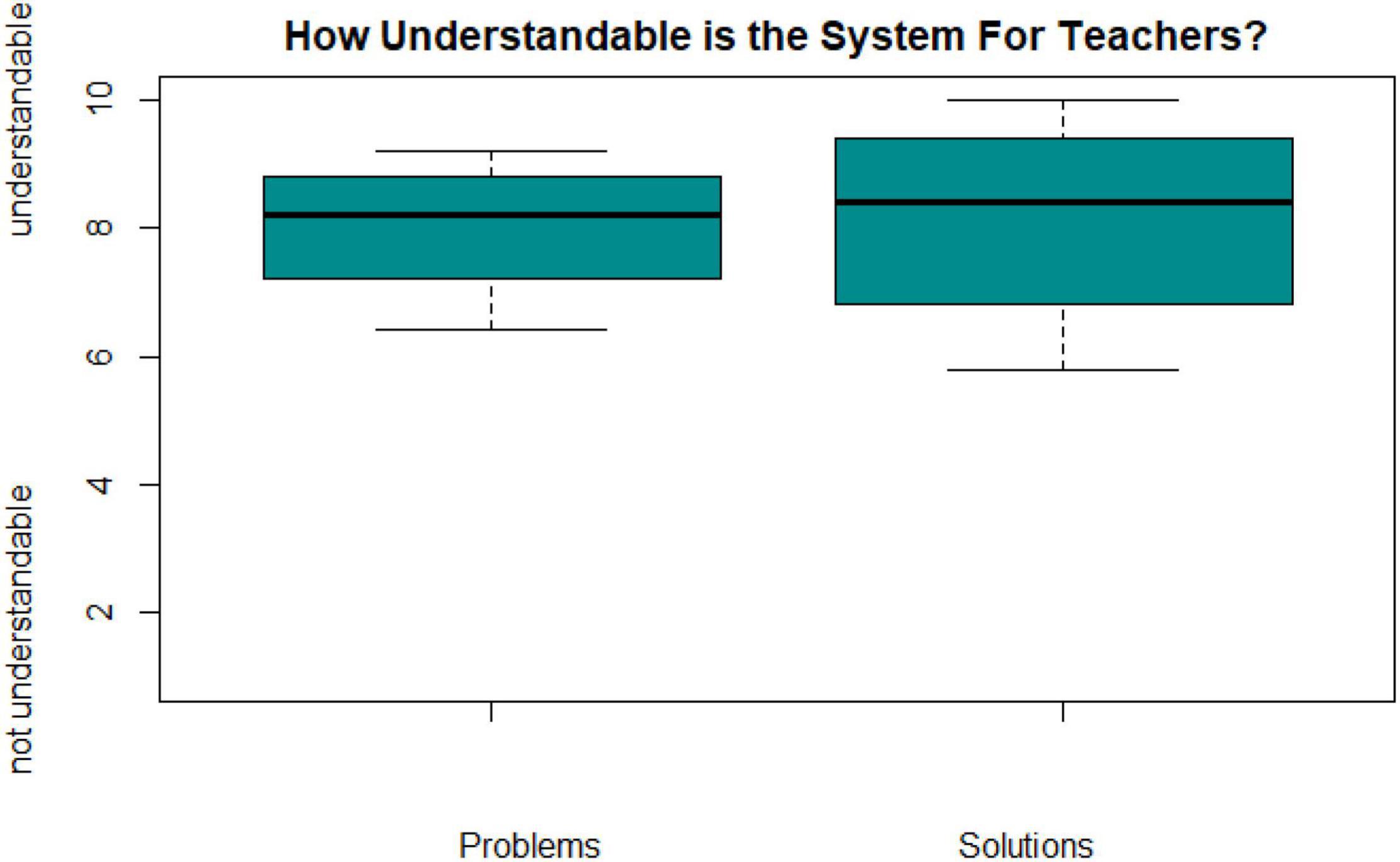
Figure 1. How did the teachers perceive the understandability of the solutions and problems provided by the AI assistant?
To further triangulate the interpretation that the participating teachers found the AI assistant understandable, is the notion of the teachers starting to employ the pedagogical actions introduced in the experiment as possible interventions in the next scenarios. For instance, 13 teachers introduced the idea of having different roles during collaboration when proposing a solution to the problem in the seen vignette after it had been introduced by the AI assistant beforehand. T2 even proposed using roles as an intervention in three subsequent scenarios after the AI assistant had suggested the idea. T9 stated later in the post-interview that he “actually started thinking differently during the experiment,” T11 added that she “would not have thought of this method on my own, but now that I was suggested it, I would try it.”
Out of 56 qualitative comments made about the suggestions provided to the teachers, in 39 cases the teachers were positively minded, only changing some aspects of the proposal (11 cases). These positively minded comments were uttered by 14 different teachers, e.g., describing the suggestions as “really cool” (mentioned nine times), “very interesting” (mentioned eight times) or even as a “brilliant idea” (three times). When discussing the changes the teachers would have liked to have made to the suggestions, many of them were connected to the timing of the interventions. In several cases, the teachers felt that the intervention strategy should be introduced to the students at the beginning of the lesson, instead of piling it on them during the collaboration. In 17 cases, the teachers felt reluctant toward the suggestions. Out of these rejecting reactions, we could identify that the teachers either felt that they did not have enough time for the intervention (T2 “Yes, I imagine that if I were to start each collaboration by making the students create a plan, then it could help make it more effective, but I do not think I have the time for that”), or they did not agree on the phrasing of the problem (T3 “The program says they were doing nothing, but I would say that were doing something”) or the method seemed too alien for them (T15 “I have heard about this six hats method, but as I am a realist, I do not like these things with weird names, I feel as if I am allergic to them”).
RQ2 Would the Teachers Find an Assistant Providing Suggestions During Collaboration Helpful?
While most teachers were positively minded about having an AI assistant assist them during collaboration (mean 6.2 on a scale 1–7, see Figure 2 below), using the AI assistant convinced the more skeptic ones of its usefulness. A paired t-test was used to compare the attitudes of the teachers before and after interacting with the AI assistant. The teachers’ opinions on the usefulness of the AI assistant showed a statistically significant difference with a medium effect (t = −2.2188, df = 16, p = 0.041, Cohen’s d = 0.621) after having been introduced to the AI assistant. Some of the teachers commented on their own limitations in the classroom and “how having technology to assist us could be of benefit” (T13), T15 said that “it is not good to be overly sure of yourself.” Several teachers (T14, T16, T7) pointed out that having an assistant would definitely not hurt them, because they feel that the final decision is still in their hands, but they have an option to outsource some information that otherwise might not be available to them. Teacher 14 is one of several teachers indicating that the AI “is an idea bank and would absolutely be helpful” in the teachers’ everyday work.
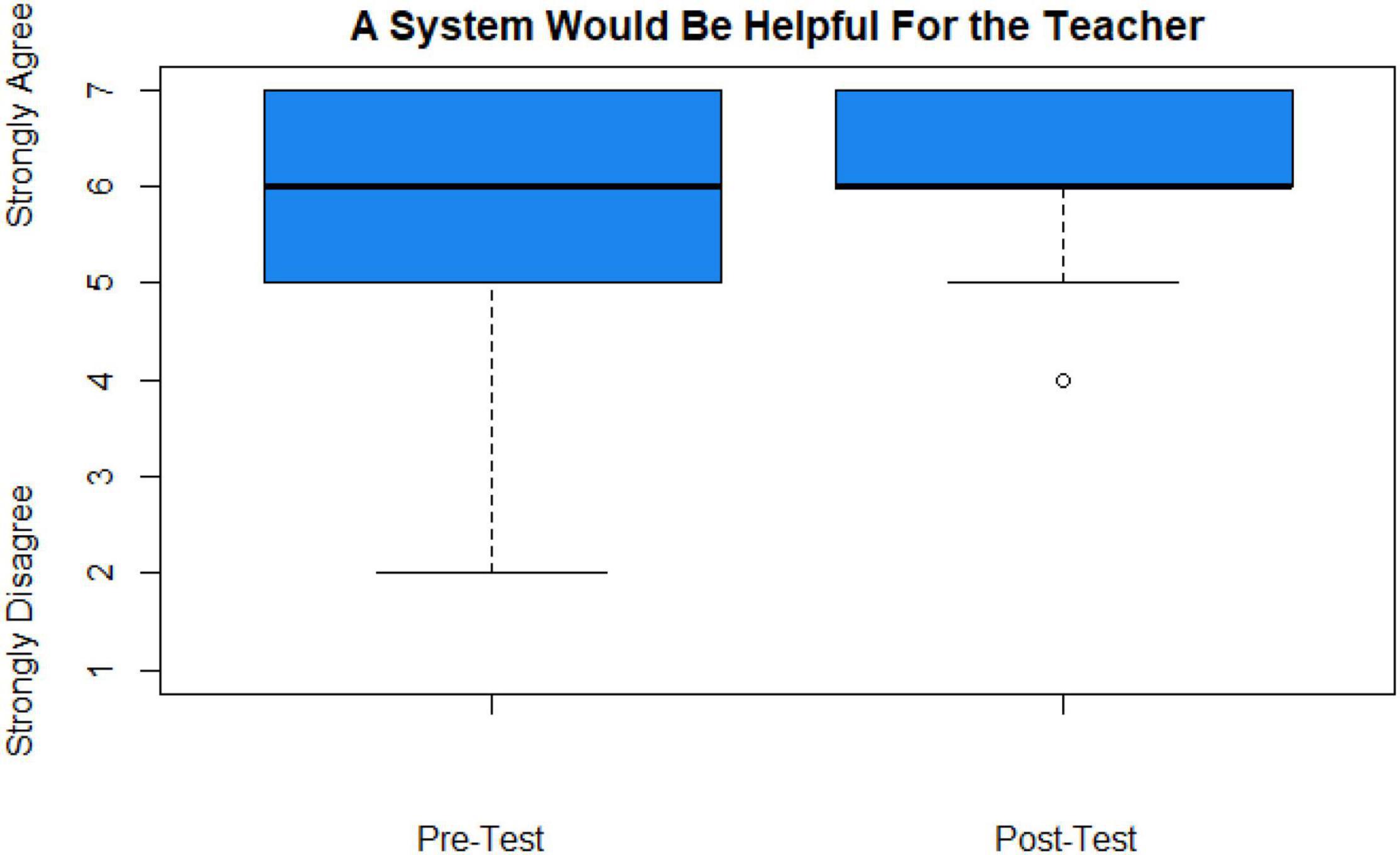
Figure 2. Boxplot of answers to the question if the perceived usefulness of the AI assistant increased after the experiment.
RQ3 How Likely Is the Teacher to Carry Out the Proposed Interventions?
The involved teachers rated the likelihood of carrying out the intervention strategies offered by the AI assistant from neutral (1) to very likely (5) with a mean of 3.9. It appears that the teachers seem rather willing to try the intervention strategies in their own classroom. To triangulate this finding, the participating teacher T13 said that “if a system gave me ideas about how to intervene, I would definitely do it.” Six teachers saw the prototype as a useful idea bank, which might only be needed to use for some amount of time. T17 and T15 stated the importance of technology enhancing the skills of the teacher: “I am not able to observe everything to the extent I would like to, this is where technology could help me.” However, the teachers also indicated that they might rather feel comfortable using it as an analysis tool for planning the next lessons: T10 said “I would not use it right away, because every intervention costs time, I would rather use this as a hint for the next lesson.” In some cases, the teachers feel hesitant to carry out the interventions because they have a feeling that it will not work either because they believe that the students are not capable of implementing the strategies or it might seem as an overreaction from the teacher’s behalf.
RQ4 How Do the Pedagogical Responses Change When an Artificial Intelligence Assistant Is Introduced?
We mapped the interventions using the Situated Model of Instructional Decision-Making (Wise and Jung, 2019). When looking at Table 5, we can see that the involved teachers before being introduced to the suggestions of the AI assistant, were more prone to opt for the wait and see strategy. However, after the provided suggestions, the teachers were more subject to carry out a pedagogical action, whether it was precisely following the directions of the model, a modified version of the model or an alternative suggestion offered by the teachers. It could be inferred that an AI assistant supporting teachers during CL could make teachers intervene more frequently during lessons.
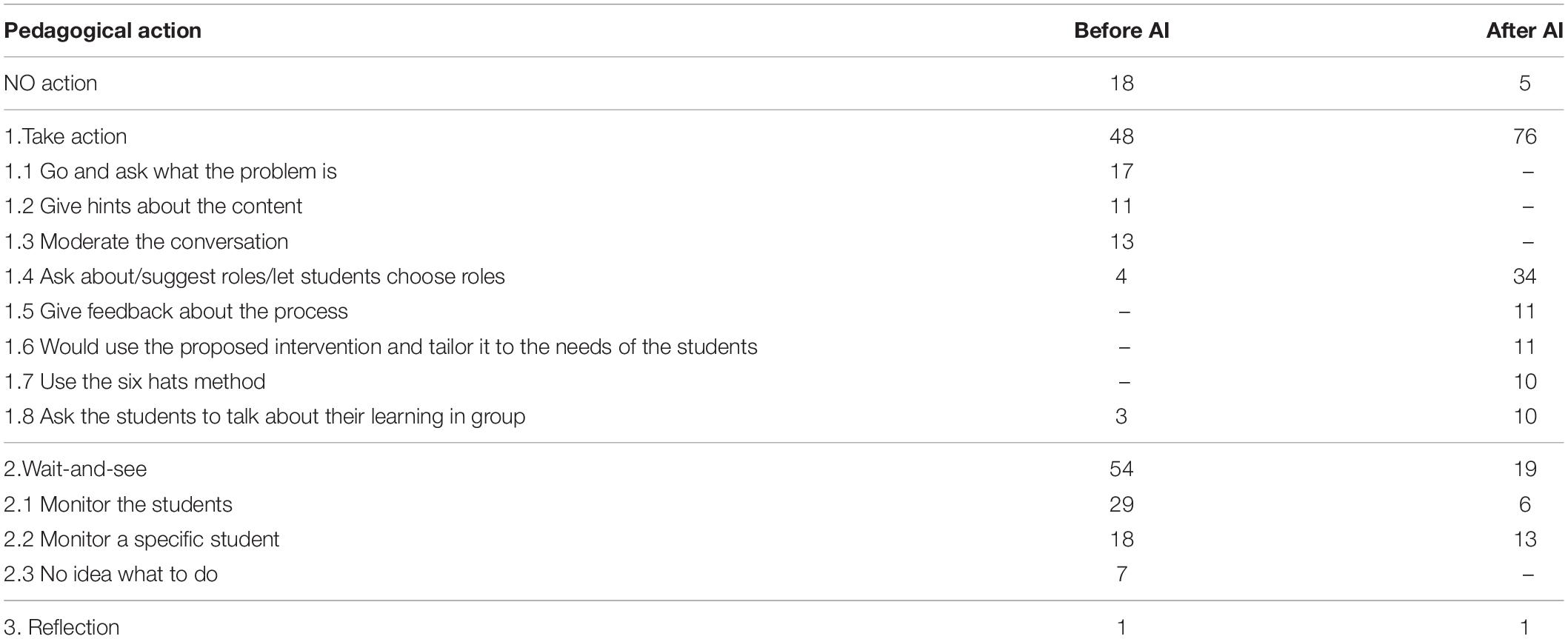
Table 5. A comparative table showing the intervention ideas the teachers had before and after seeing the suggestions by the AI assistant; the numbers indicate the number of comments.
It seems that the teachers received new ideas from the AI assistant, as well as were more ready to specify the nature of the intervention after having been exposed to the suggestions of the AI assistant. Teacher 4 is an example of a teacher before interacting with the AI assistant being unsure of which interventions to carry out commenting that “I do not know how to intervene here, I have no idea, I would just continue monitoring them.” Whereas after getting suggestions from the AI, started assigning roles to the students: “and the dashboard could inform me if they were actually adhering to them, it would not be difficult to see that.” It must be noted that even if some of the pedagogical actions were not completely new to the teachers, they still had not used them in their everyday practice.
RQ5 How Is the Teachers’ Understanding and Methods of Coregulation Affected by the Introduction of an Artificial Intelligence Assistant?
Importance of Observation
We again used a paired t-test to compare the attitudes of the teachers before and after interacting with the AI assistant. There was a difference in the teachers’ perception of how important observing the students during collaboration was (t = 2.4, df = 16, p = 0.029, Cohen’s d = 0.474), indicating a small effect. When asking about how important it was to provide feedback on the process (vs. product) of collaboration, a medium effect was witnessed (p = 0.027, t = 2.4259, df = 16, Cohen’s d = 0.625). We can say that the participating teachers’ interest in observing students during CL increased concurrently with the study. Evermore so, it increased the teachers’ urgency to provide feedback on the process of collaboration. T16 stated that “Yes, it would be very good to give students feedback about the process, but I have so big classes, I just do not normally have the information to base the feedback on. If the assistant could help me with that, I would love to do it” and T7 stressed that “now that we have gone through the videos, I understand how important it actually is to observe the students very closely and how difficult it would be to do it with several groups in order to provide feedback they clearly need.” Before the AI assistant, the teachers indicated in five cases that providing feedback on the product of the collaboration is essential, after being introduced to the AI assistant, 11 teachers would hypothetically intervene during the lesson by giving feedback about the process of collaboration.
Not Knowing How to Intervene
Some of the teachers (7 instances) noted that there was a problem with the collaboration, but they did not intervene since they did not know how to do it: “No, I would not know how to intervene at all, but I might stop to observe them a bit more” (T4), “I do not know how to answer you, I would not know how to intervene” (T2). However, after the AI assistant had suggested an intervention, the teachers were motivated to either agree with the proposed suggestion or pose an alternative intervention themselves. As can be witnessed in Figure 3, the participating teachers felt they knew more coregulation strategies after having interacted with the assistant than they did before looking at the vignettes and receiving suggested pedagogical actions from the AI assistant. However, teacher like T17 have commented that “I am feeling a lot more confident after having walked through these eight situations with you, it is not so scary anymore.”
Role of Expertise
The boxplot in Figure 3 shows that the overall knowledge has increased. A paired t-test did not show statistical significance, but as our previous study (Kasepalu et al., 2021) showed that experience was a critical factor in the projected use of a tool, we wanted to see if there was a difference in the results based on the level of experience. Using the experience threshold from The Teaching and Learning International Survey (TALIS, 2018), there were eight teachers with less than 5 years of experience and nine teachers with more than 5 years of teaching experience, three teachers had not answered the pre-and post-test. When comparing the means of these two groups before and after seeing the AI assistant using a paired t-test, the results showed that less experienced teachers had rated their knowledge of the strategies higher than more experienced teachers, and as the knowledge increased for more experienced teachers, it did not have that effect for the less experienced teachers. In order to find out if the difference between more and less experienced teachers is statistically significant, we ran a correlation test Using the Spearman method and experience as a variable. As a result we saw that in question 3 (if providing strategies to students was the teacher’s responsibility) there was a correlation (p = 0.001) that was statistically significant. When the teachers were asked whether they thought that a teacher should provide students helpful strategies with the purpose of improving collaboration, each of the more experienced teachers agreed with the statement strongly, whereas less experienced teachers were more hesitant on the matter (see Figure 4 below). In order to test the statistical significance in our non-normal population (because the values for P3 were close to 100% and our sample was small), we carried out the Fisher’s Exact test. Here, the p-value yielded 0.002, confirming the statistical significance. In addition to this, we carried out a pairwise t-test in RStudio that is used to calculate pairwise comparisons between group levels with corrections for multiple testing, which gave the result p = 0.01 meaning that the difference is statistically significant.
Self-Perception of Coregulation Knowledge
When looking at the intervention strategies employed, we noted that the teachers started employing more coregulation strategies after they had been interacting with the AI assistant for three situations. The first part was an average of four strategies, and almost 8 on average in the second part (see Table 6). We could infer from this, is that the teachers had picked up ideas from the AI assistant, possibly learned from the AI assistant, and started employing coregulation strategies more than they did in the beginning. As the teachers had stated in the questionnaire that providing feedback about the process to the students is essential, here we can see that when the teachers were shown vignettes of authentic classroom situations and after they had received some suggestions from the AI assistant, the teachers started providing more feedback, inquiring students, or adapting their behavior. Also, the variety of strategies employed increased. It must be noted that the number of interventions is lower than the number of pedagogical responses because often the teacher would give tips to the students about the content which is not considered a coregulation strategy. Overall, the intervention strategies became slightly more varied, but most probably more time is needed to interact with the AI assistant and try out different intervention strategies to make this a more general claim.
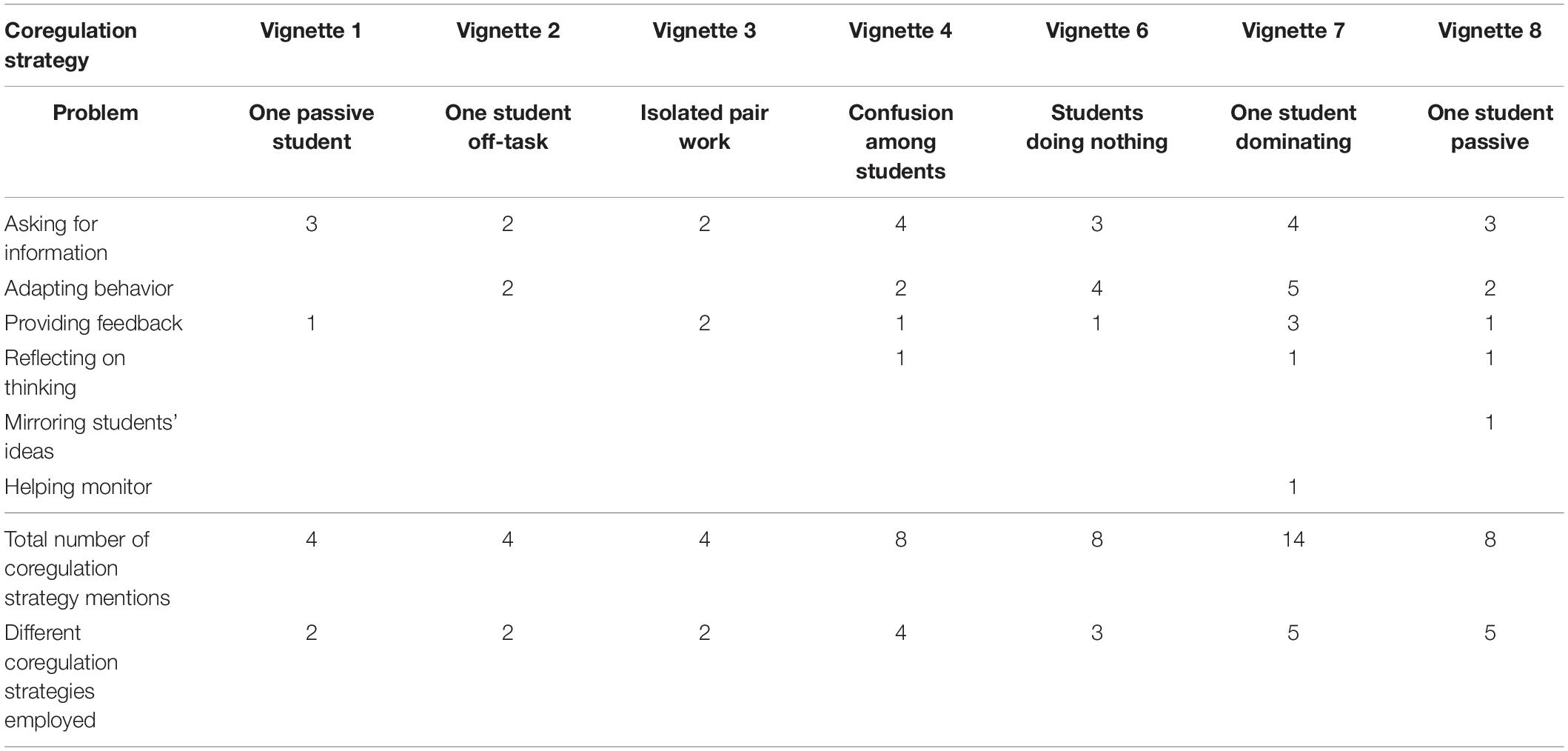
Table 6. The different coregulation strategies teachers used during the WoZ separated according to the vignettes.
Discussion
The Suggestions Provided by the Collaboration Intervention Model Are Understandable for Teachers
The reactions of the teachers to the suggestions were rather positive and the teachers even started employing the suggestions offered by the AI assistant in scenarios presented later. The results confirmed our initial idea that an AI assistant would be useful for teachers (see Figure 1). However, for some teachers, it was very difficult to form an opinion about the situation, as they were observing one group at a time and were not under very much pressure timewise. As some teachers indicated that they would have liked to have more time to look at the collaboration, the need for an AI assistant to monitor the progress of other groups while the teacher is focusing on only one group, becomes even more prevalent. Intelligence amplification entails providing teachers with data to inform their decision-making (Baker, 2016). This means that the AI assistant has the potential to conduct some analysis for the teacher, while still leaving the teacher in charge.
Teachers’ Pedagogical Actions Were Mostly Targeted at Specific Students
When we mapped the results of our study with the Situated Model of Instructional Decision-Making (Wise and Jung, 2019), it was apparent that the teachers in our study were hypothetically rather prone to intervene during the CL session. However, most of the interventions were targeted toward specific groups of students, whole-class scaffolding was rather marginal. This could possibly be explained by the fact that whole class teaching is considered to have a higher interactional complexity than teacher-student or small group teaching (Gegenfurtner et al., 2020). However, if several groups are struggling with a similar issue, but the teacher is unaware of it, an AI assistant could possibly detect this issue, alert the teacher, and when needed, increase the number of whole-class interventions.
The Artificial Intelligence Assistant Increases the Number of Pedagogical Actions Carried Out by Participating Teachers
Our study showed that an AI assistant supporting teachers during CL could make teachers take more pedagogical actions during lessons, which must be studied in authentic contexts. This confirms the notion expressed by Holstein et al. (2019a) that AI assistants might be even more effective if the strengths of AI and the teacher are combined. The students will not only get automated support, but also the teacher’s complementary strengths will be taken advantage of as their abilities to help their students are amplified. We also noted that even if some of the suggestions were not completely new to the teachers, the teachers were more prone to try the method out if they already had some previous knowledge about it. This could be connected to the notion of orchestration load, which is the level of exertion a teacher dedicates to coordinating multiple learning processes (Prieto et al., 2018). The orchestration load might be too big if the suggestions for the teachers are completely unknown.
Teachers Know Coregulation Strategies, but Do Not Use Them in Classrooms
Surprisingly, even if the knowledge of using roles in CL seems old and ubiquitous, the participating teachers in our study claimed that this is not something that is being exploited in the everyday classrooms of today. This is a classic case of research-practice gap typical to Computer Supported Collaborative Learning (Chan, 2011). Many teachers indicated that they know that roles could be used in CL, but they do not know how to do it. A methodological implication is the necessity for designing additional materials concerning different coregulation methods attached to the AI assistant for interested teachers. In addition to this, some of the participating teachers expressed the need for an introductory workshop before using the AI assistant. An implication for theory is for researchers who focus on improving collaboration among students to further elaborate the CIM that could inform AI. An implication for practice is for teachers to try and use the CIM even without the use of learning analytics or an AI assistant.
The Artificial Intelligence Affects the Teachers’ Understanding and Use of Coregulation Methods
When the teachers got the chance to interact with the AI assistant, the perception of the teacher’s role during CL changed slightly. Before interacting with the AI assistant, the teachers had the perception of the teachers simply monitoring the session in the background and intervening only when there was a colossal necessity. After analyzing the videos and assessing the collaboration, the teachers saw the increased importance of closely observing the students during collaboration and providing feedback on the process as well. The teachers started employing more coregulation strategies when they had been interacting with the AI assistant for some time, however, the teachers still mostly opted for providing feedback to the students and adapting the behavior during a CL session. It must be noted that some coregulation strategies proposed by Hadwin and Oshige (2011) like mirroring the students’ ideas, modeling thinking, and offering prompts for thinking and reflecting were either proposed once or not at all during the WoZ. This could mean that the teachers might not be aware of these strategies and thus an implication would be to introduce them to practicing teachers. We imagine that the AI assistant were to identify the problem according to the CIM with the help of machine learning models developed to classify the sub-dimensions of the collaboration quality. Our initial study of machine learning model development for collaboration showed that it’s feasible to estimate sub-dimensions using simple features (e.g., speaking time) from the data (Chejara et al., 2020). We have developed a web-based tool to conduct collaborative learning activities with monitoring functionality which captures students’ writing traces (if activity involves a collaborative text editor), perform voice activity detection and speech-to-text in real-time. These activity traces are then presented in a form of dashboard to the teacher. We envision an AI assistant integrated in this tool utilizing features (e.g., speaking time, turn taking based on voice activity detection, facial features, etc.) from the tool and feed those features to the developed machine learning models to classify the various sub-dimensions along with collaboration quality. On the basis of results, the AI assistant will give suggestions to the teacher from the CIM for the teacher to try out in the classroom. At the moment, we are in the process of data annotation of the collected data using collaboration rating scheme (Rummel et al., 2011). As the next step, we will train and evaluate the supervised machine learning models on the data. As the teacher could try out the solution in an authentic setting, this would be categorized as work-integrated learning.
The Artificial Intelligence Affects the Teacher’s Confidence and Understanding of Coregulating Collaborative Learning
While the teachers were rather insecure about their ability to provide strategies to aid collaborative learning before, after doing some thinking with the help of an AI assistant during the WoZ, the confidence had increased. Although the end goal of education and teachers is to create students who are self-regulating and sharing regulation in a collaborative setting, this is not something that happens without guidance. Coregulation is understood as a transitional process on the road of the learner becoming self-regulating (Hadwin and Oshige, 2011). A practical implication is to check whether the CIM provides teachers with strategies for mirroring the students’ ideas, requesting the students to reflect on their learning, modeling thinking, and offering prompts for thinking and reflecting. The results of our study demonstrate that some of the participating teachers did not perceive coregulation in a CL classroom as a responsibility of the teacher, which might affect the collaboration skills of the students. Research shows that a teacher can support students on the road to becoming students with socially shared regulation, but this does not happen by simply allowing the students absolute freedom in their collaboration, they need guidance and helpful strategies (Hadwin and Oshige, 2011; Järvelä and Hadwin, 2013). Our small-scale study showed that an AI assistant improved the understanding of coregulating CL for the participating teachers and AI has potential to improve collaboration.
The Teachers’ Experience Influences Their Understanding of Collaboration
Less experienced teachers in our study seemed to think they know about strategies supporting collaboration, but do not believe that a teacher needs to teach them to students. However, more experienced teachers feel they know less strategies and at the same time, they are certain that teachers are supposed to educate the students on different strategies to aid collaboration. After having been introduced to the AI assistant, less experienced teachers’ perceived knowledge on the matter decreased, whereas more experienced teachers realized they already are knowledgeable about some of the strategies that could support students working together. An implication for theory is whether this could be a matter of orchestration load. More novice teachers feel they are already overburdened with an abundance of tasks and are not capable of taking on any more responsibility, whereas more experienced teachers have automated some tasks and are able to focus on supporting the students more. Another possible explanation could be that less experienced teachers feel that students should be self-regulating learners and do not want to interfere with their learning process. Although the aim of collaboration is for students to eventually be self-regulating and socially sharing the regulation, we cannot expect them to simply start doing this on their own. As suggested earlier, coregulation is a transitional process (Hadwin and Oshige, 2011), which might not have been understood by less experienced teachers. A practical implication would be to offer courses on teaching in collaborative learning settings or providing coregulation strategy training at universities/training centers using AI assistants.
Limitations and Future Work
One of the limitations of the study entails having a small sample of twenty teachers, with seventeen teachers answering all the questions. Thus, we cannot generalize these results to other teacher populations yet. In addition, the multiple hypothesis testing problem needs to be considered. The pre- and post-test was composed based on theory, but the instrument needs to be validated in future studies due to the small sample. In addition to this, the questionnaire data could be triangulated with the use of a test or an interview to gain a deeper understanding of the attitudes of the teachers. Furthermore, we explored the factor of experience modulating the use of an AI assistant for teachers, but other factors like attitudes to technology, knowledge of CL techniques were left unexplored. In our analysis we saw that coregulation strategies varied more greatly and increased in number after the teachers had interacted with the AI assistant prototype a couple of times. However, we cannot be certain that some situations in the vignette were not inherently more prone to intervention than others. This could in the future be solved by randomizing the order of vignettes, which was not done this time. Another limitation is the question whether the interventions of the teachers would grow more varied by simply offering new ideas to the teacher through a workshop, training, etc., or whether using an AI assistant would actually be more effective. We need to point out that the teachers carried out their evaluations based on a model for an AI, rather than an actual implementation. The results might be very different from the teachers when an actual computer makes a suggestion. However, all measures were taken to maximize ecological validity of the study. Before conducting the WoZ protocol, the teachers were told that the following was not a test for them, but they were free to respond any way they felt like to the situations. The respondents were assured that there was no right or wrong answer they could be providing to avoid eliciting a socially desirable answer. All the teachers were in-service teachers who had some experience with conducting collaborative learning in their classrooms. The vignettes were video recorded in an authentic classroom and none of the teachers indicated that they were finding the situations unrealistic. Instead, they often started laughing and commenting on a similar behavior of their own students in their classrooms. On the other hand, the teachers were not interacting with a machine AI assistant, but they could see that the ideas were presented on a slip of paper. A methodological implication would be to provide longer vignettes with the option of the teacher stopping the video once they have made a decision. Another methodological implication would be to also study the perceived ease of use (Davis, 1989) which we chose not to do due to the participants not being able to interact with a real prototype (i.e., we focused on studying the perceived usefulness of the tool in this study). As a paper prototype was used for the purpose of the study, the next step in our DBR process is developing a prototype AI assistant based on the CIM to be tested in authentic settings. Then it could be seen whether the reaction of the teachers is similar to reaction using the paper prototype. In the future, we will explore experience, knowledge of coregulation strategies, experience in using CL, attitude to technology as factors moderating the use of an AI assistant.
We propose the following AI orchestration assistants’ design implications: (1) the teachers should be able to back-trace how the AI came to the potential problem to increase trust toward the system/analyses and help understand whether the assistant has made a mistake and allowing for the possibility of learning from the AI (and for the AI to learn from the teacher); (2) the (back-traceable) data needs to have pedagogical content (i.e., only showing time spent on task will not make teachers trust the dashboard); (3) the system needs to be adaptable and configurable, as the needs that a novice teacher has differ from a more experienced teacher, and other personalization aspects can also be relevant. We would also like to pose some open questions for the scientific community to further investigate: Can the use of an AI assistant help more novice teachers make decisions similarly to experienced teachers? Will an AI assistant increase the number and variety of pedagogical actions in a CL setting, or will it cause the teacher to abandon all analytical thinking and start to excessively trust the AI? What does the teacher need for such AI support to become as much a part of their natural pedagogical repertoire as a printer or a slideshow?
Conclusion
The purpose of the study was to investigate how teachers would use an AI assistant in synchronous face-to-face CL activities, whether teachers would find it useful and whether it would initiate pedagogical action, especially in terms of different coregulation strategies. The results show that the teachers found the AI assistant useful, and in several cases the teachers started employing the intervention strategies the AI assistant had introduced. The amount and variety of coregulation methods employed by teachers also increased toward the end of the study. However, most of the interventions were targeted toward specific groups of students, and whole-class scaffolding was rather marginal. Our analysis also explored the extent to which expertise can modulate the understanding of coregulation. It seems that less experienced teachers did not see coregulation as part of a teacher’s responsibility, while more experienced teachers did. Coregulation is a transitional process, where teachers with the help of an AI assistant could possibly help guide the students during collaboration better on the way of becoming self-regulating learners and learners with socially shared regulation. We propose using an AI assistant in CL to help teachers notice and analyze whether the potential problem proposed by AI is occurring and try out the intervention proposed by AI with students. With this, we are amplifying the teachers’ intelligence by increasing their awareness and offering a databank of interventions for coregulating CL.
Data Availability Statement
The raw data supporting the conclusions of this article will be made available by the authors, without undue reservation.
Ethics Statement
The studies involving human participants were reviewed and approved by CEITER Ethics Board at Tallinn University. The patients/participants provided their written informed consent to participate in this study. Written informed consent was obtained from the individual(s) for the publication of any potentially identifiable images or data included in this article.
Author Contributions
RK, LP, and TL contributed to the conception and design of the study. RK carried out the data collection, did the statistical analyses, and wrote the first draft of the manuscript. RK and PC did the qualitative analyses. All authors contributed to manuscript revision, read, and approved the submitted version
Funding
This work was partially funded by the CEITER project (htttp://ceiter.tlu.ee) under European Union’s Horizon 2020 Research and Innovation Programme grant agreement no. 669074. Open access was funded by the European Regional Development Fund through the project “TLU TEE or Tallinn University as the promoter of intelligent lifestyle” (No. 2014-2020.4.01.16-0033).
Conflict of Interest
The authors declare that the research was conducted in the absence of any commercial or financial relationships that could be construed as a potential conflict of interest.
Publisher’s Note
All claims expressed in this article are solely those of the authors and do not necessarily represent those of their affiliated organizations, or those of the publisher, the editors and the reviewers. Any product that may be evaluated in this article, or claim that may be made by its manufacturer, is not guaranteed or endorsed by the publisher.
References
Allal, L. (2020). Assessment and the co-regulation of learning in the classroom. Assess. Educ. Princ. Policy Pract. 27, 332–349. doi: 10.1080/0969594x.2019.1609411
Alumäe, T., and Tilk, O. A. (2018). “Advanced rich transcription system for estonian speech,” in Proceedings of the 8th International Conference, Baltic HLT 2018, Amsterdam.
Amarasinghe, I., Hernandez-Leo, D., Michos, K., and Vujovic, M. (2020). An actionable orchestration dashboard to enhance collaboration in the classroom. IEEE Trans. Learn. Technol. 13, 662–675. doi: 10.1109/TLT.2020.3028597
Baker, R. S. (2016). Stupid tutoring systems, intelligent humans. Int. J. Artif. Intell. Educ. 26, 600–614. doi: 10.1007/s40593-016-0105-0
Barkley, F. E., Major, C. H., and Cross, K. P. (2014). Collaborative Learning Techniques. A Handbook for College Faculty, 2nd Edn. San Fransisco, CA: Jossey-Bass. A Wiley Brand.
Baumgartner, E., Bell, P., Brophy, S., Hoadley, C., Hsi, S., Joseph, D., et al. (2003). Design-based research: an emerging paradigm for educational inquiry. Educ. Res. 32, 5–8. doi: 10.3102/0013189X032001005
Chan, C. K. K. (2011). Bridging research and practice: implementing and sustaining knowledge building in Hong Kong classrooms. Int. J. Comput. Support. Collaborat. Learn. 6, 147–186. doi: 10.1007/s11412-011-9121-0
Chejara, P., Prieto, L. P., Ruiz-Calleja, A., Rodríguez-Triana, M. J., Shankar, S. K., and Kasepalu, R. (2021). EFAR-MMLA: an evaluation framework to assess and report generalizability of machine learning models in mmla. Sensors 21, 1–27. doi: 10.3390/s21082863
Chejara, P., Prieto, L. P., Ruiz-Calleja, A., Shankar, S. K., and Kasepalu, R. (2020). “Quantifying collaboration quality in face-to-face classroom settings using MMLA,” in Collaboration Technologies and Social Computing. CollabTech 2020, Vol. 1, eds A. Nolte, C. Alvarez, R. Hishiyama, I. A. Chounta, M. Rodríguez-Triana, and T. Inoue (Cham: Springer), 159–166.
Dahlbäck, N., Jönsson, A., and Ahrenberg, L. (1993). “Wizard of Oz studies: why and how?,” in Proceedings of the 1st international conference on Intelligent user interfaces, New York, NY, 193–200. doi: 10.1109/TVCG.2017.2744198
Davis, F. D. (1989). Perceived usefulness, perceived ease of use, and user acceptance of information technology. MIS Q. Manag. Inf. Syst. 13, 319–339. doi: 10.2307/249008
Dazo, S. L., Stepanek, N. R., Chauhan, A., and Dorn, B. (2017). “Examining instructor use of learning analytics,” in Proceedings of the 2017 CHI Conference Extended Abstracts on Human Factors in Computing Systems, New York, NY, 2504–2510. doi: 10.1145/3027063.3053256
de Bono, E. (2000). Six Thinking Hats: Run Better Meetings, Make Faster Decisions. London: Penguin Life.
Gegenfurtner, A., Lewalter, D., Lehtinen, E., Schmidt, M., and Gruber, H. (2020). Teacher expertise and professional vision: examining knowledge-based reasoning of pre-service teachers, in-service teachers, and school principals. Front. Educ. 5:59. doi: 10.3389/feduc.2020.00059
Geiger, M., Waizenegger, L., Treasure-Jones, T., Sarigianni, C., Maier, R., Thalmann, S., et al. (2017). “Not just another type of resistance - towards a deeper understanding of supportive non-use,” in Proceedings of the 25th European Conference on Information Systems (ECIS) June 5-10, 2017, Guimarães, 2366–2381.
Hadwin, A., and Oshige, M. (2011). Self-regulation, coregulation, and socially shared regulation: exploring perspectives of social in self-regulated learning theory. Teach. Coll. Rec. 113, 240–264. doi: 10.1177/016146811111300204
Holstein, K., Harpstead, E., Gulotta, R., and Forlizzi, J. (2020). “Replay enactments: exploring possible futures through historical data,” in Proceedings of the 2020 ACM Designing Interactive Systems Conference, - DIS 2020, New York, NY, 1607–1618. doi: 10.1145/3357236.3395427
Holstein, K., McLaren, B. M., and Aleven, V. (2019b). Designing for Complementarity: Teacher and Student Needs for Orchestration Support in AI-Enhanced Classrooms. Cham: Springer International Publishing, doi: 10.1007/978-3-030-23204-7_14
Holstein, K., McLaren, B. M., and Aleven, V. (2019a). Co-designing a real-time classroom orchestration tool to support teacher–AI complementarity. J. Learn. Anal. 6, 27–52. doi: 10.18608/jla.2019.62.3
Jackson, D. (2015). Employability skill development in work-integrated learning: barriers and best practice. Stud. High. Educ. 40, 350–367. doi: 10.1080/03075079.2013.842221
Jacques, D. (2000). Learning in Groups: A Handbook for Improving Group Working. Melbourne, VIC: Kogan Page.
Järvelä, S., and Hadwin, A. F. (2013). New frontiers: regulating learning in CSCL. Educ. Psychol. 48, 25–39. doi: 10.1080/00461520.2012.748006
Johnson, D. W., and Johnson, R. T. (2017). “Cooperative learning,” in Innovación Educación. I Congresso Internacional, Vol. 12. ed. Gobieno de Aragon (Zaragoza: Gobieno de Aragon).
Johnson, D. W., Johnson, R. T., and Smith, K. A. (2014). Cooperative learning: improving university instruction by basing practice on validated theory. J. Excell. Coll. Teach. 25, 85–118. doi: 10.1046/j.1365-2923.1997.00671.x
Jørnø, R. L., and Gynther, K. (2018). What constitutes an ‘actionable insight’ in learning analytics? J. Learn. Anal. 5, 198–221. doi: 10.18608/jla.2018.53.13
Kasepalu, R., Chejara, P., Prieto, L. P., and Ley, T. (2021). Do teachers find dashboards trustworthy, actionable and useful? A vignette study using a logs and audio. Technol. Knowl. Learn. 19. doi: 10.1007/s10758-021-09522-5
Kasepalu, R., Prieto, P. L., and Ley, T. (2019). “Providing teachers with individual and group-level collaboration analytics: a paper prototype,” in in International Workshop on Collaboration Analytics: Making Learning Visible in Collaborative Settings. (Lyon: CSCL). Available online at: https://collaborationanalytics.files.wordpress.com/2019/06/submission-2-reet.pdf. (accessed January 31, 2022).
Kelley, J. (1984). “An iterative design methodology for user-friendly natural language information applications,” in Proceedings of the Institution of Mechanical Engineers, Part M: Journal of Engineering for the Maritime Environment, Vol. 2, New York, NY, 26–41.
Ley, T. (2019). Knowledge structures for integrating working and learning: a reflection on a decade of learning technology research for workplace learning. Br. J. Educ. Technol. 51, 331–346. doi: 10.1111/bjet.12835
Liu, A. L., and Nesbit, J. C. (2020). Dashboards for Computer-Supported Collaborative Learning. Cham: Springer International Publishing, doi: 10.1007/978-3-319-47194-5_5
Martinez-Maldonado, R., Clayphan, A., Yacef, K., and Kay, J. (2015). MTFeedback: providing notifications to enhance teacher awareness of small group work in the classroom. IEEE Trans. Learn. Technol. 8, 187–200. doi: 10.1109/TLT.2014.2365027
Molenaar, I., and Knoop-Van Campen, C. A. N. (2019). How teachers make dashboard information actionable. IEEE Trans. Learn. Technol. 12, 347–355. doi: 10.1109/TLT.2018.2851585
Prieto, L. P., Sharma, K., Kidzinski, Ł, and Dillenbourg, P. (2018). Orchestration load indicators and patterns: in-the-wild studies using mobile eye-tracking. IEEE Trans. Learn. Technol. 11, 216–229. doi: 10.1109/TLT.2017.2690687
Rummel, N., Deiglmayr, A., Spada, H., Kahrimanis, G., and Avouris, N. (2011). “Analyzing collaborative interactions across domains and settings: an adaptable rating scheme,” in Analyzing Interactions in CSCL, Vol. 12, eds S. Puntambekar, G. Erkens, and C. Hmelo-Silver (Cham: Springer), 367–390.
Schlögl, S., Doherty, G., and Luz, S. (2015). Wizard of Oz experimentation for language technology applications: challenges and tools. Interact. Comput. 27, 592–615. doi: 10.1093/iwc/iwu016
Schwarz, B. B., and Asterhan, C. S. (2011). E-moderation of synchronous discussions in educational settings: a nascent practice. J. Learn. Sci. 20, 395–442. doi: 10.1080/10508406.2011.553257
Seidel, T., and Stürmer, K. (2014). Modeling and measuring the structure of professional vision in preservice teachers. Am. Educ. Res. J. 51, 739–771. doi: 10.3102/0002831214531321
Smith, P. J. (2003). Workplace learning and flexible delivery. Rev. Educ. Res. 73, 53–88. doi: 10.3102/00346543073001053
Taherdoost, H. (2019). What is the best response scale for survey and questionnaire design; review of different lengths of rating scale / attitude scale / likert scale. Int. J. Acad. Res. Manag. 8, 1–10.
TALIS (2018). Teaching and Learning International Survey: Insights and Interpretations. Available online at: http://www.oecd.org/education/talis/TALIS2018_insights_and_interpretations.pdf (accessed January 31, 2022).
Tarmazdi, H., Vivian, R., Szabo, C., Falkner, K., and Falkner, N. (2015). “Using learning analytics to visualise computer science teamwork,” in Proceedings of the 2015 ACM Conference on Innovation and Technology in Computer Science Education, ITiCS 2015, Vilnius, 165–170. doi: 10.1145/2729094.2742613
van Leeuwen, A. (2015). Learning analytics to support teachers during synchronous CSCL: balancing between overview and overload. J. Learn. Anal. 2, 138–162. doi: 10.18608/jla.2015.22.11
van Leeuwen, A., and Janssen, J. (2019). A systematic review of teacher guidance during collaborative learning in primary and secondary education. Educ. Res. Rev. 27, 71–89. doi: 10.1016/j.edurev.2019.02.001
van Leeuwen, A., and Rummel, N. (2019). Orchestration tools to support the teacher during student collaboration: a review. Unterrichtswissenschaft 47, 143–158. doi: 10.1007/s42010-019-00052-9
van Leeuwen, A., and Rummel, N. (2020). “Comparing teachers’ use of mirroring and advising dashboards,” in Proceedings of the 10th International Conference on Learning Analytics & Knowledge (LAK’20). (New York, NY: ACM), 26–34. doi: 10.1145/3375462.3375471
van Leeuwen, A., Janssen, J., Erkens, G., and Brekelmans, M. (2014). Supporting teachers in guiding collaborating students: effects of learning analytics in CSCL. Comput. Educ. 79, 28–39. doi: 10.1016/j.compedu.2014.07.007
Wise, A. F., and Jung, Y. (2019). Teaching with analytics: towards a situated model of instructional decision-making. J. Learn. Anal. 6, 53–69. doi: 10.18608/jla.2019.62.4
Worsley, M., Anderson, K., Melo, N., and Jang, J. (2021). Designing analytics for collaboration literacy and student empowerment. J. Learn. Anal. 8, 30–48. doi: 10.18608/jla.2021.7242
Appendix 1
Keywords: collaborative learning (CL), artificial intelligence (AI), pedagogical actions, teacher intervention, coregulation, mixed methods, collaboration analytics, Wizard-of-Oz
Citation: Kasepalu R, Prieto LP, Ley T and Chejara P (2022) Teacher Artificial Intelligence-Supported Pedagogical Actions in Collaborative Learning Coregulation: A Wizard-of-Oz Study. Front. Educ. 7:736194. doi: 10.3389/feduc.2022.736194
Received: 04 July 2021; Accepted: 26 January 2022;
Published: 17 February 2022.
Edited by:
Claudia Müller-Birn, Freie Universität Berlin, GermanyReviewed by:
Bob Edmison, Virginia Tech, United StatesYacine Lafifi, 8 May 1945 University of Guelma, Algeria
Marc Berges, Friedrich-Alexander-Universität Erlangen-Nürnberg, Germany
Copyright © 2022 Kasepalu, Prieto, Ley and Chejara. This is an open-access article distributed under the terms of the Creative Commons Attribution License (CC BY). The use, distribution or reproduction in other forums is permitted, provided the original author(s) and the copyright owner(s) are credited and that the original publication in this journal is cited, in accordance with accepted academic practice. No use, distribution or reproduction is permitted which does not comply with these terms.
*Correspondence: Reet Kasepalu, cmVldGthc2VAdGx1LmVl