- 1Department of Mathematics, University of California, San Diego, San Diego, CA, United States
- 2Halıcıoǧlu Data Science Institute, University of California, San Diego, San Diego, CA, United States
- 3Joint Doctoral Program in Math and Science Education, San Diego State University, San Diego, CA, United States
- 4Joint Doctoral Program in Math and Science Education, University of California, San Diego, San Diego, CA, United States
- 5Department of Physical Therapy, Movement and Rehabilitation Science, Bouvé College of Health, Northeastern University, Boston, MA, United States
- 6Art and Design, College of Arts, Media and Design, Northeastern University, Boston, MA, United States
- 7Qualcomm Institute, University of California, San Diego, San Diego, CA, United States
The COVID-19 pandemic resulted in nearly all universities switching courses to online formats. We surveyed the online learning experience of undergraduate students (n = 187) at a large, public research institution in course structure, interpersonal interaction, and academic resources. Data was also collected from course evaluations. Students reported decreases in live lecture engagement and attendance, with 72 percent reporting that low engagement during lectures hurt their online learning experience. A majority of students reported that they struggled with staying connected to their peers and instructors and managing the pace of coursework. Students had positive impressions, however, of their instructional staff. Majorities of students felt more comfortable asking and answering questions in online classes, suggesting that there might be features of learning online to which students are receptive, and which may also benefit in-person classes.
Introduction
In Spring 2020, 90% of higher education institutions in the United States canceled in-person instruction and shifted to emergency remote teaching (ERT) due to the COVID-19 pandemic (Lederman, 2020). ERT in response to COVID-19 is qualitatively different from typical online learning instruction as students did not self-select to participate in ERT and teachers were expected to transition to online learning in an unrealistic time frame (Brooks et al., 2020; Hodges et al., 2020; Johnson et al., 2020). This abrupt transition left both faculty and students without proper preparation for continuing higher education in an online environment.
In a random sample of 1,008 undergraduates who began their Spring 2020 courses in-person and ended them online, 51% of respondents said they were very satisfied with their course before the pandemic, and only 19% were very satisfied after the transition to online learning (Means and Neisler, 2020). Additionally, 57% of respondents said that maintaining interest in the course material was “worse online,” 65% claimed they had fewer opportunities to collaborate with peers, and 42% said that keeping motivated was a problem (Means and Neisler, 2020). Another survey of 3,089 North American higher education students had similar results with 78% of respondents saying online experiences were not engaging and 75% saying they missed face-to-face interactions with instructors and peers (Read, 2020). Lastly, of the 97 university presidents surveyed in the United States by Inside Higher Ed, 81% claimed that maintaining student engagement would be challenging when moving classes online due to COVID-19 (Inside Higher Ed, 2020).
In this report, we consider the measures and strategies that were implemented to engage students in online lectures at UCSD during ERT due to the COVID-19 pandemic. We investigate student perceptions of these measures and place our findings in the larger context of returning to in-person instruction and improving engagement in both online and in-person learning for undergraduates. Before diving into the current study, we first define what we mean by engagement.
Theoretical Framework and Literature Review
Student Engagement
Student engagement has three widely accepted dimensions: behavioral, cognitive and affective (Chapman, 2002; Fredricks et al., 2004, 2016; Mandernach, 2015). Each dimension has indicators (Fredricks et al., 2004), or facets (Coates, 2007), that manifest each dimension. Behavioral engagement refers to active responses to learning activities and is indicated by participation, persistence, and/or positive conduct. Cognitive engagement includes mental effort in learning activities and is indicated by deep learning, self-regulation, and understanding. Affective engagement is the emotional investment in learning activities and is indicated by positive reactions to the learning environment, peers, and teachers as well as a sense of belonging. A list of indicators for each dimension can be found in Bond et al. (2020).
The literature also theorizes different influences for each engagement dimension. Most influencing factors are sociocultural in nature and can include the political, social, and teaching environment as well as relationships within the classroom (Kahu, 2013). In particular, social engagement with peers and instructors creates a sense of community, which is often correlated with more effective learning outcomes (Rovai and Wighting, 2005; Liu et al., 2007; Lear et al., 2010; Kendricks, 2011; Redmond et al., 2018; Chatterjee and Correia, 2020). Three key classroom interactions are often investigated when trying to understand the factors influencing student engagement: student-student interactions, student-instructor interactions, and student-content interactions (Moore, 1993).
Student-student interactions prevent boredom and isolation by creating a dynamic sense of community (Martin and Bolliger, 2018). Features that foster student-student interactions in online learning environments include group activities, peer assessment, and use of virtual communication spaces such as social media, chat forums, and discussion boards (Revere and Kovach, 2011; Tess, 2013; Banna et al., 2015). In the absence of face-to-face communication, these virtual communication spaces help build student relationships (Nicholson, 2002; Harrell, 2008). In a survey of 1,406 university students in asynchronous online courses, the students claimed to have greater satisfaction and to have learned more when more of the course grade was based on discussions, likely because discussions fostered increased student-student and student-instructor interactions (Shea et al., 2001). Interestingly, in another study, graduate students in online courses claimed that student-student interactions were the least important of the three for maintaining student engagement, but that they were more likely to be engaged if an online course had online communication tools, ice breakers, and group activities (Martin and Bolliger, 2018).
In the Martin and Bolliger (2018) study, the graduate students enrolled in online courses found student-instructor interactions to be the most important of the three interaction types, which supports prior work that found students perceive student-instructor interactions as more important than peer interactions in fostering engagement (Swan and Shih, 2005). Student-instructor interactions increased in frequency in online classes when the following practices were implemented (1) multiple open communication channels between students and instructors (Gaytan and McEwen, 2007; Dixson, 2010; Martin and Bolliger, 2018), (2) regular communication of announcements, reminders, grading rubrics, and expectations by instructors (Martin and Bolliger, 2018), (3) timely and consistent feedback provided to students (Gaytan and McEwen, 2007; Dixson, 2010; Chakraborty and Nafukho, 2014; Martin and Bolliger, 2018), and (4) instructors taking a minimal role in course discussions (Mandernach et al., 2006; Dixson, 2010).
Student-content interactions include any interaction the student has with course content. Qualities that have been shown to increase student engagement with course content include the use of curricular materials and classroom activities that incorporate realistic scenarios, prompts that scaffold deep reflection and understanding, multimedia instructional materials, and those that allow student agency in choice of content or activity format (Abrami et al., 2012; Wimpenny and Savin-Baden, 2013; Britt et al., 2015; Martin and Bolliger, 2018). In online learning, students need to be able to use various technologies in order to be able to engage in student-content interactions, so technical barriers such as lack of access to devices or reliable internet can be a substantial issue that deprives educational opportunities especially for students from lower socioeconomic households (Means and Neisler, 2020; Reich et al., 2020; UNESCO, 2020).
Engagement in Online Learning
Bond and Bedenlier (2019) present a theoretical framework for engagement in online learning that combines the three dimensions of engagement, types of interactions that can influence the engagement dimensions, and possible short term and long term outcomes. The types of interactions are based on components present in the student’s immediate surrounding or microsystem, and are largely based on Moore’s three types of interactions: teachers, peers, and curriculum. However, the authors add technology and the classroom environment as influential components because they are particularly important for online learning.
Specific characteristics of each microsystem component can differentially modulate student engagement, and each component has at least one characteristic that specifically focuses on technology. Teacher presence, feedback, support, time invested, content expertise, information and communications technology skills and knowledge, technology acceptance, and use of technology all can influence the types of interactions students might have with their teachers which would then impact their engagement (Zhu, 2006; Beer et al., 2010; Zepke and Leach, 2010; Ma et al., 2015; Quin, 2017). For curriculum/activities, the quality, design, difficulty, relevance, level of required collaboration, and use of technology can influence the types of interactions a student might encounter that could impact their engagement (Zhu, 2006; Coates, 2007; Zepke and Leach, 2010; Bundick et al., 2014; Almarghani and Mijatovic, 2017; Xiao, 2017). Characteristics that can change the quantity and quality of peer interactions and thereby influence engagement include the amount of opportunities to collaborate, formation of respectful relationships, clear boundaries and expectations, being able to physically see each other, and sharing work with others and in turn respond to the work of others (Nelson Laird and Kuh, 2005; Zhu, 2006; Yildiz, 2009; Zepke and Leach, 2010). When describing influential characteristics, the authors combine classroom environment and technology because in online learning, the classroom environment inherently utilizes technology. The influential characteristics of these two components are access to technology, support in using and understanding technology, usability, design, technology choice, sense of community, and types of assessment measures. All of these characteristics demonstrably influenced engagement levels in prior literature (Zhu, 2006; Dixson, 2010; Cakir, 2013; Levin et al., 2013; Martin and Bolliger, 2018; Northey et al., 2018; Sumuer, 2018).
Online learning can take place in different formats, including fully synchronous, fully asynchronous, or blended (Fadde and Vu, 2014). Each of these formats offers different challenges and opportunities for technological ease, time management, community, and pacing. Fully asynchronous learning is time efficient, but offers less opportunity for interactions that naturally take place in person (Fadde and Vu, 2014). Instructors and students may feel underwhelmed by the lack of immediate feedback that can happen in face to face class time (Fadde and Vu, 2014). Synchronous online learning is less flexible for teachers and students and requires reliable technology, but allows for more real time engagement and feedback (Fadde and Vu, 2014). In blended learning courses, instructors have to coordinate and organize both the online and in person meetings and lessons, which is not as time efficient. Blended learning means there is some in person engagement which provides spontaneity and more natural personal relations (Fadde and Vu, 2014). In all online formats, students may feel isolated and instructors and students need to spend more time and intention into building community (Fadde and Vu, 2014; Gillett-Swan, 2017). Often, instructors can use learning management systems and discussion boards to help facilitate student interaction and connection (Fadde and Vu, 2014). In terms of group work, engagement and participation is dependent not only on the modality of learning, but also the instructors expectations for assessment (Gillett-Swan, 2017). Given the flexibility and power of online meeting and work environments, collaborating synchronously or asynchronously are both possible and effective (Gillett-Swan, 2017). In online learning courses, especially fully asynchronous, students are more accountable for their learning, which may be challenging for students who struggle with self-regulating their work pace (Gillett-Swan, 2017). Learning from home also means there are more distractions than when students attend class on campus. At any point during class, children, pets, or work can interrupt a student’s, or instructor’s, remote learning or teaching (Fadde and Vu, 2014).
According to Raes et al. (2019), the flexibility of a blended -or hybrid- learning environment encourages more students to show up to class when they otherwise would have taken a sick day, or would not have been able to attend due to home demands. It also equalizes learning opportunities for underrepresented groups, and more comprehensive support with two modes of interaction. On the other hand, hybrid learning can cause more strain on the instructor who may have to adapt their teaching designs for the demands of this unique format while maintaining the same standards (Bülow, 2022). Due to the nature of class, some students can feel more distant to the instructor and to each other, and in many cases active class participation was difficult in hybrid learning environments. Although Bulow’s review (2022) focused on the challenges and opportunities of designing effective hybrid learning environments for the teacher, it follows that students participating in different environments will also need to adapt to foster effective active participation environments that encompass both local and remote learners.
Engagement in Emergency Remote Instruction During COVID-19
There is currently a thin literature on student perceptions of the efficacy of ERT strategies and formats in engaging students during COVID-19. Indeed, student perceptions about online learning do not indicate actual learning. This study considers student perceptions for the purpose of gathering information about what conditions help or hinder students’ comfort with engaging in online classes toward the goal of designing improved online learning opportunities in the future. The large scale surveys of undergraduate students had some items relating to engagement, but these surveys aimed to generally understand the student experience during the transition to COVID-19 induced ERT (Means and Neisler, 2020; Read, 2020). A few small studies have surveyed or interviewed students from a single course on their perceptions of the changes made to courses to accommodate ERT (Senn and Wessner, 2021), the positives and negatives of ERT (Hussein et al., 2020), or the changes in their participation patterns and the course structures and instructor strategies that increase or decrease engagement in ERT (Perets et al., 2020). In their survey of 73 students across the United States, Wester and colleagues specifically focused on changes to students’ cognitive, affective, and behavioral engagement due to COVID-19 induced ERT, but they did not inquire as to what were the key influencing factors for these changes. Walker and Koralesky (2021) and Shin and Hickey (2021) surveyed students from a single institution but from multiple courses and thus are most relevant to the current study. These studies aimed to understand the students’ perceptions of their engagement and influencing factors of engagement at a single institution, but they did not assess how often these factors were implemented at that institution.
The current study investigates the engagement strategies used in a large, public, research institution, students’ opinions about these course methods, and students’ overall perception of learning in-person versus during ERT. This study aims to answer the following questions:
1. How has the change from in-person to online learning affected student attendance, performance expectations of students, and participation in lectures?
2. What engagement tools are being utilized in lectures and what do students think about them?
3. What influence do social interactions with peers, teachers, and administration have on student engagement?
These three questions encompass the three different dimensions of engagement, including multiple facets of each, as well as explicitly highlighting the role of technology in student engagement.
Materials and Methods
Data were collected from two main sources: a survey of undergraduates, and Course and Professor Evaluations (CAPE). The study was deemed exempt from further review by the institution’s Institutional Review Board because identifying information was not collected.
Survey
The survey consisted of 50 questions, including demographic information as well as questions about both in-person and online learning (Refer to full survey in Supplementary Material.). The survey, hosted on Qualtrics, was distributed to undergraduate students using various social media channels, such as Reddit, Discord, and Facebook, in addition to being advertised in some courses. In total, the survey was answered by 237 students, of which 187 completed the survey in full, between January 26th and February 15, 2021. It was made clear to students that the data collected would be anonymous and used to assess engagement over the course of Fall 2019 to Fall 2020. The majority of the survey was administered using five-point Likert scales of agreement, frequency, and approval. The survey was divided into blocks, each of which used the same Likert scale. Quantitative analysis of the survey data was conducted using R, and visualized with the likert R package (Bryer and Speerschneider, 2016).
A number of steps were taken to ensure that survey responses were valid. Before survey distribution, 2 cognitive interviews were conducted with undergraduate students attending the institution in order to refine the intelligibility of survey items (Desmione and Carlson Le Floch, 2004). Forty-eight incomplete surveys were excluded. In addition, engagement tests were placed within the larger blocks of the survey in order to prevent respondents from clicking the same choice repeatedly without reading the prompts. The two students who answered at least one of these questions incorrectly were excluded.
Respondent Profile
Respondents were asked before the survey to confirm that they were undergraduate students attending the institution over the age of 18. Among the 187 students that filled out the survey in its entirety, 21.9% were in their first year, 28.3% in their second year, 34.2% in their third year, 11.8% in their fourth year, and 1.1% in their fifth year or beyond. It should be noted, therefore, that some students, especially first-years, had no experience with in-person college education at the institution, and these respondents were asked to indicate this for any questions about in-person learning. However, all students surveyed were asked before participating whether they had experience with online learning at the institution. 2.7% of respondents were first year transfers. 72.7% of overall respondents identified as female, 25.7% as male, 0.5% as non-binary, and 1.1% preferred not to disclose gender. In regards to ethnicity, 45.6% of respondents identified as Asian, 22.8% as White, 13.9% as Hispanic/Latinx, 1.7% as Middle Eastern, 1.6% as Black or African-American, and 2.2% as Other. 27.9% of respondents were first-generation college students, 7.7% of respondents were international students, and 9.9% of students were transfer students.
In the most recent report for the 2020–2021 academic year, the Institutional Research Department noted that out of 31,842 undergraduates, 49.8% of undergraduates are women and 49.4% are men (University of California, San Diego Institutional Research, 2021). This report states that 17% of undergraduates are international students, which is a larger percentage than is represented by survey respondents (University of California, San Diego Institutional Research, 2021). The institution reports 33% of undergraduates are transfer students, which are also underrepresented in the survey respondents (University of California San Diego [UCSD], 2021b). The ethnicity profile of the survey respondents is similar to the undergraduate student demographic at this institution. According to the institutional research report, among undergraduates, 37.1% are Asian American, 19% are White, 20.8% are Chicano/Latino, 3% are African American, 0.4% are American Indian, and 2.5% are missing data on ethnicity (University of California, San Diego Institutional Research, 2021).
Course and Professor Evaluation Reviews
Data were also collected from the institution’s CAPE reviews, a university-administered survey offered prior to finals week every quarter, in which undergraduate students are asked to rate various aspects of their experience with their undergraduate courses and professors (Courses not CAPEd for Winter 22, 2022). CAPE reviews are anonymous, but are sometimes incentivized by professors to increase participation.
Although it was not designed with Bond and Bedenlier’s student engagement framework in mind, the questions on the CAPE survey still address the fundamental influences on engagement established by the framework. The CAPE survey asks students how many hours a week they spend studying outside of class, the grade they expect to receive, and whether they recommend the course overall. The survey then asks questions about the professor, such as whether they explain material well, show concern for student learning, and whether the student recommends the professor overall.
In this study, we chose to look only at data from Fall 2019, a quarter where education was in-person, and Fall 2020, when courses were online. In Fall 2019, there were 65,985 total CAPE reviews submitted, out of a total of 114,258 course enrollments in classes where CAPE was made available, for a total response rate of 57.8% (University of California San Diego [UCSD], 2021a). The mean response rate within a class was 53.1% with a standard deviation of 20.7%. In Fall 2020, there were 65,845 CAPE responses out of a total of 118,316 possible enrollments, for a total response rate of 55.7%. The mean response rate within a class was 50.7%, with a standard deviation of 19.6%.
In order to adjust for the different course offerings between quarters, and for the different professors who might teach the same course, we selected only CAPE reviews for courses that were offered in both Fall 2019 and Fall 2020 with the same professors. This dataset contained 31,360 unique reviews (16,147 from Fall 2019 and 15,213 from Fall 2020), covering 587 class sections in Fall 2019 and 630 in Fall 2020. Since no data about the students were provided with the set, however, we do not know how many students these 31,360 reviews represent. This pairing strategy offers many interesting opportunities to compare the changes and consistencies of student reviews between both quarters in question. To keep this study focused on the three research questions and in observation of time and space limitations, analysis was only performed on the pairwise level of the general CAPE survey questions and not broken down to further granularity.
The CAPE survey was created by the designers of CAPE, not the researchers of this paper. The questions on the CAPE survey are general and only provide a partial picture of the status of student engagement in Fall 2019 and Fall 2020. The small scale survey created by this research team attempts to clarify and make meaning of the results from the CAPE data.
Data Analysis
Survey Data
Survey data was collected and exported from Qualtrics as a. csv file, then manually trimmed to include only relevant survey responses from participants who completed the survey. Data analysis was done in R using the RStudio interface, with visualizations done using the likert and ggplot2 R packages (Bryer and Speerschneider, 2016; Wickham, 2016; R Core Team, 2020; RStudio Team, 2020). Statistical tests were performed on lecture data, using paired t-tests, and Mann–Whitney U tests of the responses; for example, when comparing attendance of in-person lectures in Fall 2019 and live online lectures on Zoom in Fall 2020.
Course and Professor Evaluation Data
As previously mentioned, analysis of CAPE reviews was restricted to courses that were offered in both Fall 2019 and 2020 with the same professor, with Fall 2019 courses being in-person and Fall 2020 courses being online. This was done since the variation of interest is the change from in-person to online education, and restricting analysis to these courses allowed the pairing of specific courses for statistical tests, as well as the adjustment for any differences in course offerings or professor choices between the two quarters. In order to compare ratings for a specific item, first, negative items were recoded if necessary. The majority of questions were on a 5-point Likert scale, though some, such as expected grade, needed conversion from categorical (A–F scale) to numerical (usually 0–4). Then, the two-sample Mann–Whitney U test was conducted on the numerical survey answers, comparing the results from Fall 2019 to those from Fall 2020. Results were then visualized using the R package ggplot2 (Wickham, 2016), as well as the likert package (Bryer and Speerschneider, 2016).
Results
In this study, we aimed to take a broad look at the state of online learning at UCSD as compared to in-person learning before the COVID-19 pandemic. This assessment was split into three general categories: changes in lecture engagement and student performance, tools that professors and administrators have implemented in the face of online learning, and changes in patterns of students’ interactions with their peers and with instructors. In general, while we found that students’ ratings of their professors and course staff remained positive, there were significant decreases in lecture engagement, attendance, and perceived ability to keep up with coursework, even as expected grades rose. In addition, student-student interactions fell for the vast majority of students, which students felt hurt their learning experience.
Course and Professor Evaluation Results
How Has the Change From in-Person to Online Learning Affected Student Attendance, Performance Expectations, and Participation in Lectures?
Lecture Attendance
In the CAPE survey, students reported their answers to a series of questions relating to lecture attendance and engagement. Table 1 reports the results of the Mann–Whitney U test for each question, in which the results from Fall 2019 were compared to the results from Fall 2020. Statistically significant differences were found between students’ responses to the question “How often do you attend this course?” (rated on a 1–3 scale of Very Rarely, Some of the Time, and Most of the Time), although students were still most likely to report that they attended the class most of the time. Statistically significant decreases were also found for students’ agreement to the questions “Instructor is well-prepared for classes,” and “Instructor starts and finishes classes on time.” It should be noted that “attendance” was not clarified as “synchronous” or “asynchronous” attendance to survey respondents.

Table 1. Mean and standard deviations of student responses on CAPE evaluation questions relating to lecture attendance and engagement in Fall 2019 and 2020.
Expected Grades
Within the CAPE survey, students are asked, “What grade do you expect in this class?” The given options are A, B, C, D, F, Pass, and No Pass. The proportion of CAPE responses in which students reported taking the course Pass/No Pass stayed relatively constant from Fall 2019 to Fall 2020, going from 6.5% in Fall 2019 to 6.4% in Fall 2020. As can be seen in Figure 1, participants were more likely to expect A’s in Fall 2020; in Fall 2019, the median expected grade was an A in 56.8% of classes, while in Fall 2020, this figure was 68.0%. We used a Mann–Whitney U test to test our hypothesis that there would be a difference between Fall 2019 and Fall 2020 expected grades because of students’ and instructors’ unfamiliarity with the online modality. When looking solely at classes in which students expected to receive a letter grade, after recoding letter grades to GPA equivalents, a significant difference was found between expected grades in Fall 2019 and 2020, with a mean of 3.443 in FA19 and 3.538 in FA20 (U = 92286720, p < 0.001).
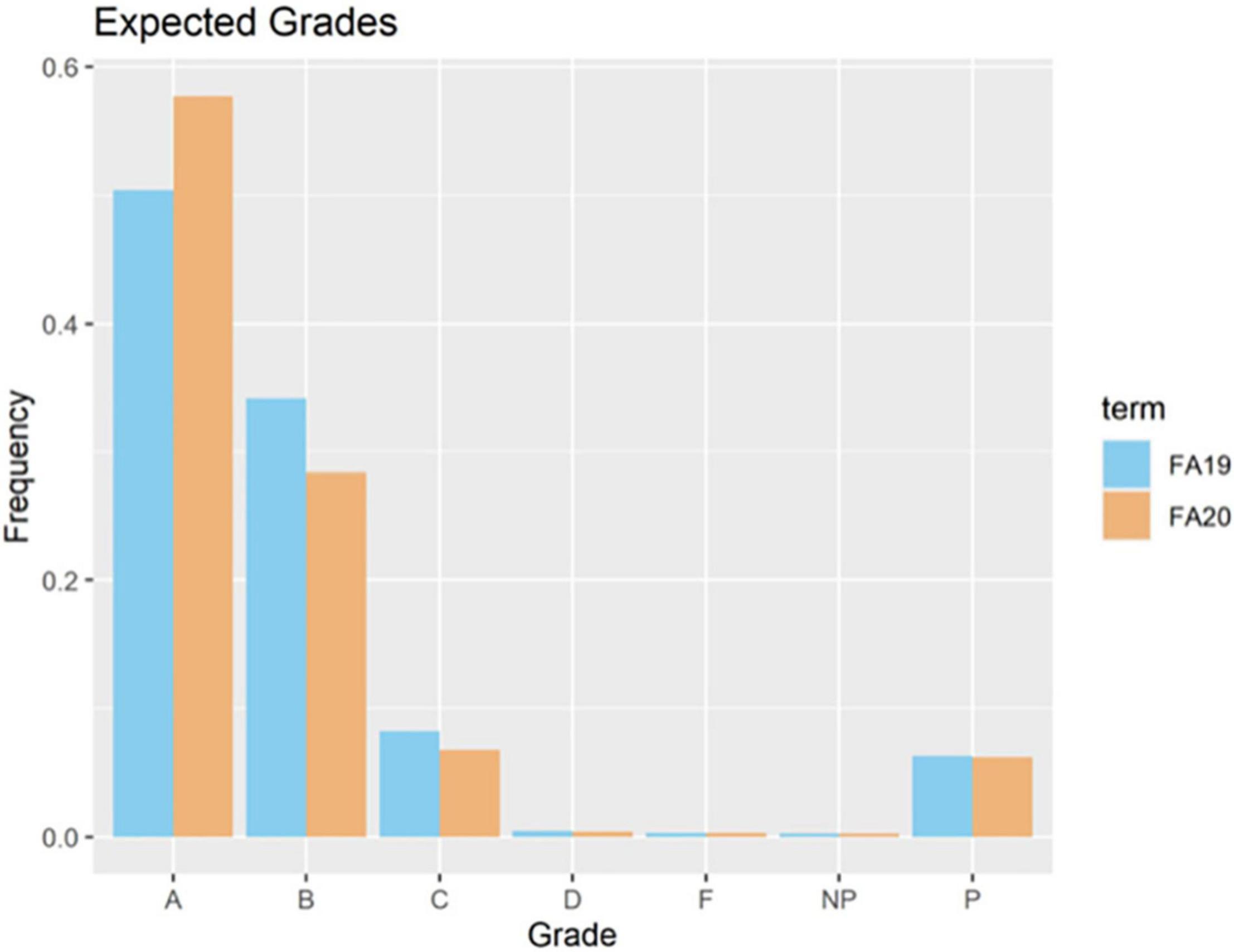
Figure 1. Distribution of grades expected by students prior to finals week in CAPE surveys in Fall 2019 and Fall 2020.
What Engagement Tools Are Being Utilized by Professors and What do Students Think About Them?
Assignments and Learning
As part of the CAPE survey, respondents were asked to rate their agreement on a 5-point Likert scale to questions about their assignments and learning experience in the class. Results are displayed in Table 2. Statistically significant increases in student agreement, as indicated by the two-sample Mann–Whitney U test, were reported in the questions “Assignments promote learning,” “The course material is intellectually stimulating,” and “I learned a great deal from this course.”

Table 2. Student responses on CAPE evaluation statements relating to assignments, course material, and quality of learning.
What Influence do Social Interactions With Peers, Teachers, and Administration Have on Student Engagement?
Professor Efficacy and Accessibility
As part of the CAPE survey, students also rated their professors in various aspects, as can be seen in Table 3. The only significant result observed between Fall 2019 and Fall 2020 was a slight increase in student agreement with the statement “Instructor is accessible outside of class.”

Table 3. Student responses on CAPE evaluation statements relating to instructor efficacy and accessibility.
Survey Results
How Has the Change From in-Person to Online Learning Affected Student Attendance, Performance Expectations, and Participation in Lectures?
General Satisfaction
Respondents were asked to indicate their agreement on a 5-point Likert scale (Strongly Disagree, Disagree, Neither Agree nor Disagree, Agree, and Strongly Agree) to the statement, “In general, I am satisfied with my online learning experience at [institution].” 36% of respondents agreed with the statement, 28% neither agreed nor disagreed, and 36% disagreed.
Perceptions of Academic Performance
Students were asked to rate their agreement on a 5-point Likert scale of agreement to a series of broad questions about their online learning experience, some of which pertained to academic performance. When assessing the statement “My current online courses are more difficult than my past in-person courses,” 42% chose Strongly Agree or Agree, 32% chose Neither Agree nor Disagree, and 26% chose Disagree or Strongly Disagree. Respondents were also split on the statement “My academic performance has improved with online education,” which 28% agreed/strongly agreed with, 34% disagreed/strongly disagreed with, and 38% chose neither.
For the statement “I feel more able to manage my time effectively with online education than with in-person education,” only 34% agreed/strongly agreed with the statement while 45% disagreed/strongly disagreed and 21% chose neither. For the statement, “I feel that it is easier to deal with the pace of my course load with online education than with in-person education,” 30% of respondents agreed/strongly agreed, 54% disagreed/strongly disagreed, and 16% neither agreed nor disagreed.
Lecture Attendance by Class Type
Since the CAPE survey question regarding attendance did not specify asynchronous or synchronous attendance, students were asked on the survey created by the authors of this paper how often they attended and skipped certain types of lectures. In response to the question “During your last quarter of in-person classes, how often did you skip live, in-person lectures?,” 11% reported doing so often or always, 14% did so sometimes, and the remaining 74% did so rarely or never. The terms “Sometimes” and “Rarely” were not clarified to the respondents. This is the same scale and language used on the CAPE survey, however, which was a benefit to synthesizing and comparing this data with CAPEs. Meanwhile, for online classes, 35% reported skipping their live classes often or always, 23% did so sometimes, and 43% did so rarely or never.
Respondents were also asked about their recorded lectures, both in-person and online; while some courses at the institution are recorded and released in either audio or video form for students, most online synchronous lectures are recorded. When asked how often they watched recorded lectures instead of live lectures in-person, 12% of respondents said they did so often or always, 12% reported doing so sometimes, and 76% did so rarely or never. For online classes where recorded versions of live lectures were available, 47% of students reported watching the recorded version often or always, 21% did so sometimes, and 33% did so rarely or never.
Meanwhile, there were also some lectures during online learning that were offered only online (asynchronous), as opposed to being recorded versions of lectures that were delivered to students live over Zoom.
Students were asked questions about their lecture attendance for in-person learning pre-COVID and for online learning during the pandemic. On a 5 point Likert scale from Never to Always, 11% of students said they skipped “live, in-person lectures” in their courses pre-COVID Often or Always. On the same scale, 35% of respondents said they skipped live online lectures Often or Always. To assess the significance of these reports, we conducted a one-sided Mann–Whitney U test with the null hypothesis that the median frequency of students skipping live online lectures is greater than the median frequency of skipping live in-person lectures. Previous research suggesting that lecture attendance decreased after the COVID-19 transition motivated our alternative hypothesis that students would skip live online lectures more often (Perets et al., 2020). The result was significant, meaning that this evidence suggests that students skip online lectures (Mdn = 3 “Sometimes”) more often than live in-person lectures (Mdn = 2 “Rarely”), U = 23328, p < 0.001. The results were also significant when a one-sided 2 sample t-test was performed to test if students were skipping online lectures (M = 2.84, SD = 1.13) more often than they skipped in-person lectures (M = 1.97, SD = 1.06), t(358.53) = 7.55, p < 0.001.
In order to clarify why students might be skipping lectures, we asked students how often they were using the recorded lecture options during in-person and online learning. 12% of respondents reported that they watched the recorded lecture “Often” or “Always” instead of attending the live lecture in-person while 47% of respondents said that they watched the recorded version of lecture, if it was offered, “Often” or “Always” rather than the live version during remote learning. When a one-sided Mann–Whitney U test was performed comparing the medians of students that utilized the recorded option during in-person classes (Mdn = 2 “Rarely”) and during online classes (Mdn = 3 “Sometimes”), the results were significant, suggesting that more students watch a recorded lecture version when it is offered during online classes, U = 6410, p < 0.001. The results are also significant with a t-test comparing the means of students that watched the recorded format during in-person classes (M = 1.95, SD = 1.08) and during online classes (M = 3.23, SD = 1.23), t(330.84) = –10.13, p < 0.001.
Students were asked how often they used course materials, such as a textbook or instructor provided notes and slideshows, rather than attending a live or recorded lecture to learn the necessary material. 10% of students said that they used course materials “Often” or “Always” during in-person learning while 19% of students said they used course materials “Often” or “Always” during online learning. The results were significant in a one-sided Mann–Whitney U test for the null hypothesis that the medians are equivalent for students using materials during in-person learning (Mdn = 1 “Never”) and during online learning (Mdn = 2 “Rarely”), U = 12644, p < 0.001. In other words, the evidence suggests that students use course materials instead of attending lectures more often when classes are online than when classes are in-person. A one-sided t-test also indicates that students during online learning (M = 2.30, SD = 1.16) utilize provided materials instead of watching lecture to learn course material more often than students during in-person learning (M = 1.76, SD = 1.03), t(364.55) = –4.72, p < 0.001.
Discussions are supplementary and sometimes mandatory classes to the lecture conducted by a teaching assistant. Students reported that during the last quarter of online classes the discussion sections tended to include synchronous live discussion instead of pre-recorded content (see Table 4).
Reported Attendance and Engagement in Lecture
Students were asked to rate their agreement on the same 5-point Likert scale to a series of questions about their in-lecture attendance and engagement. When presented with the statement “I feel more comfortable asking questions in online classes than in in-person ones,” 56% of students agreed, 22% neither agreed nor disagreed, and 22% disagreed. Here, “agreed” includes strongly agree and disagree includes “strongly disagreed.” This was similar to the result for “I feel more comfortable answering questions in online classes than in in-person ones,” to which 56% agreed, 24% neither agreed nor disagreed, and 20% disagreed.
When students who had taken both in-person and online courses were directly asked about overall attendance of live lectures, with the statement “I attend more live lectures now that they are online than I did when lectures were in-person,” 12% agreed, 19% neither agreed nor disagreed, and 69% disagreed (with 32.5% selecting “Strongly disagree”).
Issues With Online Learning
Respondents were asked to indicate on a 5-point Likert frequency scale (Never, Rarely, Sometimes, Often, and Always) how often a series of possible issues affected their online learning. These are reported in Figure 2. The most common technical issue was unreliable WiFi. 20% of students say unreliable WiFi happens “Often” or “Always,” 35% say this issue happens to them “Sometimes,” and 45% of students say unreliable WiFi affects their online learning “Never” or “Rarely.” The next common technological problem students face is unreliable devices. A poor physical environment affected students’ online learning for 32% of the respondents “Often” or “Always.” Issues with platforms, such as Gradescope, Canvas, and Zoom, were present but reported less often.
What Engagement Tools Are Being Utilized by Professors and What do Students Think About Them?
Course Structure
For a given possible intervention in course structure, students were asked how often their professors implemented the changes and to rate their opinion of the learning strategy. The examined changes were weekly quizzes, replacing exams with projects or other assignments, interactive polls or questions during lectures, breakout rooms within lectures, open-book or open-note exams, and optional or no-fault final exams – exams that will not count toward a student’s overall grade if their exam score does not help their grade.
Respondents’ reported frequencies of these interventions are displayed in Figure 3, and their ratings of them are displayed in Figure 4. In addition to being the most common intervention, open book exams were also the most popular intervention among students, with 89% of respondents reporting that they had a Good or Excellent opinion. Similarly popular were in-lecture polls, optional finals, and replacing exams with assignments, while breakout sessions had a slightly negative favorability.
Academic Tools and Resources
In the survey, students were asked to rate their agreement with the statement, “Online learning has made me more likely to use academic resources such as office hours, tutoring, or voluntary discussion sessions.” 42% of students agreed (includes “strongly agreed”), 23% neither agreed nor disagreed, and 35% disagreed (includes “strongly disagreed”). However, for the statement, “Difficulties accessing office hours or other academic resources have negatively interfered with my academic performance during online education,” 26% of students agreed/strongly agreed, 24% neither agreed nor disagreed, and 49% disagreed/strongly disagreed.
Respondents were asked to rate their opinion of various academic resources on a 5-point scale (Terrible, Poor, Average, Good, and Excellent) for both in-person and online classes (Figures 5, 6). The most notable change in rating was for the messaging platform Discord, which 67% of respondents saw as a Good or Excellent academic resource during online education, compared to 34% in in-person education. The learning management system Canvas also saw an increase in favorability, while favorability decreased for course discussions.
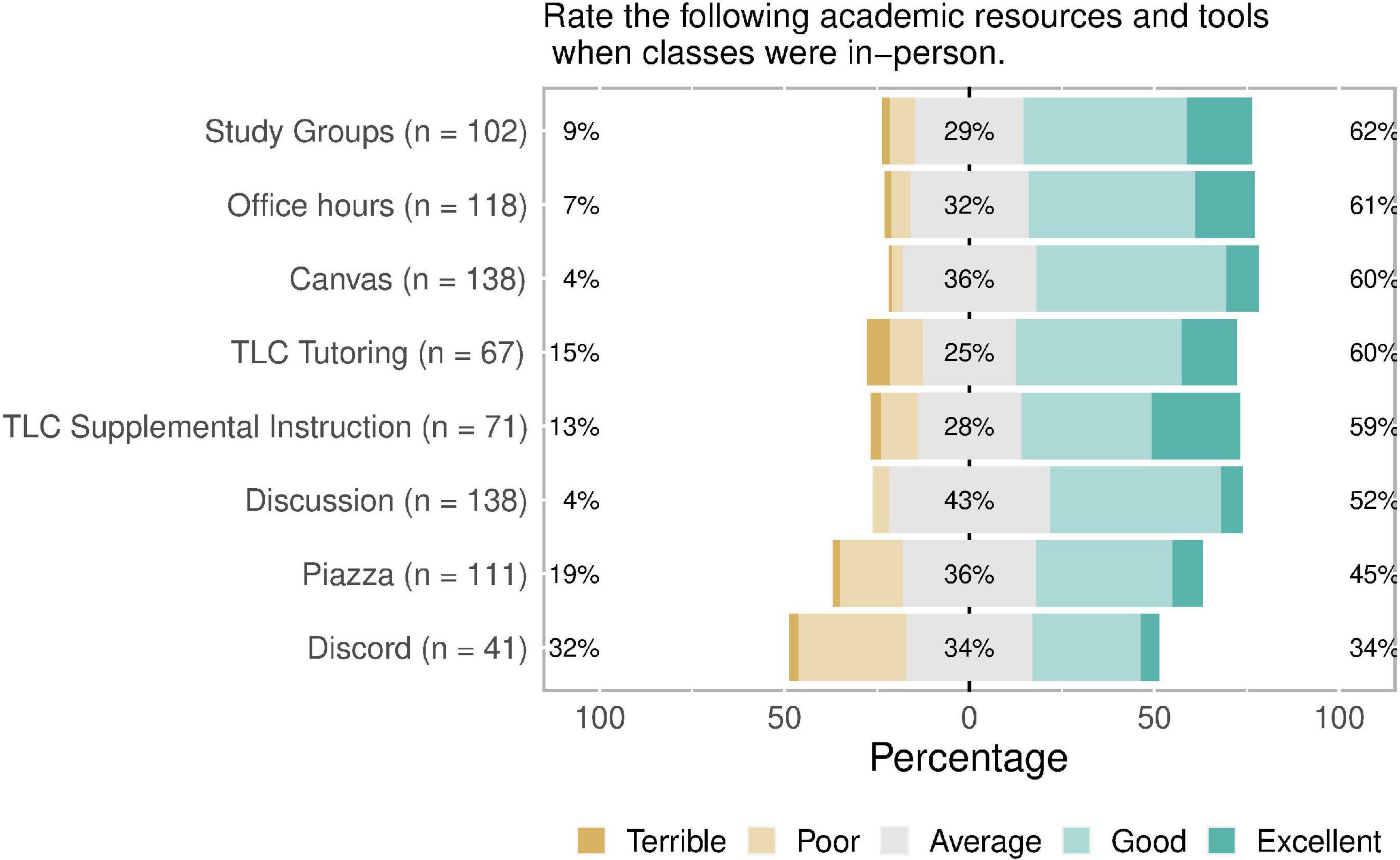
Figure 5. Students’ reported approval ratings of certain academic resources and tools when classes were in-person.
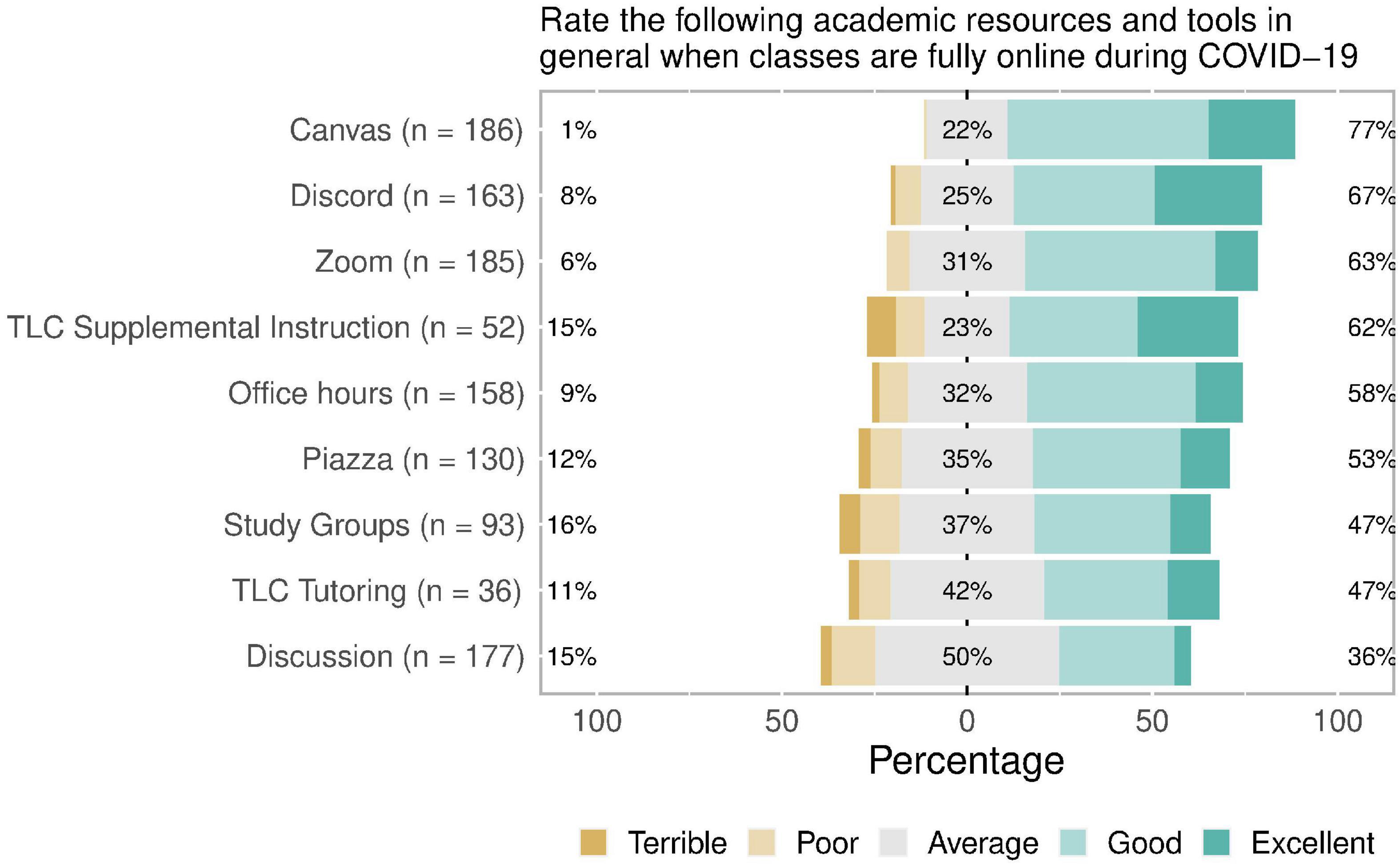
Figure 6. Students’ reported approval ratings of certain academic resources and tools when classes were online.
Cameras
Respondents were asked to rate the frequency at which they and their professors turned their cameras on during lectures. 64% of students reported keeping their cameras on never or rarely, 29% reported keeping cameras on sometimes, and 6% of students reported keeping their cameras on often or always. Meanwhile, for professors, 58% of students reported that all of their professors kept their cameras on, 28% said most kept their cameras on, 9% said about half did so, and the remaining 5% said that some or none of their professors kept cameras on.
What Influence do Social Interactions With Peers, Teachers, and Administration Have on Student Engagement?
Personal Interaction
A lack of social interaction was among the largest complaints of students about online learning. 88% of respondents at least somewhat agreed with the statement “I feel less socially connected to my peers during online education than with in-person education.” When students were asked how often certain issues negatively impacted their online learning experience, 64% of respondents indicated that a lack of interaction with peers often or always impacted their learning experience, and 44% reported the same about a lack of instructor interaction.
When we asked students how they stay connected to their peers, 78.6% said that they stay connected to peers through student-run course forums, such as Discord, a messaging app that is designed to build communities of a common interest. 72.7% said they use personal communication, i.e., texting, with peers. 48.1% of students said they use faculty-run course forums, such as Piazza or Canvas. 45.5% of students surveyed keep in touch with peers through institution clubs and organizations. 29.4% of students selected that they use student-made study groups and 19.8% stay connected through their campus job.
Ratings of University Faculty and Staff
Students were asked to rate their opinion of various faculty and staff, by answering survey statements of the form “____ have been sufficiently accommodating of my academic needs and circumstances during online learning.” For instructors, 72% agreed/strongly agreed with this statement and 11% disagreed/strongly disagreed; for teaching assistants and course tutors, 81% agreed/strongly agreed, and only 2% disagreed/strongly disagreed. Meanwhile, for university administration, 39% of students agreed/strongly agreed, 34% neither agreed nor disagreed, and 26% disagreed/strongly disagreed.
Discussion
Based on both the prior literature and this study, students seemed to struggle with engagement before the pandemic during in-person lectures, and it appears from the survey findings that students are struggling even more with engagement in online courses. A U.S. study investigating the teaching and learning experiences of instructors and students during the COVID-19 pandemic also found that when learning transitioned online, students’ main issue was engagement whereas prior to the pandemic the main issue for students was content (Perets et al., 2020). The lack of peer connection and technological issues seem to be significant problems for students during online learning and could contribute to students’ issues with engagement. The problems with attention during an online lecture might be attributed to the lack of social accountability that an in-person lecture promotes to put away distractions like cell phones and taking active notes. Additionally, CAPE data shows that students rate their professors’ efforts and course design highly and similarly before and during Fall 2019 and Fall 2020. Although every course and professor has different requirements, creating collaborative opportunities and incorporating interactive features into lectures could be beneficial to student engagement.
How Has the Change From in-Person to Online Learning Affected Student Attendance, Performance Expectations, and Participation in Lectures?
For live lectures, the increase in students reporting skipping live online lectures more often may be due to the increase in availability and ease of recorded options with online lectures. A similar study to this research found that when the university transitioned to Pass/No Pass grading rather than letter grading during ERT, students attended synchronous lectures less (Perets et al., 2020). During the pandemic, the institution’s deadline to change to P/NP grading was extended and more academic departments allowed Pass/No Pass classes to fulfill course requirements. In our study, we did not detect an increase in students who took advantage of the P/NP grading, but it is possible that students skipped more synchronous lectures knowing that they could use the Pass option as a safety net if they did not dedicate the typical amount of lecture time to learn the material. The results emphasize the vital role of the cognitive dimension in engagement.
It is clear that more students are taking advantage of recorded options with online learning. A survey of Harvard medical students indicates a preference for the recorded option because of the ability to increase the speed of the lecture video and prevent fatigue (Cardall et al., 2008). Consistent with previous research, our results suggest that students may seek more value and time management options from course material when classes are fully online (Perets et al., 2020). Recorded lectures allow freedom for students to learn at a time that works best for them (Rae and McCarthy, 2017). For discussions, students reported that they had more discussions that were live rather than recorded. Research indicates that successful online learning requires strong instructor support (Dixson, 2010; Martin and Bolliger, 2018). The smaller class setting of a discussion, even virtual, may promote better engagement through interaction among the students, content, and the discussion leader.
Based on CAPE results, which are conducted the week before final exams, students expected higher grades during the online learning period. Although expected grades rose, students concur with previous surveys that the workload was overwhelming and was not adequately adjusted to reflect the circumstances of ERT (Hussein et al., 2020; Shin and Hickey, 2020). While there are many factors that could account for this, including the fact that expected grades reported on CAPE do not reflect a student’s actual grade, one possible explanatory factor is the use of more lenient grading standards and course practices during the pandemic. In addition to relaxed Pass/No Pass standards, courses were more likely to adopt practices like open-book tests or no-fault finals, providing students with assessments that emphasized a demonstration of deeper conceptual understanding rather than memorization. It is important to note that students’ perceptions of their learning does not indicate that students are actually learning or performing better academically. This goes for the CAPE question, “I learned a great deal from this course,” the CAPE question about expected grades, and the small scale survey reports about academic performance. We took interest in these questions because they offer insight into the level of difficulty students perceived during ERT due to the shift in engagement demands from remote learning. More research should be done with students’ academic performance data before and after ERT to clarify whether there was a change in students’ learning.
Students’ preference for using a virtual platform during lecture to ask, answer, and respond to questions was surprising. This extends previous evidence from Vu and Fadde (2013), who found that, in a graduate design course at a Midwestern public university with both in-person and online students in the same lecture, students learning online were more likely to ask questions through a chat than students attending in-person lectures. In addition, during the COVID-19 pandemic, Castelli and Sarvary (2021) report that Zoom chat facilitates discussions for students, especially for those who may not have spoken in in-person classes.
When students were surveyed on the issues they faced with online learning, the most common issues had to do with engagement in lectures, interaction with instructors and peers, and having a poor physical work environment, while technical issues or issues with learning platforms were less common. The distinction between frequency and impact is key, since issues such as bad WiFi connection can be debilitating to online learning even if uncommon, and issues with technology and physical environment also correlate with equity concerns. Other surveys have found that students and faculty from equity-seeking groups faced more hardships during online learning because of increased home responsibilities and problems with internet access (Chan et al., 2020; Shin and Hickey, 2020). Promoting student engagement in class involves more than well-planned teaching strategies. Instructors and universities need to look at the resources and accessibility of their class to reduce the digital divide.
According to the CAPE data from Table 2, instructors received consistent reviews before and after the ERT switch, indicating that they maintained their effectiveness in teaching. The ratings for two CAPE prompts “Instructor is well prepared for class” and “Instructor starts and finishes class on time” had statistically significant decreases from Fall 2019 to Fall 2020. This decrease could be attributed to increased technological preparation needed for online courses and the variety of offerings for lecture modalities. For example, some instructors chose to offer a synchronous lecture at a different time than the original scheduled course time, and then provide office hours during their scheduled lecture time to discuss and review the lectures. Regardless of the statistically significant changes, the means for these two statements are high and similar to Fall 2019.
What Engagement Tools Are Being Utilized in Lectures and What do Students Think About Them?
Based on the results, a majority of students report that their professors are using weekly quizzes, breakout rooms, and polls at least sometimes in their classes to engage students. Students had highly positive ratings of in-course polling, were mostly neutral or positive about weekly quizzes (as a replacement for midterm or final exams), but were slightly negative about breakout rooms. Venton and Pompano (2021) report positive qualitative student feedback from students in chemistry classes at the University of Virginia, with some students finding it easier to speak up and make connections with peers than in an entire class; Fitzgibbons et al. (2021), meanwhile, found in a sample of 15 students at the University of Rochester that students preferred working as a full class instead of in breakout rooms, though students did report making more peer connections in breakout rooms. Breakouts have potential to strengthen student-student and student-instructor relationships, but further research is needed to clarify their effectiveness.
Changes were also made to course structure, with almost all (94%) of students reporting that open-book exams were used at least sometimes. Open-book exams were also the most popular intervention overall, although the reason for their widespread adoption (academic integrity and fairness concerns) is likely different from the reasons that students like them (less focus on memorization). Open-book tests, however, present complications. Bailey et al. (2020) notes that while students still needed a good level of understanding to succeed on open-book exams, these exams were best suited to higher-order subjects without a unique, searchable answer.
Changes were detected in the responses to the CAPE statements, “Assignments promote learning,” “The course material is intellectually stimulating,” and “I learned a great deal from this course,” noted in Table 3. Although there were statistically significant changes detected by the Mann–Whitney U Test, the means between Fall 2019 and Fall 2020 are still similar and positive. The results from this table indicate that students felt that there was not a decrease in learning and interest in their material. This might be due to instructors changing the design of assessments and assignments to accommodate for academic integrity and modality circumstances in the online learning format. The consistently positive CAPE ratings are also likely due to the fact that students are aware that CAPEs are an important factor for the departments’ hiring and retention decisions for faculty, and subsequently important for their instructors’ careers. Students may have also recognized that most of the difficulties in the switch to online learning were not the instructors’ fault. Students’ sympathy for the challenges that instructors faced may be contributing to the slightly more positive reviews during Fall 2020.
What Influence do Social Interactions With Peers, Teachers, and Administration Have on Student Engagement?
One of the most common experiences reported by students was a decrease in interaction with peers, with a strong majority of students saying that a lack of peer interaction hurt their learning experience. A study from Central Michigan University shows that peer interaction through in class activities supports optimal active learning (Linton et al., 2014). Without face-to-face learning and asynchronous classes during COVID, instructors were not able to conduct the same collaborative activities. When asked how students interacted with their peers, the most common responses were student-run course forums or texting. This seems to support the findings of Wong (2020) which indicated that during ERT, students largely halted their use of synchronous forms of communication and opted instead for asynchronous ones, like instant messaging, with possible impacts on students’ social development. Students also reported a decrease in interaction with their instructors with a plurality saying that a lack of access to their instructors affected their academic experience. At the same time, ratings of professors’ ability to accommodate for the issues students faced during online education were high, as were students’ ratings of online office hours. It seems that students sympathized with instructors’ difficulties in the ERT transition but were aware that the lack of instructor presence impacted their learning experience nonetheless.
Limitations
There are some limitations in this study that should be considered before generalizing the results more widely. The survey was conducted at just a single university, UCSD: a large, highly-ranked, public research institution in the United States with its own unique approach to the COVID-19 pandemic. These results would likely differ significantly for online education at other universities. In addition, though care was taken to distribute the survey in channels used by all students, the voluntary response of students chosen from these channels does not constitute a simple random sample of undergraduates attending this institution. For example, our survey over-represents female students, who constituted 72.7% of the survey sample. The channels chosen could also bias certain results; for example, it is possible that students who answer online surveys released on the institution’s social media channels are less likely to have technical or Internet difficulties. Results from the small survey might be skewed slightly because respondents had to recall a year prior to their experiences in Fall 2019, whereas they might have had a more accurate memory of their Fall 2020 experience. CAPEs are completed at the end of the quarter when their recollection of their experiences is fresh, so those reviews are likely less susceptible to this unconscious bias.
The issues with sampling are somewhat mitigated in the CAPE data, but these responses are not themselves without issue. CAPE reviews are still a voluntary survey, and therefore are not a random sample of undergraduates. In addition, some instructors use extra credit to incentivize students to participate in CAPEs if the class meets a threshold percentage of responses, which might skew the population of respondents. CAPE responses tend to be relatively generous and positive, with students rating instructors and educational quality much higher in CAPE reviews than in our survey. This is possibly because the CAPE forms make it easy for students to report the most positive ratings on every item without considering them individually. Additionally, students are aware that CAPEs have an impact on the department’s decisions to rehire instructors.
Teaching Implications
Online learning presented multiple challenges for instructors and students, illuminating areas to improve in higher education that were not recognized before the COVID-19 pandemic. A majority of students expressed their comfort in engaging with the Zoom chat and polling. Students might feel this way since they can ask and answer questions using the chat feature without disrupting the focus in class. Therefore, in both further online learning and in-person classes, instructors might be able to stimulate interaction by lowering the social barriers to asking and answering questions. Applications such as Backchannel Chat, Yo Teach!, and NowComment offer more features than Zoom or Google Meet to prevent fatigue and increase retention in-person or online (LearnWeaver, 2014; Hong Kong Polytechnic University, 2018; Paul Allison, 2018).
At the same time, increased interactivity in lectures, especially if required, is not necessarily a panacea for engagement issues. For example, some professors might require students to turn on their cameras, increasing accountability and giving an incentive to visibly focus as if in an in-person classroom. However, Castelli and Sarvary (2021) found, as we did, that the majority of students in an introductory collegiate biology course kept their cameras off; students cited concerns about their appearance, other people being seen behind them, and weak internet connections as the most common reasons for not keeping cameras on. Not only are these understandable concerns, but they correlate with identity as well: Castelli and Sarvary found that both underrepresented minorities and women were more likely to indicate that they worried about cameras showing others their surroundings and the people behind them.
Conclusion
Prior to the COVID-19 pandemic, online learning was a choice. Our research demonstrates that online learning has a long way to go before it can be used in an equitable manner that creates an engaging environment for all students, but that instructors adapted well to ERT to ensure courses promoted the same level of learning. The sudden nature of remote learning during the COVID pandemic did not allow for instructors or institutions to research and promote the most engaging online learning resources. Students have widely varying opinions and experiences with their higher education online learning experience during the pandemic. Our data analysis shows that distance learning during the pandemic had a toll on attendance during live lecture and peer-instructor connection. The difference in expected grades from Fall 2019 to Fall 2020 indicates that students felt differently about their ability to succeed in their online classes. In addition, students had trouble managing work loads during online learning. We gathered that instructors could be using engagement strategies more often to match students’ enthusiasm for those strategies, such as chat features and polls. Despite the challenges of online learning highlighted, this research also presents evidence that online learning can be engaging for students with the right tools. Student reviews indicated similarity before and after the switch to online learning, including indicating that course assignments promoted learning and the material was intellectually stimulating. These results propose that the courses and professors, despite the modality switch and changes to teaching and assessment strategies, maintained the level of learning that students felt they were getting out of their course.
Data Availability Statement
The data supporting the conclusions of this article contains potentially identifiable information. The authors can remove this identifying information prior to sharing the data.
Author Contributions
BH contributed to this project through formal analysis, investigation, and writing. PN contributed to the project through formal analysis, investigation, visualization, and writing. LC contributed to conceptualization, resources, supervision, writing, review, and editing. SH-L contributed methodology, supervision, writing, review, and editing. All authors contributed to the article and approved the submitted version.
Conflict of Interest
The authors declare that the research was conducted in the absence of any commercial or financial relationships that could be construed as a potential conflict of interest.
Publisher’s Note
All claims expressed in this article are solely those of the authors and do not necessarily represent those of their affiliated organizations, or those of the publisher, the editors and the reviewers. Any product that may be evaluated in this article, or claim that may be made by its manufacturer, is not guaranteed or endorsed by the publisher.
Acknowledgments
We would like to acknowledge the Qualcomm Institute Learning Academy for supporting this project.
References
Abrami, P. C., Bernard, R. M., Bures, E. M., Borokhovski, E., and Tamim, R. M. (2012). “Interaction in distance education and online learning: using evidence and theory to improve practice,” in The Next Generation of Distance Education, eds L. Moller and J. B. Huett (Boston, MA: Springer), 49–69. doi: 10.1016/j.nedt.2014.06.008
Almarghani, E., and Mijatovic, I. (2017). Factors affecting student engagement in HEIs – it is all about good teaching. Teach. Higher Educ. 22, 940–956. doi: 10.1080/13562517.2017.1319808
Bailey, T., Kinnear, G., Sangwin, C., and O’Hagan, S. (2020). Modifying closed-book exams for use as open-book exams. OSF Preprint] doi: 10.31219/osf.io/pvzb7
Banna, J., Lin, M.-F. G., Stewart, M., and Fialkowski, M. K. (2015). Interaction matters: strategies to promote engaged learning in an online introductory nutrition course. J. Online Learn. Teach. 11, 249–261.
Beer, C., Clark, K., and Jones, D. (2010). “Indicators of engagement,” in Proceedings of the Curriculum, Technology & Transformation for An Unknown. Proceedings Ascilite Sydney, eds C. H. Steel, M. J. Keppell, P. Gerbic, and S. Housego (Sydney, SA).
Bond, M., and Bedenlier, S. (2019). Facilitating student engagement through educational technology: towards a conceptual framework. J. Interact. Media Educ. 2019:11.
Bond, M., Buntins, K., Bedenlier, S., Zawacki-Richter, O., and Kerres, M. (2020). Mapping research in student engagement and educational technology in higher education: a systematic evidence map. Int. J. Educ. Technol. Higher Educ. 17:2.
Britt, M., Goon, D., and Timmerman, M. (2015). How to better engage online students with online strategies. College Student J. 49, 399–404.
Brooks, D. C., Grajek, S., and Lang, L. (2020). Institutional readiness to adopt fully remote learning. Educ. Rev.
Bryer, J., and Speerschneider, K. (2016). Likert: Analysis and Visualization Likert Items. R Package Version 1.3.5. Available online at: https://CRAN.R-project.org/package=likert (accessed August 2021).
Bundick, M., Quaglia, R., Corso, M., and Haywood, D. (2014). Promoting student engagement in the classroom. Teach. Coll. Rec. 116, 1–43.
Bülow, M. W. (2022). “Designing synchronous hybrid learning spaces: challenges and opportunities,” in Hybrid Learning Spaces. Understanding Teaching-Learning Practice, eds E. Gil, Y. Mor, Y. Dimitriadis, and C. Köppe (Cham: Springer), doi: 10.1007/978-3-030-88520-5_9
Cakir, H. (2013). Use of blogs in pre-service teacher education to improve student engagement. Comp. Educ. 68, 244–252. doi: 10.1016/j.compedu.2013.05.013
Cardall, S., Krupat, E., and Ulrich, M. (2008). Live lecture versus video-recorded lecture: are students voting with their feet? Acad. Med. 83, 1174–1178. doi: 10.1097/acm.0b013e31818c6902
Castelli, F. R., and Sarvary, M. A. (2021). Why students do not turn on their video cameras during online classes, and an equitable, and inclusive plan to encourage them to do so. Ecol. Evol. 11, 3565–3576. doi: 10.1002/ece3.7123
Chakraborty, M., and Nafukho, F. M. (2014). Strengthening student engagement: what do students want in online courses? Eur. J. Train. Dev. 38, 782–802. doi: 10.1108/ejtd-11-2013-0123
Chan, L., Way, K., Hunter, M., Hird-Younger, M., and Daswani, G. (2020). Equity and Online Learning Survey Results. Toronto, ON: University of Toronto.
Chapman, E. (2002). Alternative approaches to assessing student engagement rates. Practical Assess. Res. Eval. 8, 1–7.
Chatterjee, R., and Correia, A. (2020). Online students’ attitudes toward collaborative learning and sense of community. Am. J. Distance Educ. 34, 53–68. doi: 10.1080/08923647.2020.1703479
Coates, H. (2007). A model of online and general campus based student engagement. Assess. Eval. Higher Educ. 32, 121–141.
Courses not CAPEd for Winter 22 (2022). Course and Professor Evaluations (CAPE). Available online at: https://cape.ucsd.edu/faculty/CoursesNotCAPEd.aspx (Retrieved March 3, 2022).
Desmione, L. M., and Carlson Le Floch, K. (2004). Are we asking the right questions? using cognitive interviews to improve surveys in education research. Educ. Eval. Policy Anal. 26, 1–22. doi: 10.3102/01623737026001001
Dixson, M. D. (2010). Creating effective student engagement in online courses: what do students find engaging? J. Scholarship Teach. Learn. 10, 1–13.
Fadde, P. J., and Vu, P. (2014). Blended online learning: benefits, challenges, and misconceptions. Online learn. Common Misconceptions Benefits Challenges 2014, 33–48. doi: 10.4018/978-1-5225-8009-6.ch002
Fitzgibbons, L., Kruelski, N., and Young, R. (2021). Breakout Rooms in an E-Learning Environment. Rochester, NY: University of Rochester Research.
Fredricks, J. A., Blumenfeld, P. C., and Paris, A. H. (2004). School engagement: potential of the concept, state of the evidence. Rev. Educ. Res. 74, 59–109. doi: 10.3102/00346543074001059
Fredricks, J. A., Filsecker, M., and Lawson, M. A. (2016). Student engagement, context, and adjustment: addressing definitional, measurement, and methodological issues. Learn. Instruct. 43, 1–4.
Gaytan, J., and McEwen, B. C. (2007). Effective online instructional and assessment strategies. Am. J. Distance Educ. 21, 117–132. doi: 10.1080/08923640701341653
Gillett-Swan, J. (2017). The challenges of online learning: supporting and engaging the isolated learner. J. Learn. Design 10, 20–30. doi: 10.5204/jld.v9i3.293
Harrell, I. (2008). Increasing the success of online students. Inquiry: J. Virginia Commun. Colleges 13, 36–44.
Hodges, C. B., Moore, S., Lockee, B. B., Trust, T., and Bond, M. A. (2020). The Difference Between Emergency Remote Teaching and Online Learning. EDUCAUSE Review.
Hussein, E., Daoud, S., Alrabaiah, H., and Badawi, R. (2020). Exploring undergraduate students’ attitudes towards emergency online learning during COVID-19: a case from the UAE. Children Youth Services Rev. 119:105699. doi: 10.1016/j.childyouth.2020.105699
Inside Higher Ed (2020). Responding to the COVID-19 Crisis: A Survey of College, and University Presidents. Inside Higher Ed: Washington, DC.
Johnson, N., Veletsianos, G., and Seaman, J. (2020). U.S. faculty and administrators’ experiences and approaches in the early weeks of the COVID-19 Pandemic. Online Learn. 24, 6–21. doi: 10.24059/olj.v24i2.2285
Kahu, R. (2013). Framing student engagement in higher education. Stud. Higher Educ. 38, 758–773. doi: 10.1080/03075079.2011.598505
Kendricks, K. D. (2011). Creating a supportive environment to enhance computer based learning for underrepresented minorities in college algebra classrooms. J. Scholarsh. Teach. Learn. 12, 12–25.
Lear, J. L., Ansorge, C., and Steckelberg, A. (2010). Interactivity/community process model for the online education environment. J. Online Learn. Teach. 6, 71–77.
LearnWeaver (2014). Backchannel Chat Benefits. https://backchannelchat.com/Benefits
Lederman, D. (2020). How Teaching Changed in the (Forced) Shift to Remote Learning. How professors Changed Their Teaching in this Spring’s Shift to Remote Learning. Available online at: https://www.insidehighered.com/digital-learning/article/2020/04/22/how-professors-changed-their-teaching-springs-shift-remote (accessed April 22, 2020).
Levin, S., Whitsett, D., and Wood, G. (2013). Teaching MSW social work practice in a blended online learning environment. J. Teach. Soc. Work 33, 408–420. doi: 10.1080/08841233.2013.829168
Linton, D. L., Farmer, J. K., and Peterson, E. (2014). Is peer interaction necessary for optimal active learning? CBE—Life Sci. Educ. 13, 243–252. doi: 10.1187/cbe.13-10-0201
Liu, X., Magjuka, R., Bonk, C., and Lee, S. (2007). Does sense of community matter? an examination of participants’ perceptions of building learning communities in online courses. Quarterly Rev. Distance Educ. 8:9.
Ma, J., Han, X., Yang, J., and Cheng, J. (2015). Examining the necessary condition for engagement in an online learning environment based on learning analytics approach: the role of the instructor. Int. Higher Educ. 24, 26–34. doi: 10.1016/j.iheduc.2014.09.005
Mandernach, B. J. (2015). Assessment of student engagement in higher education: a synthesis of literature and assessment tools. Int. J. Learn. Teach. Educ. Res. 12, 1–14. doi: 10.1080/02602938.2021.1986468
Mandernach, B. J., Gonzales, R. M., and Garrett, A. L. (2006). An examination of online instructor presence via threaded discussion participation. J. Online Learn. Teach. 2, 248–260.
Martin, F., and Bolliger, D. U. (2018). Engagement matters: student perceptions on the importance of engagement strategies in the online learning environment. Online Learn. 22, 205–222. doi: 10.1186/s12913-016-1423-5
Means, B., and Neisler, J. (2020). Suddenly Online: A National Survey of Undergraduates During the COVID-19 Pandemic. San Mateo, CA: Digital Promise.
Moore, M. (1993). “Three types of interaction,” in Distance Education: New Perspectives, eds K. Harry, M. John, and D. Keegan (New York, NY: Routledge), 19–24.
Nelson Laird, T., and Kuh, D. (2005). Student experiences with information technology and their relationship to other aspects of student engagement. Res. Higher Educ. 46, 211–233. doi: 10.5811/westjem.2017.9.35163
Nicholson, S. (2002). Socializing in the “virtual hallway”: instant messaging in the asynchronous web-based distance education classroom. Int. Higher Educ. 5, 363–372.
Northey, G., Govind, R., Bucic, T., Chylinski, M., Dolan, R., and van Esch, P. (2018). The effect of “here and now” learning on student engagement and academic achievement. Br. J. Educ. Technol. 49, 321–333. doi: 10.1111/bjet.12589
Perets, E. A., Chabeda, D., Gong, A. Z., Huang, X., Fung, T. S., Ng, K. Y., et al. (2020). Impact of the emergency transition to remote teaching on student engagement in a non-stem undergraduate chemistry course in the time of covid-19. J. Chem. Educ. 97, 2439–2447. doi: 10.1021/acs.jchemed.0c00879
Quin, D. (2017). Longitudinal and contextual associations between teacher–student relationships and student engagement. Rev. Educ. Res. 87, 345–387. doi: 10.1016/j.jsp.2019.07.012
R Core Team (2020). R: A Language and Environment for Statistical Computing. Vienna: R Foundation for Statistical Computing.
Rae, M. G., and McCarthy, M. (2017). The impact of vodcast utilisation upon student learning of physiology by first year graduate to entry medicine students. J. Scholarship Teach. Learn. 17, 1–23. doi: 10.14434/josotl.v17i2.21125
Raes, A., Detienne, L., and Depaepe, F. (2019). A systematic review on synchronous hybrid learning: gaps identified. Learn. Environ. Res. 23, 269–290. doi: 10.1007/s10984-019-09303-z
Read, D. L. (2020). Adrift in a Pandemic: Survey of 3,089 Students Finds Uncertainty About Returning to College. Toronto, ON: Top Hat.
Redmond, P., Heffernan, A., Abawi, L., Brown, A., and Henderson, R. (2018). An online engagement framework for higher education. Online Learn. 22, 183–204.
Reich, J., Buttimer, C., Fang, A., Hillaire, G., Hirsch, K., Larke, L., et al. (2020). Remote learning guidance from state education agencies during the COVID-19 pandemic: a first look. EdArXiv [Preprint]. doi: 10.35542/osf.io/437e2
Revere, L., and Kovach, J. V. (2011). Online technologies for engaged learning: a meaningful synthesis for educators. Quar. Rev. Distance Educ. 12, 113–124.
Rovai, A., and Wighting, M. (2005). Feelings of alienation and community among higher education students in a virtual classroom. Int. Higher Educ. 8, 97–110. doi: 10.1016/j.iheduc.2005.03.001
Senn, S., and Wessner, D. R. (2021). Maintaining student engagement during an abrupt instructional transition: lessons learned from COVID-19. J. Microbiol. Biol. Educ. 22:22.1.47. doi: 10.1128/jmbe.v22i1.2305
Shea, P., Fredericksen, E., Pickett, A., Pelz, W., and Swan, K. (2001). Measures of learning effectiveness in the SUNY Learning Network. Online Educ. 2, 31–54.
Shin, M., and Hickey, K. (2020). Needs a little TLC: examining college students’ emergency remote teaching and learning experiences During covid-19. J. Further Higher Educ. 45, 973–986. doi: 10.1080/0309877x.2020.1847261
Shin, M., and Hickey, K. (2021). Needs a little TLC: examining college students’ emergency remote teaching and learning experiences during COVID-19. J. Furth. High. Educ. 45, 973–986.
Sumuer, E. (2018). Factors related to college students’ self-directed learning with technology. Australasian J. Educ. Technol. 34, 29–43. doi: 10.3389/fpsyg.2021.751017
Swan, K., and Shih, L. (2005). On the nature and development of social presence in online course discussions. J. Asynchronous Learn. Networks 9, 115–136.
Tess, P. A. (2013). The role of social media in higher education classes (real and virtual) – a literature review. Comp. Hum. Behav. 29, A60–A68.
UNESCO (2020). UNESCO Rallies International Organizations, Civil Society and Private Sector Partners in a Broad Coalition to Ensure #learningneverstops [Press Release]. Paris: UNESCO.
University of California San Diego [UCSD] (2021b). Transfer Students. Undergraduate Admissions. La Jolla, CA: UCSD.
University of California San Diego [UCSD] (2021a). Response Rate. Course and Professor Evaluations (CAPE). La Jolla, CA: UCSD.
University of California, San Diego Institutional Research (2021). Student Profile 2020-2021. La Jolla, CA: UCSD.
Venton, B. J., and Pompano, R. R. (2021). Strategies for enhancing remote student engagement through active learning. Anal. Bioanal. Chem. 413, 1507–1512. doi: 10.1007/s00216-021-03159-0
Vu, P., and Fadde, P. (2013). When to talk, when to chat: student interactions in live virtual classrooms. J. Interact. Online Learn. 12, 41–52.
Walker, K. A., and Koralesky, K. E. (2021). Student and instructor perceptions of engagement after the rapid online transition of teaching due to COVID-19. Nat. Sci. Educ. 50:e20038. doi: 10.1002/nse2.20038
Wimpenny, K., and Savin-Baden, M. (2013). Alienation, agency and authenticity: a synthesis of the literature on student engagement. Teach. Higher Educ. 18, 311–326. doi: 10.1080/13562517.2012.725223
Wong, R. (2020). When no one can go to school: does online learning meet students’ basic learning needs? Interact. Learn. Environ. 1–17.
Xiao, J. (2017). Learner-content interaction in distance education: the weakest link in interaction research. Distance Educ. 38, 123–135. doi: 10.1080/01587919.2017.1298982
Yildiz, S. (2009). Social presence in the web-based classroom: implications for intercultural communication. J. Stud. Int. Educ. 13, 46–65. doi: 10.1177/1028315308317654
Zepke, N., and Leach, L. (2010). Improving student engagement: ten proposals for action. Act. Learn. Higher Educ. 11, 167–177. doi: 10.1111/jocn.15810
Keywords: student engagement, undergraduate, online learning, in-person learning, remote instruction and teaching
Citation: Hollister B, Nair P, Hill-Lindsay S and Chukoskie L (2022) Engagement in Online Learning: Student Attitudes and Behavior During COVID-19. Front. Educ. 7:851019. doi: 10.3389/feduc.2022.851019
Received: 08 January 2022; Accepted: 11 April 2022;
Published: 09 May 2022.
Edited by:
Canan Blake, University College London, United KingdomReviewed by:
Mark G. Rae, University College Cork, IrelandYu-Chun Kuo, Rowan University, United States
Paul C. LePore, Arizona State University, United States
Copyright © 2022 Hollister, Nair, Hill-Lindsay and Chukoskie. This is an open-access article distributed under the terms of the Creative Commons Attribution License (CC BY). The use, distribution or reproduction in other forums is permitted, provided the original author(s) and the copyright owner(s) are credited and that the original publication in this journal is cited, in accordance with accepted academic practice. No use, distribution or reproduction is permitted which does not comply with these terms.
*Correspondence: Brooke Hollister, YmhvbGxpc3RAdWNzZC5lZHU=
†These authors share first authorship