- Department of Earth, Ocean and Ecological Sciences, University of Liverpool, Liverpool, United Kingdom
Monitoring student attendance and engagement is common practice during undergraduate courses at university. Attendance data typically show a strong positive relationship with student performance and regular monitoring is an important tool to identify students who may require additional academic provisions, wellbeing support and pastoral care, for example. However, most of the previous studies and our framework for monitoring attendance and engagement is based on traditional on-campus, in-person delivery. Accelerated by the COVID-19 pandemic, our transition to online teaching delivery requires us to re-evaluate what constitutes attendance and engagement in a purely online setting and what are the most accurate ways of monitoring. Here, I show how statistics derived from student interaction with a virtual learning environment, Canvas, can be used as a monitoring tool. I show how basic statistics such as the number and frequency of page views are not adequate and do not correlate with student performance. A more in-depth analysis of video viewing duration, rather than simple page clicks/views is required, and weakly correlates with student performance. Lastly, I provide a discussion of the potential pitfalls and advantages of collecting such data and provide a perspective on some of the associated challenges.
Introduction
Both student attendance and engagement during university level courses are commonly related to student performance (e.g., Jones, 1984; Clump et al., 2003; Gump, 2005). There have been numerous studies that relate synchronous attendance at on-campus or in-person lecture sessions to academic performance. Where performance is usually measured by final examination grade, since the success in examination commonly relates to the learner meeting the intended learning objectives. Numerous, largely discipline specific empirical studies have convincingly shown that student attendance is strongly correlated with performance (e.g., Jones, 1984; Launius, 1997; Rodgers, 2001; Sharma et al., 2005; Marburger, 2006). However, other studies have addressed how such attendance-performance relationships are not equal for all students and is dependent on numerous student characteristics. For example, more pronounced negative effects of poor attendance are observed for low-performing students (Westerman et al., 2011). Furthermore, student ambition (e.g., desire to meet job entry grade), personal study skills, work habit, self-motivation, and personal discipline all contribute to the relationship between attendance and performance (Lievens et al., 2002; Robbins et al., 2004; Credé and Kuncel, 2008; Credé et al., 2010). These interconnected factors can make attendance-performance relationships challenging to untangle.
There is also an extensive body of work showing that active learning techniques and the associated student engagement during teaching leads to a greater number of students meeting the learning outcomes and thus, by extension, improved academic performance. The methods for performing active learning and increasing student engagement are diverse and can include group pair-share exercises, benchtop demonstrations, cooperative problem solving exercises, peer-led inquiry, and research project experiences, for example (Farrell et al., 1999; Andersen, 2002; Seymour et al., 2004; Knight and Wood, 2005; Baldock and Chanson, 2006; Jones and Ehlers, 2021). However, irrespective of method, in general all these active learning strategies lead to increased student engagement and generally succeed in supporting students to meet learning outcomes (Froyd, 2007; Freeman et al., 2014). A large proportion of this previous work on student attendance, engagement and the relationship to performance has focused on synchronous in-person delivery. The extent to which these findings can be related to asynchronous online delivery and exactly what constitutes “engagement” and “attendance” in a purely online environment remains unclear and an active area of research.
There has been a growing move to online or hybrid learning. Despite the additional time investment required by the instructor to create effective online or hybrid teaching materials (McFarlin, 2008; Wieling and Hofman, 2010), there are multiple benefits and increased online learning may be an important method to widen participation across numerous and diverse student groups. Students can select the time and place to conduct their own learning, especially when all the material is delivered asynchronously. This supports students who have other commitments (e.g., caring responsibilities), live in remote geographical locations, or have health concerns, for example (Colorado and Eberle, 2012; Kahu et al., 2013; Johnson, 2015; Chung et al., 2022). It can also make the learning environment more inclusive, for example performing practical or fieldwork-based activities online enables participation by a wider number of students, especially those with disabilities (Giles et al., 2020). The move to online teaching was greatly accelerated due to the COVID-19 pandemic, forcing the global education sector, across all levels, to rapidly switch to exclusively online teaching (Mishra et al., 2020; Chung et al., 2022). Furthermore, going forward, universities worldwide are encouraged and sometimes required to offer a hybrid or blended learning approach for students (Chung et al., 2022).
Thus, given the importance and interrelationship between attendance, engagement, academic performance, and student wellbeing we must understand how to effectively monitor such factors in an online educational setting. Most of our attendance monitoring strategies have only been tested for in-person, traditional on-campus delivery. They remain untested for online teaching and such strategies might be unpractical. Specifically, here, I will address the following research questions:
• What methods can be used to effectively monitor attendance and engagement in a purely online environment?
• Does increased online attendance and engagement contribute to increased academic performance?
• What are the challenges faced when monitoring student attendance during asynchronous and synchronous online learning?
In this study, following these research questions, I show how student viewing statistics from the virtual learning environment (VLE) platform, Canvas, can and cannot be used to monitor “attendance” and “engagement.” Lastly, I also provide a perspective on the opportunities, challenges faced and potential pitfalls of using such data for student monitoring at universities.
Methods
Student Population and Setting
The data presented here were generated during an undergraduate course entitled “Volcanology and Geohazards” at the University of Liverpool, United Kingdom. The course was taken by 31 students of mixed gender during the second year of their undergraduate study. The course is a compulsory module for the undergraduate degree programs, BSc/MESci Geology and BSc/MESci Geology and Physical Geography, with 24 and 7 students enrolled on these programs respectively. The only difference between the BSc and MESci degree is the degree length, the BSc programs are 3 years whereas the MESci programs have an additional year of advanced study to form a 4-year program. The course analyzed here was taken by all students in their second year of study, thus is not impacted by variations in degree type (i.e., BSc vs. MESci). No age or ethnicity data is available for this study. This study was approved by the University of Liverpool’s ethics committee, details are provided in the Ethics Statement.
Course Information and the Virtual Learning Environment
The course analyzed in this study started on the 8th of February 2021 in the second (winter) semester of the 2020–2021 academic year and was delivered solely online. The course “Volcanology and Geohazards” ran for a total of 12 weeks. It was split equally between these topics, with the volcanology teaching occurring in the first 6 weeks and the geohazards component in weeks 7–12. The volcanology component is the sole focus here and comprised 10 pre-recorded asynchronous lectures, 2 online synchronous 1-h guest lectures and 5 online synchronous 2-h practical sessions (Table 1). The online practical sessions used handwritten calculation exercises and Excel based tasks to supplement material delivered in the online lectures. The students were assessed by an online timed quiz given in teaching week 6 and a final open book online essay exam question given in the summer examination period, due 16 weeks after the volcanology teaching began.
The synchronous delivery was performed using the video-conferencing platform, Zoom. All the asynchronous lectures and materials (e.g., lecture slides, question sheets, spreadsheets, solutions) associated with the synchronous sessions were made available to the students using the virtual learning environment (VLE), Canvas. The VLE can be accessed using a web browser on any device and is used for all courses at the University of Liverpool. Canvas is widely used in higher education settings both within the United Kingdom and globally, however, there is lots of flexibility and thus variability of how course content is structured on the VLE. Figure 1 shows the Canvas layout for the volcanology component of the course investigated here. From the volcanology component homepage (Figure 1) all the asynchronous teaching material can be accessed. The hyperlinks on the numbered and named lectures link to a separate page with the pre-recorded lecture video embedded. The hyperlinked numbered weeks at the bottom of the page (Figure 1) link to a file repository where the pdf copies of the lecture slides, the practical session questions sheets, resources, and practical solutions are located.
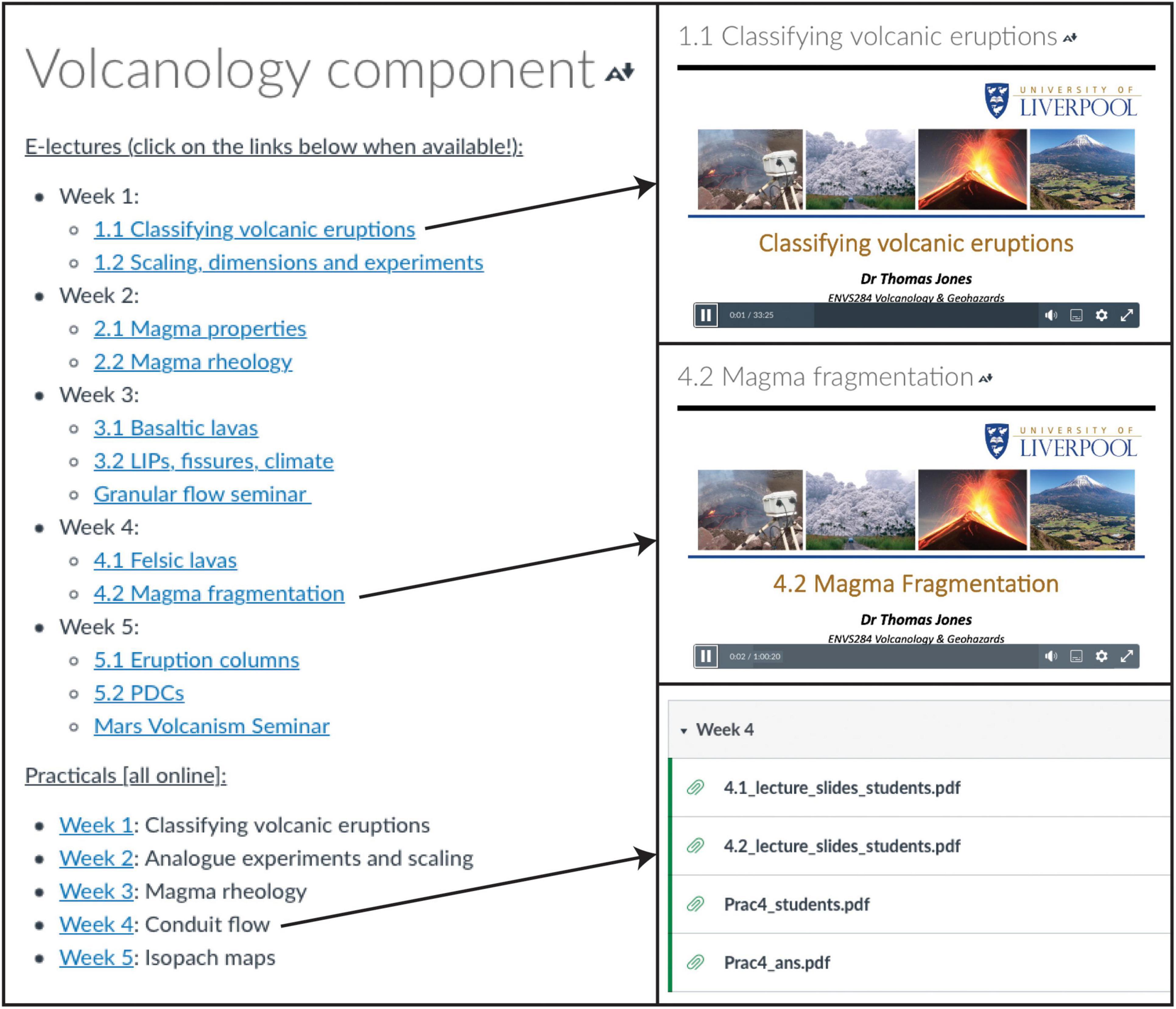
Figure 1. Screenshots of the VLE layout. The volcanology component home page is shown on the left. From this home page all the asynchronous teaching material can be accessed. The numbered lectures link to a page with the pre-recorded lecture video embedded. The hyperlinked week numbers (e.g., “Week 1”) listed at the bottom of the home page link to a file collection containing the pdf lecture slides, question sheets for the practical sessions and answers. Representative links are shown by the arrows.
Data Collection and Analysis Methods
In this study three types of data were collected: (a) synchronous attendance; (b) student grades and (c) access to materials on the VLE. For all data, immediately after collection, all student names were removed and each student was assigned a random number between 1 and 31 such that these data remained truly anonymous and could not be deduced by the alphabetical order of surnames, for example.
Attendance was recorded for all synchronous sessions (i.e., guest lectures and practical sessions). This was done by matching Zoom profile names to the class register. Student grades are used as a measure of performance and were taken from the online quiz, the essay exam, and the average of these two assignments, assuming an equal weighting to give a final volcanology grade. For comparative purposes, the average student grade obtained in the first 2 years of university study was also recorded. These data were taken directly from the university’s internal records system.
The access to the VLE, Canvas, was assessed using the built-in “new analytics” tool. For each student this tool lists the number of page/resource views in each week. The following data were manually extracted: the number of students that accessed each resource at least once; the total number of resource views for each student and, the week that each student accessed a resource. A second built-in tool entitled “Canvas studio” was used to extract viewing statistics related to the embedded asynchronous videos. For each student, and for each video, this tool shows the specific video segments that have been watched. These viewing data are reported for every 30-second video segment for videos less than 1 h in duration, and for every 1-min video segment for videos greater than 1 h in duration. Using this tool, for each asynchronous lecture video, the duration viewed by each student at least once was manually recorded. Unfortunately, it is not possible with the current VLE platform to identify how many times a student watched each asynchronous lecture video. Also, it cannot be determined whether the student viewed the lecture all at once or in a series of sittings. Once all these data had been collected, grouped into a spreadsheet, and anonymized it was imported into Matlab, where all data plotting and fitting was performed.
Results and Analysis
Engagement Depending on Course Position
As shown in the course syllabus (Table 1), each week had a unique set of learning materials assigned to it. In general, the material associated with the early teaching weeks were accessed by a greater number of students over the course duration relative to the material associated with the later teaching weeks (Figure 2). In addition to this slight reduction in engagement with the online material with position in the course (Figure 2), it can be seen that very few students went back to check their answers to the practical activities.
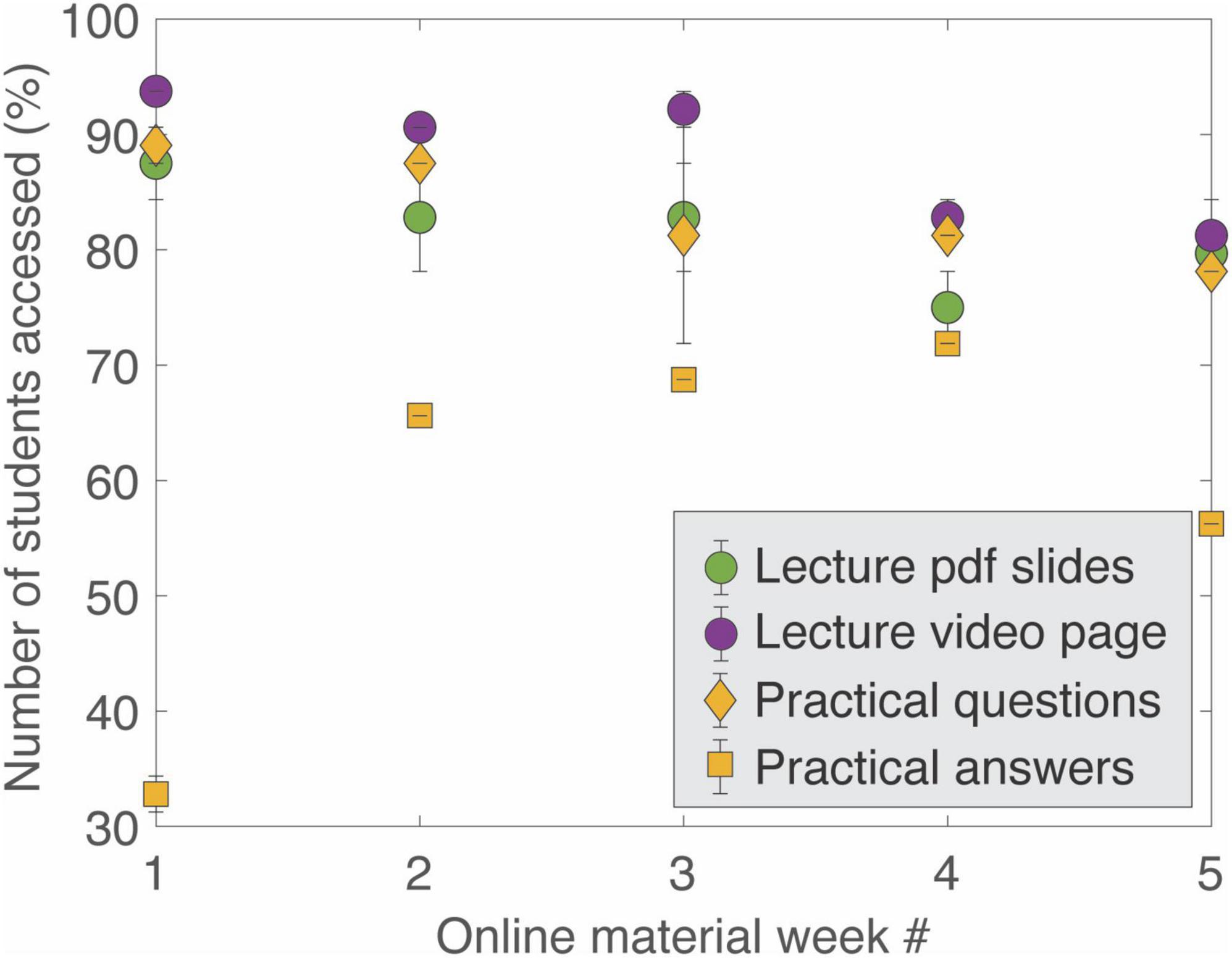
Figure 2. The proportion of students accessing the material provided on the virtual learning environment. The online material has been split per week, as detailed in the course syllabus (Table 1). Green circles represent the lecture slides in pdf format, purple circles represent the pages hosting the lecture videos, yellow diamonds represent the practical questions, and the yellow squares represent the answers to the practical exercises. Data points show the mean values, the error bars indicate the minimum and maximum values recorded.
Bulk Attendance and Access Data
Synchronous session attendance and the proportion of online page views can be compared to the students’ grades, used here as a proxy for academic performance. Comparisons were made for both assessment types; the volcanology quiz (Figure 3A) and the volcanology essay (Figure 3B) and for the final aggregated grade (Figure 3C) assuming an equal weighting between the essay and the quiz. No positive or negative correlations are observed between attendance/total page views and performance.
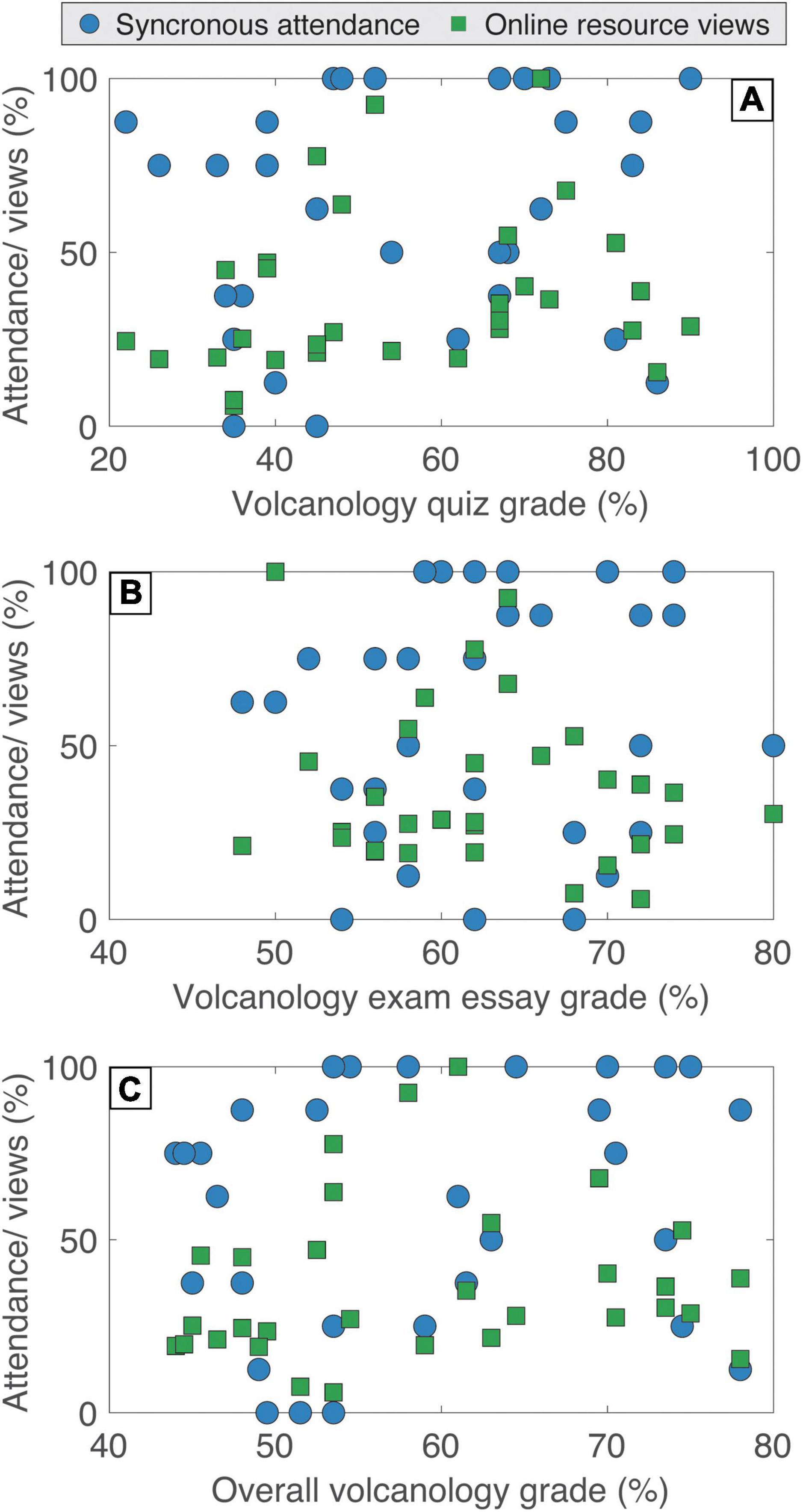
Figure 3. Relationships between student attendance and performance. The blue circles represent synchronous attendance during online guest lectures, practical sessions, and Q&A sessions. The green squares represent the virtual learning environment page views, normalized to the maximum number of page views recorded for an individual student. No correlations between attendance and student grade (a measure of performance) are observed for any of the assessments. The figure panels show (A) the quiz grade, (B) the exam essay grade and (C) the overall volcanology grade.
Time Series of Student Access
Student viewing and access data from the virtual learning environment, Canvas, also allows for the monitoring of student engagement with the online material as a function of time, rather than the bulk, course averaged data previously presented. The course was published on Canvas one week before teaching began (i.e., week 0) and included all the teaching material for week 1. Subsequent material was published on the Monday of the related teaching week. The material for week 1 received a small number (43) of views in the week before teaching began (Figure 4A). In teaching week 1 there was the second highest number of views (783), this gradually declined to reach a minimum (461) in week 3 before rising again in weeks 4 and 5. Week 6 experienced the highest number of views (1,046), although this week contained no new teaching material or timetabled synchronous sessions it hosted the volcanology quiz, which contributed to the final course grade obtained by the students. These views were dominated by pdf versions of the lecture slides, followed by the practical materials, followed lastly by the pages with the asynchronous lecture videos embedded. After the volcanology quiz in week 6 only a very small number of views (<40) occurred each week, and weeks 11 and 12 received zero views. Engagement rapidly increased the week before the final essay-based exam and reached 582 views in week 17 when the final exam was due. These views in weeks 16 and 17 were dominated by the embedded lecture videos and pdf copies of the slides.
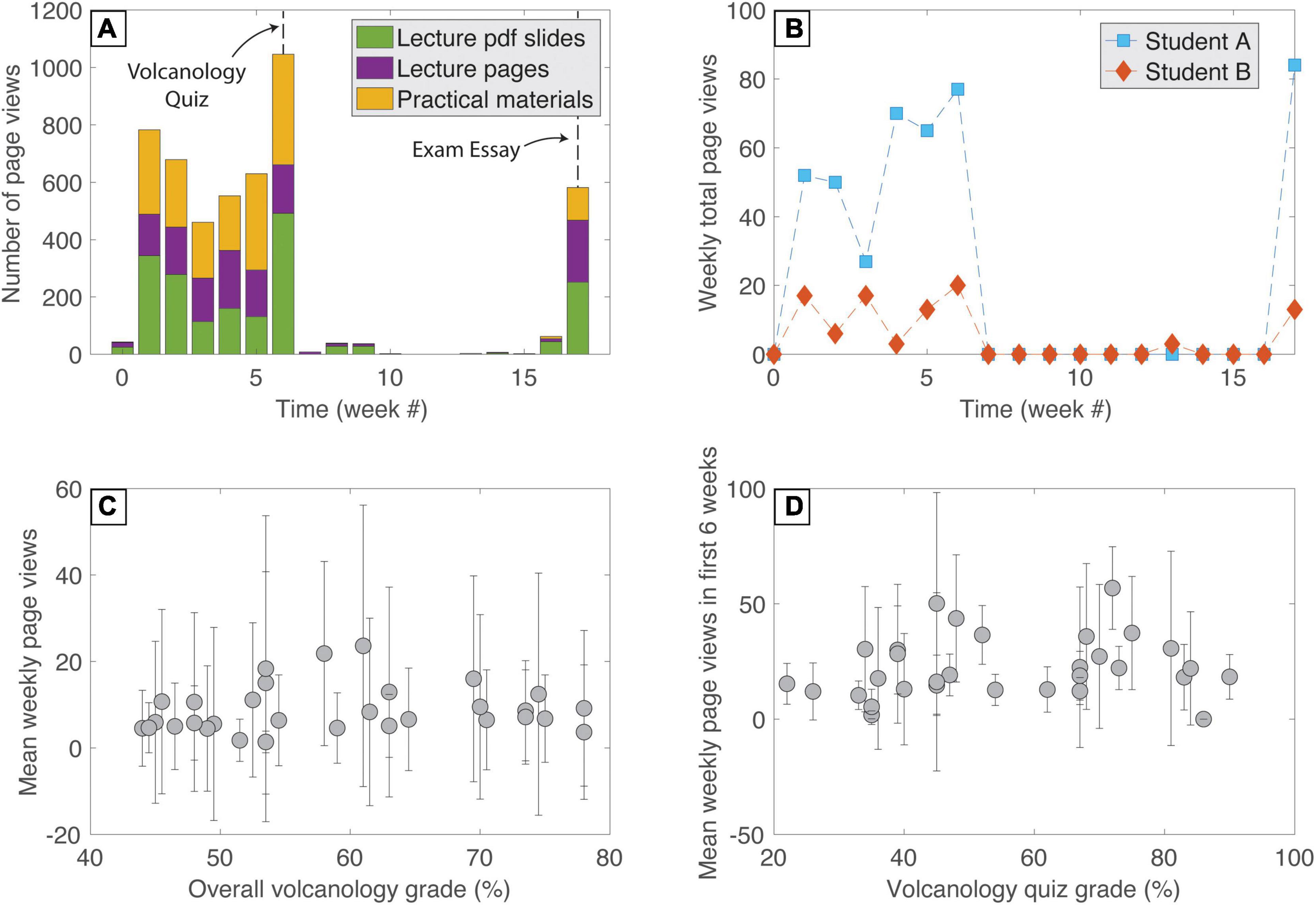
Figure 4. Time series of VLE access. (A) The total number of page views per week. The green shaded parts of the bar correspond to the pdf copies of the lecture slides. Purple corresponds to the VLE pages with the lecture video embedded and yellow corresponds to the practical question sheets and answers. Teaching began in week 1 and lasted for 5 weeks, the volcanology quiz was conducted in week 6 and the final essay-based exam was conducted in week 17. (B) Total number of page views as a function of week for two representative students. (C) Mean number of weekly page views compared to the overall volcanology grade obtained. (D) Mean number of weekly page views during weeks 1 through 6 compared to the volcanology quiz grade obtained. In both panels (C,D) the error bars represent one standard deviation of the student’s page views for weeks 0–17 and for weeks 1–6, respectively.
The time series of engagement/access to the online material can also be tracked for individual students, two examples are shown in Figure 4B. These data allow for interpretations to be made about student study style (e.g., intense “cramming” before examinations vs. sustained learning) and can be used by institutions for wellbeing checks (e.g., identifying sudden reductions in online engagement). The mean number of page views per week shows no correlation to the overall volcanology grade obtained by the student (Figure 4C). There is also no correlation between the volcanology quiz grade and the mean weekly page views within the first 6 weeks (Figure 4D). The standard deviation of weekly page views across both time frames (Figures 4C,D) can be linked to the study/viewing approach taken by the student. A small standard deviation indicates that the student regularly engaged with the online material on a weekly basis. Whereas a large standard deviation is indicative of uneven access to the online materials. The most common pattern observed was very little, to no engagement with the VLE until one or two weeks before the quiz and exam when activity rapidly increased. Over both time periods (Figures 4C,D), there is no correlation between standard deviation of views and academic performance (i.e., grade). Thus, these data do not provide evidence that support a preferred or optimum study plan.
Asynchronous Lecture Viewing Statistics
For each of the embedded lecture videos the portion of the video that was watched at least once was quantified for each student. An example of these data is shown in Figure 5A. This shows a typical pattern where the number of students viewing the video decreases with duration into the pre-recorded lecture. There were several students that accessed the VLE page that hosted the video, but did not watch the video in full, or in some cases did not watch any parts of the video. This illustrates the difference between simple page access data (e.g., Figure 4) and asynchronous lecture viewing statistics (Figure 5). To determine if video views are reflected in student performance, the portion of videos watched across the entire volcanology course (i.e., Lecture 1.1–5.2; Table 1) were calculated for each student. Figure 5B compares the total proportion of asynchronous lecture videos watched to the overall course grade obtained by the student. In general, there is a positive correlation between the proportion of the asynchronous lecture videos watched by the student and their overall course grade. However, for some students there is no correlation, despite watching all the videos in full they still achieved a low grade. To normalize these results, the student grades were compared to their average grade during the first 2 years of undergraduate study, termed the “grade difference” here (Figure 5C). Similarly, a weak positive correlation can be observed, where the students that watched more of the videos, in full, obtained a better grade relative to their personal average.
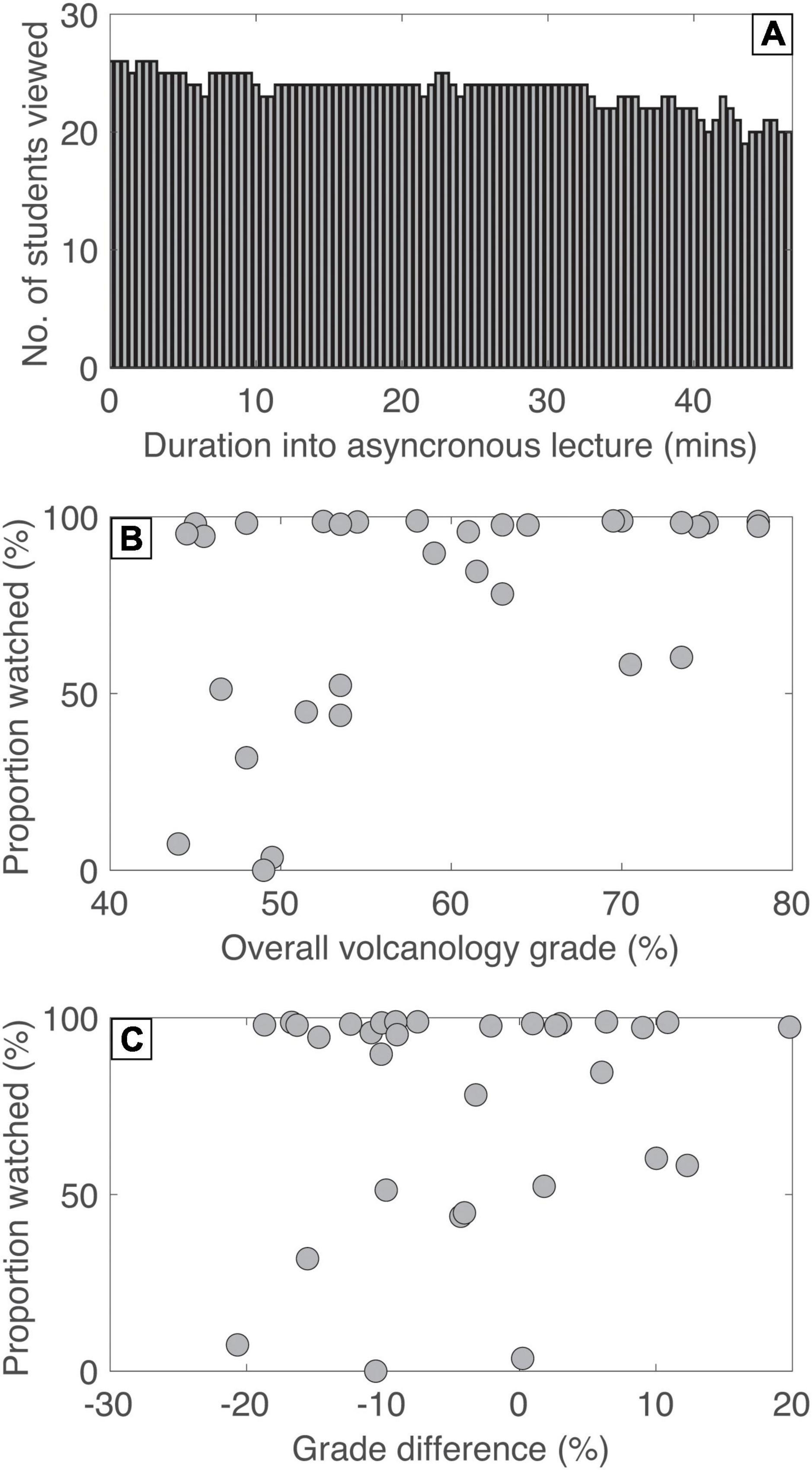
Figure 5. Viewing statistics of the embedded lecture videos. (A) The number of students that viewed each half minute segment of Lecture 1.2 at least once. Note that only one lecture (Lecture 1.2) is shown for illustrative purposes, but this analysis was performed on all asynchronous lecture videos. (B,C) The proportion of all asynchronous lecture videos that were watched at least once compared to: (B) the overall grade obtained by each student; (C) the grade difference between the average grade obtained in the first 2 years of university study and the overall volcanology grade. A positive grade difference indicates that the student did better in the volcanology course relative to their university average.
Discussion and Perspectives
Attendance in a Purely Online Environment
During traditional on-campus, in-person delivery attendance is frequently monitored using registers. This is done for multiple reasons, including, but not limited to, government reporting requirements, the link between attendance and academic performance, and pastoral care (Friedman et al., 2001; Macfarlane, 2013; Oldfield et al., 2019). Where a sudden reduction in attendance can be related to concerns over student wellbeing and student drop-out (Smith and Beggs, 2002; Boulton et al., 2019). The use of technology such as in-class quizzes or the use of personal response systems (e.g., “clickers”) can reduce the burden of collecting attendance data (Hoekstra, 2008) and when the responses are used to constitute part of the course grade they can provide an additional incentive for students to attend. Furthermore, these approaches introduce a component of active learning into an otherwise passive lecture (Gauci et al., 2009). Presenting students with clicker questions part-way through a lecture, for example, requires the learners to actively engage with the lecture material and apply their knowledge. When answers are subsequently presented it offers the students immediate feedback. The incorporation of active learning in this way is widely accepted to be beneficial to both student learning and experience (Froyd, 2007; Freeman et al., 2014). Furthermore, active learning increases student engagement during sessions which has also been shown to increase student performance (e.g., Handelsman et al., 2005; Kuh et al., 2008; Casuso-Holgado et al., 2013; Ayala and Manzano, 2018; Vizoso et al., 2018; Büchele, 2021). Thus, it is not just simple attendance that matters, the level of student engagement is also a key contributing factor.
However, effectively monitoring attendance and engagement and incorporating active learning into online delivery represents a key challenge faced during the rapid changes brought about by the COVID-19 pandemic (Andrews, 2021; Gribble and Wardrop, 2021; Symons, 2021). During online synchronous activities online equivalents to clickers can be used such as Zoom polls or polls based on web browsers. During online synchronous activities I suggest that documenting poll responses might be the best way to accurately register attendance. The use of digital bulletin or ideas boards such as Padlet can also facilitate student engagement online and have been shown to enhance cognitive engagement and learning (Ali, 2021; Gill-Simmen, 2021). Breakout rooms in video conferencing software such as Zoom or Microsoft Teams and the use of private channels in Microsoft Teams can encourage engagement and discussion between group members (Corradi, 2021; McMenamin and von Rohr, 2021). However, all these approaches require an additional, often large, time investment by the instructor. This was particularly difficult in the early stages of the pandemic when we all had to rapidly adjust to a new way of working. A quick way to monitor attendance during online synchronous delivery is to simply take a register of the attendees signed into the video conferencing software of choice (e.g., Zoom, WebEx, Microsoft Teams). This was the approach taken in this study; however, this does not necessarily constitute attendance and certainly cannot measure engagement. This may, at least in part, explain the lack of correlation observed here (Figure 3). The student could easily log on at the start of the synchronous session and then not watch the session. For example, it would be easy to mute the volume, perform other work on another device, or even leave the room. Indeed, from personal experience it is common for a small number (1 or 2 students) to remained logged on, with video cameras turned off, and not participate/engage with the material delivered. If the session ended a few minutes early, directed conversation toward these students clearly revealed that they were not listening or engaged with the session despite “attending.” Instructors should therefore use caution when documenting attendance in this way. One potential solution to this is to encourage students to turn their video cameras on, however, it is good practice not to enforce this because of the associated issues surrounding privacy invasion, inclusivity, and the access to stable internet connections (Darici et al., 2021). The instructor could explain to the students the benefits of video vs. purely audio interaction and allow the students to make their own informed decision.
Monitoring attendance and engagement during online asynchronous activities proves even more challenging. One method, as performed in this study, is to monitor student engagement with the VLE platform. A growing number of educational institutions are tracking student log in to the VLE platform to identify drops in access. Student support services can then follow up with individual students and offer further support and pastoral care as required. Despite being a quick and convenient method of quantifying online engagement, documenting the number of page views does pose challenges. Students may go through clicking on many pages on the VLE without properly reading or digesting the material, they may also download the material (e.g., lecture slide pdfs) and view them offline, rather than within the VLE browser. These caveats limit the use of VLE page viewing statistics as an appropriate metric for assessing online student engagement (cf. Figures 3, 4). This therefore questions the value and time investment spent collecting these data by instructors and administrative teams.
Effective Monitoring of Attendance Through a Virtual Learning Environment
In this study I have shown that these synchronous attendance data and the VLE page viewing statistics do not show any measurable relationship to student performance. Some key reasons for this lack in correlation have been detailed above. The only metric that showed some correlation to student performance was the proportion of the asynchronous lecture videos viewed (Figure 5). The results are broadly in line with studies of physical attendance during in-person delivery where the correlation is positive—increased attendance increases student performance (e.g., Jones, 1984; Launius, 1997; Rodgers, 2001; Sharma et al., 2005; Marburger, 2006). The proportion of video viewed by each student is therefore a better way of measuring “attendance” relative to simple page views. Although it is impossible to determine if the student was truly listening tentatively to the asynchronous lecture video, at the very least it can be determined that: (a) it was played and (b) for what specific duration. For example, I observed that a small number of students (<5) sometimes viewed a page that hosted the embedded lecture video but never watched the video. Furthermore, the metric is more robust against students trying to falsely register attendance—if another tab is opened within the web browser the VLE does not record this time as viewing. Therefore, this level of analysis can also provide some benefits over synchronous online lectures given to participants without webcams enabled. Due to these multiple factors, I suggest that video viewing statistics offer the best way to monitor “attendance” during online, asynchronous delivery.
Lessons Learnt for Providing Online Materials
There is debate within the literature surrounding what constitutes an ideal virtual learning environment, however, it is broadly agreed that building good instructor-student relations, motivating students to do their best, and increased interpersonal interaction are most beneficial (e.g., Fredericksen et al., 1999; Young, 2006; Jaggars and Xu, 2016). Additionally, some studies suggest that the exact layout and structure of the material provided on the VLE platform may not directly influence student performance (Jaggars and Xu, 2016). Here, I do not evaluate the role of the VLE layout on performance, rather, I provide a perspective on useful VLE layouts for effective monitoring of student “attendance” and “engagement.”
Separating out different course content (e.g., scientific topics) and components (e.g., practical exercises, lectures), each on a unique, separate VLE page allows for a more detailed level of engagement analysis. This is more useful than bulk access data, used to determine whether an individual student has logged into the VLE platform or not. Although I have shown that page viewing data does not show any direct, measurable relationship to student performance, these individual page viewing data can have benefits. Now, I provide three examples of how separate VLE pages and their associated access data might be useful.
First, separating out the solutions/answers to practical exercises from the question sheets allows the instructor to determine the proportion of students that are checking their answers, forming a key component of knowledge consolidation. The instructor or student support teams may then choose to approach students who are less engaged with these online materials to ascertain why and provide further support as appropriate. Second, providing the recommended reading or links to this material on a separate VLE page allows the instructor to determine the number of students further supporting their learning outside of the timetabled activities. Third, providing lecture slides in addition to the asynchronous recording is often considered good practice to facilitate different learning types (e.g., Auditory vs. reading) (Fleming, 1995). Again, separating these out on different VLE pages allows the instructor to quantitatively assess the proportion of students who read, listen or both read and listen to the lecture material. This also allows the students to tailor their study method to their own personal learning style or circumstance (e.g., internet bandwidth too low for video streaming).
However, these suggestions of restructuring VLE layouts and the associated monitoring student access data present a large additional time investment by the instructor. Given the lack of correlation between total page views and student performance reported here it is not recommended that such statistics are routinely monitored. Rather, as detailed in the examples above, instructor time should be invested in monitoring VLE engagement to address specific questions (e.g., what proportion of students are checking their answers to in-class exercises?). Furthermore, for these data to be useful, additional resources are required to interact, support and/or encourage those students who show limited engagement. Lastly, all these online VLE monitoring approaches are prone to student manipulation. Once students know what statistics (e.g., page clicks, video views) are being monitored it is easy to “cheat the system” and falsify the data. Instructors should therefore be careful about regularly reminding students that such data is being monitored.
Limitations and Future Work
A limitation of this work is the small sample size used. Only 31 students, in one undergraduate course participated in this study and a clear avenue for future work would be to test these results and perspectives on a larger sample set featuring a diverse group of students, studying a range of subjects over multiple academic years. In this study the video viewing data extracted from the VLE, Canvas, did not quantify the number of times each student viewed the asynchronous lecture video—just whether a time segment had been viewed at least once. Upgrading the viewing statistics code within the VLE would allow us to test if the number of views influences student performance. Furthermore, the VLE cannot capture data on whether students work together when they study. The VLE will only log one student as accessing a resource, even if there were a group in the room watching the recording together. Given the COVID-19 restrictions in place when this course was taught, group VLE accesses are unlikely here but should be considered in future studies.
This study is built upon the premise that the lecture and practical materials addressed learning objectives that were subsequently tested in the assessments to determine a student’s grade. This has traditionally been the case where the timetabled activities directly contribute to the learning objectives. In these cases, the link between attendance and student performance is relatively straightforward. However, with our growing transition to a hybrid or blended learning model where timetabled activities can comprise, for example, open discussion forums, flipped classrooms and student seminars, the link between (online) attendance and performance could be further complicated. It will be difficult to isolate the impact of increased attendance from the other benefits provided by a blended learning model (e.g., McFarlin, 2008; Al-Qahtani and Higgins, 2013). Despite this, the key message here remains true. Simple VLE log in or page viewing is not sufficient to determine student attendance and engagement and with our ever-increasing use of online materials this must be reconsidered.
Summary and Conclusion
In this study it has been shown that attendance during online synchronous activities and the number and frequency of VLE page views do not clearly correlate with student performance. This is in contrast with numerous studies (e.g., Jones, 1984; Launius, 1997; Rodgers, 2001; Sharma et al., 2005; Marburger, 2006; Büchele, 2021) that have demonstrated a positive relationship between increased in-person attendance and student grades (a proxy for performance). The reasons behind the disconnect between attendance and performance in an online, vs. in-person setting are complex and comprised of numerous inter-related factors. The ease of falsely registering attendance online (e.g., logging into Zoom and muting the volume) and the unknown level of engagement (e.g., clicking on VLE pages but not reading the content) are key examples. Given that basic VLE access statistics (e.g., number of page views/clicks) show no measurable relationship to performance, we need a better method to monitor attendance and engagement in an online setting. This is a key pedagogical implication of this research and one that requires further investigation.
One method that has shown some promise is the use of asynchronous lecture video viewing statistics. Specifically, the total proportion of the videos watched by a student has been shown to be weakly correlated with performance. However, this is unlikely to be the full solution. Obtaining and analyzing these data requires a considerable time investment by the instructor which might not be possible for all courses. Furthermore, the exclusive use of lecture viewing data assumes that all the learning objectives are met in this manner. It is not robust against courses where all (or a portion) of the learning objectives are met through group activities, flipped classroom sessions, or in-person laboratory tasks, for example. Looking forward we must ascertain what methods are appropriate for accurately monitoring attendance and engagement in an online and hybrid teaching model. This requires careful investigation and as a community we should be cautious when using bulk, yet easily obtainable, VLE access data.
Data Availability Statement
The original contributions presented in the study are included in the article/supplementary material, further inquiries can be directed to the corresponding author/s.
Ethics Statement
This study was approved by the University of Liverpool’s ethics committee. Before any of these data used in this study were collected an email was sent to all students. The email informed the students about the use of these data to be collected and gave the students an option to opt out of the study. No students opted out. For all data, immediately after collection, all student names were removed and each student was assigned a random number between 1 and 31 such that these data remained truly anonymous and could not be deduced by the alphabetical order of surnames, for example. No other identifying characteristics were ever collected. Written informed consent for participation was not required for this study in accordance with the national legislation and the institutional requirements.
Author Contributions
TJ led all parts of the study, from project conceptualization to manuscript writing and submission.
Conflict of Interest
The author declares that the research was conducted in the absence of any commercial or financial relationships that could be construed as a potential conflict of interest.
Publisher’s Note
All claims expressed in this article are solely those of the authors and do not necessarily represent those of their affiliated organizations, or those of the publisher, the editors and the reviewers. Any product that may be evaluated in this article, or claim that may be made by its manufacturer, is not guaranteed or endorsed by the publisher.
Acknowledgments
I would like to thank Eli Saetnan and Judith Schoch from The Academy at the University of Liverpool for discussions that led to and improved this study. Three reviewers provided constructive comments that also improved this contribution.
References
Ali, A. (2021). Using Padlet as a Pedagogical Tool. J. Learn. Dev. High. Educ. 2021:799. doi: 10.47408/jldhe.vi22.799
Al-Qahtani, A. A. Y., and Higgins, S. E. (2013). Effects of traditional, blended and e-learning on students’ achievement in higher education. J. Comput. Assist. Learn. 29, 220–234. doi: 10.1111/j.1365-2729.2012.00490.x
Andersen, C. B. (2002). Understanding carbonate equilibria by measuring alkalinity in experimental and natural systems. J. Geosci. Educ. 50, 389–403. doi: 10.5408/1089-9995-50.4.389
Andrews, J. (2021). Transition online: challenges and achievements. J. Learn. Dev. High. Educ. 2021:703. doi: 10.47408/jldhe.vi22.703
Ayala, J. C., and Manzano, G. (2018). Academic performance of first-year university students: The influence of resilience and engagement. High. Educ. Res. Dev. 37, 1321–1335. doi: 10.1080/07294360.2018.1502258
Baldock, T. E., and Chanson, H. (2006). Undergraduate teaching of ideal and real fluid flows: the value of real-world experimental projects. Eur. J. Eng. Educ. 31, 729–739. doi: 10.1080/03043790600911837
Boulton, C. A., Hughes, E., Kent, C., Smith, J. R., and Williams, H. T. P. (2019). Student engagement and wellbeing over time at a higher education institution. PLoS One 14:e0225770. doi: 10.1371/journal.pone.0225770
Büchele, S. (2021). Evaluating the link between attendance and performance in higher education: the role of classroom engagement dimensions. Assess. Eval. High. Educ. 46, 132–150. doi: 10.1080/02602938.2020.1754330
Casuso-Holgado, M. J., Cuesta-Vargas, A. I., Moreno-Morales, N., Labajos-Manzanares, M. T., Barón-López, F. J., and Vega-Cuesta, M. (2013). The association between academic engagement and achievement in health sciences students. BMC Med. Educ. 13, 1–7. doi: 10.1186/1472-6920-13-33
Chung, J., McKenzie, S., Schweinsberg, A., and Mundy, M. E. (2022). Correlates of Academic Performance in Online Higher Education: a Systematic Review. Front. Educ. 7:820567. doi: 10.3389/feduc.2022.820567
Clump, M. A., Bauer, H., and Whiteleather, A. (2003). To attend or not to attend: Is that a good question? J. Instr. Psychol. 30, 220–224.
Colorado, J. T., and Eberle, J. (2012). Student demographics and success in online learning environments. Emporia State Res. Stud. 46, 4–10.
Corradi, H. R. (2021). Does Zoom allow for efficient and meaningful group work? Translating staff development for online delivery during Covid-19. J. Learn. Dev. High. Educ. 2021:697. doi: 10.47408/jldhe.vi22.697
Credé, M., and Kuncel, N. R. (2008). Study habits, skills, and attitudes: the third pillar supporting collegiate academic performance. Perspect. Psychol. Sci. 3, 425–453. doi: 10.1111/j.1745-6924.2008.00089.x
Credé, M., Roch, S. G., and Kieszczynka, U. M. (2010). Class attendance in college: a meta-analytic review of the relationship of class attendance with grades and student characteristics. Rev. Educ. Res. 80, 272–295. doi: 10.3102/0034654310362998
Darici, D., Reissner, C., Brockhaus, J., and Missler, M. (2021). Implementation of a fully digital histology course in the anatomical teaching curriculum during COVID-19 pandemic. Ann. Anatomy-Anatomischer Anzeiger 236:151718. doi: 10.1016/j.aanat.2021.151718
Farrell, J. J., Moog, R. S., and Spencer, J. N. (1999). A guided-inquiry general chemistry course. J. Chem. Educ. 76:570. doi: 10.1021/ed076p570
Fleming, N. D. (1995). “I’m different; not dumb. Modes of presentation (VARK) in the tertiary classroom,” in Research and development in higher education, Proceedings of the 1995 Annual Conference of the Higher Education and Research Development Society of Australasia (HERDSA), (HERDSA), 308–313. doi: 10.1016/j.nedt.2008.06.007
Fredericksen, E., Swan, K., Pelz, W., Pickett, A., and Shea, P. (1999). Student satisfaction and perceived learning with online courses-principles and examples from the SUNY learning network. Proc. ALN Sum. Workshop 1999:1999.
Freeman, S., Eddy, S. L., McDonough, M., Smith, M. K., Okoroafor, N., Jordt, H., et al. (2014). Active learning increases student performance in science, engineering, and mathematics. Proc. Natl. Acad. Sci. 111, 8410–8415. doi: 10.1073/pnas.1319030111
Friedman, P., Rodriguez, F., and McComb, J. (2001). Why students do and do not attend classes: Myths and realities. Coll. Teach. 49, 124–133. doi: 10.1080/87567555.2001.10844593
Froyd, J. E. (2007). Evidence for the efficacy of student-active learning pedagogies. Proj. Kaleidosc. 66, 64–74.
Gauci, S. A., Dantas, A. M., Williams, D. A., and Kemm, R. E. (2009). Promoting student-centered active learning in lectures with a personal response system. Adv. Physiol. Educ. 33, 60–71. doi: 10.1152/advan.00109.2007
Giles, S., Jackson, C., and Stephen, N. (2020). Barriers to fieldwork in undergraduate geoscience degrees. Nat. Rev. Earth Environ. Environ. 1, 77–78. doi: 10.1038/s43017-020-0022-5
Gill-Simmen, L. (2021). Using Padlet in instructional design to promote cognitive engagement: a case study of undergraduate marketing students. J. Learn. Dev. High. Educ. 2021, 575. doi: 10.47408/jldhe.vi20.575
Gribble, L., and Wardrop, J. (2021). Learning by engaging: connecting with our students to keep them active and attentive in online classes. J. Learn. Dev. High. Educ. 2021:701. doi: 10.47408/jldhe.vi22.701
Gump, S. E. (2005). The cost of cutting class: attendance as a predictor of success. Coll. Teach. 53, 21–26. doi: 10.3200/ctch.53.1.21-26
Handelsman, M. M., Briggs, W. L., Sullivan, N., and Towler, A. (2005). A measure of college student course engagement. J. Educ. Res. 98, 184–192. doi: 10.3200/joer.98.3.184-192
Hoekstra, A. (2008). Vibrant student voices: exploring effects of the use of clickers in large college courses. Learn. Media Technol. 33, 329–341. doi: 10.1080/17439880802497081
Jaggars, S. S., and Xu, D. (2016). How do online course design features influence student performance? Comput. Educ. 95, 270–284. doi: 10.1016/j.compedu.2016.01.014
Johnson, G. M. (2015). On-campus and fully-online university students: comparing demographics, digital technology use and learning characteristics. J. Univ. Teach. Learn. Pract. 12:4.
Jones, C. H. (1984). Interaction of absences and grades in a college course. J. Psychol. 116, 133–136. doi: 10.1080/00223980.1984.9923627
Jones, T. J., and Ehlers, T. A. (2021). Using benchtop experiments to teach dimensional analysis and analogue modelling to graduate geoscience students. J. Geosci. Educ. 69, 313–322. doi: 10.1080/10899995.2020.1855040
Kahu, E. R., Stephens, C., Leach, L., and Zepke, N. (2013). The engagement of mature distance students. High. Educ. Res. Dev. 32, 791–804. doi: 10.1080/07294360.2013.777036
Knight, J. K., and Wood, W. B. (2005). Teaching more by lecturing less. Cell Biol. Educ. 4, 298–310. doi: 10.1187/05-06-0082
Kuh, G. D., Cruce, T. M., Shoup, R., Kinzie, J., and Gonyea, R. M. (2008). Unmasking the effects of student engagement on first-year college grades and persistence. J. Higher Educ. 79, 540–563. doi: 10.1353/jhe.0.0019
Launius, M. H. (1997). College student attendance: attitudes and academic performance. Coll. Stud. J. 31, 86–92.
Lievens, F., Coetsier, P., De Fruyt, F., and De Maeseneer, J. (2002). Medical students’ personality characteristics and academic performance: a five-factor model perspective. Med. Educ. 36, 1050–1056. doi: 10.1046/j.1365-2923.2002.01328.x
Macfarlane, B. (2013). The surveillance of learning: A critical analysis of university attendance policies. High. Educ. Q. 67, 358–373. doi: 10.1111/hequ.12016
Marburger, D. R. (2006). Does mandatory attendance improve student performance? J. Econ. Educ. 37, 148–155. doi: 10.3200/jece.37.2.148-155
McFarlin, B. K. (2008). Hybrid lecture-online format increases student grades in an undergraduate exercise physiology course at a large urban university. Adv. Physiol. Educ. 32, 86–91. doi: 10.1152/advan.00066.2007
McMenamin, J., and von Rohr, M.-T. R. (2021). Private online channels and student-centred interaction. J. Learn. Dev. High. Educ. 2021:689. doi: 10.47408/jldhe.vi22.689
Mishra, L., Gupta, T., and Shree, A. (2020). Online teaching-learning in higher education during lockdown period of COVID-19 pandemic. Int. J. Educ. Res. Open 1:100012. doi: 10.1016/j.ijedro.2020.100012
Oldfield, J., Rodwell, J., Curry, L., and Marks, G. (2019). A face in a sea of faces: exploring university students’ reasons for non-attendance to teaching sessions. J. Furth. High. Educ. 43, 443–452. doi: 10.1080/0309877x.2017.1363387
Robbins, S. B., Lauver, K., Le, H., Davis, D., Langley, R., and Carlstrom, A. (2004). Do psychosocial and study skill factors predict college outcomes? A meta-analysis. Psychol. Bull. 130, 261. doi: 10.1037/0033-2909.130.2.261
Rodgers, J. R. (2001). A panel-data study of the effect of student attendance on university performance. Aust. J. Educ. 45, 284–295. doi: 10.2147/AMEP.S226255
Seymour, E., Hunter, A.-B., Laursen, S. L., and DeAntoni, T. (2004). Establishing the benefits of research experiences for undergraduates in the sciences: first findings from a three-year study. Sci. Educ. 88, 493–534. doi: 10.1002/sce.10131
Sharma, M. D., Mendez, A., and O’Byrne, J. W. (2005). The relationship between attendance in student-centred physics tutorials and performance in university examinations. Int. J. Sci. Educ. 27, 1375–1389. doi: 10.1080/09500690500153931
Smith, E. M., and Beggs, B. J. (2002). “Optimally maximising student retention in Higher Education,” in SRHE Annual Conference, (Glasgow), 2002. doi: 10.1186/s12960-019-0432-y
Symons, K. (2021). “Can you hear me? Are you there?”: student engagement in an online environment. J. Learn. Dev. High. Educ. 2021:730. doi: 10.47408/jldhe.vi22.730
Vizoso, C., Rodríguez, C., and Arias-Gundín, O. (2018). Coping, academic engagement and performance in university students. High. Educ. Res. Dev. 37, 1515–1529. doi: 10.1080/07294360.2018.1504006
Westerman, J. W., Perez-Batres, L. A., Coffey, B. S., and Pouder, R. W. (2011). The relationship between undergraduate attendance and performance revisited: alignment of student and instructor goals. Decis. Sci. J. Innov. Educ. 9, 49–67. doi: 10.1111/j.1540-4609.2010.00294.x
Wieling, M. B., and Hofman, W. H. A. (2010). The impact of online video lecture recordings and automated feedback on student performance. Comput. Educ. 54, 992–998. doi: 10.1016/j.compedu.2009.10.002
Keywords: academic performance, online education, virtual learning environment, student attendance, online engagement, online higher education, course design
Citation: Jones TJ (2022) Relationships Between Undergraduate Student Performance, Engagement, and Attendance in an Online Environment. Front. Educ. 7:906601. doi: 10.3389/feduc.2022.906601
Received: 28 March 2022; Accepted: 04 April 2022;
Published: 09 May 2022.
Edited by:
Lucas Kohnke, The Education University of Hong Kong, Hong Kong SAR, ChinaReviewed by:
Di Zou, The Education University of Hong Kong, Hong Kong SAR, ChinaAndrew Jarvis, Hong Kong Polytechnic University, Hong Kong SAR, China
Mark Bedoya Ulla, Walailak University, Thailand
Copyright © 2022 Jones. This is an open-access article distributed under the terms of the Creative Commons Attribution License (CC BY). The use, distribution or reproduction in other forums is permitted, provided the original author(s) and the copyright owner(s) are credited and that the original publication in this journal is cited, in accordance with accepted academic practice. No use, distribution or reproduction is permitted which does not comply with these terms.
*Correspondence: Thomas J. Jones, dGhvbWFzLmpvbmVzQGxpdmVycG9vbC5hYy51aw==