- 1Mary Lou Fulton Teachers College, Arizona State University, Tempe, AZ, United States
- 2Mathematics Department, Northwest Vista College, San Antonio, TX, United States
- 3College of Integrative Sciences and Arts, Arizona State University, Tempe, AZ, United States
Introductory courses in biology often act as a gateway for students seeking careers in healthcare and science-related fields. As such, they provide a prime entry point for innovations seeking to enhance students’ learning of foundational content. Extant innovations and interventions have been found to positively impact students’ study strategy use with concomitant impacts on course exams and grades. These innovations, however, often have associated time and other costs, which may ultimately limit more widespread use. Our study builds on prior findings by exploring the extent to which students evidence increased use of effective study strategies after engaging in a brief (i.e., 15-min), online module requiring no financial cost for students or time commitment from instructors, and whether changes in students’ use of effective study strategies are associated with changes in exam performance. The present study employed a brief, online module designed to support undergraduate students’ (n = 98) use of effective study strategies in an introductory human anatomy and physiology course. Through a pretest-posttest design, students described the strategies they used to study and completed four cognitive and metacognitive subscales before and after engaging in a brief, online module designed to teach them about effective study strategies. Results were somewhat mixed: students evidenced a modest, statistically significant increase in the number of strategies used and changes in strategy use were associated with changes in exam score only for some measures. Notably, this relationship was not moderated by GPA, suggesting that the strength of the relationship between changes in strategy use and changes in exam scores were not different depending on students’ levels of prior academic performance. Taken together, the innovation was associated with increases in students’ exam scores, irrespective of GPA, but future research should explore the refinement and extension of the innovation to explore ways that increase efficacy and impact while still balancing sustainable implementation to account for challenges associated with instructor supervision and training, financial costs, and students’ time.
Introduction
There is often a disconnect between the effective study strategies and learning techniques that educational experts know to be most effective for learners (Kornell and Bjork, 2007; Karpicke et al., 2009; Weinstein et al., 2010; Hartwig and Dunlosky, 2012; Dunlosky et al., 2013; Vemu et al., 2022) and the ones that students employ when they study. Practice testing and distributed practice, for example, are more efficacious for learning and comprehension than summarizing, highlighting, or rereading (Dunlosky et al., 2013; Adesope et al., 2017). Despite this evidence, undergraduate students often rely on less effective techniques, such as rereading (Karpicke et al., 2009) and waiting to study until right before a test (i.e., massed practice or “cramming,” Blasiman et al., 2017). Ultimately, many students enter colleges and universities underprepared for how to learn (Kiewra, 2002; Wingate, 2007; Kritzinger et al., 2018). Recognizing this disconnect, educational researchers have long endeavored to support students’ use of effective study strategies (Hattie et al., 1996) and delineate ways to help college students use these strategies successfully (Cook et al., 2013; Zhao et al., 2014; Broadbent and Poon, 2015; Muteti et al., 2021; Theobald, 2021).
Undergraduate students enrolled in introductory biology courses are a particularly important subpopulation with contextual demands that set them apart from other majors within the university and may particularly benefit from engaging in more effective study strategies (Roediger and Butler, 2011; Hartwig and Dunlosky, 2012; Blasiman et al., 2017; Kritzinger et al., 2018; Vemu et al., 2022). For example, students taking biology-related courses, such as human anatomy and physiology, are often seeking health science careers, and thus, these courses may serve as a “gateway” into those careers, in effect, granting or limiting access (Koch, 2017; Hensley et al., 2021; Muteti et al., 2021). Students that employ better study strategies may be more likely to excel in the course, learn more, and ultimately be retained in their chosen field. For example, Schneider and Preckel (2017) found in their meta-analysis that students utilizing study strategies, such as elaboration or retrieval practice, performed at higher rates than peers who do not use those strategies. This notion is further impacted by the finding from a study by Marbach-Ad et al. (2016) where biology students that had lower grade point averages (GPAs) placed a higher value on retention skills (e.g., rote memorization of concepts, such as listing the bones in the body) over transfer skills (e.g., deeper understanding of content, such as application of concepts to health care contexts). Further, Ley and Young (1998) found that students who entered college underprepared (i.e., classified as taking a developmental or remedial class) not only used fewer total strategies but also used them with less consistency than their regular admission peers. Given that students with the greatest need may use strategies less frequently and have a greater predisposition toward reliance on less effective rehearsal strategies, they may benefit from targeted support.
A growing body of innovations designed to support biology students’ study strategy use has emerged over the past 2 decades (Minchella et al., 2002; Sebesta and Bray Speth, 2017; Bernacki et al., 2020; Hensley et al., 2021). Our study builds on prior findings by exploring the extent to which students evidence increased use of effective study strategies after engaging in a brief (i.e., 15-min), online module requiring no financial cost for students or time commitment from instructors, and whether students’ increased use of effective study strategies is associated with increased exam performance. We also examine whether the strength of that potential relationship is moderated by GPA.
Approaches to supporting students’ study strategy use have emerged from different frameworks, including self-regulated learning (SRL; Zimmerman, 2000, 2002) and desirable difficulties (Bjork and Bjork, 2011). While SRL has been conceptualized in multiple ways, many researchers have centered the three-phase approach forwarded by Zimmerman (2000, 2002), whereby learning occurs through a cyclical process involving forethought (i.e., before), performance (i.e., during), and self-reflection (i.e., after). Each of these interdependent phases plays a critical role in how individuals learn. For example, students’ self-motivation beliefs are an important subprocess of the forethought phase. The extent to which students come to an intervention already possessing intrinsic interest or value, self-efficacy, or mastery-oriented goals will impact their engagement in sustained study efforts and learning (Eccles, 1983; Bandura, 1986; Ames and Archer, 1988; Zimmerman and Schunk, 1989; Zimmerman, 2002).
Many students enrolled in introductory biology courses are pursuing careers in healthcare and science-related careers—careers that ultimately require mastery of such content (Koch, 2017; Hensley et al., 2021; Muteti et al., 2021). Therefore, students may recognize the value of learning about anatomy and physiology (Sullins et al., 1995), positively impacting the forethought phase. Yet students often cannot identify (i.e., forethought), deploy (i.e., performance), or evaluate (i.e., self-reflection) strategies that they do not know, and thus, SRL is constrained by the repertoire of study strategies and progress monitoring approaches that learners possess (Zimmerman and Schunk, 1989; Bernacki et al., 2020). This is particularly critical for introductory biology students, as Sebesta and Bray Speth (2017) found that they not only have limited knowledge of SRL strategies, but they may also be unable to properly implement them. Their findings also revealed a link between SRL strategy use and achievement that was previously found in other science content areas (see Lopez et al., 2013).
Not all study strategies are equally effective. Bjork and Bjork (2011) argue that strategies that induce desirable difficulties yield greater cognitive understanding and better enable encoding and retrieval processes. The desirable difficulties framework asserts that employing more effortful and active strategies (e.g., interleaving, spaced studying, using quizzes, or practice tests to study material) cultivates longer and deeper comprehension (Bjork and Bjork, 2011). Walck-Shannon et al. (2021) leveraged the desirable difficulties framework to examine the relationship between study strategies and performance on exams for introductory biology students. They found that students who used a greater number of active study strategies (e.g., explaining concepts, self-quizzing, and drawing diagrams) scored higher than students who used fewer active strategies or passive strategies (i.e., read textbooks, rewrote notes, and watched lectures). Each additional active strategy that students used was associated with an increase of about 2–3% on the respective exams. Further, Kritzinger et al. (2018) found that ability and willingness to persist through challenges were more evident in higher-performing students and can be predictive of student success, underscoring the impact of prior performance.
The use of interventions to support introductory biology students is not new. Minchella et al. (2002) investigated the impact of a semester-long, one-credit, biology seminar designed to help first-year students transition to college and increase their academic success. Academic advisors and a team of undergraduate teaching interns assisted first-year students through problem-solving sessions (e.g., class time devoted to modeling and teaching problem-solving strategies for the concurrent biology lab), as well as discussions and lectures, with an emphasis on fostering collaborative peer support. Throughout the semester, students developed time management systems, learned strategies to help them succeed in biology, and had time to visit research laboratories. Overall, these activities helped students build realistic expectations of a career in the field of biology. The seminar course resulted in positive outcomes including increased grades, student satisfaction, and retention in the department.
More recently, Bernacki et al. (2020) implemented a 2-h, self-guided, online training course embedded within a biology seminar. The goal of this study was to examine if a “Learning to Learn” course could change undergraduate biology students’ study habits and improve their coursework performance. The intervention contained three modules designed to teach and model the effectiveness of different learning strategies by providing opportunities for students to read and practice not only using the strategies but adapting them to their needs. The modules ended with identifying resources provided within the biology seminar’s learning management system (LMS) to help future learning. The modules had a statistically significant impact on student behavior (e.g., students utilized more self-assessment, planning, and self-monitoring resources than students that did not participate in the modules, as measured by monitoring the LMS traffic) and academic performance (e.g., students scored higher on exam scores than those that did not participate).
Interventions have the potential to yield increased learning outcomes for undergraduate students in biology-related courses and beyond. However, many of these approaches require a significant financial and time investment (e.g., training of instructors, days or hours required for students to complete the module). Comparatively fewer approaches have emphasized more sustainable implementation (e.g., brief, online, and low resource). One notable recent exception centered on a single, brief (e.g., 15 min) instructor-created presentation and discussion that focused on three high-impact strategies (Vemu et al., 2022). In their intervention, Vemu et al. (2022) encouraged students to engage in high-impact, effective study strategies (i.e., spacing, self-testing, and drawings or models) at the beginning of the semester. While there was no statistically significant growth in students’ use of key strategies from the beginning to the end of the semester, students that reported using spacing and drawing strategies by the end of the semester had higher grades.
Our study advances extant research by exploring the extent to which a study strategy intervention that is not only brief (i.e., 15-min) but also instructor-independent (e.g., not requiring additional instructor/course time) can yield a positive impact on student learning outcomes for students in an introductory anatomy and physiology course.
The present study
We employed a one-group, repeated measures design, such that all students engaged in the brief, online module between exam 2 and exam 3. This design allowed us to look at students’ strategy use over time, as well as the extent to which strategy use was linked to exam score. Further, it enabled us to look at whether that potential relationship was moderated by students’ prior academic performance, such that students with different levels of prior academic performance (e.g., comparatively higher or lower GPA) have a stronger or weaker relationship between the changes in their strategy use and exam score differences.
RQ1: Do students evidence greater use of effective study strategies after participating in a brief, online module, as evidenced by descriptions of their strategy use and ratings on cognitive and metacognitive strategies subscales?
RQ2: (a) Are changes in students’ use of effective study strategies associated with changes in exam score and (b) is this relationship moderated by self-reported GPA, as evidenced by descriptions of their strategy use and ratings on cognitive and metacognitive strategies subscales?
Materials and methods
Participants, context, and design
Undergraduate students were recruited from three, large sections of an introductory human anatomy and physiology course taught in the spring semester by two instructors at a large public, Hispanic-serving University in the southwestern United States. The course covered aspects related to the structure and function of the human body, including cells and tissues as well as the integumentary, skeletal, muscular, and nervous systems. All three sections of the course were taught predominantly via traditional lecture with an associated lab component. Participating students (n = 98) made up about 16% of the total number of initially enrolled students across the three sections (i.e., between 140 and 240 students per section, not accounting for those who withdrew from the course).
Participants (women, n = 74; men, n = 22; nonbinary, n = 1; did not respond, n = 1) were mostly (85.6%) between 18 and 21 years old (M = 19.95, SD = 2.16). Students identified as White1 (55.0%), Hispanic (28.6%), Asian (14.3%), Black (9.2%), American Indian (3.1%), Pacific Islander (2.0%), or elected not to report their race (2.0%). Over half were students in their first year of college (57.1%) with the remaining participants in their second (35.7%) or third (7.2%) year. Almost all of the participants (90%) expressed that they were taking the course at least partly because it was a required course for their major, but a substantial portion also noted that they were interested in learning the course content (39%) or that it would help them with their future career (61%).
Human subjects approval was obtained prior to conducting the study (#STUDY00008599), and all participants consented to participate in the research before beginning the first survey. APA ethical standards were followed throughout the duration of the research. Students were offered 2% extra credit in their course as compensation for completing the study. All but one participant granted permission to include exam grades as part of our data, thus that individual was excluded from analyses that involved exam grades.
We intentionally employed a one-group, pretest-posttest design that invited all students enrolled in the class to engage in the module midway through the semester. This timing allowed for a more stable measure of students’ typical strategy use at pretest, having already experienced one exam before reporting strategy use on the second exam (see also Bernacki et al., 2020). Sebesta and Bray Speth (2017) referred to this as the “settling in” (p. 9) of strategy use occurring after the second exam. Further, given the emphasis on a brief intervention, we were particularly interested in examining the impact on students’ study strategy use immediately after the module (i.e., the exam that followed several weeks later), where it would most likely be detected, before examining the potential for delayed impact (i.e., the final exam).
Materials
Brief, online module
The brief, online module focuses on six study strategies that have been largely established in the literature as effective but not commonly discussed in classrooms (Pomerance et al., 2016) or used by students (Dunlosky et al., 2013; Weinstein et al., 2023a): spaced practice (Benjamin and Tullis, 2010), retrieval practice (Roediger et al., 2011), elaboration (McDaniel and Donnelly, 1996), interleaving (Rohrer, 2012), concrete examples (Rawson et al., 2014), and dual coding (Mayer and Anderson, 1992). In alignment with our theoretical framing, Dunlosky et al. (2013) identified these strategies among those that can help students improve their comprehension and application of concepts, allowing individuals to better engage in SRL (e.g., use more effective strategies in the performance phase; Zimmerman, 2000). Likewise, Bjork and Bjork (2011) noted several of these as active study strategies that elicit desirable difficulties. Further, using these strategies in combination can help solidify the study process, given their complementary nature. For example, spaced practice focuses on spreading out study sessions, whereas dual coding and concrete examples emphasize how one can effectively study during those spaced study sessions (Weinstein et al., 2023a). Similarly, retrieval practice can not only help improve the ability to recall information, but also when spaced out over time, it can aid transfer of knowledge to new contexts (Butler, 2010).
All students participated in a brief (i.e., approximately 15 min), two-part module where they (a) learned about the six study strategies and (b) reflected on how they could use two of the strategies in their human anatomy and physiology class. First, students watched a video (8.5 min; Memorize Academy [Username] in collaboration with the Learning Scientists, 2016) that overviewed all six strategies. The video was produced in collaboration with The Learning Scientists,2 cognitive psychologists that study the science of learning, and addressed both how to use each strategy as well as an overview of research that supports their benefits on learning. Students were unable to proceed to the next page of the survey until the duration of the video had elapsed. Then, students ranked the strategies based on what they were most interested in learning about in more depth. For their two highest interest strategies, students spent 3–5 min reviewing the associated infographic (Weinstein et al., 2023b) and writing a detailed plan for how they could use that strategy to study for their human anatomy and physiology class (Figure 1). While students’ detailed plans were not evaluated as part of the data, the authors verified that students responded to the planning prompt.
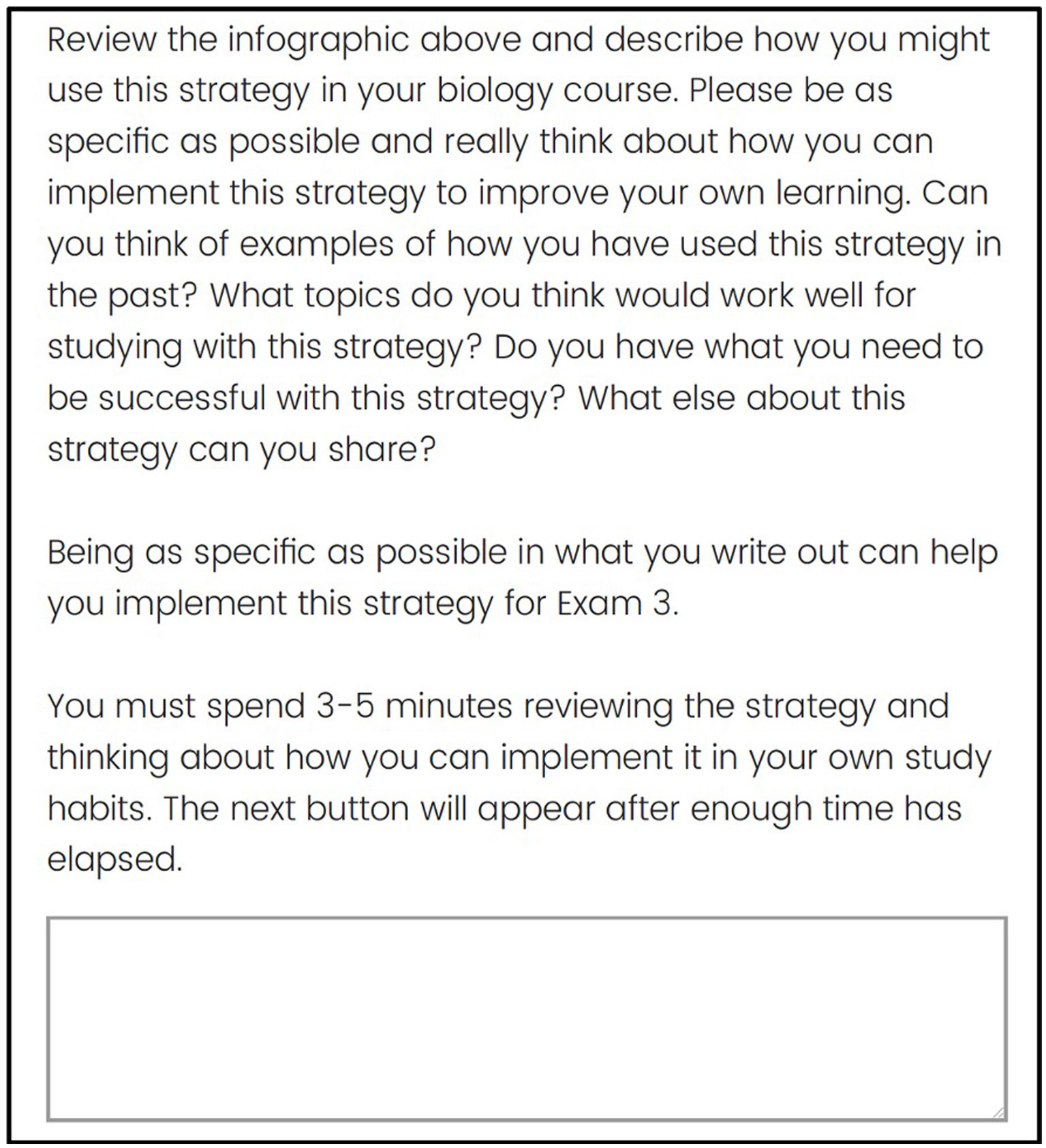
Figure 1. Strategy implementation prompt example from module. Infographics referred to in the prompt were produced by Weinstein et al., 2023b and are available at: https://www.learningscientists.org/downloadable-materials.
Quantity of effective study strategies used
After both exam 2 and exam 3, students responded to a series of open-ended questions (e.g., “please describe all of the strategies you used to study in as much detail as possible”) asking them to describe how they studied for the exam they just took (Figure 2). The responses were coded based on whether students described using each of the six different study strategies across their responses (i.e., used = 1, not = 0). A quantity score was also calculated for each student based on the total number of effective study strategies they described using for exam 2 (i.e., before the module) and for exam 3 (i.e., after the module). Scores could range from 0 (i.e., no effective strategies) to 6 (i.e., all effective strategies). For example, one student described their studying by noting, “I used quizlet to memorize terms, flash cards to test myself[,] and I drew myself pictures of types of tissues, bones, and diagrams[,] such as [a] hair follicle[,] we needed to know to help myself study and understand the structures.” This response represents a score of 2, as the student described using both retrieval practice (i.e., quizlet and/or flash cards) and dual coding (i.e., drawing pictures and/or diagrams). All responses were coded by the third author and 20% of the responses were then checked by the first author for fidelity to the scoring rubric and interrater consistency. Interrater agreement was checked separately for the identification of each strategy within a student’s response. This process allowed us to ensure that agreement was sufficient for each strategy independently [i.e., ICC (2), absolute agreement, single measure >0.698], as well as for overall quantity score [i.e., the total sum of all effective strategies used; ICC (2), consistency, single measure = 0.888]. We also calculated a strategy use change score (i.e., the quantity of strategies students described using at exam 3 minus the quantity of strategies students described using at exam 2) to gauge the extent to which students’ use of effective study strategies changed over time.
Cognitive and metacognitive strategies subscales
Students completed the Motivated Strategies for Learning Questionnaire (Pintrich et al., 1991), which included the cognitive and metacognitive strategies (CAMS) subscales, after both exam 2 and exam 3. Participants responded to each of the statements (e.g., “When reading for this course, I make up questions to help focus my reading.”) on a 7 (i.e., very true of me) to 1 (i.e., not at all true of me) Likert-type scale, and scores for each subscale were calculated averaging across all items associated with the respective subscale. Given the focus of the module, we report only data pertaining to four of the CAMS subscales (elaboration, αpre = 0.665; αpost = 0.702; organization, αpre = 0.385; αpost = 0.567; critical thinking, αpre = 0.659; αpost = 0.500; and metacognitive self-regulation, αpre = 0.733; αpost = 0.834). Notably, Cronbach alpha values for three of the subscales (i.e., elaboration, organization, and critical thinking) were right at or below the threshold of α > 0.7, potentially due to the low number of items combined with the somewhat modest sample size. For each subscale, correlations between the two administrations (i.e., at exam 2 and at exam 3) were all statistically significant and positive (all rs > 0.415), providing additional evidence of test–retest reliability of the subscale scores.
Demographic information and self-reported GPA
At the end of the second survey, participants completed a brief demographic questionnaire (e.g., age, gender, race, and enrollment), and participants were asked to self-report their college GPA. Additionally, several questions also focused on participants’ motivations for taking the course (e.g., their plans after graduation, whether the course was required for their program or major).
Exam scores
Four exams were administered in the course, roughly 4 weeks apart. Each exam was worth 40 points and together they contributed to 50% of students’ total course grade. The content assessed in each exam was independent and non-cumulative, that is, exams targeted only the content learned over the preceding 4 weeks. Difficulty was not equated between the four exams. Both instructors reported overall average scores for the four exams, indicating a progressive increase in difficulty over time (i.e., each exam had a lower average percentage than the preceding one). Specifically, the drop from exam 2 to exam 3 was 2% for one instructor and 3% for the other. Instructors noted that students typically could draw more from prior knowledge based on content learned earlier in the course (e.g., prior college chemistry course or advanced biology course in high school) than later on in the semester. Scores for the first, second, and third course exams were obtained from course instructors for students that consented to allow grades to be used as part of the research (i.e., all but one). To address RQ2, we also calculated an exam change score (i.e., exam 3 minus exam 2). This allowed us to look specifically at the extent to which changes in strategy use were associated with increased or decreased performance on the exam.
Procedures
Students were invited to participate in the research immediately after receiving their grades for exam 2. After consenting to participate in the research, students completed the pretest survey (see Figure 3), which included (a) their descriptions of how they studied for the exam, (b) the CAMS subscales, and (c) the brief, online module. Two weeks later, an email went out to all students who completed the pretest survey that included a reminder link to the video with a note prompting them to use the study strategies while preparing for exam 3. Students were emailed the link for the posttest survey immediately after the grades for exam 3 were posted. The second survey included the same measures as the first (i.e., a and b above), and it also included a series of demographic and motivation questions, including a self-report of their current GPA.
Statistical analysis
Given the ordinal nature of the quantity of effective study strategies described using (i.e., 0–6), we used Related-Samples Wilcoxon Signed Rank test to examine RQ1 and the changes in study use from exam 2 to exam 3. Prior to analyzing the data, we examined the distribution of the differences, which revealed a symmetrically shaped distribution, thus meeting the requisite assumption for interpreting the results of this test. In contrast, we used paired-samples t-tests to determine whether the mean differences on the four CAMS subscales from exam 2 to exam 3 were statistically significant, given the continuous nature of the subscale scores. After examining the boxplots for each respective subscale analysis, outliers that were more than 1.5 box-lengths from the edge of the box were removed, ranging from no outliers on the organization subscale to five outliers on the elaboration subscale. The assumption of normality was not violated for any of the subscales (i.e., Shapiro–Wilk’s test, all ps > 0.186). For RQ2, we employed PROCESS v4.1 macro of Hayes (2021) in SPSS to gauge whether a change in effective strategies (i.e., measured by the quantity of strategies participants described using and the four CAMS subscales) was associated with a change in exam performance (i.e., a higher grade on exam 3 than exam 2) and whether the strength of that relationship was moderated by students’ self-reported GPA.
Results
Descriptive statistics
Students, on average, evidenced modest strategy use for both exam 2 and exam 3, as evidenced by the quantity of study strategies reported and CAMS study strategy subscale scores (Table 1). As mentioned previously, exam 3 had a higher difficulty for students than exam 2 (i.e., the section averages for exam 3 were between 2 and 3% lower than on exam 2 scores). However, participating students only scored about two points (i.e., 0.5% of the 40-point exam) lower on exam 3 than on exam 2. GPA was overall notably high; only five students reported a GPA below 3.0, while 25 reported a GPA at or above 4.00 (i.e., grades of A+ are weighted at 4.33). We address issues related to the overall high GPA scores and the decision to collect GPA via self-report in greater detail in the discussion.
Frequency counts for each of the six study strategies are noted in Table 2. Prior to the brief, online module, retrieval practice was noted most frequently as the strategy students described using. After the module, there was a very small increase (i.e., between 3 and 8) in the number of students who reported using each of the strategies, except for dual coding. Notably, students selected spaced practice and retrieval practice as the ones they were most interested in learning about—they were selected almost twice as frequently as the other strategies—these were also strategies among those that students most often reported using prior to watching the video (i.e., they described using them for exam 2).
There was a statistically significant, positive correlation between the quantity of study strategies students used for exam 2 and exam 2 scores (r = 0.273, p = 0.007). Of note, there was no statistically significant correlation between the quantity strategies used for exam 2 and the other exam scores (i.e., exam 3, r = 0.142, p = 0.169; exam 1, r = 0.167, p = 0.105). This pattern, however, did not hold for the quantity of study strategies students used for exam 3. There was no significant correlation with any of the exams, including exam 3, r = 118, p = 0.255, although the correlation between the quantity of study strategies used at exam 3 was higher for exam 3 than it was for exam 1, r = 0.017, p = 0.870, or exam 2, r = −0.012, p = 0.906. There were no significant correlations between any of the CAMS subscales at exam 2 and any of the exams, and for exam 3, only the metacognitive self-regulation subscale had a statistically significant positive correlation with the associated exam. These correlations suggest a limited pattern whereby students’ use of effective study strategies was associated with higher exam scores (see Table 3).
Changes in study strategy use
To gather a more comprehensive understanding regarding changes in students’ study strategy use over time, we analyzed RQ1 by looking at two different indicators of strategy use. First, we examined changes in the quantity of effective study strategies based on students’ descriptions. Of the 98 participating students, 41 described using a greater number of effective study strategies in preparation for exam 3 than they did in preparation for exam 2 (i.e., after engaging with the module), 37 described using the same number, and only 20 described using fewer effective study strategies. Altogether, students evidenced a statistically significant median increase in the number of strategies from exam 2 to exam 3, z = 2.75, p = 0.006. Notably, however, the median number of strategies students used was the same (Mdn = 1) both before and after the module.
Additionally, we also looked at changes in students’ responses to the associated CAMS subscales. For both the critical thinking, t(95) = 2.02, p = 0.046, Cohen’s d = 0.207, and metacognitive self-regulation, t(94) = 2.16, p = 0.033, Cohen’s d = 0.223, subscales, there was a statistically significant mean increase over time in line with a small effect. No differences were detected for either the elaboration or organization subscales (both ps > 0.196, Cohen’s d < 0.135).
Impact of study strategy change
When looking at the impact of study strategy change, the results revealed that the overall model (i.e., change in study strategies that students described using predicting change in exam score and accounting for GPA) was statistically significant, F(3,87) = 2.92, p = 0.0384, R2 = 0.09. As predicted, the change in the number of strategies used was associated with a statistically significant change in exam score, b = 1.34, t(87) = 2.58, p = 0.012, revealing that every additional strategy used was associated with an increase of 1.34 exam points (i.e., out of 40 points total) for those scoring at the grand mean of GPA. GPA did not directly predict exam score change, b = 0.83, t(87) = 0.61, p = 0.544, and there was no interaction between changes in strategy use and GPA, b = 1.96, t(87) = 1.23, p = 0.114. As such, the association between strategy use and scores on the exam was consistent across students, irrespective of their GPA.
Additionally, we examined changes in students’ study strategy use as evidenced by their scores on the four CAMS subscales. However, the results revealed that none of the overall models were statistically significant [e.g., metacognitive self-regulation, F(3,87) = 1.44, p = 0.237, R2 = 0.05; critical thinking, F(3,87) = 1.59, p =. 197, R2 = 0.05].
Discussion
Drawing from extant interventions and grounded in the literature of SRL (Zimmerman, 2000, 2002) and desirable difficulties (Bjork and Bjork, 2011), the present study centered around examining an innovation for students taking an introductory human anatomy and physiology course to potentially increase their effective strategy use. Our approach offered a unique contribution in that it was designed for sustainable use (i.e., took only 15 min of students’ time to complete, was completed outside of class time and online via a link, required no extra materials or costs for students, and did not involve any instructor time).
We employed two different indicators of students’ strategy use. First, by systematically coding students’ descriptions of their studying, we were able to measure the degree to which students used effective strategies in a way that was sensitive to the six specific strategies embedded in the module. Second, by using the CAMS subscales of the Motivated Strategies for Learning Questionnaire, we also gathered complementary measures of strategy use via a well-established measurement tool (Pintrich et al., 1991; Kritzinger et al., 2018).
Ultimately, we found modest, statistically significant increases on some indicators of students’ strategy use. On average, students described using more effective study strategies, as well as greater critical thinking and metacognitive self-regulation, but there were no differences detected for two of the subscales (i.e., elaboration and organization). Additionally, there was limited evidence about the association between changes in strategy use and changes in exam scores, and GPA did not moderate this relationship.
Need for briefer, sustainable innovations
Numerous interventions have been designed to successfully support undergraduate students’ self-regulated learning and study strategy use (Hattie et al., 1996; Minchella et al., 2002; Roediger and Butler, 2011; Hartwig and Dunlosky, 2012; Cook et al., 2013; Zhao et al., 2014; Broadbent and Poon, 2015; Blasiman et al., 2017; Sebesta and Bray Speth, 2017; Bernacki et al., 2020; Hensley et al., 2021; Muteti et al., 2021; Theobald, 2021; Vemu et al., 2022). Each is comprised of a unique composition of features that make up how it is implemented and enacted, specifying both modality (e.g., online vs. face-to-face) and intensity (e.g., long vs. short). These features necessarily impact both the possibility for scalable implementation (e.g., the ability for other instructors to implement the intervention), as well as the likelihood for generalizability of results (e.g., whether the benefits to students are believed to apply in other contexts).
While longer interventions have shown greater efficacy (Dignath and Büettner, 2008), there are inherent challenges that come with them. Undergraduate students are faced with increasing competing demands for their time (e.g., balancing school and work) that may preclude their participation in longer-duration interventions. Shorter interventions can still be effective. The digital skills intervention designed by Bernacki et al. (2020), for example, required only 1–2 h of students’ time and yielded increased grades on course quizzes and exams, although the intervention designed by Vemu et al. (2022), which only took 15-min to complete, did not yield a statistically significant increase in strategy use. Combined with the findings of the present study, it is unclear the extent to which an intervention as brief as 15 min can yield meaningful change, despite the potential value of such brief interventions.
Measures of strategy use
In the present study, we aimed to gather students’ strategy use via complementary measures of strategy use (i.e., descriptions of their studying and CAMS subscales). Results for the two measures differed in that increases were evidenced on the former, more proximal, measure of the specific strategies described in the module, as well as some of the more distal measures (i.e., two of the four subscales). Specifically, while the descriptions of students’ study strategies were coded based on the strategies discussed in the module; the CAMS subscales were less directly aligned. For example, none of the targeted strategies explicitly targeted critical thinking, while the elaboration strategy directly aligned with the elaboration subscale. Likewise, metacognitive self-regulation, which involved planning, monitoring, and regulating, loosely aligned with multiple strategies (e.g., retrieval practice and spaced practice) and the overarching aim of the video. Moreover, strategy use is not all or nothing (Sebesta and Bray Speth, 2017). Multiple factors contribute to the effectiveness of strategy use and the impact on learning (e.g., how long, often, or correctly a strategy was used). Walck-Shannon et al. (2021) accounted for this by looking at not only the number of strategies students used when studying for the exam, but also the proportion of time they studied using each of the active strategies. However, even in that approach, it was unclear how deeply or correctly each strategy was used (e.g., superficially or in ways that align with best practices). Consequently, our measure of students’ self-reported strategy use was limited in the fact that we were not able to gauge the extent to which they used the respective strategy (e.g., just once or frequently) or how effectively they used it (e.g., in line with best practices or not).
In contrast with other interventions, our results revealed no differences for two of the CAMS subscales (i.e., elaboration and organization). Sebesta and Bray Speth (2017), for example, found that after their intervention, organizational strategies increased (i.e., keeping records, goal setting and planning, and reviewing graded work). Kritzinger et al. (2018) studied differences between students identified as at-risk, higher performing, as well as those in the “murky middle” (p. 2). They found differences between how students in each of these groups studied. For example, higher performing students were more likely to use metacognitive self-regulation and elaboration. This is particularly notable given that certain strategies can evoke desirable difficulties and may be challenging for students to utilize without additional support (Kritzinger et al., 2018; Walck-Shannon et al., 2021). Indeed, students may struggle or resist adopting new study strategies without being explicitly taught how to utilize them in their own context (Vemu et al., 2022).
Supporting all students’ learning
An increase in strategy use ultimately only matters if concomitant changes are evidenced with regard to students’ learning and performance outcomes. One of the key contributions of this study was that there was, in effect, a simple effect of changes in students’ described strategy use on changes in exam score, in line with similar findings of both Walck-Shannon et al. (2021) and Bernacki et al. (2020).
Yet, students do not all enter college with the same level of preparation (Ley and Young, 1998), and students with lower GPAs may rely on more rehearsal-based study strategies than their peers with higher GPAs (Marbach-Ad et al., 2016). In contrast, students with higher GPAs may not recognize the need for using certain strategies or admit they used or need them (Kritzinger et al., 2018). A meta-analytic review by Credé and Phillips (2011) found some subscales to correlate with GPA (e.g., metacognitive self-regulation) but not others (e.g., elaboration or organization). Given this conflicting past research, we investigated the role of GPA, but we did not find any evidence that GPA moderated the strength of the relationship between changes in strategy use and exam score—students with higher GPAs did not have a stronger or weaker relationship than those with lower GPAs.
Limitations and areas for further exploration
Similar to Hensley et al. (2021), we also focused on growth from pretest to posttest, which allowed us to take into consideration students’ extant strategy use and prior knowledge as well as the fact that exam 3 was more difficult than exam 2. While we intentionally provided all students interested in participating in the research with the brief, online module, to potentially support their learning, we recognize that without a control group, we cannot definitively attribute these changes to the module or make claims of causal inferences (see also Vemu et al., 2022). Of note, however, the sample of students who participated in the study did evidence less of a decrease in scores from exam 2 to exam 3 (i.e., 0.5%) compared to the overall average decrease in each section (i.e., between 2 and 3%), although such difference might be a result of selection bias. While it was not within the scope of the present study, we hope to see future research continue investigating the benefits of brief innovations to support students’ study strategy use using experimental designs. Of note, there was nothing related to study techniques covered in the standard course instruction, and we have no knowledge of other interventions available to students in the class that would serve as an alternative explanation for the increase in strategies used, specific to those covered in the brief, online module.
Future research should also continue to explore the role of GPA. For feasibility reasons, it was not possible to obtain official student GPAs for this study, as such we gathered GPA via self-report. Discrepancies exist between self-reported GPA and official GPA. Indeed, Kuncel et al. (2005) found in their meta-analysis that, overall, self-reported GPA had increased error and decreased reliability, as individuals tend to have positive bias in their reporting, resulting in restricted range. However, after examining the moderation effects of various individual difference variables, the authors of the meta-analysis also forwarded an “ideal situation” where “more faith can be placed” on results derived from self-reported GPA (i.e., “self-reported grades from college students who have done well in school and have high cognitive ability scores,” p. 76). We argue that while collecting GPA via self-report is less than ideal, our sample (i.e., high-achieving college students) is among those that have a more viable justification for use. While self-reported GPA may be artificially inflated, the moderately high overall nature of the sample suggests that the college students were overall high achieving. Taken together, there is a need to further investigate the impact of the module directly, using a comparison or control condition with a sample of students who have a wider range of GPA.
We hope to see continued research in this area to develop and evaluate more intensive interventions with reasonable time commitments and costs for both students and instructors. Using the present study as a case in point, students described using more effective strategies, although most still only used one or two of the strategies they learned about. This may have been related to the design of the module (i.e., students only personalized a plan for using their top two strategies) or the fact that most students selected to personalize a plan for strategies that were already commonly employed (e.g., retrieval practice). Thus, one future direction could be to extend the intervention by providing repeated (i.e., spaced) exposures to the video and allowing students to focus on different strategies each time. This would give students an opportunity to expand their repertoire of strategies and allow them to reflect on prior attempts and implement novel strategies. Alternatively, future research could explore other novel approaches to strategy interventions, for example, strategies that promote the use of collaborative or interactive strategies (e.g., forming study groups to promote small-group learning; Springer et al., 1999).
Finally, given the complexity of measuring strategy use, combined with the modest and somewhat mixed results of the present study, future research should continue to investigate ways to assess and gauge students’ strategy use with these measures and others. Of note, one limitation of the present study is that we did not account for potential family-wise error (e.g., Bonferroni correction) in our analyses of the five CAMS subscales, which would be overly conservative in this context (e.g., sample size, number of subscales, and power). Interpretation of effect sizes, which align with a small effect for both critical thinking and metacognitive self-regulation, however, can serve as additional evidence that these results are less likely a result of Type 1 error. Additionally, the measures we employed did not assess the quality with which students used the various strategies (e.g., did they use them effectively) or the extent to which they used them (e.g., just once or in every study session). Future research should explore complementary measures that can better gauge the complexity of students’ study strategy use.
Introductory human anatomy and physiology courses can serve as a “gateway” into health sciences careers, and it is a critical opportunity to examine interventions that can support students in this specific area. Supporting undergraduate biology students earlier in their academic pathways could positively impact the trajectory of their success in the field (Kritzinger et al., 2018). Even with a greater level of subjective value and motivation to succeed (Sullins et al., 1995), they may lack knowledge about SRL strategies and how to implement them (Sebesta and Bray Speth, 2017), limiting their learning and ultimately their success. Continued exploration is needed to recognize the challenges and constraints that students are navigating in order to identify and delineate brief, low-cost interventions that promote enhanced strategy use, learning, and academic performance for students in introductory human anatomy and physiology courses.
Data availability statement
The raw data supporting the conclusions of this article will be made available by the authors, without undue reservation.
Ethics statement
The studies involving human participants were reviewed and approved by Arizona State University’s Institutional Review Board. The patients/participants provided their written informed consent to participate in this study.
Author contributions
CF led the conception, design, and analysis of the study as well as manuscript writing. ES, AM, and LY contributed to the coding, analysis, and writing-up portions of the manuscript. All authors contributed to the article and approved the submitted version.
Funding
This research project was supported by internal research grants awarded by both Mary Lou Fulton Teachers College and the Institute for Social Sciences Research at Arizona State University (ASU) as well as the ASU Open Access Publication Fund.
Conflict of interest
The authors declare that the research was conducted in the absence of any commercial or financial relationships that could be construed as a potential conflict of interest.
Publisher’s note
All claims expressed in this article are solely those of the authors and do not necessarily represent those of their affiliated organizations, or those of the publisher, the editors and the reviewers. Any product that may be evaluated in this article, or claim that may be made by its manufacturer, is not guaranteed or endorsed by the publisher.
Footnotes
1. ^Total does not equal 100%, as students were permitted to select multiple race identifiers.
References
Adesope, O. O., Trevisan, D. A., and Sundararajan, N. (2017). Rethinking the use of tests: a meta-analysis of practice testing. Rev. Educ. Res. 87, 659–701. doi: 10.3102/0034654316689306
Ames, C., and Archer, J. (1988). Achievement goals in the classroom: Students' learning strategies and motivation processes. J. Educ. Psychol. 80, 260–267. doi: 10.1037/0022-0663.80.3.260
Bandura, A. (1986). Social Foundations of Thought and Action: A Social Cognitive Theory. Englewood Cliffs, NJ: Prentice-Hall.
Benjamin, A. S., and Tullis, J. (2010). What makes distributed practice effective? Cogn. Psychol. 61, 228–247. doi: 10.1016/j.cogpsych.2010.05.004
Bernacki, M. L., Vosicka, L., and Utz, J. C. (2020). Can a brief, digital skill training intervention help undergraduates “learn to learn” and improve their STEM achievement? J. Educ. Psychol. 112, 765–781. doi: 10.1037/edu0000405
Bjork, E. L., and Bjork, R. A. (2011). “Making things hard on yourself, but in a good way: creating desirable difficulties to enhance learning” in Psychology and the Real World: Essays Illustrating Fundamental Contributions to Society. eds. M. A. Gernsbacher, R. W. Pew, L. M. Hough, and J. R. Pomerantz (New York, NY: Worth Publishers), 56–64.
Blasiman, R. N., Dunlosky, J., and Rawson, K. A. (2017). The what, how much, and when of study strategies: comparing intended versus actual study behaviour. Memory 25, 784–792. doi: 10.1080/09658211.2016.1221974
Broadbent, J., and Poon, W. L. (2015). Self-regulated learning strategies & academic achievement in online higher education learning environments: a systematic review. Internet High. Educ. 27, 1–13. doi: 10.1016/j.iheduc.2015.04.007
Butler, A. C. (2010). Repeated testing produces superior transfer of learning relative to repeated studying. J. Exp. Psychol. Learn. Mem. Cogn. 36, 1118–1133. doi: 10.1037/a0019902
Cook, E., Kennedy, E., and McGuire, S. Y. (2013). Effect of teaching metacognitive learning strategies on performance in general chemistry courses. J. Chem. Educ. 90, 961–967. doi: 10.1021/ed300686h
Credé, M., and Phillips, L. A. (2011). A meta-analytic review of the motivated strategies for learning questionnaire. Learn. Individ. Differ. 21, 337–346. doi: 10.1016/j.lindif.2011.03.002
Dignath, C., and Büettner, G. (2008). Components of fostering self-regulated learning among students: a meta-analysis on intervention studies at primary and secondary school level. Metacogn. Learn. 3, 231–264. doi: 10.1007/s11409-008-9029-x
Dunlosky, J., Rawson, K. A., Marsh, E. J., Nathan, M. J., and Willingham, D. T. (2013). Improving students’ learning with effective learning techniques: promising directions from cognitive and educational psychology. Psychol. Sci. Public Interest 14, 4–58. doi: 10.1177/1529100612453266
Eccles, J. S. (1983). “Expectancies, values, and academic behaviors” in Achievement and Achievement Motives. ed. J. T. Spence (San Francisco: Freeman), 75–146.
Hartwig, M. K., and Dunlosky, J. (2012). Study strategies of college students: are self-testing and scheduling related to achievement? Psychon. Bull. Rev. 19, 126–134. doi: 10.3758/s13423-011-0181-y
Hattie, J., Biggs, J., and Purdie, N. (1996). Effects of learning skills interventions on student learning: a meta-analysis. Rev. Educ. Res. 66, 99–136. doi: 10.3102/00346543066002099
Hayes, A. F. (2021). The PROCESS macro for SPSS and SAS (version 4.1). Available at: http://processmacro.org/index.html
Hensley, L., Kulesza, A., Peri, J., Brady, A. C., Wolters, C. A., Sovic, D., et al. (2021). Supporting undergraduate biology students’ academic success: comparing two workshop interventions. CBE Life Sci. Educ. 20:ar60. doi: 10.1187/cbe.21-03-0068
Karpicke, J. D., Butler, A. C., and Roediger, H. L. (2009). Metacognitive strategies in student learning: do students practise retrieval when they study on their own? Memory 17, 471–479. doi: 10.1080/09658210802647009
Kiewra, K. A. (2002). How classroom teachers can help students learn and teach them how to learn. Theory Pract. 41, 71–80. doi: 10.1207/s15430421tip4102_3
Koch, A. K. (2017). “It’s about the gateway courses: defining and contextualizing the issue” in Improving Teaching, Learning, Equity, and Success in Gateway Courses. New Directions for Higher Education. ed. A. K. Koch, vol. 180 (Hoboken, New Jersey: Jossey-Bass), 11–17.
Kornell, N., and Bjork, R. A. (2007). The promise and perils of self-regulated study. Psychon. Bull. Rev. 14, 219–224. doi: 10.3758/BF03194055
Kritzinger, A., Lemmens, J.-C., and Potgieter, M. (2018). Learning strategies for first-year biology: toward moving the murky middle. CBE Life Sci. Educ. 17, 1–13. doi: 10.1187/cbe.17-10-0211
Kuncel, N. R., Credé, M., and Thomas, L. L. (2005). The validity of self-reported grade point averages, class ranks, and test scores: a meta-analysis and review of the literature. Rev. Educ. Res. 75, 63–82. doi: 10.3102/00346543075001063
Ley, K., and Young, D. B. (1998). Self-regulation behaviors in underprepared (developmental) and regular admission college students. Contemp. Educ. Psychol. 23, 42–64. doi: 10.1006/ceps.1997.0956
Lopez, E. J., Nandagopal, K., Shavelson, R. J., Szu, E., and Penn, J. (2013). Self-regulated learning study strategies and academic performance in undergraduate organic chemistry: an investigation examining ethnically diverse students. J. Res. Sci. Teach. 50, 660–676. doi: 10.1002/tea.21095
Marbach-Ad, G., Rietschel, C., and Thompson, K. V. (2016). Validation and application of the survey of teaching beliefs and practices for undergraduates (STEP-U): identifying factors associated with valuing important workplace skills among biology students. CBE Life Sci. Educ. 15:ar59. doi: 10.1187/cbe.16-05-0164
Mayer, R. E., and Anderson, R. B. (1992). The instructive animation: helping students build connections between words and pictures in multimedia learning. J. Educ. Psychol. 84, 444–452. doi: 10.1037/0022-0663.84.4.444
McDaniel, M. A., and Donnelly, C. M. (1996). Learning with analogy and elaborative interrogation. J. Educ. Psychol. 88, 508–519. doi: 10.1037/0022-0663.88.3.508
Memorize Academy [Username] in collaboration with the Learning Scientists (2016). How to study effectively for school or college [top 6 science-based study skills] [video]. Youtube. Available at: https://www.youtube.com/watch?v=CPxSzxylRCI
Minchella, D. J., Yazvac, C. W., Fodrea, R. A., and Ball, G. (2002). Biology resource seminar: first aid for the first year. Am. Biol. Teach. 64, 352–357. doi: 10.2307/4451310
Muteti, C. Z., Zarraga, C., Jacob, B. I., Mwarumba, T. M., Nkhata, D. B., Mwavita, M., et al. (2021). I realized what I was doing was not working: the influence of explicit teaching of metacognition on students’ study strategies in a general chemistry I course. Chem. Educ. Res. Pract. 22, 122–135. doi: 10.1039/d0rp00217h
Pintrich, P. R., Smith, D. A. F., Garcia, T., and McKeachie, W. J. (1991). A manual for the use of the motivated strategies for learning questionnaire (MSLQ), The University of Michigan, Ann Arbor.
Pomerance, L., Greenberg, J., and Walsh, K. (2016). Learning about learning: what every new teacher needs to know. National Council on Teacher Quality. Available at: https://www.nctq.org/publications/Learning-About-Learning:-What-Every-New-Teacher-Needs-to-Know
Rawson, K. A., Thomas, R. C., and Jacoby, L. L. (2014). The power of examples: illustrative examples enhance conceptual learning of declarative concepts. Educ. Psychol. Rev. 27, 483–504. doi: 10.1007/s10648-014-9273-3
Roediger, H. L., and Butler, A. C. (2011). The critical role of retrieval practice in long-term retention. Trends Cogn. Sci. 15, 20–27. doi: 10.1016/j.tics.2010.09.003
Roediger, H. L., Putnam, A. L., and Smith, M. A. (2011). Ten benefits of testing and their applications to educational practice. Psychol. Learn. Motiv. 55, 1–36. doi: 10.1016/B978-0-12-387691-1.00001-6
Rohrer, D. (2012). Interleaving helps students distinguish among similar concepts. Educ. Psychol. Rev. 24, 355–367. doi: 10.1007/s10648-012-9201-3
Schneider, M., and Preckel, F. (2017). Variables associated with achievement in higher education: a systematic review of meta-analyses. Psychol. Bull. 143, 565–600. doi: 10.1037/bul0000098
Sebesta, A. J., and Bray Speth, E. (2017). How should I study for the exam? Self-regulated learning strategies and achievement in introductory biology. CBE Life Sci. Educ. 16:ar30. doi: 10.1187/cbe.16-09-0269
Springer, L., Stanne, M. E., and Donovan, S. S. (1999). Effects of small-group learning on undergraduates in science, mathematics, engineering, and technology: a meta-analysis. Rev. Educ. Res. 69, 21–51. doi: 10.3102/00346543069001021
Sullins, E. S., Hernandez, D., Fuller, C., and Tashiro, J. S. (1995). Predicting who will major in a science discipline: expectancy–value theory as part of an ecological model for studying academic communities. J. Res. Sci. Teach. 32, 99–119. doi: 10.1002/tea.3660320109
Theobald, M. (2021). Self-regulated learning training programs enhance university students’ academic performance, self-regulated learning strategies, and motivation: a meta-analysis. Contemp. Educ. Psychol. 66:101976. doi: 10.1016/j.cedpsych.2021.101976
Vemu, S., Denaro, K., Sato, B. K., Fisher, M. R., and Williams, A. E. (2022). Moving the needle: evidence of an effective study strategy intervention in a community college biology course. CBE Life Sci. Educ. 21:ar24. doi: 10.1187/cbe.21-08-0216
Walck-Shannon, E. M., Rowell, S. F., and Frey, R. F. (2021). To what extent do study habits relate to performance? CBE Life Sci. Educ. 20:ar6. doi: 10.1187/cbe.20-05-0091
Weinstein, Y., McDermott, K. B., and Roediger, H. L. (2010). A comparison of study strategies for passages: rereading, answering questions, and generating questions. J. Exp. Psychol. Appl. 16, 308–316. doi: 10.1037/a0020992
Weinstein, Y., Smith, M., and Caviglioli, O. (2023a) Our answers to frequently asked questions. Available at: https://www.learningscientists.org/faq
Weinstein, Y., Smith, M., and Caviglioli, O. (2023b) Six strategies for effective learning: materials for teachers and students. Available at: https://www.learningscientists.org/downloadable-materials
Wingate, U. (2007). A framework for transition: supporting ‘learning to learn’ in higher education. High. Educ. Q. 61, 391–405. doi: 10.1111/j.1468-2273.2007.00361.x
Zhao, N., Wardeska, J. G., McGuire, S. Y., and Cook, E. (2014). Metacognition: an effective tool to promote success in college science learning. J. Coll. Sci. Teach. 43, 48–54. doi: 10.2505/4/jcst14_043_04_48
Zimmerman, B. J. (2000). “Attaining self-regulation: a social cognitive perspective” in Handbook of Self-Regulation. eds. M. Boekarts, P. R. Pintrich, and M. Zeidner (San Diego, CA: Academic Press), 13–39.
Zimmerman, B. J. (2002). Becoming a self-regulated learner: an overview. Theory Pract. 41, 64–70. doi: 10.1207/s15430421tip4102_2
Keywords: self-regulated learning, study strategies, online intervention, undergraduate students, learning
Citation: Firetto CM, Starrett E, Montalbano AC, Yan L, Penkrot TA, Kingsbury JS and Hyatt J-PK (2023) The impact of effective study strategy use in an introductory anatomy and physiology class. Front. Educ. 8:1161772. doi: 10.3389/feduc.2023.1161772
Edited by:
Wang-Kin Chiu, The Hong Kong Polytechnic University, ChinaReviewed by:
Joseph R. Boyle, Temple University, United StatesKit W. Cho, University of Houston–Downtown, United States
Copyright © 2023 Firetto, Starrett, Montalbano, Yan, Penkrot, Kingsbury and Hyatt. This is an open-access article distributed under the terms of the Creative Commons Attribution License (CC BY). The use, distribution or reproduction in other forums is permitted, provided the original author(s) and the copyright owner(s) are credited and that the original publication in this journal is cited, in accordance with accepted academic practice. No use, distribution or reproduction is permitted which does not comply with these terms.
*Correspondence: Carla M. Firetto, Y2ZpcmV0dG9AYXN1LmVkdQ==