- 1Department of SGP AI, Singapore University of Technology and Design, Singapore, Singapore
- 2Pillar of Information Systems Technology and Design, Singapore University of Technology and Design, Singapore, Singapore
- 3Pillar of Engineering Product Development, Singapore University of Technology and Design, Singapore, Singapore
In recent years, eye-tracking (ET) methods have gained an increasing interest in STEM education research. When applied to engineering education, ET is particularly relevant for understanding some aspects of student behavior, especially student competency, and its assessment. However, from the instructor’s perspective, little is known about how ET can be used to provide new insights into, and ease the process of, instructor assessment. Traditionally, engineering education is assessed through time-consuming and labor-extensive screening of their materials and learning outcomes. With regard to this, and coupled with, for instance, the subjective open-ended dimensions of engineering design, assessing competency has shown some limitations. To address such issues, alternative technologies such as artificial intelligence (AI), which has the potential to massively predict and repeat instructors’ tasks with higher accuracy, have been suggested. To date, little is known about the effects of combining AI and ET (AIET) techniques to gain new insights into the instructor’s perspective. We conducted a Review of engineering education over the last decade (2013–2022) to study the latest research focusing on this combination to improve engineering assessment. The Review was conducted in four databases (Web of Science, IEEE Xplore, EBSCOhost, and Google Scholar) and included specific terms associated with the topic of AIET in engineering education. The research identified two types of AIET applications that mostly focus on student learning: (1) eye-tracking devices that rely on AI to enhance the gaze-tracking process (improvement of technology), and (2) the use of AI to analyze, predict, and assess eye-tracking analytics (application of technology). We ended the Review by discussing future perspectives and potential contributions to the assessment of engineering learning.
1. Introduction
Eye tracking has been integrated into many applications, such as human-computer interaction, marketing, medicine, and engineering (e.g., assistive driving, software, and user interfaces). Recent studies revealed that eye tracking (ET) and artificial intelligence (AI), including machine (ML) and deep learning (DL), have been combined to assess human behavior (e.g., Tien et al., 2014). However, although extensive studies have focused on the application of AI techniques to eye-tracking data in some STEM disciplines, little is known about how this could be used in engineering design educational settings to facilitate instructors’ assessment of the design learning of their students, especially design competency.
1.1. Competency assessment in engineering education
1.1.1. Competency-based engineering education
The development of student competencies has become a central issue in complex fields, such as engineering education. With regard to competencies, various terminologies are used to describe a learner expertise in a situation and their ability to solve complex engineering problems; for instance, competence (pl. competences), competency (pl. competencies), capability, and so on, are generally used. The debate about terminology is still ongoing. In this paper, we refer to both “competence,” i.e., the general term, and “competency,” i.e., the components of a competence as holistic constructs, with the focus on “competency” as the ability to integrate knowledge, skills, and attitudes (KSAs; Le Deist and Winterton, 2005) and their underlying constituents (cognitive, conative, affective, motivational, volitional, social, etc.; e.g., Shavelson, 2013; Blömeke et al., 2015) simultaneously (van Merriënboer and Kirschner, 2017). From an instructional design perspective, learning, which is also the acquisition of skills and competencies, has integrative goals in which KSAs are developed concurrently to acquire complex skills and professional competencies (Frerejean et al., 2019). This approach is interesting and may help avoid core issues in instructional engineering design, such as compartmentalization, which involves the teaching of KSAs separately, hindering competency acquisition and transfer in complex engineering learning. Therefore, as suggested by Spencer (1997), competency assessment (we discuss this further in the next section) determines the extent to which a learner has competencies. Competency is assumed to be multidimensional (Blömeke et al., 2015) and discipline-specific (Zlatkin-Troitschanskaia and Pant, 2016). Competencies can be learned through training and practice. Siddique et al. (2012) noted two levels of competencies in professional fields: (1) field-specific task competencies, and (2) meta-competencies as generalized skill sets. Le Deist and Winterton (2005) argued that a multi-dimensional framework of “competence” necessarily involved conceptual (cognitive and meta-competence) and operational (functional and social competence, including attitude and behavior) competencies. They assumed competence is composed of four dimensions of competencies: cognitive dimension (knowledge), functional dimension (skills), social (behavior and attitudes), and meta-competence (Le Deist and Winterton, 2005). Engineers argue that these dimensions also apply to engineering education. With the emerging complexity involved in designing engineering systems, tackling complexity is a new requirement. As such, Hadgraft and Kolmos (2020) proposed that three basic competencies should be incorporated into engineering courses: complexity, system thinking, and interdisciplinarity. Therefore, we argue that competency and competency assessment should be described by a more holistic framework that is appropriate to learning and instruction in complex engineering education.
1.1.2. Challenges of assessing student competencies in engineering education
Instructors’ assessment of students’ engineering competencies is a critical topic that has been addressed for decades in the engineering education literature. Despite this, assessment of engineering learning suffers from several issues, such as a lack of consistency. It is still highly subjective, labor-intensive, and time-consuming. The COVID-19 pandemic has exacerbated these issues as many engineering instructions shifted from face-to-face to online or remote instructions using online platforms, thus increasing teacher workloads, cognitive loads, etc. More critically, engineering assessment suffers from an integrative approach to engineering competencies and competency assessment even with the use of advanced techniques, such as AI and other computing technologies (e.g., Khan et al., 2023). Most technologies used to assess engineering student competencies usually focus on some aspects of an engineering competence and not on a systemic holistic approach to competency.
1.2. Eye-tracking technologies: a brief history in scientific research
Several papers have reviewed the history of eye-tracking research (e.g., Wade and Tatler, 2005; Płużyczka, 2018). Płużyczka (2018) identified three developmental phases in the first 100 years of eye tracking as a research approach: the first phase of eye-tracking research dates back to the late 1870s with Javal’s studies on understanding and assessing the reading process. At that time, the eye-tracking approach was optical-mechanical and invasive. The second era of eye-tracking research originated with film recordings in the 1920s. The third phase started in the mid-1970s and refers to two main phenomena related to the development of psychology (the establishment of a theoretical and methodological basis for cognitive psychology) and technology (the use of computer, television, and electronic techniques to detect and locate the eye). Motivated by the rapid development of eye-tracking and computer processing technologies, Płużyczka (2018) also suggested that another phase led to contemporary eye-tracking research that took place in the 1990s.
Eye tracking permits the assessment of an individual’s visual attention, yielding a rich source of information on where, when, how long, and in which sequence certain information in space or about space is looked at (Kiefer et al., 2017). Different eye-tracking techniques have been referenced. For instance, Duchowski and Duchowski (2007) identified four categories of eye movement measurement methodologies: electro-oculography (EOG), scleral contact lens/search coil, photo-oculography (POG) or video-oculography (VOG), and video-based combined pupil and corneal reflection (p. 51). Li et al. (2021) also provided a similar overview. Among other techniques, they cited the earliest manual observations followed by new techniques, such as electrooculography, video and photographic, corneal reflection, and micro-electromechanical systems, and those based on machine and deep learning. For each method, they examined the benefits and limitations. They argued that CNN-based approaches offer better recognition performance and robustness; however, they require large amounts of data, complex parameter adjustments, and an understanding of black box characteristics, and involve high costs.
1.3. AI and ET to assess engineering
Artificial intelligence and computer vision (CV) have advanced significantly and rapidly over the past decade due to highly effective deep learning models, such as the CNN variants (Szegedy et al., 2015; He et al., 2016; Huang et al., 2017) and vision transformers (Dosovitskiy et al., 2020), and the availability of large high-quality datasets and powerful GPUs for training such large models. As a result of these advances in AI and CV, eye-tracking technology has reached a level of reliability sufficient for wider adoption, such as for evaluating student attention via their eye-gaze on the study materials taught. More specifically, this application has the benefit of being able to measure multiple spectrums of student attention. For example, such technology can measure whether the student is focusing more generally on the class or specifically on certain parts of the lecture material. Adding on the dimension of time, one can also measure the amount of time students spend on different parts of the course content and when their attention starts to drift.
In terms of the instructor-side, the integration of eye tracking and AI has various benefits for the assessment of engineering design education. Similar to the application of eye tracking and AI with students, these technologies can generally be used to measure which part of a student assignment an instructor focuses more on and the amount of time they spend on different parts of an assignment. In addition, we see the following potential cases for the use of eye tracking and AI:
• Studying the effectiveness of assessment criterions. Alongside a marking rubric, eye tracking and AI can be used to find a correlation between different assessment criteria and specific parts of a submitted assignment. For example, we can compare the criteria in a marking rubric that an instructor is looking at and the corresponding parts of an assignment they look at next. Pairs of these marking criteria and assignment segments can then be used for correlation studies.
• Streamlining instructor assessment workload. With explainable AI (XAI) techniques, a system can highlight portions of the student assignments that an instructor should focus on based on the different criteria. Such a model can be trained on past data of instructor assessment and student assignments, alongside the captured eye-tracking data. This model can then be transferred and fine-tuned to other assignments.
• Detecting discrepancies between instructor assessments. Different instructors may have varying standards or interpretations of engineering assessments, e.g., between newer and more experience instructors. Eye tracking and AI can be used to determine whether there are any differences between instructors in terms of the parts of the student assignments they focus on, how much time they spend on each portion, and, most importantly, any significant differences in the assigned grades for each criteria.
2. Purpose and research questions
This study aims to understand research trends in the use of AIET to assess engineering student competencies. The overall research questions (RQs) are as follows:
• RQ1: What are the current research trends (or categories) in AI- and ET-based competency assessment in engineering education over the last decade?
• RQ2: What are the most salient competency dimensions and labels to which we attribute studies related to assessing engineering education?
3. Methods
3.1. Data collection
We reviewed the literature and collected papers from the following four databases: Web of Science (WoS), IEEE Xplore (IX), Academic Search Complete (ASC), and Computers and Applied Sciences Complete (CASC) hosted by EBSCO and Google Scholar (GS). The Review was conducted with research published in the last decade, i.e., from 2013 to 2022. Focusing on title, abstracts, and keywords, we used a general equation including terms used in the topic of eye tracking and artificial intelligence in engineering education research, such as Title-Abs-Key[(“eye-track*” OR “eye-gaze” OR “eye movement”) AND (“artificial intelligence” OR “machine learning” OR “deep learning”) AND (“assess* OR evaluat* OR measur* OR test* OR screen*) AND (“competenc*” OR “skills” OR “knowledge” OR “attitudes”) AND (“engineering design” OR “engineering education”)]. The review process, which comprised three steps, namely identification, screening, and eligibility, is summarized below and in the flow diagram (Figure 1):
1. Identification: an initial record of N = 89 studies were identified by searching the databases: EBSCOhost (19 studies), WoS (24 studies), IX (26 studies), and GS (20 studies).
2. Screening: after duplicates were removed, records were screened based on the relevance of titles and abstracts.
3. Eligibility: peer-reviewed studies written in English and related to engineering education, competency assessment, and higher education were selected.
4. Finally, N = 76 studies were retained in this Review.
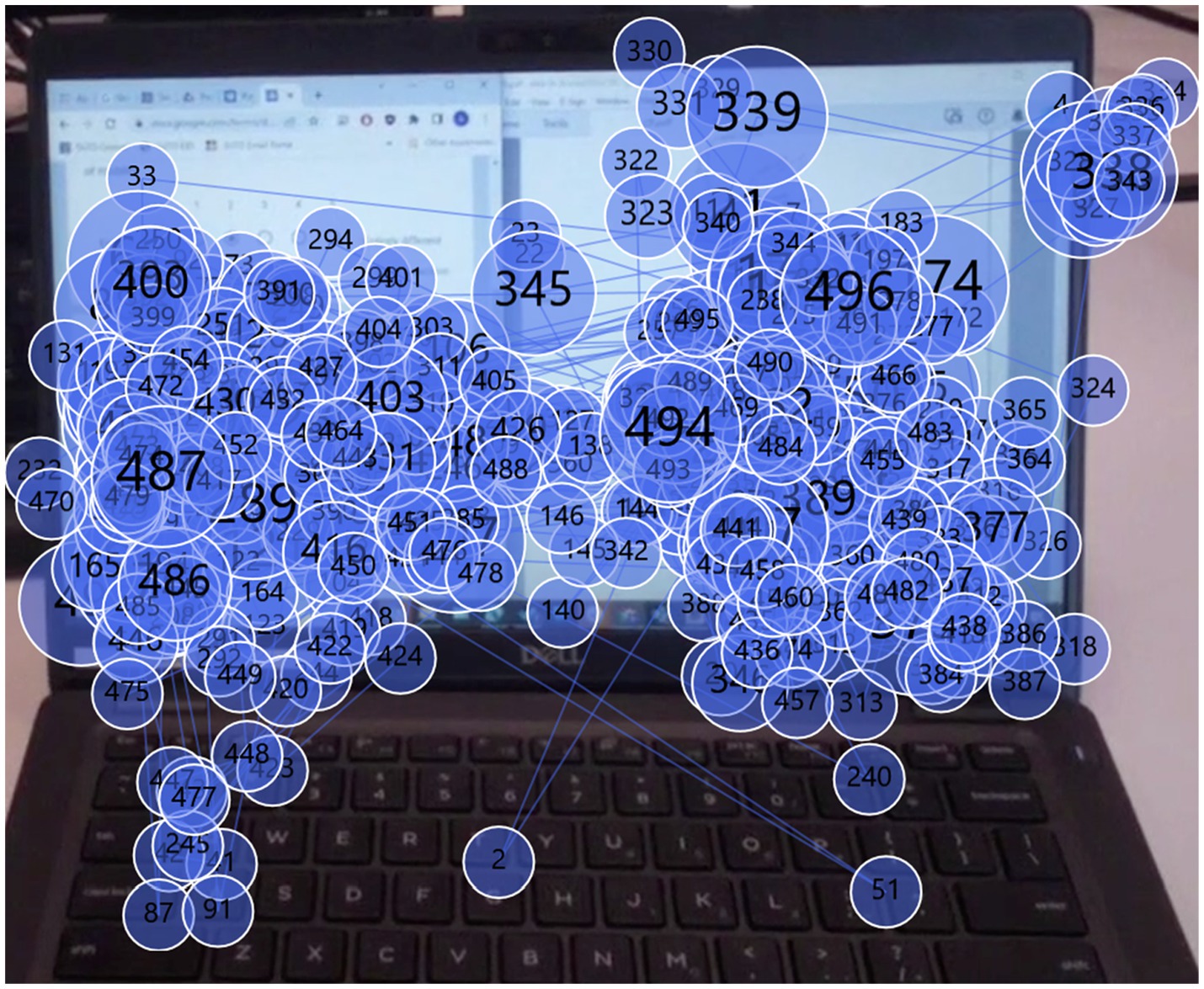
Figure 1. Instructor on-screen assessment of student design creativity. This pilot experiment (one person involved) used Tobii glasses 2 (issues related to the use of this specific tool are not discussed here). The instructor followed a rubric (left side of the screen) comprising a set of criteria to assess creativity in students’ design solutions (right side of the computer screen). The results of this study are not reported in this paper.
All references collected from the databases were imported into Rayyan, an intelligent platform for systematic review, to help in the review process. Data were then manually categorized (according to labels that fit in the dimensional aspects of a student’s competency as defined earlier) and exported in an editable format containing three variables: title, abstract, dimension, and corresponding labels. In addition, the generated format contained the following criteria: relevance to assessing EE (yes/no), higher education (yes/no), tested with instructors or students (yes/no), methodology used (type of assessment), competency dimensions (cognitive, functional, social, and meta), contributions, and limitations (Figure 2).
3.2. Data analysis
Qualitative and quantitative methods were used to analyze the data. Following the collection, we first performed a qualitative analysis (i.e., thematic analysis), manually categorizing and labeling the focus of each paper according to the competency dimensions. This helped to identify the types of AIET. Based on this corpus, we then furthered the Review with a lexicometry analysis with IRaMuTEQ 0.7 alpha 2 (Ratinaud, 2009) and RStudio 2021.09.1 + 372 for macOS. IRaMuTEQ is an R interface for multidimensional analysis of texts and questionnaires. It offers different types of analysis, such as lexicometry, statistical methods (specificity calculation, factor analysis, or classification), textual data visualization (usually called word cloud), or term network analysis (called similarity analysis).
We conducted a clustering based on the Reinert’s method (Reinert, 1990). This method includes a hierarchical classification, profiles, and correspondence analyses. To obtain the co-occurrence graphs, we then conducted a similarities analysis that used the graph theory also called network analysis to analyze trends within the reported data. Finally, we also used thematic analysis to organize the reported data into categories for the assessment types, titles of clusters, and types of AIET.
4. Results
4.1. Categories of artificial intelligence and eye tracking
Our first research question attempts to explore current research trends in AIET-based assessment in engineering education. Typically, and based on the manual thematic analysis, two relatively dependent types of AIET research categories can be identified with regard to assessment: (1) eye-tracking devices that use AI and sub-domains to improve the process of tracking (improving the technology), and (2) the use of AI to analyze and predict the eye-tracking data analytics related to student learning (the application of the technology). The first typology generally consists of combining AI and sub-domains, such as machine learning (ML) and deep learning (DL) with ET. As opposed to traditional tracking approaches that often estimate the location of visual cues, researchers developing this orientation attempt to improve the tracking process; for instance, favoring detection over tracking. Reported results from this approach detail the performance and accuracy of detection. This is receiving increasing attention. Conversely, although the second typology also utilizes AI to predict and detect behaviors, it mainly focuses on assessing and providing insights into learner behaviors afterwards based on recorded eye-tracking data. Collected data can be reinjected into the learning system afterwards to support the learners and/or educators.
Usually there are more practical applications to educational assessment. With regard to these typologies, a multimodal approach integrating ET and several signals, such as EEG (e.g., Wu et al., 2021), fNIRS (Shi et al., 2020), and skin conductance (e.g., Muldner and Burleson, 2015), is also referenced (Table 1).
4.2. Engineering competencies and dimensions
We view competency as the integration of student skills, knowledge, and attitudes and their underlying constituents simultaneously, hence highlighting different learning dimensions as defined earlier. There is no meaningful skill acquisition without suitable connections to these defined dimensions. Consequently, the competency acquisition is analyzed in terms of these dimensions, namely cognitive, functional, social, and meta. Among these dimensions, the assessment of the cognitive dimension of engineering student expertise seems to be the primary focus of AIET applications (cf. Table 2).
Moreover, our results showed an overview of learners’ mental state assessments, including cognitive, affective, and social levels of learners’ competencies. Although the visual and cognitive competency dimension was a particular focus, studies are lacking when it comes to students’ design expertise and its assessment by instructors. The reported studies examined the issues of addressing an aspect of student competency; however, they still lack focus on a holistic approach of competency assessment with competency being the integration of KSAs. Additionally, we manually analyzed references to highlight the types of assessment included in studies (see Table 3). The lexicometry analysis ran a hierarchical top-down classification that helped to identify four classes (or clusters) within the reported data (see Table 4). Class 1 (28.1% of the data), which we named “Eye-tracking method,” grouped terms related to gaze, achieve, feature, and eye, which were used to track and assess visual patterns. This class is correlated with Class 2 (13.9%) comprising the “AI functional approach” used to track and assess learners’ mental states and behaviors through the eye-tracking analytics. Such behaviors are described in Class 3 (22.1%), which we named “Mental state and behavioral assessment.” This class included terminology associated with the assessed aspect/behavior, such as cognitive, perceptual, awareness, stress, mental, and competency. Finally, we identify Class 4 (35.9%), which addressed “Instructional approach and student learning” as it included terms such as “student,” “learning,” and “team.”
In addition to this classification, we ran a correspondence analysis (CA) that showed the visual relationships of the identified clusters (cf. Figure 3). We analyzed the CA based on the first two factors, which were quite representative of the data samples (factor 1, 40.81%; factor 2, 32.19%). Results highlighted that Clusters 1 and 2 were well correlated, suggesting the relevance of the association of eye tracking and AI. However, these two clusters were in opposition, i.e., negatively correlated with Cluster 3 about mental states and behavior assessment on axis 2 (vertical), and with Cluster 4 about instructional approaches on axis 1. From these results, we identified the most well represented words of each cluster (see Table 4; “gaze” in cluster 1: χ2 = 33.78, p < 0.0001; “network” in cluster 2: χ2 = 258.56, p < 0.0001; “cognitive” in cluster 3: χ2 = 51.41, p < 0.0001; and “student” in cluster 4: χ2 = 88.44, p < 0.0001). This analysis confirmed the three clusters, namely the classes described above.
To obtain the co-occurrence graphs, we then performed a similarities analysis that used the graph theory also called network analysis to analyze trends in the literature. This analysis displayed the overall connection and grouping of terms used in the reported papers based on the co-occurrence scores of words (cf. Figures 4–6).
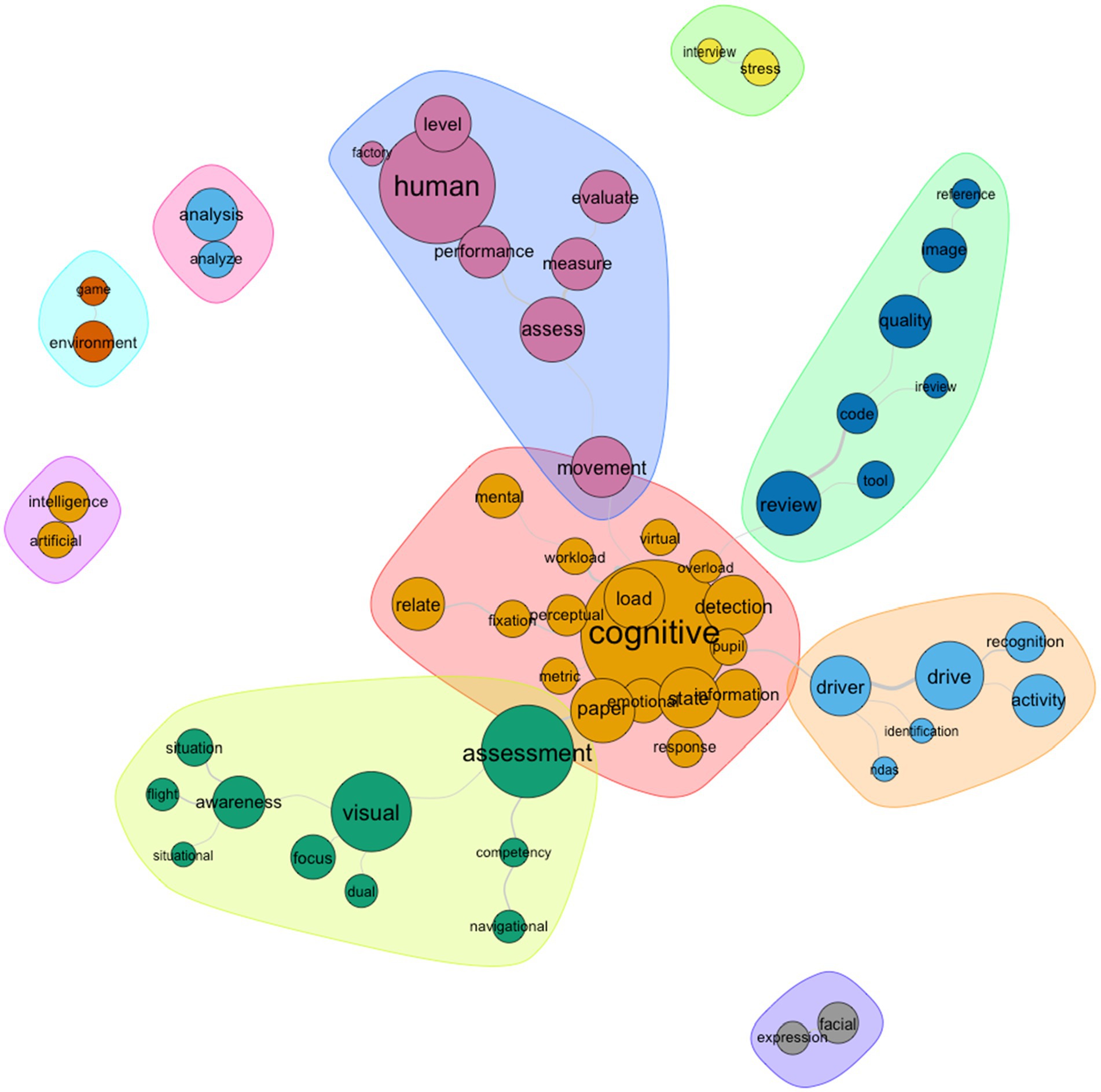
Figure 5. Cluster graph of “assessment” (χ2 = 16.17; p < 0.0001) and “assess” (χ2 = 15.96; p < 0.0001).
Whereas Figure 4 highlights grouping words from the reported literature (title, abstract, and keyword), Figure 6 shows the relationships between those three variables and our defined dimensions (cognitive, functional, social, and meta) and the labels defined in Table 2.
Regarding competency assessment in engineering education, we particularly focused on the feature “assess*” and analyzed (1) trends (Figure 7), (2) keyword-in-context (Table 5). With regard to research trends, Figure 7 (top), which depicts the absolute occurrence of the feature “assess*” across years, shows a clear trend of increasing interest in assessment with technologies, such as AI and ET, whereas Figure 7 (bottom) outlines the relative occurrence of “assess*” in comparison with all other features. Examples of citations in abstracts mentioning the purpose of analysis are provided in Table 4.
4.3. Application scenarios and model accuracy
Artificial intelligence and eye tracking has been applied in several engineering contexts with different focuses. A summary is provided in Table 6. We noticed applications in the classroom but also in lab practice, simulation training, and industry. However, despite the relevance, research is lacking on how models can help assess competency in a broader way involving the dimensions discussed earlier.
To understand the relevance of these AIET applications, we identified studies on the accuracy of developed models that integrate AI and ET to support engineering assessment broadly speaking, i.e., of and for/as learning with regard to the engineering literature (see Table 7). A relatively good average accuracy of 79.76% was found with an estimation range from 12% to 99.43%.
5. Discussion
This paper reviews the engineering literature to identify research focusing on AI and ET to support the assessment of competency in engineering education. Our study revealed that combining eye tracking and AI to assess engineering student competencies is receiving increasing attention. The association seems to be well supported, especially with the development of advanced technologies, such as AI. The Review highlights the main types of AIET, which are discussed below.
5.1. Two types of AIET in engineering competency assessment
Overall, two types of AIET focuses were reported: (1) an eye-tracking device that uses AI to improve the process of visual tracking itself, and (2) the use of AI to analyze, predict, and assess the eye tracking analytics.
5.1.1. AIET to improve the process of visual tracking
Most studies reported in this Review use this first approach to improve current eye-tracking technologies. For instance, in recent years, the prediction of eye movement scanpath can be divided into two categories: prediction models that hand-design features and powerful mathematical knowledge, and methods that intuitively obtain the sequence of eye fixes from the bottom-up salinity map and other useful indications (Han et al., 2021). With the advances of machine and deep learning, the study of computational eye-movement models has been mainly based on neural network learning models (e.g., Wang et al., 2021). For instance, in the context of robotic cars, Saha et al. (2018) proposed a CNN architecture that estimates the direction of vision from detected eyes and surpasses the latest results from the Eye-Chimera database. According to Rafee et al. (2022), previous eye-movement approaches focused on classifying eye movements into two categories: saccades and non-saccades. A limitation of these approaches is that they confuse fixations and smooth tracking by placing them in the non-saccadic category (Rafee et al., 2022). They proposed a low-cost optical motion analysis system with CCN technology and Kalman filters for estimating and analyzing the position of the eyes.
5.1.2. AIET to analyze and predict learners’ behaviors
With this approach, engineering assessments in the age of AI take a new shift and offer diverse possibilities (Swiecki et al., 2022), especially with the increase of online education platforms and environments (Peng et al., 2022). As such, research suggests that machine learning technologies can provide better detection than current state-of-the-art event detection algorithms and achieve manual encoding performance (Zemblys et al., 2018). When applied in engineering education, AIET-based approaches have the potential to provide automatic and non-intrusive assessment (Meza et al., 2017; Ahrens, 2020; Chen, 2021), higher accuracy (Hijazi et al., 2021), complex dynamic scenes such as video-based data (Guo et al., 2022), and a less consuming process. For instance, Hijazi et al. (2021) used iReview, an intelligent tool for evaluating code review quality using biometric measures gathered from code reviewers (often called biofeedback).
Costescu et al. (2019) combined GP3 Eye Tracker with OGAMA to identify learners at risk of developing attention problems. They were able to accurately assess visual attention skills, interpret data, and predict reading abilities. Ahrens (2020) tracked how software engineers navigate and interact with documents. By analyzing their areas of focus and gaze recordings, the author developed an algorithm to identify trace links between artifacts from these data. He finally concluded that eye tracking and interaction data are automatic and non-intrusive, allowing automatic recording without manual effort. This approach has interesting applications and perspectives for engineering design, namely the assessment of student visual parameters and algorithm replication for mass assessment, fairness and accuracy (objectivity, overload, and increased perception), understanding student learning behaviors, etc.
Moreover, as reported in our Review, AI and subsets for eye-tracking studies appear to be effective, as an average accuracy of approximately 80% was found for applications in engineering education, including in-class, VR, laboratory, and industrial settings.
5.2. Dimensions in engineering competency assessment of/for learning
With regard to our second research question, different dimensions of student learning have been analyzed. A somewhat unsurprising result was the prevalence of assessing student cognitive state, as eye tracking indeed relates to learners’ visual cues. As such, multiple studies can be found within the pertinent literature over the last few decades focusing on the assessment of cognitive states (e.g., Hayes et al., 2011) and visual cognition and perception (e.g., Gegenfurtner et al., 2013; Rayner et al., 2014). However, taken together and considering the sample size (N = 76 papers) reported over the last decade, this Review revealed that few studies in the field of engineering education have focused on AI- and eye-tracking-based assessment of student learning. Although the expected finding was that papers would essentially focus on the visual and cognitive aspects of student learning and competencies, this study also shows an interest in the literature that focuses on other components, such as functional (skills) and social (attitudes) aspects of student learning. Indeed, it is assumed that eye tracking is essentially used as a tool for examining cognitive processes (Beesley et al., 2019). However, references for the meta competency aspect are seriously lacking. Several reasons may explain this repartition. First, it is true that early studies in this area focused primarily on obtaining insights into learners’ visual patterns and therefore attempted to describe visual dynamics when learners look at the material in different environments and formats. Over the last decade, the focus has shifted to computational perspectives to visual attention modeling (e.g., Borji and Itti, 2012), driven by a digital transformation with the advances of attention computing, AI, machine learning, and cloud computing. Since 2013, and a bit later in 2016, as shown in Figure 7 (top), there has been a rapid rise of eye-tracking and AI-based assessments in research, especially when the field of AI becomes more accessible to cognitivists, psychologists, and engineering educational researchers. For instance, motivated by the complexity of contemporary visual materials and scenes, attention mechanism was associated with computer vision to imitate the human visual system (Guo et al., 2022). Moreover, this shift can be analyzed following the AI breakthroughs over the decade (2015: Russakovsky et al., 2015: OpenAI co-founded in 2015: deep learning models…). For instance, in January, 2023, the MIT Review published their 22nd 10 breakthrough technologies 2023 annual list (MIT Technology Review, 2023), recognizing key technological advances in many fields, such as AI. This list ranked “AI that makes images” in second position, justifying the growing interest visual computing has in contemporary research.
Shao et al. (2022) identified three waves of climax in AI advancements: in the early 60s, the second climax, and the third wave of AI, which according to LeCun et al. (2015) started with the era of deep learning, highly fostered developments and progress in society. As such, ImageNet was released in 2012, which in 2015 helped companies such as Microsoft and Google develop machines that could defeat humans in image recognition challenges. ImageNet was foundational to the advances of computer vison research (including recognition and visual computing).
We also reported the following different forms of assessments in engineering education: assessment of learning, i.e., as a summative evaluation (e.g., Bottos and Balasingam, 2020; Hijazi et al., 2021), formative assessment, i.e., assessment for learning, including feedback (e.g., Su et al., 2021; Tamim et al., 2021), self-assessment (Khosravi et al., 2022), and peer-assessment (Chen, 2021). In fact, engineering tasks are becoming increasingly complex. Therefore, current engineering instructions apply several assessments to better map student learning and their abilities, especially in active pedagogies such as project-based learning (PBL). This is reported by Ndiaye and Blessing (2023), who analyzed engineering instructors’ course review reports and highlighted several combinations of assessment (e.g., summative: 2D project, exam, review, and prototype evaluation; formative: quizzes, problem sets, and homework assignment; peer assessment: peer review…). Providing an effective competency assessment for learning, especially feedback, to all students in such complex fields is challenging and time-consuming. Therefore, as there is a strong association between AI and ET, researchers have been exploring alternative solutions within this synergy. As such, Su et al. (2021) used video to analyze student concentration. They proposed a non-intrusive computer vision system based on deep learning to monitor students’ concentration by extracting and inferring high-level visual signals of behavior, including facial expressions, gestures, and activities. A similar approach was used by Bottos and Balasingam (2020), who tracked reading progression using eye-gaze measurements and Hidden Markov models. With regard to team collaboration assessment, Guo and Barmaki (2020) used an automated tool based on gaze points and joint visual attention information from computer vision to assess team collaboration and cooperation.
5.3. Challenges of AIET
Despite the importance, AIET-based engineering assessment has some limitations. First, it suffers from a systematic and integrative approach of competency and competency assessment. Khan et al. (2023) reviewed the literature and identified a similar result for AI-based competency assessment in engineering design education. Indeed, competency, especially the measurement of student expertise, is viewed differently among researchers. There is ongoing debate about terminology within the literature (e.g., Le Deist and Winterton, 2005; Blömeke et al., 2015).
A second key challenge is the technique that is used to evaluate student learning. There are different eye-tracking methods and tools and they do not use the same tracking approach, hence not allowing tracking of the same behaviors. Consequently, further investigation is needed to achieve an appropriate network construction, followed by more efficient training to avoid common failures, such as over-training (e.g., Morozkin et al., 2017).
Other critical issues can be highlighted. AIET technologies are often too expensive and time-consuming (e.g., analysis of manual gaze data and data interpretation) to be implemented in classroom practice. Therefore, the development of low-cost approaches can be a better and more inclusive approach for engineering learning and instructions. Finally, the assessment of the student (behavior, mental state, etc.) often tends to replace the assessment of student learning (outcomes). It is not clear how studies clarify this difference.
6. Conclusion
This Review provides important insights into AI- and eye-tracking-based competency assessment in engineering education. With regard to our first research question (RQ1), this Review revealed that research trends have taken two orientations over the last decade. We showed that research generally discussed that (1) eye-tracking devices developed intrinsically with AI to enhance the gaze-tracking process (improvement of techniques), and/or (2) AI can be used to analyze, predict, and assess eye-tracking analytics (application domain). With regard to RQ2, i.e., the salient competency dimensions and labels attributed to assessing engineering education, the main finding is that visual cognitive aspects of learner competency are a primary focus. Hence, despite growing interest in advanced technologies, such as AI, attention computing, and eye-tracking, it is shown that student competency and underlying components are assessed in a fragmented way, i.e., not in a systematic and integrative approach to engineering competency and holistic assessment. Assessing engineering student expertise with AIET is essentially limited to visual aspects, and there is a lack of references and understanding about how it can be extended to more complex engineering learning. Therefore, we argue that such limitations can be situated in the technology itself, which relies on the eye (hence visual cognition and perception only) as a portal to an individual brain to understand human behavior. In addition, there is not yet a common understanding of expertise and competency. Terminologies vary depending on the subject domain.
This Review presents some limitations. Although the debate about competency or competence is still ongoing within the literature, we focus on engineering competency in terms of dimensions to analyze what is being effectively assessed. However, as preliminary research, an approach may need to be extended to other underlying engineering fields and explore different possible components in student competency acquisition. This needs to be better clarified with regard to existing frameworks. Additionally, as for every review, we only used well known terms; however, many terminologies are being used to describe eye-tracking techniques and studies (eye or gaze tracking, eye movements, visual tracking, etc.), including the variation in the syntax of the words (e.g., eye tracking or eye tracking or eye tracking) and competency (competence, ability, etc.). AI also suffers from this variation (e.g., machine learning, deep learning, NLP, etc.). Not all these terms were used, thus reducing the search.
This Review is probably one of the first to discuss trends in research on the assessment of engineering education with AIET technologies. Multiple relevant perspectives are possible. For engineering education, it is important to investigate in-depth how AIET can support complex learning and instruction. AIET may open new opportunities to better assess learning inclusively and efficiently, assuming that relevant assessment frameworks of the content to be assessed are well defined and situated. It is necessary to examine the combination of holistic approaches to assess complex engineering skills. As such, this Review may have several implications for the integration of AIET in engineering education. It may open new research perspectives on the.
AIET-based assessment of student learning, which will be worth investigating. This is a key area to be explored in-depth further.
Future research can focus on exploring multimodal approaches to better capture less-represented dimensions of engineering student competencies, helping to mitigate existing assessment shortcomings. One of the main issues is mapping student abilities and their engagement holistically during their learning with different assessments methods. Therefore, an increasing interest lies in associating different inclusive fine-grained techniques, such as electrical (EEG), physiological (heart-rate variability, galvanic skin resistance, and eye tracking), neurophysiological (fMRI) signals, and other traditional assessments (e.g., self-reported surveys, quizzes, peer-assessment, etc.), to improve assessment accuracy and efficiency. For instance, Wu et al. (2021) developed a deep-gradient neural network for the classification of multimodal signals (EEG and ET). Their model predicted emotions with 81.10% accuracy during an experiment with eight emotion event stimuli. Similar studies exploring learning and assessment are needed to gain holistic insights into student learning, instructions, and assessments.
Author contributions
YN and KL made a substantial, direct, and intellectual contribution to the work. LB reviewed and provided general comments on the work. All authors contributed to the article and approved the submitted version.
Funding
This research was funded by the Ministry of Education, Singapore (MOE2020-TRF-029).
Conflict of interest
The authors declare that the research was conducted in the absence of any commercial or financial relationships that could be construed as a potential conflict of interest.
Publisher’s note
All claims expressed in this article are solely those of the authors and do not necessarily represent those of their affiliated organizations, or those of the publisher, the editors and the reviewers. Any product that may be evaluated in this article, or claim that may be made by its manufacturer, is not guaranteed or endorsed by the publisher.
References
Ahrens, M. (2020). “Towards automatic capturing of traceability links by combining eye tracking and interaction data” In 2020 IEEE 28th International Requirements Engineering Conference (RE), Zurich, Switzerland. 434–439.
Amadori, P. V., Fischer, T., Wang, R., and Demiris, Y. (2021). Predicting secondary task performance: a directly actionable metric for cognitive overload detection. IEEE Transac. Cogn. Dev. Syst. 14, 1474–1485. doi: 10.1109/TCDS.2021.3114162
Amri, S., Ltifi, H., and Ayed, M. B. (2017). “Comprehensive evaluation method of visual analytics tools based on fuzzy theory and artificial neural network” In 2017 12th International Conference on Intelligent Systems and Knowledge Engineering (ISKE), 1–6. IEEE.
Aracena, C., Basterrech, S., Snáel, V., and Velásquez, J. (2015). “Neural networks for emotion recognition based on eye tracking data” In 2015 IEEE International Conference on Systems, Man, and Cybernetics, 2632–2637, IEEE.
Aunsri, N., and Rattarom, S. (2022). Novel eye-based features for head pose-free gaze estimation with web camera: new model and low-cost device. Ain Shams Eng. J. 13:101731. doi: 10.1016/j.asej.2022.101731
Bautista, L. G. C., and Naval, P. C. (2021) “CLRGaze: contrastive learning of representations for eye movement signals, 2021” In 29th European Signal Processing Conference (EUSIPCO), Dublin, Ireland. 1241–1245.
Beesley, T., Pearson, D., and Le Pelley, M. (2019). “Eye tracking as a tool for examining cognitive processes” in Biophysical measurement in experimental social science research. ed. G. Foster (Cambridge, MA: Academic Press), 1–30.
Bharadva, K. R. (2021). A machine learning framework to detect student’s online engagement. Doctoral dissertation. Dublin, National College of Ireland.
Blömeke, S., Gustafsson, J.-E., and Shavelson, R. J. (2015). Beyond dichotomies: competence viewed as a continuum. Z. Psychol. 223, 3–13. doi: 10.1027/2151-2604/a000194
Borji, A., and Itti, L. (2012). State-of-the-art in visual attention modeling. IEEE Trans. Pattern Anal. Mach. Intell. 35, 185–207. doi: 10.1109/TPAMI.2012.89
Bottos, S., and Balasingam, B. (2020). Tracking the progression of reading using eye-gaze point measurements and hidden markov models. IEEE Trans. Instrum. Meas. 69, 7857–7868. doi: 10.1109/TIM.2020.2983525
Bozkir, E., Geisler, D., and Kasneci, E. (2019). “Person independent, privacy preserving, and real time assessment of cognitive load using eye tracking in a virtual reality setup” In IEEE Conference on Virtual Reality and 3D User Interfaces (VR), Osaka, Japan. 2019, 1834–1837.
Chakraborty, P., Ahmed, S., Yousuf, M. A., Azad, A., Alyami, S. A., and Moni, M. A. (2021). A human-robot interaction system calculating visual focus of human’s attention level. IEEE Access 9, 93409–93421. doi: 10.1109/ACCESS.2021.3091642
Chen, X. (2021). TeamDNA: Automatic measures of effective teamwork processes from unconstrained team meeting recordings. Doctoral dissertation. Rice University.
Costescu, C., Rosan, A., Brigitta, N., Hathazi, A., Kovari, A., Katona, J., et al. (2019). “Assessing visual attention in children using GP3 eye tracker” In 10th IEEE International Conference on Cognitive Info Communications (CogInfoCom), Naples, Italy. 343–348.
Das, A., and Hasan, M. M. (2014). “Eye gaze behavior of virtual agent in gaming environment by using artificial intelligence” In IEEE International Conference on Electrical Information and Communication Technology (EICT), 1–7.
Dogan, K. M., Suzuki, H., and Gunpinar, E. (2018). Eye tracking for screening design parameters in adjective-based design of yacht hull. Ocean Eng. 166, 262–277. doi: 10.1016/j.oceaneng.2018.08.026
Dosovitskiy, A., Beyer, L., Kolesnikov, A., Weissenborn, D., Zhai, X., Unterthiner, T., et al. (2020). “An image is worth 16x16 words: transformers for image recognition at scale” In International Conference on Learning Representations.
Duchowski, A., and Duchowski, A. (2007). “Eye tracking techniques” in Eye tracking methodology: Theory and practice. ed. A. T. Duchowski (London: Springer), 51–59.
Farha, N. A., Al-Shargie, F., Tariq, U., and Al-Nashash, H. (2021). “Artifact removal of eye tracking data for the assessment of cognitive vigilance levels” In IEEE Sixth International Conference on Advances in Biomedical Engineering (ICABME), 175–179.
Frerejean, J., van Merriënboer, J. J., Kirschner, P. A., Roex, A., Aertgeerts, B., and Marcellis, M. (2019). Designing instruction for complex learning: 4C/ID in higher education. Eur. J. Dent. Educ. 54, 513–524. doi: 10.1111/ejed.12363
Gegenfurtner, A., Lehtinen, E., and Säljö, R. (2013). Expertise differences in the comprehension of visualizations: a meta-analysis of eye-tracking research in professional domains. Educ. Psychol. Rev. 23, 523–552. doi: 10.1007/s10648-011-9174-7
Gite, S., Pradhan, B., Alamri, A., and Kotecha, K. (2021). ADMT: advanced driver’s movement tracking system using spatio-temporal interest points and maneuver anticipation using deep neural networks. IEEE Access 9, 99312–99326. doi: 10.1109/ACCESS.2021.3096032
Guo, Z., and Barmaki, R. (2020). Deep neural networks for collaborative learning analytics: evaluating team collaborations using student gaze point prediction. Australas. J. Educ. Technol. 36, 53–71. doi: 10.14742/ajet.6436
Guo, M. H., Xu, T. X., Liu, J. J., Liu, Z. N., Jiang, P. T., Mu, T. J., et al. (2022). Attention mechanisms in computer vision: a survey. Computat. Visual Media 8, 331–368. doi: 10.1007/s41095-022-0271-y
Hadgraft, R. G., and Kolmos, A. (2020). Emerging learning environments in engineering education. Australas. J. Eng. Educ. 25, 3–16. doi: 10.1080/22054952.2020.1713522
Han, Y., Han, B., and Gao, X. (2021). Human scanpath estimation based on semantic segmentation guided by common eye fixation behaviors. Neurocomputing 453, 705–717. doi: 10.1016/j.neucom.2020.07.121
Hayes, T. R., Petrov, A. A., and Sederberg, P. B. (2011). A novel method for analyzing sequential eye movements reveals strategic influence on raven’s advanced progressive matrices. J. Vis. 11, 1–11. doi: 10.1167/11.10.10
He, K., Zhang, X., Ren, S., and Sun, J. (2016). “Deep residual learning for image recognition” In Proceedings of the IEEE conference on computer vision and pattern recognition. 770–778.
Hijazi, H., Cruz, J., Castelhano, J., Couceiro, R., Castelo-Branco, M., de Carvalho, P., et al. (2021). “iReview: an intelligent code review evaluation tool using biofeedback” In 2021 IEEE 32nd International Symposium on Software Reliability Engineering (ISSRE), Wuhan, China. 476–485.
Huang, G., Liu, Z., Van Der Maaten, L., and Weinberger, K. Q. (2017). “Densely connected convolutional networks” In Proceedings of the IEEE conference on computer vision and pattern recognition, 4700–4708.
Jiang, G., Chen, H., Wang, C., and Xue, P. (2022). Transformer network intelligent flight situation awareness assessment based on pilot visual gaze and operation behavior data. Int. J. Pattern Recognit. Artif. Intell. 36:2259015. doi: 10.1142/S0218001422590157
Khan, S., Blessing, L., and Ndiaye, Y. (2023). “Artificial intelligence for competency assessment in design education: a review of literature” In 9th International Conference on Research Into Design, Indian Institute of Science, Bangalore, India.
Khosravi, S., Khan, A. R., Zoha, A., and Ghannam, R. (2022) “Self-directed learning using eye-tracking: a comparison between wearable head-worn and webcam-based technologies” In IEEE Global Engineering Education Conference (EDUCON), Tunis, Tunisia, 640–643.
Kiefer, P., Giannopoulos, I., Raubal, M., and Duchowski, A. (2017). Eye tracking for spatial research: cognition, computation, challenges. Spat. Cogn. Comput. 17, 1–19. doi: 10.1080/13875868.2016.1254634
Le Deist, F. D., and Winterton, J. (2005). What is competence? Hum. Resour. Dev. Int. 8, 27–46. doi: 10.1080/1367886042000338227
LeCun, Y., Bengio, Y., and Hinton, G. (2015). Deep learning. Nature 521, 436–444. doi: 10.1038/nature14539
Li, Z., Guo, P., and Song, C. (2021). A review of main eye movement tracking methods. J. Phys. 1802:042066. doi: 10.1088/1742-6596/1802/4/042066
Li, X., Younes, R., Bairaktarova, D., and Guo, Q. (2020). Predicting spatial visualization problems’ difficulty level from eye-tracking data. Sensors 20:1949. doi: 10.3390/s20071949
Lili, L., Singh, V. S., Hon, S., Le, D., Tan, K., Zhang, D., et al. (2021). “AI-based behavioral competency assessment tool to enhance navigational safety” In 2021 International Conference on Electrical, Computer, Communications and Mechatronics Engineering (ICECCME). Mauritius. 2021, 1–6.
Lim, Y., Gardi, A., Pongsakornsathien, N., Sabatini, R., Ezer, N., and Kistan, T. (2019). Experimental characterisation of eye-tracking sensors for adaptive human-machine systems. Measurement 140, 151–160. doi: 10.1016/j.measurement.2019.03.032
Mehta, P., Malviya, M., McComb, C., Manogharan, G., and Berdanier, C. G. P. (2020). Mining design heuristics for additive manufacturing via eye-tracking methods and hidden markov modeling. ASME. J. Mech. Des. 142:124502. doi: 10.1115/1.4048410
Meza, M., Kosir, J., Strle, G., and Kosir, A. (2017). Towards automatic real-time estimation of observed learner’s attention using psychophysiological and affective signals: the touch-typing study case. IEEE Access 5, 27043–27060. doi: 10.1109/ACCESS.2017.2750758
MIT Technology Review (2023). 10 breakthrough technologies 2023, MIT technology review. Available at: https://www.technologyreview.com/2023/01/09/1066394/10-breakthrough-technologies-2023/#crispr-for-high-cholesterol (Accessed 19 August 2023).
Morozkin, P., Swynghedauw, M., and Trocan, M. (2017). “Neural network based eye tracking” in Computational collective intelligence. ICCCI 2017 Lecture Notes in Computer Science. eds. N. Nguyen, G. Papadopoulos, P. Jędrzejowicz, B. Trawiński, and G. Vossen (Cham: Springer), 10449.
Muldner, K., and Burleson, W. (2015). Utilizing sensor data to model students’ creativity in a digital environment. Comput. Hum. Behav. 42, 127–137. doi: 10.1016/j.chb.2013.10.060
Ndiaye, Y., and Blessing, L. (2023). Assessing performance in engineering design education from a multidisciplinary perspective: an analysis of instructors’ course review reports. Proc. Design Soc. 3, 667–676. doi: 10.1017/pds.2023.67
Peng, C., Zhou, X., and Liu, S. (2022). An introduction to artificial intelligence and machine learning for online education. Mobile Netw. Appl. 27, 1147–1150. doi: 10.1007/s11036-022-01953-3
Płużyczka, M. (2018). The first hundred years: a history of eye tracking as a research method. Appl. Linguist. Papers 4, 101–116. doi: 10.32612/uw.25449354.2018.4.pp.101-116
Pritalia, G. L., Wibirama, S., Adji, T. B., and Kusrohmaniah, S. (2020). “Classification of learning styles in multimedia learning using eye-tracking and machine learning” In 2020 FORTEI-International Conference on Electrical Engineering (FORTEI-ICEE), 145–150, IEEE.
Rafee, S., Xu, Y., Zhang, J. X., and Yemeni, Z. (2022). Eye-movement analysis and prediction using deep learning techniques and Kalman filter. Int. J. Adv. Comput. Sci. Appl. 13, 937–949. doi: 10.14569/IJACSA.2022.01304107
Ratinaud, P. (2009). IRAMUTEQ: Interface de R pour les analyses multidimensionnelles de textes et de questionnaires. Available at: http://www.iramuteq.org
Rayner, K., Loschky, L. C., and Reingold, E. M. (2014). Eye movements in visual cognition: the contributions of George W. McConkie. Vis. Cogn. 22, 239–241. doi: 10.1080/13506285.2014.895463
Reinert, M. (1990). ALCESTE, une méthodologie d'analyse des données textuelles et une application: Aurélia de G. de Nerval. Bull. Méthodol. Sociol. 26, 24–54. doi: 10.1177/075910639002600103
Renawi, A., Alnajjar, F., Parambil, M., Trabelsi, Z., Gochoo, M., Khalid, S., et al. (2022). A simplified real-time camera-based attention assessment system for classrooms: pilot study. Educ. Inf. Technol. 27, 4753–4770. doi: 10.1007/s10639-021-10808-5
Russakovsky, O., Deng, J., Su, H., Krause, J., Satheesh, S., Ma, S., et al. (2015). Imagenet large scale visual recognition challenge. Int. J. Comput. Vis. 115, 211–252. doi: 10.1007/s11263-015-0816-y
Saha, D., Ferdoushi, M., Emrose, M. T., Das, S., Hasan, S. M., Khan, A. I., et al. (2018) “Deep learning-based eye gaze controlled robotic car” In 2018 IEEE Region 10 Humanitarian Technology Conference (R10-HTC). IEEE. pp. 1–6.
Shao, Z., Zhao, R., Yuan, S., Ding, M., and Wang, Y. (2022). Tracing the evolution of AI in the past decade and forecasting the emerging trends. Expert Syst. Appl. 209:118221. doi: 10.1016/j.eswa.2022.118221
Shavelson, R. J. (2013). On an approach to testing and modeling competence. Educ. Psychol. 48, 73–86. doi: 10.1080/00461520.2013.779483
Shi, Y., Zhu, Y., Mehta, R. K., and Du, J. (2020). A neurophysiological approach to assess training outcome under stress: a virtual reality experiment of industrial shutdown maintenance using functional near-infrared spectroscopy (fNIRS). Adv. Eng. Inform. 46:101153. doi: 10.1016/j.aei.2020.101153
Siddique, Z., Panchal, J., Schaefer, D., Haroon, S., Allen, J. K., and Mistree, F. (2012). “Competencies for innovating in the 21st century,” In International design engineering technical conferences and computers and information in engineering conference. American Society of Mechanical Engineers. 45066, 185–196.
Singh, A., and Das, S. (2022). “A cheating detection system in online examinations based on the analysis of eye-gaze and head-pose” In THEETAS 2022: Proceedings of The International Conference on Emerging Trends in Artificial Intelligence and Smart Systems, THEETAS 2022. 16–17 April 2022, Jabalpur, India, p. 55, European Alliance for Innovation.
Singh, J., and Modi, N. (2022). A robust, real-time camera-based eye gaze tracking system to analyze users’ visual attention using deep learning. Interact. Learn. Environ. 1–22:561. doi: 10.1080/10494820.2022.2088561
Singh, M., Walia, G. S., and Goswami, A. (2018) Using supervised learning to guide the selection of software inspectors in industry. In: 2018 IEEE International Symposium on Software Reliability Engineering Workshops (ISSREW), Memphis, TN, USA, pp. 12–17.
Spencer, L. M. (1997). “Competency assessment methods” in Assessment, development, and measurement. eds. L. J. Bassi and D. F. Russ-Eft, vol. 1 (Alexandria, VA: American Society for Training & Development)
Su, M. C., Cheng, C. T., Chang, M. C., and Hsieh, Y. Z. (2021). A video analytic in-class student concentration monitoring system. IEEE Trans. Consum. Electron. 67, 294–304. doi: 10.1109/TCE.2021.3126877
Swiecki, Z., Khosravi, H., Chen, G., Martinez-Maldonado, R., Lodge, J. M., Milligan, S., et al. (2022). Assessment in the age of artificial intelligence. Comput. Educ. Artif. Intellig. 3:100075. doi: 10.1016/j.caeai.2022.100075
Szegedy, C., Liu, W., Jia, Y., Sermanet, P., Reed, S., Anguelov, D., et al. (2015). “Going deeper with convolutions” In Proceedings of the IEEE conference on computer vision and pattern recognition. 1–9.
Tamim, H., Sultana, F., Tasneem, N., Marzan, Y., and Khan, M. M. (2021) “Class insight: a student monitoring system with real-time updates using face detection and eye tracking” In IEEE World AI IoT Congress (AIIoT), Seattle, WA, USA, 2021, pp. 0213–0220.
Tien, T., Pucher, P. H., Sodergren, M. H., Sriskandarajah, K., Yang, G. Z., and Darzi, A. (2014). Eye tracking for skills assessment and training: a systematic review. J. Surg. Res. 191, 169–178. doi: 10.1016/j.jss.2014.04.032
van Merriënboer, J. J. G., and Kirschner, P. A. (2017). Ten steps to complex learning: A systematic approach to four-component instructional design. 3rd Edn. New York and London: Routledge.
Wade, N., and Tatler, B. W. (2005) The moving tablet of the eye: The origins of modern eye movement research. USA: Oxford University Press
Wang, X., Zhao, X., and Zhang, Y. (2021). Deep-learning-based reading eye-movement analysis for aiding biometric recognition. Neurocomputing 444, 390–398. doi: 10.1016/j.neucom.2020.06.137
Wu, Q., Dey, N., Shi, F., Crespo, R. G., and Sherratt, R. S. (2021). Emotion classification on eye-tracking and electroencephalograph fused signals employing deep gradient neural networks. Appl. Soft Comput. 110:107752. doi: 10.1016/j.asoc.2021.107752
Xin, L., Bin, Z., Xiaoqin, D., Wenjing, H., Yuandong, L., Jinyu, Z., et al. (2021). Detecting task difficulty of learners in colonoscopy: evidence from eye-tracking. J. Eye Mov. Res. 14:10. doi: 10.16910/jemr.14.2.5
Zemblys, R., Niehorster, D. C., Komogortsev, O., and Holmqvist, K. (2018). Using machine learning to detect events in eye-tracking data. Behav. Res. Ther. 50, 160–181. doi: 10.3758/s13428-017-0860-3
Keywords: eye tracking, artificial intelligence, competency, assessment, engineering education
Citation: Ndiaye Y, Lim KH and Blessing L (2023) Eye tracking and artificial intelligence for competency assessment in engineering education: a review. Front. Educ. 8:1170348. doi: 10.3389/feduc.2023.1170348
Edited by:
Pascal Klein, University of Göttingen, GermanyReviewed by:
Habiddin Habiddin, State University of Malang, IndonesiaShuai Liu, Hunan Normal University, China
Copyright © 2023 Ndiaye, Lim and Blessing. This is an open-access article distributed under the terms of the Creative Commons Attribution License (CC BY). The use, distribution or reproduction in other forums is permitted, provided the original author(s) and the copyright owner(s) are credited and that the original publication in this journal is cited, in accordance with accepted academic practice. No use, distribution or reproduction is permitted which does not comply with these terms.
*Correspondence: Yakhoub Ndiaye, eWFraG91Yl9uZGlheWVAc3V0ZC5lZHUuc2c=