ChatGPT in didactical tetrahedron, does it make an exception? A case study in mathematics teaching and learning
- 1Department of Mathematics Education, Universitas Pendidikan Indonesia, Bandung, Indonesia
- 2Indonesian DDR Development Center (PUSBANGDDRINDO), Universitas Pendidikan Indonesia, Bandung, Indonesia
- 3College of Education and Human Ecology, Ohio State University, Columbus, OH, United States
- 4Faculty of Teacher Training and Education, Universitas Sebelas Maret, Surakarta, Indonesia
The didactical tetrahedron model proposes a framework for integrating technology into the previous didactical triangle. This study addresses this issue through examining the role of ChatGPT in educational settings. This quantitative and qualitative study reveals differences among three groups. We observed that students relying solely on ChatGPT for learning resulted in lower performance compared to those receiving instruction from teachers, either alone or supported by ChatGPT. The findings highlight the potential of ChatGPT in enhancing mathematical understanding, yet also underscore the indispensable role of instructors. While students generally perceive ChatGPT as a beneficial tool for learning mathematical concepts, there are concerns regarding over-reliance and the ethical implications of its use. The integration of ChatGPT into educational frameworks remains questionable within a didactic context, particularly due to its limitations in fostering deep information comprehension, stimulating critical thinking, and providing human-like guidance. The study advocates for a balanced approach, suggesting that ChatGPT can augment the learning process effectively when used in conjunction with guidance. Thus, positioning technology as an independent focal point in transforming the didactic triangle into a didactical tetrahedron is not appropriate, even when represented by ChatGPT.
1 Introduction
Previous research such as that conducted by Freudenthal (1991), Brousseau (1997), and Steinbring (2005), has focused on math learning and teaching by studying the interactions among students, teachers, and the subject matter. These interactions are typically represented using the didactic triangle (see Figure 1). The didactic triangle, also known as the “instructional triangle,” is an early model introduced by the German educator Friedrich Christoph Dahlmann in the 1960s. It consists of three fundamental components: the teacher, the student, and the teaching material (Straesser, 2007). The model emphasizes the interconnectedness of these three elements in the process of teaching and learning. In the didactic triangle, the teacher is responsible for mediating and facilitating the transfer of knowledge to the students. Students play an active role in the learning process, engaging with the teaching material and interacting with the teacher. The teaching material represents the content and concepts being taught (Brousseau, 1997).
The didactic triangle provides a foundational framework for understanding the interaction among these three elements, underscoring the importance of a harmonious alignment for effective teaching and learning. To explicitly consider the role of technology in these interactions, the didactic triangle has been expanded by Tall (1986) and more recently by Olive et al. (2010) and Ruthven (2012) into a new concept known as the didactic tetrahedron (see Figure 2).
The didactical tetrahedron, a conceptual model used in education, particularly in the context of mathematics and digital technology integration, extends the traditional didactical triangle by incorporating technology as a critical fourth element (Jukić Matić and Glasnović Gracin, 2016; Prediger et al., 2019). This inclusion underscores the transformative role of technology in reshaping the educational landscape, especially in facilitating investigative approaches to teaching and learning mathematics (Remillard and Heck, 2014). It redefines the role of teachers from mere conveyors of knowledge to facilitators who guide students in a technologically enriched learning environment. The framework also brings to light the challenges of integrating technology in education, while simultaneously presenting opportunities for enhancing student engagement and understanding of mathematical concepts (Olive et al., 2010; Cao et al., 2021). Therefore, it offers a comprehensive framework for understanding the complex interactions between teachers, students, subject matter, and technology in the modern educational context, advocating for a holistic approach that encompasses these interconnected dimensions to enrich the teaching and learning experience.
Since its introduction, the didactical tetrahedron has been widely embraced and expanded upon by researchers, educators, and curriculum developers. It has influenced the design of educational materials, the development of teaching strategies, and the integration of technology in various educational contexts (Aldon et al., 2021; Cao et al., 2021; Novita and Herman, 2021). This concept remains relevant and widely used in educational research and practice, offering a holistic perspective on the teaching and learning process. According to Olive et al. (2010), the introduction of technology into didactic situations can have transformative effects, leading to a better representation using the didactic tetrahedron. This tetrahedron illustrates the interaction between the teacher, students, tools, and mathematical knowledge, all mediated by technology (p. 168).
Suryadi (2019) provides criticism regarding the independence of technology as a new point in the didactic triangle. Through the question, “Can technology stand alone in relation to didactics?” Suryadi (2019) explains that we must return to the definition of didactics, where didactics is related to the diffusion and acquisition of knowledge. Because the actors of the diffusion process are teachers, the actors of the acquisition process are students, and the substance being diffused or acquired is existing knowledge (the result of transposition processes), the idea of the didactic triangle can logically be understood as the relationship between the three main entities of the diffusion and acquisition of knowledge events. In the didactic triangle, each party has its role in the context of diffusion and acquisition of knowledge, resulting in relationships that can be explained from both the diffusion and acquisition perspectives (Bosch, 2015).
The student-material relationship is referred to as the didactic relationship, which describes the process of knowledge acquisition in the didactic context as science, epistemology, or art (Suryadi, 2019b). The teacher-student relationship is called the pedagogical relationship because interaction between the educator and the learner is required in the process of diffusion and acquisition of knowledge (Suryadi, 2019b). This interaction is fundamentally based on the learner’s need for development, both in actual and potential stages, as explained in Vygotsky’s theory (Vygotsky, 1978). The teacher-material relationship is called the anticipatory didactic-pedagogical relationship (ADP). This relationship illustrates that an educator must have predictive thinking regarding the process of diffusion and acquisition of knowledge so that every possible outcome in the acquisition of new knowledge is anticipated, both from a didactic and pedagogical perspective.
Furthermore, Suryadi (2019) explains that technology plays a crucial role in the diffusion and acquisition of knowledge. However, it is important to realize that technology is not an independent entity but a tool used by educators to enhance the learning process (Ghavifekr and Rosdy, 2015). This requires thoughtful consideration and design by educators to make the educational experience more engaging, efficient, and easily accessible. The philosophical justification for the use of technology lies in its ability to facilitate extended cognition, providing opportunities for humans to develop their cognitive abilities. Meanwhile, in the didactical tetrahedron, the interaction between technology and teacher, student, and content is described as follows:
1. Technology and teachers: Technology plays a crucial role in supporting teachers’ teaching practices and enhancing their teaching methods. Teachers can use technology to access various digital resources, teaching aids, and multimedia materials that can enrich their lessons and make them more engaging.
2. Technology and students: Technology provides students with new opportunities to learn, collaborate, and express themselves. It allows students to access a vast amount of information, research materials, and educational content beyond traditional classroom resources. Through technology, students can engage in interactive and multimedia-rich learning experiences, which can enhance their understanding and retention of course materials. Furthermore, technology enables personalized learning experiences, adaptive assessment, and feedback, catering to individual student needs and promoting independent learning. Collaborative technology also facilitates peer-to-peer learning, communication, and teamwork.
3. Technology and content: Technology can transform and augment the materials used in teaching and learning (Alneyadi et al., 2023). It provides alternative formats, multimedia presentations, simulations, and interactive resources that make course materials more accessible and engaging. Digital textbooks, e-books, online databases, and educational websites offer extensive and up-to-date information on various topics. Additionally, technology enables the creation of digital learning materials such as educational videos, interactive presentations, and online quizzes tailored to specific learning objectives. Technology also allows real-time updates and modifications to materials, ensuring that they remain current and relevant (Olive et al., 2010; Rezat and Sträßer, 2012; Ruthven, 2012).
Overall, technology serves as a catalyst in the didactical tetrahedron, supporting teachers in their teaching practices, empowering students in their learning experiences, and enriching the materials used in the teaching process. When integrated thoughtfully and purposefully, technology can enhance educational outcomes and foster creativity, critical thinking, and collaboration between teachers and students (Yang and Wu, 2012). Thus, there is no urgency to place technology as an independent point in the didactic concept. But what about the recent technology we know as Chat-GPT? Does the presence of Chat-GPT justify that technology can stand independently in the didactic concept, making the didactical tetrahedron relevant?
ChatGPT is an advanced language model developed by Open AI (Biswas, 2023; Lund and Wang, 2023). It is built upon the GPT (Generative Pre-trained Transformer) architecture, specifically GPT-3.5 (Rehana et al., 2023). This model is designed to generate human-like text responses based on the input it receives in the form of prompts or messages (Haleem et al., 2022; Adiguzel et al., 2023; Pavlik, 2023). ChatGPT is trained on a large dataset of internet text, allowing it to learn language patterns, structure, and contextual understanding (Lund and Wang, 2023). It can comprehend and produce text across various domains, covering a wide range of topics, with the goal of generating coherent and contextually relevant responses that simulate natural human conversation (Hassani and Silva, 2023). The chat-based GPT format allows users to engage in interactive and dynamic conversations with the model. Users provide instructions or messages, and the model generates responses based on the input it receives. The model’s responses are not pre-determined but generated quickly, taking into consideration the conversation context. Open AI has provided various versions of the GPT model, and ChatGPT is one specific implementation focused on providing conversational capabilities (Mhlanga, 2023). It has been used in various applications, including customer support, language translation, creative writing assistance, and education support (Mattas, 2023).
In terms of educational assistance, ChatGPT, an advanced language model, has a significant role to play in the learning process. It assists in various ways, such as information retrieval, enabling learners to quickly access and expand their knowledge on a wide range of topics (Lo, 2023). When it comes to explaining concepts, ChatGPT excels by breaking down complex ideas into understandable components, providing examples, and offering clarifications, thus deepening learners’ understanding (Coskun, 2023). As a practice partner, ChatGPT engages learners in simulated conversations or written exchanges, offering valuable feedback on grammar, vocabulary, and coherence, thereby enhancing communication skills (Shaikh et al., 2023). The interactive learning experiences facilitated by ChatGPT, such as quizzes, puzzles, and riddles, not only engage students but also allow for a more personalized learning journey through its adaptive responses (Elbanna and Armstrong, 2023).
Observing how technology is now perceived, especially given its rapid development over the past decade, it seems that ChatGPT, as a trained language model, has the potential to usher in a new reality about technology in the realm of education, and specifically in its position within this study’s framework. Justifications about technology in education that were held previously now open up new discussions and questions as breakthroughs in technology emerge and evolve too quickly. These developments may lead to a fresh interpretation of technology compared to before. It is, therefore, very important for us to continually evaluate and explore its role in education. Such dynamics will help maintain the strength of knowledge and ensure that mathematics education remains epistemic for students (Gupta and Elby, 2011). This research aims to explore the potential use of Chat-GPT in mathematics education. Through a comprehensive study, the objective is to assess the role and impact of Chat-GPT on overall student performance, engagement, and learning experiences.
Therefore, we propose three research questions that we will answer through this study:
1. Is there a significant difference in math performance between students who solely use ChatGPT without any guidance from a lecturer, those who receive instructions with ChatGPT’s assistance, and those who receive regular instructions without ChatGPT’s help?
2. What do students think about using ChatGPT for learning and grasping mathematical concepts?
3. Does the inclusion of ChatGPT provide a valid justification within the didactical tetrahedron framework?
2 Materials and methods
Referring to the research question posed, this study adopts two approaches: quantitative research and qualitative research. We’re not calling it a mixed-methods study because we believe that ontologically, quantitative and qualitative research are at odds with each other, making it challenging to combine them to investigate the same issue. Quantitative research is rooted in the ontological perspective known as positivism or postpositivism (Dieronitou, 2014). Positivism holds that there is an objective reality that can be studied and understood through empirical observation and measurement (Tuli, 2011). It argues that the social world operates according to generalizable laws, similar to those found in the natural sciences. In this view, reality is considered external and independent of the researcher, and its goal is to uncover universal patterns and cause-and-effect relationships. On the other hand, qualitative research is based on a different ontological perspective known as constructivism, interpretivism, or social constructivism (Lee, 2012). This perspective argues that reality is socially and subjectively constructed, and meaning is actively created by individuals and groups through their interactions and interpretations of the world (Fischer and Guzel, 2023). Qualitative research seeks to understand the complexity and depth of human experiences, perspectives, and social phenomena (Rahman, 2016). In short, in quantitative research, the relationship between the researcher and the research sample should be independent, whereas in qualitative research, the relationship between the researcher and the research subjects should be dependent.
In this case, the researchers conducted quantitative research first to address the first research question. During this phase, the researchers ensured that the relationship between the researcher and the research sample remained independent. Subsequently, the second and third research questions were answered using a qualitative approach.
2.1 Research design
In the quantitative research part, we adopted a static group comparison as part of a quasi-experimental design. This type of design is particularly useful when traditional experimental designs are not practical or ethical. In our case, it involved studying both an experimental group and a control group with different treatments, as described by Kirk (2009). The experimental group consisted of students who received instruction using ChatGPT. This study included two different experimental groups: experimental group 1, where students received complete instructions solely through ChatGPT during the learning process, and experimental group 2, where students engaged in collaborative learning with a teacher using ChatGPT as a tool. Meanwhile, the control group consisted of students who received treatment as usual. In the quasi-experimental design, we compared groups or conditions that already existed (e.g., different classes or schools), without randomly assigning participants to conditions or manipulating the independent variable. This approach was chosen due to the logistical constraints within schools and educational systems that often make traditional experimental designs difficult to implement. In practice, we enlisted local teachers to administer the treatments, ensuring that the treatment for the control group closely resembled their regular classroom experience. However, prior to the study, researchers also provided guidance to the respective teachers regarding the treatment for experimental group 2, which involved the integration of ChatGPT into the learning process.
On the other hand, in the qualitative research part, we employed a phenomenological design. Phenomenological qualitative research is an approach aimed at understanding the life experiences and subjective perspectives of individuals (Creswell, 2012). It seeks to explore and describe the essence and meaning of the experiences that students go through when using ChatGPT in their mathematics learning. Phenomenology focuses on phenomena that emerge in consciousness and emphasizes understanding the rich and unique qualities of an experience.
2.2 Sampling and subjects
The quantitative research was conducted in the city of Surakarta, Indonesia, involving a study population comprising students from three universities, each with the same accreditation level. These participants were sixth-semester mathematics education students enrolled in a numerical methods course. Additionally, they had undergone a relatively similar selection process for admission, in terms of both content and procedure. Due to logistical limitations and the inability to assign participants randomly, a cluster random sampling was used. One of the universities was selected as the sample, with just one class currently undertaking the numerical methods course. In the next phase, the chosen class for the study was divided into three groups through random sampling of 33 papers. Each paper contained information about its group type, and there were 11 papers for each group type in total. This random sampling of the 33 papers created a more detailed experimental framework. Class A, consisting of 9 participants, was designated as experimental group 1. Similarly, Class B, also with 9 participants, was identified as experimental group 2. Meanwhile, Class C, with 11 participants, was set as the control group. Thus, this study involved 29 undergraduate students in a mathematics education program.
Meanwhile, the qualitative research involved in-depth interviews with a total of five students who had recently used ChatGPT in their learning process. The participants were selected using a combination of criteria and snowball sampling techniques. Inclusion criteria for participants included being part of the experimental classes that had implemented ChatGPT in their learning and their ability to articulate their experiences in a thoughtful and reflective manner. To protect the confidentiality and anonymity of the participants, pseudonyms were assigned to each participant in the reporting of findings. Participants were provided with detailed information about the research, its purpose, and the voluntary nature of their participation. Informed consent was obtained from each participant before the interviews, ensuring their understanding of the research’s objectives and their rights as research subjects (Marshall et al., 2006).
2.3 Data collection and instrument
The data for this research was collected using a combination of quantitative and qualitative methods tailored to the research questions. The aim was to provide a comprehensive understanding of the impact of the new instructional intervention (ChatGPT) on students’ mathematical problem-solving abilities, their attitudes toward using ChatGPT for learning mathematics, and ultimately provide justification for the role of ChatGPT in the didactic concept.
Quantitative data collection: To assess students’ mathematical problem-solving abilities, we employed a posttest-only, non-equivalent control group design. This is a type of quasi-experimental research design that involves comparing the outcomes or effects of an intervention or treatment between two groups: the experimental group and the control group (Kirk, 2008). In this design, participants in all three groups were measured on the dependent variable (desired outcomes) after the intervention was administered, but there was no pretest measurement. Posttest scores were analyzed using statistical methods to test for significant changes and differences in students’ math performance.
Qualitative data collection: Qualitative data were gathered to gain insights into students’ attitudes toward ChatGPT and their experiences with the intervention. Semi-structured interviews were conducted with some participants to explore their perceptions, beliefs, and experiences related to learning mathematics and the instructional intervention. Interviews were audio-recorded and transcribed verbatim for analysis. The interview questions focused on students’ attitudes toward ChatGPT, their involvement in the intervention activities, and their perceptions of the intervention’s impact on their learning experience. Additionally, classroom observations were conducted to provide contextual information about the implementation of the intervention. Researchers observed the learning sessions to gather data on teaching methods, materials used, and student interactions. Field notes were taken during observations, capturing class dynamics, student engagement, and any significant observations related to the implementation of the intervention.
2.4 Data analysis technique
Quantitative Analysis: To assess the impact of the intervention on students’ math performance, the posttest scores from the experimental and control groups are the focus of statistical analysis. First, descriptive statistics such as mean, standard deviation, and frequency are calculated to summarize the data and provide an overview of the students’ math performance. Then, inferential statistical tests are used to determine whether there are any significant differences in math performance among the three groups. Specifically, a One-way ANOVA test is conducted to compare the average scores between the experimental and control groups (Kirk, 2008). Additionally, effect sizes are calculated to assess the practical significance of any observed differences.
Qualitative Analysis: Qualitative data collected through interviews and classroom observations are analyzed using thematic analysis. Transcripts of interviews and field notes from observations are carefully reviewed and coded to identify recurring themes and patterns related to students’ attitudes toward ChatGPT and their experiences with instructional interventions. The coding process involves assigning meaningful labels to segments of data, grouping similar codes into categories, and refining the coding scheme through an iterative process. The identified themes are then interpreted and supported by relevant quotes from interviews and field notes to provide a nuanced and rich understanding of the students’ perspectives.
3 Result and discussion
In this section we briefly describe the result of three different groups in learning activities, then continue with the discussions regarding the mathematical performance, students’ perspective about ChatGPT for learning and constructing mathematical concept, and the view of ChatGPT in didactics concept.
The first group that learning activities of the group using only ChatGPT as their primary instruction tool started off with enthusiasm. This method provided quick feedback on questions related to the numerical methods course. Unfortunately, this initial enthusiasm did not last long because the group became confused about what they should be asking the system. As a result, when they faced a test without ChatGPT’s assistance, they were not prepared to tackle the challenges in problem-solving.
Now the group that used ChatGPT as a learning aid with the guidance of a facilitator, the lecturer, showed more directed results. The lecturer provided guidelines on the material to be studied, enabling students to utilize ChatGPT more effectively. Interacting with the lecturer allowed students to validate the information obtained from ChatGPT and receive additional explanations if there was any confusion. Thus, the knowledge acquired from ChatGPT could be directly validated by the lecturer for accuracy. Furthermore, this approach provided effective learning where the lecturer remained actively involved in the learning process while leveraging technology.
On the other hand, in groups that solely relied on the lecturer for learning, a more traditional approach was apparent. Nevertheless, this method resulted in a better understanding of the material compared to a method without lecturer involvement. Direct interaction with the lecturer allowed students to clarify doubts and, therefore, gain a deeper understanding. While the feedback may not be as quick as what ChatGPT offers, the closeness of interaction provided by the lecturer remained invaluable. This underscores that even though technology plays a role in enhancing the learning process, communication remains a key element in grasping the material.
3.1 Students’ mathematical performance
This section aims to compare the math performance among students who exclusively used ChatGPT, students who received instructions from a lecturer with the assistance of ChatGPT, and students who only received instructions from a lecturer. The math instructions given to the students with the instuctors were identical in terms of content, duration, and difficulty level. After the instructional period, an evaluation test consisting of a series of math questions covering the taught material was conducted. Test scores represented students’ math performance. Table 1 outlines the details of the descriptive analysis results.
Based on Table 1, it is observed that Experimental Group 1, which used only ChatGPT, achieved an average score of with a standard deviation of . Experimental Group 2, which received instruction from a professor aided by ChatGPT, attained a much higher average test score of with a standard deviation of . In contrast, the control group, receiving only regular instruction, had an average score of with a standard deviation of .
Prior to hypothesis testing, assumption tests were conducted, including tests for normality and homogeneity. Referring to Figure 3, the Q-Q Plot indicates that the data appears to be normally and linearly distributed. However, in the homogeneity test, the value of p was found to be , which is less than the alpha level of , indicating that the data is heterogeneous. Therefore, while the assumption of normality is met, the assumption of homogeneity is not. Consequently, the Welch ANOVA test was chosen to compare the means between groups in the experimental design, with the following hypothesis:
except
The output of the testing using SPSS is presented in Table 2. According to Table 2, it is evident that the value of p is , which is less than the alpha level of , leading to the rejection of the null hypothesis ( ). This indicates that there is a significant difference in the mean scores among the three groups. Furthermore, the Effect Size ( ) is calculated to be , suggesting a substantial impact (referenced). Due to the rejection of , Post-hoc Tests were conducted to identify which pairs of group means differ significantly (see Table 3).
The post-hoc tests reveal significant differences between Class A and Class B ( value = ), and between Class A and Class C ( value ). However, no significant difference was found between Class B and Class C ( value ). However, given the difference in means, the use of ChatGPT as a teaching aid in mathematics education supplemented by instructor guidance, is worthy of further investigation.
Quantitatively, it is evident that the math performance of students taught by lecturers or with ChatGPT assistance is better compared to students who learn without a lecturer and solely rely on ChatGPT. This underscores the importance of the lecturer’s role in providing deeper and contextual math instruction, while ChatGPT can offer additional support in understanding concepts. This research outcome indicates the significance of the lecturer’s role in delivering context-rich, personalized instruction with direct interaction. Lecturers can adapt their teaching approach to students’ needs, clarify complex concepts, and stimulate in-depth discussions. Therefore, lecturer-student interaction remains a significant factor in enhancing students’ understanding of mathematics, as conveyed by Heggart and Yoo (2018).
Teachers (lecturers) possess a domain of knowledge and expertise in the field of mathematics, enabling them to provide tailored guidance, explanations, and clarifications to meet students’ needs (Troussas et al., 2020). ChatGPT can offer information and answers based on its data, but it may not have the same level of expertise or understanding of individual student needs as lecturers do (Baidoo-Anu and Owusu Ansah, 2023; Lecler et al., 2023). ChatGPT also lacks the ability to provide justification regarding whether the information it conveys holds absolute truth (Sun and Hoelscher, 2023). Additionally, lecturers can adapt their teaching methods based on students’ progress and learning styles, providing direct feedback. They can offer personalized instructions and modify their approaches to cater to different learning needs. On the other hand, ChatGPT can provide standard responses without the ability to adapt to each student’s learning requirements (Aydın and Karaarslan, 2023).
Mathematics can be a complex subject, and students often require in-depth explanations and clarifications of abstract mathematical concepts. Lecturers can provide real-time examples, demonstrations, and interactive discussions to help students grasp these mathematical ideas. While ChatGPT can provide information, it cannot offer the same level of dynamic and interactive explanations (Baidoo-Anu and Owusu Ansah, 2023; Ray, 2023). Lecturers are trained in pedagogical strategies and teaching methodologies designed to enhance student learning (Phuong et al., 2018). They can employ various teaching techniques, such as visual aids, problem-solving exercises, and interactive activities, to engage students and foster a deeper understanding (Brinkley-Etzkorn, 2018; Singh et al., 2021). ChatGPT, as a language model, lacks the same pedagogical training and cannot effectively use these strategies (Kasneci et al., 2023).
Low performance in groups solely relying on ChatGPT indicates limitations in the model’s ability to provide comprehensive math learning support. ChatGPT may struggle with understanding highly specific questions, offering context-appropriate examples, and solving more complex problems (Tlili et al., 2023). Study by Hassan et al. (2023) reported that ChatGPT can handle routine inquiries and tasks, even though it is only freeing up time for more complex task. Also, Ray (2023) discussed on how handling more complex tasks may still present challenges. It is essential to note that while ChatGPT can provide valuable information and assistance, it is most effective when used as a complement to human instruction rather than a substitute for human educators (Jeon and Lee, 2023; Tlili et al., 2023). The combination of human expertise and guidance with ChatGPT’s capabilities presents an opportunity to enhance students’ math performance. This finding highlights the potential use of ChatGPT technology as an effective learning tool in improving students’ math performance. Further research can explore optimal ways to integrate this technology into broader learning contexts.
3.2 Students’ perspective about ChatGPT for learning and constructing mathematical concept
This section aims to investigate students’ views on the effectiveness and benefits of using ChatGPT in the learning process and understanding mathematical concepts. The interviews focus on questions related to their experiences using ChatGPT in the context of learning mathematics, their opinions on the utility of the tool, and the impact of using ChatGPT on their understanding of mathematical concepts. There are 15 main questions posed to explore students’ perspectives and experiences regarding the use of ChatGPT in the learning process and the formation of their understanding of mathematical concepts. Table 4 presents the questions asked to the students.
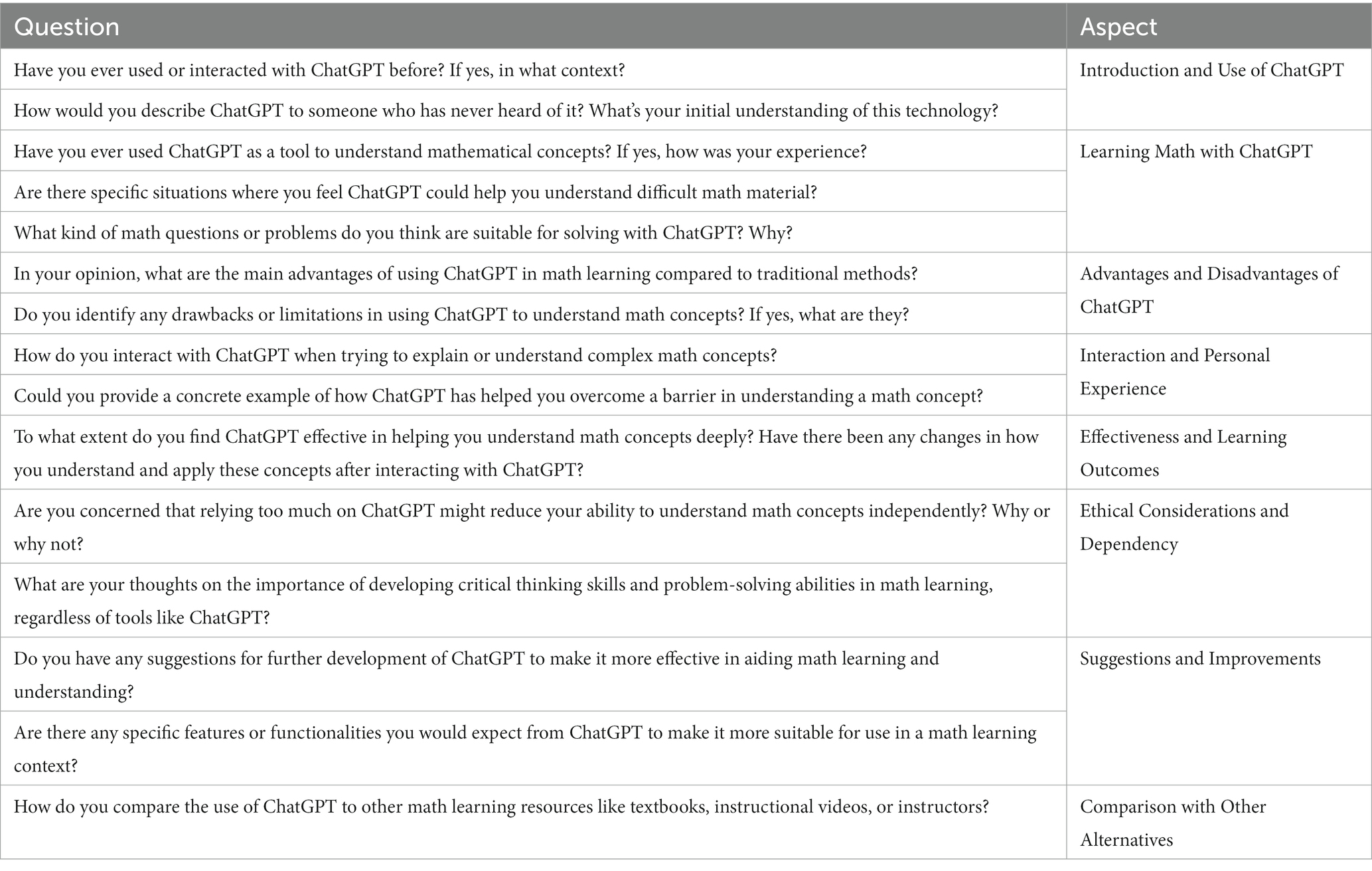
Table 4. Questions to understand students’ perspectives and experiences related to the use of ChatGPT.
The thematic analysis of the interviews reveals some important findings. Overall, the students were familiar with ChatGPT before this research was conducted. However, some students had limited knowledge about ChatGPT’s functions. In general, they described this technology as a computer program that uses artificial intelligence (AI) to generate text or respond to user requests. As a result, most of them used ChatGPT mainly to complete tasks related to paper writing or essays. Using ChatGPT to assist in essay writing could be a useful tool for students, especially when they have difficulty in constructing sentences or expressing ideas effectively.
Meanwhile, some students have used ChatGPT as a tool to understand mathematical concepts. Students who were taught by instructors with the help of ChatGPT found it to be a useful tool in learning mathematics. They appreciated ChatGPT’s ability to provide clear and structured explanations of complex mathematical concepts. Students also highlighted ChatGPT’s ability to provide solutions and problem-solving strategies that were useful in their math assignments. Students reported that using ChatGPT improved their understanding of mathematical concepts. They felt more confident and capable of overcoming difficulties in understanding with the help of ChatGPT. They also emphasized that ChatGPT’s use is suitable in the context of providing explanations about basic concepts, definitions, and procedures. This aligns with ChatGPT’s main strength in generating text that can break down concepts in a structured way.
Some students felt that ChatGPT helped them deepen their understanding of the material they were studying. They considered it a valuable resource for further understanding challenging aspects of the concepts they found difficult. However, besides these benefits, some students also expressed concerns about excessive reliance on ChatGPT. They argued that excessive dependence on technology could reduce their ability to solve problems independently. Additionally, it should be noted that ChatGPT’s effectiveness is currently limited in understanding complex mathematical contexts or providing highly personalized explanations. Moreover, while ChatGPT serves as a helpful tool, its limitations in comprehensibility and adaptability to individual learning styles cannot be overlooked. The information provided by ChatGPT, though extensive and varied, may not always align perfectly with the specific curricular context or the unique conceptual misunderstandings a student might have. This gap can lead to partial or misaligned understanding, especially in subjects where nuance and depth of knowledge are critical. Furthermore, ChatGPT’s algorithmic nature means it might not always capture the subtleties of human thought processes or the specific pedagogical approaches that a teacher might use to address a student’s unique learning needs. This limitation underscores the importance of using ChatGPT as a supplementary tool, one that complements but does not replace the personalized guidance and expertise of a human educator.
In some cases of complex math problems, students revealed their inability to understand ChatGPT’s responses. The explanations provided by the system were not consistent with the students’ prior knowledge, coupled with ChatGPT’s inability to solve more complex math problems. Consequently, students who should have benefited from ChatGPT ended up facing obstacles in their learning. Hence, there is a need for instructors to play a role as knowledge confirmers. However, for simpler math cases, students acknowledged the help provided by this system, allowing them to learn more independently and acquire various ways or tricks to answer questions. Some students added that the clarity and accuracy of ChatGPT’s answers depend on the clarity and detail of the questions asked. The clearer and more detailed the questions, the closer the response will be to what they are looking for. However, sometimes students accept ChatGPT’s concepts without any justification from the instructor or other sources. Therefore, learning would be more effective if ChatGPT is used as a tool to assist learning under the guidance of instructors, as ChatGPT cannot provide a human-like guidance. This reliance on ChatGPT for learning math without proper guidance or confirmation from educators can lead to misunderstandings and incomplete learning. Students, particularly those with less experience in self-directed learning or weaker foundational knowledge.
Next, there are students’ concerns about ethical considerations. They feel that while AI technology offers benefits, it also has the potential to raise ethical dilemmas. These students worry that excessive reliance on ChatGPT could hinder the development of their skills. There is a common sentiment that the lure of convenience may come at the expense of intellectual growth and contribute to the spread of biased information. Concerns about addiction are also felt by some students, especially after observing examples where they and their peers became overly dependent on ChatGPT for tasks that could be done independently. On the contrary, other students do not share these concerns and consider AI tools as valuable assets that greatly assist their academic achievements. They appreciate its ability to generate ideas, answers, and explanations quickly, allowing them to delve deeper into complex subjects.
The results show a dual perspective among students regarding ChatGPT, making it clear that while it offers undeniable benefits, there are growing concerns about its ethical dilemmas. This aligns with research conducted by Geis et al. (2019), Gong et al. (2019), Pedró (2019), Safdar et al. (2020), and Sit et al. (2020), which surveyed students’ attitudes toward AI-assisted learning tools, especially in terms of ethics and its implications, including privacy, bias, and transparency issues. This is consistent with the findings of this study, where students expressed their concerns about the potential for AI to contribute to the spread of biased information. Additionally, students also voiced concerns that excessive reliance on ChatGPT could hinder their intellectual development. This sentiment experienced by students aligns with Alam (2022) research on the impact of AI tools, showing that students who rely too heavily on AI-generated content demonstrate a decline in their ability to independently analyze and synthesize information. This indicates that while AI tools can provide quick solutions, they may impede the development of their cognitive skills (Vincent-Lancrin and van der Vlies, 2020).
As AI technology continues to evolve and integrate into various aspects of society, there is an urgent need to address the ethical issues it raises. Institutions should consider strategies to promote balanced AI tool usage while also encouraging ethical considerations. However, some argue that AI is the future (Gautam et al., 2022), and schools should embrace it rather than restrict it. At least, students view ChatGPT as certainly not the end of the world and not a complete suppression of independent thinking.
Overall, this section demonstrates that students have a positive perception of the usefulness and effectiveness of ChatGPT in learning and understanding mathematical concepts. Despite some concerns, the use of ChatGPT is seen as a valuable tool in enhancing mathematics education. This research provides valuable insights for the development of ChatGPT technology in an educational context and underscores the importance of considering its limitations and appropriate utilization.
3.3 ChatGPT in didactics concept
The two subtopics discussed above have highlighted the immense potential of ChatGPT to engage in the student learning process, especially in mathematics education. This section will further elaborate on how ChatGPT’s potential is viewed from a didactic perspective. In the didactic triangle, it has been explained that the connecting line between the teacher and the student is called the pedagogical relationship, the line between the student and the material is called the didactic relationship, and the line between the teacher and the material is called the didactic pedagogical anticipation (Suryadi, 2019a). By adding a new independent point, which is technology, to the didactical tetrahedron, new areas emerge: (1) teacher-student-technology; (2) student-material-technology; and (3) teacher-material-technology. Additionally, new lines emerge as well: (1) teacher-technology; (2) student-technology; and (3) material-technology. However, in its justification (Ruthven, 2009; Olive et al., 2010), both in the field and lines of technology, it is only seen as a tool to optimize the learning process. There is no comprehensive explanation as in the case of the fields and lines in the didactic triangle (teacher-student-material), so technology cannot be considered an independent point like the others.
Specifically, this section discusses the inclusion of ChatGPT (as a form of technology) in the didactical tetrahedron as an independent point. Why do not we hold the same opinion as with other educational technologies? This is because of how ChatGPT operates, which is capable of answering various questions posed to it. This discussion involves findings from the previous section. Referring to quantitative data, it is known that a group of students who only use ChatGPT without any intervention from a teacher can also generate knowledge, enabling them to achieve scores not significantly worse than two other groups. The question is, can using ChatGPT alone really build their knowledge?
A group of students who only use ChatGPT, without any intervention from a teacher, may still be able to generate knowledge. However, there are some considerations to keep in mind. Even though ChatGPT can provide useful information and stimulate understanding, the knowledge obtained may not be complete and systematically organized. This limitation primarily occurs because ChatGPT does not provide comprehensive information due to limitations in constructing sentences and other constraints (Ray, 2023). Therefore, the ability to develop cohesive and in-depth knowledge may be hindered if relying solely on ChatGPT (Jarrah, et al., 2023). Furthermore, it is important to remember that building substantial and structured knowledge in a discipline involves more than just receiving information (Langer, 2011). Students need guidance and direction from experts (such as teachers) who can help them understand complex concepts, address misconceptions, and steer them toward deeper resources (To and Carless, 2016). Teachers can also help ensure that students understand the limitations of studying a field and provide the necessary context for better understanding (Yu, 2023). The material presented by ChatGPT may only be a starting point that needs to be further explored through discussion, analysis, and deeper exploration.
Before delving further into this phenomenon, it is worth recalling the process experienced by Plato when learning from Socrates, where Plato’s learning with Socrates can be characterized by the “Socratic method” of questioning (dialectic). It is a method where Socrates engaged others in dialog by asking probing questions to stimulate critical thinking and examine one’s beliefs and assumptions (Owens, 1950). This method is similar to what can be achieved through interaction between students and ChatGPT. Any “question” can be answered by the AI’s reliability, so when the questioner has a critical mindset, it is very possible that ChatGPT’s role can build new knowledge for them. Thus, can we conclude that the presence of ChatGPT can provide justification within the didactical tetrahedron?
It is important to note that the mathematics performance in this research is derived from the students’ work on essay questions. Assessment of essay questions is subjective, meaning it is not just a binary right or wrong but also evaluates the process. There is no guarantee that the “score” obtained by students is solely the result of knowledge gained through their interactions with ChatGPT. Therefore, further analysis is needed. The first analysis is related to how the knowledge formed by each student on the same material. This is done by analyzing the questions posed by students and the answers provided by ChatGPT. Here’s an explanation.
The chosen material is about solving ordinary differential equations (ODE) with the Runge–Kutta and Euler methods. Some questions asked by students and the answers provided by ChatGPT are presented in Table 5.
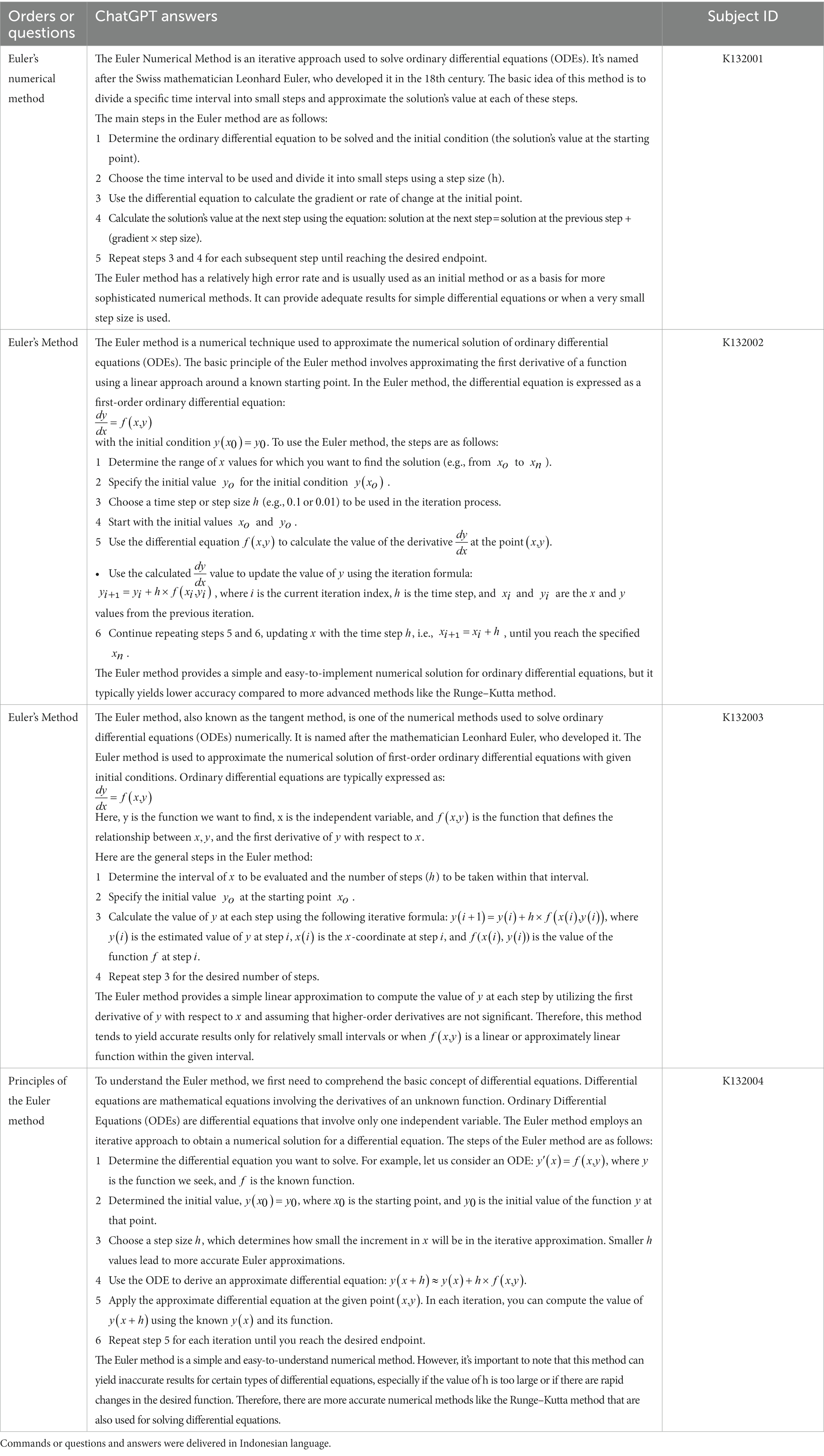
Table 5. Questions to understand students’ perspectives and experiences related to the use of ChatGPT.
Table 5 presents some findings regarding ChatGPT’s responses to questions posed by various subjects about the Euler method. Even though these questions revolve around the same concept, which is the Euler method, ChatGPT’s responses vary in terms of how the material is presented and explained. When analyzing these responses, it can be observed that ChatGPT tends to provide summaries of the Euler method. The knowledge presented in these responses is not presented systematically or epistemically. This has the potential to create obstacles in the learning process, and it may even lead to misconceptions in understanding the material. For example, the explanation about the Euler method equation displayed is: “next step solution = previous step solution + (gradient × step size)” (K132001), (K132002), (K132003), and (K132004).
In case K132001, there is a difference in the presentation model used by ChatGPT. This response uses a geometric interpretation to explain the Euler method equation. However, without additional explanation, this interpretation might be challenging for students to grasp without further insight into the origins and the fundamental ideas behind the equation. Other cases, such as K132002, K132003, and K132004, also indicate difficulties in understanding that the equations generated are derivatives of the expression with respect to using the Taylor series. Explanations may be necessary to help students connect the equations with the underlying basic concepts.
In this context, ChatGPT’s ability to provide adequate explanations depends on the suitability and clarity of the questions asked by the user. If these questions are asked more precisely and appropriately, ChatGPT is likely to provide more comprehensive and structured explanations about the Euler method concept and how the equations emerge from deeper reasoning. It is important to highlight that the information generated by ChatGPT comes from texts found on the internet (Javaid et al., 2023). Therefore, ChatGPT’s responses are based on language patterns, information, and viewpoints present in these texts (Lund and Wang, 2023). The internet resources used by ChatGPT are vast and diverse, covering a wide range of texts, including news, articles, encyclopedias, blogs, forums, websites, and more (Apostolopoulos et al., 2023). In the learning process, this model attempts to understand the relationships between words, phrases, and ideas that appear in these texts. However, it is essential to remember that although ChatGPT can generate coherent and relevant texts based on what it has learned, it does not possess understanding in the conceptual and epistemological sense (Rees, 2022; Mitrović et al., 2023). Therefore, ChatGPT cannot definitively distinguish between valid and false theories.
This occurs because GPT is based on statistics and patterns in the training data and lacks the ability to make contextual judgments and deep understanding of the truth of a statement (Zhang et al., 2023). While ChatGPT can produce responses that sound logical and reasonable based on its learned data, it does not guarantee that these responses are always correct or in line with scientific truth (Wittmann, 2023). When interpreting information provided by ChatGPT, it is important to always consider other sources of information, conduct further research, and use personal judgment to determine the validity of a theory. ChatGPT can be a useful tool for generating text and potential information, but the ultimate responsibility for evaluating the truth and reliability of information remains with the user (Javaid et al., 2023). Therefore, the interaction between students and ChatGPT is significantly different from the Socratic method.
The learning process in the Socratic method involves (Morrison, 2010): (1) Wonder, where questions are posed. Socrates would initiate conversations or dialogs with Plato and other participants, approaching individuals to discuss various topics such as ethics, politics, and knowledge. Similar to ChatGPT, this system will work best if users start by asking questions (Tlili et al., 2023); (2) Hypothesis, which is the response to wonder, where someone provides an opinion or statement regarding a question that becomes the hypothesis of the dialog. This differs from acquiring knowledge through ChatGPT, as the system lacks pedagogical capabilities similar to educators (Wardat et al., 2023), so the responses given by ChatGPT depend on what users ask; (3) Elenchus, refutation, and cross-examination. The essence of Socrates’ practice is that hypotheses are questioned, and counterexamples are provided to prove or disprove the hypotheses. While ChatGPT is a text-based system based on user questions, its responses come from a wide range of internet sources, often with unclear origins (sources not cited), and users often accept the information provided by ChatGPT without cross-checking from other sources (Oviedo-Trespalacios et al., 2023); (4) Acceptance or rejection of the hypothesis, where the choice is made between accepting or rejecting the counterexample information. Socratic methods emphasize sharp questioning and argument testing to gain a deeper understanding of concepts and sift valid from invalid information. On the other hand, interactions with ChatGPT often involve the consumption of raw information without much critical consideration. This can be a challenge if the information provided by ChatGPT is not entirely accurate, complete, or contextually suitable (Eriksson and Larsson, 2023); (5) Action, where the findings of the investigation are acted upon. In the Socratic method, taking action on findings is important for gaining a deeper understanding of arguments, identifying argument foundations, and exploring implications of discussed ideas. Socrates would encourage his interlocutors to think more critically and reflect on the arguments presented, allowing for a better understanding of the strengths and weaknesses of those arguments. On the other hand, in interactions with ChatGPT, users like students often tend to accept the information provided without much critical thought (Deiana et al., 2023). This can be a challenge if the information provided by ChatGPT is not entirely accurate, complete, or suitable within a specific context. Therefore, it is important for users to develop critical thinking skills even in interactions with technology like ChatGPT.
While ChatGPT provides information based on text from the internet, it does not have the ability to critically evaluate and understand the credibility of theories. However, it is possible that students will be able to build their knowledge independently simply by using their interactions with ChatGPT. It should be noted that there is no guarantee that the knowledge they build will be justified true belief. Therefore, the presence of ChatGPT cannot provide justification of truth in the didactic tetrahedron concept. Because the processes of diffusion and acquisition cannot be fulfilled. Its role in didactics remains and will always be as a tool that, if substituted into the didactic triangle, is likely to be on the coordinates within the pedagogical didactic anticipation (ADP) line.
Several factors reinforce that ChatGPT cannot stand independently in the didactic concept: (1) ChatGPT relies on testimonial information taken from the internet, which includes various sources, both reliable and unreliable (Lund and Wang, 2023). It does not have the ability to distinguish between the two. This limitation poses the risk of spreading false information or unverified claims, as ChatGPT lacks the characteristics of critical evaluation skills; (2) ChatGPT, on the other hand, does not have the ability to ask targeted and in-depth questions that stimulate critical thinking and encourage individuals to reflect on their own perspectives (Bishop, 2023; Yu, 2023); (3) ChatGPT does not have the capacity to engage in dialectical exchanges and refine concepts collaboratively with students (Loos et al., 2023); (4) ChatGPT, as an AI language model, does not have the capacity to guide students in the same way, limiting the depth of critical self-reflection (Loos et al., 2023); (5) ChatGPT cannot engage in inductive reasoning processes, which are essential for developing high-level thinking skills (Echenique, 2023); (6) ChatGPT cannot engage in dialectical exchanges or provide the same level of challenge and intellectual development (Echenique, 2023).
4 Conclusion
In comparing the mathematical performance among three groups of students, significant differences in mathematical performance were observed. The group that solely relied on ChatGPT achieved the lowest average scores, with statistical results indicating a difference from the other two groups. In contrast, the group receiving instruction with the assistance of ChatGPT and the group receiving instruction solely from the instructor both achieved higher average scores, with no statistical difference in their mathematical performance. Therefore, this research underscores that the use of ChatGPT as a teaching tool in mathematics instruction has the potential to enhance student performance, but the role of the instructor remains crucial in delivering in-depth instruction. While ChatGPT can provide information, explanations, and support, the combination of human expertise and ChatGPT’s capabilities holds greater potential for improving students’ understanding of mathematics.
As a form of “extended cognition,” the utilization of ChatGPT in mathematics education urgently needs development to ensure its optimal and appropriate use. The popularity of ChatGPT has reached significant levels, prompting researchers and developers to prioritize efforts in ensuring its effectiveness in the learning process rather than focusing solely on marketing aspects to attract students’ interest. The findings from this research show that students have a positive perception of ChatGPT’s use in learning and enhancing their understanding of mathematical concepts. They view ChatGPT as a valuable tool for assisting them in composing papers or essays related to mathematics and understanding complex concepts. However, concerns exist regarding excessive dependence on ChatGPT, which could diminish students’ ability to solve problems independently. There is a dual perspective among students, with some considering ChatGPT a valuable asset, while others worry about ethical implications and potential hindrances to intellectual development. Despite these concerns, students recognize the value of ChatGPT as a learning aid but emphasize the importance of its controlled use by instructors and mature ethical considerations.
The incorporation of ChatGPT does not provide valid justification for the didactical tetrahedron in the context of didactics. Although ChatGPT has the potential to provide information and answer questions, several reasons indicate that ChatGPT cannot independently stand as a valid component in didactic contexts. First, ChatGPT relies on information from the internet without the ability to distinguish between reliable and unreliable sources, potentially spreading inaccurate or unverified information. This limitation does not align with the principle of strong justification in building knowledge. Second, ChatGPT cannot pose deep questions that stimulate critical thinking and cannot engage in dialectical exchanges that promote reflection and intellectual development. Third, ChatGPT lacks the capacity to guide and provide human-like guidance to students, which is necessary for developing deep understanding and high-level thinking skills. Fourth, ChatGPT cannot engage in inductive reasoning, which is crucial for developing critical thinking skills. Therefore, while the use of ChatGPT as a teaching aid in mathematics education, supplemented by instructor guidance, is worthy of further investigation, its use in the learning process cannot validly replace the role of instructors or deep and reflective human interactions in constructing meaningful knowledge. Consequently, the inclusion of ChatGPT cannot provide valid justification for the didactical tetrahedron in the didactic context.
The findings of this research carry significant implications for teaching practices in university settings. Instructors continue to play a pivotal role in delivering in-depth and personalized mathematics instruction. While ChatGPT can offer supplementary support, human-led instruction remains irreplaceable in providing contextual explanations, discussing complex concepts, and stimulating questions and discussions. Overall, the use of ChatGPT as a teaching aid in mathematics instruction demonstrates significant potential for enhancing student performance. However, this research also emphasizes that the use of ChatGPT needs to be integrated with deep instructor-led instruction to be effective. Future research can explore the development of more advanced language models, optimization strategies for using ChatGPT in education, and the specific roles of instructors in leveraging this teaching aid effectively.
Data availability statement
The original contributions presented in the study are included in the article/supplementary material, further inquiries can be directed to the corresponding author.
Ethics statement
Ethical approval was not required for the studies involving humans because this type of research, in Indonesia, there are no ethical committee available yet. The ethical committee is commonly used in medical research. The studies were conducted in accordance with the local legislation and institutional requirements. The participants provided their written informed consent to participate in this study. Written informed consent was obtained from the individual(s) for the publication of any potentially identifiable images or data included in this article.
Author contributions
DD: Writing – original draft, Formal analysis. AH: Writing – original draft, Conceptualization, Data curation. SS: Resources, Software, Writing – original draft. DS: Formal analysis, Supervision, Validation, Writing – review & editing. LM: Project administration, Software, Writing – original draft. TC: Writing – review & editing, Supervision. LF: Project administration, Resources, Writing – review & editing.
Funding
The author(s) declare financial support was received for the research, authorship, and/or publication of this article. Several authors have received educational scholarships from the Indonesian government through the Education Fund Management Institution (LPDP). This research is partially funded by the LPDP.
Acknowledgments
The authors would like to express their gratitude to the Education Fund Management Institution (Lembaga Pengelola Dana Pendidikan—LPDP) under Ministry of Finance of the Republic of Indonesia as sponsor for their Masters’ and Doctoral studies. Authors also would like to express their sincere gratitude to all individuals and institutions whose support and contributions made this research possible.
Conflict of interest
The authors declare that the research was conducted in the absence of any commercial or financial relationships that could be construed as a potential conflict of interest.
Publisher’s note
All claims expressed in this article are solely those of the authors and do not necessarily represent those of their affiliated organizations, or those of the publisher, the editors and the reviewers. Any product that may be evaluated in this article, or claim that may be made by its manufacturer, is not guaranteed or endorsed by the publisher.
References
Adiguzel, T., Kaya, M. H., and Cansu, F. K. (2023). Revolutionizing education with AI: exploring the transformative potential of ChatGPT. Contemp. Educ. Technol. 15, 1–13. doi: 10.30935/cedtech/13152
Alam, A. (2022). Employing adaptive learning and intelligent tutoring robots for virtual classrooms and smart campuses: reforming education in the age of artificial intelligence. In Advanced computing and intelligent technologies: Proceedings of ICACIT 2022 (pp. 395–406). Springer.
Aldon, G., Cusi, A., Schacht, F., and Swidan, O. (2021). Teaching mathematics in a context of lockdown: A study focused on teachers’ praxeologies. Educ. Sci. 11, 1–21. doi: 10.3390/educsci11020038
Alneyadi, S., Abulibdeh, E., and Wardat, Y. (2023). The Impact of Digital Environment vs. Traditional Method on Literacy Skills; Reading and Writing of Emirati Fourth Graders. Sustainability, 15:3418.
Apostolopoulos, I. D., Tzani, M., and Aznaouridis, S. I. (2023). ChatGPT: ascertaining the self-evident. The use of AI in generating human knowledge. Comput. Therm. Sci. 1, 1–20. doi: 10.48550/arXiv.2308.06373
Aydın, Ö., and Karaarslan, E. (2023). Is ChatGPT leading generative AI? What is beyond expectations? Acad. Platform J. Engineer. Smart Syst. 11, 118–134. doi: 10.21541/apjess.1293702
Baidoo-Anu, D., and Owusu Ansah, L. (2023). Education in the era of generative artificial intelligence (AI): understanding the potential benefits of chatgpt in promoting teaching and learning. J. AI 7, 52–62. doi: 10.61969/jai.1337500
Biswas, S. S. (2023). Role of Chat GPT in Public Health. Ann Biomed Eng. 51, 868–869. doi: 10.1007/s10439-023-03172-7
Bosch, M. (2015). Doing research within the anthropological theory of the didactic: the case of school algebra. In Selected regular lectures from the 12th international congress on mathematical education (pp. 51–69).
Brinkley-Etzkorn, K. E. (2018). Learning to teach online: measuring the influence of faculty development training on teaching effectiveness through a TPACK lens. Internet High. Educ. 38, 28–35. doi: 10.1016/j.iheduc.2018.04.004
Brousseau, G. (1997). Theory of didactical situations in mathematics. Dordrecht: Kluwer Academic Publishers.
Cao, Y., Zhang, S., Chan, M. C. E., and Kang, Y. (2021). Post-pandemic reflections: lessons from Chinese mathematics teachers about online mathematics instruction. Asia Pac. Educ. Rev. 22, 157–168. doi: 10.1007/s12564-021-09694-w
Coskun, H. (2023). The power of ChatGPT: the breakthrough role of the language model in engineering education. Innovative Res. Engineer. 71–100. doi: 10.59287/ire.507
Creswell, J. W. (2012). Educational research: planning, conducting, and evaluating quantitative and qualitative research. Educ. Res. 4:237.
Deiana, G., Dettori, M., Arghittu, A., Azara, A., Gabutti, G., and Castiglia, P. (2023). Artificial intelligence and public health: evaluating chat GPT responses to vaccination myths and misconceptions. Vaccine 11, 1–13. doi: 10.3390/vaccines11071217
Dieronitou, I. (2014). The ontological and epistemological foundations of qualitative and quantitative approaches to research. Int. J. Econ., Commerce and Manag. United II, 1–17.
Echenique, C. R. (2023). Education in Latin America, what could we do? Revista Boletín Redipe 12, 37–60. doi: 10.36260/rbr.v12i4.1951
Elbanna, S., and Armstrong, L. (2023).Exploring the integration of ChatGPT in education: adapting for the future. Management & Sustainability: an Arab Review.
Eriksson, H., and Larsson, N. (2023). Chatting up the grade an exploration on the impact of ChatGPT on self-study experience in higher education (Umeå University). Umeå University. Retrieved from https://urn.kb.se/resolve?urn=urn:nbn:se:umu:diva-211263%0A
Fischer, E., and Guzel, G. T. (2023). The case for qualitative research. J. Consum. Psychol. 33, 259–272. doi: 10.1002/jcpy.1300
Freudenthal, H. (1991). Revisiting mathematics education: China lectures. Dordrecht: Kluwer Academic Publishers.
Gautam, A., Chirputkar, A., and Pathak, P. (2022). Opportunities and challenges in the application of artificial intelligence-based technologies in the healthcare industry. International interdisciplinary humanitarian conference for sustainability, IIHC 2022- proceedings, 18(1), 1521–1524. MDPI.
Geis, J. R., Brady, A., Wu, C. C., Spencer, J., Ranschaert, E., Jaremko, J. L., et al. (2019). Ethics of artificial intelligence in radiology: summary of the joint European and north American multisociety statement. Insights Imag 10, 101–440. doi: 10.1186/s13244-019-0785-8
Ghavifekr, S., and Rosdy, W. A. W. (2015). Teaching and learning with technology: effectiveness of ICT integration in schools. Int. J. Res. Educ. Sci. 1, 175–191. doi: 10.21890/ijres.23596
Gong, B., Nugent, J. P., Guest, W., Parker, W., Chang, P. J., Khosa, F., et al. (2019). Influence of artificial intelligence on Canadian medical students’ preference for radiology specialty: A national survey study. Acad. Radiol. 26, 566–577. doi: 10.1016/j.acra.2018.10.007
Gupta, A., and Elby, A. (2011). Beyond epistemological deficits: dynamic explanations of engineering students’ difficulties with mathematical sense-making. Int. J. Sci. Educ. 33, 2463–2488. doi: 10.1080/09500693.2010.551551
Haleem, A., Javaid, M., and Singh, R. P. (2022). An era of ChatGPT as a significant futuristic support tool: A study on features, abilities, and challenges. Bench Council Transactions on Benchmarks, Standards and Evaluations 2:100089. doi: 10.1016/j.tbench.2023.100089
Hassan, A. M., Nelson, J. A., Coert, J. H., Mehrara, B. J., and Selber, J. C. (2023). Exploring the Potential of Artificial Intelligence in Surgery: Insights from a Conversation with ChatGPT. Annals of surgical oncology 30, 3875–3878.
Hassani, H., and Silva, E. S. (2023). The role of chatGPT in data science: how AI-assisted conversational interfaces are revolutionizing the field. Big Data and Cognitive Computing 7:62. doi: 10.3390/bdcc7020062
Heggart, K. R., and Yoo, J. (2018). Getting the most from google classroom: A pedagogical framework for tertiary educators. Australian J. Teacher Educ. 43, 140–153. doi: 10.14221/ajte.2018v43n3.9
Javaid, M., Haleem, A., and Singh, R. P. (2023). ChatGPT for healthcare services: an emerging stage for an innovative perspective. BenchCouncil Transactions on Benchmarks, Standards and Evaluations 3:100105. doi: 10.1016/j.tbench.2023.100105
Jeon, J., and Lee, S. (2023). Large language models in education: A focus on the complementary relationship between human teachers and ChatGPT. Educ. Inf. Technol. 28, 15873–15892. doi: 10.1007/s10639-023-11834-1
Jukić Matić, L., and Glasnović Gracin, D. (2016). Das Schulbuch als Artefakt in der Klasse: Eine Studie vor dem Hintergrund des soziodidaktischen Tetraeders. J. Fur Mathematik-Didaktik 37, 349–374. doi: 10.1007/s13138-016-0091-7
Kasneci, E., Sessler, K., Küchemann, S., Bannert, M., Dementieva, D., Fischer, F., et al. (2023). ChatGPT for good? On opportunities and challenges of large language models for education. Learn. Individ. Differ. 103:102274. doi: 10.1016/j.lindif.2023.102274
Kirk, R. E. (2009). Experimental design. Sage handbook of quantitative methods in psychology, 23–45.
Langer, J. A. (2011). Envisioning knowledge: Building literacy in the academic disciplines. New York: Teachers College Press.
Lecler, A., Duron, L., and Soyer, P. (2023). Revolutionizing radiology with GPT-based models: current applications, future possibilities and limitations of ChatGPT. Diagn. Interv. Imaging 104, 269–274. doi: 10.1016/j.diii.2023.02.003
Lee, C. J. G. (2012). Reconsidering constructivism in qualitative research. Educ. Philos. Theory 44, 403–412. doi: 10.1111/j.1469-5812.2010.00720.x
Lo, C. K. (2023). What is the impact of ChatGPT on education? A rapid review of the literature. Educ. Sci. 13:410. doi: 10.3390/educsci13040410
Loos, E., Gröpler, J., and Goudeau, M. L. S. (2023). Using ChatGPT in education: human reflection on ChatGPT’s self-reflection. For. Soc. 13, 1–18. doi: 10.3390/soc13080196
Lund, B. D., and Wang, T. (2023). Chatting about ChatGPT: how may AI and GPT impact academia and libraries? Library Hi Tech News 40, 26–29. doi: 10.1108/LHTN-01-2023-0009
Marshall, P. A., Adebamowo, C. A., Adeyemo, A. A., Ogundiran, T. O., Vekich, M., Strenski, T., et al. (2006). Voluntary participation and informed consent international genetic research. Am. J. Public Health 96, 1989–1995. doi: 10.2105/AJPH.2005.076232
Mattas, P. S. (2023). ChatGPT: A study of AI language processing and its implications. Int. J. Res. Public. Rev. 4, 435–440. doi: 10.55248/gengpi.2023.4218
Mhlanga, D. (2023). Open AI in education, the responsible and ethical use of chatGPT towards lifelong learning. SSRN Electron. J. doi: 10.2139/ssrn.4354422
Mitrović, S., Andreoletti, D., and Ayoub, O. (2023). ChatGPT or human? Detect and explain. Explaining decisions of machine learning model for detecting short ChatGPT-generated text. Comput. Therm. Sci. 1, 1–11. doi: 10.48550/arXiv.2301.13852
Novita, R., and Herman, T. (2021). Using technology in young children mathematical learning: A didactic perspective. J. Phys. Conf. Ser. 1957, 012013–012010. doi: 10.1088/1742-6596/1957/1/012013
Olive, J., Makar, K., Hoyos, V., Kor, L. K., Kosheleva, O., and Sträßer, R. (2010). Mathematical knowledge and practices resulting from access to digital technologies. New ICMI study series 13, 133–177. doi: 10.1007/978-1-4419-0146-0_8
Oviedo-Trespalacios, O., Peden, A. E., Cole-Hunter, T., Costantini, A., Haghani, M., Rod, J. E., et al. (2023). The risks of using Chatgpt to obtain common safety-related information and advice. SSRN Electron. J. 167:106244. doi: 10.2139/ssrn.4370050
Owens, T. J. (1950). Socratic method and critical philosophy: selected essays. Thought 25, 153–154. doi: 10.5840/thought195025134
Pavlik, J. V. (2023). Collaborating with ChatGPT: considering the implications of generative artificial intelligence for journalism and media education. Journalism and Mass Communication Educator 78, 84–93. doi: 10.1177/10776958221149577
Pedró, F. (2019). Artificial intelligence in education: challenges and opportunities for sustainable development. Unesco, 46. Retrieved from https://en.unesco.org/themes/education-policy-
Phuong, T. T., Cole, S. C., and Zarestky, J. (2018). A systematic literature review of faculty development for teacher educators. High. Educ. Res. Dev. 37, 373–389. doi: 10.1080/07294360.2017.1351423
Prediger, S., Roesken-Winter, B., and Leuders, T. (2019). Which research can support PD facilitators? Strategies for content-related PD research in the three-tetrahedron model. J. Math. Teach. Educ. 22, 407–425. doi: 10.1007/s10857-019-09434-3
Rahman, M. S. (2016). The advantages and disadvantages of using qualitative and quantitative approaches and methods in language “testing and assessment” research: A literature review. J. Educ. Learn. 6:102. doi: 10.5539/jel.v6n1p102
Ray, P. P. (2023). ChatGPT: A comprehensive review on background, applications, key challenges, bias, ethics, limitations and future scope. Internet of Things and Cyber-Physical Systems 3, 121–154. doi: 10.1016/j.iotcps.2023.04.003
Rees, T. (2022). Non-human words: on GPT-3 as a philosophical laboratory. Daedalus 151, 168–182. doi: 10.1162/DAED_a_01908
Rehana, H., Çam, N. B., Basmaci, M., He, Y., Özgür, A., and Hur, J. (2023). Evaluation of GPT and BERT-based models on identifying protein-protein interactions in biomedical text. ArXiv Preprint ArXiv 10, 1–25. doi: 10.48550/arXiv.2303.17728
Remillard, J. T., and Heck, D. J. (2014). Conceptualizing the curriculum enactment process in mathematics education. ZDM-Math. Educ. 46, 705–718. doi: 10.1007/s11858-014-0600-4
Rezat, S., and Sträßer, R. (2012). From the didactical triangle to the socio-didactical tetrahedron: artifacts as fundamental constituents of the didactical situation. ZDM-Math. Educ. 44, 641–651. doi: 10.1007/s11858-012-0448-4
Ruthven, K. (2009). Towards a naturalistic conceptualisation of technology integration in classroom practice: the example of school mathematics. Éducation et Didactique 9, 131–159. doi: 10.4000/educationdidactique.434
Ruthven, K. (2012). The didactical tetrahedron as a heuristic for analysing the incorporation of digital technologies into classroom practice in support of investigative approaches to teaching mathematics. ZDM-Math. Educ. 44, 627–640. doi: 10.1007/s11858-011-0376-8
Safdar, N. M., Banja, J. D., and Meltzer, C. C. (2020). Ethical considerations in artificial intelligence. Eur. J. Radiol. 122:108768. doi: 10.1016/j.ejrad.2019.108768
Shaikh, S., Yayilgan, S. Y., Klimova, B., and Pikhart, M. (2023). Assessing the usability of ChatGPT for formal English language learning. European J. Investigation in Health, Psychol. Educ. 13, 1937–1960. doi: 10.3390/ejihpe13090140
Singh, J., Steele, K., and Singh, L. (2021). Combining the best of online and face-to-face learning: hybrid and blended learning approach for covid-19, post vaccine, & post-pandemic world. J. Educ. Technol. Syst. 50, 140–171. doi: 10.1177/00472395211047865
Sit, C., Srinivasan, R., Amlani, A., Muthuswamy, K., Azam, A., Monzon, L., et al. (2020). Attitudes and perceptions of UK medical students towards artificial intelligence and radiology: a multicentre survey. Insights Imag 11, 14–16. doi: 10.1186/s13244-019-0830-7
Steinbring, H. (2005). Analyzing mathematical teaching-learning situations - the interplay of communicational and epistemological constraints. Educ. Stud. Math. 59, 313–324. doi: 10.1007/s10649-005-4819-4
Straesser, R. (2007). Didactics of mathematics: more than mathematics and school! ZDM-Int. J. Mathematics Educ. 39, 165–171. doi: 10.1007/s11858-006-0016-x
Sun, G. H., and Hoelscher, S. H. (2023). The ChatGPT storm and what faculty can do. Nurse Educ. 48, 119–124. doi: 10.1097/NNE.0000000000001390
Tall, D. (1986). Using the computer as an environment for building and testing mathematical concepts: a tribute to richard skemp. Honour of Richard Skemp, 21–36.
Tlili, A., Shehata, B., Adarkwah, M. A., Bozkurt, A., Hickey, D. T., Huang, R., et al. (2023). What if the devil is my guardian angel: ChatGPT as a case study of using chatbots in education. Smart Learn. Environ. 10, 1–10. doi: 10.1186/s40561-023-00237-x
To, J., and Carless, D. (2016). Making productive use of exemplars: peer discussion and teacher guidance for positive transfer of strategies. J. Furth. High. Educ. 40, 746–764. doi: 10.1080/0309877X.2015.1014317
Troussas, C., Krouska, A., and Virvou, M. (2020). Using a multi module model for learning analytics to predict learners’ cognitive states and provide tailored learning pathways and assessment. Intelligent systems reference library 158, 9–22. doi: 10.1007/978-3-030-13743-4_2
Tuli, F. (2011). The basis of distinction between qualitative and quantitative research in social science: reflection on ontological, epistemological and methodological perspectives. Ethiopian J. Educ. Sci. 6, 97–108. doi: 10.4314/ejesc.v6i1.65384
Vincent-Lancrin, S., and van der Vlies, R. (2020). Trustworthy artificial intelligence (AI) in education: promises and challenges. OECD education working papers, No. 218. In OECD Publishing. France.
Vygotsky, L. S. (1978). Mind in society: The development of higher psychological processes. Cambridge: Harvard University Press.
Wardat, Y., Tashtoush, M. A., AlAli, R., and Jarrah, A. M. (2023). ChatGPT: A revolutionary tool for teaching and learning mathematics. Eurasia J. Math. Sci. Technol. Educ. 19, em2286–em2218. doi: 10.29333/ejmste/13272
Wittmann, J. (2023). Science fact vs science fiction: A ChatGPT immunological review experiment gone awry. Immunol. Lett. 256-257, 42–47. doi: 10.1016/j.imlet.2023.04.002
Yang, Y. T. C., and Wu, W. C. I. (2012). Digital storytelling for enhancing student academic achievement, critical thinking.; learning motivation: A year-long experimental study. Comp. Educ. 59, 339–352. doi: 10.1016/j.compedu.2011.12.012
Yu, H. (2023). Reflection on whether chat GPT should be banned by academia from the perspective of education and teaching. Front. Psychol. 14, 1–12. doi: 10.3389/fpsyg.2023.1181712
Keywords: ChatGPT, didactics, didactical tetrahedron, learning, teaching
Citation: Dasari D, Hendriyanto A, Sahara S, Suryadi D, Muhaimin LH, Chao T and Fitriana L (2024) ChatGPT in didactical tetrahedron, does it make an exception? A case study in mathematics teaching and learning. Front. Educ. 8:1295413. doi: 10.3389/feduc.2023.1295413
Edited by:
Kingsley Okoye, Monterrey Institute of Technology and Higher Education (ITESM), MexicoReviewed by:
James Harold Davenport, University of Bath, United KingdomYousef Wardat, Higher Colleges of Technology, United Arab Emirates
Copyright © 2024 Dasari, Hendriyanto, Sahara, Suryadi, Muhaimin, Chao and Fitriana. This is an open-access article distributed under the terms of the Creative Commons Attribution License (CC BY). The use, distribution or reproduction in other forums is permitted, provided the original author(s) and the copyright owner(s) are credited and that the original publication in this journal is cited, in accordance with accepted academic practice. No use, distribution or reproduction is permitted which does not comply with these terms.
*Correspondence: Dadan Dasari, dadan.dasari@upi.edu