- 1Department of Educational Psychology, Institute of Psychology, Johannes Gutenberg University, Mainz, Germany
- 2School of Communication and Journalism, Auburn University, Auburn, AL, United States
Emergency Remote Teaching (ERT) was introduced around the globe during the COVID-19 pandemic to ensure that students could continue with their studies during social distancing. Subsequent studies found considerable individual differences in the adjustment to ERT and identified resilience as a critical factor for coping with the challenges of independent studying. The growing evidence led to calls for resilience training in preparation for emergency situations. Against the backdrop of a three-phase model of self-regulated learning, this study examines self-regulated learning activities as an additional protective resource and a predictor for academic performance in ERT. Results from a survey of resilience, self-regulated learning strategies, and competence gain completed by students from universities in the US and Germany (N = 333) found self-regulated learning strategies to be more predictive of student competence gain than resilience. As a consequence, in addition to fostering resilience, institutions should also include self-regulation strategies in student training and support programs to better prepare students for academic success.
1 Introduction
The COVID-19 pandemic forced universities worldwide to transition to emergency remote teaching (ERT) on extremely short notice. ERT was perceived to be dramatically different from online learning (Hodges et al., 2020) as there was little time to prepare, teachers and students faced both academic challenges as well as public and personal health uncertainties. The impact of the crisis on the mental health and the psychological well-being of students quickly became a topic of interest to scholars (e.g., Sood and Sharma, 2020; Forycka et al., 2022). Early studies found that student concerns often focused less on contracting a COVID infection and more on the uncertainties resulting from changes in the traditional academic environment (see Hoofman and Secord, 2021, for an early review). These studies consistently reported mental health challenges for higher education students following the switch to ERT, including higher levels of academic stress and anxiety, the loss of cooperative learning networks, as well as depression symptoms, loneliness, and socio-economic concerns (Elmer et al., 2020; Sarasjärvi et al., 2022; Versteeg et al., 2022; Laranjeira et al., 2023; Lin et al., 2023; Salimi et al., 2023).
As the pandemic persisted, questions of how remote teaching and learning affected student study behaviors and learning outcomes arose (Gonzalez et al., 2020; Paetsch and Drechsel, 2021; Weidlich and Kalz, 2021; Imhof, 2022; Pertegal-Felices et al., 2022). At the same time, researchers sought to identify and better understand factors that may play a protective role in addressing the stressors students were experiencing (Sood and Sharma, 2020; Ye et al., 2020; Versteeg et al., 2022). These studies found considerable individual differences in the adjustment to ERT and identified resilience as a critical factor for coping with the challenges of independent studying (de la Fuente et al., 2021). The present study extends this research, examining the role and potential benefit of self-regulated learning activities on academic competence gain in an ERT context.
2 Literature review
2.1 Resilience
The level of resilience has been found to determine the ability to adapt to changing demands and to maintain well-being and psychological functioning. As a comprehensive construct used to explain differential perceptions and behavior in difficult situations, it encompasses a set of “personal qualities that enable one to thrive in the face of adversity” (Connor and Davidson, 2003, p. 76). In short, resilience is the ability to bounce back from stressful experiences and includes confidence in one’s ability to solve novel problems, optimism in the face of challenges, commitment to goals, a realistic sense of control, and tolerance of difficulties and negative affect (Connor and Davidson, 2003; Robbins et al., 2018). While individuals may face similar negative experiences, their ability to adapt to adversity differs. Previous studies suggest that resilience factors are predictive of the experienced levels of stress (see Reyes et al., 2015; San Román-Mata et al., 2020).
In ordinary times, the move to university and college life and studies is challenging for students. How they respond to the resulting stress has been linked to academic performance as well as a range of social and psychological factors (McPherson, 2012). Reviews of the resilience literature have highlighted its importance in helping students cope with academic pressures and study (Caruana et al., 2011), adapt to and recover from adverse and stressful situations in higher education (Fuller et al., 2016), as well as rise above challenges, manage personal wellbeing, and meet academic goals (Hartley, 2011; Reyes et al., 2015). In higher education, resilience has been identified as a factor affecting student engagement, persistence, and learning (Ahmed et al., 2018; Ayala and Manzano, 2018; Sood and Sharma, 2020).
The advent of the pandemic and the subsequent pivot to ERT was a major stressor for both faculty and students. In most instances, the transition to ERT was abrupt, with immediate educational goals and solutions prioritized over course design. At the same time, the transition frequently outstripped the ability of campus personnel to provide needed support to faculty and students. Among the many questions asked at the time – by parents, faculty, higher education institutions, mental health practitioners and others: how well were students coping with these stressors, what effect did the challenges and uncertainties have on student learning, and, what factors may mediate learning outcomes (Elmer et al., 2020; Kunzler et al., 2021).
Given the understandable concern of the effects of the crisis on student progress and mental health, numerous researchers began investigating the role of resilience on student behavior and success. The findings of pandemic era studies found resilience to be related to student mental well-being, academic success, and study behavior (Polizzi et al., 2020; Sood and Sharma, 2020; Ye et al., 2020; Ang et al., 2021; Nandy et al., 2021; Versteeg et al., 2022) – findings that were repeated across cultures and countries (e.g., Australia, Poland, Ecuador; Eri et al., 2021; Forycka et al., 2022; Pertegal-Felices et al., 2022). Investigations across several cultures identified low level of resilience as a risk factor for dysfunctional study behavior and dropping out of higher education (Eri et al., 2021; Forycka et al., 2022; Jehi et al., 2022; Pertegal-Felices et al., 2022).
Drawing on findings that identified intrinsic (e.g., desire to succeed, motivation) and extrinsic factors (e.g., friendships, family, teachers) factors enhancing resilience, Ang et al. (2021) recommended developing resilience training programs that take a socio-ecological approach to support gaining and maintaining a positive mindset and coping skills. More specifically, they explored the role that schools can play in developing resilience education courses for students. Based on the results of their school-based intervention programs, they concluded that such programs can provide students with the necessary skills to successfully meet academic challenges.
The organizational perspective on resilience building emphasizes the role of the institution and organizational leadership for navigating crisis and uncertainty (Nandy et al., 2021). Similar to personal resilience, organizational resilience emphasizes an organization’s “ability to anticipate potential threats, to cope effectively with adverse events, and to adapt to changing conditions” (Duchek, 2020, p. 220). Early studies of organizational resilience centered on identifying a range of “resources, behaviors, strategies, and processes that may enhance an organization’s resilience” (Duchek, 2020, p. 221), among them are improvisation and respectful interaction (Weick and Sutcliffe, 2015), sufficient and available resources (Mallak, 1998; Välikangas and Romme, 2013), redundancy (Kendra and Wachtendorf, 2003), and positive relationships (Gittell et al., 2006).
From this perspective, as large organizations, institutions of higher education are responsible for creating learning and work environments that provide a safe framework for individual members to tackle the difficulties that arise during times of crisis. At the onset of the COVID-19 pandemic, many researchers conducted literature reviews seeking to identify positive coping strategies. For example, Polizzi et al. (2020), reviewing studies conducted following natural disasters and other traumatic crises (e.g., earthquakes, floods, hurricanes, terrorist attacks), recommended a set of measures to strengthen resilience and enhance physical and mental well-being, among them: carrying on with positive activities, observing mindfulness and appreciation, keeping connected and taking good care of social contacts through digital media, and working toward acceptance-based coping, including planning ahead for the post-crisis period.
As the review above suggests, student resilience is important to educational performance and faculty and academic institutions can help foster that success (Gillham et al., 2013). One means of building student resilience in traditional academic settings is through the use classroom learning activities – activities students found to be significantly curtailed or missing as higher education institutions turned to ERT.
Notably, few empirical studies have examined both resilience and the role of the learning environment on student competence gain. It is plausible that with reduced access to the learning environment, student self-regulation competence would become even more critical for learning and academic success. Thus, this study investigates resilience and the potential impact of self-regulated learning in the wake of ERT. Study results may help identify program elements and design features academic institution decision makers should utilize when developing programs aimed at strengthening learner resilience.
2.2 Self-regulated learning
An important factor that affects student outcomes and success is student self-regulated learning (SRL). Even prior to the pandemic, SRL has long been acknowledged a key component of successful learning in both higher education (Travis and Bunde, 2020) and digital learning and distance learning (see Theobald, 2021). Self-regulation is a self-directed process, rather than a personality trait or a mental ability (Zimmerman, 2002). Pintrich (2000) defined SRL as “an active, constructive process whereby learners set goals for their learning and then attempt to monitor, regulate, and control their cognition, motivation, and behavior, guided and constrained by their goals and the contextual features in the environment” (p. 453). SRL occurs when learners assess their learning environment and processes, then use those assessments to adequately plan, subsequently modify, and actively manage their learning practices. SRL is typically described as a process involving cognitive (e.g., rehearsal, organization of material), metacognitive (e.g., goalsetting and monitoring), and motivational processes (e.g., raising value of a task, increasing perceptions of self-efficacy, introducing strategies supporting persistence in learning). Activities are cyclical in nature. They begin with a preparatory or pre-actional phase, move to the actional phase of actual handling with the materials and tasks, and conclude in the post-actional phase which contains self-evaluation, reflection, and revised goal setting (Winne, 2018).
Studies of SRL with students in higher education have found that SRL competences positively predict engagement and performance in online courses (Kizilcec et al., 2017; Theobald, 2021; Jansen et al., 2022). Moreover, students who were successful in these courses persistently demonstrated a high level of SRL behaviors in the areas of metacognition and resource management (Broadbent and Poon, 2015).
While there is accumulated evidence for the beneficial effects of SRL competences on academic achievement (Broadbent and Poon, 2015; Anthonysamy et al., 2020), research also suggests that a large proportion of students struggle with them (Alemayehu and Chen, 2021) and lack the ability to employ them efficiently. As a consequence, many institutions have established programs for beginning students to build study skills and SRL competences during the initial phases of their career in higher education (van der Zanden et al., 2018).
The abrupt transition to ERT raised important questions about the role of SRL in student coping. The crisis challenged the SRL competences of all students to a degree that might have been, in many cases, more comprehensive than before. Students had to review the practices and habits which they typically employed, and they were pushed to come up with adaptive strategies. In this context, it is plausible to assume that the more demanding aspects of SRL strategies (e.g., metacognition and resource management) might be more affected by the preoccupation with the uncertainty of the general situation than other, less complex SRL strategies (e.g., cognitive strategies, such as organizing information).
2.3 Student competences and competence gain
Outcomes of higher education involve the development of declarative knowledge and thinking skills (e.g., general content knowledge and domain specific knowledge, complex thinking, problem-solving skills) and non-cognitive outcomes (e.g., attitudes and values, motivational competences, academic self-concept, social skills). In higher education, these outcomes are typically demonstrated and measured in terms of knowledge, skills, and competences.1 In most cases, assessment of these learning outcomes is framed by subject-specific practices which rarely allow for cross-subject comparisons. In addition, both the format and content of teacher-made and course-specific tests, the most common form of assessment, have been criticized for not reflecting actual competences and student learning, particularly in cases where long-term and non-cognitive outcomes were of interest (Rychen and Salganik, 2003).
As an alternative way of assessing student competences, researchers have explored students’ ability to self-report and self-assess their own learning and the effect of these self-evaluations on student learning motivation and areas of non-cognitive learning (e.g., academic self-efficacy, academic self-concept, and professional development; Panadero et al., 2017). Panadero et al. (2017) defined self-assessment as a “… wide variety of mechanisms and techniques through which students describe (i.e., assess) and possibly assign merit or worth to (i.e., evaluate) the qualities of their own learning processes and products” (p. 75). Self-assessment is viewed as a central element of formative assessment because it is the student who “…‘close[s] that gap’ between a current performance (as revealed by assessment) and the desired standard” (Panadero et al., 2017, p. 76).
Regarding self-assessment of student achievement, the literature suggests that in spite of occasional errors, the validity of self-reported learning estimate can be assumed, provided that the design features of the questionnaires are based in theory and carefully validated (Braun et al., 2012). Braun et al. (2008) identified six areas of competency that represent student learning in a generic manner. The claim is that learning occurs in the areas of knowledge processing (e.g., subject specific facts and problems), systematic competences (e.g., information search), presentational competence (e.g., giving talks), communication competence (e.g., expressing one’s opinion), cooperation competence (e.g., contributing to group work), and personal competence (e.g., identification with the subject). It is reasonable to expect that ERT would not support student learning equally well in all of the areas listed above and that some areas are more susceptible to being negatively affected by ERT than others. Early studies on the effects of ERT on student learning suggest that there is a positive effect on students’ performance in terms of knowledge processing and subject-specific competences due to the confinement (Gonzalez et al., 2020).
2.4 Transnationally shared challenges
As the pandemic unfolded, countries around the globe imposed lockdowns and contact restrictions (Gupta, 2020; Nurunnabi et al., 2020). The resulting isolation resulted in profound changes to the psychosocial environment of these countries. In education, schools were closed and ERT was imposed. Around the world, both teachers and students were challenged by the necessity to rapidly transition to digital learning and teaching (Kizilcec et al., 2017). The global character of the pandemic and the similarity of the measures that were taken worldwide was unprecedented. However, countries and institutions were not equally prepared in terms of digital infrastructure, availability of electronic devices and software, and expertise in handling a large-scale crisis (Eri et al., 2021).
Researchers examined a variety of individual and cultural differences and their effect on how individuals differentially experienced the pandemic. For example, the way in which academic and learning culture might be affected might vary depending on a country’s position on Hofstede’s (2001) individualism – collectivism dimension. This dimension of Hofstede’s cultural values framework addresses differences in group membership and integration. Cultures emphasizing individualism emphasize personal needs, rights, and achievement, while collectivistic cultures value relationships and loyalty. Kowal et al. (2020), examining perceived stress during the COVID-19 pandemic, surveyed participants from 26 countries. They reported that stress levels did not differ on Hofstede’s individualism – collectivism dimension. Using the 10 item Perceived Stress Scale (Cohen and Williamson, 1988) (0 = never to 4 = very often which would allow for a theoretical maximum sum score of 40), they reported that countries with the lowest stress levels were Switzerland (M = 13.93, SD = 6.66), Denmark (M = 14.22, SD = 7.21), and Finland (M = 14.85, SD = 7.50), countries with the highest reported stress levels were Turkey (M = 21.71, SD = 6.67), Poland (M = 20.19, SD = 7.28), and Japan (M = 20.08, SD = 5.74), with Germany (M = 16.52, SD = 6.87) and the United States (M = 17.59, SD = 7.36) in a range between the lower and upper limits (Kowal et al., 2020, p. 955). However, perceived stress did vary with biological sex and age, with women reporting higher levels of stress than men, and younger respondents reporting higher stress than those who were older.
2.5 The role of resilience and self-regulated learning for competence gain
Resilience and SRL are typically conceptualized as processes within a given context, where the availability of relevant competences is critical for coping with challenges and addressing tasks. As resilience is defined as the ability to recover following stress exposure (Versteeg et al., 2022), planned behavior should be an essential element, particularly in challenging times. Studies demonstrating a positive relationship between resilience and academic achievement support this assumption and suggest that a high level of resilience should protect students from disengagement, aid them in maintaining motivation, and foster learning (e.g., Ayala and Manzano, 2018; Polizzi et al., 2020; Sood and Sharma, 2020; Ang et al., 2021; Nandy et al., 2021; Versteeg et al., 2022).
In addition to planned behavior, the set of SRL competences that make self-regulated learning successful (i.e., the ability to monitor effort investment, to adjust one’s goals, and to show persistence when faced with unexpected challenges), may also contribute to the general adjustment processes in times of crisis. Strong study competences and other related SRL behavior would allow students to successfully facilitate their learning while navigating the challenges of a crisis. If true, self-regulatory strength, as indicated by SRL competences, may be predictive of student competence gain (Chung et al., 2022; Edisherashvili et al., 2022).
2.6 The present study
The purpose of the present study is to identify and disentangle the effects of resilience and SRL on student competence gain in ERT. We contend that resilience alone does not safeguard academic competence gain in ERT, but that SRL makes an independent contribution to academic achievement. Acknowledging the importance of resilience for academic persistence, we expect to find a higher level of competence gain for students with higher levels of resilience. In addition, we expect to see additional variance in competence gain explained by SRL competences.
For the purpose of this study, we focus on the areas of knowledge processing and personal competence on the most likely common denominator across the subjects and course types. Based on the previous review, this study is driven by the following research question:
RQ1: Are self-regulated learning and resilience predictive of self-rated competence gain in ERT?
The research question is broken down into the following hypotheses:
H1: Self-regulated learning will be positively related to competence gain. This hypothesis is based on the broad literature that demonstrated the beneficial role of SRL competences on academic success (Broadbent and Poon, 2015; Anthonysamy et al., 2020; Travis and Bunde, 2020; Theobald, 2021). For more detailed analyses, we look into the contributions of self-regulated learning activities in the three theoretically defined phases of the learning cycle (Zimmerman, 2002).
H1a: Goal setting and Planning and Time Management– as a set of SRL learning strategies in the pre-actional phase – will have a positive effect on competence gain.
H1b: Attention Allocation – as a SRL learning strategy in the actional phase – will have a positive effect on competence gain.
H1c: Adjustment – as a SRL learning strategy in the post-actional phase –will have a positive effect on competence gain.
H2: Resilience will be positively related to competence gain. This hypothesis is based in previous research supporting the role of resilience on academic success in general and in times of pandemic in particular (e.g., Ayala and Manzano, 2018; Polizzi et al., 2020; Sood and Sharma, 2020; Ye et al., 2020; Ang et al., 2021; Nandy et al., 2021; Versteeg et al., 2022).
3 Method
3.1 Sample
Participants were drawn from higher education students enrolled in a large university in the south-west of Germany and in the southeastern United States. The total sample consisted of 333 individuals with an average age of 21.67 years (SD = 2.84), of whom about 73% were female (n = 248). Of the total sample, 192 participants were from Germany (n = 141 female; n = 1 no gender identification), while 141 participants were from the United States (n = 107 female, n = 5 no gender identification). The mean age of participants from the German sample (Mage = 23.00, SD = 2.80) was significantly higher than for the US sample (Mage = 19.74, SD = 1.39). The study utilized a convenience sample. Students were invited to participate in the survey through a variety of channels (e.g., university mailing lists, personal contacts, and social media postings). They were informed that participation was voluntary, and that no course credit or other benefits were being offered for taking the survey.
The global nature of the pandemic encouraged cross-national cooperation between the US and Germany. Ultimately, the data across the groups was collapsed. This was done for several reasons. First, the countries have been found to be similar in terms of cultural values on the individualism – collectivism dimension (Hofstede, 2001). Second, research suggests that the perceived stress levels at the time are comparable in both countries (Kowal et al., 2020). Finally, empirical data suggests that the two countries may be similar in terms of their self-regulation competences (Eggers et al., 2021). However, due to differences in the organization of the educational system, students in US higher education were somewhat younger (M = 19.7, SD = 1.4) than those in Germany (M = 23.1; SD = 2.8).
3.2 Procedure
Students were initially invited to participate in an online survey. The German version of the questionnaire was available from September 16 through November 15, 2021. This period covered the beginning of the fall term (mid-October) and went into the first 4 weeks of the fall term. This was the third semester in a row with mandatory online teaching due to the pandemic. All participants were informed about the purpose of the study and the handling of data protection. Participation was voluntary and anonymous and not connected to a specific course or class. In the case of Germany, students were asked to share the invitation to participate with peers. The US version of the survey was available online from April 2 through June 23, 2021, with data being collected at the end of students’ third semester of mandatory online teaching. As with the German version, participation was voluntary and anonymous, and participants were drawn from a variety of courses. However, unlike the German version, students could not invite others to participate.
3.3 Instruments
Data for all constructs were collected as self-report using subscales from validated instruments. Predictor variables were resilience and SRL competences as indicators of study behavior. The dependent variable was participants’ self-rated competence gain.
3.3.1 Resilience
The 10-item short version the Connor-Davidson Resilience Scale (CD-RISC; Connor and Davidson, 2003; Campbell-Sills and Stein, 2007; Sarubin et al., 2015) was utilized to measure resilience. This measurement was chosen because the theoretical underpinnings of the instrument allow for the interpretation of resilience as a protective factor and because it has validated across international samples (Gras et al., 2019; Kuiper et al., 2019; Velickovic et al., 2020; Kavčič et al., 2023; Wollny and Jacobs, 2023). The scale captures resilience as a general, trait ability to tolerate challenges and to bounce back from personal crisis. The items are worded as active self-report (e.g., “I am able to adapt to change.,” “I can achieve goals despite obstacles.”) and rated on a five-point scale (1 = not at all true to 5 = true nearly all the time). The questionnaire was available both in a validated English and German version with satisfactory psychometric characteristics, with internal consistency measured as Cronbach’s α = 0.84 for the German version (Sarubin et al., 2015) and Cronbach’s α = 0.85 for the version in English (Campbell-Sills and Stein, 2007). In the current study, reliabilities were returned at Cronbach’s α = 0.90 for both the German and the English version.
3.3.2 Self-regulated learning strategies
To measure pre-actional, actional, and post-actional learning strategies, we administered selected subscales of a short version of the Learning Strategies of University Students questionnaire (LIST-K, Klingsieck, 2018). Originally published in German (Klingsieck, 2018), the instrument was translated into English with the support of a bilingual speaker, then back-translated into German to establish equivalence of both versions. To address our hypotheses, we selected the subscales Goalsetting and Planning (e.g., “I set specific goals to guide studying.”) as well as Time Management (“I determine daily time-windows when I study.”), which in combination were taken to represent pre-actional strategies, Attention Allocation (“I am easily distracted when I study.”) as an actional strategy, and Adjusting (“I adjust my study activities when I encounter difficulties.”) as a post-actional strategy. With three items per subscale, this section of the survey contained a total of 12 items. Item responses utilized a five-point scale (1 = very rarely to 5 = very often) to indicate the degree to which these activities reflected the study behavior. Validity studies have demonstrated that the psychometric characteristics of the version (LIST-K) are sound (Loock et al., 2022; Roick et al., 2023). In the current study, the internal consistencies of the selected subscales (Cronbach’s αPre-actional = 0.77, αActional = 0.92, αPost-actional = 0.70) were in keeping with that of previous studies (Klingsieck, 2018; Loock et al., 2022; Roick et al., 2023).
3.3.3 Self-rated competence gain
The measurement of learning outcome across subject area, course type, institutions, and countries was a particular challenge as standard indicators (e.g., grades and grade point average) could not be expected to be equivalent. Additionally, due to emergency regulations, some courses had been re-scaled to a pass/fail mode. As grades were either not comparable across participants or not available, the most feasible alternative was to use an instrument that would allow guided self-evaluation of competence development.
Braun et al. (2008) developed a self-report questionnaire to measure the increase in student competences in a way that would allow comparisons across courses, subjects, years, and schools. Utilizing a survey of students in different institutions of higher education enrolled in a broad range of subjects (liberal arts, education, economics and business administration, social sciences, physics, psychology) (N = 988), Braun et al. (2008) generated a questionnaire to capture competence gain after the course of one semester. Factor analyses yielded evidence for a six factor-solution, distinguishing subject specific skills and knowledge processing, systematic competences (e.g., generic academic skills), presentational competence, communication competence, cooperation competences, and personal competence (which is interpreted as identification with the academic field). Their analysis demonstrated excellent reliabilities in terms of internal consistency and discriminant validity (values for Cronbach’s α ranged between α = 0.83 and 0.92). In particular, the authors did not find evidence for the assumption that data would contain a large self-serving bias.
For the purposes of the current study, we selected the dimensions of Knowledge Processing (“I can define core concepts and principles from this course.”) and of Personal Competences (“The course has supported my determination to continue my study program.”) as it was most plausible that progress in these aspects could be expected to occur for all students. As the scales had displayed very strong bivariate correlations in the original study (r = 0.76; Braun et al., 2008) as well as in our analyses (r = 0.63) and an exploratory factor analysis suggested a single factor-solution, we integrated the two scales. The new scale was labeled ‘competence gain’ and comprised 10 items to be rated on a five-point scale ranging from 1 = not at all true to 5 = totally true. As the questionnaire was available in German only, it was translated into English by a bilingual research assistant, then back-translated. The new scale’s internal consistency for the combined sample was satisfactory (Cronbach’s α = 0.90).
3.4 Data analysis
The data was analyzed using JASP (JASP Team, 2023). We tested our hypotheses with a critical alpha of α = 0.05. To test hypotheses H1 and H2, we used a hierarchical linear regression model with competence gain as dependent variable (see Table 1). In our stepwise procedure, we began with an intercept-only model. Next, we regressed competence gain on resilience (H2), followed by adding the SRL strategies from the pre-actional (H1a), actional (H1b), and post-actional (H1c) phase as predictors. In the last step, we inserted age and gender in our model as control variables. The online survey did not allow for item non-response, thus there were no issues related to missing data. We did not exclude outliers from our data set.
4 Results
4.1 Descriptive results
After obtaining adequate reliability estimates for all scales included in our analyses, descriptive statistics for the overall sample and calculated bivariate correlations for all model variables were generated (see Table 2).
Descriptively, the means for all constructs were well above the scale mean. Except for resilience, the full range of the scales running from 1 through 5 has been found. As seen in Table 2, students who had participated in this survey rated themselves rather strongly on resilience (M = 3.7; SD = 0.7). Self-reported competence gain is in keeping with results reported in Braun et al.’s (2008) original study, which reported an average gain of M = 3.55 (SD = 0.94) for knowledge processing and M = 3.48 (SD = 1.16) for personal competences. As seen in Table 2, competence gain was significantly related to resilience as well as to all three of the SRL learning strategies variables (Table 1 in the Appendix presents the descriptives separately for the two subsamples).
4.2 Self-reported resilience, SRL-strategies, and competence gain
To test the first set of hypotheses (H1a–H1c), we examined the predictive power of SRL (pre-actional, actional, and post-actional) as well as resilience for students’ subjective competence gain utilizing stepwise hierarchical multiple regression (see Table 3).
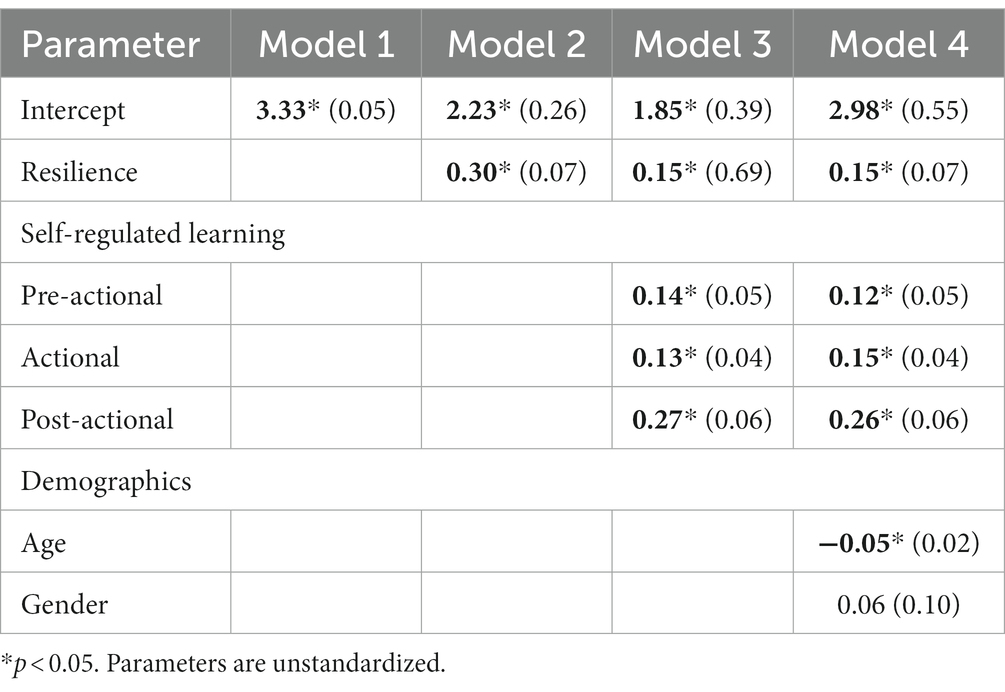
Table 3. Stepwise linear regression of competence gains on resilience, self-regulated learning, and demographics.
Results of the regression analysis suggest that all four variables – SRL activities in the pre-actional, actional, and post-actional phase and resilience – contribute to student competence gain. The standardized regression weights of all predictor variables and 95% confidence intervals are depicted in Figure 1.
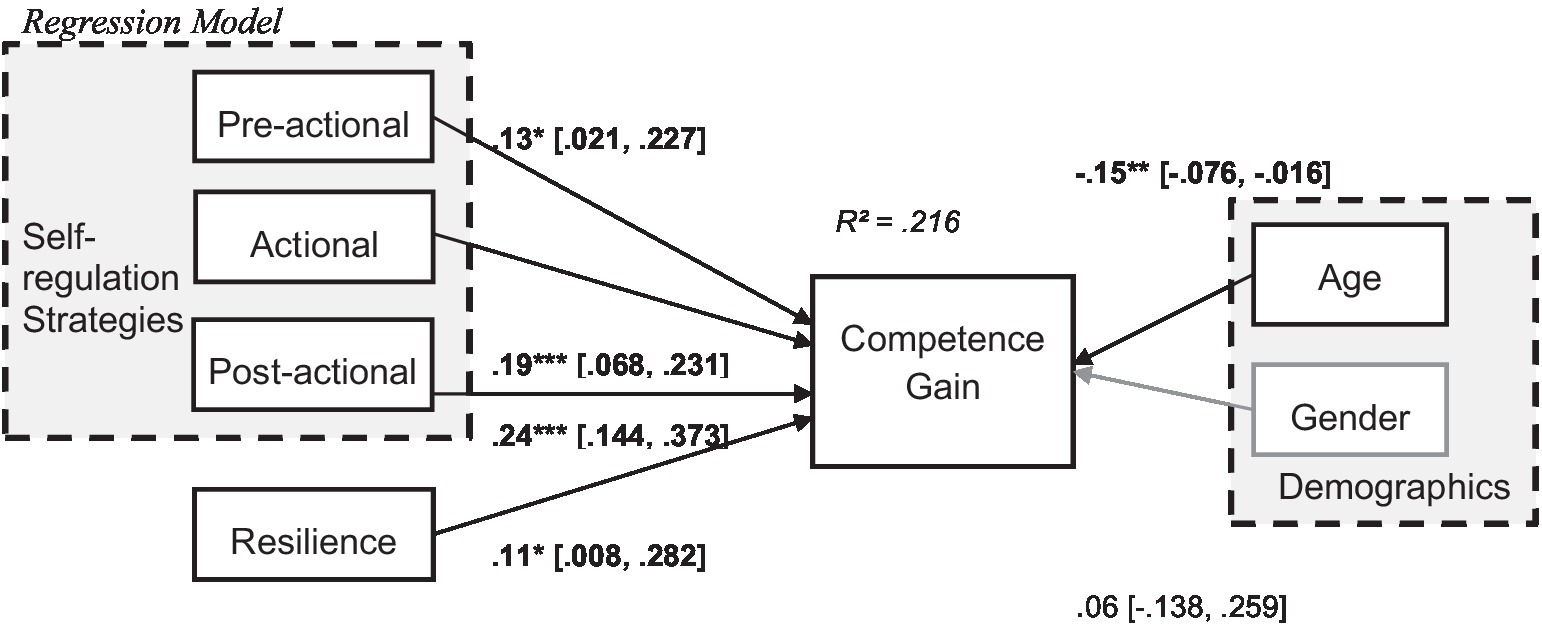
Figure 1. Regression model note. Regression weights are completely standardized and, if displayed in bold, significant at *p < 0.05, **p < 0.01, or ***p < 0.001. 95%-confidence intervals are presented in square brackets. For reasons of parsimony, the model intercept is omitted.
As can be seen, SRL strategies significantly contribute to competence gain. While resilience was also predictive of competence gain, its contributions are less than that of the SRL strategies. Based on these data, hypotheses H1 and H2 are supported – both SRL and resilience contribute to explaining competence gain in ERT. In addition, SRL explains a greater amount of the variance in competence gain when compared to resilience. The confidence interval of the regression coefficient for the predictive power of resilience is rather broad and ranges from close to zero through 0.29. The likelihood of finding similarly high coefficients for the predictive power of resilience for learning is rather limited.
While SRL activities from all three phases of the studying contribute significantly to competence gain, SRL activities in the post-actional phase appear to make a somewhat larger contribution to competence gain as demonstrated by both the value and the confidence interval for SRL activities in the post-actional phase (see Figure 1).
5 Interpretation and discussion
5.1 The learning from the current study
Resilience, the ability to cope with adverse conditions, has been identified as a critical factor for maintaining psychological well-being and functioning in academic contexts in studies conducted prior to and following the global transition to ERT (Sood and Sharma, 2020; Forycka et al., 2022; Gabrovec et al., 2022; Pertegal-Felices et al., 2022; Quintiliani et al., 2022; Versteeg et al., 2022). Subsequently, recommendations for preparing students for, and supporting them during, times of crisis have emphasized strengthening personal resilience.
This study sought to extend this research, exploring the role of self-regulated learning (SRL) as an additional protective factor supporting competence gain of higher education students. Drawing on a sample of students from two different institutions of higher education in Germany and the US, we measured resilience, self-regulated learning strategies, and competence gain.
Results of the regression analysis support the claim that both SRL strategies, and resilience are critical resources for students to make progress in challenging circumstances. Resilience and SRL strategies across all three learning phases had a separate impact on competence gain. However, the SRL post-actional phase (e.g., reflecting on one’s progress, readjusting goals for studying) was most predictive of competence gain when compared to the other variables under study (i.e., the SRL pre-actional and actional phases, and resilience).
As previous surveys had reported higher stress levels for younger people and women (Kowal et al., 2020), we also included age and gender into the regression analysis. While our data did not reveal a gender effect, younger students reported somewhat higher competence gain than older students. This finding may be related to several factors, which potentially confound the results: The US sample was composed of younger students, who were surveyed toward the end of a term and who had, therefore, a clearer and more immediate grasp of their competence gain. In contrast, German students were surveyed at the beginning of a term and asked to look back at their competence gain from the past semester.
The results of this investigation add to our understanding of factors that help protect against academic challenges and failure, particularly in during a crisis. Study results suggest that resilience alone is probably not sufficient to meet the challenges students experience in higher education. For competence gain and progress in their studies, students in higher education need to also possess a full set of self-regulated learning strategies and to use them in a meaningful way (Imhof, 2022; Klimova et al., 2022).
Based on the pattern of results of this study, we recommend preparing students for emergency situations. This preparation should include a component that equips them with strong study competences that take them through the three phases of the SLR learning cycle (Broadbent and Poon, 2015; Bellhäuser et al., 2016; Theobald, 2021). However, special emphasis should be placed the post-actional phase, where students learn how to reflect upon and adjust their learning activities. In times of crisis, these SRL competences are of substantial importance for academic competence gain in addition to strengthening resilience against adversity in general. Given that no suppressor effects were detected in our analysis, results suggest that SRL strategies and resilience are independent resources that students draw on when addressing difficulties as. While both require regulatory skills (e.g., resource allocation, finding and maintaining a focus), they also result in differing outcomes. While self-regulation in learning may lead to task completion, resilience would lead to coping with open ends that cannot yet be tackled conclusively.
5.2 Limitations
All studies have their limitations. For example, the current study addressed a narrow range of the many potential factors (e.g., the quality of the digital devices, the quality of ERT teaching) that might be related to competence gain in an ERT environment.
In addition, the study design precludes estimating the response rates and the amount of non-responses of the survey, which restrains the generalizability of the results (Dzakadzie and Quansah, 2023). Participation was totally voluntary, using multiple recruitment methods. As a result, the size of the population cannot be determined. This is a challenge inherent in survey research. However, one means of addressing the challenge is through a meta-analysis. For now, we argue that the current study produces insights that could and should prompt further research.
The study is limited by the temporal limitations of cross-sectional research. For example, the causal directions specified in our path model rely on the theory formation established and the previous findings outlined. Conducting longitudinal studies would provide a means to address this limitation, while also allowing for an exploration of the trajectories of the obtained effects.
The current study investigated the role of SRL learning activities and resilience on competence gain in a unique situation of worldwide pandemic and, consequently, ERT. As a result, there is no control group for comparison. However, it is plausible to assume that SRL competences contribute to competence gain in other learning environments. What remains, however, is the finding that a solid basis of SRL competency is critical for mastering unexpected challenges when routines are interrupted and when external anchors, such as social support provided by teachers and peers, have been removed.
While the results here reflect those of other studies reporting competence gain in ERT (Elmer et al., 2020; Gonzalez et al., 2020; Iglesias-Pradas et al., 2021), through a methodological lens, data and results may be tinged by the Common Method Bias2 (Jordan and Troth, 2020) as all constructs were measured through self-report. As a result, it remains unclear to what extent the “variance of a measure is attributable to the measurement method rather than to the construct(s) measured” (Steenkamp and Maydeu-Olivares, 2021, p. 5). This is a critical issue, as the beliefs, attitudes, and motivation of the participants to respond to the survey questions in a certain manner is unclear. The degree to which participants tweak their responses toward social desirability is hard to estimate, in particular in a binational sample as the perception of what is socially desirable can be quite different in the two countries. Future research would need to plan for a design that allows a more detailed analysis of the variance that is generated through a common method.
As noted previously, a closer examination of the German and US samples revealed that the participants from the United States were significantly younger than the group from Germany. How the age difference might have affected the results and what kind of bias might have been brought along by the age difference is unclear. In spite of the age difference, the groups were similar in terms of academic age as they were all enrolled in undergraduate programs. So, it is safe to assume that the experience with higher education was not substantially different in the subsamples.
6 Conclusion
Based on the findings of this study, institutions of (higher) education are well-advised to develop programs supporting student training in both resilience and in self-regulated learning strategies. Though resilience is a personal resource that has an impact on competence gain – as was demonstrated in a number of studies that had been conducted during the COVID-19 pandemic, our study suggests that resilience alone may not be sufficient for academic success in times of crisis. In an education context, resilience should be complemented by a set of SRL strategies to ensure competence gain. The development and practice of SRL strategies should address all three phases of learning, with a special emphasis on reflective and meta-cognitive strategies in the post-actional phase. Further research that takes personal resources, such as resilience, study competences, and study outcomes into account simultaneously, is needed to more fully understand the interaction among these and other factors that contribute to academic success.
Data availability statement
The raw data supporting the conclusions of this article will be made available by the authors, without undue reservation.
Ethics statement
The authors confirm that the study was conducted in compliance with the practice as specified by the Declaration of Helsinki. All participants had confirmed a written informed consent. The protocol was approved by the IRB Admin Office of Research Compliance at Auburn University as “Exempt” under federal regulation 45 CFR 46.101(b)(1,2).
Author contributions
MI: Conceptualization, Data curation, Investigation, Methodology, Supervision, Writing – original draft, Writing – review & editing. DW: Data curation, Writing – review & editing. JB: Data curation, Formal analysis, Visualization, Writing – review & editing. HB: Formal analysis, Methodology, Writing – review & editing.
Funding
The author(s) declare that no financial support was received for the research, authorship, and/or publication of this article.
Conflict of interest
The authors declare that the research was conducted in the absence of any commercial or financial relationships that could be construed as a potential conflict of interest.
Publisher’s note
All claims expressed in this article are solely those of the authors and do not necessarily represent those of their affiliated organizations, or those of the publisher, the editors and the reviewers. Any product that may be evaluated in this article, or claim that may be made by its manufacturer, is not guaranteed or endorsed by the publisher.
Footnotes
1. ^The European Qualifications Framework for Higher Education classifies desired competences along four dimensions as (1) knowledge and understanding, (2) application, utilization, and generation of knowledge, (3) communication and cooperation, and (4) scientific self-understanding/professionalism (HRK, 2005, p. 12). The Essential Learning Outcomes (ELOs) of the American Association of Colleges and Universities (2007) reflect the same basic cognitive and noncognitive competences.
2. ^We would like to thank our reviewer for raising the issue of the common method bias.
References
Ahmed, U., Umrani, W. A., Qureshi, M. A., and Samad, A. (2018). Examining the links between teachers support, academic efficacy, academic resilience, and student engagement in Bahrain. Int. J. Adv. Appl. Sci. 5, 39–46. doi: 10.21833/ijaas.2018.09.008
Alemayehu, L., and Chen, H.-L. (2021). Learner and instructor-related challenges for learners’ engagement in MOOCs: a review of 2014–2020 publications in selected SSCI indexed journals. Interact. Learn. Environ. 31, 3172–3194. doi: 10.1080/10494820.2021.1920430
American Association of Colleges and Universities. (2007). Essential Learning Outcomes. Available at https://www.aacu.org/trending-topics/essential-learning-outcomes#:~:text=The%20Essential%20Learning%20Outcomes%20%28ELOs%29%20define%20the%20knowledge,changed%20in%20the%20world%20and%20in%20higher%20education
Ang, W. H. D., Shorey, S., Lopez, V., Chew, H. S. J., and Lau, Y. (2021). Generation Z undergraduate students’ resilience during the COVID-19 pandemic: a qualitative study. Curr. Psychol. 41, 8132–8146. doi: 10.1007/s12144-021-01830-4
Anthonysamy, L., Koo, A.-C., and Hew, S.-H. (2020). Self-regulated learning strategies and non-academic outcomes in higher education blended learning environments: a one decade review. Educ. Inf. Technol. 25, 3677–3704. doi: 10.1007/s10639-020-10134-2
Ayala, J. C., and Manzano, G. (2018). Academic performance of first-year university students: the influence of resilience and engagement. High. Educ. Res. Dev. 37, 1321–1335. doi: 10.1080/07294360.2018.1502258
Bellhäuser, H., Lösch, T., Winter, C., and Schmitz, B. (2016). Applying a web-based training to foster self-regulated learning — effects of an intervention for large numbers of participants. Internet High. Educ. 31, 87–100. doi: 10.1016/j.iheduc.2016.07.002
Braun, E., Gusy, B., Leidner, B., and Hannover, B. (2008). Das Berliner Evaluationsinstrument für selbsteingeschätzte, studentische Kompetenzen (BEvaKomp) [the Berlin evaluation instrument for self-evaluated student competences]. Diagnostica 54, 30–42. doi: 10.1026/0012-1924.54.1.30
Braun, E., Woodley, A., Richardson, J. T. E., and Leidner, B. (2012). Self-rated competences questionnaires from a design perspective. Educ. Res. Rev. 7, 1–18. doi: 10.1016/j.edurev.2011.11.005
Broadbent, J., and Poon, W. L. (2015). Self-regulated learning strategies academic achievement in online higher education learning environments: a systematic review. Internet High. Educ. 27, 1–13. doi: 10.1016/j.iheduc.2015.04.007
Campbell-Sills, L., and Stein, M. B. (2007). Psychometric analysis and refinement of the Connor-Davidson resilience scale (CD-RISC): validation of a 10-item measure of resilience. J. Trauma. Stress. 20, 1019–1028. doi: 10.1002/jts.20271
Caruana, V., Clegg, S., Ploner, J., Stevenson, J., and Wood, J. (2011). Promoting students’ ‘resilient thinking’ in diverse higher education learning environments HE Academy Subject Centre for Sociology, Anthropology and Politics. Leeds.
Chung, J., McKenzie, S., Schweinsberg, A., and Mundy, M. E. (2022). Correlates of academic performance in online higher education: a systematic review. Front. Educ. 7:820567. doi: 10.3389/feduc.2022.820567
Cohen, S., and Williamson, G. (1988). “Perceived stress in a probability sample of the United States” in Social psychology of health. eds. S. Spacapan and S. Oskamp (Thousand Oaks, CA: Sage), 31–67.
Connor, K. M., and Davidson, J. R. (2003). Development of a new resilience scale: the Connor-Davidson resilience scale (CD-RISC). Depress. Anxiety 18, 76–82. doi: 10.1002/da.10113
de la Fuente, J., Santos, F. H., Garzón-Umerenkova, A., Fadda, S., Solinas, G., and Pignata, S. (2021). Cross-sectional study of resilience, positivity and coping strategies as predictors of engagement-burnout in undergraduate students: implications for prevention and treatment in mental well-being. Front. Psych. 12:596453. doi: 10.3389/fpsyt.2021.596453
Duchek, S. (2020). Organizational resilience: a capability-based conceptualization. Bus. Res. 13, 215–246. doi: 10.1007/s40685-019-0085-7
Dzakadzie, Y., and Quansah, F. (2023). Modeling unit non-response and validity of online teaching evaluation in higher education using generalizability theory approach. Front. Psychol. 14:1202896. doi: 10.3389/fpsyg.2023.1202896
Edisherashvili, N., Saks, K., Pedaste, M., and Leijen, A. (2022). Supporting self-regulated learning in distance learning contexts at higher education level: systematic literature review. Front. Psychol. 12:792422. doi: 10.3389/fpsyg.2021.792422
Eggers, J. H., Oostdam, R., and Voogt, J. (2021). Self-regulation strategies in blended learning environments in higher education: a systematic review. Australas. J. Educ. Technol. 175–192, 175–192. doi: 10.14742/ajet.6453
Elmer, T., Mepham, K., and Stadtfeld, C. (2020). Students under lockdown: comparisons of students’ social networks and mental health before and during the COVID-19 crisis in Switzerland. PLoS One 15:e0236337. doi: 10.1371/journal.pone.0236337
Eri, R., Gudimetla, P., Star, S., Rowlands, J., Girgla, A., To, L., et al. (2021). Digital resilience in higher education in response to COVID-19 pandemic: student perceptions from Asia and Australia. J. Univ. Teach. Learn. Pract. 18, 108–134. doi: 10.53761/1.18.5.7
Forycka, J., Pawłowicz-Szlarska, E., Burczyńska, A., Cegielska, N., Harendarz, K., and Nowicki, M. (2022). Polish medical students facing the pandemic — assessment of resilience, well-being and burnout in the COVID-19 era. PLoS One 17:e0261652. doi: 10.1371/journal.pone.0261652
Fuller, A., Belihouse, R., and Johnston, G. (2016). Get it – Creating resilient learners. Queenscliff: Inyahead Press.
Gabrovec, B., Selak, Š., Crnkovič, N., Cesar, K., and Šorgo, A. (2022). Perceived satisfaction with online study during COVID-19 lockdown correlates positively with resilience and negatively with anxiety, depression, and stress among Slovenian postsecondary students. Int. J. Environ. Res. Public Health 19:7024. doi: 10.3390/ijerph19127024
Gillham, J., Abenavoli, R. M., Brunwasser, S. M., Linkins, M., Reivich, K. J., and Seligman, M. E. P. (2013). Resilience education. Oxford Handbook Happ. doi: 10.1093/oxfordhb/9780199557257.013.0046 https://works.swarthmore.edu/fac-psychology/537
Gittell, J. H., Cameron, K., Lim, S., and Rivas, V. (2006). Relationships, layoffs and organizational resilience: airline responses to the crisis of September 11th. J. Appl. Behav. Sci. 42, 300–329. doi: 10.1177/0021886306286466
Gonzalez, T., La Rubia, M. A. de, Hincz, K. P., Comas-Lopez, M., Subirats, L., Fort, S., and Sacha, G. M. (2020). Influence of COVID-19 confinement on students’ performance in higher education. PLoS One, 15:e0239490. doi: 10.1371/journal.pone.0239490
Gras, M.-E., Font-Mayolas, S., Baltasar, A., Patiño, J., Sullman, M. J. M., and Planes, M. (2019). The Connor-Davidson resilience scale (CD-RISC) amongst young Spanish adults. Clínica Y Salud 30, 73–79. doi: 10.5093/clysa2019a11
Gupta, S. D. (2020). Ravaging pandemic of COVID-19. J. Health Manag. 22, 115–116. doi: 10.1177/2F0972063420951876
Hartley, M. T. (2011). Examining the relationships between resilience, mental health, and academic persistence in undergraduate college students. J. Am. Coll. Heal. 59, 596–604. doi: 10.1080/07448481.2010.515632
HRK. (2005). Qualifikationsrahmen für Deutsche Hochschulabschlüsse [Qualification Framework for Higher Education in Germany]. Available at www.kmk.org/doc/beschl/BS_050421_Qualifikationsrahmen_AS_Ka.pdf
Hodges, C., Moore, S., Lockee, B., Trust, T., and Bond, A. (2020), The difference between emergency remote teaching and online learning. Educause Review. Available at: https://er.educause.edu/articles/2020/3/the-difference-between-emergency-remote-teaching-and-online-learning
Hofstede, G. (2001). Culture’s consequences: comparing values, behaviors, institutions, and organizations across nations. Thousand Oaks, CA: Sage Publications.
Hoofman, J., and Secord, E. (2021). The effect of COVID-19 on education. Pediatr. Clin. N. Am. 68, 1071–1079. doi: 10.1016/j.pcl.2021.05.009
Iglesias-Pradas, S., Hernández-García, Á., Chaparro-Peláez, J., and Prieto, J. L. (2021). Emergency remote teaching and students’ academic performance in higher education during the COVID-19 pandemic: a case study. Comput. Hum. Behav. 119:106713. doi: 10.1016/j.chb.2021.106713
Imhof, M. (2022). “Studieren in den Zeiten der Pandemie. Untersuchungen zum Einfluss von Resilienz, Motivationalen Grundbedürfnissen und Lernverhalten auf die Kompetenzentwicklung von Studierenden im digitalen Studium” in Schule zwischen Wandel und Stagnation [Schools Between Change and Stagnation]. eds. L. Fuhrmann and Y. Akbaba (Wiesbaden: Springer), 457–484.
Jansen, R. S., van Leeuwen, A., Janssen, J., and Kester, L. (2022). Exploring the link between self-regulated learning and learner behaviour in a massive open online course. J. Comput. Assist. Learn. 38, 993–1004. doi: 10.1111/jcal.12675
Jehi, T., Khan, R., Dos Santos, H., and Majzoub, N. (2022). Effect of COVID-19 outbreak on anxiety among students of higher education; a review of literature. Curr. Psychol. 42, 17475–17489. doi: 10.1007/s12144-021-02587-6
Jordan, P. J., and Troth, A. C. (2020). Common method bias in applied settings: the dilemma of researching in organizations. Aust. J. Manag. 45, 3–14. doi: 10.1177/0312896219871976
Kavčič, T., Zager Kocjan, G., and Dolenc, P. (2023). Measurement invariance of the CD-RISC-10 across gender, age, and education: a study with Slovenian adults. Curr. Psychol. 42, 1727–1737. doi: 10.1007/s12144-021-01564-3
Kendra, J. M., and Wachtendorf, T. (2003). Elements of resilience after the world trade center disaster: reconstituting new York City’s emergency operations center. Disasters 27, 37–53. doi: 10.1111/1467-7717.00218
Kizilcec, R. F., Pérez-Sanagustín, M., and Maldonado, J. J. (2017). Self-regulated learning strategies predict learner behavior and goal attainment in massive open online courses. Comp. Educ. 104, 18–33. doi: 10.1016/j.compedu.2016.10.001
Klimova, B., Zamborova, K., Cierniak-Emerych, A., and Dziuba, S. (2022). University students and their ability to perform self-regulated online learning under the COVID-19 pandemic. Front. Psychol. 13:781715. doi: 10.3389/fpsyg.2022.781715
Klingsieck, K. B. (2018). Kurz und knapp – die Kurzskala des Fragebogens “Lernstrategien im Studium” (LIST) [short and sweet – the short version of the questionnaire learning strategies of university students (LIST)]. Zeitschrift für Pädagogische Psychologie 32, 249–259. doi: 10.1024/1010-0652/a000230
Kowal, M., Coll-Martín, T., Ikizer, G., Rasmussen, J., Eichel, K., Studzińska, A., et al. (2020). Who is the most stressed during the COVID-19 pandemic? Data from 26 countries and areas. Appl. Psychol. Health Well Being 12, 946–966. doi: 10.1111/aphw.12234
Kuiper, H., van Leeuwen, C. C. M., Stolwijk-Swüste, J. M., and Post, M. W. M. (2019). Measuring resilience with the Connor-Davidson resilience scale (CD-RISC): which version to choose? Spinal Cord 57, 360–366. doi: 10.1038/s41393-019-0240-1
Kunzler, A. M., Röthke, N., Günthner, L., Stoffers-Winterling, J., Tüscher, O., Coenen, M., et al. (2021). Mental burden and its risk and protective factors during the early phase of the SARS-CoV-2 pandemic: systematic review and meta-analyses. Glob. Health 17:34. doi: 10.1186/s12992-021-00670-y
Laranjeira, C., Dixe, M. A., and Querido, A. (2023). Mental health status and coping among Portuguese higher education students in the early phase of the COVID-19 pandemic. Eur. J. Invest. Health. Psychol. Educ. 13, 429–439. doi: 10.3390/ejihpe13020032
Lin, C.-H., Lin, S.-Y., Hu, B.-H., and Lo, C. O. (2023). Investigating mental health outcomes of undergraduates and graduate students in Taiwan during the COVID-19 pandemic. J. Am. Coll. Heal. 1–8, 1–8. doi: 10.1080/07448481.2022.2162824
Loock, V. S., Fleischer, J., Scheunemann, A., Froese, L., Teich, K., and Wirth, J. (2022). Narrowing down dimensions of e-learning readiness in continuing vocational education - perspectives from the adult learner. Front. Psychol. 13:1033524. doi: 10.3389/fpsyg.2022.1033524
Mallak, L. A. (1998). Measuring resilience in health care provider organizations. Health Manpow. Manag. 24, 148–152. doi: 10.1108/09552069810215755
McPherson, A. V. (2012). College student life and financial stress: an examination of the relation among perception of control and coping styles on mental health functioning. Raleigh, NC: North Carolina State University.
Nandy, M., Lodh, S., and Tang, A. (2021). Lessons from COVID-19 and a resilience model for higher education. Ind. High. Educ. 35, 3–9. doi: 10.1177/0950422220962696
Nurunnabi, M., Almusharraf, N., and Aldeghaither, D. (2020). Mental health and well-being during the COVID-19 pandemic in higher education: evidence from G20 countries. J. Public Health Res. 9:2010. doi: 10.4081/jphr.2020.2010
Paetsch, J., and Drechsel, B. (2021). Factors influencing pre-service teachers’ intention to use digital learning materials: a study conducted during the COVID-19 pandemic in Germany. Front. Psychol. 12:733830. doi: 10.3389/fpsyg.2021.733830
Panadero, E., Jonsson, A., and Botella, J. (2017). Effects of self-assessment on self-regulated learning and self-efficacy: four meta-analyses. Educ. Res. Rev. 22, 74–98. doi: 10.1016/j.edurev.2017.08.004
Pertegal-Felices, M. L., Valdivieso-Salazar, D. A., Espín-León, A., and Jimeno-Morenilla, A. (2022). Resilience and academic dropout in Ecuadorian university students during COVID-19. Sustainability 14:8066. doi: 10.3390/su14138066
Pintrich, P. R. (2000). “The role of goal orientation in self-regulated learning” in Handbook of self-regulation. eds. M. Boekaerts, P. R. Pintrich, and M. Zeidner (San Diego, CA: Academic Press), 451–502.
Polizzi, C., Lynn, S. J., and Perry, A. (2020). Stress and coping in the time of COVID-19: pathways to resilience and recovery. Clin. Neuropsychiatry 17, 59–62. doi: 10.36131/CN20200204
Quintiliani, L., Sisto, A., Vicinanza, F., Curcio, G., and Tambone, V. (2022). Resilience and psychological impact on Italian university students during COVID-19 pandemic: distance learning and health. Psychol. Health Med. 27, 69–80. doi: 10.1080/13548506.2021.1891266
Reyes, A. T., Andrusyszyn, M.-A., Iwasiw, C., Forchuk, C., and Babenko-Mould, Y. (2015). Resilience in nursing education: an integrative review. J. Nurs. Educ. 54, 438–444. doi: 10.3928/01484834-20150717-03
Robbins, A., Kaye, E., and Catling, J. C. (2018). Predictors of student resilience in higher education. Psychol. Teach. Rev. 24, 44–52. doi: 10.53841/bpsptr.2018.24.1.44
Roick, J., Poethke, P., and Richter, M. (2023). Learners’ characteristics and the mastery of digital education during the COVID-19 pandemic in students of a medical faculty in Germany. BMC Med. Educ. 23:86. doi: 10.1186/s12909-023-04012-x
Rychen, D. S., and Salganik, L. H. (2003). Key competencies for a successful life and a well-functioning society. Bern: Hogrefe and Huber Publishers.
Salimi, N., Gere, B., Talley, W., and Irioogbe, B. (2023). College students mental health challenges: concerns and considerations in the COVID-19 pandemic. J. Coll. Stud. Psychother. 37, 39–51. doi: 10.1080/87568225.2021.1890298
San Román-Mata, S., Zurita-Ortega, F., Puertas-Molero, P., Badicu, G., and González-Valero, G. (2020). A predictive study of resilience and its relationship with academic and work dimensions during the COVID-19 pandemic. J. Clin. Med. 9. doi: 10.3390/jcm9103258
Sarasjärvi, K. K., Vuolanto, P. H., Solin, P. C. M., Appelqvist-Schmidlechner, K. L., Tamminen, N. M., Elovainio, M., et al. (2022). Subjective mental well-being among higher education students in Finland during the first wave of COVID-19. Scand. J. Public Health 50, 765–771. doi: 10.1177/14034948221075433
Sarubin, N., Gutt, D., Giegling, I., Bühner, M., Hilbert, S., Krähenmann, O., et al. (2015). Erste analyse der psychometrischen Eigenschaften und Struktur der deutschsprachigen 10- und 25-item version der Connor-Davidson resilience scale (CD-RISC) [first analysis of the 10- and 25-item German version of the Connor-Davidson resilience scale (CD-RISC) regarding psychometric properties and components]. Zeitschrift für Gesundheitspsychologie 23, 112–122. doi: 10.1026/0943-8149/a000142
Sood, S., and Sharma, A. (2020). Resilience and psychological well-being of higher education students during COVID-19: the mediating role of perceived distress. J. Health Manag. 22, 606–617. doi: 10.1177/0972063420983
Steenkamp, J.-B. E., and Maydeu-Olivares, A. (2021). An updated paradigm for evaluating measurement invariance incorporating common method variance and its assessment. J. Acad. Mark. Sci. 49, 5–29. doi: 10.1007/s11747-020-00745-z
Theobald, M. (2021). Self-regulated learning training programs enhance university students’ academic performance, self-regulated learning strategies, and motivation: a meta-analysis. Contemp. Educ. Psychol. 66:101976. doi: 10.1016/j.cedpsych.2021.101976
Travis, J., and Bunde, J. (2020). Self-regulation in college: the influence of self-efficacy, need satisfaction, and stress on GPA, persistence, and satisfaction. Curr. Psychol. 41, 6185–6195. doi: 10.1007/s12144-020-01091-7
Välikangas, L., and Romme, A. G. L. (2013). How to design for strategic resilience: a case study in retailing. J. Organ. Des. 2, 44–53. doi: 10.7146/jod.2.2.7360
Van der Zanden, P. J. A. C., Denessen, E., Cillessen, A. H. N., and Meijer, P. C. (2018). Domains and predictors of first-year student success: a systematic review. Educ. Res. Rev. 23, 57–77. doi: 10.1016/j.edurev.2018.01.001
Velickovic, K., Rahm Hallberg, I., Axelsson, U., Borrebaeck, C. A. K., Rydén, L., Johnsson, P., et al. (2020). Psychometric properties of the Connor-Davidson resilience scale (CD-RISC) in a non-clinical population in Sweden. Health Qual. Life Outcomes 18:132. doi: 10.1186/s12955-020-01383-3
Versteeg, M., Kappe, R. F., and Knuiman, C. (2022). Predicting student engagement: the role of academic belonging, social integration, and resilience during COVID-19 emergency remote teaching. Front. Pub. Health 10:849594. doi: 10.3389/fpubh.2022.849594
Weick, K. E., and Sutcliffe, K. M. (2015). Managing the unexpected: sustained performance in a complex world. 3rd Edn. Hoboken, NJ: John Wiley & Sons.
Weidlich, J., and Kalz, M. (2021). Exploring predictors of instructional resilience during emergency remote teaching in higher education. Int. J. Educ. Technol. High. Educ. 18:43. doi: 10.1186/s41239-021-00278-7
Winne, P. H. (2018). “Cognition and metacognition within self-regulated learning” in Handbook of self-regulation of learning and performance. eds. D. H. Schunk and J. A. Greene (New York NY: Routledge/Taylor & Francis Group), 36–48.
Wollny, A. I., and Jacobs, I. (2023). Validity and reliability of the German versions of the CD-RISC-10 and CD-RISC-2. Curr. Psychol. 42, 3437–3448. doi: 10.1007/s12144-021-01670-2
Ye, Z., Yang, X., Zeng, C., Wang, Y., Shen, Z., Li, X., et al. (2020). Resilience, social support, and coping as mediators between COVID-19-related stressful experiences and acute stress disorder among college students in China. Appl. Psychol. Health Well Being 12, 1074–1094. doi: 10.1111/aphw.12211
Keywords: resilience, self-regulated learning, competence gain, higher education, emergency remote teaching
Citation: Imhof M, Worthington D, Burger J and Bellhäuser H (2024) Resilience and self-regulated learning as predictors of student competence gain in times of the COVID 19 pandemic – evidence from a binational sample. Front. Educ. 9:1293736. doi: 10.3389/feduc.2024.1293736
Edited by:
Ana B. Bernardo, University of Oviedo, SpainReviewed by:
Frank Quansah, University of Education, GhanaMaria Esteban, Universidad de Oviedo, Spain
Copyright © 2024 Imhof, Worthington, Burger and Bellhäuser. This is an open-access article distributed under the terms of the Creative Commons Attribution License (CC BY). The use, distribution or reproduction in other forums is permitted, provided the original author(s) and the copyright owner(s) are credited and that the original publication in this journal is cited, in accordance with accepted academic practice. No use, distribution or reproduction is permitted which does not comply with these terms.
*Correspondence: Margarete Imhof, aW1ob2ZAdW5pLW1haW56LmRl