- 1Queensland Research Centre for Peripheral Vascular Disease, College of Medicine and Dentistry, James Cook University, Townsville, QLD, Australia
- 2Ulcer and Wound Healing Consortium (UHEAL), Australian Institute of Tropical Health and Medicine, James Cook University, Townsville, QLD, Australia
- 3Faculty of Health and Medicine, School of Health Sciences, University of Newcastle, Newcastle, NSW, Australia
- 4Australian Institute of Tropical Health and Medicine, James Cook University, Townsville, QLD, Australia
- 5Department of Vascular and Endovascular Surgery, Townsville University Hospital, Townsville, QLD, Australia
Background: Remotely delivered interventions may be more efficient in controlling multiple risk factors in people with diabetes.
Purpose: To pool evidence from randomized controlled trials testing remote management interventions to simultaneously control blood pressure, blood glucose and lipids.
Data Sources: PubMed/Medline, EMBASE, CINAHL and the Cochrane library were systematically searched for randomized controlled trials (RCTs) until 20th June 2021.
Study Selection: Included RCTs were those that reported participant data on blood pressure, blood glucose, and lipid outcomes in response to a remotely delivered intervention.
Data Extraction: Three authors extracted data using a predefined template. Primary outcomes were glycated hemoglobin (HbA1c), total cholesterol (TC), low-density lipoprotein cholesterol (LDL-c), systolic and diastolic blood pressure (SBP & DBP). Risk of bias was assessed using the Cochrane collaboration RoB-2 tool. Meta-analyses are reported as standardized mean difference (SMD) with 95% confidence intervals (95%CI).
Data Synthesis: Twenty-seven RCTs reporting on 9100 participants (4581 intervention and 4519 usual care) were included. Components of the remote management interventions tested were identified as patient education, risk factor monitoring, coaching on monitoring, consultations, and pharmacological management. Comparator groups were typically face-to-face usual patient care. Remote management significantly reduced HbA1c (SMD -0.25, 95%CI -0.33 to -0.17, p<0.001), TC (SMD -0.17, 95%CI -0.29 to -0.04, p<0.0001), LDL-c (SMD -0.11, 95%CI -0.19 to -0.03, p=0.006), SBP (SMD -0.11, 95%CI -0.18 to -0.04, p=0.001) and DBP (SMD -0.09, 95%CI -0.16 to -0.02, p=0.02), with low to moderate heterogeneity (I²= 0 to 75). Twelve trials had high risk of bias, 12 had some risk and three were at low risk of bias.
Limitations: Heterogeneity and potential publication bias may limit applicability of findings.
Conclusions: Remote management significantly improves control of modifiable risk factors.
Systematic Review Registration: [https://www.crd.york.ac.uk/prospero/display_record.php?RecordID=258433], identifier PROSPERO (CRD42021258433).
Introduction
Adults diagnosed with diabetes are at high risk of major adverse events such as myocardial infarction, stroke, end stage renal failure, foot ulceration, amputation and death (1, 2). The risk of these complications can be reduced by control of blood glucose, blood pressure and lipids (3–7). Optimal control of these risk factors is infrequently achieved in routine practice, representing a missed opportunity to prevent major adverse events (6, 8). This may be due to limited access to specialists, lack of cohesive healthcare delivery and ineffective patient education (9, 10).
The medical management of people with diabetes usually involves frequent face-to-face appointments with multiple specialists (11). This can contribute to confusion about how intensively risk factors should be controlled and who is responsible for managing these risk factors (12). It also disadvantages patients in rural and remote settings who may not be able to access specialist medical services easily (13).
Remotely delivered risk factor management programs have been proposed as a more efficient way to control multiple risk factors (14–18). Risk factor monitoring, healthcare consultations, medication prescription and behavioral support can occur remotely to facilitate optimizing blood glucose, blood pressure and lipids (17–19). No previous meta-analysis or overviews have evaluated the benefit of simultaneous remote management of all these risk factors (17, 18, 20). Evidence on the effectiveness of interventions that simultaneously control multiple modifiable risk factors is needed to inform how most efficiently to deliver preventive management. This systematic review and meta-analysis aimed to pool evidence from randomized controlled trials (RCTs) testing the effectiveness of remote risk factor management programs for people with diabetes in simultaneously controlling blood glucose, blood pressure, and lipids.
Methods
This review was conducted in accordance with the Preferred Reporting Items for Systematic Reviews and Meta-Analyses (PRISMA) guidelines (21), and is registered with PROSPERO (CRD42021258433).
Data Sources and Searches
The PubMed/Medline, EMBASE, CINAHL and Cochrane library databases were searched independently by three authors (MF, LS AD) for English language articles of RCTs published from 1st January 2000 to 20th June 2021. This date restriction was applied due to the relative recent introduction of remotely-delivered healthcare and in order to provide a contemporary assessment of intervention strategies. The search combined three term groups; 1) ‘controlled trial’ (e.g. randomized, clinical trial), 2) ‘remote’ (e.g. telehealth), and 3) ‘disease and treatment’ (e.g. diabetes, dyslipidemia). The full search string is shown in Supplementary Text 1. Reference and citation lists of eligible articles were also manually searched.
Study Selection
Eligible articles were published RCTs that evaluated the effect of remote medical management interventions in comparison to usual care. The population of interest were adults ≥18 years old with either type 1 or type 2 diabetes mellitus irrespective of disease duration or history of cardiovascular disease. The interventions were remotely delivered healthcare (e.g. internet or phone-based monitoring or telehealth consultations) aimed at optimizing glycemic control, systolic blood pressure (SBP) and/or diastolic blood pressure (DBP) and total cholesterol (TC) and/or low-density lipoprotein cholesterol (LDL-c). The control group received usual medical management without remotely delivered healthcare. Each RCT identified was screened by at least two authors (MF, LS, AD, BC). Trials that did not aim to control all three risk factors or failed to report them were excluded.
Data Extraction and Quality Assessment
The primary outcome was the impact of the remotely delivered interventions on: 1) hemoglobin A1c (HbA1c %), 2) TC and LDL-c (mmol/L), and 3) SBP and DBP (mmHg) compared to the control groups. Secondary outcomes included incidence of adverse events including hypoglycemia, postural hypotension, hospital admission, death, limb-related events including leg revascularization or lower limb amputation, other medication related side-effects, major adverse cardiovascular events (MACE), development of micro-vascular complications including progression of retinopathy, neuropathy (including incident foot ulceration), or nephropathy, and all-cause mortality. Other secondary outcomes were health-related quality of life and cost-benefit analyses.
A standardized data extraction form was developed to extract the following data from each study: title, authors, year published, country of publication, number of participants, participant characteristics, intervention setting, type, frequency and duration of remote and usual care intervention(s), primary and secondary outcomes, study limitations and whether intention-to-treat or per-protocol analyses. Two authors independently extracted data, which were checked by a third author (MF, LS, AD, BC). Where studies reported multiple follow-up data, the longest follow-up duration was used. Where there were more than one intervention arm, all intervention groups were included. Meta-analyses included the number of participants completing the trial rather than numbers initially randomized as outcome data were only available for this group. Study authors were contacted for all potentially eligible studies to obtain additional and missing data.
Methodological quality was assessed independently by three authors (AD, LS and BC) using the Cochrane collaborations revised risk-of-bias tool for randomized trials (RoB 2) (22). Following independent evaluation, discussions were held between assessors to arrive at a consensus score. Where this was not possible, a final consensus on the overall risk of bias was made by an independent fourth assessor (MF). In relation to the tool, five outcomes were possible for each criterion which were ‘yes’, ‘probably yes’, ‘no information’, ‘probably no’, or ‘no’ (22). Studies were rated as low risk of bias if all domains were judged to be at low risk of bias, high risk of bias if any domain was judged to be at high risk of bias, or ‘some concerns’ of bias if any domain was judged to have some concerns but no domain had a high risk of bias (22).
Data Synthesis and Analysis
Numerical data were reported as mean and standard deviation (SD) and categorical data as number and percentage (%). Meta-analysis were performed for any primary or secondary outcome with data extractable from a minimum of three studies. The meta-analyses were conducted using the inverse-variance method for continuous outcomes and the Mantel-Haenszel statistical method for dichotomous outcomes with random effect models anticipating substantial heterogeneity (23). The results were reported as standardized mean difference (SMD) (24) or risk ratio (RR) and 95% CI for dichotomous outcomes (23). All statistical tests were two-sided and a p value <0.05 was considered significant. Heterogeneity was assessed using I2 statistic values (interpreted as 0 to 49%: low, 50 to 74%: moderate and 75 to 100%: high) (25). Several sensitivity and subgroup analyses were carried out including leave-one-out (LOO) sensitivity analyses and analysis excluding studies with high risk of bias. Several sub-group analyses were also carried out (see Supplementary Text 2). Five distinct aspects of the remote management programs tested were defined to clarify which aspects of the interventions were most important in improving outcome in subgroup meta-analysis. Subgroup meta-analysis was also planned to evaluate whether remote management was more effective in studies that only included a higher risk population at entry who were at greater risk of MACE. Higher-risk was defined as: a documented history of cardiovascular disease, a diabetes duration of greater than 10 years, HbA1c of >10.0% and/or LDL of >2.0 mmol/L and/or SBP of >130 mmHg and/or a DBP of >80 mmHg or a previous history of diabetes related complications at entry. Publication bias was assessed by funnel plots comparing the summary estimate of each study and its precision (1/standard error) (26). All analyses were conducted with Review Manager (RevMan) version 5.4. (The Cochrane Collaboration, 2020).
Results
Of 2458 unique articles identified, 46 were assessed for full-text eligibility and 27 RCTs were included (Figure 1) (27–53). Most full-text screened studies that were excluded did not target or report on the impact of the remotely-delivered intervention on all key risk factors of interest (Supplementary Table 1). Of 33 contacted authors from potentially eligible studies, four replied with the request for additional data (32, 37, 39, 44).
Study and Participant Characteristics
The included studies had a total of 9153 participants randomized and reported outcomes on 4581 participants randomized to an intervention group and 4519 to usual care. Sample sizes of individual trials ranged from 36 to 1665 (Table 1) and follow-up durations ranged from 3 to 60 months. Supplementary Table 2 reports the inclusion and exclusion criteria of each trial and the total number of participants screened and excluded. Six RCTs included people with type 1 diabetes (27, 29, 31, 33, 38, 46) and all others exclusively included participants with type 2 diabetes. Several studies excluded participants with severe complications such as foot ulcers, progressive nephropathy or retinopathy. Three studies recruited participants deemed to be at high risk of diabetes complications based on entry criteria (35, 41, 51). Participant medications at baseline and follow-up are shown in Supplementary Table 3, and baseline risk factors are shown in Supplementary Table 4, and study outcome measures, additional supports and methods of risk factor monitoring are shown in Supplementary Table 5.
Description of the Types of Interventions Tested
A detailed description of the types of interventions is given in Supplementary Table 5, with Supplementary Table 6 providing a summary overview of the key elements of the intervention provided in each trial used in the meta-analyses. Twenty out of the twenty-seven RCTs provided remote patient education without any in-person education (28–31, 35–37, 39–43, 45–50, 52, 53), nineteen RCTs provided remote risk factor monitoring (29, 31–34, 36–39, 41–47, 51–53), twenty-two RCTs provided remote coaching regarding risk factors without the use of in-person coaching (27–37, 39, 41, 43–44, 46–49, 51–53), twelve provided remote consultation without any in-person consultation (31, 35, 36, 40–47, 49) and fourteen provided remote pharmacological advice or reminders to the patient or treating team (28, 30, 34, 37–38, 41–44, 46–48, 50, 51) (see Supplementary Text 3 for further info).
Description of Control Groups
In most studies, the control group received usual care (Supplementary Table 5). This typically consisted of regular primary care physician management of participant risk factors based on guideline recommendations. Ten RCTs failed to provide a definition of usual care (27, 30, 35, 37, 39, 44, 47, 50, 52, 53). One RCT delivered non-health related text-messages to the control group (35). One RCT provided the control arm with the same blood glucose monitoring system as their intervention group, but the data was not transmitted to an online portal for further intervention (38).
Risk of Bias of Included Studies
Overall, 12 trials were deemed to be at high risk (27, 29, 33, 36, 38, 40–42, 45, 49, 51, 53), 12 trials had some concerns regarding their risk of bias (30–32, 35, 37, 39, 43, 46–48, 50, 52) and three were at low risk of bias (Supplementary Table 7) (28, 34, 44). Problems identified with high-risk studies included lack of detail on the appropriateness of analyses (27, 29), lack of allocation concealment (45, 51), differences in baseline risk factors (HbA1c) between the intervention and control groups (33), missing outcome data (36, 41, 42, 49) and differences in how data were collected between the intervention and control groups for the primary outcome (38, 40, 53).
Primary Outcome Measures
Supplementary Table 8 reports on the main risk factor outcomes based on last known follow-up included in the meta-analysis.
Impact of Remote Intervention on HbA1c
A meta-analysis of all 27 RCTs incorporating 3579 participants in the intervention group and 3726 participants in the control group found that remote risk factor management significantly reduced HbA1c compared to usual care (SMD -0.25, 95% CI -0.33 to -0.17, Z=6.17, p=<0.001; Figure 2A) with a moderate degree of heterogeneity (I²= 60%). The funnel plot was asymmetrical (Supplementary Results, Figure 1.1). LOO sensitivity analyses suggested removal of any individual RCT did not affect the significance of the finding (Supplementary Results, Table 2.1). Exclusion of studies with high risk of bias did not change the significance of the outcome (Supplementary Results, Figure 3.1). Subgroup analyses focused on remote intervention type or a high-risk population did not change the significance of the outcome (Table 2 and Supplementary Results, Figures 4.1-4.5 and 5.1).
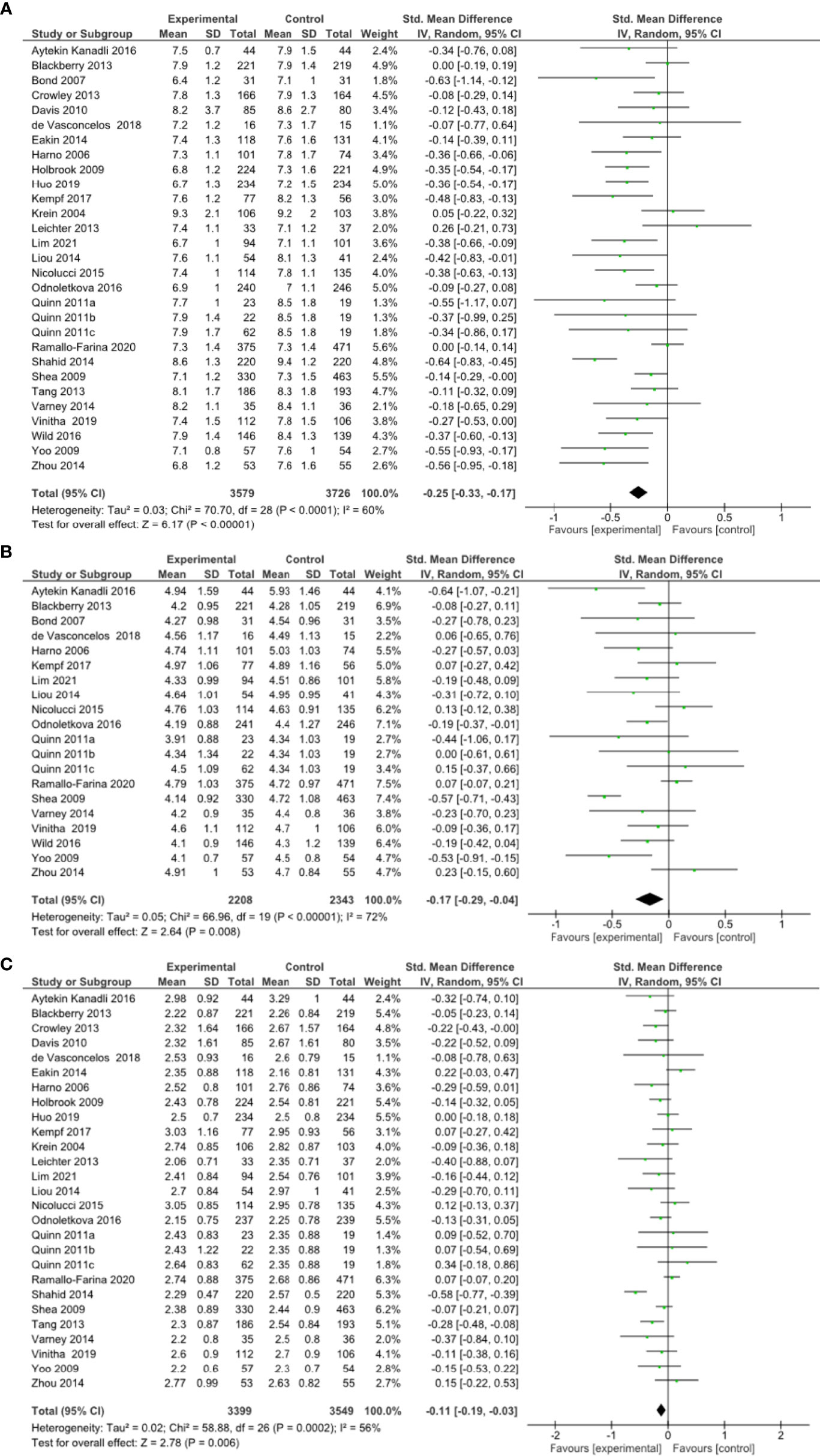
Figure 2 (A) Forest plot showing the effect of remote risk factor management on HbA1c, (B) Forest plot showing the effect of remote management on total cholesterol, (C) Forest plot showing the effect of remote risk factor management on LDL-cholesterol.
Impact of Remote Intervention on Blood Lipids
A meta-analysis of 18 RCTs incorporating 2208 participants in the intervention group and 2343 participants in the control group found that remote risk factor management significantly reduced TC compared to usual care (SMD -0.17, 95% CI -0.29 to -0.04, Z=2.64, p=0.008) with a moderate degree of heterogeneity (I²= 72%) (Figure 2B). The funnel plot was asymmetrical (Supplementary Results, Figures 1.2). LOO sensitivity analyses suggested removal of one study reduced the heterogeneity and effect size substantially (46) (Supplementary Results, Figures 2.2). Exclusion of studies with high risk of bias changed the significance of the outcome (SMD -0.20, 95% CI -0.40 to 0.00, Z=1.91, p=0.06) with a high degree of heterogeneity (I²= 81%) and the funnel plot was symmetrical (Supplementary Results, Figures 3.3, 3.4). Subgroup analyses of remote interventions showed only the coaching of risk factor modification significantly reduced TC compared to usual care (Table 2 and Supplementary Results, Figures 4.6-4.10). There were insufficient studies to undertake subgroup analysis of a high-risk population.
A meta-analysis of 25 RCTs incorporating 3399 participants in the intervention group and 3549 participants in the control group found that remote risk factor management significantly reduced LDL-c compared to usual care (SMD -0.11, 95% CI -0.19 to -0.03, Z=2.78, p=0.006) with a moderate degree of heterogeneity (I²= 56%) (Figure 2C). The funnel plot was asymmetrical (Supplementary Results, Figures 1.3). LOO sensitivity analyses suggested removal of any individual RCT did not affect the significance of the main finding (Supplementary Results, Table 2.3). Exclusion of studies with high risk of bias did not change the significance of the outcome (Supplementary Results, Figure 3.5). Subgroup analyses suggested that patient education, consultation and pharmacological management but not coaching of risk factor modification significantly reduced LDL-c compared to usual care (Table 2 and Supplementary Results, Figures 4.11–4.15). Subgroup analysis also suggested that the interventions did not significantly reduce LDL-c in the high-risk population (Supplementary Results, Figure 5.2).
Impact of Interventions on Blood Pressure
A meta-analysis of all 27 RCTs incorporating 3580 participants in the intervention group and 3726 participants in the control group found that remote risk factor management significantly reduced SBP compared to usual care (SMD -0.11, 95% CI -0.18 to -0.04, Z=3.25, p=0.001) with a low degree of heterogeneity (I²= 44%) (Figure 3A). The funnel plot was asymmetrical (Supplementary Results, Figure 1.4). LOO sensitivity analyses suggested removal of any individual RCT did not affect the significance of the main finding (Supplementary Results, Table 2.4). Exclusion of studies with high risk of bias changed the significance of the outcome (SMD -0.09, 95% CI -0.18 to 0.00, Z=1.96, p=0.05) with a moderate degree of heterogeneity (I²= 56%) and the funnel plot was asymmetrical (Supplementary Results, Figures 3.7, 3.8). Subgroup analyses focused on remote intervention type did not change the significance of the outcome (Table 2 and Supplementary Results, Figures 4.16-4.20). Subgroup analysis suggested that the interventions did not significantly reduce SBP in the high-risk population (Supplementary Results, Figure 5.3).
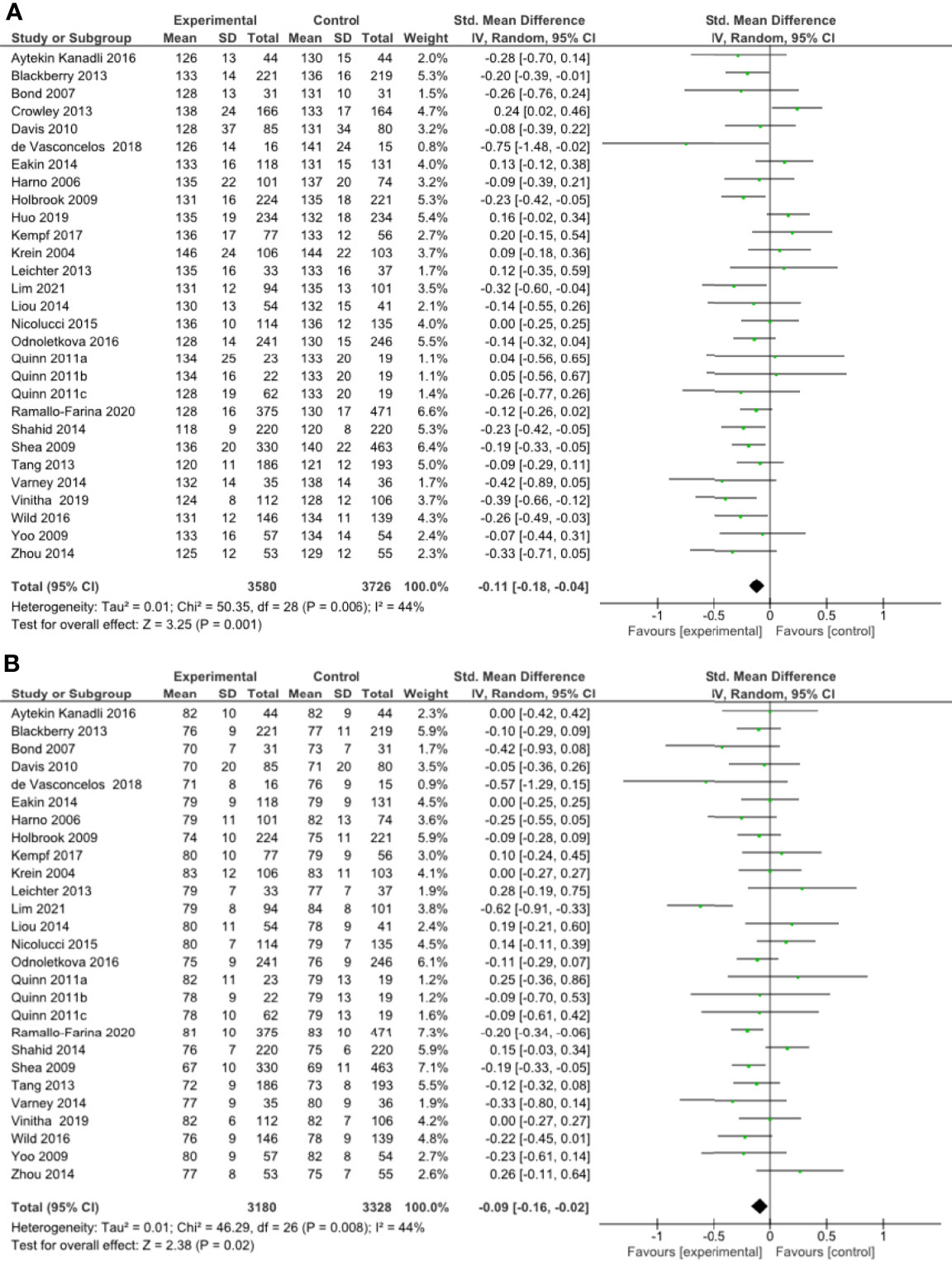
Figure 3 (A) Forest plot showing the effect of remote management on systolic blood pressure, (B) Forest plot showing the effect of remote risk factor management on diastolic blood pressure.
A meta-analysis of all 27 RCTs incorporating 3180 participants in the intervention group and 3328 participants in the control group found that remote risk factor management significantly reduced DBP compared to usual care (SMD -0.09, 95% CI -0.16 to -0.02, Z=2.38, p=0.02) with a low degree of heterogeneity (I²= 44%) (Figure 3B). The funnel plot was asymmetrical (Supplementary Results, Figure 1.5). LOO sensitivity analyses suggested removal of any individual RCT did not affect the significance of the main finding (Supplementary Results, Table 2.5). Exclusion of studies with high risk of bias did not change the significance of the outcome (Supplementary Results, Figure 3.9). Subgroup analyses suggested that monitoring of risk factors, coaching of risk factor modification and pharmacological management but not patient education and consultation significantly reduced DBP compared to usual care (Table 2 and Supplementary Results, Figures 4.21-4.25). There were insufficient studies to undertake subgroup analysis of a high-risk population.
Secondary Outcome Measures
Supplementary Tables 9 and 10 report the secondary outcome data. One study reported on major adverse cardiovascular events (51), but none of the studies reported on limb revascularization or amputation, or progression of microvascular disease or worsening of existing comorbidities. One study reported that the cost of the intervention was more than the control due to telemonitoring service costs and additional nurse phone consultations (51). None of the studies undertook a cost-benefit analysis. Quality of life data were reported in seven RCTs (28, 34–36, 41, 50, 51), but could not be combined in meta-analysis due to heterogeneity of instruments used. Two studies reported significant improvements in quality of life in the remote management group at follow-up compared to baseline (36, 41). Fifteen RCTs reported on adverse outcomes (28, 30, 32, 34–39, 41, 42, 46, 48, 51, 53). Four RCTs reported on medication related side effects including hypoglycemia and postural hypotension (32, 39, 51, 53). Mortality during follow-up was reported in 17 RCTs (27–32, 34, 35, 37, 39, 41–43, 46–48, 51).
A meta-analysis including 15 RCTs incorporated 2979 participants in the intervention group and 2955 participants in the control group found that remote risk factor management had no effect on overall adverse outcomes (RR = 0.88, 95% CI 0.70 to 1.09, Chi2 = 12.85, p=0.24) with a low degree of heterogeneity (I²= 7%) (Supplementary Results, Figure 6.1). The funnel plot was asymmetrical (Supplementary data, Figure 7.1). Additional meta-analyses of individual adverse events including mortality, hypoglycemic episodes and hospital admission showed no significant difference between groups (Supplementary Results, Figures 6.2-6.4).
Discussion
This meta-analysis suggested that remote management significantly improved control of the five modifiable risk factors for diabetes-related major adverse events. Small reductions in HbA1c and TC and modest reductions in LDL-c, SBP and DBP were found. The main findings were robust in sensitivity analyses but clarity on which components of the remote management were most effective was limited because all interventions included a composite of different intervention types. Remote risk factor management had no effect on the rate of adverse outcomes including mortality, hypoglycemic episodes and hospital admissions.
The generalizability of the findings of this meta-analysis need to consider the populations studied. These were mainly people with diabetes without a history of major adverse events but with poor risk factor control at entry (56–59). The findings may not be generalizable to populations where risk factors are already well controlled or those with a past history of diabetes-related major adverse events (60). It is also likely that the not all populations are able to engage with remote delivery of healthcare (61, 62). While some RCTs provided participants with mobile phones, computers or internet services or training (43, 44, 51, 52), most did not. There appears to be a separation between those who have access to, and the ability to understand diverse technological resources, and those who do not (‘the digital divide’) (62). For many vulnerable populations such as older persons and those from low socioeconomic, very remote and low educational backgrounds and those with physical disability and/or visual or hearing impairment, remote interventions may not be suitable or readily available. Therefore, factors such as access to the internet of things and electronic devices, user friendliness and ease of navigation of medical technology are important considerations when designing remote interventions. For some populations and certainly for some aspects of medical management, in-person models of care such as home visits are essential and therefore entirely remotely delivered models of care are unsuitable (55).
Subgroup analyses suggested that multiple components of the interventions contributed to the value of the remote management. This included patient education, monitoring of risk factors, coaching, remote consultations and pharmacological management for HbA1c and SBP. The components of patient education for TC and DBP, risk factor coaching for LDL-c, remote consultation for TC and DBP and pharmacological management for TC appeared to be less effective. Conclusions on this are however limited due to the integrated nature of all the interventions studied.
There were several limitations to this meta-analysis. First, an individual-level data analysis was not possible and thus it was not possible to analyse the effect of differing population characteristics and intervention types in detail. The interventions tested were heterogeneous and included multiple components. We sought to examine which components were more effective but since all interventions include more than one component, this analysis was incomplete. An intention to treat analysis was not possible due to loss to follow-up. Most funnel plots suggested a risk of publication bias and thus the effect of the interventions may have been over-estimated. Due to lack of consistent data, we could not perform pooled analyses of planned secondary outcomes including quality of life, cost-effectiveness, micro-vascular outcomes, limb events or MACE. A cost-benefit analysis of using remote intervention compared to standard care remains an important area of future research. Lastly, the adherence to treatments were not reported in most studies and therefore we could not evaluate the impact of adherence on outcome. There were several strengths to our study including carefully planned analyses, the inclusion of RCTs which reported on all three risk factors of interest, extensive evaluation of risk of bias and the reporting of sensitivity and subgroup analyses to evaluate relationships between subgroups and individual studies and pooled outcomes.
Conclusion
This meta-analysis suggests that remotely managing modifiable risk factors significantly lowers HbA1c, total cholesterol, LDL-cholesterol, and systolic and diastolic blood pressure in people with diabetes. Patient coaching on risk factor management and the provision of pharmacological management were identified as the most effective interventions at improving risk factor control. Further research is needed to rigorously clarify the most effective components of remote management.
Data Availability Statement
The original contributions presented in the study are included in the article/Supplementary Material. Further inquiries can be directed to the corresponding author.
Author Contributions
MF conceived the research topic, conducted the search strategy, extracted the data, analyzed the data, and wrote the manuscript. LS extracted the data, cleaned the data, and reviewed the manuscript. AD conceived the research topic, conducted the search strategy, extracted the data, cleaned the data, and reviewed the manuscript. BC extracted the data, cleaned the data, and reviewed the manuscript. JG conceived the research topic, supervised the other authors in writing the manuscript, and reviewed the manuscript. JG is the guarantor of this work, and as such had full access to the data in the study and takes responsibility for the integrity of the data and the accuracy of the data analysis. All authors contributed to the article and approved the submitted version.
Funding
This work was supported by the Townsville Hospital and Health Service Study, Education and Research Trust Account (SERTA) Fund, the James Cook University Strategic Research Investment Fund, and the Queensland Government. JG holds a Practitioner Fellowship from the National Health and Medical Research Council (1117061) and a Senior Clinical Research Fellowship from the Queensland Government, Australia. The funders played no role in study design, conduct, data collection, analysis and interpretation, and did not assist in preparation or review of this manuscript.
Conflict of Interest
The authors declare that the research was conducted in the absence of any commercial or financial relationships that could be construed as a potential conflict of interest.
Publisher’s Note
All claims expressed in this article are solely those of the authors and do not necessarily represent those of their affiliated organizations, or those of the publisher, the editors and the reviewers. Any product that may be evaluated in this article, or claim that may be made by its manufacturer, is not guaranteed or endorsed by the publisher.
Supplementary Material
The Supplementary Material for this article can be found online at: https://www.frontiersin.org/articles/10.3389/fendo.2022.848695/full#supplementary-material
References
1. Alicic RZ, Rooney MT, Tuttle KR. Diabetic Kidney Disease: Challenges, Progress, and Possibilities. Clin J Am Soc Nephrol (2017) 12:2032–45. doi: 10.2215/CJN.11491116
2. Singh N, Armstrong DG, Lipsky BA. Preventing Foot Ulcers in Patients With Diabetes. JAMA (2005) 293:217–28. doi: 10.1001/jama.293.2.217
3. Bonaca MP, Nault P, Giugliano RP, Keech AC, Pineda AL, Kanevsky E, et al. Low-Density Lipoprotein Cholesterol Lowering With Evolocumab and Outcomes in Patients With Peripheral Artery Disease: Insights From the FOURIER Trial (Further Cardiovascular Outcomes Research With PCSK9 Inhibition in Subjects With Elevated Risk). Circulation (2018) 137:338–50. doi: 10.1161/CIRCULATIONAHA.117.032235
4. Heart Protection Study Collaborative Group. Randomized Trial of the Effects of Cholesterol-Lowering With Simvastatin on Peripheral Vascular and Other Major Vascular Outcomes in 20,536 People With Peripheral Arterial Disease and Other High-Risk Conditions. J Vasc Surg (2007) 45:645–54. doi: 10.1016/j.jvs.2006.12.054
5. Anand SS, Caron F, Eikelboom JW, Bosch J, Dyal L, Aboyans V, et al. Major Adverse Limb Events and Mortality in Patients With Peripheral Artery Disease: The COMPASS Trial. J Am Coll Cardiol (2018) 71:2306–15. doi: 10.1016/j.jacc.2018.03.008
6. Golledge J, Ward NC, Watts GF. Lipid Management in People With Peripheral Artery Disease. Curr Opin Lipidol (2019) 30:470–6. doi: 10.1097/MOL.0000000000000638
7. Fernando ME, Seneviratne RM, Tan YM, Lazzarini PA, Sangla KS, Cunningham M, et al. Intensive Versus Conventional Glycaemic Control for Treating Diabetic Foot Ulcers. Cochrane Database Syst Rev (2016) 1:CD010764. doi: 10.1002/14651858.CD010764.pub2
8. Thomas Manapurathe D, Moxon JV, Krishna SM, Rowbotham S, Quigley F, Jenkins J, et al. Cohort Study Examining the Association Between Blood Pressure and Cardiovascular Events in Patients With Peripheral Artery Disease. J Am Heart Assoc (2019) 8:e010748. doi: 10.1161/JAHA.118.010748
9. Coppola A, Sasso L, Bagnasco A, Giustina A, Gazzaruso C. The Role of Patient Education in the Prevention and Management of Type 2 Diabetes: An Overview. Endocrine (2016) 53:18–27. doi: 10.1007/s12020-015-0775-7
10. Toledo FG, Triola A, Ruppert K, Siminerio LM. Telemedicine Consultations: An Alternative Model to Increase Access to Diabetes Specialist Care in Underserved Rural Communities. JMIR Res Protoc (2012) 1:e14. doi: 10.2196/resprot.2235
11. McGill M, Blonde L, Chan JCN, Khunti K, Lavalle FJ, Bailey CJ, et al. The Interdisciplinary Team in Type 2 Diabetes Management: Challenges and Best Practice Solutions From Real-World Scenarios. J Clin Transl Endocrinol (2016) 7:21–7. doi: 10.1016/j.jcte.2016.12.001
12. Espinoza P, Varela CA, Vargas IE, Ortega G, Silva PA, Boehmer KB, et al. The Burden of Treatment in People Living With Type 2 Diabetes: A Qualitative Study of Patients and Their Primary Care Clinicians. PloS One (2020) 15:e0241485. doi: 10.1371/journal.pone.0241485
13. Song HJ, Dennis S, Levesque J-F, Harris MF. What Matters to People With Chronic Conditions When Accessing Care in Australian General Practice? A Qualitative Study of Patient, Carer, and Provider Perspectives. BMC Fam Pract (2019) 20:1–13. doi: 10.1186/s12875-019-0973-0
14. Golledge J, Fernando M, Lazzarini P, Najafi B, Armstrong DG. The Potential Role of Sensors, Wearables and Telehealth in the Remote Management of Diabetes-Related Foot Disease. Sensors (2020) 20:4527. doi: 10.3390/s20164527
15. Drovandi A, Wong S, Seng L, Crowley B, Alahakoon C, Banwait J, et al. Remotely Delivered Monitoring and Management of Diabetes-Related Foot Disease: An Overview of Systematic Reviews. J Diabetes Sci Technol (2021) 19:19322968211012456. doi: 10.1177/19322968211012456
16. Su D, Zhou J, Kelley MS, Michaud TL, Siahpush M, Kim J, et al. Does Telemedicine Improve Treatment Outcomes for Diabetes? A Meta-Analysis of Results From 55 Randomized Controlled Trials. Diabetes Res Clin Pract (2016) 116:136–48. doi: 10.1016/j.diabres.2016.04.019
17. Timpel P, Oswald S, Schwarz PEH, Harst L. Mapping the Evidence on the Effectiveness of Telemedicine Interventions in Diabetes, Dyslipidemia, and Hypertension: An Umbrella Review of Systematic Reviews and Meta-Analyses. J Med Internet Res (2020) 22:e16791. doi: 10.2196/16791
18. Wu C, Wu Z, Yang L, Zhu W, Zhang M, Zhu Q, et al. Evaluation of the Clinical Outcomes of Telehealth for Managing Diabetes: A PRISMA-Compliant Meta-Analysis. Medicine (2018) 97:e12962. doi: 10.1097/MD.0000000000012962
19. Najafi B, Reeves ND, Armstrong DG. Leveraging Smart Technologies to Improve the Management of Diabetic Foot Ulcers and Extend Ulcer-Free Days in Remission. Diabetes Metab Res Rev (2020) 36(Suppl 1):e3239. doi: 10.1002/dmrr.3239
20. Marcolino MS, Maia JX, Alkmim MB, Boersma E, Ribeiro AL. Telemedicine Application in the Care of Diabetes Patients: Systematic Review and Meta-Analysis. PloS One (2013) 8:e79246. doi: 10.1371/journal.pone.0079246
21. Moher D, Liberati A, Tetzlaff J, Altman DG. Prisma Group. Preferred Reporting Items for Systematic Reviews and Meta-Analyses: The PRISMA Statement. PloS Med (2009) 6:e1000097. doi: 10.1371/journal.pmed.1000097
22. Sterne JAC, Savovic J, Page MJ, Elbers RG, Blencowe NS, Boutron I, et al. RoB 2: A Revised Tool for Assessing Risk of Bias in Randomised Trials. BMJ (2019) 366:l4898. doi: 10.1136/bmj.l4898
23. Higgins JPT TJ, Chandler J, Cumpston M, Li T, Page MJ, Welch VA. Cochrane Handbook for Systematic Reviews of Interventions Version 6.2 (Updated February 2021) (2021) (Accessed 5th July 2021).
24. Takeshima N, Sozu T, Tajika A, Ogawa Y, Hayasaka Y, Furukawa TA. Which is More Generalizable, Powerful and Interpretable in Meta-Analyses, Mean Difference or Standardized Mean Difference? BMC Med Res Methodol (2014) 14(1):30. doi: 10.1186/1471-2288-14-30
25. Higgins JP, Thompson SG. Quantifying Heterogeneity in a Meta-Analysis. Stat Med (2002) 21(11):1539–58. doi: 10.1002/sim.1186
26. Sterne JA, Gavaghan D, Egger M. Publication and Related Bias in Meta-Analysis: Power of Statistical Tests and Prevalence in the Literature. J Clin Epidemiol (2000) 53(11):1119–29. doi: 10.1016/S0895-4356(00)00242-0
27. Aytekin Kanadli K, Ovayolu N, Ovayolu O. Does Telephone Follow-Up and Education Affect Self-Care and Metabolic Control in Diabetic Patients? Holist Nurs Pract (2016) 30(2):70–7. doi: 10.1097/HNP.0000000000000137
28. Blackberry ID, Furler JS, Best JD, Chondros P, Vale M, Walker C, et al. Effectiveness of General Practice Based, Practice Nurse Led Telephone Coaching on Glycaemic Control of Type 2 Diabetes: The Patient Engagement and Coaching for Health (PEACH) Pragmatic Cluster Randomised Controlled Trial. BMJ (2013) 347:f5272. doi: 10.1136/bmj.f5272
29. Bond GE, Burr R, Wolf FM, Price M, McCurry SM, Teri L. The Effects of a Web-Based Intervention on the Physical Outcomes Associated With Diabetes Among Adults Age 60 and Older: A Randomized Trial. Diabetes Technol Ther (2007) 9(1):52–9. doi: 10.1089/dia.2006.0057
30. Crowley MJ, Powers BJ, Olsen MK, Grubber JM, Koropchak C, Rose CM, et al. The Cholesterol, Hypertension, and Glucose Education (CHANGE) Study: Results From a Randomized Controlled Trial in African Americans With Diabetes. Am Heart J (2013) 166(1):179–86. doi: 10.1016/j.ahj.2013.04.004
31. Davis RM, Hitch AD, Salaam MM, Herman WH, Zimmer-Galler IE, Mayer-Davis EJ. TeleHealth Improves Diabetes Self-Management in an Underserved Community: Diabetes TeleCare. Diabetes Care (2010) 33(8):1712–7. doi: 10.2337/dc09-1919
32. Eakin EG, Winkler EA, Dunstan DW, Healy GN, Owen N, Marshall AM, et al. Living Well With Diabetes: 24-Month Outcomes From a Randomized Trial of Telephone-Delivered Weight Loss and Physical Activity Intervention to Improve Glycemic Control. Diabetes Care (2014) 37(8):2177–85. doi: 10.2337/dc13-2427
33. Harno K, Kauppinen-Mäkelin R, Syrjäläinen J. Managing Diabetes Care Using an Integrated Regional E-Health Approach. J Telemed Telecare (2006) 12(Suppl 1):13–5. doi: 10.1258/135763306777978380
34. Holbrook A, Thabane L, Keshavjee K, Dolovich L, Bernstein B, Chan D, et al. Individualized Electronic Decision Support and Reminders to Improve Diabetes Care in the Community: COMPETE II Randomized Trial. CMAJ (2009) 181(1-2):37–44. doi: 10.1503/cmaj.081272
35. Huo X, Krumholz HM, Bai X, Spatz ES, Ding Q, Horak P, et al. Effects of Mobile Text Messaging on Glycemic Control in Patients With Coronary Heart Disease and Diabetes Mellitus: A Randomized Clinical Trial. Circ Cardiovasc Qual Outcomes (2019) 12(9):e005805. doi: 10.1161/CIRCOUTCOMES.119.005805
36. Kempf K, Altpeter B, Berger J, Reub O, Fuchs M, Schneider M, et al. Efficacy of the Telemedical Lifestyle Intervention Program TeLiPro in Advanced Stages of Type 2 Diabetes: A Randomized Controlled Trial. Diabetes Care (2017) 40(7):863–71. doi: 10.2337/dc17-0303
37. Krein SL, Klamerus ML, Vijan S, Lee JL, Fitzgerald JT, Pawlow A, et al. Case Management for Patients With Poorly Controlled Diabetes: A Randomized Trial. Am J Med (2004) 116(11):732–9. doi: 10.1016/j.amjmed.2003.11.028
38. Leichter SB, Bowman K, Adkins RA, Jelsovsky Z. Impact of Remote Management of Diabetes via Computer: The 360 Study–a Proof-of-Concept Randomized Trial. Diabetes Technol Ther (2013) 15(5):434–8. doi: 10.1089/dia.2012.0323
39. Lim SL, Ong KW, Johal J, Han CY, Yap QV, Chan YH, et al. Effect of a Smartphone App on Weight Change and Metabolic Outcomes in Asian Adults With Type 2 Diabetes: A Randomized Clinical Trial. JAMA Netw Open (2021) 4(6):e2112417–e. doi: 10.1001/jamanetworkopen.2021.12417
40. Liou JK, Soon MS, Chen CH, Huang TF, Chen YP, Yeh YP, et al. Shared Care Combined With Telecare Improves Glycemic Control of Diabetic Patients in a Rural Underserved Community. Telemed J E Health (2014) 20(2):175–8. doi: 10.1089/tmj.2013.0037
41. Nicolucci A, Cercone S, Chiriatti A, Muscas F, Gensini G. A Randomized Trial on Home Telemonitoring for the Management of Metabolic and Cardiovascular Risk in Patients With Type 2 Diabetes. Diabetes Technol Ther (2015) 17(8):563–70. doi: 10.1089/dia.2014.0355
42. Odnoletkova I, Goderis G, Nobels F, Fieuws S, Aertgeerts B, Annemans L, et al. Optimizing Diabetes Control in People With Type 2 Diabetes Through Nurse-Led Telecoaching. Diabetes Med (2016) 33(6):777–85. doi: 10.1111/dme.13092
43. Quinn CC, Shardell MD, Terrin ML, Barr EA, Ballew SH, Gruber-Baldini AL. Cluster-Randomized Trial of a Mobile Phone Personalized Behavioral Intervention for Blood Glucose Control. Diabetes Care (2011) 34(9):1934–42. doi: 10.2337/dc11-0366
44. Ramallo-Fariña Y, García-Bello MA, García-Pérez L, Boronat M, Wagner AM, Rodríguez-Rodríguez L, et al. Effectiveness of Internet-Based Multicomponent Interventions for Patients and Health Care Professionals to Improve Clinical Outcomes in Type 2 Diabetes Evaluated Through the INDICA Study: Multiarm Cluster Randomized Controlled Trial. JMIR Mhealth Uhealth (2020) 8(11):e18922. doi: 10.2196/18922
45. Shahid M, Mahar S, Shaikh S, Shaikh Z-U-D. A Randomized Controlled Trial of Mobile Phone Intervention to Improve Diabetes Care in Rural Areas. Endocr Pract (2015) 25(3):166–71.
46. Shea S, Weinstock RS, Teresi JA, Palmas W, Starren J, Cimino JJ, et al. A Randomized Trial Comparing Telemedicine Case Management With Usual Care in Older, Ethnically Diverse, Medically Underserved Patients With Diabetes Mellitus: 5 Year Results of the IDEATel Study. J Am Med Inform Assoc (2009) 16(4):446–56. doi: 10.1197/jamia.M3157
47. Tang PC, Overhage JM, Chan AS, Brown NL, Aghighi B, Entwistle MP, et al. Online Disease Management of Diabetes: Engaging and Motivating Patients Online With Enhanced Resources-Diabetes (EMPOWER-D), a Randomized Controlled Trial. J Am Med Inform Assoc (2013) 20(3):526–34. doi: 10.1136/amiajnl-2012-001263
48. Varney JE, Weiland TJ, Inder WJ, Jelinek GA. Effect of Hospital-Based Telephone Coaching on Glycaemic Control and Adherence to Management Guidelines in Type 2 Diabetes, a Randomised Controlled Trial. Intern Med J (2014) 44(9):890–7. doi: 10.1111/imj.12515
49. de Vasconcelos HCA, Lira Neto JCG, de Araujo MFM, Carvalho GCN, de Souza Teixeira CR, de Freitas RWJF, et al. Telecoaching Programme for Type 2 Diabetes Control: A Randomised Clinical Trial. Br J Nurs (2018) 27(19):1115–20. doi: 10.12968/bjon.2018.27.19.1115
50. Vinitha R, Nanditha A, Snehalatha C, Satheesh K, Susairaj P, Raghavan A, et al. Effectiveness of Mobile Phone Text Messaging in Improving Glycaemic Control Among Persons With Newly Detected Type 2 Diabetes. Diabetes Res Clin Pract (2019) 158:107919. doi: 10.1016/j.diabres.2019.107919
51. Wild SH, Hanley J, Lewis SC, McKnight JA, McCloughan LB, Padfield PL, et al. Supported Telemonitoring and Glycemic Control in People With Type 2 Diabetes: The Telescot Diabetes Pragmatic Multicenter Randomized Controlled Trial. PloS Med (2016) 13(7):e1002098. doi: 10.1371/journal.pmed.1002098
52. Yoo HJ, Park MS, Kim TN, Yang GJ, Cho TG, Hwang SH, et al. A Ubiquitous Chronic Disease Care System Using Cellular Phones and the Internet. Diabetes Med (2009) 26(6):628–35. doi: 10.1111/j.1464-5491.2009.02732.x
53. Zhou P, Xu L, Liu X, Huang J, Xu W, Chen W. Web-Based Telemedicine for Management of Type 2 Diabetes Through Glucose Uploads: A Randomized Controlled Trial. Int J Clin Exp Pathol (2014) 7(12):8848–54.
54. Zoungas S, Chalmers J, Ninomiya T, Li Q, Cooper ME, Colagiuri S, et al. Association of HbA1c Levels With Vascular Complications and Death in Patients With Type 2 Diabetes: Evidence of Glycaemic Thresholds. Diabetologia (2012) 55:636–43. doi: 10.1007/s00125-011-2404-1
55. Mach F, Baigent C, Catapano AL, Koskinas KC, Casula M, Badimon L, et al. ESC/EAS Guidelines for the Management of Dyslipidaemias: Lipid Modification to Reduce Cardiovascular Risk: The Task Force for the Management of Dyslipidaemias of the European Society of Cardiology (ESC) and European Atherosclerosis Society (EAS). Eur Heart J (2020) 41(1):111–88. doi: 10.1093/eurheartj/ehz455
56. Chou R, Dana T, Blazina I, Daeges M, Jeanne TL. Statins for Prevention of Cardiovascular Disease in Adults: Evidence Report and Systematic Review for the US Preventive Services Task Force. JAMA (2016) 316(19):2008–24. doi: 10.1001/jama.2015.15629
57. Palmer SC, Tendal B, Mustafa RA, Vandvik PO, Li S, Hao Q, et al. Sodium-Glucose Cotransporter Protein-2 (SGLT-2) Inhibitors and Glucagon-Like Peptide-1 (GLP-1) Receptor Agonists for Type 2 Diabetes: Systematic Review and Network Meta-Analysis of Randomised Controlled Trials. BMJ (2021) 372:m4573. doi: 10.1136/bmj.m4573
58. Emdin CA, Rahimi K, Neal B, Callender T, Perkovic V, Patel A. Blood Pressure Lowering in Type 2 Diabetes: A Systematic Review and Meta-Analysis. JAMA (2015) 313(6):603–15. doi: 10.1001/jama.2014.18574
59. Verma S, Bain SC, Buse JB, Idorn T, Rasmussen S, Orsted DD, et al. Occurence of First and Recurrent Major Adverse Cardiovascular Events With Liraglutide Treatment Among Patients With Type 2 Diabetes and High Risk of Cardiovascular Events: A Post Hoc Analysis of a Randomized Clinical Trial. JAMA Cardiol (2019) 4(12):1214–20. doi: 10.1001/jamacardio.2019.3080
60. Standards of Medical Care in Diabetes-2017: Summary of Revisions. Diabetes Care (2017) 40(Suppl 1):S4–5. doi: 10.2337/dc17-S003
61. Lam K, Lu AD, Shi Y, Covinsky KE. Assessing Telemedicine Unreadiness Among Older Adults in the United States During the COVID-19 Pandemic. JAMA Intern Med (2020) 180(10):1389–91. doi: 10.1001/jamainternmed.2020.2671
Keywords: blood pressure, cholesterol, lipids, systematic review, telehealth
Citation: Fernando ME, Seng L, Drovandi A, Crowley BJ and Golledge J (2022) Effectiveness of Remotely Delivered Interventions to Simultaneously Optimize Management of Hypertension, Hyperglycemia and Dyslipidemia in People With Diabetes: A Systematic Review and Meta-Analysis of Randomized Controlled Trials. Front. Endocrinol. 13:848695. doi: 10.3389/fendo.2022.848695
Received: 04 January 2022; Accepted: 17 February 2022;
Published: 15 March 2022.
Edited by:
Fariba Ahmadizar, University Medical Center Utrecht, NetherlandsReviewed by:
Soroush Mohammadi Jouabadi, Erasmus Medical Center, NetherlandsSepideh Babaniamansour, University of Michigan, United States
Peter Penson, Liverpool John Moores University, United Kingdom
Copyright © 2022 Fernando, Seng, Drovandi, Crowley and Golledge. This is an open-access article distributed under the terms of the Creative Commons Attribution License (CC BY). The use, distribution or reproduction in other forums is permitted, provided the original author(s) and the copyright owner(s) are credited and that the original publication in this journal is cited, in accordance with accepted academic practice. No use, distribution or reproduction is permitted which does not comply with these terms.
*Correspondence: Jonathan Golledge, Sm9uYXRoYW4uR29sbGVkZ2VAamN1LmVkdS5hdQ==