- 1Horizon Digital Economy Research, University of Nottingham, Nottingham, UK
- 2Warwick Business School, University of Warwick, Coventry, UK
- 3Institute of Neurology, University College London, London, UK
To solve problems such as climate change, every little push counts. Community energy schemes are a popular policy targeted to reduce a country’s carbon emissions but the effect they have on energy use depends on whether people can work together as a community. We often find ourselves caught in a dilemma: if others are not doing their bit, why should I? In our experiment, participants (N = 118) were matched in groups of 10 to play in a collective-risk game framed as a community energy purchase scheme. They made only one decision about energy use for their virtual household a day, while a full round of the game lasted 1 week in real time. All decisions were entered via personal phone or a home computer. If in the end of the week the group exceeded a pre-paid threshold of energy use all group members would share a fine. Each day participants received feedback about decisions of their group partners, and in some groups the feedback was manipulated as high (unfair condition) or low (fair condition) use. High average group use created individual risk for participants to be penalized in the end of the week, even if they did not use much themselves. We found that under the risk of having to pay a fine, participants stayed significantly below the fair-share threshold regardless of unfair behavior of others. On the contrary, they significantly decreased their consumption toward the end of the game. Seeing that others are doing their bit – using a fair-share – encouraged people to take advantage of the situation: those who played against fair confederates did not follow the normative behavior but conversely, increased their consumption over the course of the game. These opportunistic strategies were demonstrated by impulsive participants who were also low in punishment sensitivity. We discuss the findings in the light of policy research as well as literature on cooperation and prosocial behavior.
Introduction
Many environmental choices represent social dilemmas (Irwin and Berigan, 2013), whether they are large-scale decisions about climate change mitigation (Milinski et al., 2008) or everyday choices such as recycling (Lyas et al., 2004) and responsible energy use at home (Leygue et al., 2014). Social dilemmas are scenarios when the communal resources have to be maintained and individuals face a dilemma between either using more than others, while sharing the costs of usage equally – thus, free-riding; or using a fair-share that allows maintaining the consumption of a resource but often with smaller immediate personal benefits. A type of social dilemma – a collective-risk game – is relevant to various social scenarios with repeated interactions and previously has been studied in the context of climate change mitigation (e.g., Milinski et al., 2008). Understanding how people act in dilemmas, such as climate change mitigation, is of high importance. However, realization of policy makers’ decisions relies as much on small everyday choices of regular people as on large-scale choices about climate change mitigation by the leaders of policy making. Currently, there is not enough understanding of what people will do given various policy scenarios. We introduce a novel “in-the-wild” design of a social dilemma experiment. It takes an experimental laboratory game to everyday environments while still keeping the structure of the experimental social dilemma game. Through revealing people’s behavioral strategies in the situations that resemble real-world scenarios while keeping experimental control, this approach can serve as an alternative or a precursor to expensive field studies helping to understand barriers and enablers of behavior change in the domain of energy use as well as other domains of behavior.
Community Energy Purchase Schemes
Cooperation around environmental resources is vital. For example, to achieve an 80% reduction of carbon emissions target by 2050, UK energy end-users – households, businesses, and third sector – are expected to use energy more efficiently, which among other measures includes better management of supply and demand. The benefits of encouraging communities to engage in managing their energy consumption is outlined in UK government’s first Community Energy Strategy (DECC, 2014), which presents a range of initiatives that are to be supported going forward. One of these initiatives is collective purchasing, which “can make things cheaper, as buying in larger volumes usually means better deals and lower prices” (www.gov.uk/government/policies). Although examples are few and far between, the personal financial benefits to those who participate in collective purchasing have been demonstrated (Conaty and Mayo, 2012): for example, in the UK, an average saving of £131 was realized by households on the Cheaper Energy Together scheme [DECC, 2013; for similar evidence from Belgium, see Erbmann et al. (2009)]. Importantly, collective purchasing initiatives could also help to achieve carbon emissions targets by increasing engagement of community members in energy issues, and by reducing a variety of related emissions (e.g., the reduction in emissions related to the delivery of fuel, DECC, 2014, p. 6).
However, community energy purchase schemes can introduce interdependence of individual decisions, and so participating households might face a scenario alike a standard social dilemma. This is not accounted for in the policy documents, which focus on the positive outputs of a community purchasing initiative. Researchers have shown that near-future changes in energy infrastructure, e.g., forthcoming smart meter rollouts in the UK, will make it easier to identify which consumers might benefit by forming collectives (Vinyals et al., 2012) and what the tools might look like that help collectives deal with energy retailers (Ramchurn et al., 2013). However, in reality communities can be transient and marginalizing (Harvey and Braun, 1999), particularly to those not predicated toward collective action (Hoffman and High-Pippert, 2010), and reactions to energy initiatives by different communities will not necessarily remain positive (Walker et al., 2010).
If one of the households in the community, despite an agreement, uses unreasonably high amounts of energy and if there is no opportunities to punish the free-rider (Fehr and Gachter, 2002), will the rest of the community compensate for them by using less? Or will they retaliate and use more themselves, causing a rebound effect (Greening et al., 2000), thus eliminating the benefits of the deal secured by the community? Furthermore, if some households use a fair-share amount, will others follow an establishing norm of cooperation in the group? The present study used a collective-risk game, a type of experimental social dilemma, to model a communal energy purchase scenario “in the wild.” We investigated participants’ responses to free-riding or fair-share use in their group as they were going about their everyday lives, as well as what consequences the dynamic interactions over communal resources had on cooperation around energy use.
Social Dilemmas to Explain Environmental Decisions
While standard social dilemmas are conceptually applicable to many real-world scenarios, the predominant body of research in the area uses stripped down storylines where participants make decisions about money units (MUs, e.g., Fehr and Gachter, 2002). Building on previous research (Milinski et al., 2008; Jacquet et al., 2013; Leygue et al., 2014), we transformed a laboratory game to investigate whether previous experimental findings apply to more realistic, ecologically valid real-world choices. Such an approach can build a basis for establishing the constraints of current policy strategies on behaviors in schemes such as community energy purchase deals. We improved the design of the laboratory game by introducing a novel “in the wild” aspect, which aimed to enhance the ecological validity of the experiment where the decisions were made on a timeline that is closer to real-world scenarios, as well as in the familiar environment of participants’ everyday life.
A collective-risk game is a scenario where a group of players interact over a course of several rounds. They are given individual endowments and have to accumulate (or save, depending on the framing) a certain amount of money in the public pot over the course of the game. If by the end of the game they do not collect enough money (or if they overspend), they are all fined equally. We applied a collective-risk game approach to study household energy decisions by simulating everyday choices in a controlled experimental design. Will a group of households participating in a community energy purchase scheme with a pre-defined limit of energy allowance manage to stay below the threshold, given the benefits of individual use? To study this question, we modified a previously reported design (Milinski et al., 2008) to fit a community energy-buying storyline. In our experiment, if the group went over the threshold, the fine occurred with 100% probability. The fine was distributed among group members regardless of their usage from the communal resource, which is similar to previous research using experimental public goods games (e.g., Croson, 2007). Such a scenario simulates more realistically the case of community energy purchase as, unlike climate change that can happen with a particular probability, energy use in real life can be measured objectively, so its over-usage would be fined with 100% certainty.
In previously reported collective-risk games, participants interacted over a number of turns with the aim to reach a collective target of contributions (Milinski et al., 2008; Santos and Pacheco, 2011; Jacquet et al., 2013). Such give-some scenarios model social decision-making in situations such as climate change mitigation (e.g., everybody needs to contribute enough to prevent a catastrophe of climate change). However, there are many real-world scenarios in for example, community buying schemes or household energy use, which could be better represented by take-some games (Leygue et al., 2014). In these scenarios, a community has to maintain the use of a communal resource under a certain threshold to avoid negative consequences, such as exhausting the resource (Van Dijk et al., 2003) or paying a fine for over-usage.
Importantly, similar to many real-life situations, in the collective-risk game participants receive frequent feedback about the behavior of others in the group throughout the game; however, the outcome for the whole game was only evident in the end. This introduced a dilemma to each individual group member. If the target was not met, the whole group suffered: every individual had to contribute equally to the fine. However, by using more individually, participants received greater private benefit. This could be especially tempting in the short-term given the structure of the game: participants were rewarded through individual usage on each turn, but rewards for cooperation or punishment for not meeting the target were distant and were revealed only in the end of the game. Such a set up gives an opportunity to study how participants react to the behavior of others and adjust their game strategy if necessary in order to reach the target. While achieving the collective target implies some individual sacrifice, it brings benefits to everybody in the group. However, there is always uncertainty for the individual about whether others in the group choose to cooperate or to free ride. Furthermore, collective-risk games allow the study of strategies that are dependent on the behavior of others. For example, one can compensate for free-riding of others (Milinski et al., 2008). Alternatively, one can also be opportunistic and expect others to compensate. Thus, the key feature of this experimental design is to observe how the behavior of others can affect people’s choices in the game.
The behavior of others often serves as a cue eliciting certain norms of interaction, which people then can choose to follow (Biel and Thøgersen, 2007), and this is applicable to social dilemmas (Weber and Murnighan, 2008). However, do people always follow the example of the majority? Research on social norms, including energy use domain, suggests that the majority comply with normative usage after seeing the information about others’ behavior (Schultz et al., 2007). Feedback about behavior of others is referred to as a descriptive norm of behavior, which in addition to injunctive norms (rules or standards of behavior), is suggested to affect people’s choices. However, the feedback about behavior of others does not always affect decisions in a positive way, especially in the household energy use domain (Leygue et al., 2014). Field studies on household energy use also report “rebound” effects: if people find out that others use more than them, in some circumstances they can increase but not decrease their use (Schultz et al., 2007). One potential explanation for this rebound effect relates to scenarios perceived as social dilemmas where high usage by others could be perceived as unjust. In this case, instead of following the majority and using a fair-share, individuals could increase their usage in retaliation toward free-riders.
Strategies to Deal with Unfair Behavior of Others
Fairness is an important principle of human interactions. It is pervasive throughout human society: we often expect others to behave in a way that is fair to us and others (Binmore, 2014). Strong reciprocity theory suggests that violation of the fairness principle evokes strong negative emotional and behavioral reactions such as altruistic punishment of free-riders (Fehr and Gachter, 2002). Ultimatum game (UG) experiments are specifically designed to study people’s reactions to fair or unfair behavior of others. One out of two players is required to divide a pot of money in two parts and the other needs to approve the outcome for both of them to receive the allocation. Around half of participants in UGs refuse the offer, which they consider unfair, even though in this case both parties get nothing (Nowak et al., 2000), which is a way to retaliate in response to free-riding. Leygue et al. (2014) found that when faced with a hypothetical scenario in which one house member overused energy and everybody has to share the bill for their overuse, participants report heightened anger. But would they retaliate and increase their energy use, as strong reciprocity theory suggests? Many social dilemmas in the real world differ from one-shot UGs as we often interact with the same individuals over a number of occasions. Retaliation in such circumstances can have negative effects on the outcome of interactions, especially if there is no opportunity to directly punish free-riders: often retaliation causes complete elimination of cooperation (Fehr and Fischbacher, 2003). This is a highly undesirable outcome for various real-life situations, including community energy purchasing scenarios. Luckily, there are other strategies to deal with free-riding that are also at play in social dilemmas (Axelrod and Hamilton, 1981; Fudenberg et al., 2012).
The literature reports a variety of “nice” strategies in social dilemmas, which under certain circumstances lead to better payoffs for the individuals employing them. In the repeated prisoner’s dilemma between one- and two-thirds of participants, depending on conditions, demonstrate lenient strategies by not retaliating to defection straightaway and forgiving strategies by attempting to restore cooperation after inflicting punishment on the free-rider (Fudenberg et al., 2012). Furthermore, in a repeated social dilemma experiment, participants who consistently contribute a high amount to the communal account influence others through establishing a norm of cooperation in the group at no cost to themselves and often with some gain, which subsequently leads to increase in cooperation levels in those groups (Weber and Murnighan, 2008).
Previous research on collective-risk dilemmas has not looked into strategies in response to fair or unfair behavior of others, as well as whether normative behavior presented as feedback about decisions of others influences individual choices. However, Milinski et al. (2008) demonstrated that if the punishment was highly probable, more participants showed altruistic or compensating strategies, while if the punishment was expected with a low probability, a higher proportion of participants were opportunistic or free rode. This is relevant because similar to high versus low probability of punishment, unfair versus fair behavior of others throughout the game in the collective-risk dilemma, respectively, could be perceived by an individual as a higher versus lower chance of having to pay a fine in the end.
We predicted that in the community energy purchase game when others are using a fair-share [similar to Milinski et al. (2008) uncertain punishment condition], participants would realize that the fine is not likely to occur, so they could increase their usage, thus demonstrating opportunistic strategies. An alternative reaction to fair-share behavior of others would be adherence to the social norm of behavior (Biel and Thøgersen, 2007; Schultz et al., 2007) and usage of a fair-share amount.
When others are unfair, in the absence of opportunity to directly punish free-riders, two reactions are possible. First, in accord with retaliation literature, participants in the community energy purchase game could employ an emotionally driven retaliation strategy to punish free-riders or increase their usage. However, this behavior is highly undesirable from the rational point of view as it increases the risk of not meeting the target and, thus, might lead to punishment in the form of a fine for everybody. Thus, similar to Milinski et al. (2008) in certain punishment conditions, participants could use an alternative strategy and decrease their usage or compensate if others were unfair.
Individual Differences in Social Dilemma
While describing behavioral strategies in scenarios that resemble real-world situations – such as communal energy use – can help to explain and predict cooperative and non-cooperative outcomes for the group, it is equally important to understand the individual motivations behind people’s decisions. Overall, research shows heterogeneity of behavioral strategies in various types of social dilemmas (e.g., Burlando and Guala, 2005; Zhao and Smillie, 2014); however, this heterogeneity has not been yet explored in the real-world social dilemmas, such as communal energy use. To identify potential mechanisms, we review literature on individual differences in behavior in lab-based social dilemmas.
The heterogeneity in decisions in social dilemmas has been linked to a number of psychological factors, such as personality dispositions, which reflect individual differences in processing rewards and punishments (Scheres and Sanfey, 2006; Skatova and Ferguson, 2011, 2013). Dispositional reward and punishment sensitivities are key to explain individual behavior in domains where reward and punishment processing have been strongly implicated, such as prosociality and cooperation (Gintis et al., 2003; Gneezy and Fessler, 2012; Van Lange et al., 2014; Zhao and Smillie, 2014). A psychological measure that has often been used to assess individual differences in reward and punishment sensitivity includes behavioral approach system (BAS) and behavioral inhibition system (BIS) scales (Carver and White, 1994).
The BAS scale includes three subscales: two subscales measure reward reactivity aspects of reward sensitivity [BAS-reward responsiveness (BAS-RR) and BAS-drive (BAS-D)], and one measures the impulsivity aspect of reward sensitivity [BAS-Fun Seeking (BAS-FS)]. Impulsivity is associated with the tendency to engage in behaviors which are risky and often require disinhibition, while reward reactivity refers to propensity to be sensitive to opportunities for rewards and rewarding experiences (see Smillie et al., 2006, for discussion of the distinction between reward reactivity and impulsivity). These scales were previously used to explain behavior in the economic games (Scheres and Sanfey, 2006; Skatova and Ferguson, 2011, 2013) and, thus, should be applicable for explaining behavior in a collective-risk game scenario structured around communal energy use. Specifically, participants who self-reported high sensitivity to rewarding experiences (success, social interactions, etc.) in everyday life also demonstrated more strategic behavior in social dilemmas. Skatova and Ferguson (2011) showed that high BAS-RR was associated with lower contributions in a one-shot public goods game after revealing that others in the group contributed a high amount. Scheres and Sanfey (2006) found associations of BAS-RR and BAS-D with lower offers in the Dictator Game (which is similar to the UG except that the respondent does not have an opportunity to reject the offer) but not in the UG. Pothos et al. (2011) showed that participants high in BAS-RR were more likely to defect in the one-shot prisoner’s dilemma game. In all cases, participants high in reward reactivity, made a decision to defect while having full control of the situation and no dependency on the decision of other people. Therefore, their decision to defect could be interpreted as strategic and reflect the ability to better learn from reward, which they were getting in this case by defecting.
Previous studies that looked into associations between BAS scales and behavior in one-shot economic games did not find relationships between BAS-FS and individual choices. The BAS-FS scale has strongest conceptual and empirical links with impulsivity and diminished delayed reward gratification (Smillie et al., 2006; Giovanelli et al., 2013). Individual differences in behavior might be associated with differences in reward discounting when each turn of the game introduces a conflict between short-term private benefit and long-term reward by cooperation. Jacquet et al. (2013) demonstrated that discounting mechanisms affected people’s decisions in a social dilemma: a greater delay in achieving rewards by cooperation made it less likely for people to cooperate in the short term in a collective-risk dilemma. Specifically, when individuals received benefits from cooperation the day after they played the game, 7 out 10 groups succeeded in reaching a cooperation target. However, when the benefits from cooperation were delayed by 7 weeks, only 4 groups out of 11 succeeded. They also demonstrated variability in individual responses: some groups were able to reach cooperation even when the benefits were delayed by 7 weeks. Previous research using public goods games found a negative association between cooperation and impulsivity but only when the reward from free-riding was tangible (Myrseth et al., 2015). It is plausible that in a game with a longer time span, where it is easier to free ride at a given turn and get away with it, BAS-FS would be associated with more selfish behaviors. That should happen especially when the risk of loss is low, as for impulsive individuals it would be easier to disregard long-term benefits of cooperation. Instead, BAS-RR and BAS-D should be positively associated with strategic behavior, leading to high certain profits in any case.
Differences in decisions in social dilemmas were associated with low self-reported sensitivity to negative experiences in real life (e.g., social disapproval, failure, etc.) measured by the BIS scale: participants with low BIS made smaller contributions in a one-shot social dilemma while facing the risk of punishment (Skatova and Ferguson, 2013). Low BIS was also associated with higher proportion of contributing nothing in a one-shot social dilemma after finding out that others contributed a high amount to the public good (Skatova and Ferguson, 2011). Finally, research suggested that interaction between BIS and BAS traits, or broadly speaking reward and punishment sensitivity systems, is associated with various clinical and behavioral outcomes, including prosocial and antisocial behavior. Specifically, McCabe et al. (2001) demonstrated that cooperation occurs through a neural network, which provides binding joint attention to mutual gains with inhibition of immediate reward: those who cooperate inhibit the dominant response of getting a quick smaller reward in order to gain a larger delayed reward by the means of cooperation.
We predicted that in the situation when others were fair and used a small amount throughout, making the risk of group punishment for overuse low, those who were higher in BAS-FS should take advantage and use more energy to get more private immediate benefits. We predicted that if others were unfair (by using high amounts throughout the game) and the risk of a fine was high, more strategically driven participants (e.g., high in BAS-RR and BAS-D) should use less to avoid paying the fine. In terms of BIS, we predicted that those who were less sensitive to the risk of punishment (e.g., low in BIS) should use more energy when the punishment was uncertain, i.e., in the fair condition. Finally, we predicted that participants high in impulsivity (measured by BAS-FS) and low in punishment sensitivity (measured by BIS) would be more likely to demonstrate opportunistic strategies when the advantage of immediate benefits were high (e.g., in the fair feedback condition).
The Present Study
Our study extended previous research to reveal whether the fair (using the pre-agreed amount of energy) or unfair (using more energy than was pre-agreed) behavior of others influenced individual decisions over the course of a collective-risk social dilemma. Specifically, we employed an experimental game to model household energy use in the context of a community energy deal, where individuals were part of a group of households that collectively pre-paid for a certain amount of energy to use per week. If the group overused energy, a fine was distributed between all group members equally.
We manipulated feedback about the behavior of others as fair or unfair, and investigated how such feedback influenced participants’ individual decisions about energy usage in their own households, resulting in a variety of strategies: fair-share (to use as much as established by social convention), opportunistic (use more to gain private benefit even at a risk of a group-level fine), retaliatory (increase the usage after facing unfair behavior of others), or compensatory (decrease the usage in order to compensate for high use of others and so avoid the fine). We further looked at whether the different strategies were associated with individual differences in punishment (measured by BIS) and two distinct aspects of reward sensitivity: reward responsiveness (measured by BAS-RR and BAS-D) and impulsivity (measured by BAS-FS).
Our participants made decisions through a smart-phone or a home PC while going about their everyday lives as opposed to interacting with other group members in laboratory settings. In addition, unlike in laboratory settings, where participants usually make decisions every minute, our participants replied just once a day in the morning, wherever they were at the moment, and by using their mobile phone or computer at home. This is an important feature of the study as it aimed to reveal potential conflict between short-term and long-term benefits: participants were rewarded for their energy use every day, while the bill revealing potential excess would arrive only in the end of the (actual) week. Such features of the game provided a more accurate simulation of real-world decisions. The data presented in this paper are a subset of data collected within the project. Here, we focus on details of the design that are relevant to the aims presented in this paper.
Materials and Methods
Participants and Procedure
The study was conducted through Qualtrics software. Overall, 118 UK-based participants volunteered to participate (74 females; age: range 25–66, M = 35; 46 were homeowners). Out of 118, 78 participants partook in fair and unfair condition, N = 39 for each condition. For all analyses below, we used only data from these 78 participants (see Design for further explanation why only fair and unfair conditions were focus of analyses in this paper).
We aimed to recruit participants who were responsible for paying their own bills as for them the decisions in the game would have greater resemblance to real life. For that reason, we explicitly sought to recruit a non-undergraduate sample of participants. In the UK, students often have their energy bills included as a part of a rental contract. In these circumstances, there is no monetary incentive to use energy responsibly (as they pay the same amount in any case). Participants were recruited in two cities in the Midlands, UK via various university-wide mailing lists and a list of members of an energy trial conducted by a national energy company.
All participants were briefed and debriefed in person. At the briefing, they received full instructions and could try out the game. They also filled in demographic information and a BIS/BAS questionnaire. We used the BIS/BAS questionnaire (Carver and White, 1994) to measure differences in reward and punishment sensitivities. Participants rated various statements on a 4-point scale ranging from “very true for me” to “very false for me.” BIS/BAS questionnaire was scored as four scales: BIS scale (M = 3.02, SD = 0.52, α = 0.77, seven items, example item: “Criticism or scolding hurts me quite a bit”) and three BAS scales: BAS-D (M = 2.67, SD = 0.60, α = 0.74, four items, example item: “I go out of my way to get things I want”), BAS-FS (M = 2.81, SD = 0.58, α = 0.72, four items, example item: “I often act on the spur of the moment”), and BAS-RR (M = 3.38, SD = 0.47, α = 0.73, five items, example item: “When I’m doing well at something I love to keep at it”). Table 1 reports zero-order correlations between BIS and BAS subscales. For the presentation of results, BIS/BAS scores were reversed; so high rating represents high ends of the BAS and the BIS scales. There were no differences on any of BIS/BAS scales between conditions. All scales were z-scored for all analyses.
All participants who completed the study were compensated £40 (~$61) for their time. In addition, they were incentivized by being paid contingent on their choices in the experiment (see The Game). In the end, participants were paid additional £3 (~$5) on average based on their responses. The study was approved by the School of Computer Science Ethics Committee at The University of Nottingham.
Design
Participants were divided into three conditions: fair, unfair, and real. Each condition included 4 groups of 10. As only 118 participants were recruited, two participants were lacking to form 12 full groups of 10. However, for fair and unfair conditions, it did not matter if there was not a full group of 10 as the feedback about group behavior was pre-set. Therefore, we assigned 39 participants for each of manipulated conditions. Thus, we manipulated the feedback about the behavior of others in 8 out of 12 groups in a between-subjects design as fair versus unfair usage. During the game, participants in the “fair” condition received feedback, which indicated that others in their group consumed energy within the pre-agreed norm, i.e., the group’s deal allocation. The feedback was generated to represent a plausible distribution with a mean of 3.7 energy units (EUs) and SD of 0.48 EUs. The mean and SD was estimated based on the pilot study data. In the “unfair” condition, feedback indicated that their group partners consumed more than was pre-agreed (simulated in the similar way to fair condition, M = 4.4 EUs, SD = 0.47 EUs). The exact feedback on each day for each condition can be found in the Supplementary Materials. In the “real” condition, participants received accurate feedback about the consumption of others in their group (M = 3.87 EUs, SD = 0.90 EUs). To avoid deception, it was explained to participants prior to the study that some groups would receive manipulated feedback but neither experimenter nor they would know which group they were assigned to (Bardsley, 2000). As the purpose of this paper was to investigate the effect of fair and unfair behavior of others on individual strategies in a collective-risk dilemma, here we only report the results for fair and unfair conditions.
The Game
The Village Energy Deal
Participants had to imagine that they and nine other households in their virtual village were participating in a deal to purchase energy communally. The deal lasted for a week and provided a pre-paid energy amount for the village (the group of 10 households), i.e., 280 EUs shared across the whole group. Each participant received a 62-MU endowment, of which they were deducted 28 MUs for inclusion in the pre-paid deal, leaving a remainder of 34 MUs in their private account. This remainder could be spent on excess energy use (in response to hypothetical situations encountered in the game, as described later), or saved to be converted into pounds in the end of the experiment at a rate of 1 MU = £0.02. Excess energy use, i.e., any energy used over the village’s 280 EU allowance, was twice as expensive as energy paid for through the village’s deal, costing 2 MUs per 1 EU. The cost of any excess energy that was used had to be paid for communally, divided equally between all group members.
Using Energy
The only way participants used energy during the game was by setting heating in their individual virtual households. The heating was set in heat points (HPs) that reflected a subjective energy scale from very cool (1 HP) to very warm (6 HPs). HPs were introduced (as opposed to degrees Celsius or Fahrenheit) as people have different subjective perceptions of what warm or cold feels like. For example, for somebody 18°C at home might seem as quite “warm,” while for somebody else it might seem as “cold” (Li, 2005). The use of 1 HP resulted in expenditure of 1 EU.
Participants received private incentives to heat their homes: 1 HP used added 0.25 MUs to their private account. That meant the more energy they used, the more monetary benefits they would receive after the end of the game. Participants were told that all households in the group were similar in the level of energy efficiency and how much energy they used regularly.
As a result, if all participants kept their use to the norm, as suggested by the rules of the village’s energy deal (i.e., up to 4 HPs per day, for 7 days), the group would not consume excess energy and not have to pay extra at the end of the week. If the group overused, all participants had to share a fine (i.e., pay for the excess), regardless of their individual use. Therefore, the scenario represented a social dilemma, where private interest (to use as much as possible in order to gain a monetary incentive) clashed with public concern (to keep the use down in order to avoid a collective fine).
Playing the Game
Participants were instructed that one round of the game lasted a week, with seven turns. One turn took place each day of the week. In the morning of each day of the study, participants received a text message or an email with a link that they had to follow to engage in the game. The link provided the following information:
• A recap of the previous day, including the average energy consumption of other members of the village; a reminder of how much they used themselves; how many MUs they received as a benefit from previous day’s use;
• A summary of the week so far, including how much the village had consumed, how much was left in the community deal allocation for the week, and how many days were left in the week.
Following this feedback, participants had to make one decision about temperature in their virtual house for this day. This consisted of choosing a temperature setting from a scale ranging from very cool (1 HP) to very warm (6 HP).
Participants were also provided with a background story to make their hypothetical day-to-day decisions feel more real. Prior to the study, we asked participants to name three close real-life friends and/or family members who might come to visit them at Christmas. We used these names to individualize the reminders sent to participants during the game, telling participants that it was Christmas time and that those friends and/or family members had come to stay with them. Their additional goal in the game was then to make their guests happy by keeping the house warm, while also attempting to save the money in their private account by avoiding the costs incurred by the group exceeding their deal’s communal allowance.
On the eighth day of the game, participants received information about energy consumption for the preceding week, learnt whether the group had exceeded its deal’s allocation and, thus, whether they needed to contribute a payment towards the fine, if there was a fine, and how much they had to pay. In addition to the decisions about energy use in their virtual house, we also measured a number of psychological variables before and after participants set the temperature every day. As these variables were not the focus of this paper, we are omitting them from any further analyses or discussion. After the first week of the study, participants participated in an extension of the game with the same group partners. Only results from the first week are reported in this paper. A complete design of the project is available from the first author. At the end of the study, participants were rewarded based on the MUs remaining in their private accounts plus the participation fee.
Results
Response Rate
Participants responded on 6.4 days on average and there was no difference in response rate between conditions: t (68.77) = −0.62, p = 0.54. The rules of the game stated that if participants missed a response on a particular day, the temperature they set for the previous day would be carried over. We excluded from analyses participants’ data when they missed more than one response during the week. Eighty-five percent (N = 66) of participants responded on at least 6 days of the study. Only these responses were used for all further analyses. Whenever we presented aggregated responses from a specific day of the study, we also excluded participants who did not provide responses on that specific day from all relevant analyses. Response rate for each day of the study for those who responded on at least 6 days for the study was the following: 98% (N = 65) participants provided responses responded on day 1, 97% (N = 64) on day 2, 98% (N = 65) on day 3, 100% (N = 66) on day 4, day 5, and day 6, and 74% (N = 49) provided responses on day 7. The lower response rate on day 7 can be explained by failure of experimental software on that day. On that specific day, the reminder that went out to participants contained a link with incorrect feedback information. Most participants responded to this incorrect reminder, but we had to disregard those responses. Later in the same day, participants received a correct link with a request to respond again, however, not everybody responded to this second reminder. The response rate to the second reminder on day 7 was similar across conditions: 24 participants responded in the fair and 25 in the unfair condition. We further checked that all our results remained the same if we ran analyses on a restricted sample of those who responded on day 7 (results are presented in Tables 3 and 4).
Average Use Compared to the Fair-Share
Mean use across the week was 3.5 HPs (SD = 0.88) (see Table 2 for means and SDs of energy use per day, overall and per condition and Figure 1 for graphical representation). Individual use fell in the range of all possible options: participants used from 1 to 6 HPs, with 51% of all responses falling on the choice of 4 HPs, 31%, 3 HPs; 9%, 2 HPs; 3%, 1 HPs; 4%, 5 HPs; and 2%, 6 HPs. As 4 HPs seemed to be a common option for many (which is not surprising as it was suggested as a normative expenditure in the instructions), first we investigated whether the average behavior in the game deviated from a fair-share usage (i.e., a choice of 4 HPs), and whether there were any differences between conditions in this respect. On average, participants in the fair condition used 3.61 HPs during the week (SD = 0.62), while participants in unfair condition used 3.38 HPs (SD = 0.58). Both values were significantly lower than the suggested “norm” of 4 HPs: one-sample t-test comparing a mean usage in each condition to 4: t (32) = 33.48, p = 2.2e−16 for fair condition, and t (32) = 33.41, p = 2.2e−16 for unfair condition. This suggests that most people did not overuse energy to make private profits – they used a fair-share or up to 4 HPs – even though using as much as possible (up to 6 HPs) would be rational due to the structure of the game.
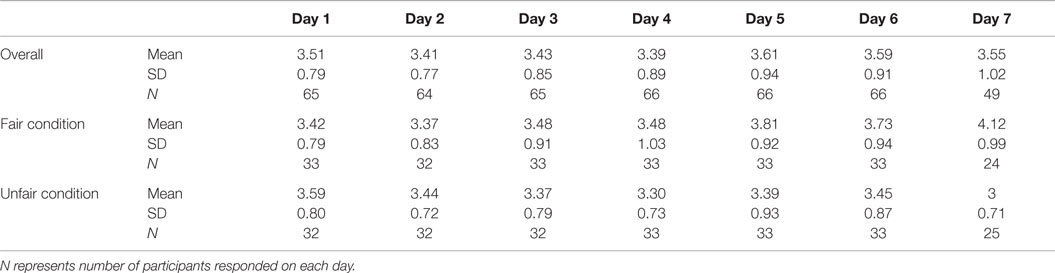
Table 2. Means and SDs of energy use for each day of the week for the whole sample, fair and unfair condition.
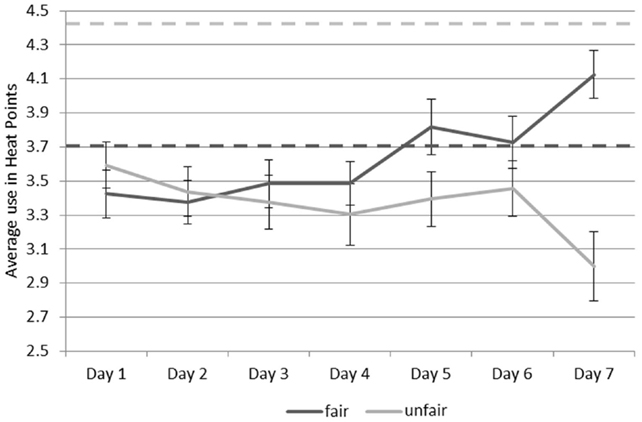
Figure 1. Average use of energy on each day of the week in fair and unfair condition. Error bars represent SE of the mean. Dotted lines show manipulated groups’ average (fair versus unfair).
Differences in Use in the Fair Versus Unfair Condition
Furthermore, we investigated the use for the week day-by-day for fair and unfair conditions separately. There was no difference in day 1 use between fair (M = 3.42, SD = 0.79, SEM = 0.14) and unfair (M = 3.59, SD = 0.80, SEM = 0.14) conditions: t (62.91) = −0.86, p = 0.39. To investigate whether fair or unfair condition had an effect on individual use, we ran a mixed-effects random intercept regression model (Model 1) estimated by maximum likelihood using lme4 package in R (Bates et al., 2014) predicting energy use on each day from condition (fair, coded as “0,” versus unfair, coded as “1”). The regression included random intercept for each participant to account for dependency between observations. We controlled for the use on day 1 to account for individual baseline. We also controlled for learning effects through using day number as a predictor: it is possible that participants would change their energy use across the week as they learn about the game and behavior of others. Predictors entered into all interaction terms were mean-centered to reduce multicollinearity.
The results (see Table 3) demonstrated that significant predictors of use were the consumption on day 1 (B = 0.38, SE = 0.05, p = 0.00001), condition (B = −0.32, SE = 0.11, p = 0.007), and interaction of the day of response by condition (B = −0.15, SE = 0.03, p = 0.00001). Table 2 reports means and SDs per condition per day of response reflecting a steady increase of overall use across the week and differences in the pattern of use for conditions: the use in the fair condition increased toward the end, while there was relative lack of change in use by participants in the unfair condition across all days apart from drastic decrease in use on the last day before the end of the game, day 7. This suggests that there was a general trend of increase in HP use over the week, however, it was reversed for unfair condition: participants in the unfair condition decreased their use toward the end of the week. Specifically, 68% decreased their use on the last day compared to the first day, 20% did not change, and 11% increased their use in the unfair condition, while 63% increased their use on the last day compared to the first day, 20% did not change, and 18% decreased their use in the fair condition. The results remained the same for the restricted sample (see Table 3).
Individual Differences and Strategies in the Game
We further investigated whether individual differences in change of strategies in the games can be attributed to personality traits. We predicted energy use on each day and used the same specification for the mixed-effects random intercept regression model as Model 1 to which we added personality traits predictors and interactions of personality with other effects. Model 2 included main effects of BIS and all BAS subscales; two-way interactions between BIS and each BAS subscale; two-way interactions between each personality subscale and day number; two-way interactions between each personality subscale and condition; three-way interactions between each personality subscale, day number, and condition; three-way interactions between BIS, each BAS subscale, and day number; three-way interactions between BIS, each BAS subscale, and condition; as well as four-way interactions between BIS, each BAS subscale, day number, and condition. See Table 4 for the full specification of the model. All predictors entered into the interaction terms were mean-centered to reduce multicollinearity. In order to assess the posterior power of the results, we ran simulations (Martin et al., 2011). First, we generated 100 samples of simulated data for all independent variables, with each variable randomly drawn from a normal distribution with a mean and SD of the respective variable from our sample (N = 65), restricted to a variable’s respective actual minimal and maximum value. As personality traits (BIS, BAS-RR, BAS-FS, and BAS-D) were correlated, their simulated scores were drawn from a normal multivariate distribution which, in addition to means and SDs from the sample and low/high limits of each variable, also accounted for covariance between each variable. Second, for each of 100 samples, we simulated 100 vectors of the dependent variable (energy use on each day) by using simulated dependent variables, as well as fixed and random effects parameters from Model 2. Third, we ran regression models, as specified in Table 4, with 10,000 sets of simulated data: 100 samples, each simulated 100 times. Finally, we determined the power of the analysis by looking at the proportion of significant results at 0.05-level for each of the fixed effect in Model 2. The results of power analyses are reported in Table 4.
The results confirmed the previous analysis with energy use predicted by the consumption on day 1 (B = 0.42, SE = 0.07, p = 0.00001), condition (B = −0.33, SE = 0.15, p = 0.032), and interactions between day number and condition (B = −0.14, SE = 0.04, p = 0.0002). In addition, there was an effect of personality traits. Specifically, there was a positive effect of the interactions between BAS-FS and day number (B = 0.08, SE = 0.02, p = 0.001), a negative effect of the interactions between BIS and day number (B = −0.08, SE = 0.02, p = 0.00001), a negative effect of the three-way interactions between BAS-FS, day number, and condition (B = −0.12, SE = 0.05, p = 0.011), a positive effect of a three-way interactions of BIS, a day number, and condition (B = 0.13, SE = 0.04, p = 0.0001), and a positive effect of a four-way interactions between BIS, BAS-FS, day number, and condition (B = 0.15, SE = 0.05, p = 0.0032). All results remained significant in the restricted sample. All effects were detected with sufficient power (>80%) at significance level of 0.05. There were no effects of BAS-RR or BAS-D on behavior in the game.
To analyze the results of the interactions, we calculated mean predicted values of consumption for high- and low-end participants of each trait, using results of regression analysis, Model 2. High- and low-end participants were identified as above or below of a respective scale of the sample’s average. We then calculated the change for each group of participants (e.g., high versus low BAS-FS group) between days 1 and 7 to illustrate changes in behavior during the week. Analysis of interactions suggests the following interpretation of results: BAS-FS and BIS can explain some variability in individual decisions. Specifically, those who are high in BAS-FS used more toward the end of the week overall, with a predicted average increase between days 1 and 7 of 0.42 EUs, compared to 0.04 EUs increase in those who are low in BAS-FS. Likewise, those who are low in BIS used more toward the end of the week overall, with a predicted average increase of 0.43 EUs compared to high BIS group, who had a predicted decrease of 0.04 EUs. The effects of personality traits were specific to the fair condition: only in the fair condition, participants high in BAS-FS demonstrated a predicted average increase of their use on 1.28 EUs on average between days 1 and 7 (0.33 increase for low BAS-FS participants), while low BIS participants demonstrated a predicted increase of 1.16 EUs (0.16 for high BIS participants). In the unfair condition, both low/high BAS-FS and low/high BIS participants decreased their consumption: high BAS-FS on 0.25, low BAS-FS on 0.31, high BIS on 0.27, and low BIS on 0.29 EUs. Thus, high BAS-FS and low BIS explain some variation in increased use toward the end of the week, but only in the fair condition.
Finally, the investigation of the four-way interaction of BAS-FS, BIS, condition, and day of response suggests that specifically in the fair condition those who are high in BAS-FS and at the same time low in BIS produce the largest increase in use: by 2.77 EUs difference between days 1 and 7, while high BAS-FS and high BIS produced a 0.42 EUs increase, low BAS-FS and low BIS – 0.62 EUs, with low BAS-FS and high BIS participants not changing their use in the fair condition between days 1 and 7. All participants in the unfair condition produced a decrease in use with high BIS/low BAS-FS and low BIS/high BAS-FS decreasing on 0.39 EUs, while high BAS-FS and high BIS on 0.11, low BAS-FS/low BIS on 0.22 EUs. The actual aggregated responses of the groups broken down by high/low BIS and BAS-FS, as well as by condition for each day are depicted on the Figure 2.
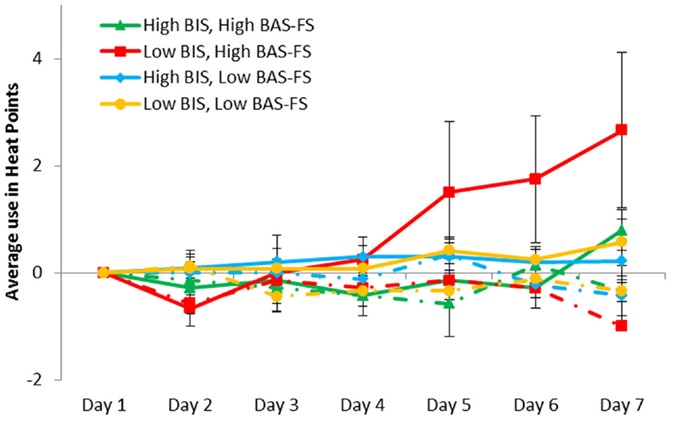
Figure 2. Average use of energy on each day of the week grouped by condition (unfair versus fair) and four different combinations of low and high scores on BIS and BAS-FS: (1) high BAS-FS and high BIS; (2) high BAS-FS and low BIS; (3) low BAS-FS and high BIS; (4) low BAS-FS and low BIS. Error bars represent SE of the mean. The scores are corrected for the baseline use in the first day. High/low groups are identified based on above/below the mean of a respective scale. Dotted lines represent fair, and solid – unfair condition.
Discussion
Behavior in social dilemmas often depends on what others do. After we get feedback about others, we adjust our strategy for future interactions. This paper presents the results of a community purchase energy game structured as an inverted collective-risk dilemma with seven turns before the final outcome is revealed. Participants entered decisions simultaneously with nine other players in their group using their own smart phones or computers at home over a course of a week-long game. Each day, before they made a decision, they also received aggregated information about behavior of others in the group for the previous day. In some groups, we manipulated behavior of the group as fair – the followed a pre-defined group norm of use – or unfair, where the rest of the group used more than was pre-defined.
We find that the majority of participants who were in the unfair condition demonstrated generous behavior: 68% decreased their use on the last day compared to the first day to compensate for the high use of others. This indicates that individuals in groups were good at dealing with effects of free-riding of others, especially as the punishment for group-level non-cooperation was certain. Further we find that the majority of participants in the fair condition demonstrated opportunistic behavior: 63% increased their use on the last day compared to the first day. The findings suggest that individuals did not follow either descriptive (“what others do”) or injunctive (“what I am supposed to do”) social norms of behavior especially under low risk of punishment for free-riding: in the fair condition participants used significantly more than their group on the last day of the study, while in the unfair condition significantly less than their group.
We find that individual differences in impulsivity, measured through BAS-FS, and punishment sensitivity, measured through BIS, were associated with opportunistic strategies in the fair condition. In particular, those who were high in BAS-FS and low in BIS used more on the last turn of the game showing over 2 EUs increase on the last day of the game compared to the first day. We further discuss contribution of the results of the paper to the literature on social dilemmas, individual differences, and understanding of energy behaviors.
Compensating and Opportunistic Strategies in Multi-Turn Social Dilemmas
Strong reciprocity theory suggests that if others are free-riding, people will punish free-riders even at a cost to themselves (Fehr and Gachter, 2002). Our results show that only a small proportion of individuals retaliated by increasing their use when others were unfair, with the majority being generous and compensating for others. Thus, we did not find evidence for strong reciprocity theory in our experiment. It is possible that strong reciprocity does not explain cooperation in social dilemmas that involve interactions over multiple turns. This is in line with previous research suggesting that strong reciprocity cannot always explain how people manage free-riding behaviors in social dilemmas (Yamagishi et al., 2012). In the case of our game, from an individual player perspective, the more others used, the higher was the likelihood of the fine for the group. Therefore, the reason why participants were generous can be explained by high risk of punishment. This is in line with Milinski et al. (2008) who found that in a multi-turn collective-risk dilemma in the high punishment risk condition more participants demonstrated compensating strategies toward the end of the game.
In addition to the risk of punishment, it is possible that participants were generous in this condition because of the take-some framing of the game. This is in line with previous research: it is often reported that people cooperate more in take-some dilemmas (Van Lange et al., 2013). Furthermore, the instructions could have had an influence too: participants knew that their task was not to use over the limit as a group. Such instructions could have enhanced a goal to achieve the results that were best for the group. Previous research demonstrated that goal-orientation has an effect on people’s behavior in social dilemmas. Specifically, people can assign the importance on self- or other-beneficial outcomes (van Lange, 1999) and depending on the framing of the outcome, this can lead to either selfish or other-regarding behavior (Van Lange et al., 2013).
However, even though in this game the majority of participants did not retaliate, this does not mean they were indifferent to the fact that others in their group were unjust. Psychological factors, such as emotions and cognitive appraisals, are strongly implicated in the way people judge a situation that involves unfairness of others (e.g., Ketelaar and Tung Au, 2003; Sanfey et al., 2003; Nelissen et al., 2007). In our case, even if our participants felt angry, most of them did not act on their emotions as demonstrated by decrease in energy use in the unfair condition. The result also cannot be explained by opportunity to “cool down” (Dickinson and Masclet, 2015) as the decision of how much to use on a particular day had to be made straight after being presented with the feedback about others. It is still not clear what the cost of generosity was for individual participants in circumstances when others were unfair. Being angry and not having an opportunity to deal with the emotion (by either reappraising or acting on it) can have detrimental effects on one’s wellbeing (Zammuner and Galli, 2005; Barrett et al., 2013) and have negative consequences for future interactions (e.g., Pillutla and Murnighan, 1996). Future research needs to investigate the role of psychological factors on behavioral strategies in multi-turn games, such as collective-risk social dilemmas with and without opportunities to impose sanctions or act on one’s emotions in some way, as well as what consequences psychological factors, such as emotions, could have on people’s behavior and interactions beyond an experimental game.
While in the unfair condition, participants were generous, the opposite was observed in the fair condition. In this condition, the majority of participants increased their use toward the end of the game. Contrary to social norms literature (Schultz et al., 2007), which suggests that both descriptive (do as others do, in our case implemented through feedback about behavior of others) and injunctive (do as you think is right, in our case implemented through the instruction of normative use of 4 HPs) norms guide people’s behavior, in our game, participants did not follow the norm of use demonstrated by their confederates through manipulated feedback, or as it was reinforced in the instructions for the experiment. This suggests the influences of social norms on behavior might be weaker if there are other motivations guiding people’s choices, such as getting individual private benefits or maximizing individual profit through avoiding a group-level fine (Charness and Rabin, 2002). However, as the reported results only cover one game, it is not clear what the participants in the unfair condition would do should they have another round of interactions with their group partners.
Participants in the fair condition significantly increased their consumption. The increase throughout the game is in line with rebound effects in energy use domain: when presented with real feedback about energy use of other households people find out that they used less than others, under some circumstances, they can increase their energy use (Leygue et al., 2014). Use of over the fair-share allowance by 30% of participants at some point in the game further indicated a different type of individual behavior in response to feedback about others – a type of weak free-riding (Keser and van Winden, 2000), which has negative consequences especially considering the environmental context of these decisions. While the findings are at odds with social norms literature, they are in line with literature on collective-risk games. Milinski et al. (2008) demonstrated that in low punishment risk condition participants free rode more. In the case of our fair condition, participants presumably also perceived risk of punishment as low; therefore, they chose to take advantage of the situation.
On the one hand, given the structure of the game, it was rational to use more when there was an opportunity, as one could get additional private benefits for usage. What is rational in the current situation could depend on the context and it is possible that our participants just aimed to optimize their profit in the game and disregarded the broader environmental picture. However, the environmental context of the game puts the decision into a different perspective, as the overarching goal of such energy purchase scheme is ultimately to decrease energy use. It is noteworthy that people still increased their energy use for private benefit, despite knowing that doing so can have consequences for the environment. While this result can be also explained by the fact that some participants might have not considered environmental framing of the game, understanding people’s motivations behind free-riding is important, as it can explain why people do not make environmentally friendly choices in the real world, including not making links between their own actions and consequences for environment. For example, future energy collectives individuals might employ such opportunistic strategies by accumulating more energy than their fair-share in personal storage. Previous research suggests that people justify free-riding behavior by denying their responsibility for the outcome (Schwartz and Howard, 1982) or by convincing themselves that their behavior would not make a difference to the group outcome (Kerr and Kaufman-Gilliland, 1997). It is possible that in the case of this game, participants felt that increasing usage would not impact the outcome, so they could behave opportunistically. It is also plausible that participants behaved rationally and optimized their profits. However, whichever reason was driving the behavior of the majority, similar choices in real world have negative implications for issues such as climate change mitigation. Our findings can be used by policy makers to develop and model approaches to predict and discourage opportunistic strategies. Based on our findings, policy makers could benefit by building in an incentive structure to encourage cooperation and to prevent opportunistic behavior in future scenarios.
Individual Differences and Opportunistic Strategies
While some participants used an opportunity to get additional profits in the fair condition, about 40% did not demonstrate such behavior: they either did not change or decreased their usage. We showed that individual differences in impulsivity and punishment sensitivity were associated with opportunistic strategies. Specifically, participants with high BAS-FS and low BIS increased their energy use toward the end of the game significantly more than other participants. Results are in line with previous findings that impulsive individuals are more likely to be biased toward an immediate reward in the situations where there is a conflict between immediate and delayed reward (Smillie et al., 2006). In our study, participants knew that using more HPs would increase their profits, so they received immediate gratification from using HPs, while the reward through cooperation was delayed by at least one day (in case of the last turn decision) or more days (in case of all other decisions). This result is in line with findings of Myrseth et al. (2015) who demonstrated negative associations between impulsivity and cooperation when immediate rewards for free-riding were more salient and tangible. Furthermore, in line with predictions, we found that participants who were low in BIS were more likely to free ride when the risk of punishment was low, i.e., in the fair condition. This supports previous research, suggesting that inhibitory mechanisms are implicated in prosocial choices as one needs to withhold an initial impulse to free ride in order to get better rewards through cooperation in the future (McCabe et al., 2001; Skatova and Ferguson, 2013).
Our findings contribute to the understanding of conditions necessary in order to maintain cooperation in groups especially around environmental issues. For example, Freytag et al. (2014) found that intermediate targets featuring environmental protection as a process helped to improve cooperation in collective-risk dilemmas. It is possible that introduction of intermediate targets and rewards in a community energy purchase scenario, for example, through messages that enhance environmental consequences of various decisions or through opportunities for reputation formation could reduce opportunistic behavior in the collective-risk game among impulsive individuals with low inhibitory control.
We did not find predicted associations between other subscales of BAS (BAS-RR and BAS-D) and behavior in the experiment. In previous studies that demonstrated associations between reward responsiveness component of BAS, namely BAS-RR and BAS-D, and free-riding behavior in economic games, participants had full information about behavior of others or control over the situation while making their decision. Thus, selfish choices of reward responsive participants could have been explained by the fact that they learned better from reward and made a selfish choice to take advantage of a certain increase in profits. In our design even for the last decision, there was some uncertainty about behavior of others. This different structure of the game can explain why there were no associations of BAS-RR and BAS-D with behavior in the unfair condition. While we did not find any associations of individual differences and behavior in the unfair condition, future research could study the motivation behind generous compensating strategies in collective-risk dilemmas.
Implications for Policy Around Energy Use
Many researchers highlight that it is important to extend lab-based paradigms and develop social dilemma research designs that help to mirror important features of real-world behavior in social dilemma-like scenarios (e.g., Van Lange et al., 2013). Such research can help to identify constraints of policies and test out model scenarios in various areas of social decision-making. The results of the study presented here suggest that community energy purchase deals could backfire as we predict that under certain conditions people will increase their energy usage, especially if there is an opportunity to gain private benefits and the risk of punishment is low. Community energy purchase schemes without a system of intermediate rewards and/or risk of punishment might not be as efficient as expected. We further suggest that it is necessary to study the implications of these schemes beyond actual energy use, because opportunistic behavior of others might lead to indirect negative consequences on interpersonal relationships in the community. Future research is needed to understand psychological cost of generous compensatory behavior that we observed in unfair condition, and whether it could spill over to other domains of interactions within community.
While our game modeled one specific case of managing energy supply and demand on a local level, our results have implications to decision-making in other areas of sustainable behavior such as household energy use (Leygue et al., 2014) and climate change mitigation (Milinski et al., 2008). Understanding how people act in dilemmas such as climate change mitigation are of high importance, however, we advocate the approach to employ social dilemmas to study more local decisions, such as community energy purchase schemes. Ultimately, for people, the climate change mitigation dilemma consists of small person-level every day dilemmas, such as the one presented in this paper. Moreover, research suggests that attempts to establish cooperation with large groups is less productive than when small groups are involved (Santos and Pacheco, 2011). Without understanding how to manage free-riding and achieve cooperation on small scale, it will also not be possible to resolve the global climate change mitigation dilemma.
Limitations
Our study had limitations. Failure in experimental software on day 7 meant that all participants whose data were submitted to the final analysis saw the feedback about behavior of others twice, and on the first occasion the feedback was incorrect. This reduced sample size that could have biased the responses and were submitted to the analyses. While our findings are consistent with previous research both in terms of behavioral outcomes (Jacquet et al., 2013) and individual differences (Myrseth et al., 2015; Skatova and Ferguson, 2013), the replication of the main findings can help to affirm the results. The heterogeneity of responses in economic games (which subsequently produces large variation around the mean) is well documented (Burlando and Guala, 2005), however, future research could also help to explain remaining variation that is visible from Figure 2’s SE: specifically, there might be other personality or cognitive factors driving variation in behavior in the fair condition.
Our study also did not account for a number of factors that could have impacted cooperation in the collective-risk game scenario: for example, reputation, anonymity, communication between group members, and other factors. Research on social dilemmas suggests that reputation (Milinski et al., 2002) is key in sustaining cooperation in groups. Reputation scenarios assume that players responses could be traced throughout the game, which was not possible in our design. Decreasing anonymity is not directly applicable to energy use at home, as it comes at privacy cost (McKenna et al., 2012; Rouf et al., 2012). However, lower levels of anonymity than we had in our game – where only group-level behavior was shared with others – and some opportunities for reputation building might have improved cooperation in a collective-risk dilemma scenario. Furthermore, our study did not involve any communication between group members, while real-world interactions certainly involve at least some level of communication. Communication provides the group with more opportunities to self-manage cooperation through, for example, imposing social sanctions, such as disapproval (Noussair and Tucker, 2005). Future research could look into whether communication between group partners helps to coordinate the efforts around energy use and reduce the level of opportunistic strategies.
Conclusion
We used a social dilemma – a collective-risk game – to model real-world decisions in a community energy purchase scenario. Our study confirms that in order to maintain cooperation the risk of punishment should be high and tangible; otherwise, people take advantage of the situation and free ride. Specifically, individuals high in impulsivity and low in sensitivity to punishment showed higher levels of opportunistic behavior. We also show that when the risk of punishment is high, people compensate for others to avoid the group-level punishment. However, the psychological cost is unclear. Compensating for others could come at an emotional toll and impact negatively on further interactions. Taking advantage at the last moment puts collective good at risk in a way that can lead to a disaster, especially in an environmental context. We suggest that people should have tangible intermittent incentives to save energy and not just be expected to follow what others do as suggested by social norms literature. Taken together, the findings of the study reported here illustrate the benefits of a social dilemma approach to study behaviors around energy use and the constraints of policies in the environmental domain.
Author Contributions
AS and BB designed the study, AS and BKS collected and analysed the data, all authors contributed to the writing and revision of the paper, approved the final draft, and agree to be accountable to all aspects of the work presented in the paper.
Conflict of Interest Statement
The authors declare that the research was conducted in the absence of any commercial or financial relationships that could be construed as a potential conflict of interest.
Acknowledgments
The study was supported by Horizon Digital Economy Research (Research Councils UK grant EP/G065802/1), from Human Data to Personal Experience (Research Councils UK grant EP/M02315X/1), Creating the Energy for Change (Research Councils UK grant EP/K002589/1), and Network for Integrated Behavioural Science (Research Councils UK grant ES/K002201/1).
Supplementary Material
The Supplementary Material for this article can be found online at http://journal.frontiersin.org/article/10.3389/fenrg.2016.00008
References
Axelrod, R., and Hamilton, W. D. (1981). The evolution of cooperation. Science 211, 1390–1396. doi:10.1126/science.7466396
Bardsley, N. (2000). Control without deception: individual behaviour in free-riding experiments revisited. Exp. Econ. 3, 215–240. doi:10.1023/a:1011420500828
Barrett, E. L., Mills, K. L., and Teesson, M. (2013). Mental health correlates of anger in the general population: findings from the 2007 national survey of mental health and wellbeing. Aust. N. Z. J. Psychiatry 47, 470–476. doi:10.1177/0004867413476752
Bates, D., Mächler, M., Bolker, B., and Walker, S. (2014). Fitting Linear Mixed-Effects Models Using LME4. arXiv preprint arXiv:1406.5823.
Biel, A., and Thøgersen, J. (2007). Activation of social norms in social dilemmas: a review of the evidence and reflections on the implications for environmental behaviour. J. Econ. Psychol. 28, 93–112. doi:10.1016/j.joep.2006.03.003
Binmore, K. (2014). Bargaining and fairness. Proc. Natl. Acad. Sci. U.S.A. 111(Suppl. 3), 10785–10788. doi:10.1073/pnas.1400819111
Burlando, R. M., and Guala, F. (2005). Heterogeneous agents in public goods experiments. Exp. Econ. 8, 35–54. doi:10.1007/s10683-005-0436-4
Carver, C. S., and White, T. L. (1994). Behavioral inhibition, behavioral activation, and affective responses to impending reward and punishment: the BIS/BAS scales. J. Pers. Soc. Psychol. 67, 319–333. doi:10.1037/0022-3514.67.2.319
Charness, G., and Rabin, M. (2002). Understanding social preferences with simple tests. Q. J. Econ. 117, 817–869. doi:10.1162/003355302760193904
Conaty, P., and Mayo, E. (2012). Towards a co-operative energy service sector. J. Cooper. Stud. 45, 46–55.
Croson, R. T. A. (2007). Theories of commitment, altruism and reciprocity: evidence from linear public goods games. Econ. Inq. 45, 199–216. doi:10.1111/j.1465-7295.2006.00006.x
DECC. (2013). Helping Customers Switch: Collective Switching and Beyond. Available at: https://www.gov.uk/government/uploads/system/uploads/attachment_data/file/253862/Helping_Customers_Switch_Collective_Switching_and_Beyond_final__2_.pdf
DECC. (2014). Community Energy Strategy. London: HMSO. Available at: https://www.gov.uk/government/uploads/system/uploads/attachment_data/file/275163/20140126Community_Energy_Strategy.pdf
Dickinson, D. L., and Masclet, D. (2015). Emotion venting and punishment in public good experiments. J. Public Econ. 122, 55–67. doi:10.1016/j.jpubeco.2014.10.008
Erbmann, R., Goulbourne, H., and Malik, P. (2009). Collective Power: Changing the Way We Consume Energy. London: Co-operative Party.
Fehr, E., and Fischbacher, U. (2003). The nature of human altruism. Nature 425, 785–791. doi:10.1038/nature02043
Fehr, E., and Gachter, S. (2002). Altruistic punishment in humans. Nature 415, 137–140. doi:10.1038/415137a
Freytag, A., Güth, W., Koppel, H., and Wangler, L. (2014). Is regulation by milestones efficiency enhancing? An experimental study of environmental protection. Eur. J. Polit. Econ. 33, 71–84. doi:10.1016/j.ejpoleco.2013.11.005
Fudenberg, D., Rand, D. G., and Dreber, A. (2012). Slow to anger and fast to forgive: cooperation in an uncertain world. Am. Econ. Rev. 102, 720–749. doi:10.1257/aer.102.2.720
Gintis, H., Bowles, S., Boyd, R., and Fehr, E. (2003). Explaining altruistic behavior in humans. Evol. Hum. Behav. 24, 153–172. doi:10.1016/s1090-5138(02)00157-5
Giovanelli, A., Hoerger, M., Johnson, S. L., and Gruber, J. (2013). Impulsive responses to positive mood and reward are related to mania risk. Cogn. Emot. 27, 1091–1104. doi:10.1080/02699931.2013.772048
Gneezy, A., and Fessler, D. M. (2012). Conflict, sticks and carrots: war increases prosocial punishments and rewards. Proc. Biol. Sci. 279, 219–223. doi:10.1098/rspb.2011.0805
Greening, L. A., Greene, D. L., and Difiglio, C. (2000). Energy efficiency and consumption–the rebound effect–a survey. Energy Policy 28, 389–401. doi:10.1016/S0301-4215(00)00021-5
Harvey, D., and Braun, B. (1999). Justice, nature, and the geography of difference. Can. Geogr. 43, 105.
Hoffman, S. M., and High-Pippert, A. (2010). From private lives to collective action: recruitment and participation incentives for a community energy program. Energy Policy 38, 7567–7574. doi:10.1016/j.enpol.2009.06.054
Irwin, K., and Berigan, N. (2013). Trust, culture, and cooperation: a social dilemma analysis of pro-environmental behaviors. Sociol. Q. 54, 424–449. doi:10.1111/tsq.12029
Jacquet, J., Hagel, K., Hauert, C., Marotzke, J., Röhl, T., and Milinski, M. (2013). Intra-and intergenerational discounting in the climate game. Nat. Clim. Chang. 3, 1025–1028. doi:10.1038/nclimate2024
Kerr, N. L., and Kaufman-Gilliland, C. M. (1997). “… and besides, I probably couldn’t have made a difference anyway”: justification of social dilemma defection via perceived self-inefficacy. J. Exp. Soc. Psychol. 33, 211–230. doi:10.1006/jesp.1996.1319
Keser, C., and van Winden, F. (2000). Conditional cooperation and voluntary contributions to public goods. Scand. J. Econ. 102, 23–39. doi:10.1111/1467-9442.00182
Ketelaar, T., and Tung Au, W. (2003). The effects of feelings of guilt on the behaviour of uncooperative individuals in repeated social bargaining games: an affect-as-information interpretation of the role of emotion in social interaction. Cogn. Emot. 17, 429–453. doi:10.1080/02699930143000662
Leygue, C., Ferguson, E., Skatova, A., and Spence, A. (2014). Energy sharing and energy feedback: affective and behavioral reactions to communal energy displays. Front. Energy Res. 2, 29. doi:10.3389/fenrg.2014.00029
Li, Y. (2005). Perceptions of temperature, moisture and comfort in clothing during environmental transients. Ergonomics 48, 234–248. doi:10.1080/0014013042000327715
Lyas, J. K., Shaw, P. J., and Van-Vygt, M. (2004). Provision of feedback to promote householders’ use of a kerbside recycling scheme: a social dilemma perspective. J. Solid Waste Technol. Manag. 30, 7–18.
Martin, J. G., Nussey, D. H., Wilson, A. J., and Reale, D. (2011). Measuring individual differences in reaction norms in field and experimental studies: a power analysis of random regression models. Methods Ecol. Evol. 2, 362–374. doi:10.1111/j.2041-210X.2010.00084.x
McCabe, K., Houser, D., Ryan, L., Smith, V., and Trouard, T. (2001). A functional imaging study of cooperation in two-person reciprocal exchange. Proc. Natl. Acad. Sci. U.S.A. 98, 11832–11835. doi:10.1073/pnas.211415698
McKenna, E., Richardson, I., and Thomson, M. (2012). Smart meter data: balancing consumer privacy concerns with legitimate applications. Energy Policy 41, 807–814. doi:10.1016/j.enpol.2011.11.049
Milinski, M., Semmann, D., and Krambeck, H.-J. (2002). Reputation helps solve the ‘tragedy of the commons’. Nature 415, 424–426. doi:10.1038/415424a
Milinski, M., Sommerfeld, R. D., Krambeck, H.-J., Reed, F. A., and Marotzke, J. (2008). The collective-risk social dilemma and the prevention of simulated dangerous climate change. Proc. Natl. Acad. Sci. U.S.A. 105, 2291–2294. doi:10.1073/pnas.0709546105
Myrseth, K. O. R., Riener, G., and Wollbrant, C. E. (2015). Tangible temptation in the social dilemma: Cash, cooperation, and self-control. J. Neurosci. Psychol. Econ. 8, 61–77. doi:10.1037/npe0000035
Nelissen, R. M. A., Dijker, A. J. M., and deVries, N. K. (2007). How to turn a hawk into a dove and vice versa: interactions between emotions and goals in a give-some dilemma game. J. Exp. Soc. Psychol. 43, 280–286. doi:10.1016/j.jesp.2006.01.009
Noussair, C., and Tucker, S. (2005). Combining monetary and social sanctions to promote cooperation. Econ. Inq. 43, 649–660. doi:10.1093/ei/cbi045
Nowak, M. A., Page, K. M., and Sigmund, K. (2000). Fairness versus reason in the ultimatum game. Science 289, 1773–1775. doi:10.1126/science.289.5485.1773
Pillutla, M. M., and Murnighan, J. K. (1996). Unfairness, anger, and spite: emotional rejections of ultimatum offers. Organ. Behav. Hum. Decis. Process 68, 208–224. doi:10.1006/obhd.1996.0100
Pothos, E. M., Perry, G., Corr, P. J., Matthew, M. R., and Busemeyer, J. R. (2011). Understanding cooperation in the Prisoner’s Dilemma game. Pers. Individ. Dif. 51, 210–215. doi:10.1016/j.paid.2010.05.002
Ramchurn, S. D., Osborne, M., Parson, O., Rahwan, T., Maleki, S., Reece, S., et al. (2013). “AgentSwitch: towards smart energy tariff selection,” in Proceedings of the 12th International Conference on Autonomous Agents and Multiagent Systems (AAMAS 2013), 2013, St. Paul, MN, eds T. Ito, C. Jonker, M. Gini, and O. Shehory.
Rouf, I., Mustafa, H., Xu, M., Xu, W., Miller, R., and Gruteser, M. (2012). “Neighborhood watch: security and privacy analysis of automatic meter reading systems,” in Proceedings of the 2012 ACM Conference on Computer and Communications Security. (Raleigh, NC: ACM), 462–473.
Sanfey, A. G., Rilling, J. K., Aronson, J. A., Nystrom, L. E., and Cohen, J. D. (2003). The neural basis of economic decision-making in the ultimatum game. Science 300, 1755–1758. doi:10.1126/science.1082976
Santos, F. C., and Pacheco, J. M. (2011). Risk of collective failure provides an escape from the tragedy of the commons. Proc. Natl. Acad. Sci. U.S.A. 108, 10421–10425. doi:10.1073/pnas.1015648108
Scheres, A., and Sanfey, A. (2006). Individual differences in decision making: drive and reward responsiveness affect strategic bargaining in economic games. Behav. Brain Funct. 2, 35. doi:10.1186/1744-9081-2-35
Schultz, P. W., Nolan, J. M., Cialdini, R. B., Goldstein, N. J., and Griskevicius, V. (2007). The constructive, destructive, and reconstructive power of social norms. Psychol. Sci. 18, 429–434. doi:10.1111/j.1467-9280.2007.01917.x
Schwartz, S. H., and Howard, J. A. (1982). “A self-based motivational model of helping,” in Cooperation and Helping Behavior: Theories and Research, eds V. Derlega and J. Grzelak (New York: Academic Press), 22–35.
Skatova, A., and Ferguson, E. (2011). What makes people cooperate? Individual differences in BAS/BIS predict strategic reciprocation in a public goods game. Pers. Individ. Dif. 51, 237–241. doi:10.1016/j.paid.2010.05.013
Skatova, A., and Ferguson, E. (2013). Individual differences in behavioural inhibition explain free riding in public good games when punishment is expected but not implemented. Behav. Brain Funct. 9, 3. doi:10.1186/1744-9081-9-3
Smillie, L. D., Jackson, C. J., and Dalgleish, L. I. (2006). Conceptual distinctions among Carver and White’s (1994) BAS scales: a reward-reactivity versus trait impulsivity perspective. Pers. Individ. Dif. 40, 1039–1050. doi:10.1016/j.paid.2005.10.012
Van Dijk, E., Wilke, H., and Wit, A. (2003). Preferences for leadership in social dilemmas: public good dilemmas versus common resource dilemmas. J. Exp. Soc. Psychol. 39, 170–176. doi:10.1016/S0022-1031(02)00518-8
Van Lange, P. A. (1999). The pursuit of joint outcomes and equality in outcomes: an integrative model of social value orientation. J. Pers. Soc. Psychol. 77, 337–349. doi:10.1037/0022-3514.77.2.337
Van Lange, P. A., Joireman, J., Parks, C. D., and Van Dijk, E. (2013). The psychology of social dilemmas: a review. Organ. Behav. Hum. Decis. Process 120, 125–141. doi:10.1016/j.obhdp.2012.11.003
Van Lange, P. A., Rockenbach, B., and Yamagishi, T. (eds). (2014). Reward and Punishment in Social Dilemmas. Oxford: Oxford University Press.
Vinyals, M., Bistaffa, F., Farinelli, A., and Rogers, A. (2012). “Coalitional energy purchasing in the smart grid,” in Energy Conference and Exhibition (ENERGYCON), 2012 IEEE International. (Florence, IT: IEEE), 848–853.
Walker, G., Devine-Wright, P., Hunter, S., High, H., and Evans, B. (2010). Trust and community: exploring the meanings, contexts and dynamics of community renewable energy. Energy Policy 38, 2655–2663. doi:10.1016/j.enpol.2009.05.055
Weber, J. M., and Murnighan, J. K. (2008). Suckers or saviors? Consistent contributors in social dilemmas. J. Pers. Soc. Psychol. 95, 1340. doi:10.1037/a0012454
Yamagishi, T., Horita, Y., Mifune, N., Hashimoto, H., Li, Y., Shinada, M., et al. (2012). Rejection of unfair offers in the ultimatum game is no evidence of strong reciprocity. Proc. Natl. Acad. Sci. U.S.A. 109, 20364–20368. doi:10.1073/pnas.1212126109
Zammuner, V. L., and Galli, C. (2005). Wellbeing: causes and consequences of emotion regulation in work settings. Int. Rev. Psychiatry 17, 355–364. doi:10.1080/09540260500238348
Keywords: cooperation, collective-risk social dilemma, public good, community energy, environmental behaviour, impulsivity, punishment sensitivity, collective purchasing
Citation: Skatova A, Bedwell B and Kuper-Smith B (2016) When Push Comes to Shove: Compensating and Opportunistic Strategies in a Collective-Risk Household Energy Dilemma. Front. Energy Res. 4:8. doi: 10.3389/fenrg.2016.00008
Received: 28 February 2015; Accepted: 16 February 2016;
Published: 14 March 2016
Edited by:
Tobias Brosch, University of Geneva, SwitzerlandReviewed by:
John M. Polimeni, Albany College of Pharmacy and Health Sciences, USAUlf J. J. Hahnel, University of Geneva, Switzerland
Copyright: © 2016 Skatova, Bedwell and Kuper-Smith. This is an open-access article distributed under the terms of the Creative Commons Attribution License (CC BY). The use, distribution or reproduction in other forums is permitted, provided the original author(s) or licensor are credited and that the original publication in this journal is cited, in accordance with accepted academic practice. No use, distribution or reproduction is permitted which does not comply with these terms.
*Correspondence: Anya Skatova, YW55YS5za2F0b3ZhQGdtYWlsLmNvbQ==