Predicting Buildings' Energy Use: Is the Occupant-Centric “Performance Gap” Research Program Ill-Advised?
- Department of Building Physics and Building Ecology, Vienna University of Technology, Vienna, Austria
The term “performance prediction” commonly denotes the analysis of building designs, for instance, in view of their future energy demand. The notion of “performance gap” is invoked, when actual performance of buildings does not match preceding predictions. There has been a recent trend to attribute the so-called energy performance gap predominantly (sometimes even entirely) to user behavior. As a consequence, a number of research efforts (subsumed hear as “the performance gap research program”) pursue more accurate predictions of user behavior and the exploitation of user-related energy efficiency potential via behavioral modification. In the present contribution, we critically revisit the premises and orientation of these efforts. Firstly, we suggest to situate the related discourse within a structured conceptual framework to objectively discuss the spectrum of potential contributors to the errors in building energy use predictions. Secondly, we examine the frequently purported utility of probabilistic methods and uncertainty analysis as an effectual remedy to the problem of performance gap.
Introduction
Numeric simulation tools can support the analysis of buildings' physical behavior (Clarke, 2007). An instance of such behavior pertains to buildings' energy demand for heating, cooling, lighting, and ventilation (De Wilde, 2018; Hensen and Lamberts, 2019). Frequently, the notion of “performance prediction” is used to denote the analysis of building designs, for instance, in view of their future energy demand. When actual performance of buildings does not match previous predictions, a further notion, namely “performance gap” is invoked. As such, the disagreement between buildings' simulated and actual energy performance can be the result of multiple sources of uncertainty, including assumed building properties, internal processes, and external boundary conditions (i.e., weather). Whereas, such causes and their relative role in prediction errors may have to be identified on a case by case basis, there has been a recent trend to attribute the so-called performance gap predominantly (sometimes even entirely) to user behavior (Yan et al., 2015; Hong et al., 2016; Jia et al., 2017; Yoshino et al., 2017). As a consequence, numerous research efforts in the building energy use domain pursue both better predictions of user behavior (Wagner et al., 2018; Mahdavi and Tahmasebi, 2019) and the exploitation of user-related energy efficiency potential via behavioral modification (Ueno et al., 2005). In the present contribution, we critically revisit these efforts, subsumed under the heading of “the performance gap research program,” from a 2-fold perspective. Firstly, a structured conceptual framework is proposed to objectively discuss the spectrum of potential occupant-related contributors to the errors in simulation-based energy use predictions. The entailed logical analysis suggests that the a priori identification of the human factor as responsible for the performance gap may be misleading. Secondly, the frequently promoted probabilistic methods and uncertainty analysis are considered in view of their potential as an effective remedy to the problem of performance gap. Thereby, a key question pertains to the usefulness of uncertainty analysis for buildings' energy evaluation processes in those cases where the assumed priors are not empirically grounded.
The Performance Gap Research Program
To start with a disclaimer, there is, to our knowledge, no explicitly formulated performance gap research program. However, a cursory review of a number of recent and ongoing research efforts reveals certain recurrent leitmotivs that may be interpreted as a kind of implicit research program (Menezes et al., 2012; Andersen et al., 2016). The premises and arguments of this research program could be stated as follows:
(i) Buildings' actual (monitored) energy use can deviate frequently and largely from prior computational (typically simulation-based) predictions. This circumstance underlies the performance gap parlance.
(ii) Even in case of similar buildings (identical designs in terms of geometry and layout, identical construction features, and same location in terms of urban context and microclimate) monitored energy use magnitudes can vary significantly. Such differences must thus be due to differences in occupancy patterns and behavior.
(iii) From statements i and ii above it follows that, in order to close the performance gap, more accurate predictions of occupancy patterns and behavior are needed that must take the stochastic nature of occupancy-related phenomena and processes into account.
(iv) To address the need stated in statement iii, we need to a) replace conventional (schedule-based) occupant presence and (rule-based) behavioral models with stochastic models and b) routinely conduct concomitant uncertainty analysis.
(v) Independent of modeling challenges, the mere fact of people's significant impact on buildings' energy performance underlines the need for concerted efforts and campaigns to encourage and guide building occupants toward energy-conscious and energy-efficient behavior.
We only briefly address a few of the inconsistencies of this program in the following (we refrain from an extensive analysis not only to circumvent the straw man fallacy criticism, but also because, as it will become more clear later, we want to focus on a specific aspect of this program):
Statement i above is as such largely correct: It is backed by empirical evidence. Statement ii appears likewise plausible. However, it must be dealt with cautiously: Even in case of seemingly identical building objects, we cannot simply exclude differences and inconsistencies in construction quality and details as well as quality fluctuations in building systems installations. Moreover, despite the unitary appearance of certain building objects (say, terrace houses), there may be differences, for instance in terms of solar exposure (e.g., due to the site layout) and air flow patterns. However, the main flaw of the research program, as described above, is to assume that statements iii and iv simply follow from statements i and ii. The entailed deduction here entails a threefold fallacy:
• First, there is not clear at all if predictions of future occupancy patterns and behavior (particularly in case of unknown populations) can be arbitrarily enhanced.
• Second, it is not established that the replacement of schedules and rules with stochastic models inevitably results in higher predictive accuracy of the values of energetically relevant key performance indicators (Mahdavi et al., 2018). Note that the emulation of the probabilistic look of energy use dynamics is not the same as provision of accurate predictions of future energy use.
• Third, even if we grant that significant improvements in occupancy-related predictions are possible (for instance, in case of existing buildings, and known populations), it does not follow that performance gap—in the sense of energy performance predictions for building designs—can be closed: This circumstance should be utterly clear, if we just reflect upon the difficulties—if not the impossibility—of mid-term and long-term weather predictions.
Nonetheless, rather than continuing with the exposition of the argumentative flaws in the performance gap research program in its entirety, we would like to draw attention to its—rather innocent sounding—second and last statements (items ii and v in the above formulation). The tacit assumptions here is not only that people's influence on buildings' energy performance is significant, but also that occupants generally have at their disposal the options and possibilities to reduce the negative implications of this influence (i.e., wasteful energy use): All they need to do is to simply acquire and display the right disposition and attitude toward energy-conscious behavior. It appears as though, from this tacit assumption, and without sufficient reflection, the occupant-centric performance gap research program derives a kind of preeminent standing for probabilistic modeling endeavors and behavioral modification projects (Hong et al., 2016). Notwithstanding the importance of the latter initiatives, we suggest that there is still a need for a deeper and more systematic reflection on the ways by which people can influence buildings' energy use. Specifically, it is important to better understand the nature and categories of such influence, if meaningful occupancy-related energy efficiency potentials are to be exploited. Toward this end, in the next section of the present contribution, we suggest and discuss a possible categorization of people's role in buildings' energy performance together with assessment of the extent to which both improved modeling techniques and informational campaigns could spark a positive impact.
Reflections on the Occupants' Influence Space
As alluded to in the introduction, people's assumed impact on buildings' energy performance has triggered a number of research efforts into the patterns and implications of occupants' control-oriented behavior in buildings. However, at times it appears that such efforts are made without prior deep reflection on nature, conditions, mechanisms, and scope of behavior impact on energy performance. It may be thus beneficial to reflect upon the logical space of occupants' influence possibilities. Ideally, such reflection should lead to a categorized list of occupant-related issues together with the mechanisms by which they influence energy usage.
A possible starting point for such a categorization effort could be a previously formulated distinction between people's passive and active effects on building performance in general and energy use in particular (Andersen et al., 2007). Passive effects are the mere consequence of people's presence in buildings. Focusing on energy-relevant matters, sensible, and latent heat emission by occupants contribute to the so-called internal energy gains and can thus influence buildings' heating and cooling loads. Other emissions related to occupants' presence in buildings (e.g., carbon dioxide and various pollutants) may not have direct relevance to buildings' heat transfer processes, but are relevant to required ventilation rates, be those modulated centrally or directly by occupants (e.g., via operation of windows for natural ventilation). Active effects are, on the other hand, the result of occupants' interactions with those building elements, components, and systems that are either explicitly meant to facilitate indoor environmental control (heating, cooling, ventilation, and lighting) or indirectly influence buildings' thermal balance (i.e., due to heat emission by computers and associated peripherals, electronic equipment, etc.). Note that indoor environmental control devices encompass both envelope elements (such as windows and blinds) and technical systems (for heating, cooling, and ventilation). The scope of energy-relevant implications of occupants' interactions with these devices is obviously a function of their availability and the extent to which they can be manipulated. For instance, the lighting systems in an office may be:
(i) centrally controlled,
(ii) operated based on occupancy sensing,
(iii) operated based on daylight sensing,
(iv) switched on and off by occupants,
(v) manipulated by occupants using a dimmer (for continuous control of the light levels).
In instances i to iii above, occupants typically cannot directly or actively influence the device state (and, consequentially, neither the resulting energy ramifications). As with the lighting systems, heating, cooling, and ventilation systems may be centrally controlled or they may afford some level of occupant interaction, from rudimentary on/off alternatives to extended menus for definition and scheduling of assorted set-points to define preferred ranges for indoor environmental variables such as temperature and humidity. Consequently, the extent of occupants' impact on energy use depends on the availability, type, and resolution of systems control opportunities, which may greatly differ from one building to another, even within typologically similar building populations (e.g., residential or commercial buildings).
This brief analysis points to the problematic nature of general and global statements regarding the scope and magnitude of occupants' influence on buildings' energy use. Even if we, notwithstanding all the above mentioned caveats, identify occupant behavior as a major contributor to the performance gap, we should be careful about the coherence of the associated research program: It can be hardly exhausted in pottering around with stochastic modeling methods or formalistic (empirically ungrounded) uncertainty analysis exercises. Likewise, to be effective, occupancy-centric energy efficiency arguments and campaigns need to be ideally based on a prior understanding of not only the diversity of occupants' needs and preferences, but also the actual degree of control-relevant affordance offered by the targeted buildings and their technical systems.
Consider a number of building devices such as envelope elements (windows, shades), electrical equipment (luminaires, fans), and building systems terminals (i.e., interfaces of building systems with indoor spaces (e.g., radiators, diffusors). The following Table 1 provides an illustrative summary representation of these devices together with:
(a) presumed reasons why the occupants would operate them,
(b) how (by which means) the occupants could operate them,
(c) the kind of energy source that could be affected by occupant actions,
(d) the reasons why occupant-based actions could lead to energy inefficiency.
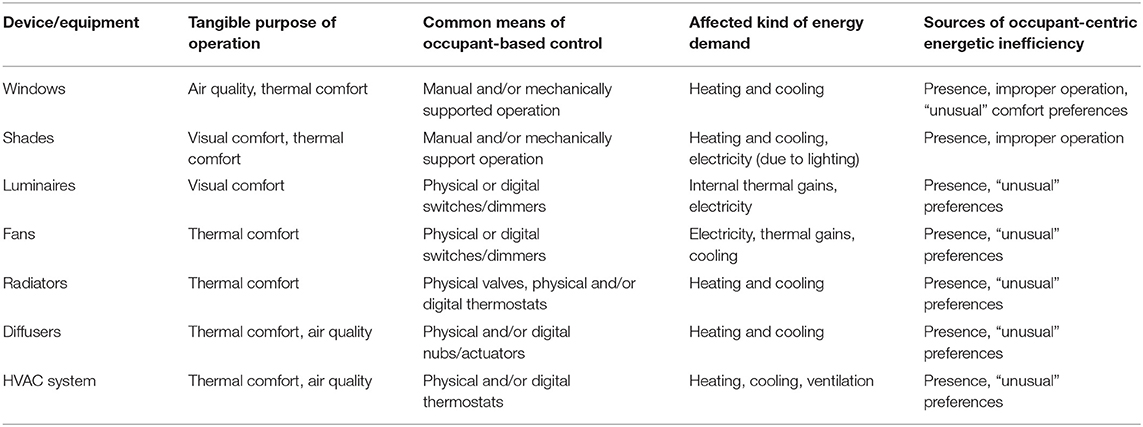
Table 1. Summary representation of buildings' control devices with associated functionality, interface, relevant energy demand categories, and sources of inefficient operation.
We refer to this representation as illustrative, as it is not meant to be either exhaustive or highly detailed. For instance, devices are organized in a number of broad categories (first column). With “tangible” purpose of operation (second column) we refer to the circumstance that devices might be operated because of reasons other than the commonly assumed default ones: For instance, windows might be opened to respond to a psychological need to establish immediate connection to the outside environment, or they may be closed to escape perceived noise and air pollution. Likewise, in certain situations, shades could be applied due to privacy concerns, rather than solar radiation, or daylight control. Nonetheless, the summary representation of Table 1 allows to pinpoint some of the challenges of the aforementioned occupant-centric energy efficiency research program. The second column of Table 1 summarizes a listing of why occupant would make use of device control opportunities (if available). They basically correspond to the reasons why we make buildings in the first place, namely to respond to occupants' need for healthy and comfortable indoor environments. Assuming (i) occupants are conscious of their needs, (ii) these needs are “reasonable,” (iii) buildings' control devices in general and means of occupants' interactions with these devices in particular are properly conceived and implemented, we are left only with a rather limited number of energy efficiency opportunities in the patterns of occupant behavior (see last column of Table 1).
With the “presence” aspect we simply mean the following: When occupants are absent, provision of services—and associated energy use—is obviously not necessary. Technical solutions may be adopted to address at least certain dimensions of this problem (e.g., occupancy sensing for lighting controls) such that all systems would automatically revert back to default, most energy-efficient states in the absence of occupants. Of course proper occupant behavior could also remediate this problem—for instance when occupants would simply turn off the lights when leaving rooms in a building. However, proper behavior is not merely a matter of occupants' attitude and consciousness. The design and configurations of building systems' hardware and software can both facilitate or impede proper behavior. For instance, heating and cooling systems would have to offer not only the possibility to schedule the course of set-point temperatures (to account for presence, time of the day/week, different rooms, different activities), but implement the possibility in terms of an intuitive and practical user interface.
Table 1 includes also, in the last column, the phrase “unusual” comfort preferences. But are comfort preferences out of the common ranges unreasonable? It seems sensible to remind occupants of the potential of adaptive measures (e.g., adjusting the clothing levels) in lieu of very high (low) heating (cooling) set-points. But what is to be done if occupants thus addressed remain unresponsive? What is to be done in cases where occupants in residential buildings insist on winter-time natural ventilation due to their attachment to a very purist notion of fresh air, other than perhaps confronting them—via dedicated energy use monitoring—with the economic implications of their behavior?
Another energetically detrimental mode of occupant behavior involves “improper” device operation. Instances of such improper control regime includes, for example, intensive winter-time (summer-time) natural ventilation when the building is in heating (cooling) mode. Likewise, under temperate conditions and when glare-free daylight is available, it would be unwise to keep shades closed and deploy electrical lighting. Also in these kinds of scenarios, the design and configuration of building control systems could contribute to or impede energy-conscious behavior. As it is not always straight-forward for occupants to gauge the type and time of proper control actions, presumably systems that would provide relevant information (outdoor conditions, heating/cooling systems' operational states) could be beneficial.
Be that as it may, it is not clear how we can integrate the above considerations pertaining to occupant presence, their comfort preferences, and the appropriateness of their control-oriented behavior into a coherent solution to the problem of performance gap as relevant to the prediction of buildings' future energy requirements. Granted, would we knew, at the design stage, the attitudinal stance (energy-consciousness) and the technical savviness of a building's future occupants (information level concerning optimal building control strategies), we would be probably in a better position to more reliably predict its future energy performance. Likewise, had we at our disposal reliable models to gauge the short-term, mid-term, and long-term effects of energy-relevant informational (or feedback) campaigns, we probably could do a better job of energy use predictions. But short of cases involving existing buildings with known occupant populations, it is difficult to fathom how we could become into possession of such kinds of information and such kinds of models. Note that it is not at all clear to which extent the typically modest sources of information that are currently available could be generalized, given the diversity of building types, locations, and populations.
The preceding principal analysis of the dependence of buildings' energy use on occupants' presence and behavioral patterns was not meant to belittle the importance of the quest for more advanced and versatile models of such patterns. Aside from the utility of data-driven occupancy models (particularly in case of operational optimization of existing buildings), all research geared toward a better understanding of occupant behavior is ultimately of great value to the building design and operation community. Rather, the objective was in a sense to point to an argumentative gap in the so-called performance gap research program (as outlined in the section The Performance Gap Research Program of this contribution). Improving the detail level and realistic feel of our occupancy models is in and of itself not a warrant for the closure of performance gap. Likewise, the extent and longevity of behavioral modification campaigns on buildings' energy use depend on a complex set of variables and are not necessarily generalizable for all kinds of building types, locations, and populations. Having said this, the performance gap research program may have the potential to incrementally improve energy demand assessment procedures, especially if the coverage and density of observational information regarding occupant behavior significantly increases in future. A relevant question in this context is as follows: In the absence of such rich and comprehensive empirical information on user behavior, can certain types of formal and simulation-supported analysis methods and tools nonetheless aid building design and operation professionals in view of systematic energy performance specification and projection, while taking the occupancy factors into account? We briefly address some aspects of this possibility in the next section of the paper.
Sensitivity and Uncertainty Analysis
In the foregoing discussion, we alluded to two shortcomings of the performance gap research program as commonly approached. The first shortcoming, addressed above, concerns the a priori assumption of occupants' behavior as a kind of default contributor. The second shortcoming is implied in the assumption that replacing single-value performance predictions with distributions of key performance indicator values could alleviate the performance gap problem, even if such distributions are based on insufficient empirical data. To give this assumption the benefit of doubt, in the following we examine two possibilities, whereby potential beneficiaries of building performance simulation are provided with distributions of predicted performance indicator values as opposed to single values. The first possibility (see section Illustration of the Performance Consequences of the Diversity of Occupants' Preferences) explores intuitive data presentation formats that are meant to visualize the consequences of the variation in occupancy-related model input assumptions on simulation results. The second possibility (see section Impact of Set-Point Distributions on Estimated Building Energy Demand) examines the effect of input variable distributions on the corresponding distributions of computed performance indicator values. Finally, we explore the possibility that, even though basic user-related regarding building occupants may be subject to inter-occupant diversity, their statistical distributions may be similar (see section Occupancy Presence Patterns in Office Buildings). This would mean that even if—without authentic empirical information—the assumptions regarding the basic value of such user-related data would be arbitrary, the assumptions regarding their stochastic distribution would not. Toward this end, the case study quoted in section Occupancy Presence Patterns in Office Buildings considers the variables pertaining to occupants' presence in an office building.
Illustration of the Performance Consequences of the Diversity of Occupants' Preferences
A typical way occupants can influence buildings' energy demand is related to their comfort-related preferences in view of indoor environmental conditions. A recent effort (Mahdavi et al., 2018) explored the degree to which the consideration of diversity in occupants' comfort-related preferences (as expressed in terms of various system operation set-points) can influence buildings' energy performance (as expressed via key performance indicators such as annual heating and cooling demand). Thereby, a mid-rise office building in Vienna, Austria was simulated with varying compositions of occupants in terms of their relative preferences concerning such set-points. In the absence of empirically-based information, the study was conducted based on the assumption of three general groups of occupants with differing preference ranges and corresponding—hypothesized—positive and negative energy performance implications. These implications were expressed in terms of typical performance indicators such as annual and peak heating and cooling loads:
(i) A first group of occupants (“H”) was assumed to have a narrower range of acceptable indoor environmental conditions expected to result in a higher energy demand.
(ii) A second group of occupants (“M”) was assumed to have more moderate expectations expected to result in a medium energy use level.
(iii) A third group of occupants (“L”) was expected to have the most flexible expectations expected to have lower energy demand implications.
The assumptions with regard to heating, cooling, lighting, and shading set-points for the three groups of occupants are summarized in Table 2. Furthermore, a number of scenarios with different compositions of occupants from the three above mentioned groups were defined and the implications of these scenarios for the simulated values of relevant key performance indicators were studied.
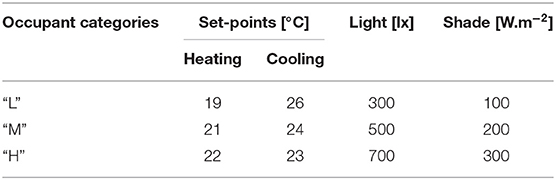
Table 2. Assumptions concerning the three occupant categories (“H,” “M,” “L”) regarding heating and cooling set-points, indoor illuminance threshold for operating electrical lighting, and vertical irradiance threshold [incident on the façade] for operating shades [adapted from Mahdavi et al. (2018) with permission from A. Mahdavi, M. Taheri, and F. Tahmasebi].
Figures 1, 2 illustrate the deviation of typical performance indicators (annual and peak heating and loads) from the benchmark (i.e., 100% type “H” occupants). The ratio of the number of offices with type “H” occupants to the total number of offices is shown on the x-axis, whereas the deviation of the key performance indicator from the benchmark is given on the y-axis. To better visualize the energy-related influence of the different buildings' populations, Figures 3, 4 illustrate the lines of equal deviation of the annual heating and cooling demand from the benchmark. The deviations depend on the ratio of the three groups of occupants (“H,” “M,” and “L”) to the total population.
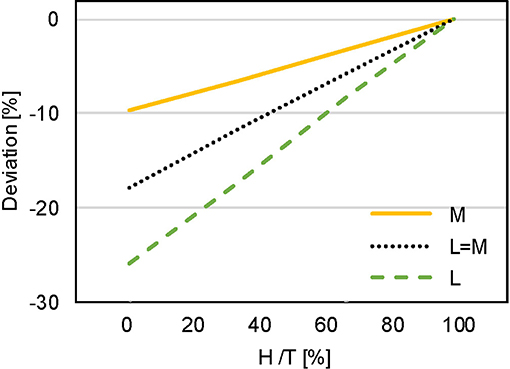
Figure 1. Deviation of the annual heating demand from the benchmark, depending on the fraction of H, M, and L in total population. Reproduced from Mahdavi et al. (2018) with permission from A. Mahdavi, M. Taheri, and F. Tahmasebi.
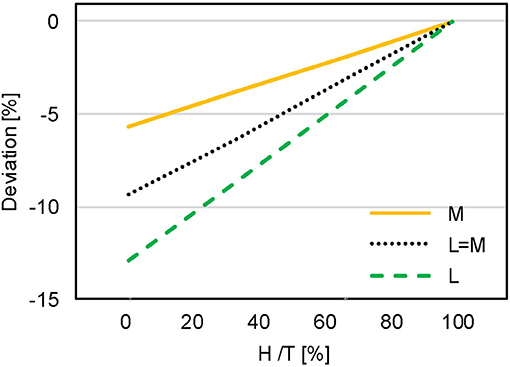
Figure 2. Deviation of the peak heating load from the benchmark, depending on the fraction of H, M, and L in total population. Reproduced from Mahdavi et al. (2018) with permission from A. Mahdavi, M. Taheri, and F. Tahmasebi.
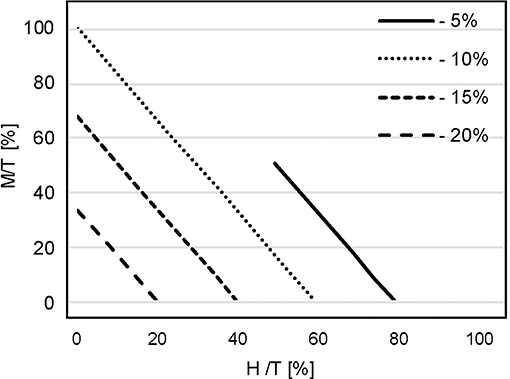
Figure 3. Lines of equal deviation (from −5 to −20%) of the annual heating demand from the benchmark, depending on the fraction of H, and M in total population. Reproduced from Mahdavi et al. (2018) with permission from A. Mahdavi, M. Taheri, and F. Tahmasebi.
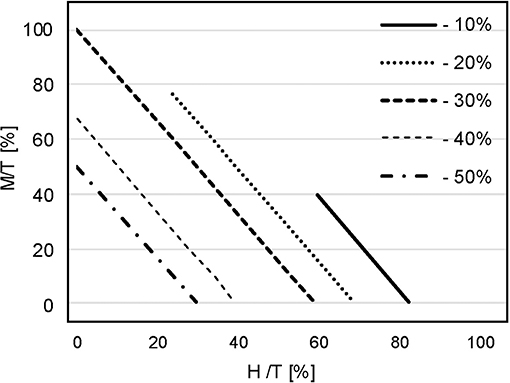
Figure 4. Lines of equal deviation (from −10 to −50%) of the annual cooling demand from the benchmark, depending on the fraction of H, and M in total population. Reproduced from Mahdavi et al. (2018) with permission from A. Mahdavi, M. Taheri, and F. Tahmasebi.
This case study illustrates both the potential and the limitations of efforts to predict occupants' influence on buildings' future energy performance. The study shows the theoretically ascertainable impact of the variability of the most basic modeling assumptions such as heating and cooling set-point temperatures on buildings' energy use predictions. Given a specific context (climatic region, urban context, building typology, building systems, and fabrics), the sensitivity of key performance indicator values (KPIs) to model input variations can be computed and visualized. But in the absence of real (empirically secured) estimation of the relevant population's traits and distributions (e.g., thermal comfort preferences of future occupants), the utility of the computed ranges of KPIs remain rather limited.
Impact of Set-Point Distributions on Estimated Building Energy Demand
As mentioned before, it has been suggested that, instead of mere single numbers, energy performance calculation results should include uncertainty ranges. As sensible as this suggestion is, it does not specify the basis for the specification of such uncertainty ranges. Again, there may be a theoretical possibility, that computed uncertainty ranges for performance indicators could be, to a certain extent, resistant to the ranges and shapes of associated input data distributions. A recent case study explored this possibility via systematic simulation runs applied to a sample building in Italy (Berger et al., 2020). Thereby, we considered the implications of different distribution shapes and ranges of occupancy-related input data assumptions for computed values of key performance indicators such as annual heating demand. To exemplify the results of this study, consider five different distributions of computed annual heating demand of a typical residential building in Milan, Italy as shown in Figure 5. The question is, what constitutes the informational value of these very different distributions for those who are meant to interpret and utilize the simulation results? In the present case, the diversity of the distributions is the consequence of the—arbitrarily assumed—input data variations with regard to heating temperature set-point frequencies, namely three normal distributions with three different widths, namely narrow (N_N), standard (N_S), and wide (N_W) as well as one left-skewed distribution (S_L) and one right-skewed distribution (S_R) (see Figure 6). It could be argued that the simulation results' distribution would be more persistent (less prone to variation of input variable distributions), if more than one input variable would be simultaneously and randomly varied in the course of Monte Carlo simulations. Figures 7, 8 display the results of a preliminary test of this possibility. Figure 7 shows three very different distributions of computed heating demand (qa, h) of the aforementioned residential object. In this case, two key input variables, namely heating temperature set-point (θsp−h) and air change rate (ACH) were concurrently randomized each with three different distribution functions (A, B, and C) as shown in Figure 8. As these preliminary results seem to suggest, there is no evidence that extensive yet arbitrary variations of input data distributions must result in reproducible and consistent distributions of computed key performance indicator values. As with the previous case study, we must conclude that the mere inclusion of uncertainty ranges in declaration of energy performance predictions may be of limited value, as long as the underlying input data variations are not empirically grounded.
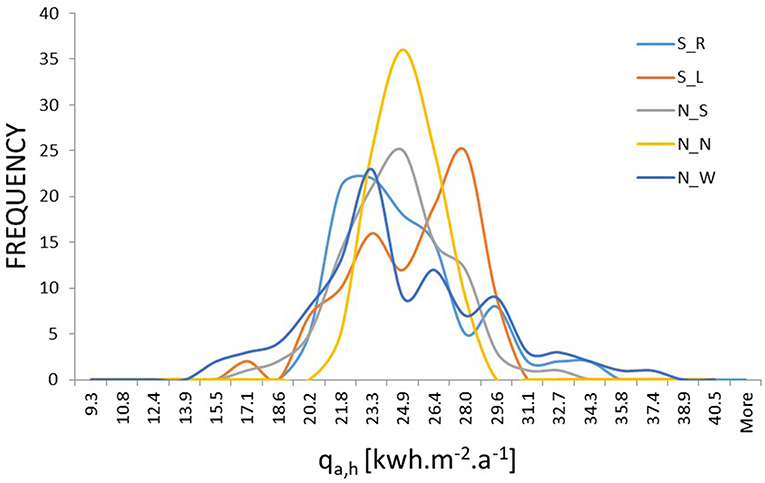
Figure 5. Computed annual heating demand distributions as a consequence of assumed input variable distributions (see Figure 6). Reproduced from Berger et al. (2020) with permission from C. Berger, E. Primo, D. Wolosiuk, V. Corrado, and A. Mahdavi.
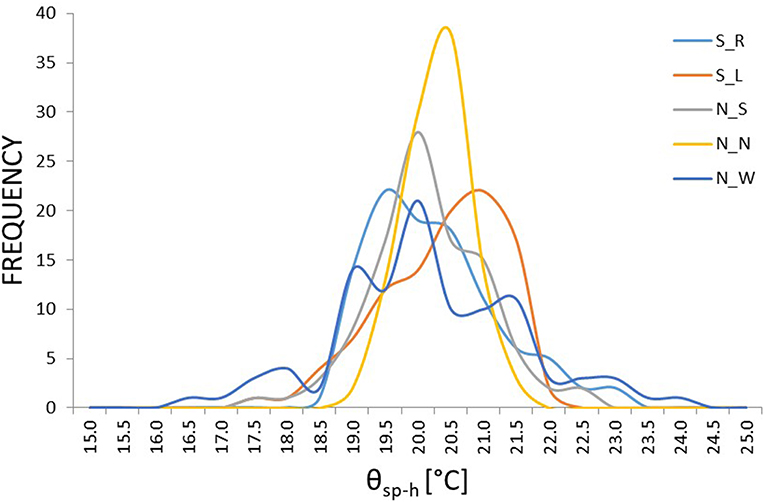
Figure 6. Assumed heating temperature set-point distributions toward computation of annual heating demand (see Figure 5). Reproduced from Berger et al. (2020) with permission from C. Berger, E. Primo, D. Wolosiuk, V. Corrado, and A. Mahdavi.
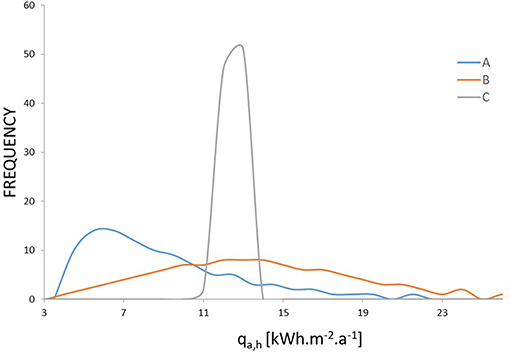
Figure 7. Computed distributions of annual heating demand as a consequence of assumed input variable distributions (see Figure 8). Reproduced from Berger et al. (2020) with permission from C. Berger, E. Primo, D. Wolosiuk, V. Corrado, and A. Mahdavi.
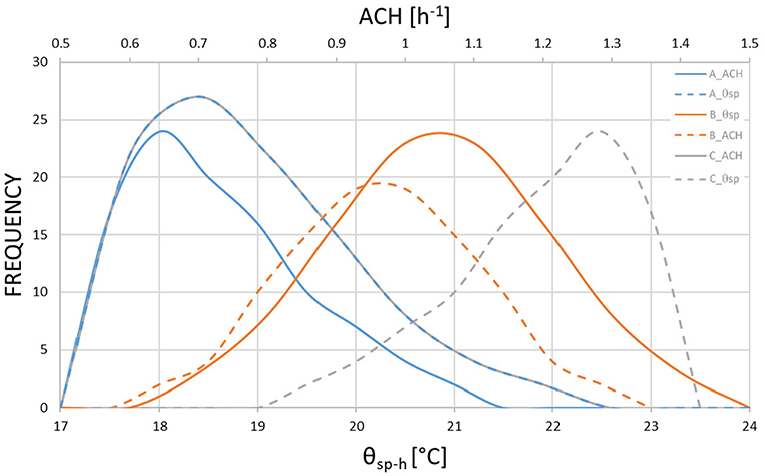
Figure 8. Selected distributions of input variables θsp−h and ACH toward computation of annual heating demand (see Figure 7). Reproduced from Berger et al. (2020) with permission from C. Berger, E. Primo, D. Wolosiuk, V. Corrado, and A. Mahdavi.
Occupancy Presence Patterns in Office Buildings
A recent study explored the statistical variance of the defining markers of presence patterns in an office building area in an educational building (see Mahdavi and Tahmasebi, 2015 for details). Such markers include, for example, occupants' first arrival times (FA), last departure times (LD), presence duration (PD), number of transitions between occupied and vacant states (NT), working hours (WH), absence duration (AD), and fraction of presence (FOP). Whereas, the mean values of such markers could be arguably assumed to be very different amongst different office workers, we could speculate that their statistical distributions could be similar. Table 3 summarizes, for the eight occupants working in this area and for some of the aforementioned markers, the observed presence data in terms of three basic statistics, namely mean, standard deviation, coefficient of variation (CV). Despite the small number of occupants, the values of the eight markers for all occupants were processed in terms of probability distribution box plots. Figures 9–11 provide instances of such box plots (for FA, WH, and FOP). The results support a number of observations:
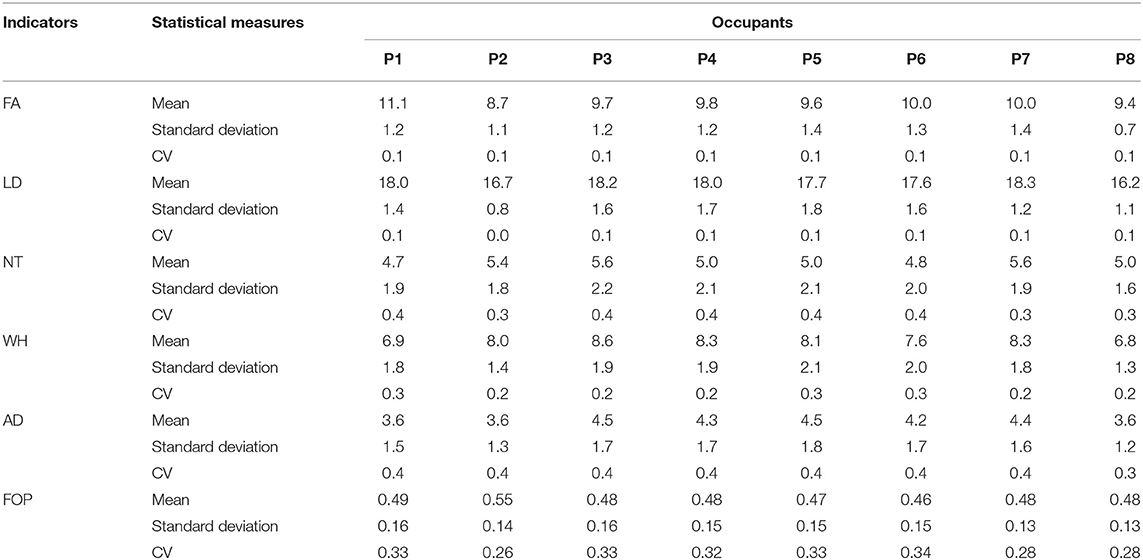
Table 3. Summary of the statistical analysis results [adapted from Mahdavi and Tahmasebi (2015) with permission from A. Mahdavi and F. Tahmasebi].
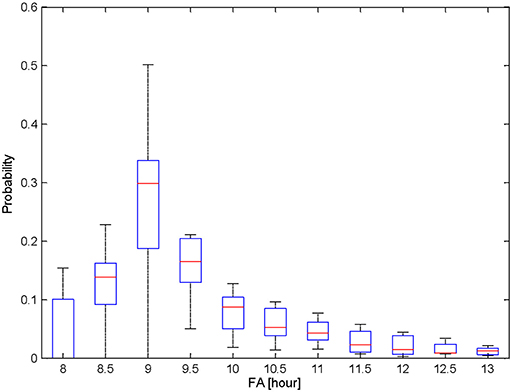
Figure 9. First arrival time boxplot. Reproduced from Mahdavi and Tahmasebi (2015) with permission from A. Mahdavi, and F. Tahmasebi.
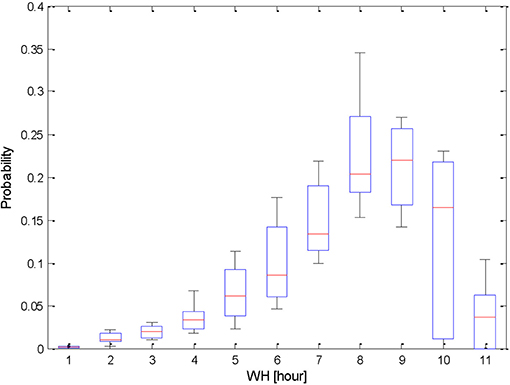
Figure 10. Working hours boxplot. Reproduced from Mahdavi and Tahmasebi (2015) with permission from A. Mahdavi, and F. Tahmasebi.
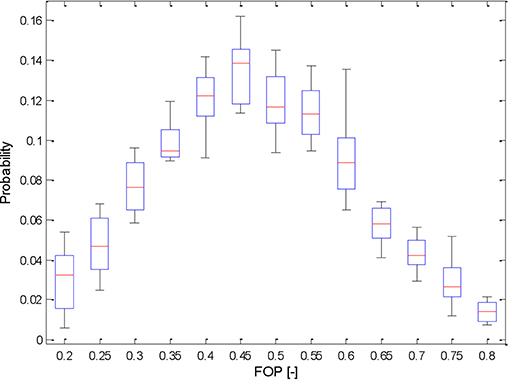
Figure 11. Fraction of presence boxplot. Reproduced from Mahdavi and Tahmasebi (2015) with permission from A. Mahdavi, and F. Tahmasebi.
Statistically speaking, some indicators (PD, NT, FOP) appear to display a normal (symmetrical) distribution pattern, whereas others (FA, LD, WH) are non-symmetric (see Figures 9–11). Specifically, as one could expect, FA is left skewed (most arrivals occur before noon) and LD is right skewed (most departures occur after noon). Likewise, WH is plausibly right skewed, as the probability of shorter than normal working hours is higher than extremely long ones. Certain markers (FOP, NT) show a somewhat higher level of consistency across multiple occupants (as expressed in the values of the statistics considered), suggesting that their values might be less prone to occupants' diversity.
More importantly, considering CV as a classical statistical dispersion measure applied to the distribution of the markers' values, we notice that its values do not vary much across different occupants (see Table 3). If confirmed by more extensive studies in the future, this finding could be of significance: Whereas the absolute averaged long-term values of occupancy pattern markers are very different from one occupant to another, the extent of variance (or dispersion) of the values could be statistically similar, consistent, and—at least to a certain degree—resistant to occupants' diversity. Consequently, basic information about the shape of distribution (normal, skewed, etc.) together with the dispersion information can provide a reliable basis for the randomization of typical occupancy schedules. This in turn can provide the basis for stochastic occupancy models suitable for deployment in building performance simulation applications. Needless to say, the credence of the above conclusions needs to be re-examined in future studies involving different settings, different building types, and generally a much broader empirical data set based on a much larger number of occupants.
Conclusion
We provided a critical analysis of the “performance gap” notion and the associated research program. This program identifies occupants as a major contributor to the observed discrepancies between predicted and actual energy performance of buildings. Consequently, it assigns priority to more accurate predictions of user presence and behavior in buildings (typically, via pursuit of probabilistic modeling approaches) as well as efforts to encourage more energy-conscious user behavior (typically, via information campaigns or dynamic energy-centric feedback mechanisms).
Notwithstanding the value of such efforts, we argued that their premises and potential have not been sufficiently and realistically gauged. Toward this end, we suggested the need for a more systematic assessment of the scope of users' energy-relevant impact on buildings' performance. To facilitate the related discourse, we introduced a structured representation of the items and categories of a conceptual user influence space. This representation suggests, that not all user-related energy inefficiency factors can be ameliorated by users themselves. Whereas, in certain cases waste of energy can be indeed caused by improper user behavior, in other instances poorly designed, configured, and operated environmental control systems might carry the bulk of responsibility. Likewise, it is not clear what is the relative weight of seemingly unreasonable user-desired indoor environmental conditions in buildings' total energy use, and if and to which extent occupants should or could be convinced toward rethinking their preferences. Given these observations, we concluded that one should be careful with across-the-board declaration of human behavior as the central contributor to performance gap and wasteful energy use.
We further addressed the potential for and usefulness of the frequently promoted probabilistic methods and uncertainty analysis in view of their potential as an effective remedy to the performance gap dilemma. Thereby, a key question pertains to the usefulness of uncertainty analysis for buildings' energy evaluation processes in those cases where the assumed priors are not empirically grounded. As such, we suggested that morphological incorporation of stochastic features in occupancy-related model input data does not necessarily translate into more accurate predictions of occupancy patterns and behavior as relevant to specific building designs. Simply replacing conventional (schedule-based) occupant presence and (rule-based) behavioral models in simulation tools with stochastic models must not result in higher predictive accuracy of the values of energetically relevant key performance indicators.
Finally, we also reflected on the potential of various forms of uncertainty analyses in the context of the performance gap problems. Toward this end, we considered a number of cases studies, whereby potential beneficiaries of building performance simulation are provided with distributions of predicted performance indicator values as opposed to single values. The results of the related treatment can be summarized as follows: Adequate data presentation formats can effectively visualize the consequences of the variation in occupancy-related model input assumptions for the simulation results. However, the value of such visualizations is proportional to the validity and robustness of the underlying empirical information. Likewise, the results of computational energy performance modeling can be of course presented not only in terms of single values, but in terms of distributions of computed performance indicator values. However, the utility of such distributions is again limited, if they are not based on empirically grounded information on the corresponding distributions of the input variable values.
Given the multiple layers of genuine uncertainty concerning the long-term evolution of a number of salient variables related to buildings and their internal and external boundary conditions, we might have to bid farewell to the idea that the so-called performance gap can be entirely closed. This does not mean that we should not aspire to a higher fidelity level with regard to occupant-specific assumptions when projecting buildings' future performance. Emulation of the stochastic feel of realistic occupancy patterns may be useful in view of a number of specific building engineering questions and problems. But a specific modeling formalism, in and of itself, does not warrant more accurate predictions into the future. Ultimately, availability of ever more comprehensive repositories of actual occupancy information (covering multiple climatic boundary conditions, building types, populations) could provide the basis for more reliable behavioral models and derivative building codes and standards as well as energy policies. While not fully closing the performance gap, this could indeed significantly improve the consistency and transparency of future evidence-based building design and operation processes and practices.
Data Availability Statement
All datasets for this study are included in the article/supplementary material.
Author Contributions
AM was primary author of this paper. He selected the topic of the inquiry, formulated the main research questions, and suggested the approach to treat those. CB participated in the development of the paper's structure and content. Specifically, she was instrumental in conducting a number of simulation studies whose results were incorporated in this paper.
Funding
The authors acknowledge TU Wien Bibliothek for financial support through its Open Access Funding Programme.
Conflict of Interest
The authors declare that the research was conducted in the absence of any commercial or financial relationships that could be construed as a potential conflict of interest.
Acknowledgments
The authors' contributions to the occupancy research in general and the specific treatment of the present paper in particular have benefited from their participation in the IEA EBC Annex 79 activities. The authors want also like to acknowledge the contributions by Dawid Wolosiuk and Farhang Tahmasebi toward generation of some of the simulation-based data used in this paper.
References
Andersen, R. K., Fabi, V., and Corgnati, S. P. (2016). Predicted and actual indoor environmental quality: verification of occupants' behavior models in residential buildings. Energy Build. 127, 105–115. doi: 10.1016/j.enbuild.2016.05.074
Andersen, R. K., Olesen, B. W., and Toftum, J. (2007). “Simulation of the effect of occupant behavior on indoor climate and energy consumption,” in Proceedings of Clima 2007, 9th REHVA World Congress: Wellbeing Indoors (Helsinki).
Berger, C., Primo, E., Wolosiuk, D., Corrado, V., and Mahdavi, A. (2020). “The impact of occupancy-related input data uncertainty on the distribution of building simulation results,” in Proceedings of the 4th Building Simulation Applications Conference – BSA 2019 (Bolzano).
Clarke, J. (2007). Energy Simulation in Building Design. London: Taylor & Francis. doi: 10.4324/9780080505640
Hensen, J., and Lamberts, R. (2019). Building Performance Simulation for Design and Operation. London: Routledge. doi: 10.1201/9780429402296
Hong, T., Taylor-Lange, S.C., D‘Oca, S., Yan, D., and Corgnati, S. P. (2016). Advances in research and applications of energy-related occupant behavior in buildings. Energy Build. 116, 694–702. doi: 10.1016/j.enbuild.2015.11.052
Jia, M., Srinivasan, R. S., and Raheem, A. A. (2017). From occupancy to occupant behavior: an analytical survey of data acquisition technologies, modeling methodologies and simulation coupling mechanisms for building energy efficiency. Renew. Sust. Energy Rev. 68, 525–540. doi: 10.1016/j.rser.2016.10.011
Mahdavi, A., Taheri, M., and Tahmasebi, F. (2018). “Derivation and representation of the energetically relevant consequences of occupants' presence and behaviour in buildings,” in Proceedings of BauSim2018, eds A. P. Wagner and von Both (Karlsruhe).
Mahdavi, A., and Tahmasebi, F. (2015). “The inter-individual variance of the defining markers of occupancy patterns in office buildings: a case study,” in Proceedings of BS2015. 14th Conference of IBPSA (Hyderabad), 2243-2247.
Mahdavi, A., and Tahmasebi, F. (2019). “People in building performance simulation,” in Building Performance Simulation for Design and Operation, eds J. Hensen and R. Lamberts (Routledge), 117–145. doi: 10.1201/9780429402296-4
Menezes, A., Cripps, A., Bouchlaghem, D., and Buswell, R. (2012). Predicted vs. actual energy performance of non-domestic buildings: Using post-occupancy evaluation data to reduce the performance gap. Appl. Energy 97, 355–364. doi: 10.1016/j.apenergy.2011.11.075
Ueno, T., Inada, R., Saeki, O., and Tsuji, K. (2005). “Effectiveness of displaying energy consumption data in residential houses - analysis on how the residents respond,” in Proceedings of ECEEE 2005 (Mandelieu-La Napoule), 1289–1299.
Yan, D., O‘Brien, W., Hong, T., Feng, X., Gunay, H. B., Tahmasebi, F., et al. (2015). Occupant behavior modeling for building performance simulation: Current state and future challenges. Energy Build. 107, 264–278. doi: 10.1016/j.enbuild.2015.08.032
Keywords: buildings, energy, performance gap, prediction, simulation
Citation: Mahdavi A and Berger C (2019) Predicting Buildings' Energy Use: Is the Occupant-Centric “Performance Gap” Research Program Ill-Advised? Front. Energy Res. 7:124. doi: 10.3389/fenrg.2019.00124
Received: 30 August 2019; Accepted: 23 October 2019;
Published: 08 November 2019.
Edited by:
Valentina Serra, Politecnico di Torino, ItalyReviewed by:
Tianzhen Hong, Lawrence Berkeley National Laboratory, United StatesCristina Becchio, Politecnico di Torino, Italy
Copyright © 2019 Mahdavi and Berger. This is an open-access article distributed under the terms of the Creative Commons Attribution License (CC BY). The use, distribution or reproduction in other forums is permitted, provided the original author(s) and the copyright owner(s) are credited and that the original publication in this journal is cited, in accordance with accepted academic practice. No use, distribution or reproduction is permitted which does not comply with these terms.
*Correspondence: Ardeshir Mahdavi, amahdavi@tuwien.ac.at