A Guideline for Life Cycle Assessment of Carbon Capture and Utilization
- 1Institute for Technical Thermodynamics, RWTH Aachen University, Aachen, Germany
- 2Department of Technical Chemistry, Technische Universität Berlin, Berlin, Germany
- 3Fraunhofer Institute for Solar Energy Systems (ISE), Freiburg, Germany
- 4Institute of Energy and Climate Research - Energy Systems Engineering (IEK-10), Forschungszentrum Jülich GmbH, Jülich, Germany
Carbon Capture and Utilization (CCU) is an emerging field proposed for emissions mitigation and even negative emissions. These potential benefits need to be assessed by the holistic method of Life Cycle Assessment (LCA) that accounts for multiple environmental impact categories over the entire life cycle of products or services. However, even though LCA is a standardized method, current LCA practice differs widely in methodological choices. The resulting LCA studies show large variability which limits their value for decision support. Applying LCA to CCU technologies leads to further specific methodological issues, e.g., due to the double role of CO2 as emission and feedstock. In this work, we therefore present a comprehensive guideline for LCA of CCU technologies. The guideline has been development in a collaborative process involving over 40 experts and builds upon existing LCA standards and guidelines. The presented guidelines should improve comparability of LCA studies through clear methodological guidance and predefined assumptions on feedstock and utilities. Transparency is increased through interpretation and reporting guidance. Improved comparability should help to strengthen knowledge-based decision-making. Consequently, research funds and time can be allocated more efficiently for the development of technologies for climate change mitigation and negative emissions.
Introduction
Carbon capture and utilization (CCU) involves the capture of the greenhouse gas CO2 from point sources or ambient air and its subsequent conversion into valuable products (Baena-Moreno et al., 2019). By converting CO2 into valuable products, CCU aims to improve economic benefits while also reducing environmental impacts such as the impact on climate change or fossil resource depletion (Al-Mamoori et al., 2017). However, the reduction of environmental impacts cannot be taken for granted: high energetic co-reactants such as hydrogen (Sternberg and Bardow, 2016) or epoxide are often needed to activate the chemically inert CO2 (von der Assen and Bardow, 2014). The production of these high energetic co-reactants, however, is associated with high environmental impacts. Thus, whether CCU technologies reduce environmental impacts can only be concluded from a detailed environmental assessment. A method for environmental assessment with broad acceptance among academic and industrial practitioners is Life Cycle Assessment (LCA).
Even though, LCA has been standardized in ISO 14040/14044 (European Committee for Standardisation, 2009, 2018), the standard leaves methodological choices, e.g., for selecting the functional unit, system boundaries, background processes, or environmental impact assessment methods (European Commission, 2018). As a result, LCA studies on CCU technologies are often not comparable, e.g., because of differences in functional units, i.e., the relative basis for which environmental impacts are assessed, or system boundaries. Even if functional units and system boundaries are comparable, LCA results often show significant variation for identical technologies, because different processes are selected for the production of feedstocks or utilities. In particular, the supply of electricity or hydrogen has been shown to vary largely between various LCA studies such that conclusions even change qualitatively (Artz et al., 2018). For example, for CO2-based methanol, LCA studies in the literature reported cradle-to-gate carbon footprints between −1.7 and +9.7 kg of CO2, eq per kg of methanol. The conclusions of these studies are thus qualitatively very different: CO2-based methanol could either even be a carbon sink over its life cycle or emits much more CO2 than fossil-based methanol. However, harmonization of assumptions regarding supply of electricity and CO2 reduced the variation such that all carbon footprints had the same sign and could be clearly distinguished from fossil-based methanol. The current lack of a consistent basis for LCA hampers proper decision making of stakeholders involved in CCU technologies and, in fact, may also lead sub-optimal decisions. As a result, both the academic literature (Cuéllar-Franca and Azapagic, 2015; Naims et al., 2015) and the Scientific Advice Mechanism (SAM) of the European Commission (European Commission, 2018) call for specific guidelines for LCA on CCU technologies.
Specific LCA guidelines have been developed in several areas such as e.g., photovoltaic electricity (Frischknecht et al., 2016), buildings (Malmqvist et al., 2011), aggregates for construction (Blengini et al., 2012), bio-based product (European Commission - Joint Research Center, 2011b, 2018), or industrial symbiosis (Mattila et al., 2012). These specific LCA guidelines have also been developed based on the ISO standard and further refined based on main research questions in the specific area. By providing a consistent framework to address these main research questions, specific LCA guidelines have been important in advancing the adoption of environmental assessment in the respective fields.
We therefore developed the first guideline for standardized LCA of CCU technologies (Zimmerman et al., 2018). The guideline aims to enhance transparency, comparability and reliability of LCA studies for CCU technologies. In particular, the guideline identifies pitfalls causing ambiguity when assessing the environmental impact reduction potential of CCU technologies and offers guidance on how to avoid these pitfalls based on existing standards and guidelines. The guideline was developed in a 1-year project in cooperation with TU Berlin, University of Sheffield and the Institute for Advanced Sustainability Studies in Potsdam. A guideline for Techno-economic Assessment (TEA) was developed in parallel (Zimmerman et al., 2018).
In this paper, we present the content of the guideline tailored for a broad scientific audience. For this purpose, we give an overview of the development process of the guidelines in section Approach. In section General Introduction to Life Cycle Assessment, we present the content of the guideline. Here, we provide a short introduction to LCA followed by the identified pitfalls sorted according to the four phases of LCA. For the first phase of LCA, the goal and scope definition, we provide examples for goal definitions for studies on CCU technologies, unified functional units and system boundaries with respect to the goal of the study and a hierarchy of methods to solve multi-functionality. For the second phase, the life cycle inventory, we present general requirements for the selection of inventory sets and reference processes and methods to bridge data gaps. For the third phase, the life cycle impact assessment, we provide guidance for the selection of impact assessment methods with respect to regions and on how to account for temporary storage of CO2. In the fourth and last phase of LCA, the life cycle interpretation, we specify requirements for uncertainty and sensitivity analysis, provide guidance on how to interpret neutral and negative environmental impacts.
Approach
The basis for the guideline was literature on LCA methodology covering the following standards (European Committee for Standardisation, 2009, 2017, 2018; BSI, 2011; AFNOR, 2016), guidelines (World Resources Institute and World Business Council for Sustainable Development; European Commission - Joint Research Center, 2011a, 2012), textbooks (Baumann and Tillman, 2004; Guinée, 2006; Curran, 2012), and scientific peer-reviewed publications on LCA methodology and LCA studies on CCU (Artz et al., 2018). Building upon this analysis, a first draft document was created and discussed with six external experts (1 industry, 2 academia, 3 policy; 5 countries; 60% women) during the first of two in-person discussion workshops. The discussions of workshop 1 provided the basis further development of guidelines, which was then discussed with a large group of 39 external participants (26% industry, 46% academia, 28% policy; 11 countries, 28% women) during the second workshop. After the second workshop, the guideline was revised and then finally reviewed by three academic researchers and an official of the German Federal Environmental Agency (UBA) (“peer-review”). After final revision, the guideline document were published in Deep Blue Data, the repository of the University of Michigan.
In this paper, we present the methodological core of the LCA guidelines, in particular shall, should and may rules. Shall rules are the minimum requirements that are recommended to achieve a standardized TEA/LCA for CCU. Every LCA produced using this guideline must cover these basic rules. All rules in this category have to be addressed. Should rules cover a recommended level of analysis and should be applied to produce LCA of greater depth. Use of may rules produces the greatest detail of LCA. These rules may not be applicable in all studies and should be applied as determined by the practitioner. Note that this work leaves out major parts of LCA basics contained in the full guideline.
General Introduction to Life Cycle Assessment
Life cycle assessment is a methodology to account for the environmental impacts of a product or service throughout its entire life cycle (Figure 1). The entire life cycle spans from cradle-to-grave: from raw material extraction through production, packaging, use, end-of-life treatment and recycling to final disposal. Through each stage, the product's life cycle interacts with the environment by consuming natural resources and emitting pollutants. Life cycle assessment is a quantitative method to describe these interactions and their potential environmental impacts1. Due to its holistic approach, LCA avoids problem shifting between both environmental impact categories and life cycle stages. Therefore, LCA is a valuable tool in various fields, e.g., product or process design, decision making in industry and policy as well as marketing. The LCA methodology was standardized in the 1990s by the international standardization organization (ISO) in ISO 14040 and 14044 and is still updated and extended regularly (most recently in May 2018).
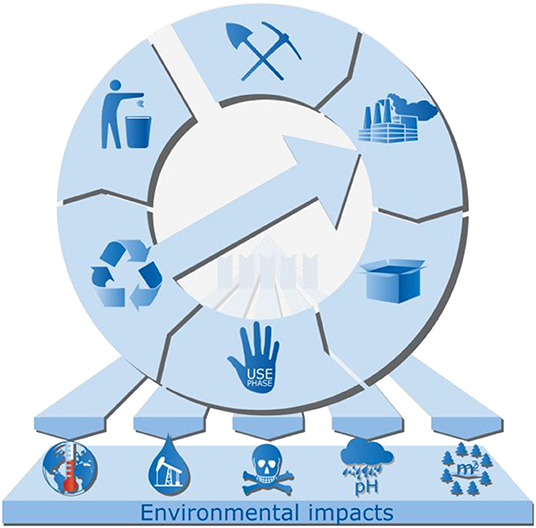
Figure 1. The holistic approach of life cycle assessment accounts for environmental impacts associated over the entire life cycle with all stages of product's life cycle (circle in the middle).
According to the ISO standard, a LCA study is sub-divided in four phases (Figure 2):
1. Goal and Scope definition
2. Life cycle inventory analysis
3. Life cycle impact assessment
4. Interpretation.
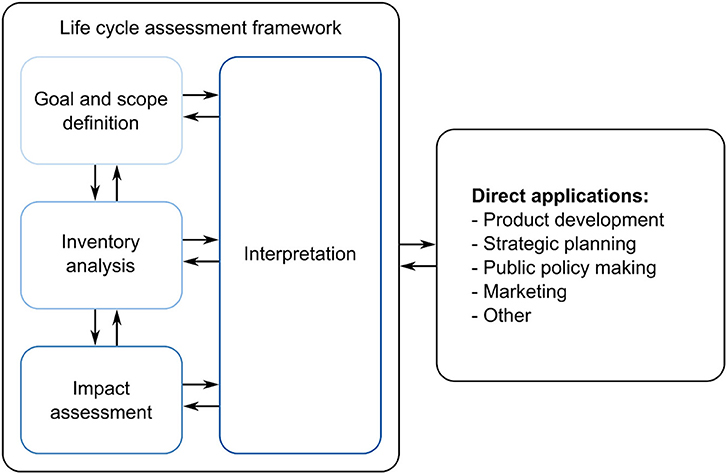
Figure 2. General framework for life cycle assessment (European Committee for Standardisation, 2009).
All phases are interdependent, e.g., the gathered life cycle inventories have to fit to the goal and scope with respect to time and space. In practice, this interdependence renders LCA an iterative approach, as data availability is often not fully known at the beginning of an LCA study. Furthermore, the entire life cycle assessment framework is influenced and by its supposed direct applications and the other way around (Figure 2).
Goal Definition
Every LCA study starts with the goal definition by unambiguously stating “the intended application of the study, the reasons for carrying out the study, the intended audience of the study and whether the results are to be used in comparative assertions disclosed to public (European Committee for Standardisation, 2018).” In other words, the central question of the study is defined. However, many questions may be answered by LCA. To get an overview, we here start by identifying typical goal definitions for CCU from literature. Most CCU technologies are in stages of early development and aim to reduce environmental impacts. Therefore, most LCA studies on CCU aim at quantifying the potential environmental impact reductions of CCU processes or products in comparison to existing processes (Aresta and Galatola, 1999; Aresta et al., 2002; Kim et al., 2011; Anicic et al., 2014; Souza et al., 2014; van der Giesen et al., 2014; von der Assen and Bardow, 2014; Luu et al., 2015; Al-Kalbani et al., 2016; Garcia-Herrero et al., 2016; Hoppe et al., 2016, 2017; Matzen and Demirel, 2016; Schakel et al., 2016; Sternberg and Bardow, 2016; Parra et al., 2017; Sternberg et al., 2017; Uusitalo et al., 2017; Zhang et al., 2017). Most studies also include a contribution analysis of environmental impacts to identify opportunities for improvement (Aresta and Galatola, 1999; Aresta et al., 2002; Kim et al., 2011; van der Giesen et al., 2014; von der Assen and Bardow, 2014; Luu et al., 2015; Garcia-Herrero et al., 2016; Matzen and Demirel, 2016; Schakel et al., 2016; Parra et al., 2017; Uusitalo et al., 2017). One study, however, focused identifying the CCU technology most efficiently using surplus energy (Sternberg and Bardow, 2015). Once CO2-based products are deployed in the markets, LCA can be used for environmental product declaration (CarbonCure; Carbon Recycling International; Audi, 2017).
From this short literature review, the most common research questions are derived:
1. What is the environmental impact reduction of a CCU-based product or service compared to the same product or service derived from fossil carbon sources?
2. What are the contributions to the environmental impacts of a CCU product/process over the life cycle and where are hot spots to reduce environmental impacts?
3. What CCU technology to make efficient use of renewable energy?
4. What are the environmental footprints of products or services used as basis for customer decisions (product declaration)?
All of these research questions imply a comparison between alternatives (explicit or implicit) and thus, intend to support decision making, e.g., which process to use, how to improve the technology or which product to buy (refers to product declaration). In most cases, CCU technologies aim to produce products that are already offered in the market. For this reason, this guideline focusses on comparative assessments, or assessments that are to be used in comparative assertions. Goal definition should use the research questions listed above to derive the specific research question of the study. In addition, the requirements of the ISO 14044 shall be fulfilled as listed in the beginning of this section.
For CCU technologies in stages of early development (low technology readiness level, TRL), studies can end up in apple vs. oranges comparisons, since most reference technologies are mature and have been optimized over decades. In contrast, low TRL processes usually have higher energy demand or solvent consumption because of not yet established heat integration and/or process optimization. At the same time, low TRL processes lack auxiliary processes such as product purification steps after reaction. Thus, LCA studies on lab-scale processes can both under- or over-estimate environmental impacts. These aspects should always be considered in comparative studies if a high TRL technology is compared to a low TRL technology. In early development stages, LCA is most useful to identify hot spots for environmental improvement via contribution followed by a sensitivity analysis. However, a comparison between a low TRL CCU technology and a high TRL reference technology can still reveal valuable insights to guide research. Furthermore, ex-ante assessments may be applied to compare the current low TRL technology at a future industrial scale-up TRL with the future reference process or the technology development (Pehnt, 2006; Gavankar et al., 2015b; Kaetelhoen et al., 2015, 2016; Arvidsson et al., 2017; Villares et al., 2017; Cucurachi et al., 2018). However, the prediction of future developments introduces another source of uncertainty.
Scope Definition
The scope definition shall describe under which conditions and assumptions the results of the study are valid. Therefore, every aspect of the scope definition is closely related to and has to be in line with the study's goal (European Committee for Standardisation, 2009, 2018; European Commission - Joint Research Center, 2010).
Defining Functional Units for CCU Technologies
Life cycle assessment quantifies the environmental impacts of a product or process system on a relative basis with respect to its function, e.g., global warming impact per kg of product (European Committee for Standardisation, 2009). This relative basis is called functional unit, which quantifies the performance of a product system or service. As most LCA studies on CCU aim at comparing CCU technologies to a benchmark or results are used for comparisons, the functional unit should ensure the sound comparison of the assessed technologies. However, different LCA studies on identical technologies may apply different functional units, which complicates comparisons between studies or even makes them incomparable (Artz et al., 2018). To increase comparability among studies, we derive functional units for each class of CCU technologies from current LCA practice and derived a decision tree to define a suitable functional unit.
For products with identical chemical structure and composition to their conventional counterparts, in general, mass shall be used as a basis for comparison since this is the most common trading unit for chemicals, materials and minerals (Figure 3). Other bases for comparison, e.g., amount of species, volume or exergy, could also be applied. However, as enhanced comparability is a major objective of this guideline, we recommend using mass for comparisons, since mass is the most common measure of trading.
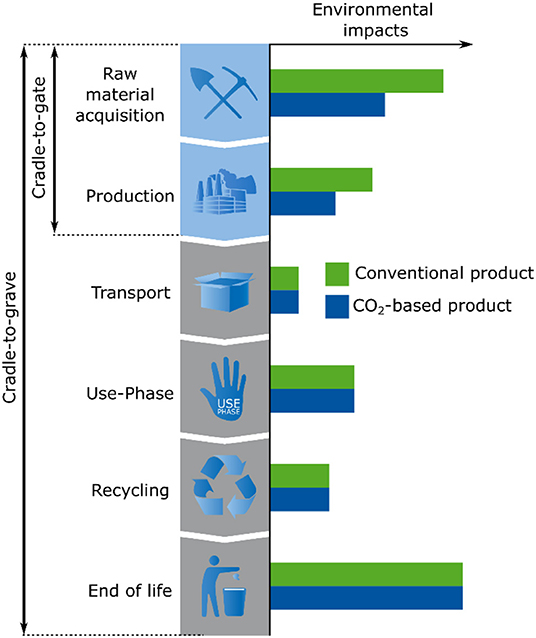
Figure 3. Illustration of a comparison of environmental impacts for products with identical chemical structure and composition over the entire life cycle. Impacts shown as bars on the x-axis only differ during the phases raw material acquisition and production and thus, comparative studies only have to consider these phases.
In case of fuels with identical chemical structure and composition, energy content (based on the lower heating value, LHV) shall be used, since the value of fuels is measured by their energy content. The lower heating value is recommended since in most energy services the condensation enthalpy of formed water is not accessible due to exhaust temperatures above 100°C, e.g., power plants, internal combustion engines and most boilers.
For CO2-based products with different chemical structure and composition to their conventional counterparts, a generic functional unit cannot be defined. Instead, the functional unit shall be defined so that the technical performance in the defined application of the products becomes comparable, e.g., compare detergents based on the washing performance and not based on mass.
The functional unit of CO2-based fuels with different chemical structure and composition shall be defined with respect to the purpose of the fuel, i.e., energy services provided. Energy services are for example the supply of electricity or heat or the transportation of persons or goods. The functional unit has to quantify either the precise energy service (e.g., 1 MJ of electricity from a gas turbine of a certain type) or the distance for freight or person transport (e.g., one person km driven in a specified vehicle/ship/aircraft), since combustion properties may be different and thus, comparability based on energy content is not guaranteed (Deutz et al., 2018).
Energy storage delays the use of energy to a later time than when it is was generated and thus, decouples supply and demand of energy (The European Parliament the council of the European Union, 2019). Through decoupling demand and supply, energy storage offer additional degrees of freedom to operate the energy generation in a more efficient way and thus, can lead to lower environmental impacts. However, potential impact reductions strongly depend on the dynamics of demand and supply through the energy system in which the energy storage operates and the energy storage characteristics, e.g., charging and discharging rated output, the power ramping capability, and the storage duration between charging and discharging. Due to the dynamic nature of energy system with or without energy storages, the functional unit may not be defined as an amount of energy. Instead, the functional unit should be defined as the satisfaction of energy demand over a period of time, e.g., as a time-series of the power demand with a temporal resolution of 1 h covering 1 year.
To compare energy storage with different storage characteristics, the energy system without any storage system shall be compared to systems with the energy storage alternatives. In a second step, the difference of environmental impacts reductions of the energy storage alternatives can be compared.
To find a suitable functional unit, we developed the decision tree shown in Figure 4 leading to functional unit by answering a maximum of three questions: (1) Is the subject of the study a CCU product or an energy storage? (2) If the subject of the study is CCU product, is it fuels or not? (3) Is the subject of the study chemically identical to the conventional product or not?
Defining System Boundaries for CCU Technologies
The system boundary defines which processes and life cycle stages are needed to fulfill the function as defined by the functional unit and thus, are part of the analyzed product system. In general, the system boundary should cover the entire life cycle from cradle-to-grave (European Committee for Standardisation, 2009). However, in situations where technical performance and, thus, downstream emissions are identical, a cradle-to-gate approach, in particular for comparative studies, is sufficient where the system boundaries only cover the product system from raw materials acquisition to the factory gate (Figure 3; Guinée, 2006). In fact, in some situations, it is practically infeasible to cover the entire life cycle, e.g., if a product has numerous but unknown potential applications. In the following, we derive a decision tree (Figure 5) with a set of system boundaries for CCU technologies, which are in line with the functional units derived in section Defining Functional Units for CCU Technologies.
For products and fuels with identical chemical structure and composition to their conventional counterparts, a cradle-to-gate approach is sufficient since the products cannot be differentiated and thus, downstream life cycle phases are identical and so are their environmental impacts (Figure 3).
System boundaries for products with different chemical structure and composition to their conventional counterparts, such as CO2-based materials (e.g., consumer products) shall cover the entire life cycle from cradle-to-grave. A cradle-to-gate approach is only applicable if differences in technical performance and end-of-life treatment not differ significantly. In all other cases, materials perform differently and environmental impacts from downstream processes will not be identical. Therefore, LCA studies shall cover the entire life cycle to avoid problem shifting from one life cycle phase to the other (Figure 5).
For fuels with different chemical structure and composition to their conventional counterparts, a cradle-to-grave approach shall cover the raw material acquisition, production, and transport as well as use and end-of-life, which often occur simultaneously during combustion. Omitting combustion can lead to qualitatively incorrect results, if fuels change engine efficiencies and tailpipe emissions (Deutz et al., 2018).
However, if the study aims to compare fuels with identical chemical structure and composition to their conventional counterparts, both fuels will behave identically in all potential applications and thus, a cradle-to-gate approach is justified. In other cases, omitting the combustion might still be necessary if the potential application is unknown, e.g., in early stages of development.
For the comparison of energy storage, the system boundaries shall cover the entire energy system from gate-to-gate, i.e., all environmental impacts arising from the operation of the energy system, and the entire life cycle of the energy storage, i.e., construction, operation, and decommissioning.
Upstream Environmental Impact From CO2 Capture
CO2 emitted to the environment is an elementary flow. Thus, captured CO2 is often treated intuitively as a consumed emission . However, captured CO2 is a product of human transformation. Consequently, CO2 is a technical flow, and a chemical feedstock for CO2 utilization. Thus, treating CO2 as negative emission is usually incorrect and captured CO2 has to be treated like any other feedstock (Heijungs and Frischknecht, 1998). CO2 sources shall be included in system boundaries as environmental impacts occur due to the CO2 supply. Assessments shall comprise all process steps leading to environmental impacts including CO2 source, CO2-purification and transport as shown in Figure 6.
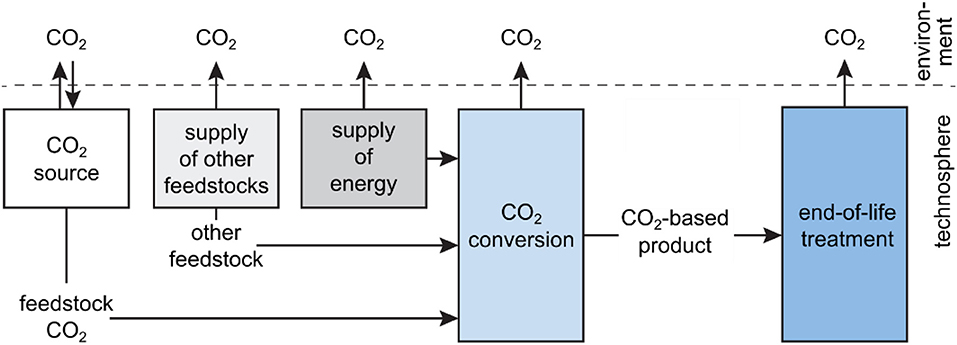
Figure 6. Schematic life cycle of CCU technologies span from the CO2 source, supply other feedstocks and energy to the end of life treatment. In all life cycle stages, environmental impacts should be considered. Adopted from von der Assen et al. (2013).
Life Cycle Inventory Modeling Framework and Solving Multi-Functionality
The life cycle inventory modeling framework defines how data is gathered and processed during the life cycle inventory stage of LCA (European Commission - Joint Research Center, 2010). The framework defines how interactions with other product systems are handled, in particular, how to solve multi-functionality problems.
Data Inventory for CCU Processes
The system boundaries for LCA studies on CCU technologies start with the acquisition of raw materials and either end at the factory gate or at the end of the products life cycle.
During an LCA study, some process data will not be available from direct measurements. A company can usually only measure data within its factory gates. Other companies or LCA databases can supply missing upstream and downstream data in the life cycle inventories. If the specific supplier of up-/downstream services is known or the production process of an input can be identified, inventory data specific to the process should be used. In other cases, this information might not be available, because products are purchased from a market, e.g., electricity traded at the stock market. In these cases, a specific technology is not available, and a market mix shall be used instead (European Commission - Joint Research Center, 2010).
The use of market mixes can be assumed until the additional demand or supply of the CCU technology triggers large-scale, structural changes2. An example for a large-scale, structural change could be the installation of additional electrical power capacities in response to an excessive electricity demand by a CCU technology, which could also affect production and consumption patterns in wide parts of the economy through changes in electricity prices. Such large-scale effects may occur for a large-scale market introduction of CCU products. Nevertheless, accessing large-scale effects is typically beyond the scope of conventional LCA studies. The development of methods for this purpose by integration of complex market models is topic of current research (Yang and Heijungs, 2018). In this guideline, we focus on the scope of conventional LCA studies.
Therefore, first, process-specific inventory data shall be used, if this information is available. Only otherwise, averaged market mixes shall be used for the regarding input.
Solving Multi-Functionality
Most CCU systems are multi-functional, because CO2 sources often provide a main product and CO2 (Figure 8; von der Assen et al., 2014). For example, ammonia is produced by reacting hydrogen with nitrogen. Hydrogen can be co-produced with CO2 in the steam-methane-reforming process. As poison to the catalyst for ammonia production, CO2 has to be separated prior the formation of ammonia and subsequently, a pure CO2 stream is released. If CO2 is now captured from ammonia synthesis, the main-product ammonia and the co-product CO2 are produced simultaneously. If the environmental impacts for the produced CO2 stream are required, the total emissions of the system need to be split between the main and the co-product.
This problem is called multi-functionality. Other co-products or functions may occur throughout the life cycle of CCU products. In general, the problem of multi-functionality is no CCU-specific problem. The problem can be addressed using established LCA methodologies. However, a number of methodological choices have to be made. Therefore, we first demonstrate how the methods can be applied to a CO2 source since the multi-functionality problem at the CO2 source is at the core of most CCU processes. Subsequently, we present a hierarchy of methods to solve multi-functionality which is valid according to ISO 14044 and other guidelines and standards (World Resources Institute and World Business Council for Sustainable Development; European Commission - Joint Research Center, 2010; BSI, 2011; AFNOR, 2016; European Committee for Standardisation, 2018).
In the following, the alternative methods to solve multi-functionality are described and applied to account for the supply of CO2. Sub-division solves the problem of multi-functionality by separating an aggregated (black box) unit process with multiple functions into smaller unit processes and gathering input and output data of these smaller unit processes, e.g., a factory with multiple products that are produced in independent processes can be sub-divided into individual production lines (European Committee for Standardisation, 2009).
Cases where sub-division is applicable are not a problem of multi-functionality in a strict sense, but a problem of missing data. If this missing data can be gathered, multi-functionality can be fully resolved and thus, sub-division shall always be applied first. Sub-division shall even be applied if multi-functional unit processes remain, as this leads to smaller and simpler product systems.
Application to CO2-source: Sub-division is not applicable to CO2-source since CO2 is always produced jointly with the main product.
System expansion expands the functional unit to include other functions of the product systems than originally stated in goal and scope definition. If this expanded function is still meaningful, the multi-functionality problem is resolved (European Committee for Standardisation, 2009).
CCU processes are often multi-functional, e.g., when the CO2 source co-produces another product such as electricity. As discussed above, CCU processes are often compared to conventional processes. To compare both product systems, each product system needs to fulfill the same functional unit and therefore, the system boundaries and the functional unit are changed for the product systems. For the comparison of the CCU process with two products (product of CO2 source and product of CO2-process) to a conventional system (Figure 7A), the main product of the CO2 source is added to the functional unit and the conventional system is expanded with the CO2 source without capture (Figure 7).
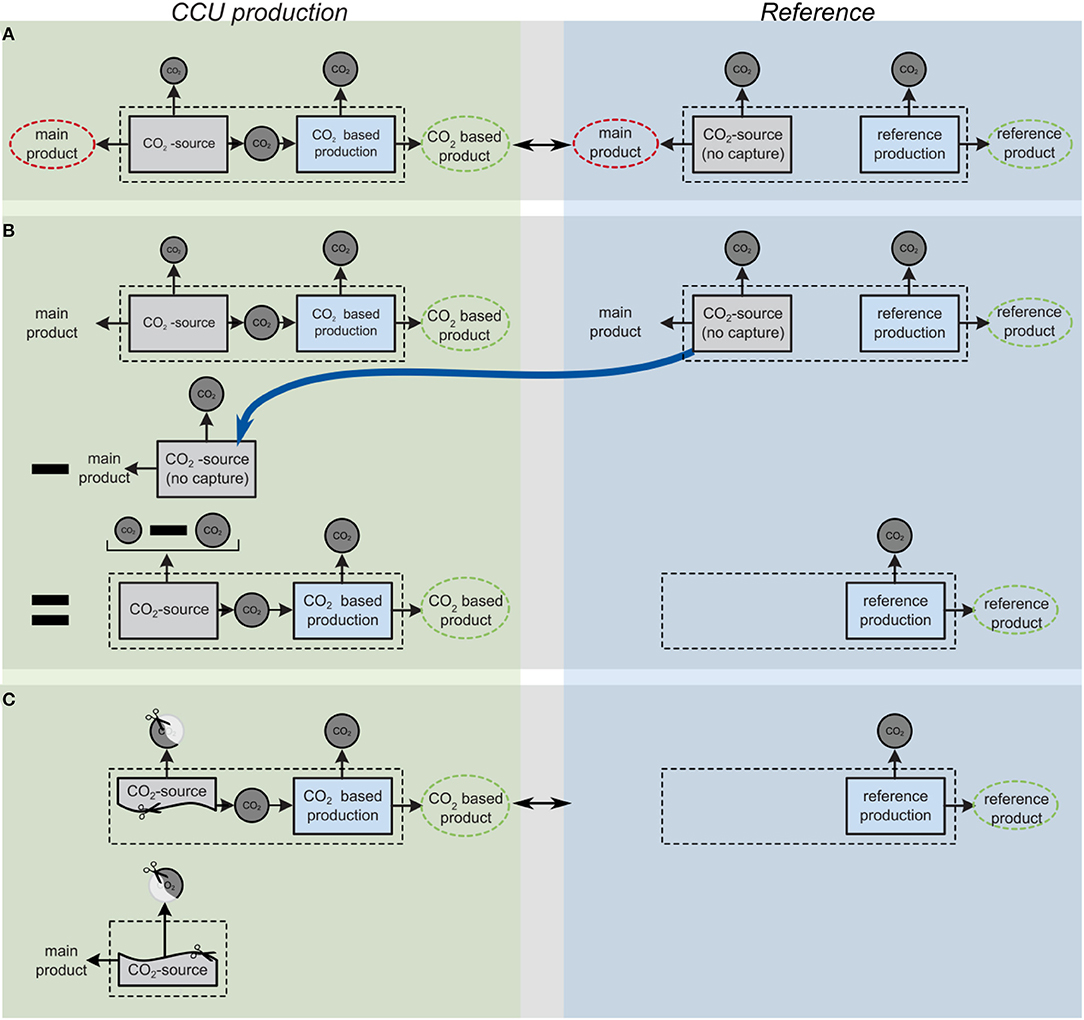
Figure 7. (A) Comparison of a CCU production and a reference production: CCU system produces a main product besides CO2-based product, i.e., the CCU system has additional function (dashed red line) besides functional unit (dashed green line). Thus, the conventional and CCU system are not comparable due to different functions. System expansion enables the comparison of the CCU production and the reference production by including the main product of CO2-source in functional unit. For a sound comparison the reference production system is expanded with the conventional production of the main product without carbon capture. (B) Substitution: The production of the main product without carbon capture is avoided and thus, the CCU system is credited for the otherwise emitted CO2, but has to carry the burdens of purification, compression and transport. (C) Allocation sub-divides the CO2-sources into two processes and distributes the environmental burdens of the CO2 source between the main product and the feedstock CO2 production using underlying physical relationship or other relationship. The CCU production system becomes a mono-functional production system and can be compared to the reference production since functional units are identical.
Note that a process used for system expansion (not in case of CO2-sources) can be multi-functional as well and subsequent system expansion may be needed. In theory, one could end up modeling the entire global technosphere. However, this endless chain of system expansion is usually interrupted by the definition of cut-off criteria for small contributions to the LCA results.
Substitution does not include additional functions in the functional unit. Instead, a credit is given for the production of the co-product. The credit represents the environmental burdens avoided by the substitution of the conventional production system which would have been used otherwise. The functional unit remains as stated in the goal and scope definition, but the system boundary is altered for the product system where substitution is applied. In comparative assessments, the system boundary and functional unit of the conventional product system(s) remains unchanged (European Commission - Joint Research Center, 2010).
Similar to the approach presented in section Data Inventory for CCU Processes, first, a specific process to be substituted shall be identified and used. In all other cases, a market-averaged process mix shall be assumed (European Commission - Joint Research Center, 2010).
For CO2 sources, the substituted process is usually the same source but without capture (Figure 7B). This assumption is meaningful as long as not all CO2 from this source is already fully utilized.
Both approaches, system expansion and system expansion via substitution, are mathematically equivalent in comparative LCA; however, results, meaning and interpretation of results are not, because system boundaries and functional unit are altered. System expansion via substitution can lead to negative environmental impacts (e.g., negative CO2 emissions), because by-products are credited. These negative environmental impacts can be misunderstood in a way that producing more of the product could offer infinite benefits to the environment. However, these negative environmental impacts are limited to the market capacity of the by-products and thus, do not offer infinite benefits. Furthermore, these negative environmental impacts do not indicate that greenhouse gas emissions are taken up by the production system from the atmosphere nor that natural resources are generated (Tanzer and Ramírez, 2019). The negative environmental impacts simply indicate that the production system has lower environmental impacts than the conventional production of all products and by-product trough the conventional production. However, as a conceptual advantage, substitution conserves the causal interaction between processes by accounting for impacts in other life cycles.
Allocation partitions the in- and outputs of the multi-functional process among the products or functions reflecting an underlying physical causal, economic, or other non-causal physical relationship (Figure 7C).
According to ISO 14044, an underlying physical causal relationship shall be applied first, by quantifying how input and outputs physically relate to a function of system. For example, the chlorination of benzene delivers mono-chlorobenzene, ortho-, and para-dichlorobenzene and hydrochloric acid. The amount of chlorine consumed by the process is directly physically related to the amount of the chlorine incorporated in the products. Therefore, the amount of chlorine in each product is the physical criterion to distribute the chlorine flow between the products of benzene chlorination. Another way to establish a physical causality is to quantitatively change the functions and observe how the inputs and outputs are affected. The distribution of the inputs and outputs should than reflect this quantitative change of inputs and outputs3. Note that more than one relationship can be applicable within one process.
In case of CO2 sources, a physical causality can be found by quantitatively changing the amount of main product and the product CO2 produced and observing how the inputs and outputs are affected. Setting the amount of main product to zero, leads to a process without inputs, outputs, and product CO2. Therefore, the amount of main product affects the inputs and outputs of the process. Varying the amount of product CO2 changes the amount of CO2 emitted, since CO2 is no longer emitted and the inputs and outputs related to the capture process. In consequence, 1 kg of CO2 provided by the CO2-source leads to an emission reduction of 1 kgCO2e and an increase of emissions related to the capture process. The result is identical to the substitution approach.
If a physical causal relationship cannot be applied, other underlying relationships shall be used. For this purpose, the multi-functional process is sub-divided into mono-functional processes and the environmental burdens of the multi-functional process are distributed among the mono-functional processes according to attributes of the product or functions. The most commonly applied attribute is economic value of products or functions. Since the multi-functional process is artificially sub-divided, the physical causality between processes is lost, i.e., the independent production of former jointly produced products. In addition, the selection of the attribute is to some extent arbitrary.
The selection of a suitable product attribute to distribute the emissions of the CO2-source among the main product and the CO2-source can be difficult. Mass can be applied to all processes except power plants, since electricity has no mass and thus, all emissions would be distributed to CO2. Energy is not a suitable attribute since CO2 does not contain any energy, or more precisely: its lower heating value is zero. The economic value of CO2 is uncertain, since the capture process is related to costs, the price of CO2 might be positive and thus, economic allocation would attribute CO2 would with positive emissions. However, it can be argued that CO2 has a negative economic value since it is a waste stream, which needs a waste treatment. In this case, the CO2 source has only one function, i.e., producing the main product and has a technical waste flow, i.e., the concentrated CO2 stream. The CO2-utilizing step would then be multi-functional in the sense that a CCU product is produced and the CO2 waste stream is treated. As waste stream per se cannot carry any environmental burdens, the environmental impacts of the CCU utilizing step would be allocated between the CCU product and the waste treatment (European Commission - Joint Research Center, 2010).
As each applied criterion would significantly alter the environmental impact attributed to CO2 and an objective selection of one allocation criterion is not possible, a sensitivity analysis is always needed.
Hierarchy of Methods for Solving Cases of Multi-Functionality
Existing standards (BSI, 2011; AFNOR, 2016; European Committee for Standardisation, 2017, 2018) and guidelines (European Commission - Joint Research Center, 2010, 2012; World Resources Institute and World Business Council for Sustainable Development, 2011) rank methods for solving multi-functionality in a hierarchy which should be consistent with stated goal definition. In the following, we present the hierarchy of methods for solving multifunctionality complying with the hierarchy of ISO standards and guidelines.
First, check if multi-functionality can be solved by gathering individual process data and apply sub-division.
If subdivision cannot solve the multi-functionality problem, apply system expansion. Note that results obtained via system expansion are joint impacts due to the production of more than one product and thus, are not specific to a single product of the CCU technology. This might be in conflict with the initial research question and a modification of the question might be needed.
If product-specific assessments are needed to answer the initial research question the following hierarchy of allocation method shall be applied. Please note that results obtained via system expansion shall always be computed to assess the overall effect of introducing the CCU technology.
For product-specific assessments, first, substitution shall be applied. If substitution is not possible, e.g., because there is no process available to be substituted, apply allocation: First, using an underlying physical relationship and then an underlying other relationship, e.g., economic value.
Special Requirements for Comparative Studies
Any study intended for external communication shall be reviewed. For comparative studies or studies to be used in comparative assertions disclosed to public, a critical review shall be conducted by an independent and qualified review panel. More information about the review process can be found in the ILCD handbook, the ISO standard and the PEF guideline (European Committee for Standardisation, 2009, 2018; European Commission - Joint Research Center, 2010, 2012; International Organization for Standardization, 2014).
Note that external review also allows to leave out confidential information in the public report and thus, can protect intellectual property.
Life Cycle Inventory (LCI)
In the life cycle inventory phase, the actual data is gathered, and the product system is modeled according to goal and scope definition.
Estimation Methods to Bridge Data Gaps
During LCA studies, practitioners are often confronted with limited data availability. To bridge data gaps, estimation methods have been developed. In the following, commonly applied estimation methods are presented, and further readings are provided. These methods may be used to bridge data gaps, but the generated data should be replaced by measured values as soon as possible.
Second-Law Analysis
With thermodynamic analysis, a second-law analysis can be conducted based on stoichiometric reaction schemes, mass-, energy-, exergy-, and entropy balances. By assuming second-law efficiency of 100%, an absolute best-case scenario is obtained. If this best-case scenario does not offer environmental benefits, the considered process will never offer any environmental benefits. In particular for low TRL technologies, the second-law analysis is an useful tool to sort out technologies. Therefore, the second-law analysis shall be used to establish a best-case scenario.
Gate-to-Gate Inventory Estimation
In cases, where specific information of chemical processes is missing, e.g., for feedstocks, Ecoinvent uses a yield of 95% based on a stoichiometric mass balance and a product averaged energy demand and other auxiliaries can be assumed as a rough estimation (Weidema et al., 2013; ecoinvent, 2017; Gendorf Chemiepark, 2017).
Jiménez-Gonzáles et al. and Kim et al. provide a design-based method to estimate gate-to-gate inventory information when direct data is not available (Jiménez-González et al., 2000; Kim and Overcash, 2003). The provided method defines transparent rules for data collection and provides rules of thumbs, e.g., for the estimation of mass balance, energy requirements, and energy recovery rates. Based on this method, Kim et al. show for 86 chemicals that the gate-to-gate process energy ranges for half of the organic chemicals from 0 to 4 MJ per kg and for half of the inorganic chemicals from −1 to 3 MJ per kg.
A method to estimate gate-to-gate process energy consumption when no process engineering is available, is provided by Bumann et al. (2010) which correlates the process energy demand with the energy index provided by Sugiyama et al. (2008). The proposed method is based on a simplified process model consisting of a reactor and separation unit and information of the chemical reaction, e.g., reactants, products, co-products, and by-products, reaction conditions and thermodynamic data. From this data, an energy index is computed and used for the estimation of gate-to-gate energy consumption. The average deviation of this method is around 30%.
Artificial Neural Networks
Environmental impacts of processes have been estimated from molecular descriptors of the desired product using neural networks (Wernet et al., 2008, 2009). The resulting software tool Finechem can be helpful if no process information is available. The neural network was trained with industrial data and thus, the method might be limited to predict molecules comparable to those in the training set. In addition, the molecular descriptors limit the range of application, as isomeric compounds and polymers cannot be differentiated. Furthermore, as this method uses solely the molecular descriptors of the product as an input, alternative production pathways cannot be assessed by this method. This is in particular a shortcoming for CCU technologies which aim to substitute identical products, fuels, or materials.
Selection of Reference Processes
The selection of a reference process has significant impact on the reduction potential of the assessed CCU technology. Therefore, the reference process has to be carefully selected. In general, reference processes shall be those processes that the CCU production system competes with in the market, i.e., the marginal process. However, the identification of the marginal process may introduce complex market interactions, in particular if the process has more than one function. Therefore, the reference process shall be modeled as the average market mix if further information is missing and if no large-scale, structural changes occur (section Data Inventory for CCU Processes).
However, CCU technologies—in particular in stages of early development—do not compete with current technologies, since their market launch lies in the future. Instead, these CCU processes compete with the technologies established in the future. Thus, comparing CCU technologies in stages of early development to currently used processes does not reflect reality. Therefore, the time dimension is crucial for assessing ecological benefits of CCU. For this purpose, future development techniques, e.g., learning curves, may be applied to both the CCU technology and the reference process, as both processes underlie development (Cucurachi et al., 2018). Methods to apply learning curves are described by Gavankar et al. (2015b) and Cespi et al. (2015). Note that forecasting techniques shall not exceed physical limitations, e.g., the second law of thermodynamics. In addition, changes in the background system shall be accounted for, e.g., the changes in the energy supply due to higher shares from renewables.
However, predicting future technologies is potentially beyond the scope and experience of many LCA practitioner and thus, if no reliable predictions on future developments are available, the current best available technology should be used as the reference technology.
Life Cycle Impact Assessment
Key driver for CCU is to lower GHG emissions and our dependence on fossil resources. Not surprisingly, global warming and fossil resource depletion (or fossil-based cumulative energy demand) are usually selected as impact categories in LCA studies on CCU (Artz et al., 2018). The introduction of CCU technologies may further affect a variety of environmental impacts and the holistic LCA approach aims to avoid problem shifting from one impact category to another. Therefore, impact categories shall not be omitted from LCA studies to avoid misleading decision-making if impact categories are:
- Relevant, i.e., accounted elementary flows contribute in these categories and
- Assessable, i.e., impact assessment methods exist and these methods are reliable.
However, the selection of impact categories and methods is not straightforward: Numerous impact categories exist and sometimes even multiple methods for one impact category exist. Furthermore, the uncertainty of impact assessment models varies as more or less complex cause-effect chains are involved and methods are more or less advanced. In consequence, different impact assessment models are used in practice leading to differences in LCA results.
Impact assessment should use the CML (Institute of Environmental Sciences, University of Leiden) impact assessment methodology in its most recent version as the “International Environmental Product Declaration (EPD) System” uses CML as a default for product category rules. To the best of the authors' knowledge (July 2018), the most recent version of CML is 2016. Additionally, a second set of methodology should be applied if this methodology is geographically more appropriate than CML4,5. In this way, comparability and geographical representativeness are guaranteed at the same time.
For Europe, the Joint Research Center provides a selection of impact categories and methods which were defined in a stakeholder's dialogue involving LCIA model developers and LCA practitioners and thus, the JRC recommendation should be followed for Europe (European Commission - Joint Research Center, 2011a). For the United States, the EPA developed TRACI 2.1 as impact assessment methodology and thus, TRACI in its most recent version should be used for studies in the U.S. (Bare, 2002).
Note that life cycle impact assessment should be limited to midpoint indicators, because the level of uncertainty increases with endpoint indicators or single point indicators. Also note that a detailed knowledge of impact assessment method is necessary to interpret and report results properly, e.g., human toxicity assessments have high uncertainty and thus, results differing by 2–3 orders of magnitude might still be interpreted correctly as “identically toxic” (Hauschild and Huijbregts, 2015).
Temporary Storage of CO2
CCU products offer temporary carbon storage. Due to temporary carbon storage, CO2 emissions can be delayed and thus, do not contribute to climate change during the time of storage. Therefore, temporary storage is no independent or additional benefit from the impact on climate change.
The relevance of temporary storage depends on the class of CO2-based product or fuel considered:
For CO2-based products and fuels with identical chemical structure and composition to their conventional counterparts, carbon storage does not offer any additional benefits since the product life is identical after leaving the factory gate for both products and the amount of carbon chemically bonded is identical (Kätelhön et al., 2019). Therefore, the time between production and end-of-life treatment and the amount of CO2 released during end-of-life treatment is identical. Thus, the emission time profile is identical after factory gate (blue and green line in Figure 8) and there is no additional effect storing CO2.
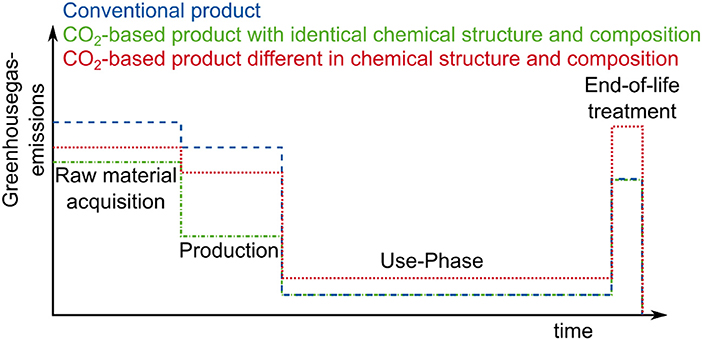
Figure 8. Emissions time profiles for different products. CO2-based products with identical chemical structure and composition to their conventional counterparts have identical emissions timing profiles after production. CO2-based products different in chemical structure and composition can have different emissions during use-phase and end-of-life treatment and different life spans and thus, the emissions timing profile can be different.
For CO2-based products different in chemical structure and composition to their conventional counterparts, emission time profiles are not identical (red line in Figure 8) and thus, temporary storage may offer climate benefits (Figure 9). However, note that temporary storage offers a benefit only once. Once all counterparts have been substituted, the composition remains constant and thus, emission time profiles are identical again.
For CO2-based fuels different in chemical structure and composition to their conventional counterparts, temporary storage is usually not significant, since the storage duration is short compared to climate change dynamics.
To decide whether or not temporary storage may offer any climate benefit, we developed the decision tree shown in Figure 9 by answering a maximum of three questions: (1) Is the subject of the study a CCU product or an energy storage? (2) If the subject of the study is CCU product, is it chemically identical to the conventional product or not? (3) If the chemical structure differs, is the subject of the study a fuel or not?
The effect of temporary CO2 storage is known from bio-based products and methods to account for temporary storage exist (Levasseur et al., 2010, 2012; Brandao et al., 2013). However, classic LCA does not account for temporary storage or emission timings, “as LCA per se is not discounting emissions over time” (ILCD handbook, p. 226), since LCA models are usually static and do not account for dynamic effects such as discounting emissions over time (Brander, 2016).
To follow the established LCA principles, delayed emission shall not be discounted over time. Instead, emission time profiles, the amount and duration of carbon stored may be reported as a separate item.
Note that for permanent storage6, a discounting method is not needed because end-of-life emission never occur and thus, are zero. If end-of-life emissions are zero, the effect of storage is thus already considered.
Life Cycle Interpretation
During life cycle interpretation, the feedback loop of the iterative steps of LCA studies is closed, e.g., through evaluating the gathered life cycle inventory in the light of the goal definition. Furthermore, the results are evaluated to derive robust conclusions and potential recommendations at the end of a LCA study.
Uncertainty and Sensitivity Analysis
In the following, methods to quantify the impact of uncertainties are described and two levels of recommendation are provided. This section is based on Igos et al. (2018). First, a basic level is described using sensitivity analysis and scenario analysis and second, an intermediate level using uncertainty analysis. The basic level shall be applied and the intermediate level should be applied if possible.
Please note that uncertainty assessment in general is already covered sufficiently by standards and guidelines. However, the following section describes how methods can be applied to CCU technologies.
Basic Level
In the basic approach, input variables shall be identified that have uncertainties with high impacts on the uncertainty of the model output. For this purpose, a sensitivity analysis shall be carried out. Sensitivity analysis is a systematic procedure to estimate the effects that alternative choices for methods and data have on the outcome of a study (European Committee for Standardisation, 2018). The most basic approach to carry out a sensitivity analysis is the one-at-a-time approach. For the one-at-a-time approach, input variables shall be varied separately one after the other to quantify the sensitivity of the model results toward the considered input variable. For this purpose, the input variables shall be varied within realistic ranges. The results of the sensitivity analysis may be sorted to identify key variables with the highest influence on the overall output uncertainty. If the variation of the input variables reveals weak points of the study that are not in line with the LCA study's goal and scope, the goal and scope definition shall either be refined or data quality and modeling approach shall be reviewed until significance of results according to goal definition is achieved.
Once the key variables are identified, either a scenario analysis, i.e., the evaluation of alternative choices, or the calculation of threshold values for key variables shall be carried out.
For a scenario analysis, a number of scenarios exploring key variables shall be defined. These scenarios shall be analyzed in relation to the model results of the baseline scenario. Typically, best- and worst-case scenarios should be defined to quantify the range of the model results.
CCU technologies often make use of energy or high energetic reactants, e.g., hydrogen to activate CO2. The production of those high energetic reactants or the supply of energy can lead to high environmental impacts. In consequence, assumptions on environmental impacts of these inputs have been identified as the major source of varying results in LCA studies on CCU technologies. Thus, the environmental impacts related to the high energetic reactants are often the key variables in studies on CCU technologies (Artz et al., 2018). Furthermore, CCU technologies are emerging technologies and thus, the derived scenarios shall consider the transition of the background system. For this purpose, practitioners shall define a scenario representing the status-quo, a fully decarbonized future and a transition scenario. An example for electricity generation is presented in Table 1. The status-quo is taken from the Energy Technology Perspectives report published by the International Energy Agency (2017). In fully decarbonized industry, the greenhouse gas emissions of the energy supply will be fairly close to zero, while in a transition scenario the emissions will lie somewhere in between the status-quo and a fully decarbonized industry (e.g., 50% of the current emissions). These scenarios are derived in a very simply way and the scenarios will perform badly at forecasting. However, valuable insights from a scenario analysis like this can be gained, e.g., the dependence on clean energy supply can be shown. Since the generation of scenarios can be time- and resource-demanding, we derived scenarios for the supply of electricity, hydrogen, CO2, heat and natural gas (as methane) for the European context is provided in the annex of this document (see Supplementary Material). These scenarios should be used per default in the European context to allow harmonization of LCA studies.
However, note that scenario analysis can suffer from ambiguity because the definition of scenarios relies on the LCA practitioner and can hardly become an automated part of LCA calculations (Jung et al., 2014).
As an alternative to scenario analysis, threshold values for key variables can be calculated. A threshold value is the smallest (or highest) value of an input variable that is sufficient to achieve environmental benefits compared to the benchmark process. For example, water electrolysis consumes 50 kWh electricity per kilogram hydrogen. To emit less greenhouse gas emissions than steam reforming of methane (10.7 kg CO2-eq per kilogram hydrogen, GaBi Software-System v8.5.0.,79 and Database for Life Cycle Engineering SP 35, 1992–2018), the greenhouse gas emissions of electricity supply for the water electrolysis would need to be 0.214 kgCO2-eq per kWh electricity or lower. In this case, the threshold value of electricity supply for hydrogen from water electrolysis compared to the benchmark process steam methane reforming of methane would be 0.214 kg CO2-eq per kWh electricity. For a sound interpretation, the calculated threshold values should lie within physical and thermodynamic limits.
Intermediate Approach
Based on the basic approach, the LCA practitioner should carry out an intermediate approach to quantify the uncertainty of the model output using uncertainty analysis. According to the ISO 14044, uncertainty analysis is a “systematic procedure to quantify the uncertainty introduced in the results of a life cycle inventory analysis due to the cumulative effects of model imprecision, input uncertainty and data variability” (European Committee for Standardisation, 2018). Therefore, uncertainty analysis is a measurement of the reliability of the model output toward the underlying decision process. Uncertainty analysis is usually carried out using stochastic methods, e.g., Monte Carlo simulation (Sonnemann et al., 2003; Schenker et al., 2009; Williams et al., 2009; Sills et al., 2013), or perturbation theory, e.g., analytical uncertainty propagation (Huijbregts et al., 2001; Lloyd and Ries, 2007; Heijungs, 2010; Groen et al., 2014; Jung et al., 2014; Pfingsten et al., 2017).
In the intermediate approach, the Monte Carlo simulation is recommended since it is the most common method to carry out an uncertainty analysis and it is integrated in current LCA software, e.g., SimaPro, OpenLCA, and GaBi. In a Monte Carlo method, all input variables are varied randomly within their defined ranges for a fixed number of model simulations. In consequence, the range of the model results is a value for the probability distribution and thus, a value for the overall model uncertainty. The Monte Carlo method requires a high number of simulations in order to obtain representative results and therefore, high computational power or high calculation time. Usually, 10,000 Monte Carlo sets are generated, but Wei et al. (2016) showed that 1 million might be necessary to achieve sufficient accuracy of results. In general, convergence cannot be guaranteed (Igos et al., 2018). Therefore, the number of Monte Carlo sets should be as high as possible but at least 10,000 (Igos et al., 2018).
In comparative studies, Monte Carlo analysis shall not be carried out independently for each alternative, since the comparison of probability distribution can lead to wrong interpretations, i.e., a large overlap of two probability distributions might be misinterpreted as an indistinctive decision. Large overlaps can be a result of identical sensitivity of both system toward one parameter (Henriksson et al., 2015). For example, if two hydrogen electrolysis with different efficiencies A and B are compared. The environmental impacts of B and A are both highly sensitive to the environmental impacts of the electricity supply, but are impacted in the same way. However, if done independently, overlaps can occur because a Monte Carlo set of B with low impact electricity supply is compared to a Monte Carlo set of A with a high impact electricity supply (Figure 10A). Here, the interpretation that both system perform equally good is wrong and can be avoided by a joint Monte Carlo simulation of the difference of both alternatives. A joint Monte Carlo analysis can show that the environmental impacts of alternative B are always higher than A in each Monte Carlo set and thus, that A is clearly advantageous (Figure 10B).
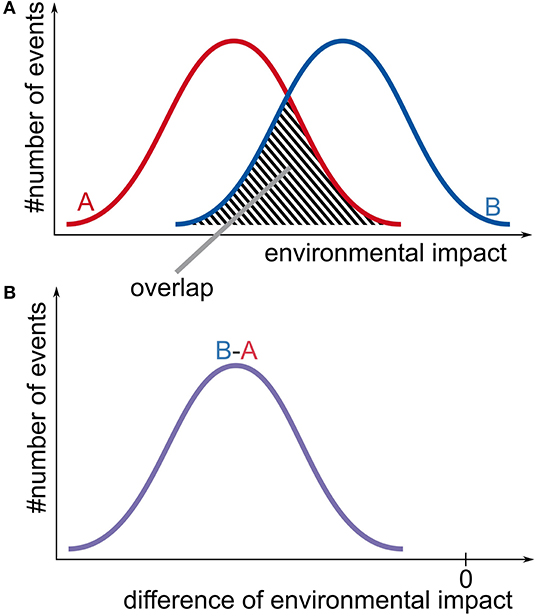
Figure 10. (A) Results of an independent Monte Carlo analysis for alternatives A and B. (B) Results of Monte Carlo analysis for the difference of technologies.
Therefore, a comparison of different technologies shall be carried out in a joint Monte Carlo simulation. Furthermore, a comparison between different technologies in a joint Monte Carlo simulation step shall always be related to the same background system to ensure consistent results. For instance, the conventional synthesis of methanol requires high amounts of carbon monoxide and hydrogen whereas the CO2-based production pathways require high amounts of carbon dioxide and hydrogen. To ensure a fair comparison between both technologies and thus, a consistent result of the uncertainty analysis, the background production system of hydrogen has to be the same for each individual Monte Carlo simulation step. For this reason, using aggregated processes in Monte Carlo analysis can be misleading and thus, should be avoided.
Uncertainty and sensitivity analysis are important for comparative studies to identify whether calculated differences of environmental impacts are significant or not. Note that significant difference may not be revealed by sensitivity analysis. This does not mean that no difference exists, but that the study could not prove any. Furthermore, note that ignorance, as an additional source of uncertainty, can neither be assessed by uncertainty nor by sensitivity analysis “but may be revealed by qualified peer review” (European Commission - Joint Research Center, 2010).
Communication of Uncertainty Assessment Results
The communication of uncertainty assessment results is important to avoid misleading interpretations and to ensure the credibility of the assessment (Gavankar et al., 2015a). Therefore, the communication of the results of the basic approach shall include parameters with high sensitivity and their effects to the overall model results. The results of the scenario analysis and calculated threshold values shall be reported separately to the results of the sensitivity analysis. The intermediate uncertainty assessment approach should furthermore include the results of the uncertainty analysis. The results of the uncertainty analysis should be interpreted with regards to their effect on the reliability of the LCA results.
Carbon-Neutral Products and Negative Emissions
CCU technologies consume CO2 to produce value-added products. Thus, intuitively CCU technologies may be thought of as technologies with potentially zero emissions or net-negative emissions.
CO2 is usually considered to be captured from fossil or biogenic point sources or directly from the atmosphere via direct air capture. Fossil point sources release carbon previously stored in underground departments, while biogenic point sources releases carbon previously consumed from the atmosphere. CCU technologies can theoretically be carbon neutral over the entire life cycle if CO2 is captured from the atmosphere (via biogenic point sources or direct air capture) and the CO2 is released at the end-of-life (Figure 11a) or if CO2 is captured from fossil point sources and CO2 is sequestered or permanently stored in the product (Figure 11b) and if all other GHG emissions are zero over the life cycle. CCU technologies have potentially negative emissions (Figure 11c) if CO2 is captured from the atmosphere (via biogenic point sources or direct air capture) and if CO2 is sequestered or permanently stored in the product and if overall life cycle GHG emissions are lower than the amount of CO2 fixated. If the amount of atmospheric CO2 capture and fixation is equal to other fossil emissions over the life cycle, the process is carbon neutral.
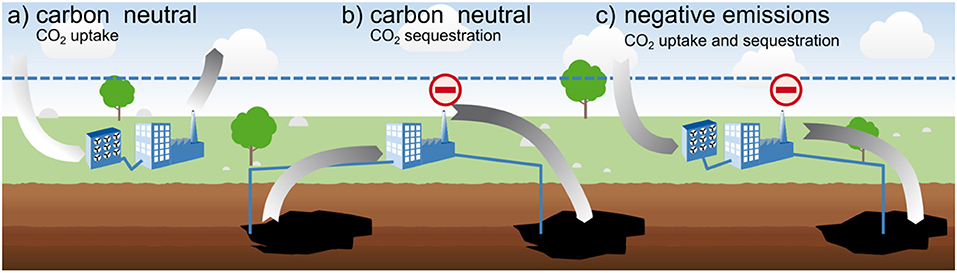
Figure 11. (a) Carbon neutral CO2-uptake: CO2 is taken from the atmosphere and is re-emitted after the product life cycle. (b) Carbon-neutral CO2 sequestration: Fossil carbon is taken from underground reservoirs and CO2 is sequestered after product life cycle. (a,b) are only carbon neutral if no emissions occur during the product life cycle. (c) Negative emissions: CO2 is taken from the atmosphere and sequestered after the product life cycle. (c) will only have negative emissions if emissions over the entire lifecycle are <1 kg CO2-eq. per kg CO2 up taken.
In all other cases, CCU technologies have positive CO2 emissions over the life cycle. Still, emissions can be lower than for competing conventional processes (Figure 12). In this case, the CCU process also contributes to climate change mitigation through substitution, and hence is carbon reducing. Even though such processes lower CO2 emissions compared to the status quo, they are not carbon negative. In particular, this also holds for carbon-reducing processes with negative CO2 emissions obtained from substitution. Through applying substitution (section Solving Multi-Functionality) or cradle-to-gate analysis, negative LCA results can be computed. However, the negative LCA results does only reflect a comparison. In particular, negative LCA results do not necessarily imply that the CCU product is carbon neutral or even has negative emissions over its life cycle. Therefore, negative CO2 emissions obtained from substitution shall be clearly stated as environmental benefit compared to the benchmark technology and not as negative CO2 emissions over the life cycle. In addition, avoided CO2 emissions and other environmental impact from substitution shall be reported separately (Tanzer and Ramírez, 2019).
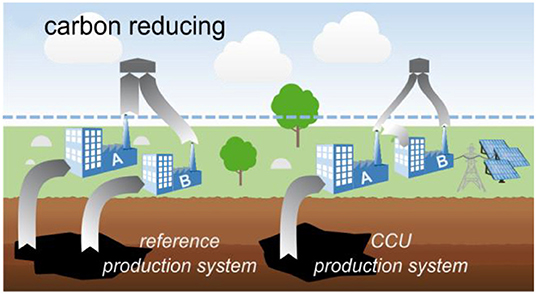
Figure 12. Carbon reducing: CCU technologies can offer lower CO2 emissions than the status quo and thus, may be considered as carbon-reducing technologies.
Reporting
The final step of an LCA study is a report. According to ISO 14044, “The results and conclusions of the LCA shall be completely and accurately reported without bias to the intended audience.” Assumptions made on data and methods should be transparently reported and enable the reader to understand limitations of the results. Presented results should enable readers to understand the complexity and trade-offs of the LCA study. Results and interpretation presented should be in line with the goals of the study. The reports may be reduced if sensitive or confidential information and data may not be published.
The report shall include an executive summary and a technical summary table to provide easy access to the data used in the assessment. The main report shall report all assumptions, data for calculation, methods, results and limitations as transparently and detailed as possible. This is also important to guarantee reproducibility and full traceability by the reader. The assessment results shall be clearly reported to the audience in order to avoid ambiguity and misinterpretation.
The supporting information provides a checklist for an executive summary, a technical summary table and main report. This checklist is derived from the ISO 14044 and the ILCD handbook and additionally includes CCU-specific items.
Discussion
Building upon established LCA standards and guidelines, our work is tailored to the needs of LCA practitioners assessing CCU technologies by identifying and resolving CCU-specific pitfalls. The resulting guidelines substantially reduce the space for methodical choices in LCA with the goal to foster comparable and transparent studies on the environmental impacts of CCU.
Even though our guideline already defines a broad framework for the environmental comparison of CCU technologies, some aspects specific to CCU technologies are still unaddressed. First, the guideline is not an ISO standard or document, even though reviewed by around 50 international experts from industry, academia and policy in two workshops. Introducing an ISO standard or document report could strongly enhance the adoption of the guidelines. However, the process of standardization is driven by industry and thus, can only be initiated by us.
Second, the LCA guideline was developed in parallel to a guideline for techno-economic assessment (TEA). Both TEA and LCA are sub-divided into identical phases and both build upon mass and energy balances and thus, share the same data foundation. However, currently, the goal and scope of both assessments are different: while LCA studies aim to quantify the overall environmental impacts, TEA studies usually aim to compare production costs, prices or analyze the market situation. Therefore, both assessments are currently not aligned and thus, TEA and LCA studies can be done at the same data basis, but goal and scope may not necessarily be aligned. In consequence, results of TEA and LCA have to be interpreted separately and combined indicators such as abatement costs have to be interpreted with caution. Therefore, a stronger alignment between both guidelines would be beneficial, in particular, for the identification of trade-offs between environmental and economic indicators.
Third, in many cases, CCU technologies promise to reduce environmental impacts and achieve costs competitiveness. The promises can, however, not be proven with high certainty for technologies with early maturity, because data availability is low at these stages. For this reason, further methods are needed to assess the potential of technologies with low maturity.
Fourth, the last unsolved issue is that LCA reports are usually of high complexity and long including trade-offs and if-then conclusions. Decision-makers, such as policy makers, often lack time and technological expertise to derive policies from these highly complex LCA reports. Therefore, guidance is missing for policy makers on how to commission and understand LCA results. LCA practitioners should develop methods on how to write effective LCA reports for a target audience.
Conclusion
This publication summarizes our guideline for standardized life-cycle assessment of technologies for carbon capture and utilization. This guideline aims to reduce the ambiguity in methodological choices and enhances the transparency of LCA studies. These goals have been achieved by substantially restricting the methodological choices currently left open by LCA standards. To reduce methodological choices, we identified typical goal definitions for LCA studies on CCU and provided suitable scope definitions in line with these goal definitions. We further predefined functional units and system boundaries with respect to a CCU classification and provided a hierarchy for methods to solve multi-functionality with a focus on system-wide assessments. For the life cycle inventory phase, we defined rules to select data in line with major guidelines. Furthermore, we recommended impact assessment methods with respect to regional differences, in order to reduce the variation of results. As CCU technologies are often set in a utopian future, we define scenarios for the uncertainty assessment for the most common inputs in order to show the dependency of clean feedstock. The presented guidelines should hopefully help to strengthen the development of environmentally beneficial CCU technologies.
Data Availability Statement
The datasets for harmonized scenario analysis is provided in the electronic supporting information.
Author Contributions
LM worked on the conception of this work, literature collection, analysis, and drafted the article. MB contributed by drafting section Uncertainty and Sensitivity analysis. AK, AS, AZ, and AB contributed through to the conception of the work and revising it critically for important intellectual content. All authors contributed by giving final approval of the version to be published.
Funding
Funding for this work from the European Institute of Technology (EIT) Climate-KIC grant number 180409 and by The Global CO2 Initiative is thankfully acknowledged.
Conflict of Interest
The authors declare that the research was conducted in the absence of any commercial or financial relationships that could be construed as a potential conflict of interest.
Acknowledgments
The authors would like to thank Benedikt Winter (RWTH Aachen) for his support in the scenario modeling as well as Issam Dairanieh (formerly The Global CO2 Initiative), Sira Saccani (EIT Climate-KIC) and Ted Grozier (formerly EIT Climate-KIC) as well as all participants of the co-collaboration workshops and reviewers for their fruitful discussions and feedback.
Supplementary Material
The Supplementary Material for this article can be found online at: https://www.frontiersin.org/articles/10.3389/fenrg.2020.00015/full#supplementary-material
Footnotes
1. ^In this paper, the term “environmental impacts” is used instead of “potential environmental impacts” to improve readability. However, LCA is not able to assess actual environmental impacts.
2. ^Following the ILCD handbook (European Commission - Joint Research Center, 2010) this shall be assumed as long as the additional supply or demand of the production system under study does not exceed a threshold value of 5% of the annual market size of a supplied or demanded product. The threshold value of 5% refers to an estimated share of production capacity which is annually decommissioned, i.e., production plants in the end of their life time (European Commission - Joint Research Center, 2010). If the additional supply or demand of the production under study exceeds 5% production capacity, plants are decommissioned that would otherwise still produce and thus, large scale, structural changes apply. This might be the case if CCU technologies are deployed on a global scale and thus, CCU technologies trigger large-scale changes. The ILCD handbook refers to this as the distinction between goal situation A and B.
3. ^The ILCD handbook refers to this as “virtual sub-division.”
4. ^CML-IA Characterisation Factors. Available online at: https://www.universiteitleiden.nl/en/research/research-output/science/cml-ia-characterisation-factors#features (accessed May 17, 2018).
5. ^The International ® System - Environmental Product Declarations. Available online at: https://www.environdec.com/ (accessed May 17, 2018).
6. ^Permanent storage can be assumed if CO2 is sequestered for 100,000 years.
References
AFNOR (2016). BP X30-323-0 General Principles for an Environmental Communication on Mass Market Products: Part 0: General Principles and Methodological Framework. Paris: AFNOR
Al-Kalbani, H., Xuan, J., Garcia, S., and Wang, H. (2016). Comparative energetic assessment of methanol production from CO2: chemical versus electrochemical process. Appl. Energy 165, 1–13. doi: 10.1016/j.apenergy.2015.12.027
Al-Mamoori, A., Krishnamurthy, A., Rownaghi, A. A., and Rezaei, F. (2017). Carbon capture and utilization update. Energy Technol. 5, 834–849. doi: 10.1002/ente.201600747
Anicic, B., Trop, P., and Goricanec, D. (2014). Comparison between two methods of methanol production from carbon dioxide. Energy 77, 279–289. doi: 10.1016/j.energy.2014.09.069
Aresta, M., Caroppo, A., Dibenedetto, A., and Narracci, M. (2002). “Life Cycle Assessment (LCA) applied to the synthesis of methanol. Comparison of the use of syngas with the use of CO2 and dihydrogen produced from renewables,” in Environmental Challenges and Greenhouse Gas Control for Fossil Fuel Utilization in the 21st Century, Boston, MA: Publisher Name Springer, 331–347.
Aresta, M., and Galatola, M. (1999). Life cycle analysis applied to the assessment of the environmental impact of alternative synthetic processes. The dimethylcarbonate case: part 1. J. Clean. Prod. 7, 181–193. doi: 10.1016/S0959-6526(98)00074-2
Artz, J., Müller, T. E., Thenert, K., Kleinekorte, J., Meys, R., Sternberg, A., et al. (2018). Sustainable conversion of carbon dioxide: an integrated review of catalysis and life cycle assessment. Chem. Rev. 118, 434–504. doi: 10.1021/acs.chemrev.7b00435
Arvidsson, R., Tillman, A. M., Sandén, B. A., Janssen, M., Nordelöf, A., Kushnir, D., et al. (2017). Environmental assessment of emerging technologies: recommendations for prospective LCA. J. Ind. Ecol. 80:40. doi: 10.1111/jiec.12690
Audi AG (2017). Audi g-Tron Models With Audi e-Gas: The Energy Revolution in the Tank. Available online at: https://www.audi-mediacenter.com/en/techday-on-combustion-engine-technology-8738/audi-g-tron-models-with-audi-e-gas-the-energy-revolution-in-the-tank-8749 (accessed March 27, 2018).
Baena-Moreno, F. M., Rodríguez-Galán, M., Vega, F., Alonso-Fariñas, B., Vilches Arenas, L. F., and Navarrete, B. (2019). Carbon capture and utilization technologies: a literature review and recent advances. Energy Sourc. A 41, 1403–1433. doi: 10.1080/15567036.2018.1548518
Bare, J. C. (2002). TRACI 2.0: the tool for the reduction and assessment of chemical and other environmental impacts 2.0. J. Ind. Ecol. 6, 49–78. doi: 10.1162/108819802766269539
Baumann, H., and Tillman, A.-M. (2004). The Hitch Hikers's Guide to LCA: An Orientation in Life Cycle Assessment Methodology and Application. Lund: Studentlitteratur.
Blengini, G. A., Garbarino, E., Šolar, S., Shields, D. J., Hámor, T., Vinai, R., et al. (2012). Life Cycle Assessment guidelines for the sustainable production and recycling of aggregates: the Sustainable Aggregates Resource Management project (SARMa). J. Clean. Prod. 27, 177–181. doi: 10.1016/j.jclepro.2012.01.020
Brandao, M., Levasseur, A., Kirschbaum, M. U. F., Weidema, B. P., Cowie, A. L., Jorgensen, S. V., et al. (2013). Key issues and options in accounting for carbon sequestration and temporary storage in life cycle assessment and carbon footprinting. Int. J. Life Cycle Assess. 18, 230–240. doi: 10.1007/s11367-012-0451-6
Brander, M. (2016). Transposing lessons between different forms of consequential greenhouse gas accounting: lessons for consequential life cycle assessment, project-level accounting, and policy-level accounting. J. Clean. Prod. 112, 4247–4256. doi: 10.1016/j.jclepro.2015.05.101
BSI (2011). PAS 2050 - Specification for the Assessment of the Life Cycle Greenhouse Gas Emissions of Goods and Services 13.310; 91.190. London: BSI.
Bumann, A. A., Papadokonstantakis, S., Sugiyama, H., Fischer, U., and Hungerbühler, K. (2010). Evaluation and analysis of a proxy indicator for the estimation of gate-to-gate energy consumption in the early process design phases: the case of organic solvent production. Energy 35, 2407–2418. doi: 10.1016/j.energy.2010.02.023
Carbon Recycling International (2019). Vulcanol. Available online at: http://carbonrecycling.is/projects-1/ (accessed March 27, 2018).
CarbonCure (2019). Contribution to LEED. Available online at: http://carboncure.com/sustainability/contribution-to-leed/ (accessed March 27, 2018)
Cespi, D., Beach, E. S., Swarr, T. E., Passarini, F., Vassura, I., Dunn, P. J., et al. (2015). Life cycle inventory improvement in the pharmaceutical sector: assessment of the sustainability combining PMI and LCA tools. Green Chem. 17, 3390–3400. doi: 10.1039/C5GC00424A
Cucurachi, S., van der Giesen, C., and Guinée, J. (2018). Ex-ante LCA of emerging technologies. Proc. CIRP 69, 463–468. doi: 10.1016/j.procir.2017.11.005
Cuéllar-Franca, R. M., and Azapagic, A. (2015). Carbon capture, storage and utilisation technologies: a critical analysis and comparison of their life cycle environmental impacts. J. CO2 Util. 9, 82–102. doi: 10.1016/j.jcou.2014.12.001
Curran, M. A. (2012). Life Cycle Assessment Handbook: A Guide for Environmentally Sustainable Products. Beverly, MA: Wiley-Scrivener.
Deutz, S., Bongartz, D., Heuser, B., Kätelhön, A., Schulze Langenhorst, L., Omari, A., et al. (2018). Cleaner production of cleaner fuels: Wind-to-wheel – environmental assessment of CO2 -based oxymethylene ether as a drop-in fuel. Energy Environ. Sci. 55:7296. doi: 10.1039/C7EE01657C
ecoinvent (2017). Data on the Production of Chemicals Created for the EU Product Environmental Footprint (PEF) Pilot Phase Implementation. Zürich: ecoinvent Association. Available online at: www.ecoinvent.org
European Commission - Joint Research Center (2010). ILCD Handbook - General Guide for Life Cycle Assessment - Detailed Guidance. Luxembourg: Publications Office.
European Commission - Joint Research Center (2011a). ILCD Handbook: Recommendations for Life Cycle Impact Assessment in the European Context. Luxembourg: Publications Office.
European Commission - Joint Research Center (2011b). Supporting Environmentally Sound Decisions for Bio-Waste Management: A practical guide to Life Cycle Thinking (LCT) and Life Cycle Assessment (LCA). Ispra: European Commission - Joint Research Center
European Commission - Joint Research Center (2012). Product Environmental Footprint (PEF) Guide. Ispra: European Commission - Joint Research Center.
European Commission - Joint Research Center (2018). Biomass Production, Supply, Uses and Flows in the European Union: First Results From an Integrated Assessment. Ispra: European Commission - Joint Research Center.
European Commission (2018). Scientific Advice Mechanism (SAM): Novel Carbon Capture and Utilisation Technologies. Luxembourg: European Commission.
European Committee for Standardisation (2009). ISO 14040- Environmental Management – Life Cycle Assessment – Principles and Framework 13.020.10. Berlin: Beuth Verlag GmbH.
European Committee for Standardisation (2017). ISO 14067 Greenhouse Gases – Carbon Footprint of Products – Requirements and Guidelines for Quantification and Communication 13.020.40. Berlin: Beuth Verlag GmbH.
European Committee for Standardisation (2018). ISO 14044 - Environmental Management – Life Cycle Assessment – Principles and Framework 13.020.10. Berlin: Beuth Verlag GmbH.
Frischknecht, R., Heath, G., Raugei, M., Sinha, P., and de Wild-Scholten, M. (2016). Methodology Guidelines on Life Cycle Assessment of Photovoltaic Electricity 3rd: EA PVPS Task 1. International Energy Agency Photovoltaic Power Systems Programme.
GaBi Software-System v8.5.0 79 Database for Life Cycle Engineering SP 35 (1992–2018). Leinfeld-Echterdingen: Thinkstep.
Garcia-Herrero, I., Cuellar-Franca, R. M., Enriquez-Gutierrez, V. M., Alvarez-Guerra, M., Irabien, A., and Azapagic, A. (2016). Environmental assessment of dimethyl carbonate production: comparison of a novel electrosynthesis route utilizing CO2 with a commercial oxidative carbonylation process. ACS Sustainable Chem. Eng. 4, 2088–2097. doi: 10.1021/acssuschemeng.5b01515
Gavankar, S., Anderson, S., and Keller, A. A. (2015a). Critical components of uncertainty communication in life cycle assessments of emerging technologies. J. Ind. Ecol. 19, 468–479. doi: 10.1111/jiec.12183
Gavankar, S., Suh, S., and Keller, A. A. (2015b). The role of scale and technology maturity in life cycle assessment of emerging technologies: a case study on carbon nanotubes. J. Ind. Ecol. 19, 51–60. doi: 10.1111/jiec.12175
Groen, E. A., Heijungs, R., Bokkers, E. A. M., and de Boer, I. J. M. (2014). Methods for uncertainty propagation in life cycle assessment. Environ. Model. Softw. 62, 316–325. doi: 10.1016/j.envsoft.2014.10.006
Guinée, J. B. (2006). Handbook on Life Cycle Assessment: Operational Guide to the ISO Standards. Dordrecht: Kluwer Academic Publishers.
Hauschild, M., and Huijbregts, M. A. J. (eds.). (2015). Life Cycle Impact Assessment. Dordrecht: Springer.
Heijungs, R. (2010). Sensitivity coefficients for matrix-based LCA. Int. J. Life Cycle Assess. 15, 511–520. doi: 10.1007/s11367-010-0158-5
Heijungs, R., and Frischknecht, R. (1998). A special view on the nature of the allocation problem. Int. J. LCA 3, 321–332. doi: 10.1007/BF02979343
Henriksson, P. J. G., Heijungs, R., Dao, H. M., Phan, L. T., Snoo, G. R. de, and Guinée, J. B. (2015). Product carbon footprints and their uncertainties in comparative decision contexts. PLoS ONE. 10:e0121221. doi: 10.1371/journal.pone.0121221
Hoppe, W., Bringezu, S., and Thonemann, N. (2016). Comparison of global warming potential between conventionally produced and CO2-based natural gas used in transport versus chemical production. J. Clean. Prod. 121, 231–237. doi: 10.1016/j.jclepro.2016.02.042
Hoppe, W., Thonemann, N., and Bringezu, S. (2017). Life cycle assessment of carbon dioxide-based production of methane and methanol and derived polymers. J. Ind. Ecol. 7:181. doi: 10.1111/jiec.12583
Huijbregts, M. A. J., Norris, G., Bretz, R., Ciroth, A., Maurice, B., Bahr, B., et al. (2001). Framework for modelling data uncertainty in life cycle inventories. Int. J. Life Cycle Assess. 6, 127–132. doi: 10.1007/BF02978728
Igos, E., Benetto, E., Meyer, R., Baustert, P., and Othoniel, B. (2018). How to treat uncertainties in life cycle assessment studies? Int. J. Life Cycle Assess. 176:359. doi: 10.1007/s11367-018-1477-1
International Energy Agency (2017). Energy Technology Perspectives 2017: Catalysing Energy Technology Transformations. Paris: OECD.
International Organization for Standardization (2014). ISO 14071 - Environmental Management - Life Cycle Assessment - Critical Review Processes and Reviewer Competencies: Additional Requirements and Guidelines to ISO 14044:2006. Berlin: International Organization for Standardization.
Jiménez-González, C., Kim, S., and Overcash, M. R. (2000). Methodology for developing gate-to-gate Life cycle inventory information. Int. J. Life Cycle Assess. 5, 153–159. doi: 10.1007/BF02978615
Jung, J., von der Assen, N., and Bardow, A. (2014). Sensitivity coefficient-based uncertainty analysis for multi-functionality in LCA. Int. J. Life Cycle Assess. 19, 661–676. doi: 10.1007/s11367-013-0655-4
Kaetelhoen, A., Bardow, A., and Suh, S. (2016). Stochastic technology choice model for consequential life cycle assessment. Environ. Sci. Technol. 50, 12575–12583. doi: 10.1021/acs.est.6b04270
Kaetelhoen, A., von der Assen, N., Suh, S., Jung, J., and Bardow, A. (2015). Industry-cost-curve approach for modeling the environmental impact of introducing new technologies in life cycle assessment. Environ. Sci. Technol. 49, 7543–7551. doi: 10.1021/es5056512
Kätelhön, A., Meys, R., Deutz, S., Suh, S., and Bardow, A. (2019). Climate change mitigation potential of carbon capture and utilization in the chemical industry. Proc. Natl. Acad. Sci. U.S.A. 116, 11187–11194. doi: 10.1073/pnas.1821029116
Kim, J., Henao, C. A., Johnson, T. A., Dedrick, D. E., Miller, J. E., Stechel, E. B., et al. (2011). Methanol production from CO2 using solar-thermal energy: process development and techno-economic analysis. Energy Environ. Sci. 4, 3122–3132. doi: 10.1039/c1ee01311d
Kim, S., and Overcash, M. (2003). Energy in chemical manufacturing processes: gate-to-gate information for life cycle assessment. J. Chem. Technol. Biotechnol. 78, 995–1005. doi: 10.1002/jctb.821
Levasseur, A., Lesage, P., Margni, M., Brandao, M., and Samson, R. (2012). Assessing temporary carbon sequestration and storage projects through land use, land-use change and forestry: comparison of dynamic life cycle assessment with ton-year approaches. Clim. Change 115, 759–776. doi: 10.1007/s10584-012-0473-x
Levasseur, A., Lesage, P., Margni, M., Deschênes, L., and Samson, R. (2010). Considering time in LCA: dynamic LCA and its application to global warming impact assessments. Environ. Sci. Technol. 44, 3169–3174. doi: 10.1021/es9030003
Lloyd, S. M., and Ries, R. (2007). Characterizing, propagating, and analyzing uncertainty in life-cycle assessment - a survey of quantitative approaches. J. Ind. Ecol. 11, 161–179. doi: 10.1162/jiec.2007.1136
Luu, M. T., Milani, D., Bahadori, A., and Abbas, A. (2015). A comparative study of CO2 utilization in methanol synthesis with various syngas production technologies. J. CO2 Util. 12, 62–76. doi: 10.1016/j.jcou.2015.07.001
Malmqvist, T., Glaumann, M., Scarpellini, S., Zabalza, I., Aranda, A., Llera, E., et al. (2011). Life cycle assessment in buildings: the ENSLIC simplified method and guidelines. Energy. 36, 1900–1907. doi: 10.1016/j.energy.2010.03.026
Mattila, T., Lehtoranta, S., Sokka, L., Melanen, M., and Nissinen, A. (2012). Methodological Aspects of Applying Life Cycle Assessment to Industrial Symbioses. Journal of Industrial Ecology 16, 51–60. doi: 10.1111/j.1530-9290.2011.00443.x
Matzen, M., and Demirel, Y. (2016). Methanol and dimethyl ether from renewable hydrogen and carbon dioxide: Alternative fuels production and life-cycle assessment. J. Clean. Prod. 139, 1068–1077. doi: 10.1016/j.jclepro.2016.08.163
Naims, H., Olfe-Kräutlein, B., Lorente Lafuente, A. M., and Bruhn, T. (2015). CO2 Recycling – An Option for Policymaking and Society? Twelve Theses on the Societal and Political Significance of Carbon Capture and Utilisation (CCU) Technologies. Institute for Advanced Sustainability Studies (IASS).
Parra, D., Zhang, X., Bauer, C., and Patel, M. K. (2017). An integrated techno-economic and life cycle environmental assessment of power-to-gas systems. Appl. Energy 193, 440–454. doi: 10.1016/j.apenergy.2017.02.063
Pehnt, M. (2006). Dynamic life cycle assessment (LCA) of renewable energy technologies. Renew. Energy 31, 55–71. doi: 10.1016/j.renene.2005.03.002
Pfingsten, S. von, Broll, D. O., von der Assen, N., and Bardow, A. (2017). Second-order analytical uncertainty analysis in life cycle assessment. Environ. Sci. Technol. 51, 13199–13204. doi: 10.1021/acs.est.7b01406
Schakel, W., Oreggioni, G., Singh, B., Stromman, A., and Ramirez, A. (2016). Assessing the techno-environmental performance of CO2 utilization via dry reforming of methane for the production of dimethyl ether. J. CO2 Util. 16, 138–149. doi: 10.1016/j.jcou.2016.06.005
Schenker, U., Scheringer, M., Sohn, M. D., Maddalena, R. L., McKone, T. E., and Hungerbühler, K. (2009). Using information on uncertainty to improve environmental fate modeling: a case study on DDT. Environ. Sci. Technol. 43, 128–134. doi: 10.1021/es801161x
Sills, D. L., Paramita, V., Franke, M. J., Johnson, M. C., Akabas, T. M., Greene, C. H., et al. (2013). Quantitative uncertainty analysis of Life Cycle Assessment for algal biofuel production. Environ. Sci. Technol. 47, 687–694. doi: 10.1021/es3029236
Sonnemann, G. W., Schuhmacher, M., and Castells, F. (2003). Uncertainty assessment by a Monte Carlo simulation in a life cycle inventory of electricity produced by a waste incinerator. J. Clean. Prod. 11, 279–292. doi: 10.1016/S0959-6526(02)00028-8
Souza, L. F. S., Ferreira, P. R. R., Medeiros, J. L. de, Alves, R. M. B., and Araújo, O. Q. F. (2014). Production of DMC from CO2 via indirect route: technical–economical–environmental assessment and analysis. ACS Sustain. Chem. Eng. 2, 62–69. doi: 10.1021/sc400279n
Sternberg, A., and Bardow, A. (2015). Power-to-What? - Environmental assessment of energy storage systems. Energy Environ. Sci. 8, 389–400. doi: 10.1039/c4ee03051f
Sternberg, A., and Bardow, A. (2016). Life cycle assessment of power-to-gas: syngas vs methane. ACS Sustain. Chem. Eng. 4, 4156–4165. doi: 10.1021/acssuschemeng.6b00644
Sternberg, A., Jens, C. M., and Bardow, A. (2017). Life cycle assessment of CO2 -based C1-chemicals. Green Chem. 19, 2244–2259. doi: 10.1039/C6GC02852G
Sugiyama, H., Fischer, U., Hungerbühler, K., and Hirao, M. (2008). Decision framework for chemical process design including different stages of environmental, health, and safety assessment. AIChE J. 54, 1037–1053. doi: 10.1002/aic.11430
Tanzer, S. E., and Ramírez, A. (2019). When are negative emissions negative emissions? Energy Environ. Sci. 12, 1210–1218. doi: 10.1039/C8EE03338B
The European Parliament and the council of the European Union (2019). Directive (EU) 2019/944. Brussels: The European Parliament and the council of the European Union.
Uusitalo, V., Vaisanen, S., Inkeri, E., and Soukka, R. (2017). Potential for greenhouse gas emission reductions using surplus electricity in hydrogen, methane and methanol production via electrolysis. Energ. Convers. Manag. 134, 125–134. doi: 10.1016/j.enconman.2016.12.031
van der Giesen, C., Kleijn, R., and Kramer, G. J. (2014). Energy and climate impacts of producing synthetic hydrocarbon fuels from CO2. Environ. Sci. Technol. 48, 7111–7121. doi: 10.1021/es500191g
Villares, M., Işildar, A., van der Giesen, C., and Guinée, J. (2017). Does ex ante application enhance the usefulness of LCA? A case study on an emerging technology for metal recovery from e-waste. Int. J. Life Cycle Assess. 22, 1618–1633. doi: 10.1007/s11367-017-1270-6
von der Assen, N., and Bardow, A. (2014). Life cycle assessment of polyols for polyurethane production using CO2 as feedstock: insights from an industrial case study. Green Chem. 16, 3272–3280. doi: 10.1039/c4gc00513a
von der Assen, N., Jung, J., and Bardow, A. (2013). Life-cycle assessment of carbon dioxide capture and utilization: avoiding the pitfalls. Energy Environ. Sci. 6, 2721–2734. doi: 10.1039/c3ee41151f
von der Assen, N., Voll, P., Peters, M., and Bardow, A. (2014). Life cycle assessment of CO2 capture and utilization: a tutorial review. Chem. Soc. Rev. 43, 7982–7994. doi: 10.1039/c3cs60373c
Wei, W., Larrey-Lassalle, P., Faure, T., Dumoulin, N., Roux, P., and Mathias, J.-D. (2016). Using the reliability theory for assessing the decision confidence probability for comparative life cycle assessments. Environ. Sci. Technol. 50, 2272–2280. doi: 10.1021/acs.est.5b03683
Weidema, B., Bauer, C., Hischier, R., Mutel, C., Nemecek, T., Reinhard, J., et al. (2013). Overview and Methodology. Data Quality Guideline for the Ecoinvent Database Version 3. St. Gallen. Available online at: https://www.ecoinvent.org/files/dataqualityguideline_ecoinvent_3_20130506.pdf
Wernet, G., Hellweg, S., Fischer, U., Papadokonstantakis, S., and Hungerbühler, K. (2008). Molecular-structure-based models of chemical inventories using neural networks. Environ. Sci. Technol. 42, 6717–6722. doi: 10.1021/es7022362
Wernet, G., Papadokonstantakis, S., Hellweg, S., and Hungerbühler, K. (2009). Bridging data gaps in environmental assessments: Modeling impacts of fine and basic chemical production. Green Chem. 11:1826. doi: 10.1039/b905558d
Williams, E. D., Weber, C. L., and Hawkins, T. R. (2009). Hybrid framework for managing uncertainty in life cycle inventories. J. Ind. Ecol. 13, 928–944. doi: 10.1111/j.1530-9290.2009.00170.x
World Resources Institute and World Business Council for Sustainable Development (2011). Greenhouse Gas Protocol: Product Life Cycle Accounting and Reporting Standard. Washington, DC; Geneva: World Resources Institute; World Business Council for Sustainable Development.
Yang, Y., and Heijungs, R. (2018). On the use of different models for consequential life cycle assessment. Int. J. Life Cycle Assess. 23, 751–758. doi: 10.1007/s11367-017-1337-4
Keywords: CO2 utilization, CCU, carbon capture and use, life cycle assessment, LCA, standardization, carbon capture and utilization, guideline
Citation: Müller LJ, Kätelhön A, Bachmann M, Zimmermann A, Sternberg A and Bardow A (2020) A Guideline for Life Cycle Assessment of Carbon Capture and Utilization. Front. Energy Res. 8:15. doi: 10.3389/fenrg.2020.00015
Received: 18 October 2019; Accepted: 23 January 2020;
Published: 14 February 2020.
Edited by:
Camille Petit, Imperial College London, United KingdomReviewed by:
Hannu-Petteri Mattila, Independent Researcher, Parainen, FinlandRosa Marisa Cuellar-Franca, University of Manchester, United Kingdom
Copyright © 2020 Müller, Kätelhön, Bachmann, Zimmermann, Sternberg and Bardow. This is an open-access article distributed under the terms of the Creative Commons Attribution License (CC BY). The use, distribution or reproduction in other forums is permitted, provided the original author(s) and the copyright owner(s) are credited and that the original publication in this journal is cited, in accordance with accepted academic practice. No use, distribution or reproduction is permitted which does not comply with these terms.
*Correspondence: André Bardow, andre.bardow@ltt.rwth-aachen.de