- 1Research Institute of Circular Economy, Tianjin University of Technology, Tianjin, China
- 2College of Humanities, Tianjin Agricultural University, Tianjin, China
In response to climate change and continued reliance on traditional high-carbon fossil fuels, promoting the transition toward sustainable energy systems by development of low-carbon energy resources has been seen as the main strategy for mitigating and solving global climate change. However, the promotion of low-carbon energy also faces material supply risks. To provide a reference for the steady and rapid development of renewable energy and other energy in the future energy market, this paper considers renewable energy prediction based on a long- and short-term memory network model as well as the growth rate changes of crude oil, natural gas, nuclear energy, financial revenues, and expenditure. In the prediction process, it is found that natural gas will be a strong competitor for the development of renewable energy in the future. When natural gas grows too quickly, the growth of renewable energy will be negative. On the other hand, when the monthly growth rate of natural gas and crude oil is smaller than that of nuclear energy, renewable energy will display a growth trend, and the rate will increase with the growth of natural gas and nuclear energy. What is more, wind and solar energy will be limited by metallic materials, such as Dy, Nd, Te, and In. Improving the energy density of metals plays a key role in China’s transition to a low-carbon energy structure.
Introduction
Renewable energy is an important means to control and reduce carbon emissions in the process of heat generation and transportation. Its main output form is power supply, which can be directly put into production and application. It does not need a large amount of investment in transportation infrastructure like traditional energy required in the past. China’s overall electricity demand has been growing rapidly, according to the International Energy Agency (IEA). The total electricity generation capacity in China increased significantly from 621.268 TWh in 1990 to 6282.607 TWh in 2016. During the same period, the renewable energy generation capacity increased from 126.788 to 1570.566 TWh, among which wind power generation capacity increased from 0.002 to 237.071 TWh and solar photovoltaic power generation capacity increased from 0.002 to 75.256 TWh. The data show that China’s electricity consumption and demand for renewable energy have been growing steadily.
In terms of electricity supply, hydropower accounts for a large proportion of China’s total renewable energy generation, compared with wind and solar. For now, however, China’s main means of generating electricity is still through the burning of fossil fuels. According to the IEA’s statistics on China’s electricity generation from fossil fuel combustion, the share of thermal power generation in China’s total electricity generation decreased from 79.59% in 1990 to 70.40% in 2016. Although China has gradually attached importance to nuclear power plant construction and renewable energy power generation since 1990, it has gradually realized that coal, oil, and natural gas, which will eventually run out, cause great harm to the environment and are the key factors leading to the greenhouse effect and global warming. However, the data show that China’s dependence on traditional energy sources remains a serious problem.
In 2016, China issued the 13th 5-year plan for renewable energy development, which aims to achieve emission reduction targets and accelerate the construction of a low-carbon and safe modern energy system. In the same year, the share of renewable energy in the total power generation rose from 16.14% in 2005 to 25% in 2016, which was largely dependent on national investment in renewable energy. According to statistics from the national energy administration of China, in 2018, when the total electricity consumption was 6844.9 TWh, the year-on-year growth was 8.5%, indicating that China’s electricity demand is still on the rise. However, in 2017, China abandoned 55 TWh of water energy, 41.9 TWh of wind energy, and 7.3 TWh of solar energy, and the total amount of waste exceeded 100 TWh, equivalent to the annual electricity output of the Three Gorges power station in 2018, the world’s largest power station. As a result, the capacity of renewable energy has not been perfectly matched with that of the energy storage equipment in the past few years.
More than that, the development and utilization of renewable energy are inseparable from many mineral resources, especially critical metals which are under high supply owing to high concentration of production and lack of available suitable substitutes. This issue has aroused worldwide concern. For the German wind industry, Shammugam et al. (2019) sees copper and dysprosium as the most critical materials for development, both of which clearly exceed Germany’s quota for renewable energy technologies and will face stiff competition from other sectors for access to raw materials. In the United States, renewable energy (mainly wind and solar) is particularly constrained by Te and Dy (Nassar et al., 2016). However, the concern of China’s energy–mineral nexus is far behind the environmental benefits and costs brought by renewable energy.
The characteristics of renewable energy are production of energy from renewable sources and environmental protection. The development of renewable energy is an important way to reduce traditional energy dependence and economic decarbonization. At the same time, Apergis et al. (2018) concluded in his research that investing in renewable energy and medical treatment would help reduce carbon emissions, and abundant renewable energy could cope with climate change and improve citizens’ health. Therefore, it is particularly important to ensure the technology supply of renewable energy and promote the transformation of China’s energy system. Forecasting the generation capacity of renewable energy can’t only prevent the waste of power resources, such as the excess capacity of electric power and the mismatch between the power supply and the development speed of the power grid, but also further promote the healthy development of China’s renewable energy power generation industry. Therefore, this paper adopts the long- and short-term memory network (LSTM) renewable energy generation prediction method to try to determine an accurate prediction value and then further discuss the key metal demand on this basis, hoping to provide an important basis for China’s future renewable energy policy.
Literature Review
Selection of Relevant Indicators for Renewable Energy Prediction
Many renewable energy prediction studies began in 2000 (Tsai et al., 2017). Because of the uncertainty of renewable energy (Notton et al., 2018), the perspective of its prediction is diversified, which prompts each study to selectively consider different factors in its prediction, such as traditional energy (Nadimi and Tokimatsu, 2017), nuclear energy (Kok and Benli, 2017), policy (Black et al., 2014), installed capacity (Zhou et al., 2018), technology (Ruedabayona et al., 2019), and economy (Ozcan and Ozturk, 2019). Therefore, it is very difficult to accurately summarize and classify existing studies, but summarizing and reviewing existing studies is helpful to highlight the characteristics of this study.
The application of renewable energy can replace the burning of fossil fuels. Some studies have predicted that increasing the proportion of renewable energy in energy consumption can reduce traditional energy consumption and reduce carbon emissions (Chen et al., 2019). Research on the prediction of renewable energy based on production cost shows that the extraction time and technological cost of fossil fuels are gradually increasing, and the investment yield of renewable energy is expected to be lower than that of oil and natural gas production (Baranes et al., 2017). The advantages of renewable energy power production are increasingly prominent. So, the difference between renewable energy and traditional energy mainly lies in the sustainable use of energy and the low cost and impact on the environment (Craig et al., 2019). There are many forms of renewable energy sources that provide electricity, such as solar energy, wind energy, biological energy, and hydropower, and can provide power in an isolated or mixed way (Amri, 2019). This has led to many parts of the world making use of renewable energy sources. For example, solar energy is the easiest way to get free energy and is friendly to the environment; solar radiation on the surface of the earth is about 1.5 × 1018 KWh/year, which is about 10,000 times the global energy consumption (Sharma and Kakkar, 2018). In addition, for renewable energy resources, the extraction, processing, and power generation operations tend to be a single operation (Stokes and Breetz, 2018). Hydropower stations only need to collect water from rivers and mountain runoffs to provide power. Wind farms can generate electricity simply by turning blades with the force of the wind.
However, renewable energy also has its shortcomings and brings difficulties to its prediction. The associated research found that the dependency of solar and wind energy resources on the weather, with its randomness and volatility, makes electric power production and distribution uncertain and intermittent (Das et al., 2018), and can lead to sudden emergency power supply problems. Therefore, other forms of energy are needed to serve as a necessary supplement (Jenkins et al., 2018). For this reason, research on the prediction of renewable energy by nuclear energy shows that the joint investment return rate of nuclear energy, natural gas, and renewable energy is higher than 30%, whereas the investment return rate of only using renewable energy is only between 5 and 30% (Walmsley et al., 2018). It is expected that when building an energy system that relies on primary natural resources such as hydropower, wind power, geothermal power, and solar energy, this can make up for the shortage of natural resources that cannot meet the power supply demand.
High energy dependence and environmental degradation can provide strong incentives for the utilization of renewable energy. Cadoret and Padovano (2016) find that when the government only considers the environment and does not pay enough attention to the energy market, renewable energy may increase, and these environmental policies ignore energy costs which will have an impact on economic development. From this perspective, government financial support can also play an important role. In developed countries, economic efficiency is an important factor for the success of renewable energy projects (Belaid and Youssef, 2017). A major problem with renewable energy production is its direct economic cost (Newbery, 2016), which also depends on economic development. Renewable energy technology is a capital-intensive technology (Huh and Lee, 2014), so it needs a lot of investment, especially in the early stages of its development. The cost for developing countries is much higher than that of developed countries (Moriarty and Wang, 2015). In addition, in developing countries, labor force is often insufficient and the investment environment is more unstable, which makes the economic value created by renewable energy uncertain (Reddy, 2018). However, economic growth usually leads to an increase in renewable energy production (Narayan and Doytch, 2017). As a result, the government is making investments in renewable energy more valuable by changing the direction of the market through fiscal and policy measures.
National or regional renewable energy forecasting research should be a comprehensive study of all types of energy and fiscal policies. Through the analysis of the above renewable energy prediction research, it can be found that this only focuses on the advantages of renewable energy from an environment perspective, and mostly lacks the consideration of its intermittent power supply, which can easily cause a sudden power supply shortage. However, nuclear energy can only compensate for the shortage of renewable energy in a limited way owing to the construction cycles of the nuclear power industry and the limitations of its safety problems (Dong et al., 2018). The prediction of government finance and policy support can indeed achieve the purpose of accurately predicting renewable energy, but it ignores the impact of other energy sources. This paper forecasts renewable energy from a more comprehensive perspective based on comprehensive indicators such as traditional energy, nuclear energy, and fiscal revenue and expenditure, and further discusses its impact on renewable energy.
Prediction Methods for Renewable Energy
Renewable energy prediction research can be divided into two categories. One kind is the medium- and long-term forecast which takes the social economic index or some depend on long-term measurements as the characteristic input. The other is a short-term prediction based on wind speed or illuminance. The two types of prediction use different methods because of different data volumes. The first kind of prediction research mentioned earlier is relatively mature; in this kind of study, the most widely used prediction method is the use of regression model (Wu et al., 2019) as well as fuzzy logic-based prediction (Sivaneasan et al., 2017), autoregressive integrated moving average model (ARIMA) (Aasim et al., 2019), Gray model (GM) (Liu et al., 2016), support vector regression (SVR) (Almusaylh et al., 2018), and artificial neural network (ANN) (Ghimire et al., 2019). These prediction methods have their own advantages. For example, for short-term prediction, linear multivariable prediction, fuzzy logic prediction, and neural networks based on regression models are the most accurate, whereas the SVR model is the most appropriate for a single variable. For long-term prediction, ARIMA and GM are the most accurate models for linear univariate prediction. The accuracy and stability of the prediction model can be improved, and accurate judgment can be made quickly and with high probability. Although these prediction models each have advantages, they also have disadvantages. The results obtained by the regression prediction method cannot reflect the periodicity, and the fuzzy logic prediction lacks specific algorithm formula. Although the GM is accurate, it cannot reflect the dynamic change, whereas the SVR and the ANN often fail to show high accuracy because of insufficient data.
In the second category of short-term prediction research, the methods are relatively uniform, mostly using LSTM. The LSTM model has the ability to memorize the characteristics of time series. Compared with other neural networks, LSTM models can effectively use historical data to predict future time points. This is widely used in prediction because of its prominent advantages for time series prediction. Zheng et al. (2019) used an LSTM model to predict electricity consumption. Zhang et al. (2019) used an LSTM model to predict short-term power generation. Cortez et al. (2018) confirmed that an LSTM model is more suitable for predicting emergencies than traditional time series models and machine learning methods. Srivastava and Lessmann (2018) applied an LSTM model to predict solar energy and concluded that this is a reliable prediction model. Yu et al. (2019) used a combined LSTM-EFG model to predict wind speed.
Recently, China’s national bureau of statistics released monthly renewable energy supply data to provide support for relevant researchers. In this paper, an LSTM model is introduced that combines five characteristic inputs: crude oil output, natural gas output, nuclear power generation, and fiscal revenue and expenditure. It is expected that the construction of a prediction model that factors in the interaction of traditional energy, nuclear energy, and financial support will enable the prediction and comparison of the generation of renewable energy.
Key Metal for Renewable Energy
In recent years, many official reports and academic studies have shown concern about the mineral density of renewable energy (Phadke, 2017; Vidal et al., 2017). For example, the European Union formulated the Strategic Energy Technology Plan (SET-Plan) to ensure the competitiveness of industrial enterprises in the new energy supply value chain. The plan prioritized six techniques: nuclear fission, solar photovoltaic (PV) and concentrating solar power, wind energy, biological energy, carbon capture and storage, and power grid. Some scholars further studied on the metal materials in the use of the six low-carbon energy technologies for large-scale technology deployment and identified the five metals with high risk (Moss et al., 2013). Although a comprehensive deployment of renewable energy is necessary, considering the development of renewable energy in China, this study focuses on sorting out metal material requirements related to hydropower, wind, and solar energy.
Hydropower
Installed hydropower capacity is the largest source of renewable energy in the power sector, accounting for about 17% (Mallin, 2018) of global electricity demand. In terms of electricity supply, hydropower also accounts for a larger share of China’s total renewable energy generation than wind and solar. Hydropower plants are mainly divided into four categories: run-of-river, reservoir, pumped storage, and in-stream technology. Each type requires different materials as a result of different scenarios of technical application. Roughly, steel and concrete make up the largest proportion of the pipes and pressure pipes used in the construction of hydropower stations. Steel is the main material for turbines, copper (about 8%) is needed for generators and aluminum is the main component for transmission lines.
Wind and Solar Power
Nassar et al. (2016) analyzed the by-product metal demand of wind and solar PV power generation in the United States by 2040 and summarized 11 by-product metals related to 5 key renewable energy technologies (crystalline silicon solar cells (c-Si), cadmium telluride solar cells (CdTe), copper indium gallium (di), selenide solar cells (CIGS), amorphous silicon germanium solar cells (a-SiGe), and wind turbines with Nd2Fe14B permanent magnets), as follows: silver (Ag), cadmium (Cd), selenium (Se), tellurium (Te), indium (In), germanium (Ge), neodymium (Nd), praseodymium (Pr), dysprosium (Dy), terbium (Tb), and gallium (Ga). Shammugam et al. (2019), who studied wind turbines, conducted a comprehensive assessment of the metals used in the development of the German wind industry and identified steel as the most needed material for wind turbines, followed by copper and alloys such as chromium, manganese, and nickel. In addition, copper and dysprosium, which form the basis of wind turbines, will face particular competition from PV technology on a large scale. Based on the deployment of China’s six wind and solar PV technologies, Wang et al. (2019) proposed eight key materials (neodymium, dysprosium, cadmium, tellurium, indium, gallium, selenium, and germanium). He believed that in the future, wind energy–related materials will increase by 230 to 312 times, and solar energy–related materials will increase by 20 to 137 times. In addition, China needs more critical materials to support future renewable energy targets than Germany and the United States.
Data and Methods
Data Sources and Methods
The data used in this paper are all from the monthly data from nuclear power generation, crude oil, natural gas, renewable energy generation, fiscal revenue, expenditure, and six other indicators in the China national statistical yearbook. Considering the historical stage of China’s renewable energy development, here, renewable energy generation is the sum of hydropower generation, wind power generation, and solar power generation. The data are divided according to a 9:1 ratio, which is determined by sample experiments. The research sample in this paper is a total of 108 sets of data from September 2009 to August 2018. For training, 90% of the data were used; that is, 97 sets of data from September 2009 to September 2017 were taken as the training samples. The remaining 10% were used as test samples, i.e., 11 sets of data samples from September 2017 to August 2018. Of the training samples, 90% are used for model construction, and the assessment of prediction accuracy is performed with the remaining 10%.
As the country with the largest population and consumption, China is the cradle of “digital capital.” In recent years, China has continuously released more detailed data to facilitate research on various issues, especially monthly data concerning energy and fiscal revenue and expenditure. This can provide a new perspective for the prediction of renewable energy. A large amount of macro data supports the research and enables the use of more advanced LSTM prediction models. Compared with the traditional time series approach, which requires only a small amount of data to achieve a very high accuracy, training with more data will also make the results more accurate and reliable. To illustrate the function of the LSTM storage unit, in Figure 1 we use arrows to indicate the operation of a single local LSTM unit in a layer of the network at time step t. Through activation functions sigmoid, tanh, and ϕ, the corresponding Input Gate (it), Cell (Ct), Output Gate (ot), and Forget Gate (ft) values of the gate are calculated at the same time with the previous time step (h(t–1)). The output is the same but also receives the input data related to the current time step (xt). These are shown in Eqs 1–6. Here, θxn and θhn are the values before the input and output data of the unit weight matrix, and bn with n∈(i,g,f,o) of the bias vector identify the gate.
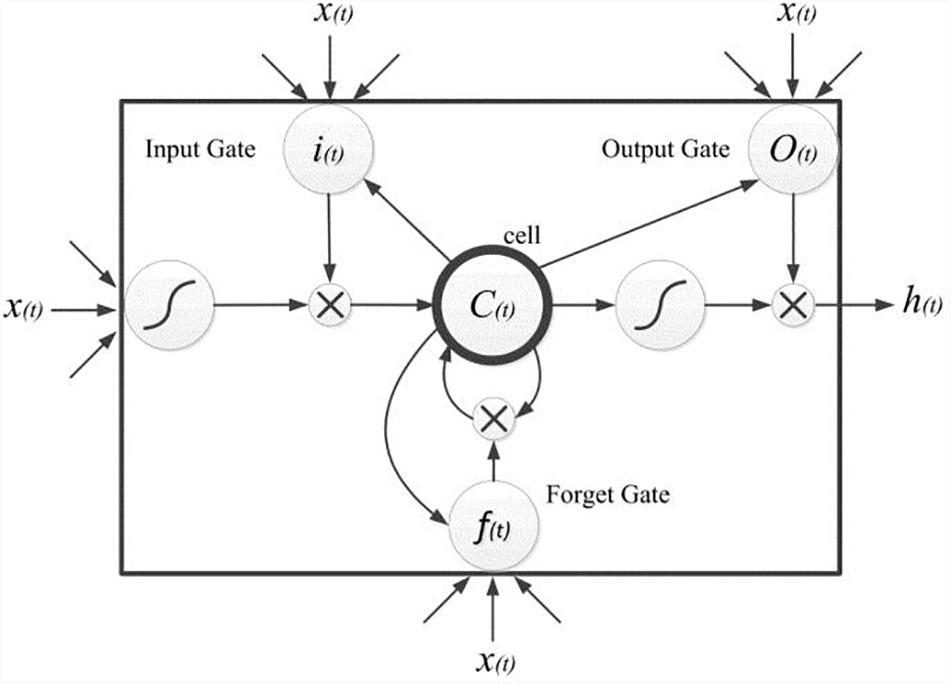
Figure 1. LSTM unit structure (Graves, 2013).
Model Building and Scenario Setting
In their forecast of China’s energy demand and self-sufficiency, Xie et al. (2015) proposed that the self-sufficiency of crude oil would drop from 40.6 to 35.9% and that of natural gas from 73.1 to 68.6% from 2015 to 2020. From Figure 2, it can be seen that renewable energy, crude oil, natural gas, and nuclear power vary over time regarding financial revenue and expenditure, but from 2015 to 2018 there is a downward trend for crude oil and the other data. This confirms Xie et al. (2015) on the study of the self-sufficiency rate of crude oil, but the trend of natural gas production has not declined over the same period, but gradually rose in more than 10 years. Perhaps because natural gas is a clean source of energy, it can be regarded as a supplement to renewable energy without being restricted in exploitation. Thus, to ensure the integrity of experiments and the reliability of the forecasting model, based on 2.1, 2.2, and 2.3, the data are divided into three types of prediction index of renewable energy. The first category is crude oil, natural gas on behalf of non-renewable energy, and nuclear energy forecast of renewable energy (Merge1). The second is the projections of renewable energy by nuclear energy and fiscal revenues and expenditures (Merge2). The third category is the prediction of renewable energy by crude oil, natural gas, and financial balance (Merge3). In the end, seven methods can be used to predict renewable energy in a single and combined form.
The results can be seen in Table 1. The RMSE is the root mean square error, as shown in Eq. 7. The emergence of abnormal points (such as abnormally large and abnormally small values) will lead to errors in the model. According to the characteristics of intermittent renewable energy, abnormal values are considered to be normal, so the use of RMSE to measure the error is not reasonable. MAPE obtains the ratio between the true value and the predicted value, which is more appropriate for determining the accuracy of renewable energy prediction, and is shown in Eq. 8. In similar studies, Lu (2019) used MAPE as an indicator to measure the accuracy of the model. Thus, it is concluded that the three indexes are the most accurate in predicting renewable energy (Merge4), and the best prediction model is shown in Figure 2.
where N is the total number of data samples, y is the actual value, and x is the predicted value.
According to the IEA, the share of the world’s energy supply for renewable energy will be 22% by 2015 and 31% by 2035. In the medium- and long-term development plan for renewable energy, the key areas for development from 2010 to 2020 are hydropower, bio-energy, wind energy, solar energy, and other renewable energy, including geothermal energy and marine energy. In recent years, the utilization of renewable energy has been assigned great importance in China. Renewable energy rose 15.1% from 2014. China’s renewable energy now accounts for 16.7% of the world’s total, up from 1.2% a decade ago. According to the 13th 5-year plan (2015–2020), non-fossil energy will account for 15% of the total primary energy consumption by 2020. Data shows that China’s renewable energy generation capacity (including hydropower generation) has reached 14,386.7 TWh in 2017, indicating that renewable energy resources are very rich, but using them involves some special technical, economic, and environmental issues. In BP’s 2019 energy outlook forecast for China’s energy market in 2040, demand for almost all fuels is growing, with oil up 19%, natural gas up 1.66 times, nuclear up 4.05 times, and renewable power up 5.84 times. Renewable energy is becoming a bigger part of the global energy mix, especially in the power sector. Therefore, in the discussion concerning renewable energy growth trends, three scenarios are considered:
Scenario 1: although China’s major energy and fiscal monthly data show an overall upward trend in recent years, as a result of the implementation of the tax reduction policy, the pressure of balance of fiscal revenues and expenditures has gradually increased, as shown in Figure 3. Therefore, the lowest growth rate (non-negative) of the fiscal revenue and expenditure and other data is calculated based on the growth of the historical data, so as to predict the future trend of renewable energy and provide the basis for scenarios 2 and 3.
Scenario 2: considering the intermittency of renewable energy and the difficulty in matching power production and actual installed capacity, it is assumed that the renewable energy power supply displays stable growth under the restraining effect of historical crude oil, natural gas exploitation and nuclear power supply and the constraint of financial revenue and expenditure.
Scenario 3: to recognize the role of renewable energy in environmental improvement and emissions reduction, assuming that the development of renewable energy is based on financial incentives, this presents a kind of average (non-negative) rate of additional growth.
In Eqs (9–12), R1, R2, and R3 are the monthly growth, rates of scenario 1, scenario 2, and scenario 3, including crude oil, natural gas, nuclear energy, fiscal expenditure, and fiscal revenue. V(t0) is the value of the starting month, V(tn) is the value of the ending month, n is the number of months in the range, and N is the number of data samples.
Results and Discussion
Results
It has been shown in section “Model Building and Scenario Setting” that the prediction of renewable energy by the LSTM model requires more accurate input of the five data indexes: crude oil, natural gas, nuclear energy, and fiscal revenue and expenditure. Therefore, the selection of input characteristics in various prediction studies is also an important factor affecting the accuracy of the model. On this basis, the monthly growth rate of renewable energy in 2019 is estimated from three scenarios according to the outlook of BP, an authoritative international institution, on renewable energy in China and the historical trend analysis concerning renewable energy related data, as shown in Table 2.
According to the different rate of all data, it can be divided into three different growth trends. The nuclear energy in scenario 1 will remain unchanged for the next 16 months, with the fastest growth in scenario 3. Natural gas, as a clean energy with higher core security, shows an increasing trend in all three scenarios, among which scenario 1 has the fastest growth rate of 3.3% per month. Crude oil grew the fastest in scenario 3 and the lowest in scenario 1, which was only 0.02%. In terms of fiscal revenue and expenditure, the growth rates of scenario 1 and scenario 2 are the same, respectively 0.28 and 0.87%, lower than scenario 3.
Based on the aforementioned scenarios, renewable energy output was predicted, and the trend variation and predicted values for the three scenarios were obtained as shown in Figure 4. The comparison of the growth rate of the predicted results for renewable energy under the three scenarios is shown in Figure 5. In scenario 1, the renewable energy fluctuates between 1288.81 and 1297.13 TWh, with the lowest and highest growth rates of −21 and 0.06%, respectively. In scenario 2, the renewable energy fluctuates between 1295.12 and 1513.07 TWh, with the lowest and highest growth rates of 0.28 and 1.55%, respectively. In scenario 3, the renewable energy fluctuates between 1295.12 and 1549.18 TWh, with the lowest and highest growth rates of 0.51 and 1.8%, respectively.
Here, we consider a comparative analysis of the indicators. For scenario 1, nuclear power remains the same, whereas other indexes show a state of growth. However, the predicted results indicated a decline. Also, in this scenario, the growth of natural gas is faster than other indicators. Therefore, it is concluded that gas is a strong competitor to the future development of renewable energy. In scenario 2, all the indexes showed an upward trend. Scenario 1 compared various indicators of growth, and the growth rate here reduced to 0.68% for natural gas, nuclear energy growth rose to 1.66%, and other indicators of growth essentially remained the same. The result showed an upward trend in sharp contrast to the results from scenario 1, and demonstrated the likely development of renewable energy in the future, the importance of nuclear energy to the synergy of renewable energy, and at the same time once again verified safe, clean energy is a development in the future of the enemy. In scenario 3, the monthly growth rate of the indicators is similar to scenario 2, where growth increased month by month. The renewable energy prediction results showed an upward trend to nearly the same extent as scenario 2, and the predicted values were higher than that month. This confirms that when crude oil, natural gas has increased less than nuclear power, regardless of the trend of financial revenue and expenditure, renewable energy will still increase. This growth is likely to increase with the increase of the indicators.
Discussion
Influence of Traditional Energy on Renewable Energy
China’s energy demand is still rising, but environmental problems cannot be ignored. It is an inevitable trend to develop new energy sources to replace traditional energy sources and reduce environmental pollution. Nearly 200 parties to the 2015 Paris agreement agreed to limit global warming to 1.5°C, and China pledged to reduce its carbon intensity by 40 to 45% by 2020. To fulfill its commitment to the world, China has made efforts to develop renewable energy through a number of policies, making renewable energy increasingly competitive in terms of efficiency, energy production, and economic efficiency. Both in terms of investment efficiency and environmental factors, renewable energy has become the most suitable energy source for China’s development, which is also the main reason for the reduction of carbon emissions. Similarly, although natural gas is a kind of non-renewable energy, it is undeniable that it is a kind of clean energy and plays an important role in reducing environmental pollution. Therefore, the competition between natural gas and renewable energy is the most obvious among non-renewable energy. Furlan and Mortarino (2018) have verified that renewable energy and coal, oil, natural gas, and nuclear power are competitors. The study in this paper also found that there is a negative relationship between natural gas and renewable energy, and that the conditions for the existence of this relationship, namely crude oil, mean that natural gas is growing faster than nuclear power indicators such as financial revenue growth. Therefore, the appropriate restriction of natural gas production in the future energy policies of the Chinese government may have a positive impact on the development of renewable energy.
Influence of Nuclear Energy on Renewable Energy
In 2016, nuclear energy accounted for 2% of China’s total power generation, and the IEA also announced that nuclear energy is expected to only account for 4% of China’s total power generation in 2040. However, at the end of 2017, China’s carbon intensity has been reduced by 46%, and non-fossil energy accounts for 14.3% of primary energy consumption in 2018. Compared with the target of reaching 15% by 2020, the gap is only 0.7%. It can be seen that China cannot solely rely on nuclear energy to reach the emissions reduction goal. Thus, renewable energy, as an energy market, is in a state of rapid development with remarkable achievements, but the intermittency problem will increase with the proportion of renewable energy power generation, with its long construction period and relatively small, but clean, energy. The stability of nuclear power can be used to supplement renewable energy sources. This research also found that when the nuclear growth is faster than for natural gas, renewable energy power will increase. In the case of fiscal revenue and expenditure, the growth rate of gas is less than the growth rate of nuclear energy, and renewable energy will increase. This means that the limited production of natural gas and control of its consumption can lead to more renewable energy, reducing carbon emissions. As Li and Lu (2019) proposed, natural gas consumption will be limited to between 9 and 14%; China’s 2020 carbon intensity will be 15.27% lower than in 2015.
Assessment of Supply Risks for Individual Metals
Based on the results mentioned previously, it is estimated that the cumulative supply of renewable energy in the next 16 months will be between 20,677.25 and 22,816.83 TWh. In 2018, China’s renewable energy generation capacity reached 1.9 trillion KWh, including 1.2 trillion KWh of hydropower, 366 billion KWh of wind power, and 177.5 billion KWh of PV power, accounting for 63, 19, and 9%, respectively (Ministry of Ecology and Environment).1 On this basis, it is roughly estimated that in the next 16 months, the cumulative wind energy supply will be between 3928 and 4335 TWh, and the cumulative solar energy supply will be between 1860 and 2053 TWh. Referring to the analysis of the most common metal requirements for each energy technology by Moss et al. (2013) and production and reserve of seven metals in Table 3, the demand of Te and In is more than two times that of the production in China in 2015. Both metals also face significant supply risks from their holdings in China. Dy and Nd, as key materials for wind energy, are close to the total amount of mining in 2015 and may face the threat of competition with other sectors in the future.
The future of solar energy in China will be limited by the need for metal materials, and the deployment of wind materials will need to be accelerated. To solve this dilemma, the transformation of China’s energy system needs to proceed from the following aspects: (1) encouraging the application of technologies to improve the energy density of metals, thus easing the dependence on materials; (2) increase recycling techniques to improve mine recovery rates, mineral processing, and smelting recoveries; (3) promote the reasonable extension and combination of industrial chain of circular economy and improve the recycling of waste; (4) in terms of hydrogen energy infrastructure, promote the advanced system of hydrogen production, hydrogen supply, and hydrogenation and the main role of enterprises, and improve the technical standards and testing system.
Conclusion
The accurate prediction of renewable energy can be achieved. It can provide a strong basis for the state to invest in the construction of capacitors in the power industry, and effectively solve the waste of wind, water, and solar energy and other energy caused by the lack of energy storage equipment in China’s current renewable energy power supply field, and contribute to China’s sustainable development. Based on non-renewable energy, nuclear energy, fiscal balance, and other factors related to the development of renewable energy, this paper establishes a prediction model and forecasts China’s renewable energy consumption from September 2018 to December 2019 based on various monthly data recently released by the Chinese bureau of statistics. Through the verification and analysis in this paper, the following three important conclusions can be drawn:
(1) Through the process of selecting indicators through the LSTM prediction model, it is concluded that China’s renewable energy is intrinsically related to five variables, including crude oil, natural gas, nuclear energy, financial revenue, and expenditure. The prediction of China’s renewable energy consumption can be achieved by monitoring these five variables.
(2) In the process of data collection and experimental comparison, this paper concludes that in the future development of renewable energy, nuclear energy has an important synergistic effect on its development, and at the same time verifies that natural gas, a safe and clean energy source, is a strong competitor to the development of renewable energy in the future.
(3) It is confirmed that when the growth rate of crude oil and natural gas is smaller than that of nuclear energy, regardless of whether the financial balance remains unchanged or grows, renewable energy will still grow, and this growth may increase with the increase of various indicators. Conversely, when natural gas production increases rapidly, renewable energy capacity declines.
(4) The development of China’s renewable energy, especially solar energy, is restricted by metal materials, among which Te and In are the most prominent. Improving the energy density of metals plays a key role in China’s transition to a low-carbon energy structure.
In addition, there are some deficiencies in the prediction process of China’s renewable energy. Although the Chinese government has provided researchers with a larger amount of data in recent years, the limited sample size caused by the relatively difficult data collection limits the prediction ability of the LSTM model adopted in this paper. Therefore, the prediction accuracy of renewable energy is not obviously superior to other traditional energy prediction models. It is hoped that with the accumulation of data, the LSTM approach will have more advantages in predicting renewable energy.
Data Availability Statement
Publicly available datasets were analyzed in this study. This data can be found here: https://data.stats.gov.cn/english/easyquery.htm?cn=C01.
Author Contributions
BL and JC carried out the concepts, design, definition of intellectual content, literature search, data acquisition, data analysis, and manuscript preparation. HW provided assistance for data acquisition, data analysis, and statistical analysis. JC carried out literature search, data acquisition, and manuscript editing. QW performed manuscript review. All authors have read and approved the content of the manuscript and have made substantial contributions to all of the following: (1) the conception and design of the study, or acquisition of data, or analysis and interpretation of data, (2) drafting the manuscript or revising it critically for intellectual content, (3) final approval of the version to be submitted.
Funding
The funding sources of this work include the National Natural Science Foundation of China (grant number 71503180) and Science Foundation of Ministry of Education of China (grant number 20YJA630042).
Conflict of Interest
The authors declare that the research was conducted in the absence of any commercial or financial relationships that could be construed as a potential conflict of interest.
Footnotes
References
Aasim, Singh, S. N., and Mohapatra, A. (2019). Repeated wavelet transform based ARIMA model for very short-term wind speed forecasting. Renew. Energy 136, 758–768. doi: 10.1016/j.renene.2019.01.031
Almusaylh, M. S., Deo, R. C., Adamowski, J., and Li, Y. (2018). Short-term electricity demand forecasting with MARS, SVR and ARIMA models using aggregated demand data in Queensland, Australia. Adv. Eng. Informat. 35, 1–16. doi: 10.1016/j.aei.2017.11.002
Amri, F. (2019). Renewable and non-renewable categories of energy consumption and trade: do the development degree and the industrialization degree matter? Energy 173, 374–383. doi: 10.1016/j.energy.2019.02.114
Apergis, N., Jebli, M. B., and Youssef, S. B. (2018). Does renewable energy consumption and health expenditures decrease carbon dioxide emissions? Evidence for sub-Saharan Africa countries. Renew. Energy 127, 1011–1016. doi: 10.1016/j.renene.2018.05.043
Baranes, E., Jacqmin, J., and Poudou, J. (2017). Non-renewable and intermittent renewable energy sources: friends and foes? Energy Policy 111, 58–67. doi: 10.1016/j.enpol.2017.09.018
Belaid, F., and Youssef, M. (2017). Environmental degradation, renewable and non-renewable electricity consumption, and economic growth: assessing the evidence from Algeria. Energy Policy 102, 277–287. doi: 10.1016/j.enpol.2016.12.012
Black, G., Holley, D., Solan, D., and Bergloff, M. (2014). Fiscal and economic impacts of state incentives for wind energy development in the Western United States. Renew. Sust. Energy Rev. 34, 136–144. doi: 10.1016/j.rser.2014.03.015
Cadoret, I., and Padovano, F. (2016). The political drivers of renewable energies policies. Energy Econ. 56, 261–269. doi: 10.1016/j.eneco.2016.03.003
Chen, Y., Wang, Z., and Zhong, Z. (2019). CO2 emissions, economic growth, renewable and non-renewable energy production and foreign trade in China. Renew. Energy 131, 208–216. doi: 10.1016/j.renene.2018.07.047
Cortez, B., Carrera, B., Kim, Y. J., and Jung, J. (2018). An architecture for emergency event prediction using LSTM recurrent neural networks. Expert Syst. Appl. 97, 315–324. doi: 10.1016/j.eswa.2017.12.037
Craig, M. T., Carreno, I. L., Rossol, M., Hodge, B., and Brancucci, C. (2019). Effects on power system operations of potential changes in wind and solar generation potential under climate change. Environ. Res. Lett. 14:034014. doi: 10.1088/1748-9326/aaf93b
Das, P., Mathur, J., Bhakar, R., and Kanudia, A. (2018). Implications of short-term renewable energy resource intermittency in long-term power system planning. Energy Strategy Rev. 22, 1–15. doi: 10.1016/j.esr.2018.06.005
Dong, K., Sun, R., Jiang, H., and Zeng, X. (2018). CO2 emissions, economic growth, and the environmental Kuznets curve in China: what roles can nuclear energy and renewable energy play? J. Cleaner Prod. 196, 51–63. doi: 10.1016/j.jclepro.2018.05.271
Furlan, C., and Mortarino, C. (2018). Forecasting the impact of renewable energies in competition with non-renewable sources. Renew. Sust. Energy Rev. 81(Pt 2), 1879–1886. doi: 10.1016/j.rser.2017.05.284
Ghimire, S., Deo, R. C., Downs, N., and Raj, N. (2019). Global solar radiation prediction by ANN integrated with European Centre for medium range weather forecast fields in solar rich cities of Queensland Australia. J. Clean. Prod. 216, 288–310. doi: 10.1016/j.jclepro.2019.01.158
Graves, A. (2013). Generating sequences with recurrent neural networks. arXiv. Neural and Evolutionary Computing [Preprint] Avaliable at: https://arxiv.org/abs/1308.0850 (accessed June 5, 2014).
Huh, S., and Lee, C. (2014). Diffusion of renewable energy technologies in South Korea on incorporating their competitive interrelationships. Energy Policy 69, 248–257. doi: 10.1016/j.enpol.2014.02.028
Jenkins, J. D., Zhou, Z., Ponciroli, R., Vilim, R. B., Ganda, F., De Sisternes, F., et al. (2018). The benefits of nuclear flexibility in power system operations with renewable energy. Appl. Energy 222, 872–884. doi: 10.1016/j.apenergy.2018.03.002
Kok, B., and Benli, H. (2017). Energy diversity and nuclear energy for sustainable development in Turkey. Renew. Energy 111, 870–877. doi: 10.1016/j.renene.2017.05.001
Li, W., and Lu, C. (2019). The multiple effectiveness of state natural gas consumption constraint policies for achieving sustainable development targets in China. Appl. Energy 235, 685–698. doi: 10.1016/j.apenergy.2018.11.013
Liu, X., Moreno, B., and Garcia, A. S. (2016). A grey neural network and input-output combined forecasting model. Primary energy consumption forecasts in Spanish economic sectors. Energy 115(Pt 1), 1042–1054. doi: 10.1016/j.energy.2016.09.017
Lu, S. (2019). Integrating heuristic time series with modified grey forecasting for renewable energy in Taiwan. Renew. Energy 133, 1436–1444. doi: 10.1016/j.renene.2018.08.092
Mallin, M. A. (2018). From sea-level rise to seabed grabbing: the political economy of climate change in Kiribati. Mar. Policy 97, 244–252. doi: 10.1016/j.marpol.2018.04.021
Moriarty, P., and Wang, S. J. (2015). Assessing global renewable energy forecasts. Energy Proc. 75, 2523–2528. doi: 10.1016/j.egypro.2015.07.256
Moss, R. L., Tzimas, E., Kara, H., Willis, P., and Kooroshy, J. (2013). The potential risks from metals bottlenecks to the deployment of Strategic Energy Technologies. Energy Policy 55, 556–564. doi: 10.1016/j.enpol.2012.12.053
Nadimi, R., and Tokimatsu, K. (2017). Analyzing of renewable and non-renewable energy consumption via bayesian inference. Energy Proc. 142, 2773–2778. doi: 10.1016/j.egypro.2017.12.224
Narayan, S., and Doytch, N. (2017). An investigation of renewable and non-renewable energy consumption and economic growth nexus using industrial and residential energy consumption. Energy Econ. 68, 160–176. doi: 10.1016/j.eneco.2017.09.005
Nassar, N. T., Wilburn, D. R., and Goonan, T. G. (2016). Byproduct metal requirements for U.S. wind and solar photovoltaic electricity generation up to the year 2040 under various Clean Power Plan scenarios. Appl. Energy 183, 1209–1226. doi: 10.1016/j.apenergy.2016.08.062
Newbery, D. M. (2016). Towards a green energy economy? The EU Energy Union’s transition to a low-carbon zero subsidy electricity system – Lessons from the UK’s electricity market reform. Appl. Energy 179, 1321–1330. doi: 10.1016/j.apenergy.2016.01.046
Notton, G., Nivet, M. L., Voyant, C., Paoli, C., Darras, C., Motte, F., et al. (2018). Intermittent and stochastic character of renewable energy sources: consequences, cost of intermittence and benefit of forecasting. Renew. Sust. Energy Rev. 87, 96–105. doi: 10.1016/j.rser.2018.02.007
Ozcan, B., and Ozturk, I. (2019). Renewable energy consumption-economic growth nexus in emerging countries: a bootstrap panel causality test. Renew. Sust. Energy Rev. 104, 30–37. doi: 10.1016/j.rser.2019.01.020
Phadke, R. (2017). Green energy futures: responsible mining on Minnesota’s Iron Range. Energy Rese. Soc. Sci. 13, 163–173. doi: 10.1016/j.erss.2017.10.036
Reddy, B. S. (2018). Economic dynamics and technology diffusion in Indian power sector. Energy Policy 120, 425–435. doi: 10.1016/j.enpol.2018.05.044
Ruedabayona, J. G., Guzman, A., Eras, J. J., Silvacasarin, R., Bastidasarteaga, E., and Horrillocaraballo, J. M. (2019). Renewables energies in Colombia and the opportunity for the offshore wind technology. J. Clean. Prod. 220, 529–543. doi: 10.1016/j.jclepro.2019.02.174
Shammugam, S., Gervais, E., Schlegl, T., and Rathgeber, A. W. (2019). Raw metal needs and supply risks for the development of wind energy in Germany until 2050. J. Clean. Prod. 221, 738–752. doi: 10.1016/j.jclepro.2019.02.223
Sharma, A., and Kakkar, A. (2018). Forecasting daily global solar irradiance generation using machine learning. Renew. Sust. Energy Rev. 82(Pt. 3), 2254–2269. doi: 10.1016/j.rser.2017.08.066
Sivaneasan, B., Yu, C. Y., and Goh, K. P. (2017). Solar forecasting using ANN with fuzzy logic pre-processing. Energy Proc. 143, 727–732. doi: 10.1016/j.egypro.2017.12.753
Srivastava, S., and Lessmann, S. (2018). A comparative study of LSTM neural networks in forecasting day-ahead global horizontal irradiance with satellite data. Solar Energy 161, 232–247. doi: 10.1016/j.solener.2018.01.005
Stokes, L. C., and Breetz, H. L. (2018). Politics in the U.S. energy transition: case studies of solar, wind, biofuels and electric vehicles policy. Energy Policy 113, 76–86. doi: 10.1016/j.enpol.2017.10.057
Tsai, S., Xue, Y., Zhang, J., Chen, Q., Liu, Y., Zhou, J., et al. (2017). Models for forecasting growth trends in renewable energy. Renew. Sust. Energy Rev. 77, 1169–1178. doi: 10.1016/j.rser.2016.06.001
Vidal, O., Rostom, F., Francois, C., and Giraud, G. (2017). Global trends in metal consumption and supply: the raw material–energy nexus. Elements 13, 319–324. doi: 10.2138/gselements.13.5.319
Walmsley, T. G., Walmsley, M. R., Varbanov, P. S., and Klemes, J. J. (2018). Energy Ratio analysis and accounting for renewable and non-renewable electricity generation: a review. Renew. Sust. Energy Rev. 98, 328–345. doi: 10.1016/j.rser.2018.09.034
Wang, P., Chen, L., Ge, J., Cai, W., and Chen, W. (2019). Incorporating critical material cycles into metal-energy nexus of China’s 2050 renewable transition. Appl. Energy 253:113612. doi: 10.1016/j.apenergy.2019.113612
Wu, W., Ma, X., Zeng, B., Wang, Y., and Cai, W. (2019). Forecasting short-term renewable energy consumption of China using a novel fractional nonlinear grey Bernoulli model. Renew. Energy 140, 70–87. doi: 10.1016/j.renene.2019.03.006
Xie, N., Yuan, C., and Yang, Y. (2015). Forecasting China’s energy demand and self-sufficiency rate by grey forecasting model and Markov model. Int. J. Electr. Power Energy Syst. 66, 1–8. doi: 10.1016/j.ijepes.2014.10.028
Yu, R., Gao, J., Yu, M., Lu, W., Xu, T., Zhao, M., et al. (2019). LSTM-EFG for wind power forecasting based on sequential correlation features. Future Gen. Comput. Syst. 97, 33–42. doi: 10.1016/j.future.2018.09.054
Zhang, J., Yan, J., Infield, D., Liu, Y., and Lien, F. (2019). Short-term forecasting and uncertainty analysis of wind turbine power based on long short-term memory network and Gaussian mixture model. Appl. Energy 241, 229–244. doi: 10.1016/j.apenergy.2019.03.044
Zheng, Z., Chen, H., and Luo, X. (2019). Spatial granularity analysis on electricity consumption prediction using LSTM recurrent neural network. Energy Proc. 158, 2713–2718. doi: 10.1016/j.egypro.2019.02.027
Keywords: renewable energy, critical metals, sustainability, LSTM model, forecasting
Citation: Liu B, Chen J, Wang H and Wang Q (2020) Renewable Energy and Material Supply Risks: a Predictive Analysis Based on An LSTM Model. Front. Energy Res. 8:163. doi: 10.3389/fenrg.2020.00163
Received: 14 June 2020; Accepted: 26 June 2020;
Published: 23 September 2020.
Edited by:
Jianliang Wang, China University of Petroleum, ChinaReviewed by:
Guowei Hua, Beijing Jiaotong University, ChinaYue Che, East China Normal University, China
Yuan Wang, Tianjin University, China
Copyright © 2020 Liu, Chen, Wang and Wang. This is an open-access article distributed under the terms of the Creative Commons Attribution License (CC BY). The use, distribution or reproduction in other forums is permitted, provided the original author(s) and the copyright owner(s) are credited and that the original publication in this journal is cited, in accordance with accepted academic practice. No use, distribution or reproduction is permitted which does not comply with these terms.
*Correspondence: Bingchun Liu, dGp1dGxiY0AxNjMuY29t