- 1Faculty of Engineering and Physical Sciences, University of Southampton, Boldrewood Campus, Southampton, United Kingdom
- 2Department of Planning, Design, Technology of Architecture, Sapienza University of Rome, Rome, Italy
- 3Department of Architecture, University of Bologna, Cesena, Italy
The reduction of energy usage and environmental impact of the built environment and construction industry is crucial for sustainability on a global scale. We are working towards an increased commitment towards resource efficiency in the built environment and to the growth of innovative businesses following circular economy principles. The conceptualization of change is a relevant part of energy and sustainability transitions research, which is aimed at enabling radical shifts compatible with societal functions. In this framework, building performance has to be considered in a whole life cycle perspective because buildings are long-term assets. In a life cycle perspective, both operational and embodied energy and carbon emissions have to be considered for appropriate comparability and decision-making. The application of sustainability assessments of products and practices in the built environment is itself a critical and debatable issue. For this reason, the way energy consumption data are measured, processed, and reported has to be progressively standardized in order to enable transparency and consistency of methods at multiple scales (from single buildings up to building stock) and levels of analysis (from individual components up to systems), ideally complementing ongoing research initiatives that use open science principles in energy research. In this paper, we analyse the topic of linking design and operation phase’s energy performance analysis through regression-based approaches in buildings, highlighting the hierarchical nature of building energy modelling data. The goal of this research is to review the current state of the art of in order to orient future efforts towards integrated data analysis workflows, from design to operation. In this sense, we show how data analysis techniques can be used to evaluate the impact of both technical and human factors. Finally, we indicate how approximated physical interpretation of regression models can help in developing data-driven models that could enhance the possibility of learning from feedback and reconstructing building stock data at multiple levels.
Introduction
It is widely acknowledged that a lower environmental impact from the construction industry and built environment is crucial for sustainability and that this problem has to be tackled on a global scale (Berardi, 2017). Carbon emission reduction goals (i.e., decarbonisation) require pressing needs be met, such as increasing energy efficiency in end-uses, reducing demand, and providing a relevant quota of energy supply by renewable sources. Energy efficiency paradigms that consider the entire building life cycle performance (Berardi, 2018) are emerging both for new and existing buildings (e.g., Nearly Zero Energy Buildings, or NZEBs) (D’Agostino et al., 2016). At the EU level, we are working towards an increasing commitment towards resource efficiency in the built environment (Dodd et al., 2015) and the growth of innovative business opportunities following circular economy principles (McKinsey, 2014), because buildings are long-term assets. Built environment sustainability strategies can be inscribed within the more general (and rapidly growing) field of sustainability transitions research (Köhler et al., 2019). Sustainability transitions research focuses on the conceptualization of radical changes that have to be compatible with societal functions. For the construction industry, a conceptualization is proposed by Thuesen et al. (2016), identifying three generic knowledge domains: project, product, and service. Further, methods such as life cycle assessment are fundamental for the development of innovative economic paradigms, such as Circular Economy, in the built environment (Pomponi and Moncaster, 2017), but the assessment of the sustainability of products and practices through life cycle assessment is itself a critical issue. In fact, there could be a large variability in the way this method is currently used in practice (Pomponi and Moncaster, 2018), creating difficulties in terms of transparent comparability of results and performance benchmarking. From a life cycle perspective, the environmental impact of buildings should account for both embodied (products and construction practices) and operational energy consumptions. Both the measurement of embodied energy and carbon equivalent of buildings (De Wolf et al., 2017) and operational energy consumption (de Wilde, 2017; Imam et al., 2017) represent critical (and debatable) issues that can ultimately determine a relevant “performance gap.” Finally, energy transition strategies have to address complementarities (Markard and Hoffmann, 2016) which are crucial for the co-evolution of built environment and energy infrastructures (Junker et al., 2018; Dominković et al., 2020). In this framework, empirically grounded and tested methods that can help in standardizing the way energy consumption data are measured, processed, and reported are particularly valuable, because they can provide reliable evidence, inform policies, and support decision-making processes adequately. In the next sections, some introductory examples in this sense will be given, indicating the motivation for the review work.
Background and Motivation
The energy modelling research community at present is emphasizing the fundamental importance of open energy data and models (Pfenninger et al., 2017; Pfenninger et al., 2018), and we can envision an evolution towards systems of models (Bollinger et al., 2018) created to tackle fundamental problems in energy transitions, eventually exploiting soft-linking approaches (Deane et al., 2012; Dominković et al., 2020). The way energy consumption data are measured, processed, and reported can be standardized in order to enable transparency and consistency of methods at multiple scales and levels of analysis, as well as to complement initiatives applying open science principles in energy research (Openmod; Hilpert et al., 2018). Some research projects in this direction have been developed in recent years (CalTRACK; Jayaweera et al., 2013; Miller and Meggers, 2017; Firth et al., 2018).
The methods proposed in these projects are empirically grounded and could be useful in providing evidence regarding innovative practices, services, and technologies. As an example, providing robust and empirically tested methods is fundamental if we think about issues such as “re-bound” (Herring and Roy, 2007) and “pre-bound” effects related to energy efficiency practices (Sunikka-Blank and Galvin, 2012; Rosenow and Galvin, 2013), that are determining a substantial difference between simulated and measured performance.
Further, the methods proposed are data-driven, using surrogate models, i.e., meta-models or reduced-order models (Manfren et al., 2013; Wei et al., 2018; Westermann and Evins, 2019). Surrogate models are approximations of detailed simulation models that enable efficient computation (within a given accuracy and temporal constraints)in, for example, design optimization (Evins, 2013; Nguyen et al., 2014; Westermann and Evins, 2019) and calibration (Coakley et al., 2014) and control (Aste et al., 2017; Serale et al., 2018).
Further, surrogate models are flexible and can be used to link design and operation phase performance analysis (Allard et al., 2018; Tronchin et al., 2018a) in an integrated workflow (Manfren and Nastasi, 2020). The choice of a specific surrogate modelling technique depends on multiple factors (Koulamas et al., 2018; Østergård et al., 2018). In this review work, we focus on regression-based approaches that can be used from design to operation phase energy analysis. The basic reasons for this choice are its conceptual simplicity, availability of technical standardization (ISO, 2013; ASHRAE, 2014), availability of technical guidelines and protocols for field applications (EVO, 2003; FEMP, 2008), and availability of open software (CalTRACK; Paulus, 2017). Other fundamental aspects, that will be discussed later in more detail, include the scalability of building stock analysis (Meng et al., 2020), the affinity with variable-base degree days methods (Kohler et al., 2016; Meng and Mourshed, 2017), and the possibility to perform analysis at a utility scale (Acquaviva et al., 2015) or a city scale (Qomi et al., 2016), including the assessment of the impact of users’ behaviour (Oh et al., 2020). Finally, the possibility to analyse thermal, electrical, and fuel demands with these methods opens up new possibilities in terms of model soft-linking, for example, by using regression-based approaches to create energy demand scenarios (e.g., considering technological evolution, climate change, behavioural change, etc.) in multi-commodity systems models (Adhikari et al., 2012a; Manfren, 2012; Kraning et al., 2014; Dorfner, 2016; Nastasi, 2019), thereby supplementing open science oriented approaches in energy research (Hilpert et al., 2018).
Linking Design and Operational Energy Performance Analysis at Scale
Building performance can be studied by means of Key Performance Indicators (KPIs) (Ghaffarianhoseini et al., 2016; Kylili et al., 2016; Yoshino et al., 2017; Talele et al., 2018) that aggregate a larger set of data in a single representative quantity. In energy transition strategies for the built environment, the efficiency dimension plays a key role (Abu Bakar et al., 2015). From a techno-economic perspective (Fabbri et al., 2011; Aste et al., 2013; Corgnati et al., 2013; Tronchin et al., 2014) the analysis is generally concentrated on the trade-off between performance indicators, such as primary energy demand and investment and operation costs (Ferrara et al., 2018), following definitions from technical standardization (ISO, 2017). However, in order to enable an effective performance assessment, additional indicators have to be considered regarding IEQ (Fabbri and Tronchin, 2015; Manfren et al., 2019), RES on-site generation and self-consumption (Kurnitski, 2013), load matching, and grid interaction (Voss et al., 2010; Frontini et al., 2012). Further, indicators are essential to address innovative solutions (Mancini and Nastasi, 2019) and operation strategies for buildings aimed at flexibility (Clauß et al., 2017) and relevant problems, such as soft-linking simulation models with energy planning tools (Noussan and Nastasi, 2018; Dominković et al., 2020). Indeed, the scalability of analysis methods and tools up to district (Adhikari et al., 2012b), city (Cipriano et al., 2017), and regional scales (Aste et al., 2014; Kuster et al., 2019) is fundamental to ensure the credibility of policies and practices.
For all these reasons, KPIs are essential to address building performance problems in a wider sense (de Wilde, 2018), especially when the goal is understanding performance uncertainty and variability. These issues can be addressed, from a computational perspective, by means of a parametric and probabilistic analysis, used as an exploratory tool and for optimization purposes (Tronchin et al., 2016; Østergård et al., 2020). The importance of accounting for multiple performance scenarios (Shiel et al., 2018), considering the impact of both technical and human factors (Yoshino et al., 2017), is becoming evident, and the Design Of Experiments technique is being used in many cases for building energy performance simulations (Jaffal et al., 2009; Kotireddy et al., 2018; Schlueter and Geyer, 2018; Tronchin et al., 2018a). With respect to human factors influencing performance, generally occupants’ comfort preferences and behaviours (Menezes et al., 2012; Tagliabue et al., 2016; Gaetani et al., 2018) are overlooked, even though they can create a relevant gap between simulated and measured performance. This fact can clearly undermine the effectiveness of policies that have to propose techno-economically feasible solutions and, at the same time, consider human behaviour realistically (Herring and Roy, 2007; Rosenow and Galvin, 2013).
In conclusion, understanding the variability of building energy performance outcomes, both in design phase simulation and in actual operation, requires the definition of appropriate KPIs and of parametric/probabilistic analysis strategies, involving multiple input-output combinations. These analyses can be performed by means of reduced order models, following the argumentation reported in Introduction and Background and Motivation regarding the necessity to address multiple problems and the creating methods that are computationally efficient; in the subsequent sections, we will discuss data and modelling strategies in this direction. In Hierarchical Structure of Building Energy Modelling Data, we analyse the hierarchical structure of building performance data, while in Regression Models in Design Phase Analysis and Regression Models in Operational Phase Analysis we propose examples of regression models for design phase and operational phase analysis.
Hierarchical Structure of Building Energy Modelling Data
Building energy modelling data can be organized using a hierarchical structure to improve the level of transparency in modelling and the possibility to achieve reliable results. Examples of hierarchical structures in building energy modelling data can be found in EU legislation regarding the definition of cost-optimal levels of performance (European Commission, 2012) and in the EU Building Stock Observatory web portal (Arcipowska et al., 2016). In the United States, technical standardization was tested with the definition of reference building models (Deru et al., 2011; Goel et al., 2014), considering also costs of different technological options (Thornton et al., 2011). Further, the use of hierarchical structures in datasets for building energy modelling can be found, for example, in studies about performance gaps (Imam et al., 2017), automation systems’ efficiency (Aste et al., 2017), and occupancy modelling (Gaetani et al., 2016). Additionally, we can find examples of hierarchical data in multi-level calibration frameworks (Yang and Becerik-Gerber, 2015) and in other cases where macro-parameters (Calleja Rodríguez et al., 2013) are used to facilitate and guide the uncertainty and sensitivity analysis, or where they are used to support the definition of archetypes (Korolija et al., 2013). In turn, the definition of archetypes is essential for reference building analysis (statistically representative buildings) and for parametric studies, where an appropriate level of simplification is crucial to enable a correct analysis process. Examples in this sense can be found for the simulation of simplified building models (Pernigotto et al., 2014), for city scale modelling (Delmastro et al., 2016; Dogan et al., 2016; Dogan and Reinhart, 2017; Ghiassi and Mahdavi, 2017), and for building stock modelling (Ballarini and Corrado, 2017). An explicative example of the hierarchical structure of data in building energy modelling is reported in Table 1. The table is by no means exhaustive, but aims to outline a way to organize building energy modelling data in a transparent and possibly reproducible way, using current technical standardization and validated simulation software as a reference.
Alternatively, the hierarchy of building energy modelling data can also be conceived for the “vertical integration” of information, from the user level up to energy infrastructures, with a subdivision in levels such as meter, building, building zones, individual rooms, individual spaces within the room, and user. Examples of research in this direction can be found in international research initiatives on “Energy Flexibility in Buildings” (IEA, 2018a) and “Occupant-Centric Building Design and Operation” (IEA, 2018b). Further research developments in this direction appear particularly promising for Internet of Things applications (Breiner et al., 2016; Reka and Dragicevic, 2018).
Regression Models in Design Phase Analysis
In Hierarchical Structure of Building Energy Modelling Data, the multi-level nature of building data was illustrated using examples from the current state of the art. Following the argumentation reported at the beginning of Linking Design and Operational Energy Performance Analysis at Scale, we can understand how linking design and operation phase performance analysis using standardized and consistent methods is crucial to enable integrated data analysis workflows, from design to operation. In this section, we illustrate some examples of regression models used in design phase’s performance analysis, which makes use of datasets that partially overlap with the ones reported in Table 1. First of all, there are many examples of applications of regression models for early stage design evaluation (Catalina et al., 2008; Hygh et al., 2012; Asadi et al., 2014; Al Gharably et al., 2016; Ipbüker et al., 2016), also using a Design of Experiments approach (Jaffal et al., 2009) to create multiple input combinations in a rational way. Further, we can find examples of application of energy signatures analysis from design to operation (Allard et al., 2018; Tronchin et al., 2018a), leading to a continuity in the data analysis workflow. Additionally, we can find examples of this type of model for techno-economic analysis (using Life Cycle Cost as a KPI) based on optimization techniques (Aparicio-Ruiz et al., 2019), and Data Envelopment Analysis (Kavousian and Rajagopal, 2013). Finally, we can find an example of regression models used for Energy Performance Contracting (EPC) to control operation costs using models trained on parametric simulations (Ligier et al., 2017), thereby creating a continuity between design and operational phase analysis. A summary of the topics and sub-topics emerging from the review of regression-based approaches for design phase analysis is reported in Table 2.
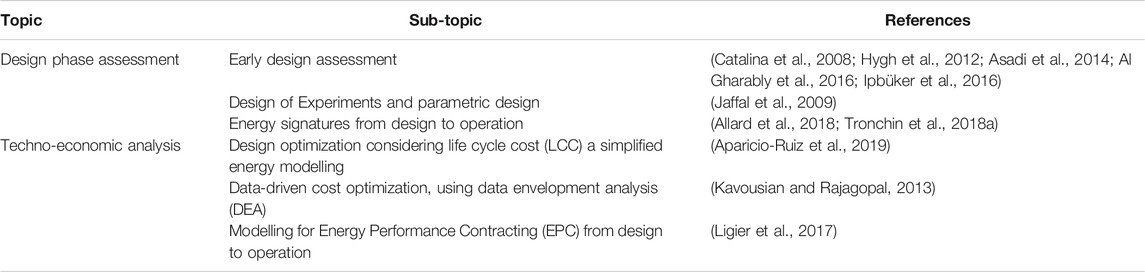
TABLE 2. Regression-based approaches for design phase performance assessment and techno-economic analysis.
While many of the research works reported above focus on design phase analysis and are, therefore, based on models trained on simulation data, the applicability of these models has also been shown for the analysis of energy performance databases (Walter and Sohn, 2016), considering the actual energy consumption data collected from surveys.
Regression Models in Operational Phase Analysis
As anticipated, regression modelling approaches have been chosen because of their standardization (ISO, 2013; ASHRAE, 2014) and the fact that they are adopted in (M&V) protocols (EVO, 2003; FEMP, 2008), where specific thresholds (expressed as statistical KPIs) are given for the acceptability of models (Fabrizio and Monetti, 2015). Therefore, they represent empirically grounded and tested methods that can be successfully used to provide and document evidence of results of energy efficiency measures (Mathieu et al., 2011; Jayaweera et al., 2013), providing results that are normalized with respect to weather and operational conditions (Price, 2010). They are based on energy interval data (dependent variable) and weather data (independent variables), together with other independent variables that may be derived from contextual information. External air temperature is the most important independent variable, used for weather normalization of energy consumption (Masuda and Claridge, 2014; Lin and Claridge, 2015; Westermann et al., 2020). Additionally, rather than using energy data directly, we can transform them to derive the average power, called energy signature (ISO, 2013), over the amount of operating hours in the time interval considered.
Scalability constitutes one of the essential pre-requisites for the applicability of these methods at scale and we consider in this section both temporal and spatial scalability. On the one hand, with respect to temporal scalability, regression-based approaches can be used with monthly, daily, and hourly energy interval data and weather data. Monthly data are the most easily accessible (e.g., utility bills or periodic meter readings) and they can used for multiple purposes, such as targeting energy savings from single buildings up to utility scale and establishing priorities (Hallinan et al., 2011b) and for recommissioning and refurbishment interventions (Hallinan et al., 2011a). Further, they can be used to estimate energy savings in industrial buildings (Server et al., 2011) and to perform the disaggregation of weather-dependent and production-dependent energy consumption in industrial facilities (Abels et al., 2011). Finally, they can be used as a basis to measure energy efficiency progress with Normalized Energy Intensity (Lammers et al., 2011), using a methodology compatible with energy management systems standardization (ISO, 2018). Regression with daily data extends these capabilities further (Masuda and Claridge, 2014; Lin and Claridge, 2015), giving the possibility to include an autoregressive term in the model formulation to improve goodness of fit (Masuda and Claridge, 2012b; Danov et al., 2013). Finally, hourly models represent an even more detailed formulation (Jalori and Reddy, 2015b; Abushakra and Paulus, 2016), which can be used effectively to understand dynamic patterns of energy demand to, for example, optimize operation interval and control strategies, considering issues such as dynamic energy tariffs and interactions with the grid and on-site generation.
On the other hand, in terms of spatial scalability, we can see how regression-based approaches can be used to model the performance of construction technologies, considering building fabric heat transfer (Bauwens and Roels, 2014; Erkoreka et al., 2016; Giraldo-Soto et al., 2018; Uriarte et al., 2019), or whole building energy behaviour (Masuda and Claridge, 2014; Lin and Claridge, 2015; Paulus et al., 2015). Going beyond single buildings, we can find examples of applications regarding building stock (Meng and Mourshed, 2017; Meng et al., 2020) and community and city scale systems (Qomi et al., 2016; Pasichnyi et al., 2019), considering also complex interactions with the urban environment and physical-statistical interpretation of models (Afshari et al., 2017). A summary of the topics and sub-topics emerging from the review of regression-based approaches for operation phase analysis is reported in Table 3.
As shown above, regression-based approaches are temporally and spatially scalable and can be employed for different types of end-uses and for different aggregations of users, making them suitable for analytics aimed at energy retrofit (Pistore et al., 2019) and decarbonisation (Tronchin et al., 2019), considering performance variability due to realistic operational patterns (Tagliabue et al., 2016; Oh et al., 2020) or the impact of properties of construction components such as thermal inertia (Aste et al., 2015).
We already mentioned conceptual simplicity as one of the advantages of this type of model compared to other meta-modelling techniques (Manfren et al., 2013). Additionally, given the standard structure of the basic models, automated or partially automated model selection techniques (Paulus et al., 2015; Paulus, 2017) can be applied to compare the performance of multiple modelling options. Clearly, the presence of different operating conditions in time (e.g., different types of operational profiles) may determine the need to cluster operational conditions on a daily basis (Jalori and Reddy, 2015a; Miller et al., 2015; Richard et al., 2017). The need for integration of clustering and regression in data analysis workflow is also indicated in recent literature reviews regarding energy transition strategies for the built environment (Tronchin et al., 2018b).
Further Research
In the previous sections we illustrated how a regression-based approach can be used to analyse performance from design to operation phase in the building life cycle using standardized methods that are conceptually simple, scalable (temporally and spatially), and easily interpretable. Future research efforts should be oriented, first of all, to the exploitation of the approximated physical interpretation of regression model coefficients (Masuda and Claridge, 2012a; Bauwens and Roels, 2014; Tronchin et al., 2016, Tronchin et al., 2019). Indeed, this interpretation depends on the formulation of an approximated physical model, which can be created, for example, according to current technical standardization (ISO, 2017). This, in turn, could enable a harmonized definition of quantities and methods at multiple levels (Manfren and Nastasi, 2019). Other relevant issues to be considered are Monte Carlo simulation methods to test the robustness of models’ estimates with respect to variable operational conditions (Cecconi et al., 2017) and Bayesian analysis as an extension of conventional regression paradigms (Li et al., 2016). In particular, Bayesian analysis can be used to reconstruct built environment data (Booth et al., 2013; Zhao et al., 2016; Lim and Zhai, 2017), considering the hierarchical data structure outlined in Hierarchical Structure of Building Energy Modelling Data. Further, regression-based approaches could become suitable for projections about energy consumption in future climate change scenarios (Jentsch et al., 2008; Jentsch et al., 2013; Bravo Dias et al., 2020) and to create load profiles when designing decentralized energy systems from buildings (Stadler et al., 2018) up to community scales (Adhikari et al., 2012a; Orehounig et al., 2014; Orehounig et al., 2015), also using clustering techniques to identify typical (recurrent) operational conditions.
In terms of technologies, regression-based approaches can complement the analysis of performance of technologies such as heat pumps and cooling machines (Busato et al., 2012; Busato et al., 2013), considering also exergy balance (Tronchin and Fabbri, 2008; Meggers et al., 2012), where temperature dependence is fundamental.
In conclusion, standardized and harmonized regression-based approaches can be used to complement recent advances in research regarding end-use energy demand based on epidemiology concepts (Hamilton et al., 2013; Hamilton et al., 2017), providing suitable evidence (Jack et al., 2018; Lomas et al., 2018) aimed at informing decision-making processes and future policies by means of robust and empirically grounded methods.
Conclusion
Understanding and conceptualizing innovation processes is crucial to respond to global sustainability issues for the built environment. Research has to be able to address the whole building life cycle, assessing the sustainability of products and practices transparently and consistently. The correct assessment of operational and embodied energy and carbon emission depends critically on the way energy data are measured, processed, and reported. The methods to perform energy-related data analysis workflows during building life cycle have to be progressively standardized and harmonized in order to enable transparency and consistency at multiple scales, from single components up to city scale and building stocks, and levels of analysis, from individual components up to systems. In other words, the evolution of methods should be aimed at creating continuity between energy performance analysis across life cycle phases (e.g., by means of data-driven model based analysis and linked open data standards), using parametric simulations in design phases and progressively calibrating building models to measured data. In this way, parametric data generated in the design phase can be analysed to detect the most relevant factors influencing performance and potentially critical assumptions, while (multi-level) model calibration can help in deriving insights on the actual performance and show transparently any potential misalignment between simulation assumptions and measured values. On the one hand, the possibility to learn constantly from measured performance could help in providing robust evidence of the impact of innovative products and practices. On the other hand, the possibility to exploit an approximated physical interpretation of regression model structure could greatly enhance the interpretability and explainability of data-driven methods, learning from feedback to enhance the performance of both single technologies and systems. The goal of this research was mapping the ongoing research in these areas, with a focus on regression-based approaches that could be used to create scalable (temporally and spatially) integrated data analysis workflows from design to operation in buildings. In this sense, we showed how data analysis techniques could be used to evaluate the impact of both technical and human factors, with the aim of reconstructing building stock data at multiple levels. In turn, these data could be used for the development of next-generation products and services in the built environment, following a continuous improvement approach, which is already recommended (and standardized) in energy management practices at the state of the art. In particular, these methods will be crucial for the further development of innovative building design paradigms (e.g., NZEBs) and will be necessary for the development of innovative energy services (e.g., exploiting energy flexibility and considering user behaviour) and technologies (e.g., energy management and automation systems). Finally, energy transition strategies have to address “complementarities” for the co-evolution of built environment and energy infrastructures, and the possibility to create an improved “soft-linking” among open energy modelling tools (and data) is particularly valuable, because they can provide reliable evidence, inform policies, and support decision-making processes.
Author Contributions
MM: Conceptualization, Design, Methodology, Writing. BN: Review, Writing. LT: Writing.
Conflict of Interest
The authors declare that the research was conducted in the absence of any commercial or financial relationships that could be construed as a potential conflict of interest.
Acknowledgments
This work was part of the activities carried out within the project n.201594LT3F, funded by PRIN (Programmi di Ricerca Scientifica di Rilevante Interesse Nazionale) of the Italian Ministry of Education, University and Research and the project “SIPARIO - Il Suono: arte Intangibile delle Performing Arts – Ricerca su teatri italiani per l'Opera POR–FESR 2014–20” , n. PG/2018/632038, funded by the Regione Emilia Romagna under EU Commission.
References
Abels, B., Sever, F., Kissock, K., and Ayele, D. (2011). Understanding industrial energy use through lean energy analysis. SAE Int. J. Mater. Manuf. 4, 495–504. doi:10.4271/2011-01-0326
Abu Bakar, N. N., Hassan, M. Y., Abdullah, H., Rahman, H. A., Abdullah, M. P., Hussin, F., et al. (2015). Energy efficiency index as an indicator for measuring building energy performance: a review. Renew. Sustain. Energy Rev. 44, 1–11. doi:10.1016/j.rser.2014.12.018
Abushakra, B., and Paulus, M. T. (2016). An hourly hybrid multi-variate change-point inverse model using short-term monitored data for annual prediction of building energy performance, part III: results and analysis (1404-RP). Sci. Technol. Built Environ. 22, 984–995. doi:10.1080/23744731.2016.1215659
Acquaviva, A., Apiletti, D., Attanasio, A., Baralis, E., Bottaccioli, L., Castagnetti, F. B., et al. (2015). “Energy signature analysis: knowledge at your fingertips,” in Big data (BigData congress), 2015 IEEE international congress on. Piscataway, NJ (IEEE), 543–550.
Adhikari, R. S., Aste, N., and Manfren, M. (2012a). Multi-commodity network flow models for dynamic energy management – smart Grid applications. Energy Procedia. 14, 1374–1379. doi:10.1016/j.egypro.2011.12.1104
Adhikari, R. S., Aste, N., and Manfren, M. (2012b). Optimization concepts in district energy design and management – a case study. Energy Procedia. 14, 1386–1391. doi:10.1016/j.egypro.2011.12.1106
Afshari, A., Friedrich, L. A., Liu, N., and Friedrich, L. A. (2017). Inverse modeling of the urban energy system using hourly electricity demand and weather measurements, Part 1: black-box model. Energy Build. 157, 126–138. doi:10.1016/j.enbuild.2017.01.053
Al Gharably, M., DeCarolis, J. F., and Ranjithan, S. R. (2016). An enhanced linear regression-based building energy model (LRBEM+) for early design. J. Build. Perform. Simul. 9, 115–133. doi:10.1080/19401493.2015.1004108
Allard, I., Olofsson, T., and Nair, G. (2018). Energy evaluation of residential buildings: performance gap analysis incorporating uncertainties in the evaluation methods. Build. Simul. 11, 725–737. doi:10.1007/S12273-018-0439-7
Aparicio-Ruiz, P., Guadix-Martín, J., Barbadilla-Martín, E., and Muñuzuri-Sanz, J. (2019). Applying renewable energy technologies in an integrated optimization method for residential building’s design. Appl. Sci. 9, 453. doi:10.3390/app9030453
Arcipowska, A., Rapf, O., Faber, M., Fabbri, M., Tigchelaar, C., Boermans, T., et al. (2016). Support for setting up an observatory of the building stock and related policies. Brussels, Belgium: European Commission.
Asadi, S., Amiri, S. S., and Mottahedi, M. (2014). On the development of multi-linear regression analysis to assess energy consumption in the early stages of building design. Energy Build. 85, 246–255. doi:10.1016/j.enbuild.2014.07.096
ASHRAE (2014). ASHRAE guideline 14-2014: measurement of energy, demand, and water savings, Atlanta, GA: American society of heating, refrigerating and air-conditioning engineers.
Aste, N., Adhikari, R. S., and Manfren, M. (2013). Cost optimal analysis of heat pump technology adoption in residential reference buildings. Renew. Energy. 60, 615–624. doi:10.1016/j.renene.2013.06.013
Aste, N., Buzzetti, M., Caputo, P., and Manfren, M. (2014). Local energy efficiency programs: a monitoring methodology for heating systems. Sustain. Cities Soc. 13, 69–77. doi:10.1016/j.scs.2014.04.006
Aste, N., Leonforte, F., Manfren, M., and Mazzon, M. (2015). Thermal inertia and energy efficiency – parametric simulation assessment on a calibrated case study. Appl. Energy. 145, 111–123. doi:10.1016/j.apenergy.2015.01.084
Aste, N., Manfren, M., and Marenzi, G. (2017). Building Automation and Control Systems and performance optimization: a framework for analysis. Renew. Sustain. Energy Rev. 75, 313–330. doi:10.1016/j.rser.2016.10.072
Ballarini, I., and Corrado, V. (2017). A new methodology for assessing the energy consumption of building stocks. Energies 10, 1102. doi:10.3390/en10081102
Bauwens, G., and Roels, S. (2014). Co-heating test: a state-of-the-art. Energy Build. 82, 163–172. doi:10.1016/j.enbuild.2014.04.039
Berardi, U. (2017). A cross-country comparison of the building energy consumptions and their trends. Resour. Conserv. Recycl. 123, 230–241. doi:10.1016/j.resconrec.2016.03.014
Berardi, U. (2018). “ZEB and nZEB (definitions, design methodologies, good practices, and case studies),” in Handbook of energy efficiency in buildings. A life cycle approach. Amsterdam, Netherlands: Elsevier Science.
Bollinger, L. A., Davis, C. B., Evins, R., Chappin, E. J. L., and Nikolic, I. (2018). Multi-model ecologies for shaping future energy systems: design patterns and development paths. Renew. Sustain. Energy Rev. 82, 3441–3451. doi:10.1016/j.rser.2017.10.047
Booth, A. T., Choudhary, R., and Spiegelhalter, D. J. (2013). A hierarchical Bayesian framework for calibrating micro-level models with macro-level data. J. Build. Perform. Simul. 6, 293–318. doi:10.1080/19401493.2012.723750
Bravo Dias, J., Carrilho da Graça, G., and Soares, P. M. M. (2020). Comparison of methodologies for generation of future weather data for building thermal energy simulation. Energy Build. 206, 109556. doi:10.1016/j.enbuild.2019.109556
Breiner, S., Subrahmanian, E., and Sriram, R. D. (2016). “Modeling the Internet of Things: a foundational approach,” in Proceedings of the seventh international workshop on the web of things. New York, NY (ACM), 38–41.
Busato, F., Lazzarin, R. M., and Noro, M. (2012). Energy and economic analysis of different heat pump systems for space heating. Int. J. Low-Carbon Technol. 7, 104–112. doi:10.1093/ijlct/cts016
Busato, F., Lazzarin, R. M., and Noro, M. (2013). Two years of recorded data for a multisource heat pump system: a performance analysis. Appl. Therm. Eng. 57, 39–47. doi:10.1016/j.applthermaleng.2013.03.053
Calleja Rodríguez, G., Carrillo Andrés, A., Domínguez Muñoz, F., Cejudo López, J. M., and Zhang, Y. (2013). Uncertainties and sensitivity analysis in building energy simulation using macroparameters. Energy Build. 67, 79–87. doi:10.1016/j.enbuild.2013.08.009
CalTRACK. CalTRACK methods. Available at: (http://docs.caltrack.org/en/latest/methods.html). (Accessed April 30, 2020)
Catalina, T., Virgone, J., and Blanco, E. (2008). Development and validation of regression models to predict monthly heating demand for residential buildings. Energy Build. 40, 1825–1832. doi:10.1016/j.enbuild.2008.04.001
Cecconi, F. R., Manfren, M., Tagliabue, L. C., Ciribini, A. L. C., and De Angelis, E. (2017). Probabilistic behavioral modeling in building performance simulation: a Monte Carlo approach. Energy Build. 148, 128–141. doi:10.1016/j.enbuild.2017.05.013
Cipriano, X., Gamboa, G., Danov, S., Mor, G., and Cipriano, J. (2017). Developing indicators to improve energy action plans in municipalities: an accounting framework based on the fund-flow model. Sustain. Cities Soc. 32, 263–276. doi:10.1016/j.scs.2017.03.004
Clauß, J., Finck, C., Vogler-Finck, P., and Beagon, P. (2017). “Control strategies for building energy systems to unlock demand side flexibility--A review,” in 12th international conference of IBPSA building simulation 2017, San Francisco, CA, August 7–9, 2017.
Coakley, D., Raftery, P., and Keane, M. (2014). A review of methods to match building energy simulation models to measured data. Renew. Sustain. Energy Rev. 37, 123–141. doi:10.1016/j.rser.2014.05.007
Corgnati, S. P., Fabrizio, E., Filippi, M., and Monetti, V. (2013). Reference buildings for cost optimal analysis: method of definition and application. Appl. Energy. 102, 983–993. doi:10.1016/j.apenergy.2012.06.001
D’Agostino, D., Zangheri, P., Cuniberti, B., Paci, D., and Bertoldi, P. (2016). Synthesis report on the national plans for nearly Zero energy buildings (NZEBs). Ispra, Italy: JRC EU Commission.
Danov, S., Carbonell, J., Cipriano, J., and Martí-Herrero, J. (2013). Approaches to evaluate building energy performance from daily consumption data considering dynamic and solar gain effects. Energy Build. 57, 110–118. doi:10.1016/j.enbuild.2012.10.050
de Wilde, P. (2017). The building performance gap: are modellers literate?. Build. Serv. Eng. Res. Technol. 38, 757–759. doi:10.1177/0143624417728431
de Wilde, P. (2018). Building performance analysis. Hoboken, NJ: Wiley Available at: https://onlinelibrary.wiley.com/doi/book/10.1002/9781119341901.
De Wolf, C., Pomponi, F., and Moncaster, A. (2017). Measuring embodied carbon dioxide equivalent of buildings: a review and critique of current industry practice. Energy Build. 140, 68–80. doi:10.1016/j.enbuild.2017.01.075
Deane, J. P., Chiodi, A., Gargiulo, M., and Gallachóir, B. P. Ó. (2012). Soft-linking of a power systems model to an energy systems model. Energy 42, 303–312. doi:10.1016/j.energy.2012.03.052
Delmastro, C., Mutani, G., and Corgnati, S. P. (2016). A supporting method for selecting cost-optimal energy retrofit policies for residential buildings at the urban scale. Energy Pol. 99, 42–56. doi:10.1016/j.enpol.2016.09.051 | .
Deru, M., Field, K., Studer, D., Benne, K., Griffith, B., Torcellini, P., et al. (2011). US Department of Energy commercial reference building models of the national building stock. Golden, CO: National Renewable Energy Laboratory.
Dodd, N., Donatello, S., Garbarino, E., and Gama-Caldas, M. (2015). Identifying macro-objectives for the life cycle environmental performance and resource efficiency of EU buildings. Ispra, Italy: JRC EU Commission.
Dogan, T., and Reinhart, C. (2017). Shoeboxer: an algorithm for abstracted rapid multi-zone urban building energy model generation and simulation. Energy Build. 140, 140–153. doi:10.1016/j.enbuild.2017.01.030
Dogan, T., Reinhart, C., and Michalatos, P. (2016). Autozoner: an algorithm for automatic thermal zoning of buildings with unknown interior space definitions. J. Build. Perform. Simul. 9, 176–189. doi:10.1080/19401493.2015.1006527
Dominković, D. F., Junker, R. G., Lindberg, K. B., and Madsen, H. (2020). Implementing flexibility into energy planning models: soft-linking of a high-level energy planning model and a short-term operational model. Appl. Energy. 260, 114292. doi:10.1016/j.apenergy.2019.114292
Dorfner, J. (2016). Open source modelling and optimisation of energy infrastructure at urban scale. PhD thesis. Munich, Germany: Technical University of Munich
Erkoreka, A., Garcia, E., Martin, K., Teres-Zubiaga, J., and Del Portillo, L. (2016). In-use office building energy characterization through basic monitoring and modelling. Energy Build.. 119, 256–266. doi:10.1016/j.enbuild.2016.03.030
European Commission (2012). Commission delegated regulation (EU) No 244/2012 of 16 January 2012. Brussels, Belgium: European Commission Available at: https://eurlex.europa.eu/LexUriServ/LexUriServ.do?uri=OJ:L:2012:081:0018:0036:EN:PDF (Accessed April 20, 2020)
Evins, R. (2013). A review of computational optimisation methods applied to sustainable building design. Renew. Sustain. Energy Rev. 22, 230–245. doi:10.1016/j.rser.2013.02.004
EVO (2003). “IPMVP new construction subcommittee,” in International performance measurement and verification protocol: concepts and option for determining energy savings in new construction. Washington, DC: Efficiency Valuation Organization (EVO), Vol. 3.
Fabbri, K., and Tronchin, L. (2015). Indoor environmental quality in low energy buildings. Energy Procedia. 78, 2778–2783. doi:10.1016/j.egypro.2015.11.625
Fabbri, K., Tronchin, L., and Tarabusi, V. (2011). Real Estate market, energy rating and cost. Reflections about an Italian case study. Procedia Eng. 21, 303–310. doi:10.1016/j.proeng.2011.11.2019
Fabrizio, E., and Monetti, V. (2015). Methodologies and advancements in the calibration of building energy models. Energies 8, 2548–2574. doi:10.3390/en8042548
FEMP (2008). Federal energy management program, M&V guidelines: measurement and verification for federal energy projects version 3.0. Washington, DC: U.S. Department of Energy Federal Energy Management Program.
Ferrara, M., Monetti, V., and Fabrizio, E. (2018). Cost-optimal analysis for nearly zero energy buildings design and optimization: a critical review. Energies 11, 1478. doi:10.3390/en11061478
Firth, S. K., Cole, G., Kane, T., Fouchal, F., and Hassan, T. M. (2018). An open science approach for building performance studies. Montréal, QC, Canada, May 9--10, 2018. Available at: http://www.ibpsa.org/proceedings/eSimPapers/2018/2-3-A-2.pdf
Frontini, F., Manfren, M., and Tagliabue, L. C. (2012). A case study of solar technologies adoption: criteria for BIPV integration in sensitive built environment. Energy Procedia. 30, 1006–1015. doi:10.1016/j.egypro.2012.11.113
Gaetani, I., Hoes, P.-J., and Hensen, J. L. M. (2016). Occupant behavior in building energy simulation: towards a fit-for-purpose modeling strategy. Energy Build. 121, 188–204. doi:10.1016/j.enbuild.2016.03.038
Gaetani, I., Hoes, P.-J., and Hensen, J. L. M. (2018). Estimating the influence of occupant behavior on building heating and cooling energy in one simulation run. Appl. Energy. 223, 159–171. doi:10.1016/j.apenergy.2018.03.108
Ghaffarianhoseini, A., Berardi, U., AlWaer, H., Chang, S., Halawa, E., Ghaffarianhoseini, A., et al. (2016). What is an intelligent building? Analysis of recent interpretations from an international perspective. Archit. Sci. Rev. 59, 338–357. doi:10.1080/00038628.2015.1079164
Ghiassi, N., and Mahdavi, A. (2017). Reductive bottom-up urban energy computing supported by multivariate cluster analysis. Energy Build. 144, 372–386. doi:10.1016/j.enbuild.2017.03.004
Giraldo-Soto, C., Erkoreka, A., Mora, L., Uriarte, I., and Del Portillo, L. A. (2018). Monitoring system Analysis for evaluating a building’s envelope energy performance through estimation of its heat loss coefficient. Sensors 18, 2360. doi:10.3390/s18072360
Goel, S., Athalye, R. A., Wang, W., Zhang, J., Rosenberg, M. I., Xie, Y., et al. (2014). Enhancements to ASHRAE standard 90.1 prototype building models. Technical Report PNNL-23269, BT0400000. doi:10.2172/1129366
Hallinan, K. P., Brodrick, P., Northridge, J., Kissock, J. K., and Brecha, R. J. (2011a). Establishing building recommissioning priorities and potential energy savings from utility energy data. Atlanta, GA: American Society of Heating, Refrigerating, and Air-Conditioning Engineers, (ASHRAE)
Hallinan, K. P., Kissock, J. K., Brecha, R. J., and Mitchell, A. (2011b). Targeting residential energy reduction for city utilities using historical electrical utility data and readily available building data. Atlanta, GA: American Society of Heating, Refrigerating, and Air-Conditioning Engineers, (ASHRAE)
Hamilton, I., Summerfield, A., Oreszczyn, T., and Ruyssevelt, P. (2017). Using epidemiological methods in energy and buildings research to achieve carbon emission targets. Energy Build.. 154, 188–197. doi:10.1016/j.enbuild.2017.08.079
Hamilton, I. G., Summerfield, A. J., Lowe, R., Ruyssevelt, P., Elwell, C. A., and Oreszczyn, T. (2013). Energy epidemiology: a new approach to end-use energy demand research. Build. Res. Inf. 41, 482–497. doi:10.1080/09613218.2013.798142 | .
Herring, H., and Roy, R. (2007). Technological innovation, energy efficient design and the rebound effect. Technovation 27, 194–203. doi:10.1016/j.technovation.2006.11.004
Hilpert, S., Kaldemeyer, C., Krien, U., Günther, S., Wingenbach, C., and Plessmann, G. (2018). The Open Energy Modelling Framework (oemof)-A new approach to facilitate open science in energy system modelling. Energy Strateg. Rev. 22, 16–25. doi:10.1016/j.esr.2018.07.001
Hitchin, R., and Knight, I. (2016). Daily energy consumption signatures and control charts for air-conditioned buildings. Energy Build. 112, 101–109. doi:10.1016/j.enbuild.2015.11.059
Hygh, J. S., DeCarolis, J. F., Hill, D. B., and Ranjithan, S. R. (2012). Multivariate regression as an energy assessment tool in early building design. Build. Environ. 57, 165–175. doi:10.1016/j.buildenv.2012.04.021
IEA (2018a). Annex 67 - energy flexible buildings. Available at: http://www.annex67.org
IEA (2018b). Annex 79 - occupant-centric building design and operation. Available at: https://annex79.iea-ebc.org/
Imam, S., Coley, D. A., and Walker, I. (2017). The building performance gap: are modellers literate? Build. Serv. Eng. Res. Technol. 38, 351–375. doi:10.1177/0143624416684641
Ipbüker, C., Valge, M., Kalbe, K., Mauring, T., and Tkaczyk, A. H. (2016). Case study of multiple regression as evaluation tool for the study of relationships between energy demand, air tightness, and associated factors. J. Energy Eng. 143, 4016027. doi:10.1061/(ASCE)EY.1943-7897.0000375
ISO (2013). ISO 16346:2013, Energy performance of buildings — assessment of overall energy performance. Available at: https://www.iso.org/standard/65601.html
ISO (2017). ISO 52000-1:2017, Energy performance of buildings — overarching EPB assessment – Part 1: general framework and procedures. Available at: https://www.iso.org/standard/65601.html
ISO (2018). ISO 50001:2018, Energy management systems - requirements with guidance for use. Available at: https://www.iso.org/standard/69426.html
Jack, R., Loveday, D., Allinson, D., and Lomas, K. (2018). First evidence for the reliability of building co-heating tests. Build. Res. Inf. 46, 383–401. doi:10.1080/09613218.2017.1299523
Jaffal, I., Inard, C., and Ghiaus, C. (2009). Fast method to predict building heating demand based on the design of experiments. Energy Build. 41, 669–677. doi:10.1016/j.enbuild.2009.01.006
Jalori, S., and Reddy, T. A. (2015a). A new clustering method to identify outliers and diurnal schedules from building energy interval data. ASHRAE Trans. 121, 33.
Jalori, S., and Reddy, T. A. (2015b). A unified inverse modeling framework for whole-building energy interval data: daily and hourly baseline modeling and short-term load forecasting. ASHRAE Trans. 121, 156.
Jayaweera, T., Haeri, H., and Gowans, D. (2013). The uniform methods project: methods for determining energy efficiency savings for specific measures. Contract 303, 275–3000.
Jentsch, M. F., Bahaj, A. S., and James, P. A. B. (2008). Climate change future proofing of buildings—generation and assessment of building simulation weather files. Energy Build. 40, 2148–2168. doi:10.1016/j.enbuild.2008.06.005
Jentsch, M. F., James, P. A. B., Bourikas, L., and Bahaj, A. S. (2013). Transforming existing weather data for worldwide locations to enable energy and building performance simulation under future climates. Renew. Energy. 55, 514–524. doi:10.1016/j.renene.2012.12.049
Junker, R. G., Azar, A. G., Lopes, R. A., Lindberg, K. B., Reynders, G., Relan, R., et al. (2018). Characterizing the energy flexibility of buildings and districts. Appl. Energy. 225, 175–182. doi:10.1016/j.apenergy.2018.05.037
Köhler, J., Geels, F. W., Kern, F., Markard, J., Onsongo, E., Wieczorek, A., et al. (2019). An agenda for sustainability transitions research: state of the art and future directions. Environ. Innov. Soc. Transitions. 31, 1–32. doi:10.1016/j.eist.2019.01.004
Kavousian, A., and Rajagopal, R. (2013). Data-driven benchmarking of building energy efficiency utilizing statistical Frontier models. J. Comput. Civ. Eng. 28, 79–88. doi:10.1061/(ASCE)CP.1943-5487.0000327
Kohler, M., Blond, N., and Clappier, A. (2016). A city scale degree-day method to assess building space heating energy demands in Strasbourg Eurometropolis (France). Appl. Energy. 184, 40–54. doi:10.1016/j.apenergy.2016.09.075
Korolija, I., Marjanovic-Halburd, L., Zhang, Y., and Hanby, V. I. (2013). UK office buildings archetypal model as methodological approach in development of regression models for predicting building energy consumption from heating and cooling demands. Energy Build. 60, 152–162. doi:10.1016/j.enbuild.2012.12.032
Kotireddy, R., Hoes, P.-J., and Hensen, J. L. M. (2018). A methodology for performance robustness assessment of low-energy buildings using scenario analysis. Appl. Energy. 212, 428–442. doi:10.1016/j.apenergy.2017.12.066
Koulamas, C., Kalogeras, A. P., Pacheco-Torres, R., Casillas, J., and Ferrarini, L. (2018). Suitability analysis of modeling and assessment approaches in energy efficiency in buildings. Energy Build. 158, 1662–1682. doi:10.1016/j.enbuild.2017.12.002
Kraning, M., Chu, E., Lavaei, J., and Boyd, S. (2014). Dynamic network energy management via proximal message passing. Found. Trends Optim. 1, 73–126. doi:10.1561/2400000002
Kuster, C., Hippolyte, J.-L., Rezgui, Y., and Mourshed, M. (2019). A simplified geo-cluster definition for energy system planning in Europe. Energy Procedia. 158, 3222–3227. doi:10.1016/j.egypro.2019.01.1001
Kylili, A., Fokaides, P. A., and Lopez Jimenez, P. A. (2016). Key Performance Indicators (KPIs) approach in buildings renovation for the sustainability of the built environment: a review. Renew. Sustain. Energy Rev. 56, 906–915. doi:10.1016/j.rser.2015.11.096
Lammers, N., Kissock, K., Abels, B., and Sever, F. (2011). Measuring progress with normalized energy intensity. SAE Int. J. Mater. Manuf. 4, 460–467. doi:10.4271/2011-01-0320
Li, Q., Augenbroe, G., and Brown, J. (2016). Assessment of linear emulators in lightweight Bayesian calibration of dynamic building energy models for parameter estimation and performance prediction. Energy Build. 124, 194–202. doi:10.1016/j.enbuild.2016.04.025
Ligier, S., Robillart, M., Schalbart, P., and Peuportier, B. (2017). “Energy performance contracting methodology based upon simulation and measurement,” in Building simulation. San Francisco, CA: IBPSA. Available at: https://hal.archives-ouvertes.fr/hal-01556848.
Lim, H., and Zhai, Z. J. (2017). Review on stochastic modeling methods for building stock energy prediction. Build. Simul. 10, 607–624. doi:10.1007/s12273-017-0383-y
Lin, G., and Claridge, D. E. (2015). A temperature-based approach to detect abnormal building energy consumption. Energy Build. 93, 110–118. doi:10.1016/j.enbuild.2015.02.013
Lomas, K. J., Oliveira, S., Warren, P., Haines, V. J., Chatterton, T., Beizaee, A., et al. (2018). Do domestic heating controls save energy? A review of the evidence. Renew. Sustain. Energy Rev. 93, 52–75. doi:10.1016/j.rser.2018.05.002
Mancini, F., and Nastasi, B. (2019). Energy retrofitting effects on the energy flexibility of dwellings. Energies 12, 2788. doi:10.3390/en12142788
Manfren, M. (2012). Multi-commodity network flow models for dynamic energy management – mathematical formulation. Energy Procedia. 14, 1380–1385. doi:10.1016/j.egypro.2011.12.1105
Manfren, M., Aste, N., and Moshksar, R. (2013). Calibration and uncertainty analysis for computer models – a meta-model based approach for integrated building energy simulation. Appl. Energy. 103, 627–641. doi:10.1016/j.apenergy.2012.10.031
Manfren, M., and Nastasi, B. (2019). From in-situ measurement to regression and time series models: an overview of trends and prospects for building performance modelling. AIP Conf. Proc. 2123, 20100. doi:10.1063/1.5117027
Manfren, M., and Nastasi, B. (2020). Parametric performance analysis and energy model calibration workflow integration—a scalable approach for buildings. Energies 13, 621. doi:10.3390/en13030621
Manfren, M., Nastasi, B., Piana, E., and Tronchin, L. (2019). On the link between energy performance of building and thermal comfort: an example. AIP Conf. Proc. 2123, 20066. doi:10.1063/1.5116993
Markard, J., and Hoffmann, V. H. (2016). Analysis of complementarities: framework and examples from the energy transition. Technol. Forecast. Soc. Change. 111, 63–75. doi:10.1016/j.techfore.2016.06.008
Masuda, H., and Claridge, D. (2012a). Estimation of building parameters using simplified energy balance model and metered whole building energy use. Available at: http://hdl.handle.net/1969.1/148946
Masuda, H., and Claridge, D. E. (2012b). Inclusion of building envelope thermal lag effects in linear regression models of daily basis building energy use data.
Masuda, H., and Claridge, D. E. (2014). Statistical modeling of the building energy balance variable for screening of metered energy use in large commercial buildings. Energy Build. 77, 292–303. doi:10.1016/j.enbuild.2014.03.070
Mathieu, J. L., Price, P. N., Kiliccote, S., and Piette, M. A. (2011). Quantifying changes in building electricity use, with application to demand response. IEEE Trans. Smart Grid. 2, 507–518. doi:10.1109/TSG.2011.2145010
McKinsey (2014). Growth within: a circular economy vision for a competitive Europe. McKinsey Center for Business and Environment.
Meggers, F., Ritter, V., Goffin, P., Baetschmann, M., and Leibundgut, H. (2012). Low exergy building systems implementation. Energy 41, 48–55. doi:10.1016/j.energy.2011.07.031
Menezes, A. C., Cripps, A., Bouchlaghem, D., and Buswell, R. (2012). Predicted vs. actual energy performance of non-domestic buildings: using post-occupancy evaluation data to reduce the performance gap. Appl. Energy. 97, 355–364. doi:10.1016/j.apenergy.2011.11.075
Meng, Q., and Mourshed, M. (2017). Degree-day based non-domestic building energy analytics and modelling should use building and type specific base temperatures. Energy Build. 155, 260–268. doi:10.1016/j.enbuild.2017.09.034
Meng, Q., Xiong, C., Mourshed, M., Wu, M., Ren, X., Wang, W., et al. (2020). Change-point multivariable quantile regression to explore effect of weather variables on building energy consumption and estimate base temperature range. Sustain. Cities Soc. 53, 101900. doi:10.1016/j.scs.2019.101900
Miller, C., and Meggers, F. (2017). The Building Data Genome Project: an open, public data set from non-residential building electrical meters. Energy Procedia. 122, 439–444. doi:10.1016/j.egypro.2017.07.400
Miller, C., Nagy, Z., and Schlueter, A. (2015). Automated daily pattern filtering of measured building performance data. Autom. Constr. 49, 1–17. doi:10.1016/j.autcon.2014.09.004
Nastasi, B. (2019). “Hydrogen policy, market, and R&D projects.” in. Solar hydrogen production: Processes, systems and technologies 31–44
Nguyen, A.-T., Reiter, S., and Rigo, P. (2014). A review on simulation-based optimization methods applied to building performance analysis. Appl. Energy. 113, 1043–1058. doi:10.1016/j.apenergy.2013.08.061
Noussan, M., and Nastasi, B. (2018). Data analysis of heating systems for buildings—a tool for energy planning, policies and systems simulation. Energies 11, 233. Available at: http://www.mdpi.com/1996-1073/11/1/233.
Østergård, T., Jensen, R. L., and Maagaard, S. E. (2018). A comparison of six metamodeling techniques applied to building performance simulations. Appl. Energy. 211, 89–103. doi:10.1016/j.apenergy.2017.10.102
Østergård, T., Jensen, R. L., and Mikkelsen, F. S. (2020). The best way to perform building simulations? One-at-a-time optimization vs. Monte Carlo sampling. Energy Build. 208, 109628. doi:10.1016/j.enbuild.2019.109628
Oh, S., Haberl, J. S., and Baltazar, J.-C. (2020). Analysis methods for characterizing energy saving opportunities from home automation devices using smart meter data. Energy Build. 216, 109955. doi:10.1016/j.enbuild.2020.109955
Openmod. Open energy modelling initiative (Openmod) - open models. Available at:https://wiki.openmod-initiative.org/wiki/Open_Models.
Orehounig, K., Evins, R., and Dorer, V. (2015). Integration of decentralized energy systems in neighbourhoods using the energy hub approach. Appl. Energy. 154, 277–289. doi:10.1016/j.apenergy.2015.04.114
Orehounig, K., Mavromatidis, G., Evins, R., Dorer, V., and Carmeliet, J. (2014). Towards an energy sustainable community: an energy system analysis for a village in Switzerland. Energy Build. 84, 277–286. doi:10.1016/j.enbuild.2014.08.012
Pasichnyi, O., Wallin, J., and Kordas, O. (2019). Data-driven building archetypes for urban building energy modelling. Energy 181, 360–377. doi:10.1016/j.energy.2019.04.197
Paulus, M. T. (2017). Algorithm for explicit solution to the three parameter linear change-point regression model. Sci. Technol. Built Environ. 23, 1026–1035. doi:10.1080/23744731.2016.1267998
Paulus, M. T., Claridge, D. E., and Culp, C. (2015). Algorithm for automating the selection of a temperature dependent change point model. Energy Build. 87, 95–104. doi:10.1016/j.enbuild.2014.11.033
Pernigotto, G., Prada, A., Gasparella, A., and Hensen, J. L. M. (2014). Development of sets of simplified building models for building simulation.
Pfenninger, S., DeCarolis, J., Hirth, L., Quoilin, S., and Staffell, I. (2017). The importance of open data and software: is energy research lagging behind? Energy Pol. 101, 211–215. doi:10.1016/j.enpol.2016.11.046
Pfenninger, S., Hirth, L., Schlecht, I., Schmid, E., Wiese, F., Brown, T., et al. (2018). Opening the black box of energy modelling: strategies and lessons learned. Energy Strateg. Rev. 19, 63–71. doi:10.1016/j.esr.2017.12.002
Pistore, L., Pernigotto, G., Cappelletti, F., Gasparella, A., and Romagnoni, P. (2019). A stepwise approach integrating feature selection, regression techniques and cluster analysis to identify primary retrofit interventions on large stocks of buildings. Sustain. Cities Soc. 47, 101438. doi:10.1016/j.scs.2019.101438
Pomponi, F., and Moncaster, A. (2017). Circular economy for the built environment: a research framework. J. Clean. Prod. 143, 710–718. doi:10.1016/j.jclepro.2016.12.055
Pomponi, F., and Moncaster, A. (2018). Scrutinising embodied carbon in buildings: the next performance gap made manifest. Renew. Sustain. Energy Rev. 81, 2431–2442. doi:10.1016/j.rser.2017.06.049
Price, P.. (2010). Methods for analyzing electric load shape and its variability. Available at: https://www.osti.gov/servlets/purl/985909
Qomi, M. J. A., Noshadravan, A., Sobstyl, J. M., Toole, J., Ferreira, J., Pellenq, R. J.-M., et al. (2016). Data analytics for simplifying thermal efficiency planning in cities. J. R. Soc. Interface. 13, 20150971. doi:10.1098/rsif.2015.0971
Reka, S. S., and Dragicevic, T. (2018). Future effectual role of energy delivery: a comprehensive review of Internet of Things and smart grid. Renew. Sustain. Energy Rev. 91, 90–108. doi:10.1016/j.rser.2018.03.089
Richard, M.-A., Fortin, H., Poulin, A., Leduc, M.-A., and Fournier, M. (2017). Daily load profiles clustering: a powerful tool for demand side management in medium-sized industries. in ACEEE summer study on energy efficiency in industry, Denver, CO.
Rosenow, J., and Galvin, R. (2013). Evaluating the evaluations: evidence from energy efficiency programmes in Germany and the UK. Energy Build. 62, 450–458. doi:10.1016/j.enbuild.2013.03.021
Schlueter, A., and Geyer, P. (2018). Linking BIM and Design of Experiments to balance architectural and technical design factors for energy performance. Autom. Constr. 86, 33–43. doi:10.1016/j.autcon.2017.10.021
Serale, G., Fiorentini, M., Capozzoli, A., Bernardini, D., and Bemporad, A. (2018). Model predictive control (MPC) for enhancing building and HVAC system energy efficiency: problem formulation, applications and opportunities. Energies 11, 631. Available at: http://www.mdpi.com/1996-1073/11/3/631.
Server, F., Kissock, J. K., Brown, D., and Mulqueen, S. (2011). Estimating industrial building energy savings using inverse simulation. ASHRAE Trans. 117 348–355
Shiel, P., Tarantino, S., and Fischer, M. (2018). Parametric analysis of design stage building energy performance simulation models. Energy Build. 172, 78–93. doi:10.1016/j.enbuild.2018.04.045
Stadler, P., Girardin, L., Ashouri, A., and Maréchal, F. (2018). Contribution of model predictive control in the integration of renewable energy sources within the built environment. Front. Energy Res. 6, 22. doi:10.3389/fenrg.2018.00022
Sunikka-Blank, M., and Galvin, R. (2012). Introducing the prebound effect: the gap between performance and actual energy consumption. Build. Res. Inf. 40, 260–273. doi:10.1080/09613218.2012.690952
Tagliabue, L. C., Manfren, M., Ciribini, A. L. C., and De Angelis, E. (2016). Probabilistic behavioural modeling in building performance simulation—the Brescia eLUX lab. Energy Build.. 128, 119–131. doi:10.1016/j.enbuild.2016.06.083
Talele, S., Traylor, C., Arpan, L., Curley, C., Chen, C.-F., Day, J., et al. (2018). Energy modeling and data structure framework for Sustainable Human-Building Ecosystems (SHBE) — a review. Front. Energy. 9, 1226. doi:10.1007/s11708-017-0530-2 LB - Talele2018
Thornton, B. A., Rosenberg, M. I., Richman, E. E., Wang, W., Xie, Y., Zhang, J., et al. (2011). Achieving the 30% goal: energy and cost savings analysis of ASHRAE Standard 90.1–2010. Report No. PNNL-20405.
Thuesen, C., Koch-Ørvad, N., and Maslesa, E. (2016). “Organising sustainable transition: understanding the product, project and service domain of the built environment,” in 32nd Annual ARCOM conference.
Tronchin, L., and Fabbri, K. (2008). Analysis of buildings’ energy consumption by means of exergy method. Int. J. Exergy. 5, 605–625. doi:10.1504/IJEX.2008.020828
Tronchin, L., Manfren, M., and James, P. A. B. (2018a). Linking design and operation performance analysis through model calibration: parametric assessment on a Passive House building. Energy 165, 26–40. doi:10.1016/j.energy.2018.09.037
Tronchin, L., Manfren, M., and Nastasi, B. (2018b). Energy efficiency, demand side management and energy storage technologies – a critical analysis of possible paths of integration in the built environment. Renew. Sustain. Energy Rev. 95, 341–353. doi:10.1016/j.rser.2018.06.060
Tronchin, L., Manfren, M., and Nastasi, B. (2019). Energy analytics for supporting built environment decarbonisation. Energy Procedia. 157, 1486–1493. doi:10.1016/j.egypro.2018.11.313
Tronchin, L., Manfren, M., and Tagliabue, L. C. (2016). Optimization of building energy performance by means of multi-scale analysis – lessons learned from case studies. Sustain. Cities Soc. 27, 296–306. doi:10.1016/j.scs.2015.11.003
Tronchin, L., Tommasino, M. C., and Fabbri, K. (2014). On the “cost-optimal levels” of energy performance requirements and its economic evaluation in Italy. Int. J. Sustain. energy Plan. Manag. 3, 49–62. doi:10.5278/ijsepm.2014.3.5
Uriarte, I., Erkoreka, A., Giraldo-Soto, C., Martin, K., Uriarte, A., and Eguia, P. (2019). Mathematical development of an average method for estimating the reduction of the Heat Loss Coefficient of an energetically retrofitted occupied office building. Energy Build. 192, 101–122. doi:10.1016/j.enbuild.2019.03.006
Voss, K., Sartori, I., Napolitano, A., Geier, S., Gonçalves, H., Hall, M., et al. (2010). “Load matching and grid interaction of net zero energy buildings,” in EUROSUN 2010 international conference on solar heating, cooling and buildings.
Walter, T., and Sohn, M. D. (2016). A regression-based approach to estimating retrofit savings using the building performance database. Appl. Energy. 179, 996–1005. doi:10.1016/j.apenergy.2016.07.087
Wei, Y., Zhang, X., Shi, Y., Xia, L., Pan, S., Wu, J., et al. (2018). A review of data-driven approaches for prediction and classification of building energy consumption. Renew. Sustain. Energy Rev. 82, 1027–1047. doi:10.1016/j.rser.2017.09.108
Westermann, P., Deb, C., Schlueter, A., and Evins, R. (2020). Unsupervised learning of energy signatures to identify the heating system and building type using smart meter data. Appl. Energy. 264, 114715. doi:10.1016/j.apenergy.2020.114715
Westermann, P., and Evins, R. (2019). Surrogate modelling for sustainable building design – a review. Energy Build. 198, 170–186. doi:10.1016/j.enbuild.2019.05.057
Yang, Z., and Becerik-Gerber, B. (2015). A model calibration framework for simultaneous multi-level building energy simulation. Appl. Energy. 149, 415–431. doi:10.1016/j.apenergy.2015.03.048
Yoshino, H., Hong, T., and Nord, N. (2017). IEA EBC annex 53: total energy use in buildings—analysis and evaluation methods. Energy Build. 152, 124–136. doi:10.1016/j.enbuild.2017.07.038
Keywords: building energy performance, open energy data, open software, data-driven methods, multivariate regression
Citation: Manfren M, Nastasi B and Tronchin L (2020) Linking Design and Operation Phase Energy Performance Analysis Through Regression-Based Approaches. Front. Energy Res. 8:557649. doi: 10.3389/fenrg.2020.557649
Received: 30 April 2020; Accepted: 01 October 2020;
Published: 24 November 2020.
Edited by:
Michel Feidt, UMR7563 Laboratoire d'énergétique et de mécanique théorique et appliquée (LEMTA), FranceReviewed by:
Marco Caniato, Free University of Bozen-Bolzano, ItalyTong Yang, Middlesex University, United Kingdom
Copyright © 2020 Manfren, Nastasi and Tronchin. This is an open-access article distributed under the terms of the Creative Commons Attribution License (CC BY). The use, distribution or reproduction in other forums is permitted, provided the original author(s) and the copyright owner(s) are credited and that the original publication in this journal is cited, in accordance with accepted academic practice. No use, distribution or reproduction is permitted which does not comply with these terms.
*Correspondence: Benedetto Nastasi, YmVuZWRldHRvLm5hc3Rhc2lAb3V0bG9vay5jb20=