- 1Department of Energy and Technology, Swedish University of Agricultural Sciences, Uppsala, Sweden
- 2MaREI Centre, Environmental Research Institute, University College Cork, Ireland
- 3Department of Biochemical Conversion, Deutsches Biomasseforschungszentrum gemeinnützige GmbH, Leipzig, Germany
Wind power coupled to hydrogen (H2) production is an interesting strategy to reduce power curtailment and to provide clean fuel for decarbonizing agricultural activities. However, such implementation is challenging for several reasons, including uncertainties in wind power availability, seasonalities in agricultural fuel demand, capital-intensive gas storage systems, and high specific investment costs of small-scale electrolysers. To investigate whether on-site H2 production could be a feasible alternative to conventional diesel farming, a model was built for dynamic simulations of H2 production from wind power driven by the fuel demand of a cereal farm located on the island of Gotland, Sweden. Different cases and technological scenarios were considered to assess the effects of future developments, H2 end-use, as well as production scale on the levelised- and farmers’ equivalent annual costs. In a single-farm application, H2 production costs varied between 21.20–14.82 €/kg. By sharing a power-to-H2 facility among four different farms of 300-ha each, the specific investment costs could be significantly decreased, resulting in 28% lower H2 production costs than when facilities are not shared. By including delivery vans as additional H2 consumers in each farm, costs of H2 production decreased by 35% due to the higher production scale and more distributed demand. However, in all cases and technological scenarios assessed, projected diesel price in retailers was cheaper than H2. Nevertheless, revenues from leasing the land to wind power developers could make H2 a more attractive option even in single-farm applications as early as 2020. Without such revenues, H2 is more competitive than diesel where power-to-H2 plants are shared by at least two farms, if technological developments predicted for 2030 come true. Also, out of 20 different cases assessed, nine of them showed a carbon abatement cost lower than the current carbon tax in Sweden of 110 €/tCO2, which demonstrate the potential of power-to-H2 as an effective strategy to decarbonize agricultural systems.
Introduction
Renewable energy sources can be exploited in remote areas with limited interconnection such as islands and/or agricultural farmlands to increase energy independence and security. Recently, declining costs of solar and wind power combined with policies and incentives to tackle climate change have created favorable conditions to further expand renewable energy production in such regions. However, due to its intermittency and uncertainty (especially for wind), high levels of variable renewable energy (VRE) are challenging to integrate into current energy systems, frequently resulting in a mismatch between supply and demand. Such imbalances cause fluctuations in grid voltage and frequency, as well as curtailment of power production, considerably increasing the overall costs of the system.
For this reason, different energy storage technologies have been developed for several applications, in particular to avoid curtailment of power production, and to support stable operations of electric grids (Fischer et al., 2018b; Koohi-Fayegh and Rosen, 2020). To compensate for production fluctuations as well as providing benefits at the system level (e.g., control reserve energy), H2 based storage systems have been proposed (Grueger et al., 2017). Also, as a clean and versatile energy carrier, H2 may have an important role in future low-carbon pathways, for instance, to produce gaseous (e.g., CH4 and NH3) and liquid fuels (e.g., methanol, gasoline, and dimethyl ether), heat or even directly used as fuel for mobility (Hanley et al., 2018).
In grain-based agricultural systems, nitrogen fertilizers and fossil fuel consumption are responsible for the majority of the GHG emissions (Yan et al., 2015). Where VRE is deployed on farmland, an interesting concept to decarbonize agricultural activities is to also include H2 storage. Thus, curtailment could be avoided and local renewable electricity could be used to produce H2 to displace diesel as a fuel in tractors and/or used to make NH3 for fertilizer via the Haber-Bosch process (Moreda et al., 2016; Allman and Daoutidis, 2018). While the latter may be restricted to large farming operations (minimum megawatt-scale equipment), H2 as fuel could potentially be used in small- and mid-size farms since it has been successfully implemented at the kilowatt-scale in industrial applications such as welding and brazing, material handling vehicles (e.g., forklifts and airport towing trucks) as well as for mobility (e.g., golf cart and long-range passenger cars) (Allman et al., 2017; Apostolou et al., 2019). Thanks to its higher energy density compared to lithium-ion batteries, H2-based fuel cell agricultural machinery (FCAM) may be preferred to manned battery-electric since agricultural operations often require continuous hours of heavy fieldwork (NHA, 2012; Wu et al., 2019; Lagnelöv et al., 2020). In addition, during the conversion of electricity to H2 via water electrolysis, oxygen (O2) and low-temperature waste heat (WH) at 60–90°C are produced which could be valorized (Buttler and Spliethoff, 2018). For instance, WH could be used for drying grains or heating greenhouses, while O2 could be used in aquaculture, in particular for sensitive species like salmon and trout (García et al., 1998; Mariani et al., 2016; Linde, 2017b). These applications should improve the sustainability of the concept as well as reduce costs associated with H2 production.
However, the on-farm production of H2 to be used in FCAM is challenging: 1) farming is a highly seasonal activity in which typical operations like harrowing, sowing, fertilizing, plowing and harvesting occur over short periods of time, resulting in peaks of fuel demand; 2) VRE production is uncertain by nature increasing risks of mismatch between supply and demand; 3) Large gas storage to compensate for such seasonalities are capital-intensive, and 4) decentralized small-scale electrolysers have higher specific investment costs increasing production costs compared to larger facilities.
Small-scale H2 production via water electrolysis has been investigated for different applications. For instance, Fischer et al. (2018a) developed a predictive control model for a 120 kW proton exchange membrane (PEM) electrolyser, injecting H2 into the natural gas grid according to fluctuating electricity prices in the spot market and within network limitations. In an energy system dominated by hydropower production, Ulleberg et al. (2020) examined the deployment of small-scale electrolysers coupled to H2 refueling stations for fuel cell electric vehicles. Similarly, Apostolou et al. (2019) further down-scaled the process proposing the use of a 50 kW wind turbine coupled to a 70 kW alkaline electrolyser to supply H2 for fuel cell electric bicycles in a green urban mobility concept. Also, H2 refueling stations with electrolysers smaller than 500 kW to supply the demand of H2 cars, and the optimization of an electrolyser operation employing wind, electricity prices, and H2 demand have been investigated elsewhere (Grüger et al., 2018; Grüger et al., 2019). Furthermore, the feasibility of stationary power-to-gas systems to store excess electricity from renewable sources in buildings with different heat and power requirements have been assessed combined with oxy-fuel boilers to produce concentrated CO2 stream and facilitate further methanation of H2 (Bailera et al., 2018; Bailera et al., 2019). Even though previous studies addressed H2 production from solar PV to fuel an all-wheel drive vehicle on a winery (Carroquino et al., 2018; Roda et al., 2018), to the best of the authors’ knowledge, techno-economic assessments of on-farm H2 production based on wind power to supply the fuel demand of heavier agricultural machineries like tractors and harvesters have never been reported. Such a concept could provide multiple benefits, curtailment could be avoided increasing the income of wind power project developers, locally produced clean fuel would be provided to decarbonize agricultural activities, and land leasing payments would be provided to farmers.
In Sweden, the local authorities of Gotland’s island have committed to an ambitious plan to be self-sufficient in energy by 2025. For this reason, local wind power production is planned to increase 5-fold (to around 1,000 MW) while grid interconnection to the mainland will be restricted to 500 MW. Nowadays, major efforts are being made by different research initiatives to develop feasible options to store and manage excess electricity that may occur (GEAB, Vatenfall, ABB, and KTH, 2011; Byman, 2015; Mohseni et al., 2017; Wallnerström and Bertling Tjernberg, 2018). Our study differentiates from previous investigations by focusing on developing a modeling tool for discrete-event simulation of H2 production according to the fuel demand of cereal-based farms located on Gotland. The model was used to find optimal plant configurations that minimized the levelized cost of H2 (LCOH2) according to the following cases: 1) single-farm H2 production for FCAM; 2) shared infrastructure between two farms for FCAM and fuel cell minivan (FCMV); and 3) increased scale production by sharing the PtH2 plant among four farms for FCAM and FCMV. Optimal plant configurations were used for further assessment of the equivalent annual cost (EAC) to compare the cost of ownership of FCAM and conventional diesel agricultural machinery in different technological scenarios (2020 and 2030). Additionally, to provide insights for policymakers on possible decarbonization strategies, the carbon abatement cost of each case assessed was also calculated.
Methodology
System Description
The power-to-hydrogen (PtH2) plant refers to an electrolyser, compressor, storage system, and a dispenser located on a farm on the island of Gotland, Sweden (57°30′N 18°33′E/57°50′N 18°55′E). A proton-exchange membrane (PEM) electrolyser was chosen due to its suitability for small-scale applications. The overall reaction of H2 production by water electrolysis is shown in Eq. 1:
The electricity is primarily obtained from wind turbines located inside the farm boundary. However, during system downtime and for safety infrastructure, electricity is also obtained from the grid (regulated market) in small volumes. To allow storage at 500 bar, H2 is compressed as soon as it is produced in the stacks (Linde, 2014). The H2 is supplied according to the demand of FCAM and where applicable FCMV used for delivery (see Agricultural H2Demand). Additionally, the economic benefits of utilizing low-temperature WH at 60°C in a greenhouse for growing tomatoes (see Appendix A), and O2 for on-site fish farming are considered (Linde, 2017a; Linde, 2017b; Törnfet and Nypelius, 2020).
In this system, farmers cooperate with wind power project developers in a business model where farmland is leased to wind power production securing additional revenues to farmers and improving wind power output, in case H2 is produced at times of constrained power grid. Figure 1 and Table 1 show the technical system boundary and an overview of the characteristics of PEM electrolyser considered in the present study.
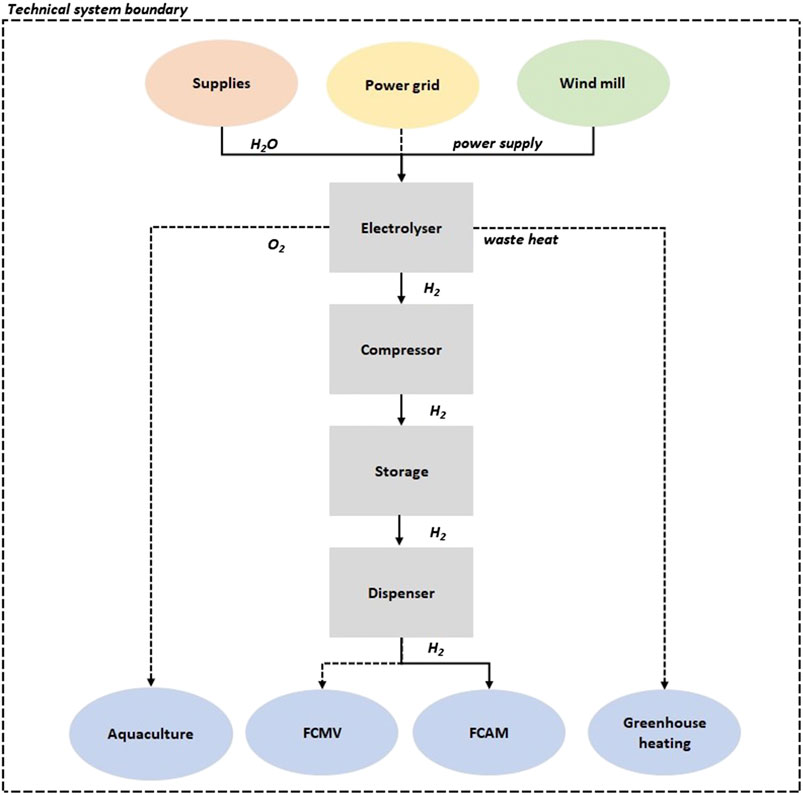
FIGURE 1. Schematic diagram of the technical system boundary. Note: Dotted arrow lines represent optional H2 and/or by-products use; FCMV - fuel cell minivan; FCAM - fuel cell agricultural machinery.
Dynamics of the Power-to-Hydrogen Plant Operation
The H2 demand of FCAM and FCMV and the H2 level in the storage tank determine whether the electrolyser should enter in operation. Therefore, whenever H2 storage is low and wind power production is sufficient to run the electrolyser on full-load, H2 is produced until the storage tank is full. At times of no wind power production or full H2 storage, the system is put directly on cold standby since the time required to ramp-up a PEM electrolyser is negligible (Buttler and Spliethoff, 2018). Thus, cold standby solely defines the non-operating hours (NOH) of the system. The energy consumed during NOH and by the safety infrastructure is purchased from the regulated market with a fixed tariff of 100 €/MWh as the quantities are too low to qualify for a cheaper tariff (e.g., day-ahead spot market). A schematic diagram of the dynamic PtH2 plant operation is presented in Figure 2 (additional information is provided in Power-to-Hydrogen Model and Optimization Procedure).
Cases Assessed
To assess the influence of H2 demand on the economic performance of the agricultural PtH2 plant, different cases were investigated under the current and future technological scenarios (2020 and 2030). A single-user PtH2 plant was used as a reference in case 1, while the option of sharing the PtH2 with more than one farm was investigated in Cases 2a and 3a. The influence of having FCMV for delivery in addition to the H2 demand of FCAM was investigated in Cases 2b and 3b. In all cases, wind power per land area was calculated as an average value according to local conditions found in Sweden (approx. 6.5 MW/km2) (Stadkraft, 2020). Thus, wind power capacity was used to determine land lease revenues and, combined with the specific wind power production (see Wind Power Production), it was also used for modeling wind power production/availability for PtH2 applications. A summary of all cases assessed is found in Table 2.
Wind Power Production
Historical wind speed measurements were used to simulate wind power production. Hourly values for 2017 were obtained from the meteorological station at the Visby Airport (57°66′N 18°34′E) on Gotland, Sweden. The station is located at 42 m above sea level and measures wind at 10 m high from the ground (SMHI, 2017). The wind speed and wind direction are shown as a wind rose in Figure 3A as well as the wind speed frequency (Figure 3B). Wind speed was extrapolated to the turbine hub height of 95 m using the power law with an exponent of 0.13 (McDonagh et al., 2020). The power curve of the V90 2.0 MW wind turbine (Vestas, Denmark) was used to convert wind speed into power for parks with 10–40 wind turbines depending on the case assessed (see Agricultural H2Demand). Such turbine cuts in at 4 m/s, is rated at 13 m/s, and cuts out at 25 m/s (Vestas, 2019).
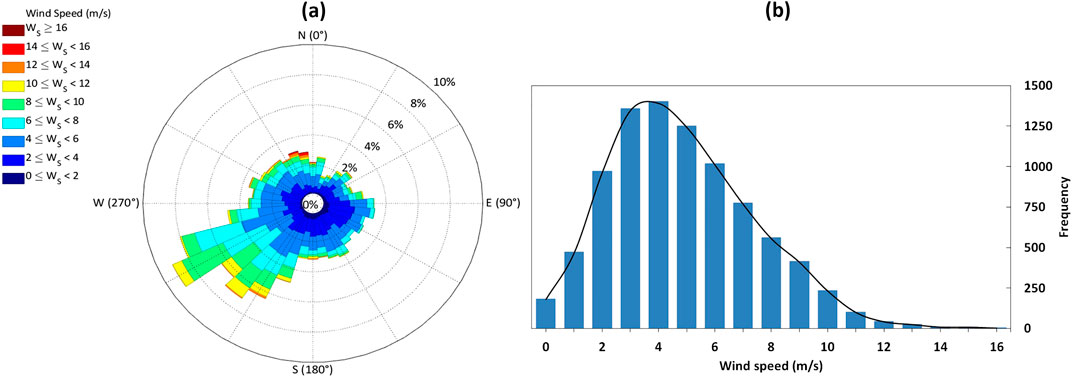
FIGURE 3. Wind rose (A) and wind speed frequency distribution (B) at the Visby Airport on Gotland, Sweden.
Price of Electricity Used for H2 Production
Hourly values from the day-ahead spot market of the Nord Pool power exchange were used to calculate the electricity costs to run the PtH2 plant (NordPool, 2019). Thus, the electricity used is accounted for as an opportunity cost if the wind power operator would have the option to sell electricity to the grid. Even though electricity prices can vary significantly when different years are compared (Janke et al., 2020), 2017 was chosen since the average price found in this year is representative of historical values between 2013–2018 in Sweden. The price distribution in the day-ahead market of the Nord Pool power exchange for the SE3 region in 2017 is shown in Figure 4. We do not consider discounting the electricity price as the benefits offered to the wind farm developer (reduced curtailment, system flexibility) are captured in the land leasing payment made to the landowner/farmer (see Power-to-Hydrogen Model and Optimization Procedure).
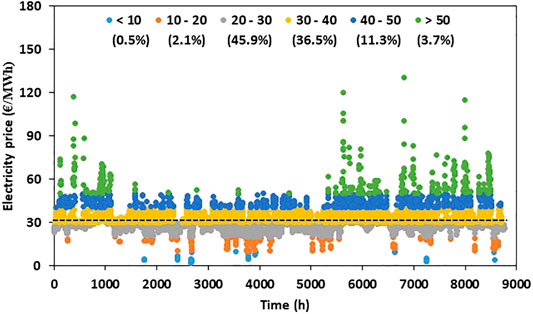
FIGURE 4. Day-ahead price distribution in the spot market of the Nord Pool for SE3 region. Note: Dotted line represents the average price in the year.
Agricultural H2 Demand
In the present study, H2 demand is modeled according to the requirements of two different consumers, namely FCAM (agricultural machinery) and FCMV (minivan). FCAM H2 demand was estimated for a cereal farm in Sweden according to the model described by Lagnelöv et al. (2020) based on dynamic discrete-event simulation with embedded state-based logic for decision making. The simulated farm encompassed 300-ha equally distributed between barley, oats, spring wheat, and winter wheat crops. The model used a conventional cropping system with work beginning in mid-March and ending at the start of November (Lagnelöv et al., 2020). One of the main aspects of this model is a workability control based on weather conditions and soil moisture content, which considered the water balance model described in (Witney, 1988) and tested by (Nilsson and Hansson, 2001). In the present study, weather and soil data from the island of Gotland (Sweden) were used instead of the values in the original report. The soil type on Gotland is mainly sand or sandy loam (Lundblad, 2015; Paulsson et al., 2015) and the soil density, field capacity, saturation, permanent wilting point, and plastic limit of sandy loam described in (Witney, 1988) was assumed adequate and used. Weather data on monthly mean air temperature, number of daily sunshine hours, and hourly precipitation were obtained from the meteorological station at the Visby Airport on Gotland, Sweden (57°66′N 18°34′E). Even though for wind power production and electricity prices data from 2017 was considered, to model the agricultural H2 demand, data of precipitation, air temperature, and sunshine hours from 2016 were considered since they better represented average values in the region for the period 1989–2018 (SHMI, 2020). The H2 demand during crop harvesting was modeled based on 28% of total fuel demand in a cereal farm according to Safa et al. (2010). The instantaneous power demand for the FCAM and the refuelling station was measured separately and were both assumed to be linear average values. The average refuelling time considered was 0.32 h and the refuelling station was assumed to have a constant H2 flow for the duration. The input values used to simulate the H2 demand of FCAM are shown in Table 3.
H2 demand of FCMV was estimated for an average driving of 35,000 km/year with a diesel-equivalent consumption of 4.43 L/100 km. The total consumption of 1,825 L/year (8.86 L/day) was equally distributed throughout the year and added to the H2 demand of FCAM when applicable (see Agricultural H2Demand).
By-Products Recovery
As the primary aim of the current study is to investigate H2 production on-demand, by-products production is not optimized. However, it is nevertheless possible to recover the produced WH and O2 for individual end-use applications. To valorize the WH stream, the size of the greenhouse was varied from 1,000 to 10,000 m2 for tomato production according to the rated power of each simulated electrolyser size (50–500 kW). A water tank (heat capacity of 70 kWh/m3; 5% whole system thermal losses assumed) with up to 24 h of full load capacity is used to account for short-term imbalances between heat supply and demand such as daily fluctuations during summertime (Novo et al., 2010; Guelpa and Verda, 2019). Along with the varying greenhouse size, different sizes of water tanks were also considered from 3 to 30 m3 with a specific investment cost of 40 €/m3 (Guelpa and Verda, 2019). More information about the heat demand estimation can be found in Figure A1 in Appendix A.
For the on-site use of O2, it was considered that all assessed farm configurations are combined with a 2,500 m3 tank for rainbow trout cultivation where O2 is injected for controlling the dissolved oxygen levels in the water. A stock density of 15 kg/m3 was applied with an average specific O2 consumption of 350 mg O2/kg/h (Boyd, 2011; Woynarovich et al., 2011). As the produced O2 from the electrolyser can only offset 23–27% of the total demand for fish farming, each tank is equipped with a dedicated O2 generation system based on pressure swing adsorption (PSA) technology with a power consumption of 1.1 kWh per m3 of O2 (85% v/v) (Aquaculture Technology, 2020). Given an electricity tariff of 100 €/MWh (regulated market), those characteristics result in an O2 production cost of around 0.19 €/kg. Hence, this value is further used to monetize the O2 production from the electrolyser.
Power-to-Hydrogen Model and Optimization Procedure
The PtH2 model was implemented in the Matlab-based Simulink environment version R2019b (MathWorks, USA). Individual equations are discretized for a fixed step size (sampling time) of 1 h. It is based on variable hourly values of wind power production, day-ahead spot market price, and fuel demand. The PEM electrolyser was modeled in combination with a compressed gas storage system to assist H2 production and delivery on-demand. The model calculates H2, WH, and O2 production as well as run hours and total electricity cost. The decision whether the electrolyser should enter into operation is dependent on the amount of H2 available in the gas storage and the availability of wind power to run the electrolyser on full-load as described below (Eq. 2):
where
H2 production in each hour (
where
O2 production
where
where
Waste heat production in hour i (
where
The run hours of the system per year (
The costs associated with electricity use during the electrolyser operation (
where
The yearly costs to keep the electrolyser on cold standby (
The power consumption during cold standby and for safety infrastructure is based on a 1.074 MW plant and is proportionally adjusted to each size of electrolyser assessed (Frank et al., 2018). To allow gas storage at 500 bar, H2 is compressed requiring 2.2 kWh/kg H2 (
Finally, the total electricity cost of the PtH2 plant (
To determine the optimal plant configuration a total number of 256 simulations were run for each case assessed. Each simulation corresponded to a combination of electrolyser size between 50 and 500 kW (30 kW increments) and gas storage capacity between 10 and 50 m3 (2.66 m3 increments). For each plant configuration, specific CAPEX (€/kW), capacity factor, the average price paid for the electricity, and the LCOH2 were calculated and used for assessment. To verify whether the PtH2 plant configurations were fulfilling the consumers' fuel requirement, the delivery of H2 on-demand was considered a mandatory criterion. The characteristic dependencies of different plant configurations on each performance indicator were visualized using Matlab function contour 3-days plot (MathWorks, USA). For each case assessed, the combination of electrolyser size and gas storage capacity that resulted in the lowest LCOH2 and simultaneously fulfills H2 demand was considered the optimal plant configuration.
Economic Assessment
The economic performance of the system was assessed based on two economic indicators, namely the LCOH2 and equivalent annual cost (EAC). While the LCOH2 is used to optimize the PtH2 plant configuration in terms of electrolyser size and H2 storage capacity, the EAC is used to compare the H2 system with a conventional diesel-fueled one. To determine the EAC, the net present value (NPV) is first calculated as follows (Eq. 12):
where
The net cash flow (NCF) is the operational expenditures subtracted by the land lease over the lifespan of the project as per Eq. 13:
where
Different diesel prices are considered to depict the influence of agricultural diesel tax relief as well as a future fuel prices in Sweden. A summary of the different prices considered in the present study are shown in Table 4.
Finally, the EAC is calculated as the cost per year of owning and operating the PtH2 and diesel-fueled agricultural systems over the lifespan of the project as follows (Eq. 14):
The LCOH2 is the breakeven selling price of the H2 produced and is given by Eq. 15 below:
All indicators are calculated in 2018 euros.
The timeline for relevant calculations includes a 3-year commissioning phase, 25 years of operation, and one-year decommissioning. Also, additional costs, such as land, permits, transport, site preparation, engineering, and design costs, grid connection as well as contingency were assumed to be equivalent to 10% of the electrolyser’s CAPEX (Benjaminsson et al., 2013).The economic model does not consider reductions in electrolyser performance over time, however, component replacement costs are included in economic assessment (2 replacements over project’s lifetime). Even though our study uses the most recent literature available, unavoidable uncertainties exist in capital expenditures (Schmidt et al., 2017). CAPEX and OPEX values of PEM electrolyser used in this study are shown in Table 5.
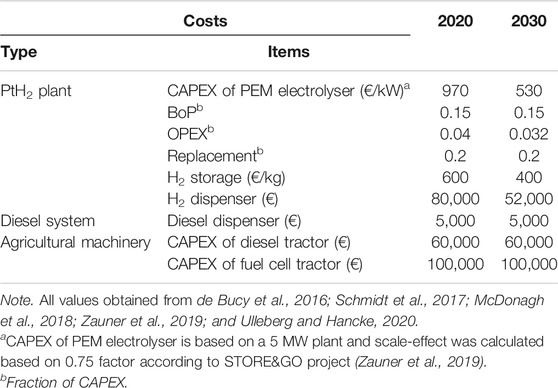
TABLE 5. Capital expenditures (CAPEX), balance of the plant (BoP) and operational expenditures of the PtH2 plant in different technological scenarios.
Results and Discussions
H2 Demand
As described in Agricultural H2Demand, H2 demand was modeled for different farm cases which include FCAM, some also include FCMV. As an example, Figure 5 shows the demand profile at the dispenser for case 2b where two farms share a PtH2 plant to fuel their agricultural machinery and one FCMV each. As expected, H2 demand for FCAM is highly seasonal, there is no demand during winter, extended parts of the summer, and some interim periods when no fieldwork is required. In contrast, H2 demand of FCMVs occurs on a year-round basis, however, requiring much less energy than FCAM per refill. In fact, the total H2 demand of the FCMVs in case 2b was just 28% of the total fuel demand.
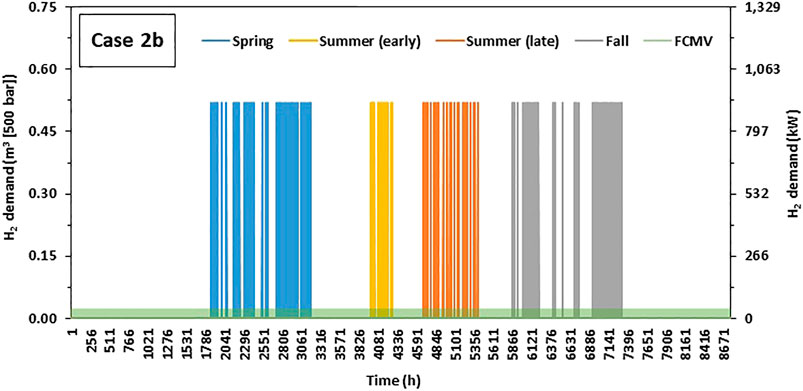
FIGURE 5. H2 demand profile of the cereal farm system for the case 2b. Note: Detailed description of the agricultural activities carried out in each season is provided by Lagnelöv et al. (2020); FCMV - fuel cell minivan.
When the PtH2 plant is scaled-up to fulfill the H2 demand of four farms including one FCMV each (case 3b), the fuel demand is double that seen in Figure 4 with the same demand profile. Conversely, where a single farm operates a PtH2 plant (case 1) FCAM demand is halved and FCMV is disregarded.
Optimization of H2 Production
For the optimization of the PtH2 plant, the electrolyser and storage capacity sizes were varied to find plant configurations that resulted in the lowest possible LCOH2. This procedure was performed for each farm case as well as for different technological scenarios assessed (Figure A2 in Appendix B). Again, case 2b (2020) is used as an example (Figure 6).
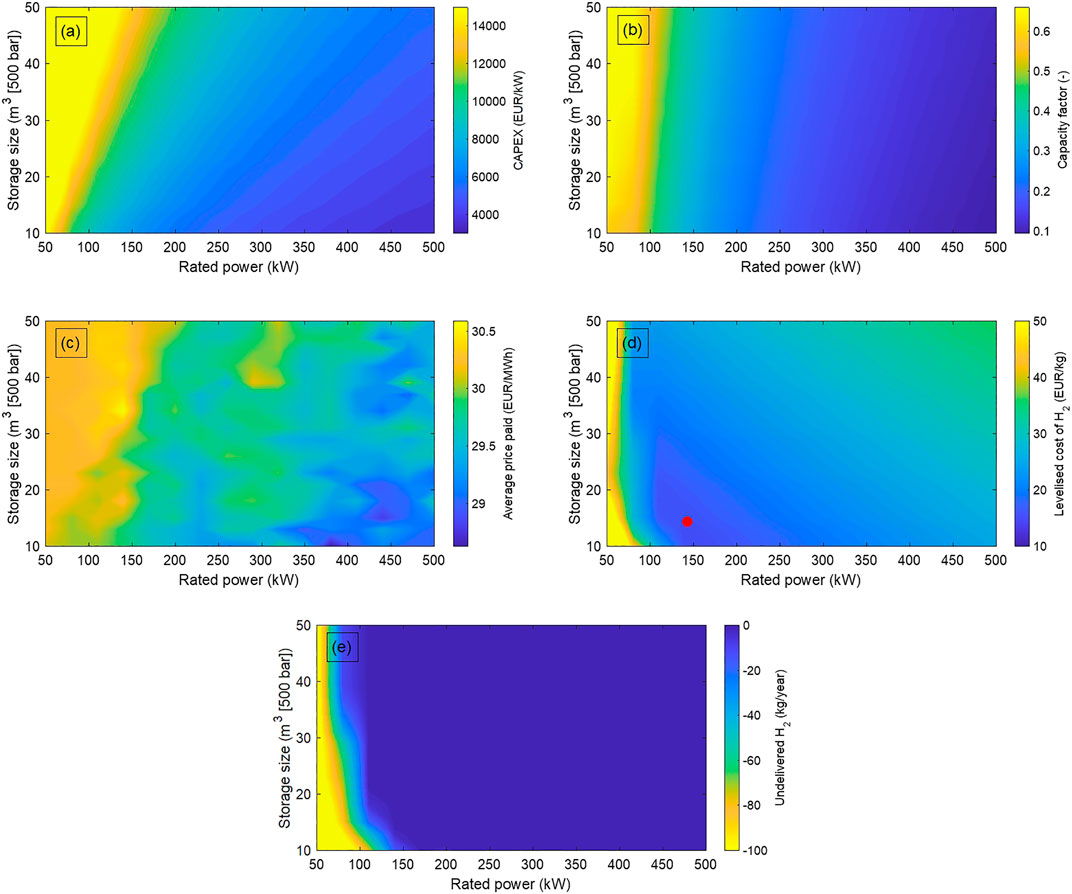
FIGURE 6. Results of electrolyser and storage capacity optimization for case 2b in 2020. (A) specific capital expenditures (CAPEX); (B) capacity factor; (C) average price paid for the electricity; (D) levelized cost of H2 (LCOH2); (E) H2 delivery check. Note: Red dot shows the optimal plant configuration to minimize LCOH2.
Economies of scale are significant in the ranges examined and heavily influenced the economic performance of the agricultural PtH2 plant (Zauner et al., 2019). However, as shown in Figure 6B, increasing electrolyser sizes also led to lower capacity factors. Such behavior is explained by the plant being driven according to the specific H2 demand, thereby increasing electrolyser capacity did not necessarily result in higher H2 production. Previous studies on electrofuels production showed that the number of running hours of the plant and the price paid for the electricity were the most important factors to minimize the production costs for a fixed capacity (McDonagh et al., 2019; Janke et al., 2020). In the present study, as the average price paid for the electricity varied less than 10% among all simulated conditions, it was indeed the capacity factor that most influenced the H2 production costs. For instance, in case 2b (2020) the lowest LCOH2 was found for a plant with a 140 kW of electrolyser size and 15 m3 (500 bar) of storage capacity (i.e., equivalent to 11 days of full-load operation). This plant configuration resulted in 3,060 h/year of operation and it was able to produce H2 at a cost of 15.87 €/kg. When the H2 demand of FCMN is disregarded (case 2a–2020), a comparable PtH2 plant would operate 11% less (2,715 h/year), which in turn results in around 6% higher H2 production costs. In contrast, if the electrolyser size would be reduced to lower than 140 kW, the number of operating hours would increase, which in theory could potentially reduce the production costs. As observed in Figure 6B, however, if smaller electrolysers are used H2 is not delivered on-demand, thus excessively small electrolysers are not considered suitable for farm operations even if coupled to large storage capacities (expensive option).
In fact, due to the highly seasonal fuel demand and relatively high cost of additional storage capacity, it is challenging to design a PtH2 plant with sufficient run hours able to truly minimize the LCOH2. A previous study on PtCH4 showed that at least 5,000 operating hours per year (57% capacity factor) would be required, and values lower than 4,000 h/year would likely result in prohibitive production costs (McDonagh et al., 2019). For case 2b (2020) the LCOH2 of 15.87 €/kg is equivalent to a diesel price of 4.02 €/L which is indeed prohibitive when compared to the assumed diesel retail price of 1.35 €/L. Such diesel price includes a carbon tax of 110 €/tCO2 applied for fossil fuel consumption. In countries like Sweden where farmers pay less for consuming fossil fuel due to the relief on the existing carbon tax, the adoption of alternative fuels by farmers becomes even more challenging since the real diesel price paid by farmers is around 1.17 €/L.
In case farmers organize themselves in a small cooperative where four farms share the same PtH2 infrastructure to supply fuel for their agricultural machinery and one FCMV in each farm (case 3b), the system is up-scaled to an optimal configuration of 290 kW electrolyser and 26 m3 (500 bar) of storage capacity. Even though this higher H2 demand does not necessarily result in major changes in the capacity factor of the plant, the specific CAPEX is reduced by 17% compared to sharing the infrastructure with just two farms (case 2b), which in turn proportionally reduces the H2 production costs (Table 6).
New composite materials for compressed H2 storage and reduced use of noble metals like platinum and titanium in PEM electrolysis will result in lower costs in the future (Schmidt et al., 2017; Moradi and Groth, 2019). As no changes in optimal plant configurations were found in 2030 compared to 2020, these technological developments are considered the main reason for the 30% reduction in LCOH2 observed. Interestingly, a previous study from our group based on H2 production without demand constraints, showed a lower reduction of 18% in production costs when comparing 2020 and 2030 technological scenarios (Janke et al., 2020). In that case, the higher capacity factor of the electrolyser (≥75%) increased the total expenses with electricity purchase, thereby reducing the effect of CAPEX on the LCOH2.
Effect of By-Products Recovery
The PtH2 plant produces and delivers H2 according to the demand of FCAM and FCMV, however, the process of water electrolysis also results in O2 production mediated by an exothermic reaction (Eq. 1 described in System Description). As PEM electrolysers are operated under controlled temperature (50–80°C), a water-based cooling system needs to be integrated to avoid overheating of the cell (>100°C), thereby also allowing the recovery of low-temperature waste heat (Buttler and Spliethoff, 2018). The feasibility of valorizing these by-products depends on local demand. For instance, our farm includes intensive tomato cultivation in a greenhouse, which requires temperature control for year-round production. In this case, it is assumed that the electrolyser’s cooling system could be integrated to the heating system of the greenhouse, offsetting the heat required from conventional sources (Wiederholm et al., 2018). Furthermore, O2 use in aquaculture has gained attention in recent years, in particular in recirculating aquaculture systems that require high levels of dissolved oxygen to allow high production densities. As O2 would be usually generated on-site via energy-intensive PSA systems, water electrolysis could partly supply O2 to aquaculture offsetting costs associated with the oxygenation process. Both WH and O2 valorization would positively impact the economic performance of the PtH2 plant. Such benefits in terms of LCOH2 reduction are shown in Figure 7.
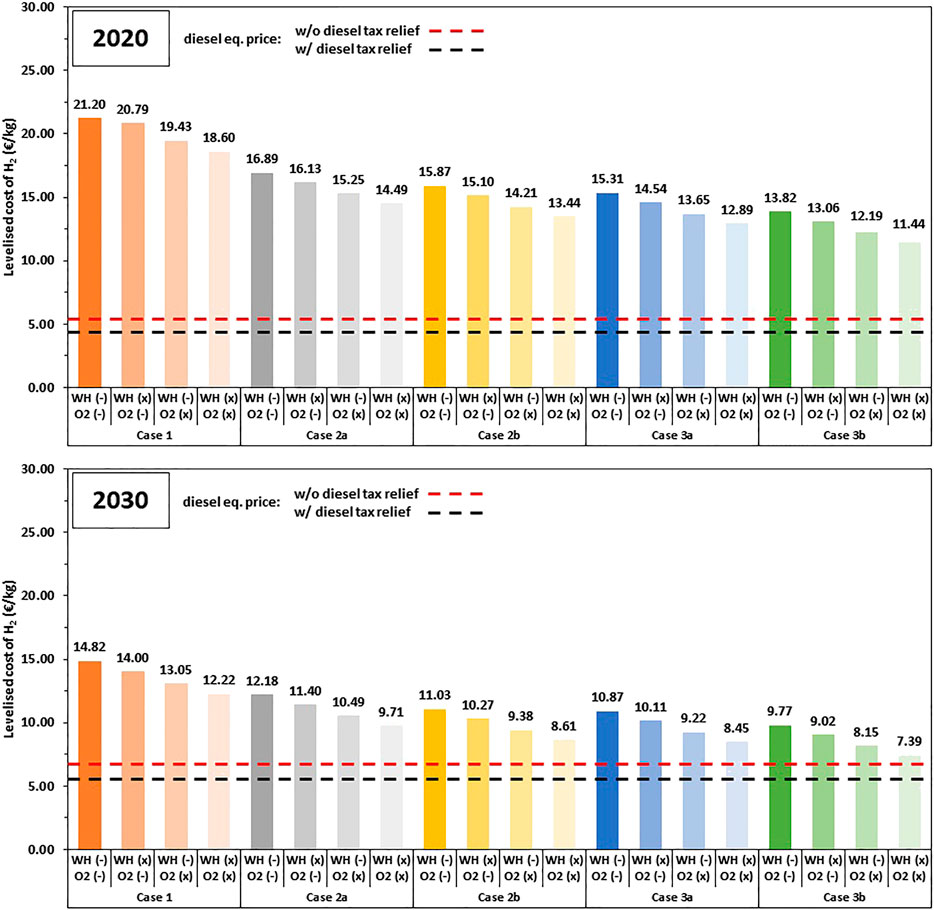
FIGURE 7. Effect of by-products recovery on the levelized cost of H2 (LCOH2) according to the different cases assessed. Note: Red dotted lines represent the diesel equivalent price without tax relief (2020–1.35 €/L or 5.33 €/kg H2; 2030–1.62 €/L or 6.40 €/kg H2); black dotted lines represent the diesel equivalent price with tax relief (2020–1.17 €/L or 4.62 €/kg H2; 2030–1.40 €/L or 5.53 €/kg H2); WH–waste heat; O2–oxygen; (-) without; and (x) with.
Independent of the case and/or year assessed recovering O2 showed to be more valuable compared to WH. On average, a reduction by 12% on the LCOH2 was possible by recovering O2, while WH was able to reduce the production costs by approximately 5%. This is mostly explained by the large quantities of O2 generated by the water electrolysis process, i.e., 88% of H2O mass becomes O2. Thus, assuming a price of 0.19 €/kg, O2 recovery substantially improves the economic performance of the process. When both O2 and WH are valorized, the LCOH2 is reduced on average by 17%.
However, diesel is still cheaper than H2 in all cases and years at the given prices. For instance, in 2020 the lowest LCOH2 found in case 3b (11.44 €/kg) was between 2.14 and 2.47 times higher than diesel with and without carbon tax respectively. In 2030, when diesel prices are expected to be 20% higher and H2 production costs 23% lower than in 2020 (case 3b with WH and O2 recovery), such differences are reduced to 1.15–1.33 times higher than diesel depending on the carbon tax scheme considered.
As clearly observed, purchasing diesel is cheaper than on-farm H2 production, for all PtH2 cases and technological scenarios considered. However, due to significant differences in terms of tank-to-wheel efficiency and purchase costs between FCAM and conventional diesel agricultural machinery, further analysis is required to understand the competitiveness of small-scale H2 production for farming activities.
Equivalent Annual Cost
The equivalent annual cost (EAC) was assessed as an additional economic indicator to understand the H2 system from the farmers' perspective. The EAC is used to compare the cost of owning fuel cell or diesel vehicles over the lifetime of the PtH2 plant. In addition, a scenario where farmers finance H2 production and use by means of leasing land to wind power project developers is also considered. Such a business model is considered advantageous for both parties: 1) farmers obtain additional revenues by leasing their land for wind power production; 2) wind power project developers potentially enhance their wind power production by selling curtailed electricity to farmers; 3) farmers can locally produce clean fuel to decarbonize their activities, and 4) support for the wind farm will likely be much greater with local involvement. The EAC according to the different farm cases and technological scenarios assessed are found in Figure 8.
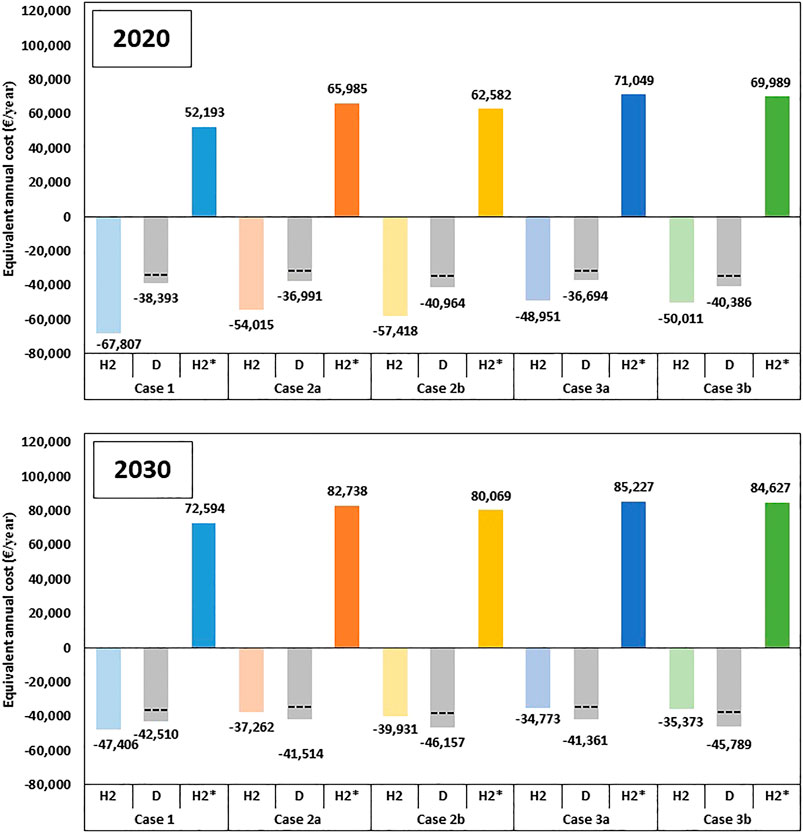
FIGURE 8. Equivalent annual cost (EAC) of the different cases assessed. Note: H2 means PtH2 without land lease revenues; D means diesel-fueled farm; H2* means PtH2 with land lease revenues; Values are presented per farm in Cases 2a,b, and 3a,b; black dotted lines represent EAC of diesel with tax relief; EAC values of PtH2 cases do not consider waste heat (WH) or O2 recovery.
Important differences were observed among the farm cases, in which sharing the PtH2 plant between two farms reduced the EAC by 21% on average, and sharing the PtH2 plant among four farms reduced annual costs by 27%. In contrast, no major benefits were found if farmers share the same diesel refueling infrastructure since the CAPEX of the diesel system is considerably lower than the H2 one. Nevertheless, EAC values associated with H2 production and use were always higher than conventional diesel farming for the period 2020 unless land lease revenues are counted.
Similarly to the LCOH2, the EAC of the H2 system will be considerably lower in 2030. In this case, annual costs would be around 30% lower compared to 2020 values. In the meantime, diesel prices are expected to increase by around 20%, reaching values between 1.40 and 1.62 €/L depending on the carbon tax scheme considered. Due to these factors, the EAC of the H2 system becomes competitive with diesel, except for case 1 which still will be more expensive. For case 2a, H2 becomes cheaper than diesel if farmers are not entitled to carbon tax relief on diesel consumption. For all remaining cases in 2030 H2 shows lower or equal EACs than diesel. Unsurprisingly, the case that presented the lowest EAC (3a–2030, no FCMV) was not the same case that showed the lowest LCOH2 (3b–2030, inc. FCMV). This is explained by the LCOH2 being inversely proportional to the amount of H2 produced (Eq. 5) which increases with the inclusion of FCMV demand, while the EAC is only marginally influenced by production via the NPV (Eq. 2-4). Thus, by adding the H2 fuel demand of FCMV, the increase in cost is greater than the savings produced from having H2 production, however, we did not compare this to diesel minivans as FCAM was the focus of this study.
When the revenues for leasing the land to wind power project developers are taken into account (6,000 €/MW/year), a major impact on the EAC is observed in favor of the H2 system. In this case, instead of having costs associated with agricultural machinery, farmers would have annual gains by operating the PtH2 plant in all farm cases and technological scenarios assessed. In cases where H2 production and use is less competitive than diesel, only a minor share of the land lease revenues would be required to make H2 competitive with diesel. For instance, under the current technological scenario, between 10–26% (600–1,560 €/MW/year) is needed to finance H2 production and use. In the 2030 scenario, only case 1 requires additional assistance from land lease revenues to make it competitive with diesel. In this case, the fraction of land lease revenues needed would be reduced from 26% to just 8% (498 €/MW/year).
Carbon Abatement Cost
The implementation of a farm-based PtH2 plant results in carbon emission reductions from different sources, namely direct fossil fuel displacement by H2, power consumption from the grid by recovering O2 from the electrolyser, and reductions in district heating use also by recovering WH from the electrolyser. As the latter two are dependent on local characteristics such as variable emission factor from the grid and use of fossil fuel in district heating systems, a simplified approach to calculate the cost of carbon mitigation was performed solely focusing on diesel displacement by the produced H2.
Considering a diesel consumption of 19,446 L/farm/year without FCMV, 27,131 L/farm/year with FCMV and the diesel emission factor of 2.64 kgCO2/L, the carbon emission reductions provided by the PtH2 plant could be estimated. In addition, the difference in EAC between H2 and diesel with and without carbon tax relief was used to calculate the carbon abatement cost of each case in different technological scenarios (Figure 9).
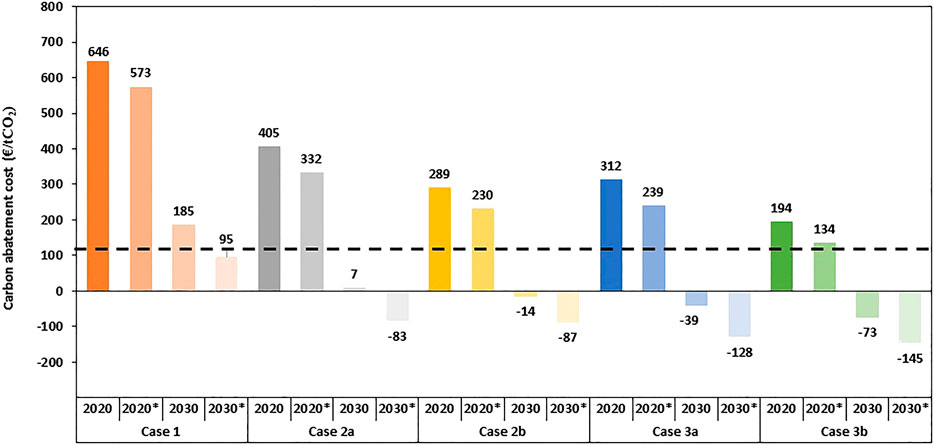
FIGURE 9. Carbon abatement cost of the different cases assessed. Note: * means without diesel tax relief; black dotted line represents the carbon tax in Sweden (2020).
It is possible to observe that under the current technological scenario without land lease revenues the carbon abatement cost is considered high, with values above 100 €/tCO2. The case 3b showed, however, the lowest carbon abatement cost in 2020 with values close to the current carbon tax in Sweden (110 €/tCO2), in particular if the diesel tax relief entitled to farming activities would be excluded. In fact, state subsidies and taxes often influence positively or negatively the cost efficiency of carbon abatement costs of different mitigating measures (Eory et al., 2018). For instance, incentives for the production and use of H2 could reduce its carbon abatement costs, but the existing tax relief on fossil fuel consumption prevents the adoption of low carbon fuels by the agricultural sector in Sweden.
In 2030, the carbon abatement costs are negative in most farm cases examined. Negative carbon abatement costs have been previously reported for different activities such as lighting switch, methane recovery from landfills, retrofit insulation in buildings, among others (McKinsey and Company, 2009). They owe negative values due to the advantage of having higher economic benefits than their implementation costs. In our case, this is translated by lower annual costs than diesel farming in most of 2030 cases. Such a favorable situation is not only due to the expected technological developments but also due to the 20% increase in diesel prices in the future. Thereby, emphasizing the importance of the price paid for diesel on the development of efficient climate protection strategies by policymakers.
Alternative Demand Profiles
As discussed in H2Demand and Optimization of H2Production, H2 demand has a major impact on the optimal plant configuration and performance of the H2 system. Where additional H2 consumers could be integrated, resulting in alternative demand profiles, significant improvements in terms of production costs could be achieved. For instance, in case 2b (2030) the LCOH2 of 11.03 €/kg is equivalent to a diesel price of 2.79 €/L, which is 2.4 times more expensive than currently found in retailers, including diesel tax relief. Such high production costs can be largely attributed to the low capacity factor of the PtH2 plant. If the H2 demand of FCMV in case 2b were to be multiplied by 10, i.e., 88.6 L of diesel eq. per day, a PtH2 plant with an electrolyser size of 200 kW and 18 m3 (500 bar) of capacity storage would be able to fulfill the demand of FCAM and FCMV, and at the same time operate during 4,241 h/year (48% of capacity factor). The PtH2 plant, thus, could lower H2 production costs to 7.04 €/kg in 2030, reaching a diesel equivalent price of 1.78 €/L (without by-products recovery). However, such a case is more akin to a small commercial filling station forecourt than a farm-based system and would require significant conversion of the local fossil fuel fleet to hydrogen fuel cells, or a medium-sized captive fleet.
Beyond sharing facilities across multiple farms as examined in this study, other farm types could be investigated for suitability for conversion to FCAM. In this case, ley crops for a dairy farm could show a more distributed H2 demand throughout the year, reducing gas storage requirements as well as allowing the PtH2 plant reach higher capacity factors. In addition, if these type of crops were integrated into a small pool of cereal-based farms sharing the same H2 production infrastructure, the seasonality of fuel demand observed in the current study would certainly be reduced, potentially resulting in better economic performances.
Ultimately, strategies similar to a demand-side management approach could be applied even to farmers sharing the same PtH2 plant with the same rotating crop system (e.g., present study). In this case, farmers could slightly adapt their agricultural operations to the availability of H2, in particular during fall for plowing operations. Such strategy is considered a key aspect to improve the economic performance and it should be addressed in future studies on small-scale green H2 production for agricultural applications.
Alternatively, H2 surplus to FCAM demand could also be injected into agricultural biogas plants in a so-called in-situ biomethanation concept (Voelklein et al., 2019). Such synergies with agricultural biogas plants could be explored in different ways: 1) to increase biomethane output of biogas plants by reacting H2 with CO2; and/or 2) to use H2 to partly displace costly energy crops as substrate like maize silage while keeping the same energy output of the biogas plant. Both concepts would increase the capacity factor of electrolysers and potentially decrease the costs of biogas production. However, care must be taken to ensure that the value of the methane-based H2 and the economies of scale it allows for are greater than the sum of the additional costs.
Small-scale Haber-Bosch process (minimum of 1.5 MW) for ammonia fertilizer production could also be explored to provide an alternative demand for H2 in the agricultural sector (Proton Ventures, 2018). This could quickly become the main demand for H2 and would provide the required economies of scale to result in a more competitive H2 either as fuel or platform for PtX processes.
Conclusions
This study examined the potential costs of an optimized system designed predominately to replace diesel-powered agricultural machinery with that powered by hydrogen (H2) fuel cells. Several scenarios or cases were examined which included the addition of fuel cell light-duty vans, the sharing of H2 facilities across neighboring farms, and valorization of the by-products (oxygen and waste heat). Results are presented in terms of levelized cost of hydrogen (LCOH2), equivalent annual cost (EAC) to the farmer (consumer), and carbon abatement cost. Even though sharing the same H2 facility among four farms decreased the LCOH2 by 28% and by adding fuel demand for delivery vans further decreased production costs by 35%, given the current cost of diesel and associated carbon taxes, H2 is not competitive in 2020. However, anticipated reductions in H2 costs coupled with increases in diesel prices mean that by 2030 H2 fuel cells may represent an economic option in many cases. Therefore, the carbon abatement costs varied drastically from −145 €/tCO2 when H2 becomes competitive with diesel in 2030, up to 646 €/tCO2 in 2020. Nevertheless, when a PtH2 plant is financed by the land lease revenues from a wind farm, H2 becomes more competitive than diesel in all analyzed scenarios. Managing the demand profiles to decrease H2 storage requirements and/or introducing an additional demand like for ammonia fertilizer production are effective strategies to reduce costs and should be addressed in future studies on H2 production for low carbon agriculture.
Data Availability Statement
All relevant data is contained within the article: The original contributions presented in the study are included in the article/supplementary material, further inquiries can be directed to the corresponding author.
Author Contributions
LJ: Conceptualization, Methodology, Software, Validation, Formal analysis, Investigation, Data curation, Writing–original draft, Writing–reviewing and editing, Visualization. SM: Conceptualization, Methodology, Software, Validation, Formal analysis, Investigation, Writing–original draft, Writing–reviewing and editing. SW: Methodology, Software, Validation, Writing–reviewing and editing. DN: Conceptualization, Methodology. PH: Conceptualization. ÅN: Conceptualization, Writing–reviewing and editing, Supervising.
Conflict of Interest
The authors declare that the research was conducted in the absence of any commercial or financial relationships that could be construed as a potential conflict of interest.
Acknowledgments
The authors would like to acknowledge Oscar Lagnelöv for providing the required data on fuel demand of agricultural machinery and Gabriel Åkerman for having initiated the development of PtH2 model.
Glossary
List of abbreviations
CAPEX capital expenditures
CCU carbon capture and utilization
CH4 methane
CO2 carbon dioxide
FCAM fuel cell agricultural machinery
FCMV fuel cell minivan
GHG greenhouse gas
H2 hydrogen
H2O water
KOH Potassium hydroxide
LCOH2 levelized cost of hydrogen
NCF net cash flow
NH3 Ammonia
NOH non-operating hours
NPV net present value
O2 oxygen
OPEX operational expenditures
PEM proton-exchange membrane
PSA pressure swing adsorption
PtH2 power-to-hydrogen
PtCH4 power-to-methane
PtX power-to-X
TRL technology readiness levels
VRE variable renewable electricity
WH waste heat
List of model parameters and symbols
Appendix A. Greenhouse Heat Demand
The produced waste heat was assumed to be used in a nearby greenhouse for tomato production. A simplified model was used to calculate the hourly demand for heat (H) per greenhouse ground area according to the method described below (García et al., 1998):
where
For appropriate growing conditions, the temperature in the greenhouse (
Based on weather data for the year 2019, it was observed a significant demand for heat in winter, spring, and autumn. Also, heat demand was observed in summer, however mostly during night time. Considering data between 1961 and 1990, the summertime in 2019 was around 2.0°C warmer than average values on Gotland, and the average temperature during 2019 was on average 2.1°C warmer than what could be considered as normal.
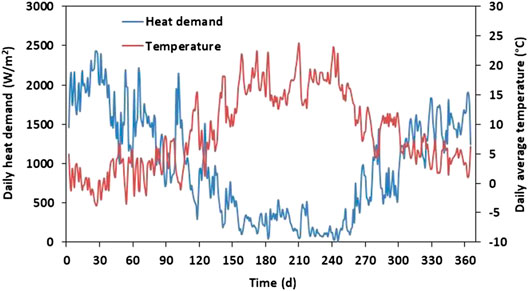
FIGURE A1. Simulated daily heat demand in a greenhouse for the production of tomatoes and the daily average air temperature on Gotland, Sweden.
Appendix B. Optimazation of All Cases Assessed
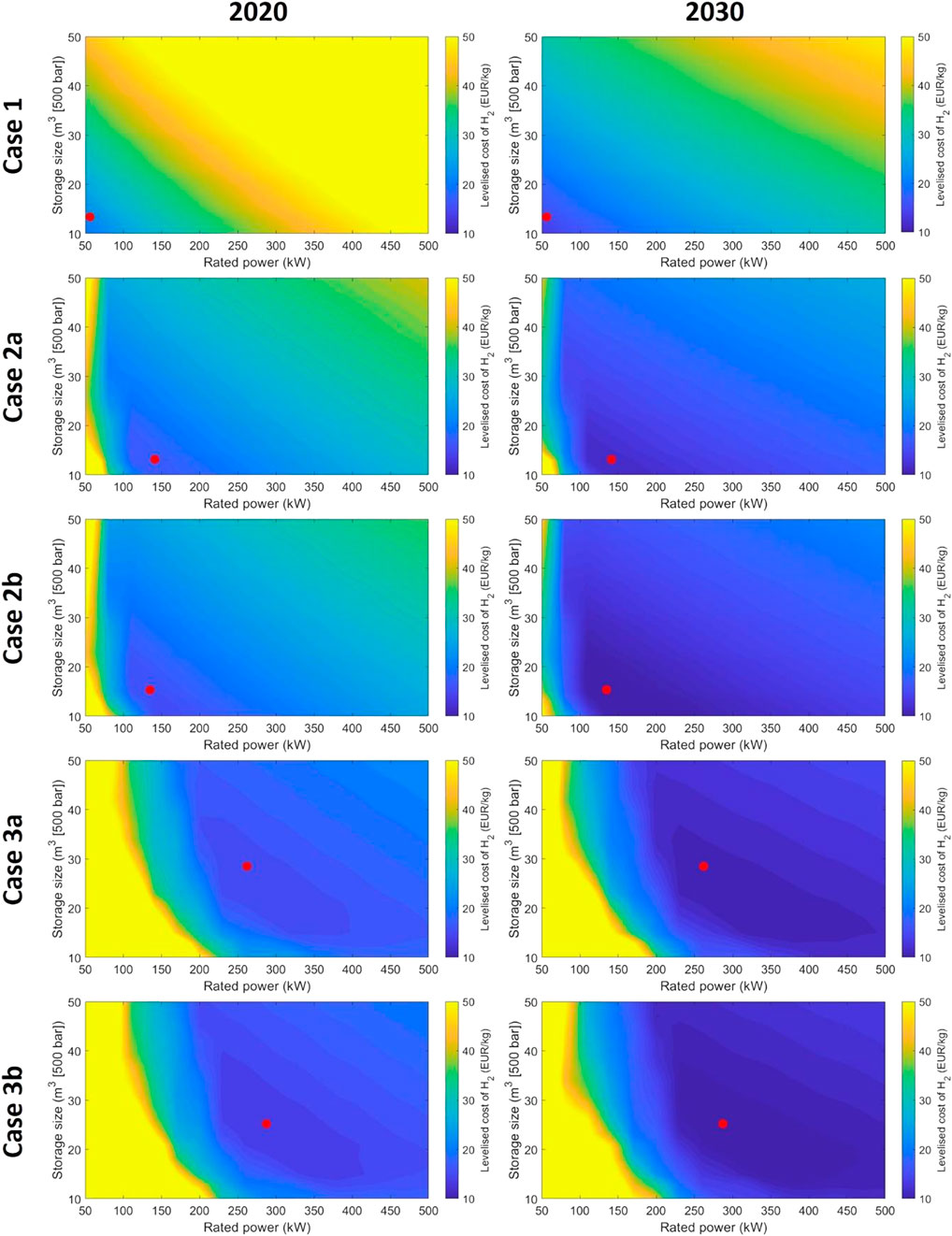
FIGURE A2. Optimization of PtH2 plant configuration (LCOH2). Note: Red dot shows the optimal plant configuration to minimize LCOH2.
References
Allman, A., and Daoutidis, P. (2018). Optimal scheduling for wind-powered ammonia generation: effects of key design parameters. Chem. Eng. Res. Des. 131, 5–15. doi:10.1016/j.cherd.2017.10.010
Allman, A., Daoutidis, P., Tiffany, D., and Kelley, S. (2017). A framework for ammonia supply chain optimization incorporating conventional and renewable generation. AIChE J. 63, 4390–4402. doi:10.1002/aic.15838
Apostolou, D., Enevoldsen, P., and Xydis, G. (2019). Supporting green urban mobility–the case of a small-scale autonomous hydrogen refuelling station. Int. J. Hydrog. Energy. 44, 9675–9689. doi:10.1016/j.ijhydene.2018.11.197
Aquaculture Technology (2020). Injection aerators for aeration, circulation, destratification and oxygenation. Available at: http://www.aquaculture-com.net/aeration.htm. (Accessed July 15, 2020).
Bailera, M., Peña, B., Lisbona, P., and Romeo, L. M. (2018). Decision-making methodology for managing photovoltaic surplus electricity through power to gas: combined heat and power in urban buildings. Appl. Energy. 228, 1032–1045. doi:10.1016/j.apenergy.2018.06.128
Bailera, M., Lisbona, P., Llera, E., Peña, B., and Romeo, L. M. (2019). Renewable energy sources and power-to-gas aided cogeneration for non-residential buildings. Energy. 181, 226–238. doi:10.1016/j.energy.2019.05.144
Benjaminsson, G., Benjaminsson, J., and Rudberg, R. (2013). Power-to-Gas: a technical review. Repport No.: 2013:284. Available at: http://sgc.camero.se/ckfinder/userfiles/files/SGC284_eng.pdf.
Bensinpriser.nu (2020). Diesel price on Gotland (Sweden). Available at: www.bensinpriser.nu (Accessed June 2, 2020).
Boyd, C. E. (2011). Dissolved oxygen requirements in aquatic animal respiration. Available at: https://www.aquaculturealliance.org/advocate/dissolved-oxygen-requirements-in-aquatic-animal-respiration/ (Accessed July 10, 2020).
Buttler, A., and Spliethoff, H. (2018). Current status of water electrolysis for energy storage, grid balancing and sector coupling via power-to-gas and power-to-liquids: a review. Renew. Sustain. Energy Rev. 82, 2440–2454. doi:10.1016/j.rser.2017.09.003
Byman, K. (2015). Locational study—Power to gas. Available at: http://www.energiforsk.se/program/energigasteknik/rapporter/locational-study-power-to-gas/.
Carroquino, J., Roda, V., Mustata, R., Yago, J., Valiño, L., Lozano, A., et al. (2018). Combined production of electricity and hydrogen from solar energy and its use in the wine sector. Renew. Energy. 122, 251–263. doi:10.1016/j.renene.2018.01.106
de Bucy, J., Lacroix, O., and Jammes, L. (2016). The potential of power-to-gas. Available online: https://www.enea-consulting.com/en/the-potential-of-power-to-gas/ (Accessed April 29, 2019).
Eory, V., Pellerin, S., Carmona Garcia, G., Lehtonen, H., Licite, I., Mattila, H., et al. (2018). Marginal abatement cost curves for agricultural climate policy: state-of-the art, lessons learnt and future potential. J. Clean. Prod. 182, 705–716. doi:10.1016/j.jclepro.2018.01.252
Fischer, D., Kaufmann, F., Hollinger, R., and Voglstätter, C. (2018a). Real live demonstration of MPC for a power-to-gas plant. Appl. Energy. 228, 833–842. doi:10.1016/j.apenergy.2018.06.144
Fischer, D., Kaufmann, F., Selinger-Lutz, O., and Voglstätter, C. (2018b). Power-to-gas in a smart city context-influence of network restrictions and possible solutions using on-site storage and model predictive controls. Int. J. Hydrogen Energy. 43, 9483–9494. doi:10.1016/j.ijhydene.2018.04.034
Frank, E., Gorre, J., Ruoss, F., and Friedl, M. J. (2018). Calculation and analysis of efficiencies and annual performances of power-to-gas systems. Appl. Energy. 218, 217–231. doi:10.1016/j.apenergy.2018.02.105
García, J. L., De La Plaza, S., Navas, L. M., Benavente, R. M., and Luna, L. (1998). Evaluation of the feasibility of alternative energy sources for greenhouse heating. J. Agric. Eng. Res. 69, 107–114. doi:10.1006/jaer.1997.0228
Grueger, F., Möhrke, F., Robinius, M., and Stolten, D. (2017). Early power to gas applications: reducing wind farm forecast errors and providing secondary control reserve. Appl. Energy. 192, 551–562. doi:10.1016/j.apenergy.2016.06.131
Grüger, F., Dylewski, L., Robinius, M., and Stolten, D. (2018). Carsharing with fuel cell vehicles: sizing hydrogen refueling stations based on refueling behavior. Appl. Energy. 228, 1540–1549. doi:10.1016/j.apenergy.2018.07.014
Grüger, F., Hoch, O., Hartmann, J., Robinius, M., and Stolten, D. (2019). Optimized electrolyzer operation: employing forecasts of wind energy availability, hydrogen demand, and electricity prices. Int. J. Hydrogen Energy. 44, 4387–4397. doi:10.1016/j.ijhydene.2018.07.165
Guelpa, E., and Verda, V. (2019). Thermal energy storage in district heating and cooling systems: a review. Appl. Energy. 252, 113474. doi:10.1016/j.apenergy.2019.113474
Hanley, E. S., Deane, J. P., and Gallachóir, B. P. Ó. (2018). The role of hydrogen in low carbon energy futures–a review of existing perspectives. Renew. Sustain. Energy Rev. 82, 3027–3045. doi:10.1016/j.rser.2017.10.034
IVL (2012). Petrol costs in 2030. Available at: https://www.ivl.se/toppmeny/pressrum/nyheter/nyheter---arkiv/2012-10-18-sa-mycket-kostar-bensinen-ar-2030.html (Accessed June 5, 2020).
Janke, L., McDonagh, S., Weinrich, S., Murphy, J., Nilsson, D., Hansson, P-A., et al. (2020). Optimizing power-to-H2 participation in the Nord Pool electricity market: effects of different bidding strategies on plant operation. Renew. Energy. 156, 820–836. doi:10.1016/j.renene.2020.04.080
Koohi-Fayegh, S., and Rosen, M. A. (2020). A review of energy storage types, applications and recent developments. J. Energy Storage. 27, 101047. doi:10.1016/j.est.2019.101047
Lagnelöv, O., Larsson, G., Nilsson, D., Larsolle, A., and Hansson, P. (2020). Performance comparison of charging systems for autonomous electric field tractors using dynamic simulation. Biosyst. Eng. 194, 121–137. doi:10.1016/j.biosystemseng.2020.03.017
Linde (2017a). Pure Water Knowledge: gases and application technologies for water treatment. Available at: http://www.linde-gas.com/watertreatment
Linde (2017b). Taking Oxygenation to a new level: innovative aquaculture solutions. Available at: http://www.linde-gas.lv/.
Mariani, L., Cola, G., Bulgari, R., Ferrante, A., and Martinetti, L. (2016). Space and time variability of heating requirements for greenhouse tomato production in the Euro-Mediterranean area. Sci. Total Environ. 562, 834–844. doi:10.1016/j.scitotenv.2016.04.057
McDonagh, S., O’Shea, R., Wall, D. M., Deane, J. P., and Murphy, J. D. (2018). Modelling of a power-to-gas system to predict the levelised cost of energy of an advanced renewable gaseous transport fuel. Appl. Energy. 215, 444–456. doi:10.1016/j.apenergy.2018.02.019
McDonagh, S., Deane, P., Rajendran, K., and Murphy, J. D. (2019). Are electrofuels a sustainable transport fuel? Analysis of the effect of controls on carbon, curtailment, and cost of hydrogen. Appl. Energy. 247, 716–730. doi:10.1016/j.apenergy.2019.04.060
McDonagh, S., Wall, D. M., Deane, P., and Murphy, J. D. (2019). The effect of electricity markets, and renewable electricity penetration, on the levelised cost of energy of an advanced electro-fuel system incorporating carbon capture and utilisation. Renew. Energy. 131, 364–371. doi:10.1016/j.renene.2018.07.058
McDonagh, S., Ahmed, S., Desmond, C., and Murphy, J. D. (2020). Hydrogen from offshore wind: investor perspective on the profitability of a hybrid system including for curtailment. Appl. Energy. 265, 114732. doi:10.1016/j.apenergy.2020.114732
McGreevy, R. (2013). Midlands landowners offered €18,000 a year per wind turbine. Ir. Times. 1. Available at: https://www.irishtimes.com/news/midlands-landowners-offered-18-000-a-year-per-wind-turbine-1.1320270 (Accessed March 15, 2020).
McKinsey and Company (2009). Pathways to a low-carbon economy: version 2 of the global greenhouse abatement cost curve.New York, USA: McKinsey and Company.
Mohseni, F., Görling, M., Llindén, M., and Larsson, M. (2017). Genomförbarhetsstudie för power to gas på Gotland. Stockholm: SWECO, 378.
Moradi, R., and Groth, K. M. (2019). Hydrogen storage and delivery: review of the state of the art technologies and risk and reliability analysis. Int. J. Hydrogen Energy. 44, 12254–12269. doi:10.1016/j.ijhydene.2019.03.041
Moreda, G. P., Muñoz-García, M. A., and Barreiro, P. (2016). High voltage electrification of tractor and agricultural machinery-a review. Energy Convers. Manag. 115, 117–131. doi:10.1016/j.enconman.2016.02.018
NHA (2012). New Holland’s NH2 fuel cell powered tractor to enter service. Fuel Cell. Bull. 2012, 3–4. doi:10.1016/s1464-2859(12)70004-4
Nilsson, D., and Hansson, P-A. (2001). Influence of various machinery combinations, fuel proportions and storage capacities on costs for co-handling of straw and reed canary grass to district heating plants. Biomass Bioenergy. 20, 247–260. doi:10.1016/S0961-9534(00)00077-5
NordPool (2019). Historical market data. Lysaker (Norway). Available at: https://www.nordpoolgroup.com/historical-market-data/ (Accessed November 15, 2019).
Novo, A. V., Bayon, J. R., Castro-Fresno, D., and Rodriguez-Hernandez, J. (2010). Review of seasonal heat storage in large basins: water tanks and gravel-water pits. Appl. Energy. 87, 390–397. doi:10.1016/j.apenergy.2009.06.033
Paulsson, R., Djodjic, F., Ross, C., and Hjerpe, K. (2015). Nationell jordartskartering-Matjordens egenskaper i åkermarken. Report No.: 2015:19.
Proost, J. (2019). State-of-the art CAPEX data for water electrolysers, and their impact on renewable hydrogen price settings. Int. J. Hydrogen Energy. 4, 4406–4413. doi:10.1016/j.ijhydene.2018.07.164
Proton Ventures B. V. (2018). Sustainable ammonia for food and power. Nitrogen. 1–10. Available at: https://www.protonventures.com/wp-content/uploads/2018/09/NS-354-Small-scale-plant-design-PROTON-VENTURES-3-1.pdf (Accessed April 1, 2020).
Roda, V., Carroquino, J., Valiño, L., Lozano, A., and Barreras, F. (2018). Remodeling of a commercial plug-in battery electric vehicle to a hybrid configuration with a PEM fuel cell. Int. J. Hydrogen Energy. 43, 16959–16970. doi:10.1016/j.ijhydene.2017.12.171
Safa, M., Samarasinghe, S., and Mohssen, M. (2010). Determination of fuel consumption and indirect factors affecting it in wheat production in Canterbury, New Zealand. Energy. 35, 5400–5405. doi:10.1016/j.energy.2010.07.015
Schmidt, O., Gambhir, A., Staffell, I., Hawkes, A., Nelson, J., and Few, S. (2017). Future cost and performance of water electrolysis: an expert elicitation study. Int. J. Hydrogen Energy. 42, 30470–30492. doi:10.1016/j.ijhydene.2017.10.045
Skatteverket (2020). Tax refund on electrticity and fuel. Available at: https://www.skatteverket.se/foretagochorganisationer/skatter/punktskatter/energiskatter/aterbetalningavskattpaelochbransle.4.109dcbe71721adafd252816.html.
SMHI (2017). Historical wind speed measurements at Visby Flygplats. Available at: https://www.smhi.se/en (Accessed October 10, 2019).
SMHI (2020). Metereological observations at Visby Flygplats 2020. Available at: https://www.smhi.se/data/meteorologi/ladda-ner-meteorologiska-observationer/#param=precipitation24HourSum.
Stadkraft (2020). Wind parks in Sweden. Available at: https://www.statkraft.se/energikallor/kraftverk/sverige/bjorkhojden-wind-farm/ (Accessed March 15, 2020).
Thornton, G. (2019). Renewable energy discount rate survey result–2018. Available at: (Accessed November 15, 2019).
https://doi.org/https://www.grantthornton.co.uk/en/insights/renewable-energy-discount-rate-survey-2018/ | Google Scholar
Törnfet, A., and Nypelius, A. (2020). Vätgas och energilagring. Romakloster (Sweden). Available at: https://www.lrf.se/mitt-lrf/regioner/gotland/aktuellt-arbete/projekt1/ (Accessed June 1, 2020).
Ulleberg, Ø., and Hancke, R. (2020). Techno-economic calculations of small-scale hydrogen supply systems for zero emission transport in Norway. Int. J. Hydrogen Energy. 45, 1201–1211. doi:10.1016/j.ijhydene.2019.05.170
Vestas (2019). Specifications of wind turbine Vestas V90 2MW. Available at: https://en.wind-turbine-models.com/turbines/1681-hummer-h25.0-200kw (Accessed January 5, 2020).
Voelklein, M. A., Rusmanis, D., and Murphy, J. D. (2019). Biological methanation: strategies for in-situ and ex-situ upgrading in anaerobic digestion. Appl. Energy. 235, 1061–1071. doi:10.1016/j.apenergy.2018.11.006
Wallnerström, C. J., and Bertling Tjernberg, L. (2018). “11 -analysis of the future power systems’s ability to enable sustainable energy—using the case system of Smart Grid Gotland,” in Application of small grid technologies. Editors L. A. Lamont and Sayigh, A. (Stockholm: Academic Press), 373–393.
Wiederholm, J., Castegren, G., Ulaner, M., Persson, M.-L., and Lindbäck, M. (2018). Nils Holgerssons underbara resa genom Sverige. Stockholm: Nils Holgerssongruppen.
Witney, B. (1988). Choosing and using farm machines. Madison, WI: Longman Scientific and Technology.
Woynarovich, A., Hoitsy, G., and Moth-Poulsen, T. (2011). Small-scale rainbow trout farming. Rome: FAO.
Wu, D., Ren, J., Davies, H., Shang, J., and Haas, O. (2019). Intelligent hydrogen fuel cell range extender for battery electric vehicles. World Electr. Veh. J. 10, 181573753. doi:10.3390/wevj10020029
Yan, M., Cheng, K., Luo, T., Yan, Y., Pan, G., and Rees, R. M. (2015). Carbon footprint of grain crop production in China - based on farm survey data. J. Clean. Prod. 104, 130–138. doi:10.1016/j.jclepro.2015.05.058
Keywords: green hydrogen, modeling and simulation, process optimization, techno-economic assessment, CO2 emission reduction
Citation: Janke L, McDonagh S, Weinrich S, Nilsson D, Hansson P-A and Nordberg Å (2020) Techno-Economic Assessment of Demand-Driven Small-Scale Green Hydrogen Production for Low Carbon Agriculture in Sweden. Front. Energy Res. 8:595224. doi: 10.3389/fenrg.2020.595224
Received: 15 August 2020; Accepted: 20 October 2020;
Published: 26 November 2020.
Edited by:
Benedetto Nastasi, Sapienza University of Rome, ItalyReviewed by:
Manuel Bailera, University of Zaragoza, SpainQingchun Yang, Hefei University of Technology, China
Copyright © 2020 Janke, McDonagh, Weinrich, Nilsson, Hansson and Nordberg. This is an open-access article distributed under the terms of the Creative Commons Attribution License (CC BY). The use, distribution or reproduction in other forums is permitted, provided the original author(s) and the copyright owner(s) are credited and that the original publication in this journal is cited, in accordance with accepted academic practice. No use, distribution or reproduction is permitted which does not comply with these terms.
*Correspondence: Leandro Janke, bGVhbmRyby5qYW5rZUBzbHUuc2U=