- 1State Grid Jilin Electric Power Company Limited, Changchun, China
- 2Department of Electrical Engineering, Northeast Electric Power University, Jilin, China
Power-to-gas (P2G) facilities and natural gas fired power units provide flexibility to integrated electricity and natural gas systems (IENGS) for wind power accommodation and ramp deployment. This paper proposes a stochastic coordinated scheduling model for IENGS considering ramping costs with P2G storage and wind power. The operation model of natural gas system with P2G is presented, and the benefits of P2G integration are analyzed. To address the uncertainties of wind power and energy loads, multiple representative scenarios are generated. The flexible ramping requirements and costs are incorporated and analyzed, and flexible ramp can be provided by P2G in this work. The coordinated scheduling model for IENGS is formulated as a two-stage stochastic programming problem, in which day-ahead scheduling for electricity systems is modeled in the first stage model and scheduling of natural gas systems is carried out in the second stage model. Numerical case studies on a modified PJM 5-bus electricity system with a 7-node natural gas system and the IEEE 118-bus system with a 20-node Belgian natural gas system verify that P2G can help accommodate wind power, provide additional flexible ramping capacities, and reduce the gas supply from gas suppliers and gas load shedding.
Introduction
In the last decade, the use of natural gas for power generation has increased significantly throughout the world (Zhang et al., 2020). The growth of natural gas-fired generation increases the interaction between electricity systems and natural gas systems (Li et al., 2008; Chaudry et al., 2014), and, together with renewable generation, makes the operation of electricity systems more environmentally friendly (Zhang et al., 2016).
In integrated electricity and natural gas systems (IENGS), the integration of large-scale intermittent and uncertain renewable energy brings major challenges to the operation of the electricity system. Curtailment of renewable energy frequently occurs due to the lack of system flexibility. As the variability increases, fast-ramp thermal units can provide flexible ramp deployment to maintain power balance (Wang et al., 2008). The fast ramping capacities of gas-fired generation units can provide operational flexibility (Baldick, 2014). In (Zhang et al., 2016) and (Alabdulwahab et al., 2015), stochastic day-ahead scheduling and security-constrained unit commitment models are proposed, respectively, and the flexibility and quick ramping capability of gas-fired generation units demonstrate the possibility of firming the variability of wind power when it is constrained by the natural gas network.
Power-to-gas (P2G) technology can achieve the conversion of electric energy to hydrogen or synthetic natural gas (SNG). Gas-fired generation units and P2G facilities realize bidirectional coupling of IENGS (Clegg and Mancarella, 2016; He et al., 2017). P2G technology is treated as a promising approach to realize high penetration of renewables and low carbon emission in (Li et al., 2017a; Yang et al., 2019). The electricity consumption of P2G can reshape the load profile, reducing wind power curtailment in valley periods and the load difference between peak and valley periods. The SNG produced as a result can then be stored or utilized in the gas network. Some existing publications have studied the operation strategy for P2G. Day-ahead scheduling of P2G storage is studied in (Khani and Farag, 2018), in which P2G storage is optimally scheduled in both electricity and gas markets. In (Clegg and Mancarella, 2015), a methodology to investigate various P2G processes is proposed and their impacts on electricity and gas networks are analyzed. In (Alkano and Scherpen, 2018), the coordination of supply from P2G facilities is studied based on a model predictive control approach. The above works verify that P2G with storage can reduce renewable energy curtailment and that P2G can play a role in the operation of natural gas systems. P2G can also help ramp by regulating the amount of consumed electricity energy. However, the benefit of P2G on flexible ramp deployment has not been considered in the existing works.
Variability and uncertainty of large-scale wind power can be handled by ramping capacities of thermal units, including gas-fired generation units. The fast ramping capability of gas-fired units makes them an important resource for providing flexibility and addressing uncertainties in a power system. Thus, the natural gas network needs to be optimally scheduled to ensure an available fuel supply for the gas-fired units to address any realization of uncertain scenarios. Moreover, the ramping costs should be included. The existing works on coordinated optimal operation of IENGS mainly focus on integrated planning (Zhang et al., 2015; Zhang et al., 2016), day-ahead scheduling (Liu et al., 2010; Alabdulwahab et al., 2015; Bai et al., 2016; He et al., 2017), unit commitment (Wang et al., 2008), and reserve scheduling (Liu et al., 2019). However, the optimal scheduling of natural gas system is barely considered.
To bridge these gaps, a coordinated stochastic scheduling model of IENGS with P2G and wind power is proposed in this paper. The primary goal of this work is to carry out coordinated stochastic optimal scheduling of IENGS utilizing P2G storage to promote wind power integration and reduce ramping costs for electricity systems. The major contributions are summarized as below.
(1) Compared to the work in (Li et al., 2017b), the coordinated scheduling model is formulated as a two-stage stochastic programming problem. Uncertainties of wind power, electricity loads, and natural gas loads are considered. Day-ahead stochastic scheduling of electricity systems is carried out in the first stage to determine the gas loads of gas-fired generation units and produced SNG of P2G for all scenarios, which are utilized in the second stage optimization model. Stochastic scheduling of natural gas systems is modeled in the second stage model to check the feasibility of natural gas transmission for all scenarios and optimally schedule the gas supply and P2G storage. The two stage models are formulated as a linear programming (LP) problem and a mixed-integer linear programming (MILP) problem, respectively.
(2) The benefits of the integration of P2G storage for IEGNS are analyzed in this paper. For electricity systems, a P2G facility can regulate its power consumption to change the electricity load profile to reduce wind power curtailments and ramping costs. For natural gas systems, the produced SNG in P2G storage can participate in the operation of natural gas systems to reduce the gas consumption from natural gas suppliers and help reduce gas load shedding, especially for loads of gas-fired generation units under uncertain scenarios.
(3) Flexible ramping requirements and costs are analyzed and presented. Ramping costs are considered, and flexible ramps can be provided by P2G storage. Natural gas network constraints with P2G storage are considered, and an evaluation of the optimal scheduling of gas suppliers and storage is conducted.
The rest of this paper is organized as follows. In Natural Gas Networks Model With Power-to-Gas Storage, a model of a natural gas network with P2G is presented. Uncertainties of Wind Power Sources and Energy Loads and Flexible Ramp presents the uncertainties of wind power, energy loads, and flexible ramps. In Stochastic Coordinated Scheduling Model, the stochastic day-ahead scheduling model is formulated, and the solution approach is proposed. In Case Studies, case studies are conducted to demonstrate the effectiveness of the model. Concluding remarks are presented in Conclusion.
Natural Gas Networks Model With Power-to-Gas Storage
Natural Gas Network
In natural gas systems, the network consists of gas wells (gas suppliers), pipelines, storage, and compressors. The majority of natural gas is produced from gas wells. The gas supply Sj from gas wells is limited by upper and lower boundaries Sj,max and Sj,min, as shown in Eq. 1:
The pipeline flow Fmj relates to pressures of node m and j in Eq. 2 and the nodal pressure is constrained in Eq. 3:
where sgn () represents sign function, which shows gas flows from a high pressure node to a low pressure node.
The compressor can be driven by power from the electricity power grid (Li et al., 2017b). The power consumption is:
Power-to-Gas Process
P2G facilities are suppliers in natural gas systems and loads in electricity systems. SNG produced by P2G facilities can be stored for use in later scheduling periods. Faster ramping rates of P2G facilities with the technology of a proton exchange membrane (PEM) can better follow power fluctuations (Grond et al., 2013; Clegg and Mancarella, 2015). In this paper, the motivation behind studying P2G and the benefits of considering P2G can be summarized as follows:
(1) From the perspective of wind power accommodation, P2G can reduce wind power curtailment by increasing electricity load. In valley electricity load periods, thermal power units cover a large portion of the power load due to lower output limits and spinning reserve constraints, resulting in a heavy curtailment of wind power. P2G can utilize the surplus wind power to generate SNG.
(2) The power consumption regulating ability of P2G can provide additional flexible ramping capacities to account for power fluctuations and uncertainties. During periods in which thermal units should ramp down dramatically to follow power load and wind power fluctuation and uncertainty, P2G can increase load values and hence reduce ramping requirements.
(3) The SNG generated by P2G can be utilized in a natural gas system to reduce gas consumption from natural gas suppliers and may help to reduce gas load shedding when the P2G storage is integrated at certain nodes. The operation constraints of P2G are stated as Eqs 6–8
The energy conversion process of P2G can be described by Eq. 6, and volumetric quantity is calculated by Eq. 7. The hourly produced SNG should be positive and limited by the upper bounds in Eq. 8.
Uncertainties of Wind Power Sources and Energy Loads and Flexible Ramp
In this section, uncertainties of wind power, electricity loads, and natural gas loads are represented by a set of scenarios. The flexible ramping requirements and costs are also analyzed.
Wind Power and Energy Loads Uncertainty and Sampling Method
Uncertainties of wind power, electricity loads, and natural gas loads with inevitable forecasting errors are modeled based on probability distribution functions. Normal distribution function has been utilized to obtain hourly wind power and energy load forecasting errors.
To simulate the uncertainties of wind power and electricity and natural gas loads, the Monte Carlo method is utilized to generate proper number of uncertain scenarios. Large numbers of scenarios would increase the computation burden, and scenario reduction techniques should be utilized. The scenario reduction algorithms would determine a scenario subset and assign new probabilities to the preserved scenarios. In this paper, the SCENRED tool in the General Algebraic Modeling System (GAMS) is executed for scenario reduction process, which contains several reduction algorithms. In this paper, fast backward reduction method is employed. The probabilities for all generated scenarios before reduction are assumed to be the same, with a cumulative sum equal to one (ΣPs = 1). After reduction, smaller number of scenarios would be obtained with corresponding probabilities.
Flexible Ramping Requirements and Costs
Controllable thermal power units in traditional power systems are adjusted to follow load fluctuations. However, the integration of renewable energy sources (RES) increases variability in power system operation. The net load, which equals to total load minus total available RES generation, is more volatile and requires more ramping capability from thermal units between operating periods in case of power imbalance. Moreover, the forecasting errors of loads and RES should also be balanced by upward and downward flexible ramping of thermal units. Hence, the flexible ramping requirements of thermal units include the following two parts:
• Flexible ramping requirements must follow hourly net load fluctuation. Thermal generation units ramp up/down to follow net load fluctuation between scheduling periods. The more volatile the net load is, the more ramping requirements are expected. The ramping capacity of a thermal generation unit is constrained by its ramping rate and minimum/maximum output limits. In the day-ahead electricity market, the flexible ramping offer is included in a generator's offer and cleared at the bidding prices of up/down ramping together with the energy offer (Wu et al., 2015). The costs for flexible ramping between adjacent scheduling periods are calculated as:
• Flexible ramping requirements must guarantee secure operation under uncertainties of load and RES, as represented in the uncertain scenarios. In each scenario, a ramping cost similar to that of the base case is considered. Moreover, flexible ramping can ensure a transition from the scheduled operation status of the base case to all possible scenarios. The feasible corrective dispatch of thermal units from the base case to all possible scenarios ensures the power balance, but the ramp offer should be optimally determined and allocated. The corrective ramping costs are calculated as:
The bidding prices of flexible ramping are assumed to be the same as up and down ramping costs in the base case.
The total ramping costs are shown as:
Remark 1: Natural gas-fired units can provide operational flexibility through their fast-ramp capacities. However, the power output of gas-fired units relates to natural gas consumption, which is constrained by the limits of the natural gas network. So the flexible ramp provided by natural gas-fired units is also constrained, especially in peak natural gas load periods. In these periods, heavy nodal loads lead to violations of the natural gas network, such as the nodal pressure of an end node of long pipeline falling below its lower limit.
Stochastic Coordinated Scheduling Model
In this section, the proposed two stage stochastic scheduling model is formulated.
First Stage Model
The objective function of the first stage stochastic scheduling model for electricity system is to minimize the expected operation cost of the electricity system, including electricity bidding costs, wind power curtailment costs, ramping costs and P2G operation costs, as shown in Eq. 12.
Objective Function
Constraints
For each scenario s, the electricity balance constraint is shown in Eq. 13, power generation constraints of thermal units and wind power are shown in Eqs 14 and 15. Ramping and spinning reserve constraints are presented in Eqs 18–21. Constraint Eq. 19 ensures the transition of operation status from the base case to all scenarios. Transmission constraints based on a DC power flow model are shown in Eq. 22.
Second Stage Model
In the second stage, the stochastic scheduling for natural gas systems is carried out based on the scheduling results of the first stage model. The hourly gas loads (including those of gas fired power units) are optimally allocated among different gas suppliers according to various bidding prices. Moreover, P2G storage can participate in the optimal operation of natural gas systems. The storage can charge gas flow from the P2G process and gas network and discharge it as gas supply.
Objective Function
The objective function of the stochastic scheduling model of natural gas systems is to minimize the expected purchasing costs. The objective function is formulated as:
Constraints
Constraints Eqs 1–5 in each scenario s:
Natural gas balance:
Constraints of gas storage integrated with P2G:
Eq. 25 gives the calculation of state of charge (SOC) of P2G storage.
To handle the nonlinear constraints of natural gas pipeline flow, the linearization method of our previous work (Li et al., 2017b) is introduced in this paper, and the second stage scheduling model can be formulated as a MILP problem.
Solution Approach
The flow chart of the solution approach for the proposed stochastic scheduling model is shown in Figure 1. Major steps of this method are summarized as follows.
Step 1: Input data of networks, wind power and energy loads in IENGS. The initial stochastic scenarios are generated by Monte Carlo. In this paper, the SCENRED tool in the GAMS is applied to perform scenario reduction to reduce computation burden (GAMS/SCENRED Documentation, 2002), and the fast backward reduction method is employed.
Step 2: Solve the first stage stochastic scheduling model of the electricity system considering P2G and ramping costs, which is formulated as a LP problem and solved to obtain gas demand of gas-fired power units and SNG produced by P2G.
Step 3: Solve the second stage stochastic optimal scheduling model of natural gas systems, which is formulated as a MILP problem and the variable of virtual load shedding of natural gas is introduced to ensure that the second stage scheduling problem is feasible.
Step 4: Given the results, if the load shedding results of gas-fired units are not equal to 0, the gas demand of gas-fired units would be modified based on the load shedding results and added into the first stage model with fixed values.
Step 5: The first stage model would be resolved until the load shedding results of gas-fired units in the second stage model equal to 0, and the results would be output and analyzed.
Remark 2: It’s noted that the modified gas demand of gas-fired units would lead to different scheduling results of P2G. To ensure the operation feasibility of P2G storage in a natural gas network, the created reserve margin
The scheduling model is implemented in GAMS, and the LP and MILP optimization models are solved utilizing CPLEX.
Case Studies
In this section, the proposed day-ahead stochastic scheduling model is performed on a PJM 5-bus power system integrated with a 7-node natural gas system and on the IEEE 118-bus power system with the Belgian natural gas system.
PJM 5-Bus System
Figure 2 provides a diagram of the utilized IENGS network including a PJM 5-bus power system integrated with a seven-node natural gas system, in which the generation capacities and bidding prices are also shown. The ramping bids factor is 20%, which means ramping bids are 20% of bidding prices of thermal units. A wind farm with a capacity of 400 MW is installed at Bus A, and the bidding cost is set to $8 per MW. The P2G operation cost coefficient is $2 per MW and the wind power curtailment cost coefficient is $60 per MW. Unit one and two at Bus A are assumed to be gas-fired power units, which are integrated at NG one and three in the natural gas network. The residential and industrial natural gas load is distributed to three load buses. The detailed parameters of the natural gas system can be found in (Liu et al., 2009). The initial state of P2G storage is 2300 kcf. The up and down spinning reserve requirements are 0.1 of hourly forecasting electricity load. The forecasted output of wind power and energy loads (including residential natural gas loads and electricity loads) are shown in Figure 3. The wind power and energy loads forecasting errors are simulated by 1,000 scenarios generated by Monte Carlo simulation. The number of scenarios is reduced to seven in GAMS.
To verify the impact of P2G on the scheduling results of IENGS, the following two cases are conducted:
Case 1: Stochastic scheduling with P2G and storage.
Case 2: Stochastic scheduling without P2G but with storage. The gas storage is integrated in the natural gas system.
The scheduling results of the two cases are compared to illustrate the impact of P2G on the electricity system and the natural gas system, respectively.
Impact of Power-to-Gas on Electricity System
The impact of P2G on electricity systems primarily assists in wind power accommodation and provides additional flexible ramping capacities to account for power fluctuations and uncertainties by increasing electricity load in certain periods. The results of scheduled wind power and the electricity consumed by P2G in Case 1 and 2 in the base case are compared in Figure 4. As depicted in Figure 4, more wind power is scheduled in Case 1 in periods 1, 2, 4–6 and 22 compared to Case 2. It can be seen that in those periods, which are off-peak hours, the P2G facility consumes power to increase electricity load and more wind power can be utilized in Case 1, while wind power would be curtailed in Case 2. The results verify that P2G can reduce wind power curtailments and aid wind power accommodation.
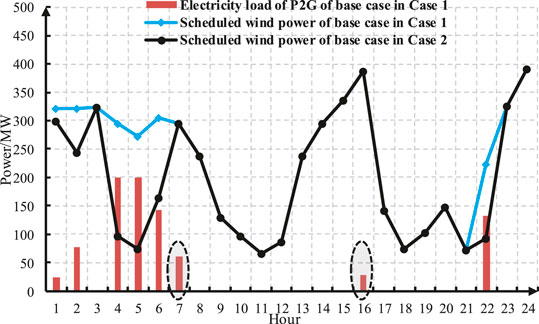
FIGURE 4. Comparison of scheduled wind power and electricity load of P2G in Case 1 and 2 in base case.
It should be noted that in periods 7 and 16 in Figure 4, the utilized wind power is the same in Case 1 and 2, but P2G facility consumes a certain amount of electricity. The reason is that the flexible ramping cost is considered in the proposed scheduling model, and in these periods P2G can provide additional flexible ramping capacities to reduce ramping costs. Moreover, electricity load by P2G can flatten the net load profile by reducing the difference between peak and valley net loads, which also reduces the ramping requirements across the scheduling horizon. A comparison of net load profiles in Case 1 and 2 is shown in Figure 5. Hourly net load in Case 1 equals to fixed electricity load plus the electricity load of P2G and minus wind power forecasting value, while Case 2 does not include a P2G electricity load. The net load curve in Case 1 fluctuates between a smaller range than the Case 2 curve. The difference between peak and valley loads is reduced from 842.86 to 642.86 MW. The above results show that a P2G facility can provide additional flexible ramping capacities and flatten the net load profile.
To further demonstrate the impact of P2G on the scheduling of electricity systems, a comparison of the scheduling results of Case 1 and 2 is shown in Table 1. Comparing Case 1 and 2, the expected total cost in Case 1 is reduced to $8.24 * 105 from $9.06 * 105 of Case 2. The expected ramping cost in Case 1 is lower than that of Case 2, which demonstrates that P2G can help reduce the ramping cost. The wind power utilization rate in Case 1 is increased 13.8% compared to Case 2.
The above results illustrate that integration of P2G facility can reduce wind power curtailment and provide additional flexible ramping capacities to reduce ramping costs for electricity systems.
Impact of Power-to-Gas Storage on Natural Gas Systems
A P2G facility generates SNG utilizing electricity energy, and the obtained SNG can be stored in P2G storage. The storage can be scheduled as either load or supply. To verify the impact of P2G storage on natural gas systems, the following case (Case 3) is conducted:
Case 3: Stochastic scheduling without P2G and storage.
The comparison of scheduling results of natural gas systems for Case 1–3 is listed in Table 2. It shows that the expected production cost in Case 1 will be decreased by almost $1,000, and the expected total supply from gas wells will be decreased 400 kcf if P2G is considered. SNG from P2G decreases the supply from gas wells. Note that the expected costs and supplies in Case 2 and 3 are almost the same because no other gas supply (P2G) is integrated, but more gas load shedding occurs in Case 3. Case 1 features the least gas load shedding, while Case 3 features the most because gas storage can regulate the gas load by charging and supply nodal loads by discharging during the scheduling horizon. SNG from P2G in storage can supply nodal gas loads, replacing gas from gas wells. The results in Table 2 suggest that the integration of P2G can reduce production cost and gas supply of natural gas system, and gas storage can help reduce load shedding, which is more effective with P2G. Natural gas storage can improve security by supplying additional gas reserves to gas-fired power units. It should be noted that the impact of gas storage on gas load shedding and operation cost relates to the location node of the storage, which can be included in the co-planning of an electricity system and natural gas system.
Impact of Ramping Cost Coefficient
In this subsection, we compare the following three cases with various ramping bid factors to illustrate the impact of the ramping cost coefficient on the scheduling of P2G.
Case 4.1: Ramping bid factor is 0;
Case 4.2: Ramping bid factor is 20%;
Case 4.3: Ramping bid factor is 50%;
Hourly power consumption results of P2G in Cases 4.1–4.3 are shown in Figure 6. In Case 4.1, the flexible ramping cost is not considered. Total power consumptions of P2G in Cases 4.1–4.3 are 7,148.11, 7644.69, 8,533.056 MW, respectively. The power consumption of P2G increases as we increase the ramping bid factor, especially in hours 14, 16 and 22 as shown in Figure 6. When the ramping cost coefficient increases, the P2G facility is operated to provide additional flexible ramping capacities and flatten the net load profile to reduce total costs. The results once again demonstrate the benefits of P2G on an electricity system for providing additional flexible ramping capacities and flattening the net load profile.
IEEE 118-Bus System
The IEEE 118-bus system integrated with the 20-node Belgian natural gas system, shown in Figure 7, is applied here to further demonstrate the applicability of the proposed model on large systems. The generator bidding data are similar to those in (Fang et al., 2015). The detailed data of Belgian natural gas system can be found in (Wolf and Smeers, 2000). Wind farms and P2G devices with the same parameters as the PJM 5-Bus system are connected at Buses 8 and 43 and node 16 and three in the natural gas network. Nine generators are assumed to be gas-fired units, and gas loads at nodes 3, 6, 7, 10, 15 and 16 are consumed by power units 7, 13, 16, 23, 42 and 29. Hourly residential gas loads are the 80% of those of the 7-node natural gas system and distributed at load nodes according to the proportion in (Fang et al., 2015). 1,000 initial scenarios are generated, and then reduced to five scenarios.
Table 3 presents the scheduling results for the five uncertain scenarios. The operation cost for the base case of electricity system is $2.036 * 104. The production cost of gas suppliers in Scenario one is the largest. The corrective ramping cost and utilized wind power in S5 are the largest. The reason is that greater corrective ramping capacities are required for greater utilization of wind power. The net load in S4 is the most fluctuant with the largest standard deviation of 998.8 MW, resulting in the largest ramping cost. The results verify the effectiveness of the proposed model on larger systems.
Conclusion
In this paper, a novel stochastic day-ahead scheduling model for IENGS considering ramping costs with P2G and wind power is proposed. The uncertainties of wind power and energy loads are represented in multiple scenarios. Natural gas network constraints are considered to ensure the availability of output of gas-fired units. P2G facility with storage is integrated to reduce wind power curtailment, provide ramping capacities, and reduce supply and load shedding in natural gas systems. The coordinated scheduling model is formulated as a two-stage stochastic programming problem, and the solution method is proposed. Numerical case studies on a modified PJM 5-bus electricity system with a 7-node natural gas system and the IEEE 118-bus system with the 20-node Belgian natural gas system demonstrate the rationality and effectiveness of the proposed model. The key findings of the case studies are summarized as follows.
(1) By utilizing abundant wind power, the integration of P2G can reduce wind power curtailment. The increment on wind power utilization rate can reach 13.8% in the test system.
(2) P2G facility provides additional flexible ramping capabilities, smoothing the net load profile and reducing total ramping costs in electricity system.
(3) The integration of P2G can reduce the total production cost, gas supply and load shedding of a natural gas system. It is more effective to reduce load shedding when gas storage works with P2G coordinately.
(4) A larger ramping cost coefficient would result in increased P2G power consumption.
The impact and benefits of P2G integration with storage relate to its capacity and location in an IENGS. Therefore, future research will focus on studying the siting and sizing of P2G facilities with storage.
Data Availability Statement
The original contributions presented in the study are included in the article/Supplementary Material, further inquiries can be directed to the corresponding author/s.
Author Contributions
XS: data curation, writing and software. CL: supervision, writing - reviewing and editing. RZ: conceptualization and methodology. TJ: software and formal analysis. HC: project administration.
Funding
This paper was supported in part by science and technology project of State Grid Jilin Corporation (SGJL0000DKJS2000287). The authors declare that this study received funding from science and technology project of State Grid Jilin Corporation. The funder had the following involvement with the study data collection.
Conflict of Interest
Author XS was employed by the company State Grid Jilin Electric Power Company Limited, China.The remaining authors declare that the research was conducted in the absence of any commercial or financial relationships that could be construed as a potential conflict of interest.
Glossary
πm/πj nodal pressure at node m/j
ηP2G energy conversion efficiency of P2G
B/Z constant parameter of compressor
ci/cw bidding prices of conventional unit/wind power
Cj,gas bidding prices of gas suppliers
Cmj pipeline constant
crui/crdi bidding prices of up/down ramp
cwc/cP2G wind power curtailment/P2G cost coefficient
Fmj gas flow of pipeline mj
GLm,max maximum gas load of gas fired units
GLm,t,gas gas load of gas-fired units
GSF generation shift factor
HHVgas higher heating values of natural gas
Limitl limit for power flow of line l
Hcom/Pcom energy/electricity consumed by compressor
Pi,t,gas power output of gas-fired unit i
Pimax/Pimin maximum/minimum limit of thermal units
Ps Probability
Rd reserve requirement of electricity system
Sc,max/Sd,max maximum charging/discharging flow
SP2G,t,gas volumetric quantity of SNG
STini/
STmax/STmin maximum/minimum SOC of P2G storage
References
Alabdulwahab, A., Abusorrah, A., Zhang, X. P., and Shahidehpour, M. (2015). Coordination of interdependent natural gas and electricity infrastructures for firming the variability of wind energy in stochastic day-ahead scheduling. IEEE Trans. Sustain. Energy. 6 (2), 606–615. doi:10.1109/tste.2015.2399855
Alkano, D., and Scherpen, J. M. A. (2018). Distributed supply coordination for power-to-gas facilities embedded in energy grids. IEEE Trans. Smart Grid. 9 (2), 1012–1022. doi:10.1109/tsg.2016.2574568
Bai, L., Li, F., Cui, H., Jiang, T., Sun, H., and Zhu, J. (2016). Interval optimization based operating strategy for gas-electricity integrated energy systems considering demand response and wind uncertainty. Appl. Energy. 167, 270–279. doi:10.1016/j.apenergy.2015.10.119
Baldick, R. (2014). Flexibility and availability: can the natural gas supply support these needs?. IEEE Power Energy Mag. 12 (6), 101–104. doi:10.1109/mpe.2014.2340774
Chaudry, M., Jenkins, N., Qadrdan, M., and Wu, J. (2014). Combined gas and electricity network expansion planning. Appl. Energy. 113 (6), 1171–1187. doi:10.1016/j.apenergy.2013.08.071
Clegg, S., and Mancarella, P. (2015). Integrated modeling and assessment of the operational impact of power-to-gas (P2G) on electrical and gas transmission networks. IEEE Trans. Sustain. Energy. 6 (4), 1234–1244. doi:10.1109/tste.2015.2424885
Clegg, S., and Mancarella, P. (2016). Storing renewables in the gas network: modelling of power-to-gas seasonal storage flexibility in low-carbon power systems. IET Gener. Transm. Distrib. 10 (3), 566–575. doi:10.1049/iet-gtd.2015.0439
Fang, X., Hu, Q., Li, F., and Wang, B. (2015). Coupon-based demand response considering wind power uncertainty: a strategic bidding model for load serving entitie. IEEE Trans. Power Syst. 31 (2), 1025–1037. doi:10.1109/tpwrs.2015.2431271
GAMS/SCENRED Documentation (2002). [Online]. Available: http://www.gams.com/docs/document.htm
Grond, L., Schulze, P., and Holstein, J. (2013). Tech. Rep. GL 13.R.23579. Systems analyses power to gas: technology review. Groningen, The Netherlands: DNV KEMA Energy Sustainability.
He, C., Wu, L., Liu, T., and Shahidehpour, M. (2017). Robust co-optimization scheduling of electricity and natural gas systems via ADMM. IEEE Trans. Sustain. Energy. 8 (2), 658–670. doi:10.1109/tste.2016.2615104
Khani, H., and Farag, H. E. Z. (2018). Optimal day-ahead scheduling of power-to-gas energy storage and gas load management in wholesale electricity and gas markets. IEEE Trans. Sustain. Energy. 9 (2), 940–951. doi:10.1109/tste.2017.2767064
Li, T., Eremia, M., and Shahidehpour, M. (2008). Interdependency of natural gas network and power system security. IEEE Trans. Power Syst. 23 (4), 1817–1824. doi:10.1109/tpwrs.2008.2004739
Li, G., Zhang, R., Jiang, T., Chen, H., Bai, L., and Li, X. (2017a). Security-constrained bi-level economic dispatch model for integrated natural gas and electricity systems considering wind power and power-to-gas process. Appl. Energy. 194, 696–704. doi:10.1016/j.apenergy.2016.07.077
Li, G., Zhang, R., Jiang, T., Chen, H., Bai, L., Cui, H., et al. (2017b). Optimal dispatch strategy for integrated energy systems with CCHP and wind power. Appl. Energy. 192, 408–419. doi:10.1016/j.apenergy.2016.08.139
Liu, C., Shahidehpour, M., Fu, Y., and Li, Z. (2009). Security-constrained unit commitment with natural gas transmission constraints. IEEE Trans. Power Syst. 24 (3), 1523–1536. doi:10.1109/tpwrs.2009.2023262
Liu, C., Shahidehpour, M., and Wang, J. (2010). Application of augmented Lagrangian relaxation to coordinated scheduling of interdependent hydrothermal power and natural gas systems. IET Gener. Transm. Distrib. 4 (12), 1314–1325. doi:10.1049/iet-gtd.2010.0151
Liu, F., Bie, Z., and Wang, X. (2019). Day-ahead dispatch of integrated electricity and natural gas system considering reserve scheduling and renewable uncertainties. IEEE Trans. Sustain. Energy. 10 (2), 646–658. doi:10.1109/tste.2018.2843121
Wang, J., Shahidehpour, M., and Li, Z. (2008). Security-constrained unit commitment with volatile wind power generation. IEEE Trans. Power Syst. 23 (3), 1319–1327. doi:10.1109/tpwrs.2008.926719
Wolf, D. D., and Smeers, Y. (2000). The gas transmission problem solved by an extension of the simplex algorithm. Manag. Sci. 46 (11), 1454–1465. doi:10.1287/mnsc.46.11.1454.12087
Wu, H., Shahidehpour, M., Alabdulwahab, A., and Abusorrah, A. (2015). Thermal generation flexibility with ramping costs and hourly demand response in stochastic security-constrained scheduling of variable energy sources. IEEE Trans. Power Syst. 30 (6), 2955–2964. doi:10.1109/tpwrs.2014.2369473
Yang, J., Zhang, N., Cheng, Y., Kang, C., and Xia, Q. (2019). Modeling the operation mechanism of combined P2G and gas-fired plant with CO2 recycling. IEEE Trans. Smart Grid. 10 (1), 1111–1121. doi:10.1109/tsg.2018.2849619
Zhang, X., Shahidehpour, M., Alabdulwahab, A. S., and Abusorrah, A. (2015). Security-constrained co-optimization planning of electricity and natural gas transportation infrastructures. IEEE Trans. Power Syst. 30 (6), 2984–2993. doi:10.1109/tpwrs.2014.2369486
Zhang, X., Che, L., Shahidehpour, M., Alabdulwahab, A., and Abusorrah, A. (2016). Electricity-natural gas operation planning with hourly demand response for deployment of flexible ramp. IEEE Trans. Sustain. Energy. 7 (3), 996–1004. doi:10.1109/tste.2015.2511140
Keywords: power-to-gas, ramping cost, wind power accommodation, stochastic programming, natural gas system
Citation: Song X, Lin C, Zhang R, Jiang T and Chen H (2020) Two-Stage Stochastic Scheduling of Integrated Electricity and Natural Gas Systems Considering Ramping Costs With Power-to-Gas Storage and Wind Power. Front. Energy Res. 8:596774. doi: 10.3389/fenrg.2020.596774
Received: 20 August 2020; Accepted: 02 October 2020;
Published: 05 November 2020.
Edited by:
Peng Li, Tianjin University, ChinaCopyright © 2020 Song, Lin, Zhang, Jiang and Chen. This is an open-access article distributed under the terms of the Creative Commons Attribution License (CC BY). The use, distribution or reproduction in other forums is permitted, provided the original author(s) and the copyright owner(s) are credited and that the original publication in this journal is cited, in accordance with accepted academic practice. No use, distribution or reproduction is permitted which does not comply with these terms.
*Correspondence: Tao Jiang, dGppYW5nQG5lZXB1LmVkdS5jbg==