- 1High-Performance Architecture Laboratory (HAL), Department of Architecture, Tarbiat Modares University, Tehran, Iran
- 2CyPhyLab, Bio-Inspired Architecture and Sensoric, Institute of Architecture, Technische Universität Berlin, Berlin, Germany
Domestic greywater produced via household chores has a major contribution to environmental pollution and is also the best-untapped energy source. Since the past decade, in the different fields, enormous efforts have been made to reach the bio-energy from bio-waste. These efforts consist of a wide range of thermochemical, bio-chemical, and microbial fuel cells; etc. however, all these efforts are in their infancy. They have high cost and are efficient on a large-scale; furthermore, these efforts used a non-intelligent process that has led to lack of development in this type of review. This ongoing research presents the smart design process that leads to hybrid energy via the intersection of computation, wastewater, and microorganisms and aims to introduce the novel bio-computational machine based on particle Physics. Therefore, we will investigate the mixture of the microbial fuel cells, specifically “Spirulina” micro-algae, and household chores greywater. In the computational framework, we propose high-precise fluid simulation with the advantage of particle position and dynamic physics for the conversion of the mixture of “Spirulina medium,” and household chore greywater makes energy control possible through flow, and management of the type of solution mix, by transferring data from the modules to the digital environment. Finally, these datasets simulate behavior based on the physical properties of each particle. The procedure works in a parametric computer-aided design (CAD) environment and through Grasshopper within Rhinoceros Software by syncing bio-computational machine to the digital environment. The solenoid valve and fluid flow controller applied between the nutritious tank and energy conversion tank measure nutritional consumption and sends it to the digital environment numerically with an Arduino-Uno board kit. These batch numerical signals are stored in our local database then are combined with the particle physics engine and simulate the material behavior; also, they provide the ability to control our bio-computational machine digitally.
Introduction
Buildings are among the significant wastewater producers, whose production volume is increasing due to population growth, improved living conditions, economic and technological development (Asano et al., 2007). domestic wastewater, produced by buildings, which consists of blackwater (from toilets) and greywater (from kitchens and bathing) is a critical source of toxic chemical compounds, gases, and biological contaminations (Strauss et al., 2003; Adamus-Białek et al., 2015). Which transfer and collect them on a large scale may have a direct impact on the surrounding environment. After being transferred to municipal wastewater treatment plants, this type of wastewater can be reused through large-scale processes, high cost, and equipment complexity (Kalogo and Monteith, 2012). “In the last decade, enormous efforts were done to reuse wastewater, each of which benefited from technological developments such as: microfiltration, ultra-filtration, and reverse osmosis membranes; the use of ozone coupled with biological filtration, low, medium, and high energy UV disinfection; high energy UV advanced oxidation, these treatment processes can now be used to remove acute toxicity (e.g., microorganisms) and chronic toxicity (e.g., chemical constituents; Angelakis et al., 2018). Most of these efforts are aimed to refine and reuse wastewater to support various applications water resources” (Sato et al., 2013). Lack of attention to the potential of other phases of wastewater, inability to have computational control of processes and the complexity of large-scale equipment have led to wastewater's use as an untapped energy resource available at the initial stages (Lahnsteiner et al., 2013). Domestic greywater produced via residential buildings at the architectural scale has great potential for reuse as an energy resource before entering the municipal sewage system due to its high-volume production and unique contents (Bond and Lovley, 2003; Johnson and Wen, 2009; Doulaye et al., 2010; Harun et al., 2010; Ozkan et al., 2012; Kara and Georgoulias, 2013; Kesaano and Sims, 2013; AQUASTAT, 2014; Global Water Intelligence, 2014; Samsonoff et al., 2014) (see Figure 1).
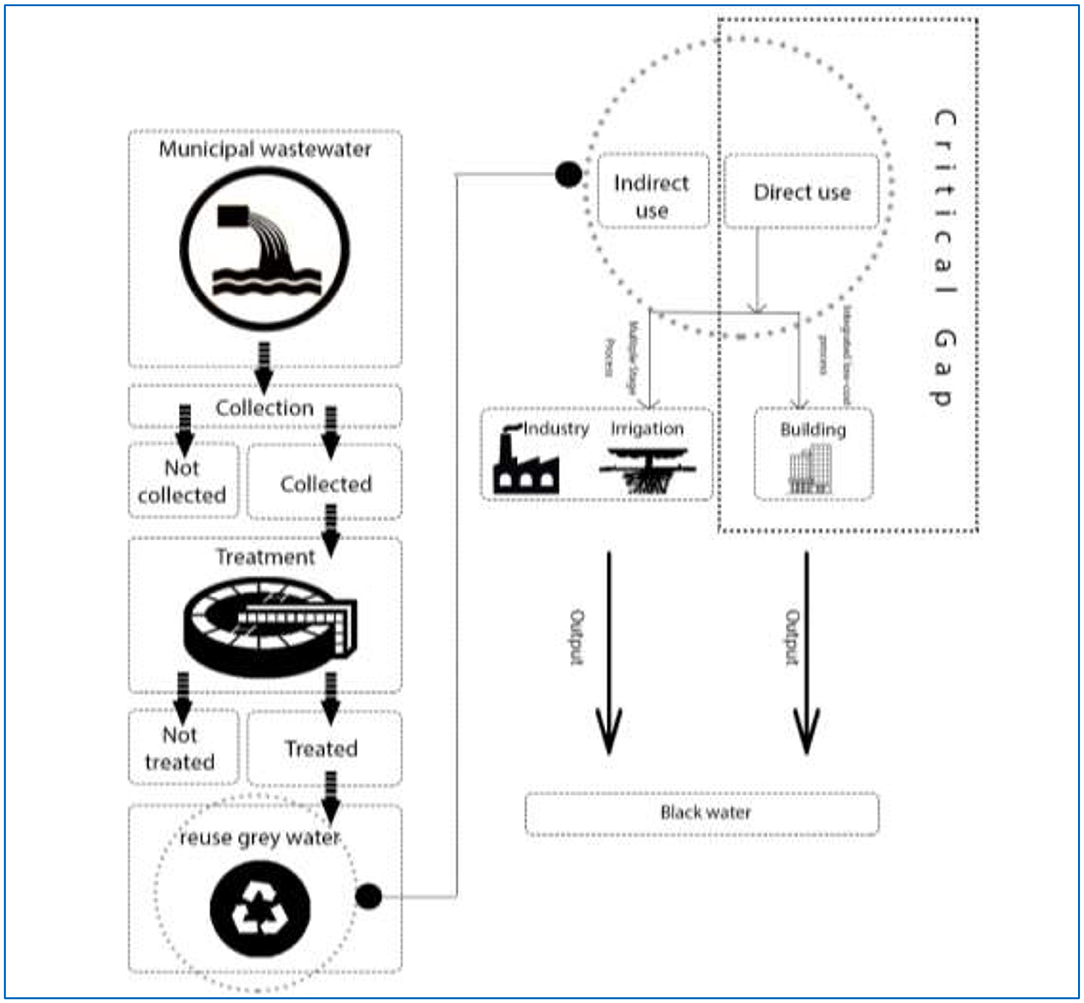
Figure 1. The process of municipal wastewater production to reuse [adapted from AQUASTAT (2014)].
Microorganisms, as an agent, perform an essential role in wastewater treatment, the use of microalgae and their combination with wastewater to separate toxins has been investigated in several types of research. “These types of microorganisms are a diverse group of photosynthetic Microorganisms, which can produce several economically valuable bio-products, including proteins, omega-3 fatty acids, and powerful antioxidants, as well as feedstocks for biofuels” (Ozkan and Berberoglu, 2013; AQUASTAT, 2014; Adamus-Białek et al., 2015). Micro-algae biofilm cultivation has been gaining attention as an alternative to the suspended growth of micro-algae for metabolite production and wastewater treatment for many years. large biomass concentrations can be obtained with minimal energy and water consumption by using these systems (Chang, 2004; Chisti, 2007; Boelee et al., 2012; Bruno et al., 2012; Ozkan and Berberoglu, 2013; De Wilde, 2014; Drechsel et al., 2015; Duro Royo, 2015). “Attached algae cultivation can be applied in photosynthetic microbial fuel cell systems (MFCs) for electricity production. In these systems, the photosynthetic microorganisms are cultivated as biofilms over the anode, electrons generated from water splitting by the photosynthetic organisms are harvested at the electrode, creating an electrical current” (Ozkan and Berberoglu, 2013; Global Water Intelligence, 2014; Gajda et al., 2015). As microalgae carry electrons, they can be deionized through electrodes, and with the advantage of this process, reach bio-energy can be obtained from these microorganisms. Due to this ability and detoxification by this type of microorganisms, they can be used as a multifunctional microorganism to purify and extract energy from wastewater in a controlled electrochemical environment. The deionized microalgae's electrons by electrodes and the residential buildings' greywater contents provide a direct energy extraction substratum from combining these solutions (De Wilde, 2014; Gajda et al., 2015). On the other hand, the physical environment's connection to the digital environment can fill the significant gap existing between predictable output energy and desired energy by controlling the effective parameters and controlling the mixture between microalgae medium and residential building's greywater in the structure of bio-computational machine (Heidari and Mahdavinejad, 2017; Heidari et al., 2018). The transfer of required information from the physical environment to the controlled electrochemical environment provides an opportunity to adapt the tools of both domains, ultimately leading to customized control at various scales and affecting multiple manufacturing industry applications.
This study aims to produce a controllable biocomputational machine as a novel method for producing functions of microorganisms in various applications. This research investigates the residential buildings' greywater (handwashing greywater and Kitchen activity greywater) with Spirulina microalgae medium used as the bio-computational machine ingredients, as an example, and the output hybrid energy as its function. Since the behavior of this machine's components is based on the constituent particles, particle physics has been used to implement the project goal. The use of particle physics enables us to control each particle's behavior in a digital environment based on the spatial points. Particle control will lead to fluid control and ultimately control the bio-computational machine itself and the desired output function. The behavior of materials simulated by high-precision engines in the CAD environment through Grasshopper within Rhinoceros software can be changed and controlled through various modules used in different parts of the bio-computational machine. Modules control the output function or hybrid output energy by changing the amount of material mixing and controlling their injection timing. Two solenoid valves regulate fluid injection timing and a double relay, then the fluid flow module controls the fluid flow rate, and the solution level control module controls the fluid level via theoretical framework. The design of intelligent structures in the present era focuses on using the potential of materials to increase the performance of these structures and eliminate the gap between design and manufacturing (Fleischmann et al., 2011; Schleicher et al., 2015). This method usually starts with experimentation materials in a physical environment. It proceeds with simulation and modeling of behaviors in a parametric environment. In the next step, the behavior of materials can reach the designer's goal by regulating the parameters affecting these behaviors (Schleicher et al., 2015). “Despite all these efforts, fully integrated, material driven digital models are yet to be implemented in off-the-shelf software to enable design processes that are fully informed by material behavior” (Tamke et al., 2014; Schleicher et al., 2015). Success and development in reviewed methods occur when the structure's components are not homogeneous, and the diversity of information in the processes is entirely interdisciplinary.
This strategy increases the power of control over processes to predict and test multi-objective goals for intelligent structures (Kara and Georgoulias, 2013). The materials and details in these structures act as agents, embedding the data where needed and moving it through various environments of different materials to capture physical Environment data. The designer also receives this data through written algorithms and modules installed in the real environment. This method is very efficient by translating the desired physical environment's data through materials and modules and finally shifting it to the digital environment to adapt and respond to contemporary problems and transform the complex design process into simple processes. The development of intelligent systems and machines to achieve the designer's desired goals confronts a crisis of information management affecting system's mechanism. The increase of parameters affecting the design, manufacturing, and internal mechanism of systems has made it difficult to control them simultaneously in the physical environment. It has created many limitations in the installation process to achieve the desired goal of the designer. These machine's information modeling creates multi-functional capabilities and shows digital flexibility on a real scale with minimal cost and power. In hybrid systems, due to the diversity of components, whether they are alive or not, it is impossible to achieve the desired output without computing materials and modeling machine information. The computer-aided design and manufacturing process helps to integrate intelligent systems' design and installation and customize the target process in the machine mechanism by designer-written algorithms. In typical examples in various industries, this is achieved by exporting the virtual design into a specific machine file format (Chang, 2004; Sass and Oxman, 2006; Sheil, 2013). With the development of computational design in the second half of the twentieth century, early ideas of computation in material evolved into generative design approaches in architecture and other fields. In architecture, material computation is a type of generative computational method in which functional complex development drives by algorithms and adapts them to a real environment (Hensel et al., 2004; Steadman, 2008). However, during the last decade or so, as computational simulation methods advanced, there has been a turn toward integrating material properties and behavior. As a result, invention techniques and material systems develop to integrate sustainable, responsive, renewable biological materials into Hybrid architectural components (Menges, 2012; Oxman et al., 2015).
Position-Based Dynamics and Particle Physics
“A particle-based method in general refers to the class of mesh-free methods that employ a set of finite number of discrete particles to represent the state of a flow system and to record the evolution of the system (i.e., their positions and velocities)” (Liu and Liu, 2003; Ye et al., 2016). In this process, the behavior of fluids is predicted based on the action of particles that makes them up so that each particle runs based on the properties defined for them (see Figure 2). These properties include particle index in a group of particle type, three-dimensional spatial position, velocity, mass, type of fluid, and the activities relative to other particles. In fluids composed of two different materials, grouping is required; it means that two groups of particles with different properties are fused (Van Zon and ten Wolde, 2005; Yu et al., 2012). When two materials are mixed, another critical factor is formed in particle physics: how particles collide and how they behavior after the collision. The simulation of such behavior is based on the dynamics of position in three-dimensional coordinates. Exploring the recent state in this field of science presents an optimal view of the previous approaches and context. Past approaches were performed to simulate particle dynamics based on internal and external forces. Internal forces include elasticity, and external forces include gravitational forces (Müller et al., 2007; Tsai, 2017). This type of approach is based on Newton's second law and the control of forces based on time steps. “Then a numerical integration technique like explicit or implicit Euler integrator is further applied to estimate the new velocity, and then position at the end of a time step. The former is conditionally stable and accurate if a small-time step is adopted. In contrast, the latter is unconditionally stable even though a large time step is adopted, but inaccurate. In order to achieve a trade-off between them, position-based method simply adopts a fast semi-integrator, simplistic Euler, to evaluate external force like gravity. Furthermore, instead of gathering internal forces to compute acceleration and velocity, the vertex position is directly manipulated and controlled to solve internal constraints, like stretching, bending, or volume constraint, and collision constraints such as mutual-collisions between objects or self-collisions of an object” (Müller et al., 2007; Tsai, 2017). The simulation of these particles with two different engines is done by dynamic and static data mining. In the dynamic mode, the dataset is transmitted simultaneously, and the system makes decisions based on the instant behavior of the material. In the static mode, the data is transmitted at specific periods and is checked by a high-precision engine. High-precision physics engines are often contrasted with real-time simulation, and in some particular examples, the real-time technique is used to increase the simulations' accuracy. Contrary to public belief, real-time physics engines despite simulation materials and optimal capacity for effective interaction between digital and physical environment have little accuracy and is in fact the appearance of particles' behavior based on shape changes. In this project, we used high-precise simulation with the advantage of position-based dynamics to increase the details and accuracy of simulated data in the digital environment. And we simulated the real-time signals based on the high-precise engine. Finally, depending on the simulated behaviors through high-precision simulation engines, we controlled the modules.
Methodology
Materials
The biocomputational machine proposed in this study generally consists of three tanks, two primary tanks and one final tank to combine materials (hybrid tank); two primary tanks consist of two separate materials: residential buildings' greywater (including handwashing greywater, kitchen activity greywater) and Spirulina microalgae medium. The second material is investigated as an agent material to reuse the first one, deionized through electrodes simultaneously for energy extraction. To perform desired domestic greywater potential from residential buildings and investigate the different output energy related to material contents, two types of greywater were combined with microalgae culture medium (see Table 3). The first was greywater produced in the kitchen activity (washing the dishes) (see Table 1) and the next type was greywater produced by handwashing) with a special hand wash liquid (see Table 2) in the residential buildings, each of which had different nutrients. each material was injected into a primary tank and the input tanks were set for the hybrid tank. The ingredients of the primary tanks were not directly related to the main sources of materials, and in this study, these tanks were manually filled once to the extent of their volume capacity. In the hybrid tanks, the materials mentioned in the primary tanks above were mixed in two different types: type A. Combination of residential building's greywater nutrients type A- kitchen activity (washing the dishes) with Spirulina microalgae medium culture nutrients and type B. Residential building's greywater nutrients type B- handwashing) with a special hand wash liquid (with Spirulina microalgae medium culture nutrient. how to combine them or how to time the opening of the solenoid valves in each of the primary tanks is written through our defined controlled electrochemical environment in CAD with the advantage of python “PyFirmata” library. The solenoid valves and primary tanks are connected to the hybrid tank through two plastic pipes, and a 1/2 conversion has been used to connect the solenoid valves to these pipes (see Figure 3). The microorganism was grown in the initial culture medium tank, to the maximum liquid density, and was injected to the hybrid tank by solenoid valve timer within plastic pipes. This process was repeated for each test on different types of greywater in the initial tanks. Simultaneous with controlling the injection of algae-saturated culture medium through electrical valves, each type of greywater was injected into the hybrid tank with a separate electrical valve by different timings. When these two materials were combined, the greywater acidity enhanced the deionization of the solution in the hybrid tank. It regenerated the final solution into a powerful electrolyte.
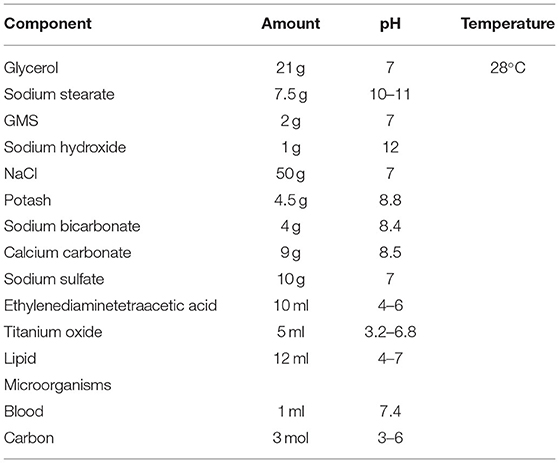
Table 2. Residential building's gray water nutrients type B-handwashing (with a special handwash liquid).
Computational
Writing to Environment
The computational part of this research in CAD environment consists of two parts: A. Writing to environment and B. Reading from environment, in the first part, the machine control computation and timing of module activation are performed by connecting the digital environment to the physical environment. The components of a biocomputational machine, including materials and modules, make up the physical environment. In this study, electronic modules receive pulses from a script written in Grasshopper in combination with the Python component by using the “PyFirmata” library via the Arduino-Uno Kit. Various electronic modules and the Arduino Uno kit are used to control the effective parameters in the bio-computational machine. These modules include the solenoid valve, fluid flow module, and solution surface level module installed on the Arduino kit through the breadboard. The solenoid valve input voltage is 12 V, and the Arduino kit cannot accept such voltages, so a double relay is used to convert the voltage of the solenoid valves to connect to the Arduino. By connecting this tool kit to the digital environment, we can write the desired commands through them in the bio-computational machine and control how to combine different fluids with different injection timing through the digital environment. Plastic pipes are used to connect modules to the hybrid tank with a diameter of 1 inch. Because the module outlet diameter is not the same as the plastic pipes' diameter, to connect the modules to these pipes, we used a 1/2 steel converter. At the connection points of these pipes to the hybrid tank, the same converter with plastic material was used in reverse type. All tanks (two primary tanks and hybrid tanks) are made of glass, and because the primary tanks are filled manually, the inlet valves are mechanical. An algorithm is used to provide the CAD environment's controlling process through Grasshopper within Rhinoceros software. The pulses are read from the solution surface level module (located in the hybrid tank) and are synced with the commands written by the injection modules (in the primary tanks' path to the hybrid tank) intelligently. To measure the output function of this bio-computational machine or hybrid energy, aluminum and copper electrodes with a multimeter connected to them have been used. A change in the inputs causes a variance in the output energy, and by connecting its numerical value to the digital environment, these values are placed next to other controlling parameters of the bio-computational machine. How to combine the materials in the primary tanks is done through a timing algorithm connected to a set of algorithms (written to solenoid valves) then the combined values of each primary tank are estimated according to the timing of solenoid valves and pipe terminal flow; in the digital environment, they can be calculated simultaneously. In this study, in addition to simulating the behavior of materials within a biocomputational machine, through four ultrasonic modules, the initial growth data required to simulate the behavior of materials based on particle physics are given to the digital environment. Ultrasonic sensors in the upper part of the medium, with a 15-degree operating radius, scanned the environment in real-time. Each sensor had a power frequency of 40 kHz and a precision of 2 mm, which covered 1/4 range of the cultured surface. Each sensor was triggered 20 times per second. Ultrasonic sensors were designed only to detect the first echo. Thus, other echoes might be affected when the sensor was triggered again. Therefore, we designed an algorithm that reflected the receiver system. It was able to achieve 5 more pulses in each trigger. As a result, every 5 ms we got the numerical value of 5 echoes in the form of an array. We divided the level of the receiving waveforms into four parts. Each segment received its own unique five-point signal. These batch numerical signals are called by Gh-python remote in the Rhinoceros environment. We used the “pyserial” library to read port serials (Figure 4 represents the ultrasonic sensors scanning zones).
Reading From Environment
To simulate the behavior of materials in the digital environment, we used a high-precise simulation engine based on the particle Physics and position-based dynamics of the materials in different tanks. Given that the particles of the material in each of the tanks were on a micro-scale, and because real-time analysis engines had errors at high data transfer speeds, unlike the writing section, the reading section was combined with electronic modules (ultrasonic) with high-precise fluid simulation based on the particle physics to transfer data from the bio-computational machine to the digital environment. To simulate the position-based dynamics of the particles, we used the “Flexhopper” plug-in through Grasshopper. This engine is a high-precision simulator based on material's particle-physics that simulates the behavior of different groups of materials in defined environments based on the properties of each particle. The properties of each particle included particle index in the grouping of materials, velocity, three-dimensional spatial position, mass, type of activity, particle group, collision mode, and optimal particle radius. The engine simulated the behavior of existing materials and fluid flows within the bio-computational machine with precision beyond real-time mode and was synchronized with the control section. It means that by burning the injection command to the solenoid valves in the monitoring environment, this transfer also occurred with the same time frames and the amount of solution, based on particle physics. In general, in this research, the computational method is a combination of real-time simulation and high-precise simulation, in which the second part helped to detail and increase the accuracy in the first part. In the digital environment, the actual dimensions and proportions of the bio-computational machine were considered, and the staging of the material behavior was quite similar to the platform in the real environment.
Data Analysis
Simulation
Before starting the main simulation, we had a pre-process in the primary tanks. In the tank, which was composed of spirulina microalgae cultured medium, this microorganism's ideal growth conditions were considered. Furthermore, the main simulation started after reaching 100% density of microalgae in the cultured medium after 12 days. During this period, the cultured medium was exposed to light for 12 h daily with an intensity of 2,000 lux and 12 h in a dark environment. In general, the process of analysis and simulation of this tank were done in two separate cases with the other primary tank's two components (see Tables 1–3). Several modules have been used in the tank's path and inside the hybrid tank to connect the controlled electrochemical environment to the physical environment. These equipment types included four Ultra sonic modules, two solenoid valves, two flow modules, a solution level module, and a double relay to control the injection timing of the primary material tanks and voltage conversion through the solenoid valve. Each of the types mentioned above equipment was efficient for transferring data in a specific part to make controllable changes in other parts, and finally changed the output energy. The movement and dispersion of microalgae were simulated by high-precise “Flexhopper” engine based on the position-based dynamics of particles over 12 days. This data was simulated from the hybrid tank every four days at 8 am, which reached its maximum density over 12 days (in the hybrid tank). The solenoid valves were timed by a double relay switch that transmits the materials from the first two tanks in a controlled manner to the hybrid tank, each of which was a cube 20 cm long and 30 cm wide, and 30 cm high. To combine 50% of the wastewater and the algae cultured medium, the injection time interval from the valves was 60 s, during which an average of 250 ml of each tank was transferred to the energy conversion tank. Every 8 min, an equal amount of mixture was transferred from both Tanks, and within 64 min, the solution volume reached the water level sensor in the energy conversion tank, which was 20 cm high from the tank, and the terminals would be closed computationally. The fluid flow controller between the initial tanks and the hybrid tank measured the nutritious consumption in real-time (in defined period of time) and sent it to the defined virtual environment numerically with an Arduino-Uno board kit that was connected with. Then high-precise engine simulated fluid flow behavior through the path. After intelligent shut-off of the inputs, 4 l from each tank was transferred to the energy conversion tank, and the microalgae growth medium provided in this environment. To test this machine, we simulated two 12-day periods. The first was from November 20, 2020, to December 2, 2020, for residential building's greywater nutrients type A- kitchen activity (washing the dishes, the second was from December 4, 2020, to December 16, 2020, for residential building's greywater nutrients type B- handwashing with a special hand wash liquid). These periods were selected to achieve the maximum algae growth in the hybrid tank.
Output
As mentioned, fundamental purpose of this study is to create a controllable bio-computational machine based on particle physics. This process leads to reusing residential buildings' greywater in a novel computational framework (see Figure 5). Hybrid energy extraction from this process was done directly to reduce costs and the need for complex equipment through two electrodes, including aluminum and cooper electrodes as cathode and anode. Output hybrid energy was tested with two types of hybrid cultured medium (type A/B) at four dates while the microorganisms were alive and in perfect growth condition. The output electrical energy in all samples varied in the range between ½ to 6 V depending on the type of ingredients and the density of microorganisms in the hybrid tank. Tested samples of kitchen activity greywater generated more electrical energy than handwashing samples in the toilet due to interferer parameters, including the type of diet and the use of dishwashing liquid, which itself can deionize the solution. The maximum electrical energy output of the similar composition of these materials with microalgae was 6 V, while is another simulated model was 3.5 V (toilet handwashing greywater). In this research, we use the gravitational force and heightened the tanks to shift the force of solutions from primary tanks to the energy conversion tank to reduce costs and dependence on input energy. For this purpose, instead of pumps, solenoid valves were used to control the flow at intervals with the Double Switch relay. This intelligent machine consisted of three tanks with 12 l capacity, including two primary tanks and an energy conversion tank and solution transfer paths. The final hybrid solution in the energy conversion tank was limited to a maximum of 8 l or 2/3 of the tank's total capacity by the solution-level control sensor. The extraction of direct electrical energy by using the potential of microorganisms and materials combined in solutions eliminated the various processes for multi-stage energy conversion with complex equipment and high costs. With the help of instant outputs of the built-in device, we could store the parameters that influenced the growth rate and its orientation. We stored this received data in datasets due to vast correlated parameters of nutrient and medium environmental conditions that affected the growth rate (see Figure 6). We stored each experiment in our dataset. The visualized data was received statistically and at specified periods and was stored in the predicted virtual environment in the computational framework (see Figures 7–9). Due to the need for time to complete microorganism's growth in the energy conversion tank's mixed culture medium, the data were received simultaneously only at the periods defined in the simulation part and were stored statistically in the final set. Because of the ability to control the modules in this machine, by changing the timing of the release of solutions from primary tanks to energy conversion tank and transfer of cultured medium and the increase the density of microorganisms, the period to reach maximum density in microalgae was limited and the maximum expected output energy was obtained in a shorter period. Six volts of electrical energy output from 8 l of the mixed solution in 12 days with static computations paves the way for future development to control more parameters computationally and reduce time to achieve optimal hybrid energy through the reuse of greywater.
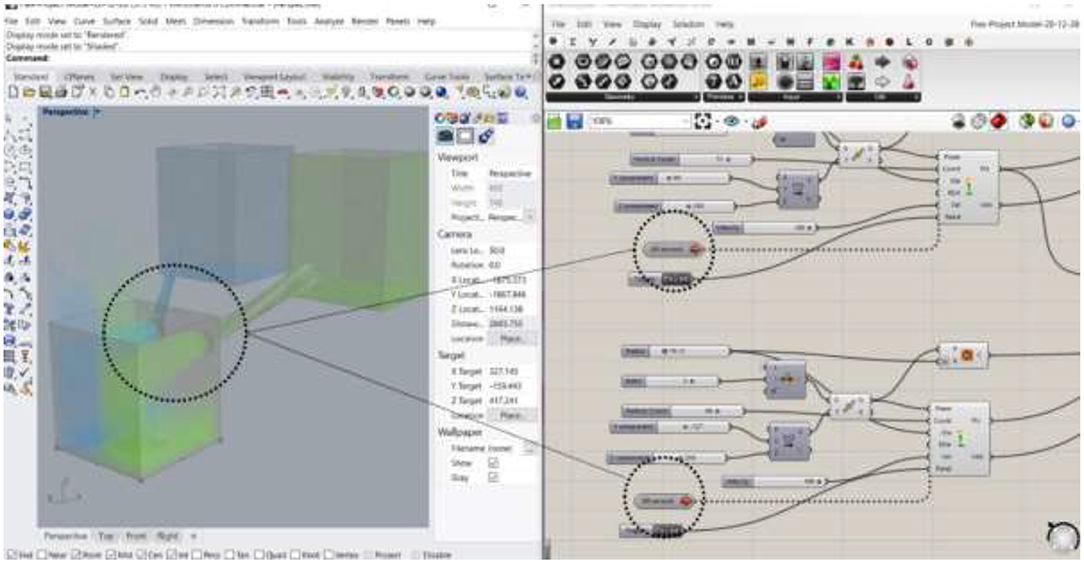
Figure 6. Solenoid valves injection timing control in the virtual environment (writing commands to the bio-computational machine).
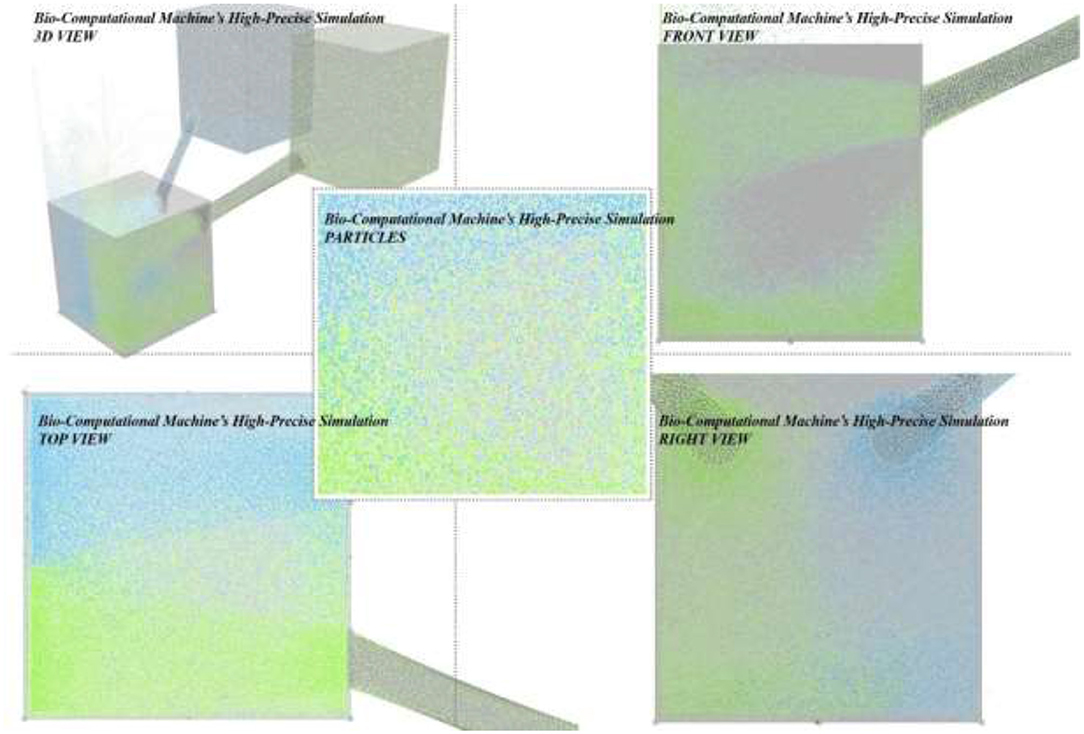
Figure 7. Bio-computational machine's high-precise simulation by position-based dynamics engine and particle physics.
Conclusions
Domestic greywater produced in the buildings proceeds up a significant part of the total greywater returned. To the take advantage of domestic greywater potential in buildings, we need a small, low-cost intelligent process at the building and architectural scale to reuse it before its turning to the municipal sewage system. The use of multi-functional microorganisms in intelligent systems reduces the extra steps in reusing wastewater and saves time and costs. For this purpose, this research has directly produced electrical energy by combining microalgae cultured medium and domestic greywater, including toilet handwashing greywater and kitchen with an intelligent machine. It combines two fields of waste and biological materials in a controlled computational framework (see Figure 10). Although this achievement has been done on a limited scale, it provides a platform for research and optimization of these machines at the architectural scale, expanding in volume, scale, number of them, and adding other computational parameters (see Figures 11, 12). These parameters directly affect the amount of output energy and its scalable scale and can be used as a part of the Building Information Management (BIM) system to control the waste generation, by reusing and converting it into energy. In addition to its partial purification, the intelligent machine's output can be used for other building functions, including the toilet and bathroom, and can be connected to other devices in an integrated network. Between the reasons, the lack of development of intelligent and low-cost machines on an architectural scale to reuse wastewater is the lack of computational control in different parts of these machines and lack of control over the outputs and its type. In the computational process defined in this project, by connecting the physical environment to the digital environment through modules, the reuse of wastewater is controlled in all conversion stages. This section can be connected to an intelligent computational engine for inhuman decisions regarding the significant parameters around and inside the hybrid environment of microorganisms and wastewater. Furthermore, new processes and functions are defined for the waste produced inside the building and they provide multi-functional components from the buildings' waste. This project is a part of ongoing research that, with a different aesthetic approach, sees waste as an architectural component and wants to use them as the main components by regulating and controlling them toward creating various functions in buildings that can be used as a component in various parts of it, including the envelope. These systems in high numbers, and after the optimization of their internal processes can provide lighting and various electrical equipment energies in different building parts. The ability to control brightness and final voltage according to output energy control can be predicted in its computational framework. In the next versions of this research, by changing microorganism's genetics, the ionization rate of solutions with a small amount of microorganism bank can be increased. Because of this, we can combine a large volume of wastewater, including purifying it and getting more electrical output energy.
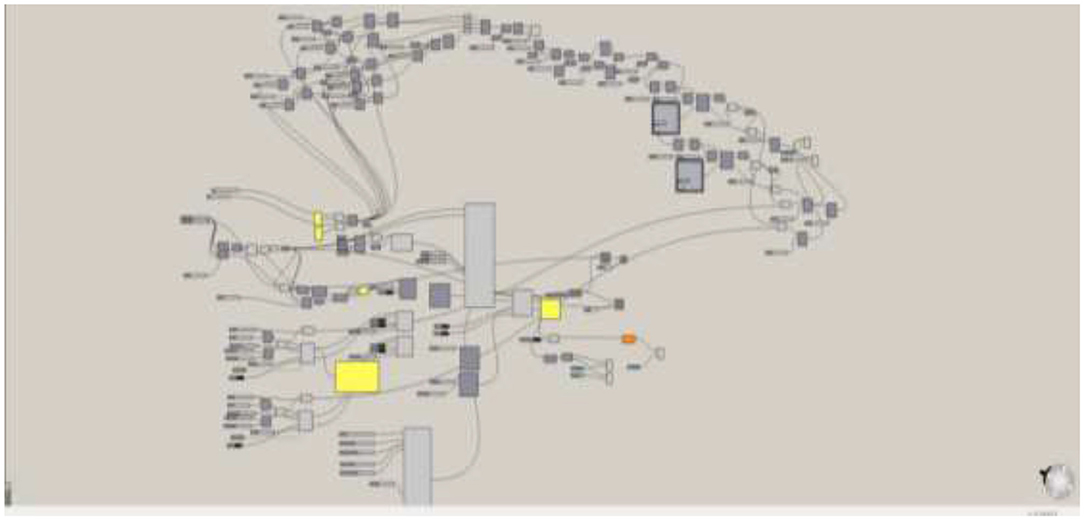
Figure 12. Written scripts through grasshopper for writing to environment and reading from environment.
Data Availability Statement
The original contributions presented in the study are included in the article/supplementary material, further inquiries can be directed to the corresponding author/s.
Ethics Statement
Ethical review and approval was not required for the study on human participants in accordance with the local legislation and institutional requirements. Written informed consent for participation was not required for this study in accordance with the national legislation and the institutional requirements.
Author Contributions
FH contributed to all aspects of this work and conducted data analysis. MR and HS wrote the main manuscript text. LW and MM gave useful comments, suggestions to this work, and affect the process of the research. All authors reviewed the manuscript.
Conflict of Interest
The authors declare that the research was conducted in the absence of any commercial or financial relationships that could be construed as a potential conflict of interest.
References
Adamus-Białek, W., and Wawszczak, M., Swiercz, A. (2015). Impact of sewage treatment plant on local environment. Proc. ECOpole 9, 397–404. doi: 10.2429/proc.2015.9(2)047
Angelakis, A. N., Asano, T., Bahri, A., Jimenez, B. E., and Tchobanoglous, G. (2018). Water reuse: from ancient to modern times and the future. Front. Environ. Sci. 6:26. doi: 10.3389/fenvs.2018.00026
AQUASTAT (2014). FAO Global Information System on Water and Agriculture. Wastewater Section. Available online at: http://www.fao.org/nr/water/aquastat/wastewater/index.stm (accessed October 1, 2020).
Asano, T., Burton, F. L., Leverenz, H. L., Tsuchihashi, R., and Tchobanoglous, G. (2007). Water Reuse: Issues, Technologies, and Applications, Eddy, Inc. 13.
Boelee, N., Temmink, H., Janssen, M., Buisman, C., and Wijffels, R. (2012). Scenario analysis of nutrient removal from municipal wastewater by microalgal biofilms. Water 4, 460–473. doi: 10.3390/w4020460
Bond, R. D., and Lovley, R. D. (2003). Electricity production by geobacter sulfurreducens attached to electrodes. Am. Soc. Microbiol. J. 69, 1548–1555. doi: 10.1128/AEM.69.3.1548-1555.2003
Bruno, L., Di Pippo, F., Antonaroli, S., Gismondi, A., Valentini, C., and Albertano, P. (2012). Characterization of biofilm-forming cyanobacteria for biomass and lipid production. J. Appl. Microbiol. 113, 1052–1064. doi: 10.1111/j.1365-2672.2012.05416.x
Chang, C. C. (2004). Direct slicing and G-code contour for rapid prototyping machine of UV resin spray using PowerSOLUTION macro commands. Int. J. Adv. Manufact. Technol. 10, 136–145. doi: 10.1108/13552540410527006
Chisti, Y. (2007). Biodiesel from microalgae. Biotechnol. Adv. 25, 294–306. doi: 10.1016/j.biotechadv.2007.02.001
De Wilde, P. (2014). The gap between predicted and measured energy performance of buildings: a framework for investigation. Automat. Constr. 41, 40–49. doi: 10.1016/j.autcon.2014.02.009
Doulaye, K., Cofie, O. O., and Kara, N. (2010). Low-Cost Options for Pathogen Reduction and Nutrient Recovery From Fecal Sludge. CGIAR. International Water Management Institute. Book Chapters, 171–188.
Drechsel, P., Qadir, M., and Wichelns, D. (2015). Global wastewater and sludge production, treatment and use. Waste Water. 6, 15–18. doi: 10.1007/978-94-017-9545-6
Duro Royo, J. (2015). Towards Fabrication Information Modeling (FIM): Workflow and Methods for Multi-Scale Trans-Disciplinary Informed Design. (Master of Science in Media Arts and Sciences Thesis). Massachusetts Institute of Technology, Cambridge, MA, United States. doi: 10.1557/opl.2015.647
Fleischmann, M., Lienhard, J., and Menges, A. (2011). “Computational design synthesis: embedding material behaviour in generative computational processes. Respecting fragile places,” in 29th eCAADe Conference Proceedings (Ljubljana), 759–767.
Gajda, I., Greenman, J., Melhuish, C., and Ieropoulos, I. (2015). Self-sustainable electricity production from algae grown in a microbial fuel cell system. Bio-mass Bio-Energy 82, 87–93. doi: 10.1016/j.biombioe.2015.05.017
Global Water Intelligence (2014). Global water market 2014. Volume 12. Oxford: Introduction and the Americas; Media Analytics Ltd.
Harun, R., Singh, M., Forde, G., and Danquah, M. (2010). Bioprocess engineering of microalgae to produce a variety of consumer products. Renew. Sustain. Energy Rev. 14, 1037–1047. doi: 10.1016/j.rser.2009.11.004
Heidari, F., and Mahdavinejad, M. (2017). “High-performance Sonitopia (Sonic Utopia): hyper intelligent material-based architectural systems for acoustic energy harvesting,” in Proceedings of the 2nd International Conference on Green Energy Technology (Rome: SAPIENZA University of Rome), 83:011001. doi: 10.1088/1755-1315/83/1/012021
Heidari, F., Mahdavinejad, M., and Sotodeh, S. H. (2018). Renewable energy and smart hybrid strategies for high-performance architecture and planning in case of Tehran Iran. Proceedings of the 4th International Conference on Environment and Renewable Energy. 159, 012030. doi: 10.1088/1755-1315/159/1/012030
Hensel, M., Menges, A., and Weinstock, M. (2004). Emergence: Morphogenetic Design Strategies. London: Academy Press.
Johnson, B. B., and Wen, Z. (2009). Development of an attached microalgal growth system for biofuel production. Appl. Microbiol. Biotechnol. 85, 525–534. doi: 10.1007/s00253-009-2133-2
Kalogo, Y., and Monteith, H. (2012). Energy and Resource Recovery From Sludge. doi: 10.2166/9781780404653
Kara, K., and Georgoulias, A. (2013). Interdisciplinary Design: New Lessons from Architecture and Engineering. ACTAR Publishers.
Kesaano, M. C., and Sims, R. (2013). Algal biofilm-based technology for wastewater treatment. Algal Res. 5, 231–240. doi: 10.1016/j.algal.2014.02.003
Lahnsteiner, J., Pisani, P., Menge, J., and Esterhizen, J. (2013). “More than 40 years of direct potable reuse experience in Windohek,” in Milestones in Water Reuse, the Best Success Stories, eds V. Lazarova, T. Asano, A. Bahri, and J. Anderson (London: IWA Publishing), 351–364.
Liu, G. R., and Liu, M. B. (2003). Smoothed Particle Hydrodynamics:A Mesh Free Particle Method. Singapore: World Scientific Publishing Co.Pte. Ltd. doi: 10.1142/9789812564405
Menges, A. (2012). Material computation: higher integration in morphogenetic design. AD 82, 14–21. doi: 10.1002/ad.1374
Müller, M., Heidelberger, B., Hennix, M., and Ratcliff, J. (2007). Position based dynamics. J. Vis. Commun. Image Represent. 18, 109–118. doi: 10.1016/j.jvcir.2007.01.005
Oxman, N., Christine, O., Fabio, G., and Kohler, M. (2015). Material ecology. Comput. Aided Design 60, 1–2. doi: 10.1016/j.cad.2014.05.009
Ozkan, A., and Berberoglu, H. (2013). Physico-chemical surface properties of microalgae. Coll. Surf. B Biointerf. 112, 287–293. doi: 10.1016/j.colsurfb.2013.08.001
Ozkan, A., Kinney, K., Katz, L., and Berberoglu, B. (2012). Reduction of water and energy requirement of algae cultivation using an algae biofilm photobioreactor. Bioresour. Technol. 114, 542–548. doi: 10.1016/j.biortech.2012.03.055
Samsonoff, N. D., Ooms, M., and Sinton, D. (2014). A photosynthetic-plasmonic-voltaic cell: excitation of photosynthetic bacteria and current collection through a plasmonic substrate. Appl. Phys. Lett. 104:043704. doi: 10.1063/1.4863679
Sass, L., and Oxman, R. (2006). Materializing design: the implications of rapid prototyping in digital design. Design Stud. 27, 325–355. doi: 10.1016/j.destud.2005.11.009
Sato, G., Qadir, M., Yamamoto, S., Endo, T., and Zahoor, A. (2013). Global, regional, and country level need for data on wastewater generation, treatment, and use. Agri. Water Manag. 130, 1–13. doi: 10.1016/j.agwat.2013.08.007
Schleicher, S., Lienhard, J., Poppinga, S., Speck, T., and Knippers, J. (2015). A methodology for transferring principles of plant movements to elastic systems in architecture. Comput. Aided Design J. 60, 105–117. doi: 10.1016/j.cad.2014.01.005
Sheil, R. (2013). De-Fabricating Protoarchitecture. In Prototyping Architecture. Building Centre Trust: London.
Steadman, Ph. (2008). The Evolution of Designs: Biological Analogy in Architecture and the Applied Arts. Routledge.
Strauss, M., Drescher, S., Zurbrügg, C., Montangero, A., Cofie, O., and Drechsel, P. (2003). Co-Composting of Fecal Sludge and Municipal Organic Waste a Literature and State-of-Knowledge Review. Ghana: Swiss Federal Institute of Environmental Science & Technology (EAWAG), International Water Management Institute (IWMI).
Tamke, M., Quinn, G., Leander Evers, H., Holden Deleuran, A., and Gengnagel, C. (2014). “The challenge of the bespoke design, simulation and optimisation of a computationally designed plywood gridshell,” in Fusion Proceedings of the 32nd eCAADe Conference (Newcastle upon Tyne), 2, 29–38.
Tsai, T.-C. (2017). Position based dynamics. Encycl. Comput. Graph. Games 92, 1–5. doi: 10.1007/978-3-319-08234-9_92-1
Van Zon, J. S., and ten Wolde, P. R. (2005). Green's-function reaction dynamics: a particle-based approach for simulating biochemical networks in time and space. J. Chem. Phys. 123:234910. doi: 10.1063/1.2137716
Ye, T., Phan-Thien, N., and Lim, C. T. (2016). Particle-based simulations of red blood cells—a review. J. Biomech. 49, 2255–2266. doi: 10.1016/j.jbiomech.2015.11.050
Keywords: bio-energy, microbial fuel cells, biocomputation, residential buildings greywater, particle-based systems, position-based dynamics, high-performance architecture
Citation: Heidari F, Mahdavinejad M, Werner LC, Roohabadi M and Sarmadi H (2021) Biocomputational Architecture Based on Particle Physics. Front. Energy Res. 9:620127. doi: 10.3389/fenrg.2021.620127
Received: 22 October 2020; Accepted: 19 April 2021;
Published: 08 July 2021.
Edited by:
Dinh Duc Nguyen, Kyonggi University, South KoreaReviewed by:
Bachirou Guene Lougou, Harbin Institute of Technology, ChinaDuong La, Ton Duc Thang University, Vietnam
Copyright © 2021 Heidari, Mahdavinejad, Werner, Roohabadi and Sarmadi. This is an open-access article distributed under the terms of the Creative Commons Attribution License (CC BY). The use, distribution or reproduction in other forums is permitted, provided the original author(s) and the copyright owner(s) are credited and that the original publication in this journal is cited, in accordance with accepted academic practice. No use, distribution or reproduction is permitted which does not comply with these terms.
*Correspondence: Mohammadjavad Mahdavinejad, bWFoZGF2aW5lamFkQG1vZGFyZXMuYWMuaXI=