A two-layer multi-energy management system for microgrids with solar, wind, and geothermal renewable energy
- 1School of Automation, China University of Geosciences, Wuhan, China
- 2Hubei Key Laboratory of Advanced Control and Intelligent Automation for Complex Systems, Wuhan, China
- 3Engineering Research Center of Intelligent Technology for Geo-Exploration, Ministry of Education, Wuhan, China
- 4Intelligent Electric Power Grid Key Laboratory of Sichuan Province, Chengdu, Sichuan, China
- 5Department of Electrical and Computer Engineering, Faculty of Science and Technology, University of Macau, Zhuhai, China
The inherent intermittency of high-penetrated renewable energy poses economic and reliable issues of microgrid energy management. This study proposes a two-layer predictive energy management system (PEMS) for high-renewable multi-energy microgrid (MEM). In this MEM, geothermal, solar, and wind energy is converted and conditioned for electricity, thermal, and gas supplies, in which multi-energy complementarities are fully exploited based on electrolytic thermos-electrochemical effects. The proposed microgrid multi-energy management is a complicated and cumbersome problem because of their increasingly tight energy couplings and uncertainties of renewable energy sources (RESs). This intractable problem is thus processed by means of a two-layer PEMS with different time scales, where the system operating costs are minimized in the upper layer and the renewable fluctuations are coped with in the lower layer. Simulation studies on a high-renewable MEM are provided to indicate its effectiveness and superiority over a single time scale scheme. Simulations results show that the operating cost can be reduced by 22.2% with high RESs accommodation.
1 Introduction
Due to the increase of environmental stress caused by global climate changes, renewable energy sources (RESs) have attracted considerable attention (Xu et al., 2019; Huang et al., 2021), and a microgrid is an effective way to integrate high penetration of RESs. However, the system economical and reliability problems of a microgrid are affected by fluctuant RESs (Wei et al., 2022). In order to compensate the renewables fluctuations and supply and demand mismatch, energy storage systems (ESSs) are generally equipped in microgrids for storing and exporting energy. Battery energy storage (BES), as an effective and economical type of ESS, can provide energy flexibility for end-users based on various operational strategies (Jin et al., 2021; Yang et al., 2021). Extensive studies on BESs in various wind and solar microgrids focus on active power coordination (Bao et al., 2020a), real-time charging/discharging management (Zhou et al., 2017a; Zhou et al., 2017b), and long-term microgrid management (Ju et al., 2017). Nevertheless, the battery service life would considerably degrade during frequent charging and discharging.
Power-to-gas (P2G) technology is another creative and advanced ESS, which is explored by extensive researchers as a promising and cost-effective solution to handle fluctuant RESs (Clegg and Mancarella, 2015). Through the thermo-electrochemical reactions in an electrolyzer, surplus power from fluctuant RESs can be converted to gaseous energy carriers, for example, methane and hydrogen (H2) are subsequently stored in a gas storage tank. The hybrid integration of P2G and BES can inherit the advantages of two ESSs in terms of operational costs, power and energy density, and flexibility, which is a cost-effective and highly reliable solution to accommodate fluctuant RESs.
With the increasing utilization of hybrid ESS and combined heat and power (CHP), the electric microgrid gradually transforms toward a multi-energy microgrid (MEM) to simultaneously provide electricity, thermal, and gas energy supplies. By buying from market or producing from P2G, natural gas is used in Xu et al. (2014) and Long et al. (2018) to meet multi-energy demand and offset the fluctuations of wind and solar energy. Biogas, as a renewable substitute gas (Yang et al., 2020; Wu et al., 2021), can be converted via CHP and direct utilization to provide electricity, heating, and lighting services, which is formed for remote areas.
In order to cope with the economic issues and environmental pollution, the utilization of 100% renewables have received the attention of extensive researchers and investment opportunities from operators because of its carbon neutrality and cost reduction performance (Bao et al., 2020b; Bao et al., 2020c; Jin et al., 2020). Based on the energy hub concept, the biogas, solar, and wind 100% renewables (Zhou et al., 2018; Xu et al., 2021; Zhang et al., 2021) are formulated as a MEM for multi-energy carriers, including electricity, thermal, and biogas. Since it can be predicted easily, hydro energy is integrated in Dasgupta et al. (2020), Neto et al. (2020), and Li et al. (2022) to provide local complementarity of solar and wind energy. Based on the P2G technology, ammonia is formed with solar and wind as a 100% renewable MEM (Xu et al., 2021) for coupled electricity, heat, and H2 supplies. Geothermal energy, as a potential RES, can provide multi-energy services, and high-temperature water can be directly fed in a geothermal-to-hydrogen (GTH) electrolyzer for electrolytic gas production (Xu et al., 2020). Extensive efforts by Tasnin and Saikia (2018) and Yilmaz (2020) have explored several different geothermal source combined heat and power systems (GSCHPs) and developed general thermo-economic property. Wind, solar , and geothermal energy are proposed for electrical and multi-energy supplies in Jordehi et al. (2021) and Xu et al. (2022). However, the multi-time scale energy management based on complementary couplings of geothermal, solar. and wind RESs to cope with their inherent intermittency and undispatchability is not involved yet.
In this study, a two-layer predictive energy management system (PEMS) is proposed for geothermal, solar, and wind MEM. Table 1 summarized the differences of typical works. The contributions of the study are as follows:
1) Geothermal, solar, and wind 100% renewable complementarities is proposed to form a MEM, where the input community renewables are efficiently conditioned/converted for output multi-energy supplies. Compared to other electrical microgrids with constant P2G efficiency, thermodynamic behaviors of GTH under fluctuant solar and wind energy feedback are fully analyzed based on a resistor-capacitor network to exploit the 100% renewable multi-energy complementarities. A multi-energy coupling matrix is formulated to systematically express the multi-energy integrated processes.
2) A novel two-layer PEMS is proposed for geothermal, solar, and wind MEM where the system operating costs are minimized in the upper layer and multi-energy supply and demand uncertainties are minimized in the lower layer. Compared to previous PEMS with single time scale, such PEMS allows the optimization of multi-time scale interactive multi-energy couplings for cost-effective enhancement.
3) A short-term multi-energy supply and demand forecasting method is developed based on multi-factor fusion. Instead of only using the supply and demand data in previous literatures, the meteorological factors are also considered, which are extracted based on copula theory to identify their importance and subsequently used to facilitate multi-energy supply and demand forecasting in the extreme gradient boosting (XGBoost) algorithm.
The rest of this article is organized as follows. The problem formulation of multi-energy microgrid is presented in Section 2. In Section 3, the framework of two-layer management system is formulated. Comparative simulation studies under several different schemes are implemented in Section 4 to demonstrate the superiority of the proposed method. Finally, the study is concluded in Section 5.
2 Problem formulation
The geothermal, solar, and wind microgrid energy management involves not only the individual management of multi-energy carriers but also their multi-time scale interactive multi-energy couplings. While the solar and energy can be directly delivered to multi-energy consumers, geothermal energy can be utilized properly to take complementarity into consideration by tapping abundant high-temperature geothermal water to produce clean hydrogen. The thermo-electrochemical reaction performed in the GTH electrolyzer is a fast dynamic process in comparison to other conversion and storage devices (Xu et al., 2020). In such cases, it may be uneconomic to coordinate all the microgrid devices in a single scale because of their different response and degradation cost characteristics. Also, hybridization of geothermal, solar, and wind renewables need to consider individual variability in energy supplies and operational availability. Therefore, the geothermal, solar, and wind microgrid management cannot be easily solved using traditional approaches owing to uncertainties of multi-energy supply and demand, multi-energy couplings, and multi-time scale coordination.
To utilize the geothermal, solar, and wind multi-energy complementarities, this study aims to 1) model and explore the multi-energy complementarities and 2) solve the microgrid multi-energy management problem in a multi-time scale way.
2.1 Multi-energy microgrid structure
Figure 1 shows a geothermal, solar, and wind MEM for multi-energy supply and demand based on the energy hub concept. The proposed MEM functions as a crucial bridge between RESs at the input port and energy-consuming. In this microgrid framework, the hybrid renewable energy is first integrated into photovoltaic thermal system (PVT), wind turbine (WT), electrolyzer, and GSCHP into multi-energy carriers, which are delivered to the consumption of community electricity, heat, and H2 loads.
In this microgrid system, when solar power is unavailable at night, wind turbines could run to generate electricity, which took solar and wind energy complementarity into consideration. Different from solar and wind energy that is greatly affected by environment and meteorological conditions, stable geothermal energy is a flexible RES and can be converted to different energy carriers. The obtained H2 is an advantageous gas, which can keep variable RES in reserve and alternatively convert into electricity and heat. BES and H2 tank serve as energy storage devices to store and condition superfluous energy. When solar and wind energy is scarce, the electrical and gas energy in ESS could be discharged to energy conversion devices in order to obtain an economical and reliable energy supply solution. Flexible energy storage and conversion provide more flexible multi-energy pathways for enhancing economical energy management and RESs accommodation.
2.2 Hybrid energy storage
The aim of hybrid ESS is to inherit the advantages of heterogeneous ESSs in the aspect of response characteristics, operational costs, power and energy density, and flexibility. The hybrid energy storage in this study consists of a H2 storage system and BES because of their mutually complementarities. The H2 storage system is generally composed of an electrolyzer and a H2 tank. The degradation cost of ESS is a common metric to evaluate economic operation of the microgrid. While the main degradation BES is derived from battery, the main degradation of the H2 storage system is derived from the electrolyzer. The degradation of battery lifetime includes the degradation of cycle life and capacity. The number and rates of charging and discharging make a difference on the battery lifetime. Contrary to the complicated battery degradation, only the operating time and on/off cycling conditions have effects on electrolyzer degradation. Remarkable advantages of the electrolyzer in the aspect of low customer acquisition cost, easy to scale up, and long durability is propitious to maintain a longer lifespan and not suffer from cycling issues (Xu et al., 2020). Especially, because of the small capacity in the microgrid, the degradation cost of the electrolyzer or the H2 storage tank is relatively small compared to the BES degradation cost. The hybrid ESS plays a role in offsetting the fluctuations from multi-energy supply and demand and alleviating unnecessary discharging/charging of BES. Consequently, the hybrid ESS has different objectives, where H2 storage is introduced to operate as the primary energy storage mode for supply and demand balance and the BES should be developed to cope with the instant energy unbalances.
2.3 Energy forecasting
In the microgrid, the energy balance between energy production and consumption must be stay at balance to avoid system operation interruptions. Compared to traditional generation systems, the multi-energy supply and demand are generally undispatchable. Thus, energy substitutes are needed to offset the fluctuations of renewable energy when they are scarce in the short term. Better forecastings allow the microgrid manger to deploy less energy storage; however, the problem gets more complex when considering multi-energy carriers. Here, the short-term multi-energy supply and demand forecasting includes forecasting multi-energy loads and solar and wind renewable energy. Though short-term forecasting can be offered up to 7 days in advance, day-ahead multi-energy supply and demand forecasting is adopted to accommodate the market mechanisms and energy management systems. Here is the implementation process of the developed forecasting method:
1) Quantitative analysis is performed based on Copula theory to shed light on the inherent nonlinear relationships among external meteorological factors (including meteorological condition, and geographical location) and multi-energy supply and demand. The key meteorological factors are thus obtained to explore the model for forecasting accuracy enhancement;
2) Multi-energy supply and demand forecasting is performed based on XGBoost algorithm (Liu et al., 2022). XGBoost arranges all the column data in advance and stores them in blocks in the form of compression. Different blocks can be stored in a distributed way, or even in a hard disk. In feature selection, these column data can be processed in parallel to achieve parallelization, and multithreading can be used to achieve acceleration.
3) The commonly used performance indexes, including root mean square error, mean absolute error, and mean absolute percentage error are adopted to evaluate the multi-energy supply and demand forecasting accuracy.
3 Two-layer predictive energy management system
3.1 System model
3.1.1 Thermo-electrochemical model
Based on thermo-electrochemical effects, GTH is another potential energy storage since excrescent electricity and thermal energy can be used to produce storable H2. The model of producing H2 is shown as follows:
Supposing that reaction environment and electrolytic solution are given, and temperature of electrolyte would be the prime affecting factor on reaction courses and H2 generation. By using the piecewise linear, the H2 can be modeled as shown in (2) by fitting the measured data (Clegg and Mancarella, 2015; Xu et al., 2020; Yilmaz, 2020).
The GTH reaction affected by many internal and external factors is complicated, and a RC thermodynamics model is introduced to be convenient for the electrolytic reaction analysis. With the classical lumped parameter method (Xu et al., 2020) the temperature, heat storage and transfer properties are processed into equivalent thermal resistance and capacitance at their geometric center. Based on the Fourier’s law, the GTH dynamics can be expressed in (3) and (4).
From the thermodynamics model in (1)–(4), the gain H2 yield owing to feedback of excess electrical and thermal energy in electrolyzer can be obtained, and multi-energy complementarities can be fully exploited.
With respect to (3), the nonlinear differential thermodynamic model of electrolyzer is handled by means of a Jacobian linearization approach, which are discretized to further problem solving:
where Tk and Tk+1 are temperature state vector; A and B are state and input matrix; uk represents the RESs heating and GTH enthalpy uxes. This approach is proved in (Zhou et al., 2018) to be effective and feasible for nonlinear differential equations.
3.1.2 Multi-energy coupling matrix
According to the converter efficiencies and dispatch factors of conversion and storage devices, microgrid is formulated as a multi-energy coupling matrix in (6) to provide multi-energy flexibility for cost-effective supplies.
By assigning outputs of multi-energy conversion and storage devices as state variables, the nonlinearity in (6) can be handled. The input vector E in (6) could combine with state variables as E′ in (7), as follows:
3.1.3 Energy supply and demand constraints
Constraint (8) represents multi-energy supply and demand balance, which includes total, supplied, and shed loads. Constraints (9) and (10) limit load shedding and maximum electricity to market.
3.1.4 Energy storage system constraints
Constraints (11)–(18) represent state of charge (SOC) balance in hybrid ESS, which includes physics ranges and limits.
3.1.5 Energy conversion constraints
Constraints (19) represents the limits of multi-energy converters.
3.2 Mathematical Model for upper layer predictive energy management system
The operational objective function of the upper layer is the minimization of the system operating costs. Since the fuels of conversion devices are obtained from GTH, the system operating costs only includes power procurement cost PCk and demand shedding cost DCk.
The upper layer PEMS problem in the can be formulated as follows:
3.3 Mathematical model for lower layer predictive energy management system
The operational objective function of the lower layer PEMS is to minimize the effects raised from multi-energy supply and demand forecasting errors. By obtaining parameters from (Zhou et al., 2018), the battery degradation cost BCk can be calculated as follows (24):
The effects raised from multi-energy supply and demand forecasting errors in short-time scales can be formulated as the deviations of references from the upper layer PEMS. The deviations can be augmented with penalty costs to form the penalty terms and can be formulated as a quadratic function:
By integrating the degradation cost of BES and quadratic penalty factors, the lower layer PEMS problem can be formulated as follows:
3.4 Problem solving
As for the two-layer PEMS, the objective is to optimize the energy dispatch in a finite period, so that a reasonable allocation of energy resources is explored to operate economically under volatility and intermittent of multi-energy supply and demand. Based on the model predictive control framework, the two-layer PEMS is formulated as a discrete-time optimization problem with different time scales, where multi-energy supply and demand uncertainties can be corrected through feedback mechanism. Figure 2 shows the flowchart of the proposed two-layer PEMS. The upper layer PEMS is composed of a receding model predictive controller, and the lower layer PEMS is a quadratic model predictive controller. In the upper layer, system operating costs is minimized by solving objective function at the current moment and looking ahead the remaining time slots considering the forecasting of multi-energy supply and demand. Only the optimal results at the current time will be delivered as the base values to the lower layer. After the awareness of multi-energy supply and demand forecasting errors, energy fluctuations each short time interval is minimized in the lower layer PEMS. The lower layer PEMS would return the value to correct the associated value obtained from the upper-layer, and the PEMS problem will start for the next time until the last moment.
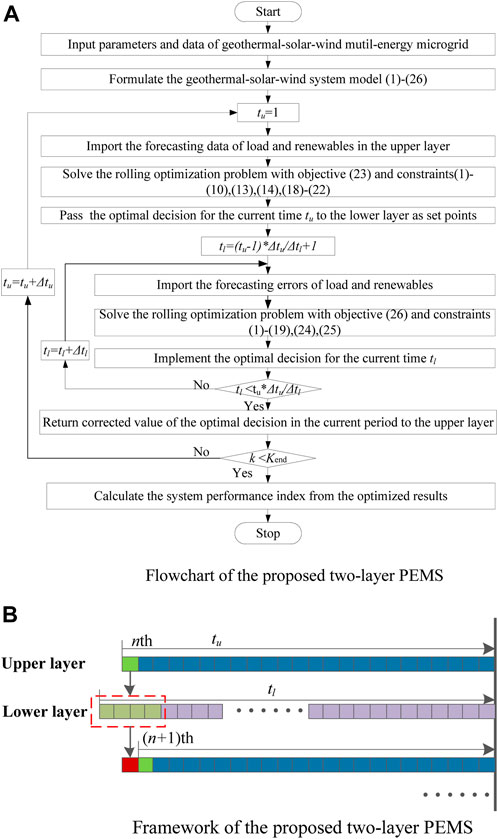
FIGURE 2. (A) Flowchart of the proposed two-layer PEMS. (B) Framework of the proposed two-layer PEMS.
4 Case study
4.1 Base data
Here, the proposed two-layer predictive PEMS is verified on geothermal, solar, and wind MEM. The diagrammatic drawing of MEM and the flowchart of PEMS are illustrated in Figures 1, 2. In order to evaluate its practical performance, the system is equipped with PVT, WT, GSCHP, boiler, CHP, furnace, BES, and H2 tank whose installed capacities are set as 1 MW, 1 MW, 500 kW, 1 MW, 1 MW, 1 MW, 1 MWh and 1000 m3, respectively. To achieve interaction with the electricity market and promote the diversified renewable accommodation, the feed-in price is obtained from (Zhou et al., 2017a) and it is set as 0.04 $/kWh. The actual parameters of the all kinds of multi-energy devices in microgrid system obtained from (Xiao et al., 2010; Zhou et al., 2018; Xu et al., 2020) in demand are listed in Table 2.
4.2 Comparative results
To demonstrate the validity and superiority of the proposed two-layer PEMS of geothermal, solar, and wind MEM, comparative schemes are performed:
1) Scheme 1 is the proposed two-layer PEMS for geothermal, solar, and wind MEM in Sections 2, 3;
2) Scheme 2 is the proposed two-layer PEMS without consideration of the battery degradation cost in (24);
3) Scheme 3 is the single-layer PEMS for the proposed MEM in Section 2.
The three operation schemes are performed for a 24 h with upper and lower layer time intervals setting as 1 h and 15 min, respectively. All the schemes are coded on GAMS on a laptop with 2.3 GHz Intel Core i5 CPU and 8GB RAM, and solved with its default settings.
Figure 3 shows the purchase and sale of daily electricity of schemes 1–3. It can be shown that, because of abundant solar and wind energy during noontime and lower electricity price, a large amount of electricity will be bought at dawn. Scheme 2 is performed with very similar purchase and sale as scheme 1 though without considering the degradation cost. Because of single-layer PEMS, the electricity in scheme 3 is tended to be bought timely to accommodate the shorter time scale fluctuations of solar and wind energy. When considering the intra-hour energy fluctuations, the microgrid in scheme 3 has to buy more electricity for multi-energy supply and demand balance.
Figures 4, 5 show the daily outputs of BES and H2 tanks. Energy storage units are in the state of charging when renewable energy is abundant and sufficient, and are discharged to replenish energy when renewable energy is insufficiency. Although the curve of scheme 1 is performed similarly as scheme 2, less electricity is charged in scheme 1 due to the consideration of the degradation cost. It also can be found that the hybrid ESS in the microgrid can efficiently accommodate the fluctuant solar and wind energy based on their complementary characteristics. While the H2 tank is charged during hours 1–3 and 11–14 to cope with intermittent solar and wind energy to reduce unnecessary BES degradation, the BES is charged and discharged on a short time scale to compensate unstable renewable energy and load owing to their short-term volatility.
The daily outputs of multi-energy converters in schemes 1–3 are shown in the Figures 6–8. The three forms of energy, electricity, thermal and gas can be flexibly converted in multiple energy conversion devices. The high-efficiency CHP and BES in system can meet unstable renewable energy and fluctuant multi-energy loads, while boiler and furnace are used as auxiliary equipment. The output of CHP is at high level to provide electricity and thermal energy because of low-level SOC of BES during hours 18–24. It also can be concluded from Figures 3–8 that the proposed two-layer PEMS scheme can reach efficient coordination among diverse multi-energy conversion equipment, hybrid ESS to regulate the multi-energy carriers. For example, during 6–8 hours, the outputs of BES, furnace, and CHP in schemes 1 and 2 stay at low level, while boiler is used to follow the peak thermal demand.
Table 3 lists the system operating cost, battery degradation cost, electricity procurement, load shedding, and renewable accommodation of schemes 1–3. It can be found that the proposed two-layer PEMS can cost-effectively explore the multi-energy complementarities to supply the multi-energy loads. It is shown that the battery degradation cost of scheme 1 is decreased by 5.5% comparing with schemes 2, which mitigate unnecessary BES charging/discharging actions because of the consideration of battery degradation costs in scheme 1. In terms of scheme 3, the SOC of H2 tanks always stays at high level and electricity is purchased and sold timely with electricity market, which results in lower degradation cost. While sufficient electricity is purchased in the single-layer PEMS, the proposed scheme 1 takes the fluctuation of renewable energy in 15 min into account to reduce system operating cost. As a result, the electricity procurement cost and operating cost of scheme 1 is decreased by 29.5% and 22.2% compared to schemes 3. Because of the synergistic effects of hybrid renewables and electricity market, load shedding is 0 and solar and wind accommodation is 100%. In conclusion, the comparative schemes confirm the advantages of the proposed two-layer PEMS.
5 Conclusion
A geothermal, solar, and wind MEM is proposed for sustainable multi-energy supplies and a two-layer PEMS is proposed to exploit their 100% renewable complementarities from multi-time scales. The two-layer PEMS is performed by considering hybrid ESS degradation characteristics, in which the operational cost is minimized in the upper layer for a long term and fluctuations by multi-energy supply and demand forecast errors are minimized with shorter time intervals in the lower layer. It can be concluded:
1) With the multi-time scale mutual multi-energy couplings, cost-effective utilization of geothermal, solar, and wind RESs can be achieved. More specifically, the operating cost and electricity procurement cost of the proposed two-layer PEMS are decreased by 22.2% and 29.5%.
2) The hybrid energy storage can be utilized complementarily at two layers for multiple decision-making objectives, and the battery degradation cost is decreased by 5.5% because of the consideration of degradation cost.
3) The proposed two-layer PEMS can coordinate energy sources efficiently and accommodate renewable energy at high penetration.
Further research would focus on the multi-time scales of multiple energy carriers.
Data availability statement
The original contributions presented in the study are included in the article/Supplementary Material; further inquiries can be directed to the corresponding author.
Author contributions
All authors listed have made a substantial, direct, and intellectual contribution to the work and approved it for publication.
Acknowledgments
The authors gratefully acknowledge the support of the National Natural Science Foundation of China under Grant 62203413, Macau Young Scholars Program under Grant AM2022005, China Postdoctoral Science Foundation funded project under Grant 2021M692992, Intelligent Electric Power Grid Key Laboratory of Sichuan Province under Grant 2022-IEPGKLSP-KFYB06, and the Fundamental Research Funds for the Central Universities, China University of Geosciences (Wuhan) (No. CUG2106349).
Conflict of interest
The authors declare that the research was conducted in the absence of any commercial or financial relationships that could be construed as a potential conflict of interest.
Publisher’s note
All claims expressed in this article are solely those of the authors and do not necessarily represent those of their affiliated organizations, or those of the publisher, the editors, and the reviewers. Any product that may be evaluated in this article, or claim that may be made by its manufacturer, is not guaranteed or endorsed by the publisher.
References
Bao, W., Ding, L., Liu, Z., Zhu, G., Kheshti, M., Wu, Q., et al. (2020c). Analytically derived fixed termination time for stepwise inertial control of wind turbines—Part I: Analytical derivation. Int. J. Electr. Power & Energy Syst. 121, 106120. doi:10.1016/j.ijepes.2020.106120
Bao, W., Wu, Q., Ding, L., Huang, S., Teng, F., and Terzija, V. (2020b). Synthetic inertial control of wind farm with BESS based on model predictive control. IET Renew. Power Gener. 14, 2447–2455. doi:10.1049/iet-rpg.2019.0885
Bao, W., Wu, Q., Ding, L., Huang, S., and Terzija, V. (2020a). A hierarchical inertial control scheme for multiple wind farms with BESSs based on ADMM. IEEE Trans. Sustain. Energy 12, 751–760. doi:10.1109/TSTE.2020.2995101
Clegg, S., and Mancarella, P. (2015). Integrated modeling and assessment of the operational impact of power-to-gas (P2G) on electrical and gas transmission networks. IEEE Trans. Sustain. Energy 6, 1234–1244. doi:10.1109/TSTE.2015.2424885
Dasgupta, K., Roy, P. K., and Mukherjee, V. (2020). Power flow based hydro-thermal-wind scheduling of hybrid power system using sine cosine algorithm. Electr. Power Syst. Res. 178, 106018. doi:10.1016/j.epsr.2019.106018
Huang, S., Wu, Q., Liao, W., Wu, G., Li, X., and Wei, J. (2021). Adaptive droop-based hierarchical optimal voltage control scheme for VSC-HVDC connected offshore wind farm. IEEE Trans. Ind. Inf. 17, 8165–8176. doi:10.1109/TII.2021.3065375
Jin, X., Wu, Q., Jia, H., and Hatziargyriou, N. (2021). Optimal integration of building heating loads in integrated heating/electricity community energy systems: A bi-level mpc approach. IEEE Trans. Sustain. Energy 12, 1741–1754. doi:10.1109/TSTE.2021.3064325
Jin, X., Wu, Q., and Jia, H. (2020). Local flexibility markets: Literature review on concepts, models and clearing methods. Appl. Energy 261, 114387. doi:10.1016/j.apenergy.2019.114387
Jordehi, A. R., Javadi, M. S., and Catalao, J. P. S. (2021). Optimal placement of battery swap stations in microgrids with micro pumped hydro storage systems, photovoltaic, wind and geothermal distributed generators. Int. J. Electr. Power & Energy Syst. 125, 106483. doi:10.1016/j.ijepes.2020.106483
Ju, C., Wang, P., Goel, L., and Xu, Y. (2017). A two-layer energy management system for microgrids with hybrid energy storage considering degradation costs. IEEE Trans. Smart Grid 9, 6047–6057. doi:10.1109/TSG.2017.2703126
Li, Y., Ming, B., Huang, Q., Wang, Y., Liu, P., and Guo, P. (2022). Identifying effective operating rules for large hydro–solar–wind hybrid systems based on an implicit stochastic optimization framework. Energy 245, 123260. doi:10.1016/j.energy.2022.123260
Liu, B., Xu, D., Jiang, L., Chen, S., and He, Y. (2022). A temporal-spatial model based short-term power load forecasting method in COVID-19 context. Front. Energy Res. 10, 923311. doi:10.3389/fenrg.2022.923311
Long, C., Wu, J., Zhou, Y., and Jenkins, N. (2018). Peer-to-peer energy sharing through a two-stage aggregated battery control in a community Microgrid. Appl. energy 226, 261–276. doi:10.1016/j.apenergy.2018.05.097
Neto, P. B. L., Saavedra, O. R., and Oliveira, D. Q. (2020). The effect of complementarity between solar, wind and tidal energy in isolated hybrid microgrids. Renew. Energy 147, 339–355. doi:10.1016/j.renene.2019.08.134
Tasnin, W., Saikia, L. C., and Raju, M. (2018). Deregulated AGC of multi-area system incorporating dish-Stirling solar thermal and geothermal power plants using fractional order cascade controller. Int. J. Electr. Power & Energy Syst. 101, 60–74. doi:10.1016/j.ijepes.2018.03.015
Wei, J., Wu, Q., Li, C., Huang, S., Zhou, B., and Chen, D. (2022). Hierarchical event-triggered MPC-based coordinated control for HVRT and voltage restoration of large-scale wind farm. IEEE Trans. Sustain. Energy 13, 1819–1829. doi:10.1109/TSTE.2022.3172933
Wu, T., Bu, S., Wei, X., Wang, G., and Zhou, B. (2021). Multitasking multi-objective operation optimization of integrated energy system considering biogas-solar-wind renewables. Energy Convers. Manag. 229, 113736. doi:10.1016/j.enconman.2020.113736
Xiao, W., Cheng, Y., Lee, W. J., Chen, V., and Charoensri, S. (2010). Hydrogen filling station design for fuel cell vehicles. IEEE Trans. Ind. Appl. 47, 245–251. doi:10.1109/TIA.2010.2090934
Xu, D., Wu, Q., Zhou, B., Li, C., Bai, L., and Huang, S. (2019). Distributed multi-energy operation of coupled electricity, heating, and natural gas networks. IEEE Trans. Sustain. Energy 11, 2457–2469. doi:10.1109/TSTE.2019.2961432
Xu, D., Yuan, Z. L., Bai, Z., Wu, Z., Chen, S., and Zhou, M. (2022). Optimal operation of geothermal-solar-wind renewables for community multi-energy supplies. Energy 249, 123672. doi:10.1016/j.energy.2022.123672
Xu, D., Zhou, B., Liu, N., Wu, Q., Voropai, N., Li, C., et al. (2021). Peer-to-peer multienergy and communication resource trading for interconnected microgrids. IEEE Trans. Ind. Inf. 17, 2522–2533. doi:10.1109/TII.2020.3000906
Xu, D., Zhou, B., Wu, Q., Chung, C. Y., Li, C., Huang, S., et al. (2020). Integrated modelling and enhanced utilization of power-to-ammonia for high renewable penetrated multi-energy systems. IEEE Trans. Power Syst. 35, 4769–4780. doi:10.1109/TPWRS.2020.2989533
Xu, X., Jia, H., Chiang, H. D., Yu, D. C., and Wang, D. (2014). Dynamic modeling and interaction of hybrid natural gas and electricity supply system in microgrid. IEEE Trans. Power Syst. 30, 1212–1221. doi:10.1109/TPWRS.2014.2343021
Yang, H., Li, C., Shahidehpour, M., Zhang, C., Zhou, B., Wu, Q., et al. (2020). Multistage expansion planning of integrated biogas and electric power delivery system considering the regional availability of biomass. IEEE Trans. Sustain. Energy 12, 920–930. doi:10.1109/TSTE.2020.3025831
Yang, X., Xu, C., He, H., Yao, W., Wen, J., and Zhang, Y. (2021). Flexibility provisions in active distribution networks with uncertainties. IEEE Trans. Sustain. Energy 12, 1–567. doi:10.1109/TSTE.2020.3012416
Yilmaz, C. (2020). Life cycle cost assessment of a geothermal power assisted hydrogen energy system. Geothermics 83, 101737. doi:10.1016/j.geothermics.2019.101737
Zhang, K., Zhou, B., Li, C., Voropai, N., Li, J., Huang, W., et al. (2021). Dynamic modeling and coordinated multi-energy management for a sustainable biogas-dominated energy hub. Energy 220, 119640. doi:10.1016/j.energy.2020.119640
Zhou, B., Xu, D., Chan, K. W., Li, C., Cao, Y., and Bu, S. (2017a). A two-stage framework for multi-objective energy management in distribution networks with a high penetration of wind energy. Energy 135, 754–766. doi:10.1016/j.energy.2017.06.178
Zhou, B., Xu, D., Li, C., Cao, Y., Chan, K. W., Xu, Y., et al. (2017b). Multi-objective generation portfolio of hybrid energy generating station for mobile emergency power supplies. IEEE Trans. Smart Grid 9, 5786–5797. doi:10.1109/TSG.2017.2696982
Zhou, B., Xu, D., Li, C., Chung, C. Y., Cao, Y., Chan, K. W., et al. (2018). Optimal scheduling of biogas–solar–wind renewable portfolio for multicarrier energy supplies. IEEE Trans. Power Syst. 33, 6229–6239. doi:10.1109/TPWRS.2018.2833496
Nomenclature
Indices and sets
k Time index
∆k Time interval
tu, tl Two-layer time index
Δtu and Δtl Two-layer time interval
n Number of optimizations
Parameters
a1, a2, a3, and a4 Parameters of BES degradation cost
Cz Thermal capacitance of electrolyte
DLe,k,max, DLh,k,max, and DLg,k,max Upper shedding value of multi-energy loads
ER Battery capacity
fWT, fe,PVT, and fh,PVT Energy transformation factors of WT and PVT
Le0,k, Lh0,k, and Lg0,k Total multi-energy loads
Pch,max and Pdis,max Maximum charging and discharging of battery
QH2 Heating value of H2
Rz Rair Equivalent thermal resistances
ri Rate of the ith segment of curve
Sbuy,max and Ssell,max Maximum amount of electricity bought and sold
Ri,j Total thermal resistance between node i and j
SB,max, SCHP,max, and SF,max Upper value of multi-energy converters
SOCBES,ref and SOCBES,avr Reference and average SOC
SOCH2,min and SOCH2,max Minimum and maximum SOC of gas storage
SOCBES,min and SOCBES,max Minimum and maximum SOC of battery
TZ,min and TZ,max Minimum and maximum temperature of electrolysis
Ti Break points
Tout,k External temperature
μbuy,k and μsell,k Price of electricity bought and sold
μBES and rBES Capital cost and rated cycle life of BES
μp and μcd Unit cost of load shedding and battery degradation
VR Total H2 tank volume
VGS,min and VGS,max Minimum and maximum capacity of gas storage
σi,k Coefficients of the i’s penalty costs
σEC and σBC Coefficients of the penalty cost and battery degradation cost
WWT,k, GPVT,k, and Egeo,k Input wind, solar, and geothermal energy
ηch and ηdis Efficiencies of battery charging and discharging
ηB, ηF, ηe,CHP, ηh,CHP, ηe,GS, and ηh,GS Efficiencies of multi-energy converters
Variables
BCk Battery degradation cost
DLe,k, DLh,k, and DLg,k Shedding value of multi-energy loads
DCk Load shedding cost
Epg,k Net electricity procurement
Le,k, Lh,k, and Lg,k Supplied multi-energy loads
Pdis,k and Pch,k Power of battery discharging and charging
PBES,k and VGS,k Net outputs of BES and gas storage
Pgth,k GTH input
PCk Electrical purchasing cost
QRES,k Thermal energy for GTG
Qreaction,k GTH enthalpy uxes
Fu Upper layer system objective function
Fl Lower layer system objective function
Sbuy,k and Ssell,k Amounts of electricity bought and sold
SOCBES,k and SOCH2,k SOC of battery and H2 storage
SB,k, SF,k, and SCHP,k Outputs of boiler, furnace, and CHP
Sef,k and Shf,k Renewable energy for reaction heating
TZ,k Temperature of electrolysis
VH2,k H2 generation
νB,k, νCHP,k, νF,k, νe,k, νh,k, and νg,k Factors of input energy flowed to devices and loads
ηH2,k Electrolytic efficiency of the GTH
Keywords: energy management, renewable energy, energy storage, multi-energy systems, microgrid
Citation: Xu D, Zhong F and Bai Z (2023) A two-layer multi-energy management system for microgrids with solar, wind, and geothermal renewable energy. Front. Energy Res. 10:1030662. doi: 10.3389/fenrg.2022.1030662
Received: 29 August 2022; Accepted: 15 September 2022;
Published: 05 January 2023.
Edited by:
Xiaoshun Zhang, Northeastern University, ChinaReviewed by:
Dawei Chen, Shanghai Jiao Tong University, ChinaShuaifeng Wang, Hunan University, China
Copyright © 2023 Xu, Zhong and Bai. This is an open-access article distributed under the terms of the Creative Commons Attribution License (CC BY). The use, distribution or reproduction in other forums is permitted, provided the original author(s) and the copyright owner(s) are credited and that the original publication in this journal is cited, in accordance with accepted academic practice. No use, distribution or reproduction is permitted which does not comply with these terms.
*Correspondence: Ziyi Bai, eeziyibai@gmail.com