- 1Key Laboratory of Measurement and Control of Complex Systems of Engineering, Ministry of Education, Southeast University, Nanjing, China
- 2NARI Group Corporation (State Grid Electric Power Research Institute), Nanjing, China
Nowadays, developing an integrated energy system (IES) is considered as an effective pattern to improve energy efficiency and reduce energy supply costs. This study proposes a new index—convertibility index (CI)—to quantitatively assess the flexibility of the IES regarding the energy conversion processes between different energy flow types. Based on the CI constraint, a planning problem is modeled as a bi-level optimization problem. To solve the proposed bi-level problem, a hybrid genetic algorithm (GA)—MILP algorithm—is developed. A case study is carried out to verify the effectiveness of the proposed method. The results show that the total cost of the IES will increase with the CI constraint. For a given case study, the total cost increases by 26.2% when the CI decreases to 0.7 and increases by 3.7% when the CI increases to 1.6. Sensitivity analysis shows that the total numbers and capacities of conversion devices show an overall increasing trend with the increase in the CIs. Meanwhile, the total cost decreases quickly at first and then slightly increases, which, in a whole, shows a “Nike” shape. With different CI constraints, the IES MW per CI ranges from 31.8 to 37.5 MW, and the average cost increase is 2.229 million yuan (2.1%/0.1 CI).
1 Introduction
Nowadays, developing an IES is considered an effective pattern to improve energy efficiency and reduce energy supply costs. IESs break the limitation of a single-energy system, which is the development trend of future energy systems (Mancarella, 2014; Fan et al., 2021). IESs contain various energy flow types in energy transmission and conversion systems, including electricity, heating water, cooling water, and natural gas. In conventional non-IESs, each type of energy system is operated independently. However, energy can be converted between various energy flow types in the IESs. For example, the energy carried by electricity, heating water, cooling water, and gas flows can be mutually converted in many devices such as CHP (combined heat and power), CCHP (combined cold, heat, and power supply), EHPs (electric heat pumps), and air conditioners (ACs) (Mancarella, 2014).
This study aims to solve the IES planning problem considering the flexibility requirement. Conversion availability between different energy flow types is a critical feature and advantage of the IES (Jiang et al., 2020), which increases the flexibility of the IES operation. For example, if a fault occurs in the gas transmission system, the gas consumer can have access to gas by power-to-gas (P2G) devices. Another example is that the consumer can alternatively select energy types according to real-time prices. It is worth noting that more energy conversion devices often indicate higher flexibility of the IES, although the cost will be higher, while less energy conversion devices might provide insufficient energy and result in high operation cost. Therefore, the planning problems of the IES are highly related to the flexibility of the IES, and there is a tradeoff between IES flexibility and the total cost.
1.1 Existing Methods About Assessment of IES Flexibility
In the area of power systems, flexibility assessment has been extensively studied. Generation adequacy metrics, such as loss of load expectation (LOLE), expected energy not served (EENS), loss of largest unit (LLU), and insufficient ramping resource expectation (IRRE), have been widely used in flexibility assessment of power systems (Lannoye et al., 2012). Nosair and Bouffard (2015) proposed the concept of a flexibility envelope to describe the flexibility potential dynamics of a power system and its individual resources in the operational planning time frame. For a power system with sustainable generation, Ma et al. (2013) presented an “offline” index to estimate the technical ability of both individual generators and the generation mix to provide the required flexibility. In the field of the IES, Clegg and Mancarella (2016) focused on the power system and the gas system and presented a methodology to quantify the flexibility of the gas network of the power system. Coelho et al. (2020) assessed the flexibility of multi-energy residential and commercial buildings. The authors of this study previously proposed a metric named “load replaceability” to assess the flexibility of the IES in the demand side (Zheng et al., 2019). Meanwhile, more assessment has been made from the perspective of energy efficiency, such as utilization efficiency (EUE) and exergy efficiency (EXE) (Su et al., 2021). Huang et al. (2021) presented indices for IES vulnerability assessment to find the key components for improving IES reliability. Moreover, several literature studies have been undertaken to improve the flexibility of the IES (for example, Good and Mancarella, 2019).
1.2 Existing Methods for Assessment of IES Planning
IES planning refers to making optimal plans for constructing an IES. In recent years, much work on IES planning has been carried out (Fan et al., 2021). Mendes et al. (2011), Mirakyan and De Guio (2013), Mirakyan and De Guio (2015), Farrokhifar et al. (2020) reviewed the research studies in the field of IES planning. Xiang et al. (2020) and Wang et al. (2022) studied the IES planning problem from economic aspects, in which the cost–benefit and exergy efficiencies are analyzed. The investment constraints in IES planning problems are included in Wang et al. (2019a). The environmental issues in IES planning problems are considered in Qin et al. (2019). The electricity–hydrogen IES planning problem is studied in Pan et al. (2020). To consider the participants in the market, a game-theoretic planning method is proposed for IESs (Zhang et al., 2019). Wang et al. (2021) proposed a planning method for the IES based on the life cycle. In Lei et al. (2020), a pipeline risk index for energy network expansion planning is defined as an IES planning problem. As for the mathematical models of IES planning, Ma et al. (2018) optimized the system structure and size of an IES and modeled the problem as a mixed integer linear programming (MILP) problem. Koltsaklis et al. (2014) presented a linear mixed integer programming model for optimal designing and operational planning of energy networks. Koltsaklis and Knápek (2021) presented an optimization framework for optimal scheduling of a multi-energy microgrid based on mixed integer programming techniques, consisting of a number of aggregated end users. In Nicolosi et al. (2021), a novel mixed integer linear programming (MILP) optimization algorithm was developed to compute the optimal management of a micro-energy grid. Zhou et al. (2013) provided a generic energy system engineering framework for optimal designing of IESs in China. Wang et al. (2022), Jing et al. (2018), Wang et al. (2019b), and Qin et al. (2019) built up the optimal planning problem of IESs as multi-objective optimization problems, and the evolutional algorithms were used to solve the problems. A hybrid algorithm combining tabu search algorithm and GA was used in Wang et al. (2022), and a hybrid algorithm combining differential evolution and particle swarm optimization was used in Jing et al. (2018). Wang et al. (2019b) presented a two-stage optimization method for a coupled capacity planning and operation problem, in which the first stage is to optimize the type and capacity of the IES by the non-dominated sorting genetic algorithm-II (NSGA-II) and the second stage is to solve the optimal dispatch and operation problem by MILP. Xiao et al. (2018) modeled IES planning as a bi-level problem in which the optimal operation of the IES under different probability scenarios is the lower-level problem and the optimal planning and design of the IES is the upper-level problem. Wang et al. (2019c) proposed an expansion planning model for the IES to minimize the total cost over the planning horizon and proposed a second-order cone programming and a modified piecewise linearization approach to convert the original mixed integer non-linear programming model to a mixed integer second-order cone programming model.
Based on the aforementioned review, the advantages of the existing models mainly include the following: 1) detailed models of different devices of the IES have been well explored and established; 2) many factors, such as the uncertainties and environmental factors, have been considered. The disadvantages of the existing models mainly include the following: 1) very less attention has been paid directly to the flexibility assessment of the IES, and the existing work on IES flexibility assessment is mainly focused on capacity adequacy. Therefore, there remains a gap in the literature regarding quantitative assessment of the conversion flexibility of the IES. 2) Although plenty of efforts have been focused on IES planning problems, the planning problem has not been fully considered with the flexibility indexes. As IES planning techniques evolve with the challenge of integrating energy conversion devices, the appropriate flexibility to manage different types of energy flows and energy conversions needs to be included.
In this work, we studied the flexibility assessment of the IES regarding the energy conversion process between different energy flow types and proposed a planning method for IESs with CI constraints. The proposed method will be useful to IES planners, investors, or owners who are interested in the following: how to assess IES flexibility? What is the appropriate degree of IES flexibility? How to keep the cost efficiency with appropriate conversion flexibility? The main contributions include the following: 1) an index for quantitative assessment of the conversion flexibility of the IES is proposed. Different from the existing indexes in Clegg and Mancarella (2016), Zheng et al. (2019), and Coelho et al. (2020), the proposed index places the emphasis on flexibility assessment regarding the energy conversion process between different energy flow types. 2) We built a bi-level optimal planning problem of the IES with CI constraints. Different from the existing bi-level planning problems of the IES in Wang et al. (2019b), Xiao et al. (2018), and Wang et al. (2019c), the CI constraints are included in the IES planning to emphasize the conversion flexibility of the IES. A new feature of considering CI is that the planner can obtain the most cost-saving IES plans and meet their CI requirement at the same time.
The remaining of the article is organized as follows: Section 2 presents the CI definition and calculation method; Section 3 presents the bi-level optimization model of IES planning with CI constraints; Section 4 conducts a case study to validate the proposed method; and Section 5 gives conclusions and discussions.
2 Convertibility Index of Integrated Energy System
IESs contain a variety of energy flow types in energy transmission and conversion systems, including electricity, heating water, cooling water, and natural gas. Different energy flows are coupled and connected by energy conversion devices. Figure 1 shows energy conversions between the conversion devices in an IES.
2.1 Definition of Convertibility
Compared to the traditional single-energy system, the IES provides extra availabilities in energy conversion. Here, we define such conversion availability as convertibility. For example, if an energy flow type, for example, A, can be converted from other types of energy flows, we say that energy flow A has convertibility. From the perspective of the end-users, if energy flow A can be converted from other energy flows, it means that energy flow A can be obtained from other types of energy flow and has convertibility. In Figure 1, the heat energy in the heat water pipeline can be converted from the energy in gas by the CHP and a heat exchanger; thus, the heat energy in this system has convertibility.
2.2 Definition of Convertibility Index of a Given Energy Flow Type
If we want to quantitatively assess convertibility, we need an index to measure it. Here, we define the CI of an energy flow type to reflect the conversion flexibility of this energy flow type. As for an IES, there exist four possible types of energy flows in the system. Denote the CI of the cooling flow, heating flow, electric power flow, and gas flow by
where
With the abovementioned definition, the CI of a given energy flow type can reflect the conversion capabilities between the given energy flow and the other energy flow types. If energy can be obtained from larger capacities of the energy conversion devices, the CI of the given energy flow type will be higher. When the CI of a given energy flow is larger than 1, it means that the energy it needs can be fully converted from other energy flow types.
2.3 Definition of Convertibility Index of an Integrated Energy System (αIES)
where
3 Bi-Level Optimization Model of Integrated Energy System Planning Considering Convertibility Index
3.1 Framework
The optimal planning problem aims to minimize the total cost of the IES, and we built up a bi-level optimization problem to achieve the aim. Bi-level optimization is a special kind of optimization where one problem is embedded within another. The outer optimization task is commonly referred to as the upper-level optimization task, and the inner optimization task is commonly referred to as the lower-level optimization task. These problems involve two kinds of variables, referred to as the upper-level variables and the lower-level variables.
The bi-level problem of the IES planning is shown in Figure 2. The upper level of the model determines the types and numbers of the devices in the IES, and these decisions are the inputs of the lower-level model. As for the objective functions, the objective function of the upper-level model is to minimize the annual investment cost and the operation costs, while the objective function of the lower-level model is to minimize the operation cost with a given planned IES.
3.2 Upper-Level Planning Model
The upper-level planning model takes the minimum annual total cost of the system as the objective function. The annual total cost mainly includes the investment cost of the devices and the yearly system operation cost. The decision variables are the types and numbers of the IES devices. The constraints include the system maximum capacity constraints. For studying the impacts of the CI, the constraints with the CI are also included.
3.3 Lower-Level Planning Model
The lower-level planning model takes the minimum operation cost in the typical day as the objective function. The decision variables are the dispatch values of the conversion devices determined by the upper-level problem. The constraints include the supply–demand balance constraints of the cooling, heating, gas, and power; the operation constraints of energy conversion devices; the power limits of the energy conversion devices; the capacity limits of the energy storage; and the power-from-outside limits.
Note that some assumptions have been made for this problem (Mancarella, 2014; Zheng et al., 2019). 1) All the parameters needed for the optimization are assumed to be known. 2) The changes in the parameters along with the operation status are neglected to simplify the model. For example, the temperature impacts on the efficiencies are not considered. Considering that the planning problem covers a long time period, the changes in the parameters are marginal, and therefore the changes in the parameters are neglected. 3) The energy flow optimization is not included. Since the studied IES is not a huge system with long-distance transmission, the energy transmission limits can be neglected. 4) The storage within the gas, cold, and hot water pipes is not considered. For an IES without long-distance transmission, the amount of gas and water within the pipes is minor. Thus, the storage within the pipes is neglected.
3.4 Upper-Level Model
The objective function of the upper-level problem is to minimize the annual total cost, as shown by Eq. 6. The annual total cost includes the annual investment cost of the devices and the yearly system operation cost in the whole year. Here, the investment cost is represented by the annual investment cost (
where
The annual investment cost (
where
The constraints include maximum capacity constraints. To study the impacts of the CI, the constraints with the CI are also included.
3.4 1 Maximum Capacity Constraints
The capacities of each type of the devices should not be larger than a given value. For a given type which might be with more than one device, the total capacities of the given type should not be larger than a given value, as shown by Eq. 9
where
3.4 2 Convertibility Index Constraints
The main difference of the proposed IES planning model from the previous research is that the CI constraints are included. The CI can be calculated by Eq. 10 in which some variables are decision variables of the upper-level model. In the upper-level model, the CI of the IES is fixed at a given value
where
3.5 Lower-Level Model
The objective function of the lower-level problem is to minimize the daily operation cost. The daily operation cost consists of four parts, as shown by (Eq. 12): the energy cost (Eq. 13), the maintenance cost (Eq. 14), the degradation cost of the energy storage (Eq. 15), and the carbon emission cost (Eq. 16). For simplification, the subscript “s” representing the scenarios is neglected in the lower-level model.
where
where
The constraints include the following: the supply–demand balance constraints of the cooling, heating, gas, and power; the operation constraints of energy conversion devices; the power limits of the energy conversion devices; the capacity limits of the energy storage; and the power-from-outside limits.
3.5.1 Supply–Demand Balance Constraints
Similar to the power balance equation in electric grids, the energy supply and demand balances of each energy flow type need to be constrained in the IES. Note that the energy supply side of the type A energy flow might contain the energy flow to be converted to type A, and the energy flow to be converted to other types. Moreover, the energy in and out of the energy storage is also considered. Finally, the supply–demand balance constraints of cooling energy (Eq. 17), heating energy (Eq. 18), electric power energy (Eq. 19), and gas energy (Eq. 20) are guaranteed.
where
3.5.2 Operation Constraints of Energy Conversion Devices: Combined Heat and Power
The CHP plant generates electricity and heat simultaneously. The relationships between input gas power and output electricity or heat power of the backpressure CHP plant are given by Eq. 21 and Eq. 22. The surplus heat, generated by the CHP plant, is partly converted to cooling energy by an absorption refrigerator and partially converted to heating energy by a heat exchanger. The output cooling power of the absorption refrigerator and the output heating power of the heat exchanger are given by Eq. 23 and Eq. 24:
where
where
3.5.3 Operation Constraints of Energy Conversion Devices: Gas Turbine
A gas turbine converts gas energy into electricity energy. The relationship between unit output and gas input per unit time is as follows (Eq. 25):
where
3.5.4 Operation Constraints of Energy Conversion Devices: Gas Boiler
A gas boiler converts gas energy into heat energy. The relationship between output heat power and gas input per unit time is as follows (Eq. 26):
where
3.5.5 Operation Constraints of Energy Conversion Devices: Electric Refrigerator and Electric Heater
An electric refrigerator and electric heater convert electricity power to cooling power and heat power, respectively. The relationships between output power and input power are given by Eq. 27 and Eq. 28:
where
3.5.6 Power Limits of Energy Conversion Devices
The output of the conversion system has its lower/upper limit. The lower/upper output power limits of all kinds of energy conversion devices are given as (Eqs 29–32)
where
3.5.7 Operation Constraints of Energy Storage Devices
The operation constraints of cooling storage, heating storage, and power storage devices mainly include the energy storage state constraints of each device, the energy balance constraints of the energy storage and discharge process, and the energy storage and discharge power constraints. Taking the power energy storage as an example, the operation constraints are given as follows (Eqs 33–39):
where
3.5.8 Power-From-Outside Limits
The lower/upper limits of purchase power of electricity, gas, cooling, and heating energy from the outside system are given as (Eqs 40–43)
where
3.6 Solving algorithm
The proposed model is a bi-level problem. In this study, a hybrid GA–MILP algorithm (Shu et al., 2019) is selected to solve it. That is, GA (Grefenstette, 1988) is implemented in MATLAB to solve the upper-level problem for deriving optimal types and numbers of the devices in the IES. The lower-level problem which seeks for the optimal scheduling of IES and production tasks is an MILP problem, and it is solved by a commercial MILP solver in GAMS with ILOG/CPLEX (ILOG CPLEX, 2018). The connection between GAMS and MATLAB, which iteratively exchanges information between the upper-level and lower-level models, is realized by module GDXMRW. The detailed calculation procedure is presented in Figure 3, and the abstract pseudocode is shown in Table 1.
It is worth mentioning that following three detailed settlements need to be made when applying the proposed model.
3.6.1 Penalty Function for Convertibility Index Constraint
In order to deal with the CI constraint, the penalty function is introduced into the model. The penalty function in GA is formulated as follows (Eq. 44):
where
3.6.2 Fitness Function
In this study, the fitness function of GA is developed by using the ranking method, which is an off-the-shelf function provided by MATLAB. The principle of the ranking method is as follows: 1) sort the objective function of the individual and get the value of position of each individual. 2) According to the value of
where
3.6.3 Termination Criterion
Considering that GA usually only ensures the local optimum, the termination criterion of this problem is set as one of the following two conditions: the optimal fitness value stays within a given threshold or the computation time has reached the maximum iteration time.
4 Case Study
This section presents a case study to verify the effectiveness of the proposed model. Section 4.1 provides the case data and settings. Section 4.2 presents the results without CI constraint. Section 4.3 studies the results with different CI constraints.
4.1 Case Settings and Data
In this section, we present a virtual case to validate the proposed method. This case includes 14 types of candidate energy conversion devices as shown in Figure 4. Some parameters are set according to Wenxia et al. (2020), Zhou et al. (2020), and Jie et al. (2021) and show the energy flow of the IES with all candidate devices. Table 2 presents the parameters of the IES, including capacities of energy conversion devices and energy storage devices, investment cost and maintenance cost per unit, conversion efficiencies, and service life. Three types of energy storage devices are set as must kept devices. Partial data and code are available online.1
4.1.1 Electricity Prices and Gas Prices
In China, electricity prices and gas prices for end users are mostly regulated and set by the provincial National Development and Reform Commission. In this study, we used “time-of-use” price data. The prices of peak, valley, and flat hours are given in Table 3. Note that the time periods of the peak–valley–flat of different places could be different. When using the proposed method, the users can set the prices based on the realized prices in their locations.
4.1.2 Heating and Cooling Prices
The heating prices are 0.225 yuan/kWh and the cooling prices are 0.65 yuan/kWh.
4.1.3 Carbon Emission Tax Prices
The carbon emission tax price is set at 0.14 yuan/kg. The emission efficiency of the IES system is set as 0.25 kg/kWh for electricity, 1.85 kg/m3 for gas, and 0 for cooling and heating.
4.1.4 Load Data
Load curves of three typical days, representing winter, summer, and spring/autumn days, respectively, are selected for the case study. The load curves of the three typical days are shown in Figures 5–7. The number of the three typical days is set as the same. The unit of the y-axis is the load ratio. The real load power is the product of the load ratio and the maximum load. The maximum loads are 7,000 kW for cooling, 8,000 kW for heating, 15,000 kW for power, and 10,000 kW for gas. For example, if the cooling power ratio is 0.9 for a given hour, the actual cooling power is 0.9 * 7,000 kW = 6,300 kW.
The methods and parameters of the GA are given in Table 4.
4.2 Results Without Convertibility Index Constraint
In this section, the results of the planning model without the CI constraint are presented. The IES planning results are shown in Table 5. After optimization, we can calculate the CI of the IES. The CI is calculated according to the number and capacity of energy conversion devices. The CI result is 1.2, and the total cost is 102.392 million yuan.
4.3 Results with Convertibility Index Constraint and Sensitivity Analysis
In order to analyze the results with different CIs, we used 10 cases with different CIs. The
Tables 7, 8 show the planning results of IES under different
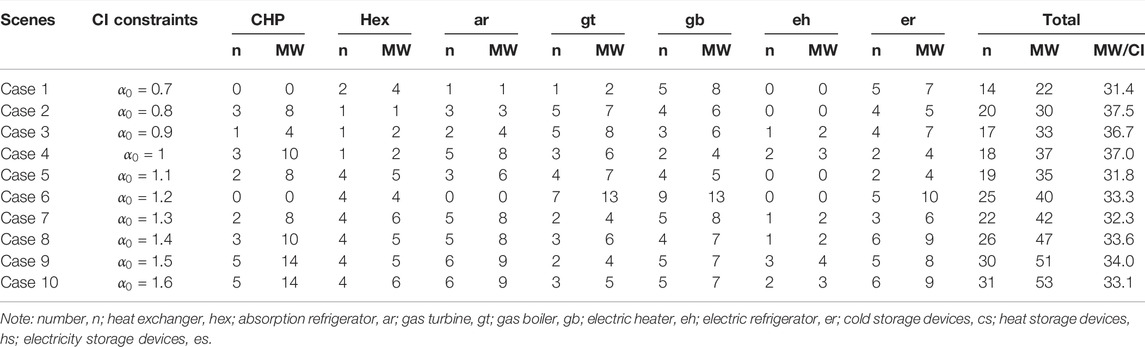
TABLE 8. Planning results with different CIs. (b) Numbers and capacities of each type of energy conversion device.
Table 9 shows the costs of IES planning results in different cases. The annual total cost with different CIs is shown in Figure 8. With the increase in the CIs, the annual total cost at first decreases quickly and then slightly increases, which on a whole shows a “Nike” shape. Compared with the result without CI constraint, when CI (
Looking into the detailed results of the investment cost and operation cost, which are shown in Figures 9, 10, respectively, some more interesting results can be found. The results show that with the increase in the CI, the investment cost continuously increases gradually. When the CI (
4.4 Performance of the Algorithm
The experiments are performed on a Windows-based server at Key Laboratory of Measurement and Control of Complex Systems of Engineering with an Intel(R) i7-9800X CPU (8 cores and 16 threads) and 32 GB of RAM. The hybrid GA–MILP solving algorithm was carried out in MATLAB, GAMS, and ILOG/CPLEX. The average solving time is 10653s. The bi-level planning annual total cost curve is shown in Figure 11. After nearly 120 iterations, the optimal annual total cost is 102.392 million yuan.
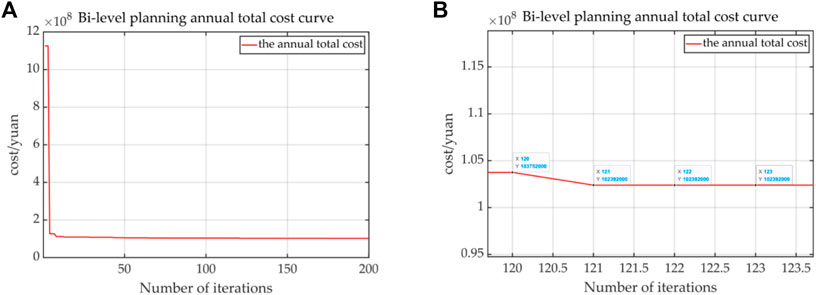
FIGURE 11. Bi-level planning annual total cost curve. (A) Overall convergence curve of the annual total cost. (B) After 120 times of convergence, the convergence value is obtained.
5 Conclusion and Discussion
This study proposes a new index—CI—to quantitatively assess the conversion flexibility of an IES system and builds up a planning problem for the IES with consideration of the impacts of the CI.
The main results of this study are as follows: 1) compared with the results without CI constraint, the CI constraints will increase the total cost. For the given case, the total cost increases by 26.2% when the CI decreases to 0.7 and increases by 3.7% when the CI increases to 1.6. With the continuous increase in the CI, the total cost at first decreases quickly and then slightly increases, which on a whole shows a “Nike” shape. 2) With the continuous increase in the CI, the total numbers and capacities of the conversion devices show an overall increase trend. When the CI increases from 0.7 to 1.6, the total number of the conversion devices increases by 121%, while the total capacity increases by 141%. 3) For the optimal results with different CIs, the MW per CI ranges from 31.8 to 37.5 MW, and the average cost increase is 2.229 million yuan (2.1%/0.1 CI). 4) The problem can be solved within 120 times iterations.
The advantages of the proposed methods are as follows: 1) the proposed index places the emphasis on flexibility assessment regarding the energy conversion process between different energy flow types, which can reflect the overall conversion flexibility of the IES. 2) The planner can obtain the most cost-saving IES plans and meet their CI requirement with CI constraints. The disadvantages of the proposed method are as follows: 1) the planning model is based on several assumptions, for example, the changes in the parameters with the operation status are neglected; and 2) the GA-based algorithm cannot ensure global optimum and is time consuming. The future research direction will be two-folded: 1) considering more practical issues in the planning problem, for example, using a practical system with real system data for simulation; and 2) exploring more efficient modeling and solving algorithms, for example, modeling it as a dual-objective optimization problem with the largest CI and the smallest annual total cost to find the Pareto surface.
Data Availability Statement
The original contributions presented in the study are included in the article/Supplementary Material, further inquiries can be directed to the corresponding author.
Author Contributions
Conceptualization: KZ; methodology: YW; software: FK; validation: TZ; writing: YW, KF and JZ; funding acquisition: KZ. All authors have read and agreed to the published version of the manuscript.
Funding
This study received funding from the National Natural Science Foundation of China (No. 51907025) and “Zhishan Young Scholar” of Southeast University (No. 2242021R41176). The funder was not involved in the study design, collection, analysis, interpretation of data, the writing of this article, or the decision to submit it for publication.
Conflict of Interest
Author TZ was employed by the company NARI Group Corporation (State Grid Electric Power Research Institute).
The remaining authors declare that the research was conducted in the absence of any commercial or financial relationships that could be construed as a potential conflict of interest.
Publisher’s Note
All claims expressed in this article are solely those of the authors and do not necessarily represent those of their affiliated organizations, or those of the publisher, the editors and the reviewers. Any product that may be evaluated in this article, or claim that may be made by its manufacturer, is not guaranteed or endorsed by the publisher.
Footnotes
1Data [Online] is available at: https://github.com/wangyingrice/IES_planning.git.
References
Clegg, S., and Mancarella, P. (2016). Integrated Electrical and Gas Network Flexibility Assessment in Low-Carbon Multi-Energy Systems. IEEE Trans. Sustain. Energ. 7 (2), 718–731. doi:10.1109/TSTE.2015.2497329
Coelho, A., Soares, F., and Peças Lopes, J. (2020). Flexibility Assessment of Multi-Energy Residential and Commercial Buildings. Energies 13 (11), 2704. doi:10.3390/en13112704
Fan, H., Yu, Z., Xia, S., and Li, X. (2021). Review on Coordinated Planning of Source-Network-Load-Storage for Integrated Energy Systems. Front. Energ. Res. 9, 138. doi:10.3389/fenrg.2021.641158
Farrokhifar, M., Nie, Y., and Pozo, D. (2020). Energy Systems Planning: A Survey on Models for Integrated Power and Natural Gas Networks Coordination. Appl. Energ. 262, 114567. doi:10.1016/j.apenergy.2020.114567
Good, N., and Mancarella, P. (2019). Flexibility in Multi-Energy Communities with Electrical and thermal Storage: A Stochastic, Robust Approach for Multi-Service Demand Response. IEEE Trans. Smart Grid 10, 503–513. doi:10.1109/TSG.2017.2745559
Grefenstette, J. J. (1988). Genetic Algorithms and Machine Learning. Machine Learn. 3 (2), 95–99. doi:10.1023/A:1022602019183
Huang, W., Du, E., Capuder, T., Zhang, X., Zhang, N., Strbac, G., et al. (2021). Reliability and Vulnerability Assessment of Multi-Energy Systems: An Energy Hub Based Method. IEEE Trans. Power Syst. 36, 3948–3959. doi:10.1109/TPWRS.2021.3057724
ILOG CPLEX. Homepage (2018). Available at: http://www.ilog.com (Accessed October 10, 2019).
Jiang, Y., Wan, C., Botterud, A., Song, Y., and Xia, S. (2020). Exploiting Flexibility of District Heating Networks in Combined Heat and Power Dispatch. IEEE Trans. Sustain. Energ. 11 (4), 2174–2188. doi:10.1109/TSTE.2019.2952147
Jie, D., Fei, J., Wenye, W., Guixiong, H., and Xinhe, Z. (2021). Study on Cascade Optimization Operation of Park-Level Integrated Energy System Considering Dynamic Energy Efficiency Model. Power Syst. Techn. 46 (03), 1027–1039. doi:10.13335/j.1000-3673.pst.2021.0484
Jing, R., Zhu, X., Zhu, Z., Wang, W., Meng, C., Shah, N., et al. (2018). A Multi-Objective Optimization and Multi-Criteria Evaluation Integrated Framework for Distributed Energy System Optimal Planning. Energ. Convers. Manage. 166, 445–462. doi:10.1016/j.enconman.2018.04.054
Koltsaklis, N. E., and Knápek, J. (2021). Optimal Scheduling of a Multi-Energy Microgrid. Chem. Eng. Trans. 88, 901–906. doi:10.3303/CET2188150
Koltsaklis, N. E., Kopanos, G. M., and Georgiadis, M. C. (2014). Design and Operational Planning of Energy Networks Based on Combined Heat and Power Units. Ind. Eng. Chem. Res. 53 (44), 16905–16923. doi:10.1021/ie404165c
Lannoye, E., Flynn, D., and O'Malley, M. (2012). Evaluation of Power System Flexibility. IEEE Trans. Power Syst. 27 (2), 922–931. doi:10.1109/TPWRS.2011.2177280
Lei, Y., Wang*, D., Jia*, H., Chen, J., Li, J., Song, Y., et al. (2020). Multi-objective Stochastic Expansion Planning Based on Multi-Dimensional Correlation Scenario Generation Method for Regional Integrated Energy System Integrated Renewable Energy. Appl. Energ. 276. doi:10.46855/2020.06.11.05.59.391937
Ma, J., Silva, V., Belhomme, R., Kirschen, D. S., and Ochoa, L. F. (2013). Evaluating and Planning Flexibility in Sustainable Power Systems. IEEE Trans. Sustain. Energ. 41, 200–209. doi:10.1109/tste.2012.2212471
Ma, T., Wu, J., Hao, L., Lee, W.-J., Yan, H., and Li, D. (2018). The Optimal Structure Planning and Energy Management Strategies of Smart Multi Energy Systems. Energy 160, 122–141. doi:10.1016/j.energy.2018.06.198
Mancarella, P. (2014). MES (Multi-energy Systems): An Overview of Concepts and Evaluation Models. Energy 65, 1–17. doi:10.1016/j.energy.2013.10.041
Mendes, G., Ioakimidis, C., and Ferrão, P. (2011). On the Planning and Analysis of Integrated Community Energy Systems: A Review and Survey of Available Tools. Renew. Sustain. Energ. Rev. 15 (9), 4836–4854. doi:10.1016/j.rser.2011.07.067
Mirakyan, A., and De Guio, R. (2013). Integrated Energy Planning in Cities and Territories: A Review of Methods and Tools. Renew. Sustain. Energ. Rev. 22, 289–297. doi:10.1016/j.rser.2013.01.033
Mirakyan, A., and De Guio, R. (2015). Modelling and Uncertainties in Integrated Energy Planning. Renew. Sustain. Energ. Rev. 46, 62–69. doi:10.1016/j.rser.2015.02.028
Nicolosi, F. F., Alberizzi, J. C., Caligiuri, C., and Renzi, M. (2021). Unit Commitment Optimization of a Micro-grid with a MILP Algorithm: Role of the Emissions, Bio-Fuels and Power Generation Technology. Energ. Rep. 7, 8639–8651. doi:10.1016/j.egyr.2021.04.020
Nosair, H., and Bouffard, F. (2015). Flexibility Envelopes for Power System Operational Planning. IEEE Trans. Sustain. Energ. 6 (3), 800–809. doi:10.1109/TSTE.2015.2410760
Pan, G., Gu, W., Lu, Y., Qiu, H., Lu, S., and Yao, S. (2020). Optimal Planning for Electricity-Hydrogen Integrated Energy System Considering Power to Hydrogen and Heat and Seasonal Storage. IEEE Trans. Sustain. Energ. 11, 2662–2676. doi:10.1109/TSTE.2020.2970078
Qin, C., Yan, Q., and He, G. (2019). Integrated Energy Systems Planning with Electricity, Heat and Gas Using Particle Swarm Optimization. Energy 188, 116044. doi:10.1016/j.energy.2019.116044
Shu, J., Guan, R., Wu, L., and Han, B. (2019). A Bi-level Approach for Determining Optimal Dynamic Retail Electricity Pricing of Large Industrial Customers. IEEE Trans. Smart Grid 10 (2), 2267–2277. doi:10.1109/TSG.2018.2794329
Su, H., Huang, Q., and Wang, Z. (2021). An Energy Efficiency Index Formation and Analysis of Integrated Energy System Based on Exergy Efficiency. Front. Energ. Res. 9. doi:10.3389/fenrg.2021.723647
Wang, J., Du, W., and Yang, D. (2021). Integrated Energy System Planning Based on Life Cycle and Emergy Theory. Front. Energ. Res. 9, 713245. doi:10.3389/fenrg.2021.713245
Wang, J., Hu, Z., and Xie, S. (2019c). Expansion Planning Model of Multi-Energy System with the Integration of Active Distribution Network. Appl. Energ. 253, 113517. doi:10.1016/j.apenergy.2019.113517
Wang, Y., Huang, F., Tao, S., Ma, Y., Ma, Y., Liu, L., et al. (2022). Multi-objective Planning of Regional Integrated Energy System Aiming at Exergy Efficiency and Economy. Appl. Energ. 306, 118120. doi:10.1016/j.apenergy.2021.118120
Wang, Y., Li, R., Dong, H., Ma, Y., Yang, J., Zhang, F., Zhu, J., and Li, S. (2019a). Capacity Planning and Optimization of Business Park-Level Integrated Energy System Based on Investment Constraints. Energy 189, 116345. doi:10.1016/j.energy.2019.116345
Wang, Y., Wang, Y., Huang, Y., Li, F., Zeng, M., Li, J., et al. (2019b). Planning and Operation Method of the Regional Integrated Energy System Considering Economy and Environment. Energy 171, 731–750. doi:10.1016/j.energy.2019.01.036
Wenxia, L., Zhengzhou, L., Yue, Y., Fang, Y., and Yao, W. (2020). Collaborative Optimal Configuration for Integrated Energy System Considering Uncertainties of Demand Response. Automation Electric Power Syst. 44 (10), 9. doi:10.7500/AEPS20190731013
Xiang, Y., Cai, H., Gu, C., and Shen, X. (2020). Cost-benefit Analysis of Integrated Energy System Planning Considering Demand Response. Energy 192, 116632. doi:10.1016/j.energy.2019.116632
Xiao, H., Pei, W., Pei, W., Dong, Z., and Kong, L. (2018). Bi-level Planning for Integrated Energy Systems Incorporating Demand Response and Energy Storage under Uncertain Environments Using Novel Metamodel. Csee Jpes 4 (2), 155–167. doi:10.17775/CSEEJPES.2017.01260
Zhang, X., Wang, H., Hu, J., Zhou, B., Zhang, Y., and Qiu, J. (2019). Game-theoretic Planning for Integrated Energy System with Independent Participants Considering Ancillary Services of Power-To-Gas Stations. Energy 176, 249–264. doi:10.1016/j.energy.2019.03.154
Zheng, T., Dai, Z., Yao, J., Yang, Y., and Cao, J. (2019). Economic Dispatch of Multi-Energy System Considering Load Replaceability. Processes 7, 570. doi:10.3390/pr7090570
Zhou, X., Yonghui, S., Dongliang, X., Jianxi, W., and Yongjie, Z. (2020). Optimal Configuration of Energy Storage for Integrated Region Energy System Considering Power/Thermal Flexible Load. Automation Electric Power Syst. 44 (2), 7. doi:10.7500/AEPS20190620005
Keywords: integrated energy system, convertibility index, optimal planning, bi-level optimization, hybrid genetic algorithm
Citation: Wang Y, Zhao J, Zheng T, Fan K and Zhang K (2022) Optimal Planning of Integrated Energy System Considering Convertibility Index. Front. Energy Res. 10:855312. doi: 10.3389/fenrg.2022.855312
Received: 15 January 2022; Accepted: 14 March 2022;
Published: 14 April 2022.
Edited by:
Xiaoxian Yang, Shanghai Second Polytechnic University, ChinaReviewed by:
Bingyuan Hong, Zhejiang Ocean University, ChinaNikolaos Koltsaklis, Czech Technical University in Prague, Czechia
Yongtu Liang, China University of Petroleum, China
Honghao Gao, Shanghai University, China
Copyright © 2022 Wang, Zhao, Zheng, Fan and Zhang. This is an open-access article distributed under the terms of the Creative Commons Attribution License (CC BY). The use, distribution or reproduction in other forums is permitted, provided the original author(s) and the copyright owner(s) are credited and that the original publication in this journal is cited, in accordance with accepted academic practice. No use, distribution or reproduction is permitted which does not comply with these terms.
*Correspondence: Kaifeng Zhang, a2FpZmVuZ3poYW5nQHNldS5lZHUuY24=