- Dipartimento di Elettronica, Informazione e Bioingegneria, Politecnico di Milano, Milano, Italy
This paper presents a comparative analysis of different modeling and control techniques that can be used to tackle the energy efficiency and management problems in buildings. Multiple resources are considered, from generation to storage, distribution and delivery. In particular, it is shown what are the real needs and advantages of adopting different techniques, based on different applications, type of buildings, boundary conditions. This contribution is based widely on the experience performed by the authors in the recent years in dealing with existing residential, commercial and tertiary filed buildings, with application ranging from local temperature control up to smart grids where buildings are seen as an active node of the grid thanks to their ability to shape the thermal and electrical profile in real time. As for control models, a wide range of modeling techniques are here investigated and compared, from linear time-invariant models, to time-varying, to nonlinear ones. Similarly, control techniques include adaptive ones and real-time predictive ones.
1 Introduction
The idea of smartness or highly energy-efficient and decarbonized building sector has gained remarkable popularity over the last few years. Recent studies showed that about 40% of total energy consumption accounts for the building sectors, residential, commercial and industrial ones (European Union, 2010).
In this context, the need for advanced control systems for efficient usage of thermo-electrical devices has become crucial to contribute to energy-efficient buildings and cities, as well as to gain flexibility for the loads in a future scenario dominated by erratic renewable sources. In this regard, there have been studies on building energy management associated with the control algorithms that covers classic to advanced methods. These approaches focused on temperature control (Hazyuk et al., 2012), optimal control of HVAC systems (Manjarres et al., 2017), energy optimization for a group of buildings connected to heat pumps (Staino et al., 2016), HVAC, battery energy storage, and renewable generation coordinator (Biyik and Kahraman, 2019).
To this scope, in this paper, a brief review on energy management systems, available control approaches and modeling algorithms is performed. Special attention is devoted to heat pumps, not only for their effectiveness in generating thermal energy from electrical energy, but also because they show wide margins for saving further energy. Actually, their inner efficiency is a nonlinear function of the operating conditions and disturbances, thus paving the way to optimal strategies, in real time, to store energy–in any form–when it is more convenient and use it when it is needed to meet userrequirements. Such a special behavior will be well detailed in the paper. Notice that due to the wide variety of topics discussed in the paper a detailed literature review has been performed in each of the following chapters.
1.1 Aim of Study
This paper provides a systematic approach to the energy management and control problem for buildings of different sizes. It is mostly based on a structured analysis of the recent authors’ investigations over the last decade in the following fields:
• Control-oriented modeling of thermal and electrical components as well as building envelopes and their couplings
• Model Predictive Control approaches (from linear to nonlinear techniques)
• Role of heat pump COP estimation and prediction for energy efficiency
• Experimental testing in small to large buildings with HVAC system
The study aims at providing new researchers with a quick start on the topic and experienced researchers with a summary of recent practical and theoretical experiences for future research.
1.2 Paper Organization
The paper is organized as follows. Section 2 reviews the modeling approaches used for the energy efficiency application in buildings. A review of the control strategies is developed in Section 3, while the guideline for controller selection based on application case study is given in Section 4. The possible challenges and real applications are given in Section 5. Section 6 concludes the paper.
2 Building Modeling for Energy Efficiency Applications
An accurate estimation and prediction of the energy consumption of buildings is crucial to enforce efficiency. However, one should first ask himself: what is the meaning of energy efficiency? And how many different types of models are available? A comprehensive comparative analysis of the available modeling technologies is provided in (Koulamas et al., 2018) where the authors encompasses bottom-up and top-down approaches, white/gray/black box models, calculation-based and data-based models.
A widespread calculation-based quasi-steady-state physical model is provided by the ISO 13790 standard discussed also in (Kalogeras et al., 2019). In the paper, the model is successfully compared to ISO 52016 standard, updating ISO 13790. In addition, the predictive capability of this model is ascertained using the simulation results of EnergyPlus dynamic simulator, as a benchmark software.
Apart from some numerical errors that appear in some mid-season months, the above models are very useful to compute a monthly or yearly consumption of a building.
However, from a control point of view, suitable short-time “control-oriented” dynamic models need to be developed to evaluate the building energy performance during the transient behaviour within a time frame typical of temperature control systems, usually measured in minutes/hours.
In this perspective, there are several different modelling techniques to represent the thermal behavior of buildings.
The resistor-capacitor (RC) network model has been widely used in the application of building energy efficiency programs. This kind of first principle modeling approach is linear based on an RC equivalent network corresponding to lumped-parameter energy balance equations. In (Yang et al., 2018) a method is proposed to develop an integrated state-space model for indoor air and radiant temperature together with a quality index, namely Predicted Mean Vote, suitable for fast real-time optimization. Similar approach is used in (Ferrarini et al., 2017) for control-oriented modeling of an office-size space.
In these approaches, generally, some parameters can be identified in a white-box fashion using physical information related to the envelope, materials, indoor volumes and so on. Some others are instead more difficult to be computed on a priori knowledge. Thus, they are either estimated using data from literature or regulations or tuned using directly measurement data information, which makes them gray-box models.
Such a hybrid white/gray box approach is applied in some application cases discussed in the following sections. In particular, in Section 5. For more case studies you may refer to (Dong et al., 2013; Lin and Yang, 2018; Zhang et al., 2022).
3 Classic vs Predictive Control Strategies
In the technical literature, there are many control approaches for energy management applications in buildings. The widest applied techniques are classic feedback regulators and predictive optimal controllers. For the sake of brevity, only a quick hint is provided for classic controls, while more attention is paid to predictive ones.
3.1 Classic Control Algorithms
Classic feedback controllers are often used for their simplicity and effectiveness. These are used for indoor temperature and humidity control, or for heating water temperature control, or even power control of the compressor of a heat pump.
There are two main categories: discrete and continuous ones. The first category is based on a hysteretical behaviour over a threshold with a predefined interval (for example, for indoor temperature we can set 20°C with 0.5°C tolerance, through a thermostat). The second category is based on PI/PID controllers.
Although both of them are effective, discrete controllers induce an oscillating behavior on the control variables. Such fluctuations may reduce the comfort as well as the residual life of components (e.g., electro-valves).
But there is one more important drawback: the discrete approach is definitely not good in presence of high-inertia systems. As an example, consider a radiant panel heating system. Typically, the water/pavement is at around 30–35°C to have an indoor temperature of 20°C. If the discrete controller switches off the heating at 20.5°C of indoor temperature, the pavement remains at 30–35°C for quite some time, still heating the room. The unwanted result is that the room temperature will then increase much beyond the original set point, and the original threshold.
On the contrary, continuous PI/PID controllers avoid fluctuations, but have the problem of tuning, that threatens many technicians during commissioning. However, PI/PID tuning (online or offline) is a well-addressed topic, especially for buildings applications. The interested reader may refer to (Salpakari and Lund, 2016; Ferrarini et al., 2017; Ferrarini and Babini, 2020) for interesting case studies in residential and tertiary field applications. Results show that even a simple clustering technique allows finding proper tuning, and trivial rule-based enhancement can increase energy efficiency of about 12.6%, without the need for structural retrofitting.
Although PI/PID algorithms provide a reliable and safe solution, there is no simple way to enforce constraints and physical limitations of the system. Furthermore, PIDs are usually single-input single-output control algorithms and they are not able to deal with multi-input/output systems. In this case, the interaction between different loops cannot be effectively seen by the controller which leads to a longer time response or more fluctuations.
3.2 Advanced Predictive Control Approaches
As said, classic control methods are applied in the majority of building energy management systems. This is mainly due to their simplicity (in both design and implementation), while still showing good performance. So, why should other control techniques be investigated?
An easy and technical answer is that the recent progress in communication protocols and industrial processors opened the way for implementation of advanced control algorithms. But what are the advantages in doing that? Concrete advantages of using advanced control algorithms are undeniable, such as:
• the ability to include forecasts
• the possibility to handle constraints of the system
• the ability to track multiple objectives (e.g., comfort vs energy saving)
• the flexibility towards changing boundary conditions
• the ability to operate the system around the optimum point
• the possibility to handle system nonlinearities
• the possibility to handle a time-varying behavior
As an example, if heat pumps are exploited for air conditioning, it is well known that their efficiency is not constant at all, being a nonlinear function of specific quantities of the operational condition as well as weather disturbances (at least for air-to-water pumps). With advanced control techniques, it is possible to make the heat pump work when it is more efficient, transforming the potential disadvantage of a complex nonlinear behavior (efficiency changing over time), into a concrete advantage.
In addition, it is possible to find a compromise in real time between storing energy when it is cheap and using it when it is necessary. Would that be possible with traditional control schemes?
Here in this section, different types of model predictive control algorithms (MPC) are analysed and compared for building energy management scenarios. MPC techniques achieved a high performance in a wide range of industries as they can accommodate a performance index (optimal solution) and constraints satisfaction (actuator and operational limitations). It has been also used successfully with the application of smart buildings, especially the ones integrated with heat pumps [see (Verhelst et al., 2012; Kajgaard et al., 2013)]. In particular cases with variable electricity prices, it shows a significant energy saving (cost reduction up to 35%).
In this regard, the type of model and mathematical formulation of the predictor defines the type of MPC algorithm. Generally, it ranges from linear to nonlinear methodologies (Rastegarpour et al., 2020a).
The aim is always to optimize the building operation with respect to both thermal comfort and energy cost. Obviously, those are conflicting objectives, so that a multi-objective optimization problem is to be selected.
Therefore, a weighted sum of the energy cost (Je) and thermal discomfort cost (Jd) is evaluated over a prediction horizon of N, as follows:
where γ1 and γ2 are the respective normalized weighting factors. The MPC aims at calculating the optimal control profile to minimize the total cost Jt over time. The optimization problem is subject to the system dynamics, actuator limitations and system constraints.
3.2.1 Linear MPC
Usually, in practical applications, the computational performance is prioritized over model accuracy. In such cases, a linear representation of the system dynamics should be obtained and used as the predictor agent inside the optimization problem. Therefore, linear or convex quadratic problems are formulated to find the optimal control profile, which provides cheaper computational effort.
The linear representation of the system dynamic can be obtained either through classic linearization techniques (Rastegarpour et al., 2020a) or developing first principle linear models (Rastegarpour et al., 2018). The latter includes many strong assumptions in order to separate the linear part of the model from the nonlinear one, tuning the MPC on the linear process model only (Mantovani and Ferrarini, 2015).
On the contrary, classic linearization technique obtains a linearized state-space model of the process around a certain operating point. In this case, there could be some stability issues depending on the level of nonlinearity of the system.
In the application of building energy management, however, the dynamics of thermal storages is nonlinear with respect to the circulated water flow rate within the tank layers. The heat pump dynamic is another potential source of nonlinearity due to its strong load dependency. The dynamical behavior of this thermo-electrical equipment can dramatically change during the transition from one operating point to another. Hence, a single linear MPC designed at a particular operating point does not necessarily give satisfactory control performance over a wide operating range.
In (Rastegarpour et al., 2020a), authors studied a MPC algorithm with successive linearization, named SMPC. In the SMPC approach, the model is linearized at each sampling instant based on the current operating point. The linearized model remains fixed over the prediction horizon. In this way, at every sampling time, the “best” linearized model is used to perform prediction and control.
However, as discussed in (Rastegarpour et al., 2020a), the SMPC achieves acceptable performance as long as the system has a behavior close to linear within the prediction horizon, while it may fail when the dynamic behavior of the system varies significantly during the prediction horizon. In the latter case, the state prediction is not accurate anymore. To tackle this problem, the proposed SMPC is refined in (Rastegarpour et al., 2020a), where the system dynamic is linearized around a reference trajectory instead of the current operating point. The approach is called linear time-varying MPC (namely LTV-MPC) and takes into account also time-varying behaviour.
The reference trajectory can be initialized with a simple off-line open-loop optimization with the prediction horizon of N, and then, starting from the second sampling time onwards, it is updated at every sampling time using the trajectory computed as an optimization result of the MPC at the previous sampling time.
It is worth noticing that the proposed linear MPC algorithms yield a convex quadratic program (QP) for which specific tools exist.
The simulation results of these algorithms show that the LTV-MPC approach obtains a significantly better performance when compared to the SMPC. Even more, the numerical solution is quite close to the optimal one and performs similarly to the nonlinear algorithms (Rastegarpour et al., 2019a; Rastegarpour et al., 2020a; Rastegarpour et al., 2021).
3.2.2 Nonlinear MPC
If the nonlinear dynamics of the system are used in the optimization problem, then the resulting optimal control problem holds nonlinear constraints. Therefore, the available linear and convex solutions are not valid anymore. Therefore, nonlinear control solutions should be employed.
The type of nonlinear predictive controller differs depending on the type of information used to predict the system’s dynamics. In general, we can divide them into the following categories:
• fuzzy models: these are mainly based on if-then rules which play the role of expert knowledge to represent the system’s behavior (Škrjanc et al., 2001).
• nonlinear autoregressive network (with exogenous inputs): it is using historical or current measurements to learn a pattern and predict its future value; artificial neural networks are the most common ones in this category (Raza and Khosravi, 2015).
• explicit first principle models: these are mainly based on the energy conservation laws on the control volume under study.
The nonlinear MPC algorithms usually go under the category of model-based methods where a representation of the physical system is provided through the explicit first principle approach. This model is then used to formulate the optimization problem for finding an optimal control trajectory.
In this case, shooting methods and interior point optimization are used for the solution of the optimal control problem.
In (Rastegarpour et al., 2020a) authors used an RK4 technique to discretize the nonlinear dynamic of the system. The optimal control profile is calculated through the solution of a nonlinear program solved by a primal–dual interior point method, using the software IPOPT. The paper also compares the linear and nonlinear MPC algorithms for the application of building energy management. A quality index is introduced to better evaluate the impact of model uncertainties on the control performance.
A similar nonlinear MPC method is used for the economic optimization of multiple floor-heating buildings (Rastegarpour et al., 2020b), which will be reviewed in Section 5.
4 Controller Selection for Building Energy Management
As reviewed in previous section, different control algorithms can be exploited to obtain a higher level of sustainability in buildings. To this scope, different types of predictive or non-predictive algorithms can be formulated depending on the dynamical behavior of the system, from linear, to nonlinear, to time-varying, to time-invariant ones. Table 1 summarizes the proposed modeling and control approaches for building energy management purposes. This is an easy guide for those who are going to select a modeling or control approach depending on their applications and their limitations.
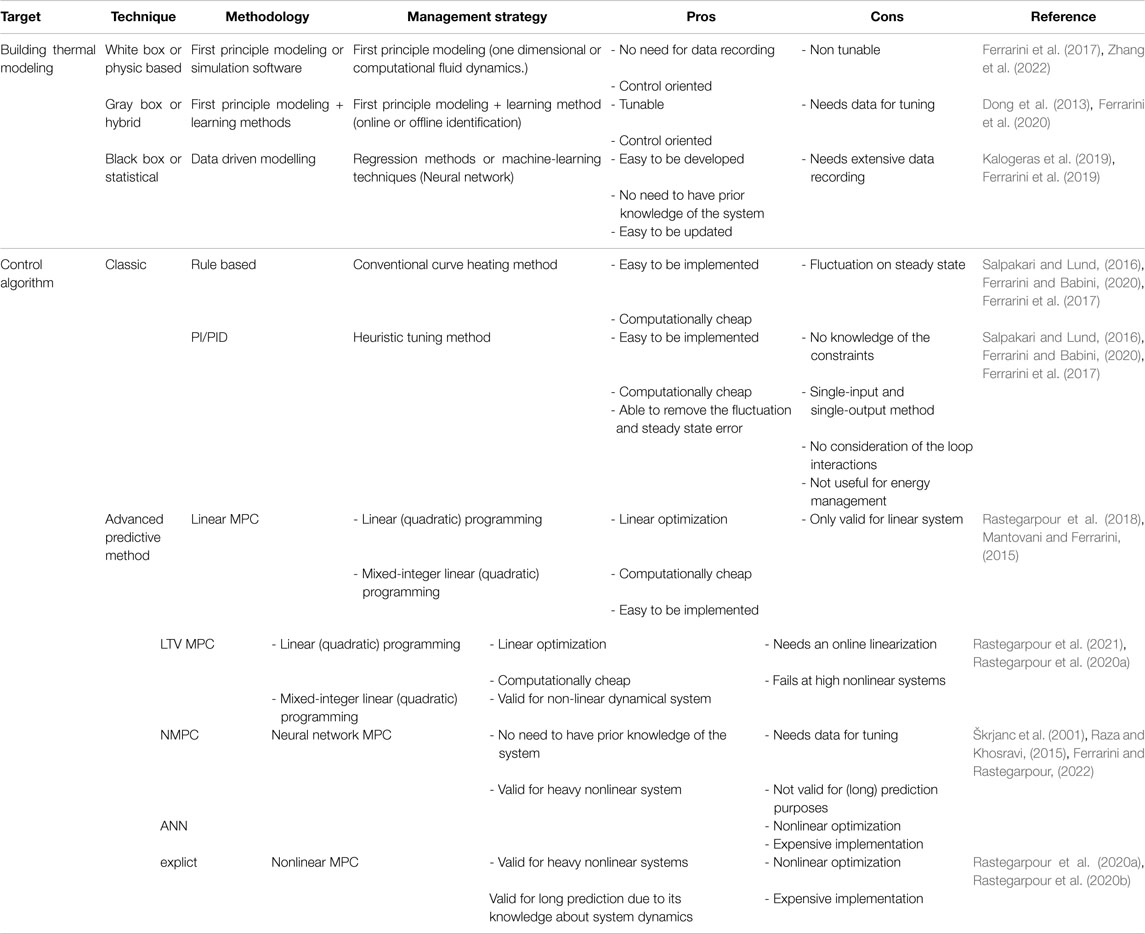
TABLE 1. Summary of the proposed modelling and control approaches with their advantages, and limitations.
However, selection of a suitable control methodology significantly depends on the time horizon during which the energy performance is going to be evaluated.
From a monthly or yearly perspective, there is no need to take care of the transitory behavior of the thermo-electrical equipment (such as heat pumps or fan coils), while they can be all studied in steady-state conditions with a set of algebraic equations. In this case, the optimization problem focuses more on the seasonal issues like monthly climatic conditions and occupancy profile when a long-term planning is usually foreseen [see (Ferrarini et al., 2019) (Rastegarpour et al., 2017)].
On the contrary, the dynamical behavior of the system’s components are more important once the controller aims at improving the buildings’ performance in a short time interval of hours or minutes level. In this case, depending on the number of variables and constraints, the resulting optimization problem could be a very complex one. So, one practical solution is to use a multi-level architecture for the control, a so-called hierarchical predictive control algorithm. This can be a combination of classic and advanced control approaches which are reviewed in Section 3.
In this view, building energy management interfaces with different boundary levels and control loops. It all depends on the level of complexity to put into the mathematical formulation. However, from a very high-level perspective, three different control hierarchies can be introduced:
• Power generation/distribution system: this includes a top level view of the control hierarchy, where a building (as individual load) or a smart grid (as a set of loads) are integrated with electrical and thermal generation systems (PV panels, wind turbines, diesel generators, industrial heat pumps etc) for energy market optimization purposes. The control system in this level designs in a larger sampling time, usually in hour(s) range.
• Load level: This includes the control of an individual building (residential or commercial) including all thermal and electrical devices such as heat pumps, water tanks, batteries, local renewable and building’s thermal zones. The control system in this level designs in a medium sampling time, usually in minute(s) level.
• Thermo/electrical equipment: This includes the bottom level of the control hierarchy, where the inner control loops of the thermo/electrical devices are taken into account, as for example:
– control of the refrigerant cycle including fans, valves and compressor
– control of the water flow rate within the heat pump condenser
– control of the heat exchangers
– control of battery energy flow
– control of air handling units and fan coils
The control system at this level is operated with a small sampling time, usually in second(s) range.
Figure 1 shows levels of integration in a control hierarchy based on the sampling time. In fact, it shows how to study the system’s dynamic from a control perspective on different levels.
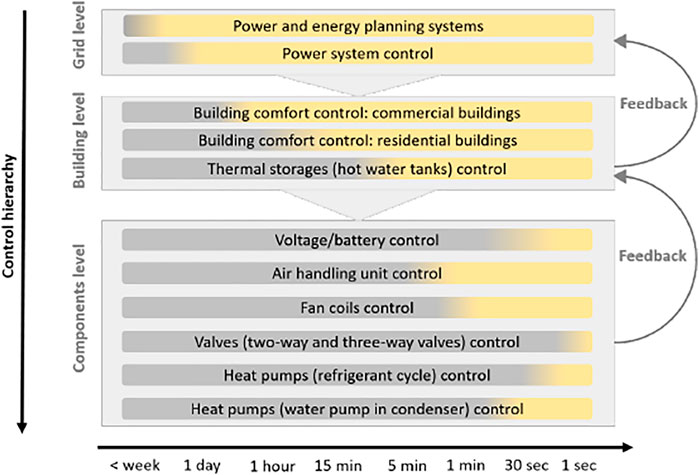
FIGURE 1. Control hierarchy and levels of integration: the yellow(light) zone shows the part where the system behaves dynamically.
5 Challenges in Real Applications
In this section, some relevant energy control applications for building are analyzed and compared. They are listed based on increasing size of the application:
• heat pump with thermal storage modeling and control
• building energy control integrated with electrical storage
• complex HVAC modeling and control
• multiple buildings in a smart grid
5.1 Heat Pump With Thermal Storage Modeling and Control
A common vapor compression heat pump cycle consists of two parallel heat exchangers, one acting as an evaporator (where heat is absorbed) and another as a condenser (where heat is released). There are several different types of heat pumps depending on the heat and sink source environments, as follows:
• heat sources: ambient air, ground, water
• heat sinks: space heating, domestic hot water
The amount of heat delivered by a heat pump strongly depends on the environmental conditions where the heat pump is operating in. In fact, this load-dependent efficiency of the heat pump is the main reason which makes it an interesting component in energy efficiency programs and opens the way for optimization problems.
In this perspective, it is possible to operate efficiently any heat pump in their efficient period by estimating and possibly predicting their coefficient of performance (COP) based on the available measurements. Heat pump COP is a practical index representing the rate at which the heat pump generates heat with respect to a specific electrical power consumption.
In this perspective, it is important to model the heat pump components in order to accurately estimate or predict its COP value. There are mainly three different approaches for modeling a heat pump, here briefly recalled.
• Exploiting the available simulation software and thermodynamic libraries such as thermolib or TRNSYS: In this approach, models are mainly developed based on thermodynamic principles already developed in some libraries. These types of simulators are useful for the overall evaluation of a heat pump cycle or for solving sizing problems. They are not absolutely useful for control system design or dynamic and online optimization solutions. However, they are quite interesting to be used as a reference model for testing different high-level control scenarios, similar to the application presented in (Rastegarpour et al., 2019a).
• Physics-based models, based on first principle thermodynamic rules. This type of modeling technique exploits the energy conservation laws and balance equations in order to evaluate the transient behavior of the system. This is a suitable approach for designing control systems for the heat pump cycle and its local controller as it gives a correct insight about thermal behavior of the refrigerant and consequently an acceptable calculation of COP value. However, in a building efficiency level, the dynamic of the heat pump is almost negligible as it operates in seconds level (see Figure 1). Moreover, from a practical point of view, the transient behavior of a heat pump is not an interesting issue, while the steady state conditions are more remarkable.
• Data-driven models. This is one of the best and most practical solutions for the application of heat pumps in building energy management programs. In fact, in this approach, the most effective parameters on COP value is used to train a network or a polynomial to be used as a predictor in higher level control algorithms. This type of models can be both dynamic or static, depending on the sampling time used for the network training. For example, a simple method is used in (Boait et al., 2011) to take the Carnot ratio and modify it with a multiplier in order to catch the heat pump COP value. A similar approach for heat pump modeling has been employed by other authors, for example (Magraner et al., 2010). In the rest of this section, we review more precisely this modeling approach.
5.1.1 Control-Oriented Models of a Domestic Air-To-Water Heat Pump
The application scenario of this section composes of a domestic air-to-water heat pump connected to a hot water tank (Figure 2). A set of control-oriented models of the heat pump is studied in (Rastegarpour et al., 2019a). Those models are basically a quadratic and linear combinations of subsets of the main factors that affect the heat pump COP, which are identified through a sensitivity analysis on the following parameters:
• external air temperature Toa
• external humidity H
• backward water temperature to condenser stage TB
• compressor power COMB
It is also proved that the dependency on only one of the many factors, like air temperature, is not enough to formulate a reliable model-based controller.
The numerical analysis of the maximum variation of the COP (ΔCOP) with respect to the variation of each parameter is summarized as follow:
• COPmin to COPmax → 1 to 7
• ΔCOP w.r.t Toa → 3.1
• ΔCOP w.r.t H → 0.21
• ΔCOP w.r.t TB → 4.83
• ΔCOP w.r.t COMB → 1.3
It becomes visible that the temperature lift in evaporator and condenser (Toa and TB) have a major impact on efficiency, which has been proven by the proposed sensitivity analysis.
The proposed models are usable if there are enough sensors to provide the designer with the required information. While, there are cases where the lack of sensors will be a critical issue for training such models. As a vivid case, the profile of the water mass flow rate along the condenser manifold is highly uncertain in absence of specific sensors. Therefore, the buffer tank temperature at the level corresponding to the heat pump condenser can not be accurately estimated at the end of each sampling period k, which results in an erroneous COP estimation.
Therefore, in case of the lack of sensors, it is possible to tune an equivalent control-oriented model for the system, i.e. heat pump and buffer tank, under variable flow rate conditions on both the condenser and load side, while still preserving good prediction capabilities of the model, with no tank temperature nor mass flow rate sensors.
The proposed equivalent model is able to enhance the performance of the designed predictive controller such that it improves the heat pump operation (COP value) by approximately 53% and saves up to 21% electrical power consumption with respect to the classic heating-curve(HC) technique. Figure 3 shows about 2 days of experimental test for the proposed algorithm. The experiment is performed on a typical winter day in Denmark, with a daily mean temperature of 0 °C for a time-of-use electricity price profile. The nonlinear optimal control problem is compared with the current internal control unit in the real modulating heat pump, which computes the supply water temperature set point based on a conventional weather-dependent heating curve. This experiment highlights the advantages of the NMPC approach against the classic control algorithm.
More details and results discussion are provided in (Ferrarini et al., 2020).
5.1.2 Dynamic COP Predictor
The trained static models presented in the previous subsection proved to provide satisfactory results in many applications. However, the approach there presented needs an intensive data collection procedure. Moreover, there are some issues, such as aging or dramatic changes in environmental conditions or any changes in the size of the buffer tank, which may enforce the retuning of the proposed polynomials.
In order to avoid such a retuning procedure, it is possible to propose a practical and generic solution for the heat pump COP prediction, thanks to the available on-line estimation theoretical framework.
In this approach, the proposed linear and nonlinear approximations are merged with an on-line estimation technique (Extended Kalman Filter is suggested) in order to update the proposed model according to the current situation of the plant. The updated model of the COP is then used in a recursive algorithm to run the Nth-step ahead prediction of the COP value. In this case, it is possible to capture the future behavior of the heat pump COP with an acceptable accuracy for a prediction horizon sufficiently enough for the control purposes in such application.
This technique is used in an experimental setup for prediction of the COP value of a domestic air-to-water heat pump in Denmark. Figure 4 shows the predictive capability of the proposed COP model for a prediction horizon of 30 min, where each point corresponds to the 60-step prediction error of the COP value.
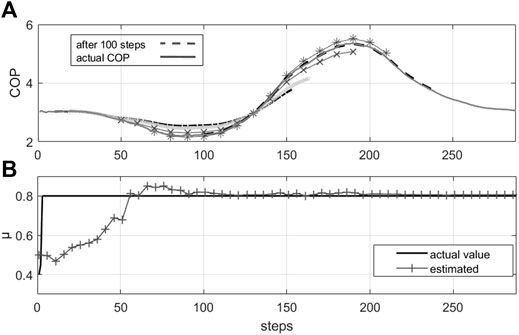
FIGURE 4. Predictive capability analysis of the proposed COP predictors for 300 steps analysis (2.5 h).
The proposed predictor is used also in a predictive optimization problem which proved promising results. For more info and details please refer to (Rastegarpour et al., 2021).
5.2 Building Energy Control Integrated With Electrical Storage
There are many strategies to improve the energy consumption trend in building sectors. Recent uses of building energy resource management system as well as complex optimization techniques uncovered new ways to achieve thermal and electrical energy saving.
According to (Zame et al., 2018), energy storage systems provides a remarkable flexibility to improve the operation of smart buildings in terms of energy consumption and user satisfaction.
In this scope, a distributed and hierarchical control algorithm is developed in (Rastegarpour et al., 2019b) for smart buildings application with the micro-grids (MGs) interaction. In fact, the proposed control architecture aims at exploiting the advantages of using thermal and electrical energy storages to shift the power generated by renewable resources to be more coincident with peak times for optimal pricing concepts. On the other hand, it guarantees the comfort level satisfaction for the building’s occupants in presence of different disturbances.
The experimental validation of the proposed control algorithm has been performed in a well-equipped smart grid research laboratory belonging to the ERIGrid research infrastructure, equipped with energy storages, controllable loads, PV panel, wind turbine and a point of common connection connected to the national grid. The selected technologies for such experiment is summarized as follows:
• Battery: Inverter SMA (Niestetal, Germany) Sunny Island 4500 with nominal power of 3.3 kW250 Ah, 60 V lead-acid batteries.
• Controllable loads: 15 kW resistors, 1 kW lamps, 0.5 HP motor, and 2.5 kVAR inductive load.
• Photovoltaics panels: inverter SMA Sunny Boy 100 E with rating of 1.1kW nominal power.
• Wind turbine: Inverter SMA Windy Boy 1700 with nominal power of 1.7 kW.
• Thermal energy storage: a 400 L tank
• Building dimension: an office with a volume of 72 m3)
In this application, the building pipelines are supplied through a three-way valve, which introduces more complexity due to variable position of valve. Despite the fact that thermal energy storage dynamical model is nonlinear due to variable water flow rate, the optimization problem is more complicated due to the logical/algebraic model of battery with variable charging/discharging efficiency, which makes the overall model a mixed-logic dynamical system.
Figure 5 shows the overall control architecture and experimental configuration of the proposed energy resources management strategy.
The experimental and simulation results show the effectiveness of the proposed solution. In fact, the proposed algorithm saves up to 12.5% in load energy consumption and it has 22% improvement of total cost benefit. As it has been shown in Figure 6, the optimal control algorithm exploits the capacity of the storages in the peak periods to serve the load and sell the excess power to the grid resulting in a more cost benefit.
5.3 Complex HVAC Modeling and Control
The problem of performance improvement and energy consumption reduction of the heating, ventilation and air conditioning system (HVAC) of a large-scale university building through the application of nonlinear predictive control strategies is studied in (Rastegarpour and Ferrarini, 2021).
This paper also significantly extends the application case in (Ferrarini and Babini, 2020), where no models of the generation and distribution units were given and only feed-forward/feedback PID control loops were evaluated. Also, indoor disturbances due to a variable occupancy level are addressed.
The experimental setup of the proposed study includes a detailed reference model composed of the integration of a multi-zone thermal model of the educational building with two air handling units (each one endowed with air extractors with heat recovery units and temperature control loops), two heat pumps (a water-to-water and an air-to-water type), a water tank and aero-hydraulic circuits, see Figure 7.
Furthermore, a three-level hierarchical NMPC (H-NMPC) algorithm is tested on this application (see Figure 8). A centralized version of NMPC is also tested for comparison purposes. The objective of the control system is twofold. First, to optimally decide how to distribute the thermal load on the two heat pumps by manipulating the water and air circulation actuators in order to guarantee the thermal comfort and air quality in the building. Second, to minimize the overall electrical energy consumption in presence of all nonlinear dynamics, equality and inequality constraints, physical limitations and disturbances.
The experimental results show that The proposed algorithm is able to improve significantly the heat pumps operation up to 21 and 34% in air-to-water and water-to-water heat pumps, receptively, compared to the traditional control system, without sacrificing the comfort conditions.
The problem of performance improvement and energy consumption reduction of the HVAC system in large scale buildings are also studied in (Mantovani and Ferrarini, 2015) for a shopping center (Campo dei Fiori), which is located in northern Italy. As a matter of fact, when HVAC plants are introduced, a heavy nonlinear behavior due to the presence of heat exchangers as plant terminals (such as radiators or fan coils) is introduced and cannot be properly modeled with a constant heat exchange coefficient, i.e., a thermal resistance.
However, the idea was to use a nonlinear block, which filters the controller’s output (thermal power) for obtaining real-world manipulated variables (mass flows or temperatures). This nonlinear compensator proves to enhance closed-loop performances with respect to classic linearization, which is not suitable for the heavy nonlinearities of heat exchangers. This way, it is possible to separate the linear part of the model from the nonlinear one, tuning the MPC on the linear—and more reliable—process model (see Figure 9).
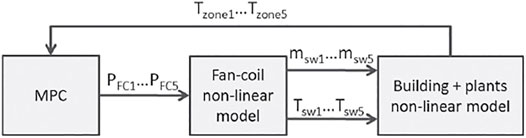
FIGURE 9. Control architecture. MPC computes a power value to be delivered to each zone. This value is filtered by a compensator to become a valid value for the HVAC system.
The experimental analysis shows that MPC allows for energy saving of about 4.5% with respect to the current practice, thanks to the predicting capabilities and the accuracy in estimating disturbance inputs.
5.4 Multiple Buildings in a Smart Micro-grid
From a pure user’s perspective, a PID may be good enough for the temperature control in residential buildings with radiators [(Ferrarini et al., 2017) (Ferrarini and Babini, 2020)]. However, more advanced control algorithms, such as MPC, may improve performance if we have radiant-panel building, although some specific alternative solutions could be exploited.
On the contrary, once the controller aims at optimizing the performance of thermo-electrical devices and improving the overall energy efficiency of the smart micro-grid in a demand-response scenario, the need of MPC algorithms is undeniable.
The results discussed in the previous subsections indicate that the use of a simplified heat pump representation provides a better suboptimal solution and consequently improves the building energy performance. However, the problem is more challenging when a group of users (building in different sizes) are taken into account.
The scenario addressed in this section extends the classic building heated by a heat pump, and consists of a set of different buildings with different desired comfort levels and different levels of flexibility. A combination of heat pumps with different types of storages (electrical and thermal) and different types of generators (renewable, diesel generators or main utility grid) is an attractive solution to shape the load in a demand-response scenario and to shift the heat pumps operation to their efficient period.
The case study and corresponding control algorithms are well described in (Rastegarpour et al., 2020b). The paper aims at finding the best compromise among different goals:
• make the heat pump work when it is more efficient
• store electrical energy when it is cheap
• store thermal energy in the tank when the heat pump is more effective
• modulate the inlet water temperature of the building pipelines to satisfy the user’s comfort
• exploit the building thermal inertia
The results of the experimental analyses were extremely encouraging. The optimal control formulation proposed there was a nonlinear MPC algorithm which proved to be consistent in many different scenarios. simulation results showed that it is possible to cut electricity cost by about 20% in a variable price scenario, thanks to the optimized flow of energy among storages and users.
6 Conclusions and Recommended Future Directions
In this paper, the application of different control algorithms was analyzed and discussed, in many different control perspectives. In fact, two main control categories have been investigated: classic (adaptive and rule-based control) and advanced (linear and nonlinear MPC). Several different applications of those control algorithms in smart grid and building levels were reviewed.
The investigated studies show how to use different types of predictive control algorithms to optimize the flow of energy among different types of storages and users, while still preserving the demand, in particular in smart building applications. In this regard, the advantages and disadvantages of linear and nonlinear MPC algorithms were discussed.
The studied application scenarios proved that, although a simple classic control (such as PID controllers) can be used for a temperature control in buildings, more advanced algorithms are required to increase the overall efficiency of the buildings.
Although numerical conclusions are difficult to generalize, depending on the specific application, adopted devices, environmental conditions, and operational conditions generic results can be conducted.
Generally, we may say that ranging from linear, to linearized, to linear time-varying, to nonlinear MPC for applications with heat pumps the efficiency increase over linear solutions is respectively in the range of 4–12% and for nonlinear control up to 20–40% in the load level.
In some cases, it is possible to decouple the linear and nonlinear part of the system, tuning a linear MPC on the liner parts. Although the performance is not as good as a complex nonlinear MPC, still it allows up to 4.5% energy saving with respect to the current practice.
The results show up to 22% cost benefit once the load (including HVAC system) is integrated with different types of storages in a microgrid application. In these scenarios a mixed-logic optimization problem is solved as it includes some binary values due to the if-then rules.
For the goal of improving the performance of the HVAC system, NMPC showed an interesting result where the heat-pump performance was improved up to 21 and 34% for air-to-water and water-to-water, respectively.
The case studies discussed here are also extremely informative for the variety of different applications. First, the proposed methods are all computationally feasible where the simulation steps (of usually 15-min) is executed in less than 2 s on a standard office computer. Then, the optimal control algorithms showed a consistent performance among several different scenarios.
There were also many practical issues related to the implementation of the proposed control strategies. As a vivid case, the prediction errors due to the model mismatches caused by the variable load profile and by the heat pump local controller for water pump and compressor speed regulation were investigated.
However, the possibility of using data driven control algorithms and combination of the learning algorithms with MPC concept are interesting future directions.
Data Availability Statement
The raw data supporting the conclusion of this article will be made available by the authors, without undue reservation.
Author Contributions
LF and SR contributed to conception and design of the work. SR organized the simulations. LF and SR performed the numerical analysis and evaluation. All authors contributed to manuscript revision, read, and approved the submitted version.
Conflict of Interest
The authors declare that the research was conducted in the absence of any commercial or financial relationships that could be construed as a potential conflict of interest.
Publisher’s Note
All claims expressed in this article are solely those of the authors and do not necessarily represent those of their affiliated organizations, or those of the publisher, the editors and the reviewers. Any product that may be evaluated in this article, or claim that may be made by its manufacturer, is not guaranteed or endorsed by the publisher.
References
Biyik, E., and Kahraman, A. (2019). A Predictive Control Strategy for Optimal Management of Peak Load, Thermal Comfort, Energy Storage and Renewables in Multi-Zone Buildings. J. Build. Eng. 25, 100826. doi:10.1016/j.jobe.2019.100826
Boait, P. J., Fan, D., and Stafford, A. (2011). Performance and Control of Domestic Ground-Source Heat Pumps in Retrofit Installations. Energy Build. 43, 1968–1976. doi:10.1016/j.enbuild.2011.04.003
Dong, B., Gorbounov, M., Yuan, S., Wu, T., Srivastav, A., Bailey, T., et al. (2013). Integrated Energy Performance Modeling for a Retail Store Building. Build. Simul. 6, 283295–295. doi:10.1007/s12273-013-0109-8
European Union (2010). Directive 2010/31/EU of the European Parliament and of the Council of 19 May 2010 on the Energy Performance of Buildings. Official J. Eur. Union, L.153/13–L.153/35.
Ferrarini, L., and Rastegarpour, S. (2022). “Neural Network Predictive Schemes for Building Temperature Control: a Comparative Study,” in Accepted for presentation at IEEE 18th International Conference on Automation Science and Engineering (CASE) Mexico.
Ferrarini, L., and Babini, R. (2020). “Digital Techniques for Energy Optimization in a University Building: a Case Study,” in 25th IEEE International Conference on Emerging Technologies and Factory Automation (ETFA) Vienna, Austria. doi:10.1109/ETFA46521.2020.9212118
Ferrarini, L., Fathi, E., Disegna, S., and Rastegarpour, S. (2019). “Energy Consumption Models for Residential Buildings: a Case Study,” in 24th IEEE International Conference on Emerging Technologies and Factory Automation (ETFA). 2019 Zaragoza, Spain. doi:10.1109/ETFA.2019.8869151
Ferrarini, L., Rastegarpour, S., and Caseri, L. (2020). Predictive Control-Oriented Models of a Domestic Air-To-Water Heat Pump Under Variable Conditions. IEEE Robot. Autom. Lett. 5 (4), 53635369–5369. doi:10.1109/LRA.2020.3007474
Ferrarini, L., Rastegarpour, S., and Petretti, A. (2017). “An Adaptive Underfloor Heating Control with External Temperature Compensation,” in Proceedings of the 14th International Conference on Informatics in Control, Automation and Robotics (ICINCO), 636. doi:10.5220/0006432906290636
Hazyuk, I., Ghiaus, C., and Penhouet, D. (2012). Optimal Temperature Control of Intermittently Heated Buildings Using Model Predictive Control: Part II - Control Algorithm. Build. Environ. 51, 388–394. doi:10.1016/j.buildenv.2011.11.008
Kajgaard, M. U., Mogensen, J., Wittendorff, A., Veress, A. T., and Biegel, B. (2013). “Model Predictive Control of Domestic Heat Pump,” in In2013 American control conference Washington, DC, USA. doi:10.1109/ACC.2013.6580131
Kalogeras, G., Rastegarpour, S., Koulamas, C., Kalogeras, A. P., Casillas, J., and Ferrarini, L. (2019). Predictive Capability Testing and Sensitivity Analysis of a Model for Building Energy Efficiency. Build. Simul. 13, 3350–50. doi:10.1007/s12273-019-0559-8
Koulamas, C., Kalogeras, A. P., Pacheco-Torres, R., Casillas, J., and Ferrarini, L. (2018). Suitability Analysis of Modeling and Assessment Approaches in Energy Efficiency in Buildings. Energy Build. 158, 1662–1682. doi:10.1016/j.enbuild.2017.12.002
Lin, Y., and Yang, W. (2018). Application of Multi-Objective Genetic Algorithm Based Simulation for Cost-Effective Building Energy Efficiency Design and Thermal Comfort Improvement. Front. Energy Res. 6, 1–19. doi:10.3389/fenrg.2018.00025
Magraner, T., Montero, Á., Quilis, S., and Urchueguía, J. F. (2010). Comparison between Design and Actual Energy Performance of a HVAC-Ground Coupled Heat Pump System in Cooling and Heating Operation. Energy Build. 42, 13941401–1401. doi:10.1016/j.enbuild.2010.03.008
Manjarres, D., Mera, A., Perea, E., Lejarazu, A., and Gil-Lopez, S. (2017). An Energy-Efficient Predictive Control for HVAC Systems Applied to Tertiary Buildings Based on Regression Techniques. Energy Build. 152, 409–417. doi:10.1016/j.enbuild.2017.07.056
Mantovani, G., and Ferrarini, L. (2015). Temperature Control of a Commercial Building with Model Predictive Control Techniques. IEEE Trans. Ind. Electron. 62 (4), 26512660–2660. doi:10.1109/TIE.2014.2387095
Rastegarpour, S., Caseri, L., Ferrarini, L., and Gehrke, O. (2019a). “Experimental Validation of the Control-Oriented Model of Heat Pumps for MPC Applications,” in IEEE 15th International Conference on Automation Science and Engineering (CASE), 1255. doi:10.1109/COASE.2019.8843211
Rastegarpour, S., Ferrarini, L., and Palaiogiannis, F. (2019b). A Distributed Predictive Control of Energy Resources in Radiant Floor Buildings. J. Dyn. Syst. Meas. Control 141 (10), 101013–101015. doi:10.1115/1.4043935
Rastegarpour, S., Gros, S., and Ferrarini, L. (2020a). MPC Approaches for Modulating Air-To-Water Heat Pumps in Radiant-Floor Buildings. Control Eng. Pract. 95, 104209. doi:10.1016/j.conengprac.2019.104209
Rastegarpour, S., Ferrarini, L., and Gros, S. (2020). Economic NMPC for Multiple Buildings Connected to a Heat Pump and Thermal and Electrical Storages. IFAC-PapersOnLine 53 (2), 17089–1709417094. doi:10.1016/j.ifacol.2020.12.1620
Rastegarpour, S., and Ferrarini, L. (2021). “Hierarchical Nonlinear MPC for Large Buildings HVAC Optimization,” in IEEE 17th International Conference on Automation Science and Engineering (CASE) Lyon, France. doi:10.1109/CASE49439.2021.9551423
Rastegarpour, S., Ferrarmi, L., Pacheco-Torres, R., Kalogeras, A., and Koulamas, C. (2017). “Sensitivity Analysis of Medical Centers Energy Consumption with EnergyPlus,” in 22nd IEEE International Conference on Emerging Technologies and Factory Automation (ETFA). 2017 (Limassol).
Rastegarpour, S., Ghaemi, M., and Ferrarini, L. (2018). “A Predictive Control Strategy for Energy Management in Buildings with Radiant Floors and Thermal Storage,” in SICE International Symposium on Control Systems (SICE ISCS), 73. doi:10.23919/SICEISCS.2018.8330158
Rastegarpour, S., Scattolini, R., and Ferrarini, L. (2021). Performance Improvement of an Air-To-Water Heat Pump through Linear Time-Varying MPC with Adaptive COP Predictor. J. Process Control 99, 6978–78. doi:10.1016/j.jprocont.2021.01.006
Raza, M. Q., and Khosravi, A. (2015). A Review on Artificial Intelligence Based Load Demand Forecasting Techniques for Smart Grid and Buildings. Renew. Sustain. Energy Rev. 50, 13521372–1372. doi:10.1016/j.rser.2015.04.065
Salpakari, J., and Lund, P. (2016). Optimal and Rule-Based Control Strategies for Energy Flexibility in Buildings with PV. Appl. Energy 161, 425436–436. doi:10.1016/j.apenergy.2015.10.036
Škrjanc, I., Zupančič, B., Furlan, B., and Krainer, A. (2001). Theoretical and Experimental Fuzzy Modelling of Building Thermal Dynamic Response. Build. Environ. 36 (9), 1023–1038. doi:10.1016/S0360-1323(00)00053-6
Staino, A., Nagpal, H., and Basu, B. (2016). Cooperative Optimization of Building Energy Systems in an Economic Model Predictive Control Framework. Energy Build. 128, 713–722. doi:10.1016/j.enbuild.2016.07.009
Verhelst, C., Degrauwe, D., Logist, F., Van Impe, J., and Helsen, L. (2012). Multi-objective Optimal Control of an Air-To-Water Heat Pump for Residential Heating. Build. Simul. 5, 281291–291. doi:10.1007/s12273-012-0061-z
Yang, S., Wan, M. P., Ng, B. F., Zhang, T., Babu, S., Zhang, Z., et al. (2018). A State-Space Thermal Model Incorporating Humidity and Thermal Comfort for Model Predictive Control in Buildings. Energy Build. 170, 2539–39. doi:10.1016/j.enbuild.2018.03.082
Zame, K. K., Brehm, C. A., Nitica, A. T., Richard, C. L., and Schweitzer III, G. D., (2018). Smart Grid and Energy Storage: Policy Recommendations. Renew. Sustain. Energy Rev. 82, 16461654–1654. doi:10.1016/j.rser.2017.07.011
Keywords: smart buildings, energy management, heat pump, linear MPC, real-time NMPC
Citation: Rastegarpour S and Ferrarini L (2022) Energy Management in Buildings: Lessons Learnt for Modeling and Advanced Control Design. Front. Energy Res. 10:899866. doi: 10.3389/fenrg.2022.899866
Received: 19 March 2022; Accepted: 03 June 2022;
Published: 02 September 2022.
Edited by:
Giorgos Georgiou, Cyprus University of Technology, CyprusReviewed by:
Elias B. Kosmatopoulos, Democritus University of Thrace, GreeceHossam A. Gabbar, Ontario Tech University, Canada
Copyright © 2022 Rastegarpour and Ferrarini. This is an open-access article distributed under the terms of the Creative Commons Attribution License (CC BY). The use, distribution or reproduction in other forums is permitted, provided the original author(s) and the copyright owner(s) are credited and that the original publication in this journal is cited, in accordance with accepted academic practice. No use, distribution or reproduction is permitted which does not comply with these terms.
*Correspondence: Luca FerrariniTHVjYS5GZXJyYXJpbmlAcG9saW1pLml0