- 1Department of Electrical and Electronics Engineering, B. V. Raju Institute of Technology, Telangana, India
- 2School of Electronics and Electrical Engineering, Lovely Professional University, Phagwara, Punjab, India
- 3Symbiosis Institute of Technology, Symbiosis International (Deemed University), Pune, India
- 4Department of Electrical Power and Machines, Faculty of Engineering, Alexandria University, Alexandria, Egypt
- 5Wolfson Centre for Magnetics, School of Engineering, Cardiff University, Cardiff, United Kingdom
- 6Engineering and Information Technology Research Center (EITRC), Bani Walid, Libya
Electric vehicles (EVs) have become a feasible alternative to conventional vehicles due to their technical and environmental benefits. The rapid penetration of EVs might cause a significant impact on the distribution system (DS) due to the adverse effects of charging the EVs and grid integration technologies. In order to compensate for an additional EV load to the existing load demand on the DS, the distributed generators (DGs) are integrated into the grid system. Due to the stochastic nature of the DGs and EV load, the integration of DGs alone with the DS can minimize the power losses and increase the voltage level but not to the extent that might not improve the system stability. Here, the EV that acts as a load in the grid-to-vehicle (G2V) mode during charging can act as an energy source with its bidirectional mode of operation as vehicle-to-grid (V2G) while in the discharging mode. V2G is a novel resource for energy storage and provision of high and low regulations. The article proposes a smart charging model of EVs, estimates the off-load and peak load times over a period of time, and allocates charging and discharging based on the constraints of the state of charge (SoC), power, and intermittent load demand. A standardized IEEE 33-node DS integrated with an EV charging station (EVCS) and DGs is used to reduce the losses and improve the voltage profile of the proposed system. Simulation results are carried out for various possible cases to assess the effective utilization of V2G for stable operation of the DS. The cost–benefit analysis (CBA) is also determined for the G2V and V2G modes of operation for a 24-h horizon.
1 Introduction
The transportation industry in India is presently the fifth largest in the world, with plans to make it the third largest by 2030. The traditional means of fuel-intensive transit will not be able to meet the needs of such a large domestic market. In India, the government is pursuing new paths to deliver efficient, clean, and cost-effective transportation services, reduce consumption of oil imports, and reduce human and environmental health impacts. With the transition to electric vehicles (EVs), India stands to benefit on several fronts, including the abundance of renewable energy sources (RES) and the availability of skilled workers in the technical and industrial sectors. Per the FAME II policy, 70% of commercial vehicles, 30% of private vehicles, 40% of buses, and 80% of two- and three-wheelers could be electrified by 2030 (Rocky Mountain Institute and Niti Aayog, 2019). As a result, India is actively encouraging the use of EVs throughout the country by providing further incentives to consumers and production companies at both the central and provincial levels. It is critical for a nation that still relies largely on coal for producing electricity to adopt stringent measures to meet its goals for the future. Regardless of the EV charging method utilized, the rapid proliferation of EVs and fast chargers will eventually result in increased electricity consumption and system congestion. However, the widespread development of EVs might cause grid instability (particularly during peak demand periods) and a slew of issues and limits (Tarroja et al., 2015). Many challenges originating from the fast proliferation of EVs and charging stations can be resolved using distributed generators (DGs), in which electricity production is often generated in the same structure, close to the load. The probabilistic and unpredictable features of EVs, such as charging station arrival/departure timings, driving range, storage capacities, and modes of charging/discharging, are significant elements that influence power system stability. Furthermore, the intermittent nature of RES is another factor that affects the system. Many technical and economic factors must be considered while developing dispersed power sources. In some cases, where demand is inherently intermittent, DGs alone will not be able to meet the need. Here, the vehicles are only utilized for around 5% of the day on average, and they are parked in lots for the other 90% (Mehrjerdi and Rakhshani, 2019). This massive quantity of unused automobiles. As a result, the idea of V2G was developed to address existing power grid challenges and concerns by concurrently governing demand and supply. V2G is a novel resource for power storage and provision of high and low regulations. Additionally, it offers a means of alleviating grid congestion and mitigating the need to upgrade grid infrastructure, which is a solution to both issues. The incorporation of the V2G method with the generating units is an effective way to address potential issues connected to high EV adoption and intermittent power supply. The aforementioned method increases energy supply and quality, decreases emissions from automobile engines, and promotes a cleaner environment through the use of V2G, which is a new model for the circular economy. The city infrastructure and way of life will be rebuilt using EVs and V2G, resulting in a massive rise in global development. The most significant goal of the V2G system development is to increase grid stability by reducing peak demand (Amamra and Marco, 2019). Interpreting the V2G impact on the system load profile is a good technique for assessing the V2G efficiency. The organization of this paper is as follows: Section 2 addresses the literature review and research gaps, Section 3 introduces aformulation of the problem, Section 4 presents the V2G smart charging framework in the radial distribution system (DS), cost analysis of G2V and V2G and the results are discussed in Section 5, and Section 6 concludes the work.
2 Literature review
One of the most significant sources of energy performance characteristics for electric cars is the amount of power used per mile traveled (mile/kWh). This efficiency indicator might have a significant influence on the electricity grid capacity to handle the increased load imposed by EV demand, together with the increased penetration of EVs. Sami et al. (2019) demonstrated a network of charging stations used to connect a fleet of EVs to a DS for a bidirectional V2G operation. Through active engagement in services for frequency and voltage control, the system can schedule EV charging and discharging a day in advance to lower the cost of EV ownership charging. The initial state of charge (SoC) of the battery, the EV charging time, the targeted time of EV departure, the cost of battery deterioration, and the needs for charging the EV are all considered in an optimization method for V2G scheduling. A conventional IEEE 33-node DS with five EV charging stations (EVCSs) is used to show the efficacy of the proposed system. An effort is made to optimally plan the G2V and V2G operating modes of EVs (Velamuri et al., 2022) to minimize losses of power in the DS when DG is present. Dominant parameters such as the SoC, journey circumstances, the size of the EV battery, and levels of charging/discharging are considered while modeling EVs. The proposed technique determines the voltage stability index, the enhanced grasshopper optimization algorithm (EGOA), and the smart charging technique to determine the size of DGs to be integrated into the system. In order to decrease power loss and enhance the voltage profile, the suggested hybrid strategy aims for EVs and DGs should be scheduled together. Sovacool et al. (2017) demonstrated how EVs, energy storage systems, and smart buildings (SB) may work together to shift grid load, minimize peak demand, and reduce annual energy use. The primary issues that are presented and assessed are battery backup, quick charging and draining, V2G interface, and stability. In addition, the simulation models for V2G and G2V to illustrate key characteristics impacting the grid-interface network are presented. The case study assessed several controller settings that had an impact on the control, stability, and dynamics of the grid-connected V2G and G2V systems. Vehicle grid integration (VGI) systems that regard plug-in electric vehicles (PEVs) as a controllable load with a unidirectional power flow consider smart or “controlled” charging or time-of-use (ToU) pricing as examples (Chtioui and Boukettaya, 2020). Typically, VGI includes the V2G perspective. The PEV is considered a storage device because of its bidirectional flow of electricity to and from the grid. For the most part, our research shows that VGI studies to this point have often been concerned with technical issues such as load balancing and power cost reduction using V2G systems. Chai et al.’s (2019) main objective was to investigate a suggested simulation environment that simulates a microgrid with a small number of V2G connections. The discharge mode is seldom employed in practice when there is a big surge in demand. A model and simulation of the components that make up this microgrid are provided, along with information on how they function. This study examines the charging and discharging situations in more detail and evaluates the management techniques utilized to control the power in this simulation.
The charging–discharging schedule for EV owners is optimized using the linear programming model presented by Raveendran et al. (2017) while considering restrictions on the amount of travel required, the cost of battery maintenance, and the cost of battery deterioration. The suggested approach is adaptable to accommodate different driving styles by enabling owners to choose their preferred parking location and on-road duration based on their travel schedule. The suggested approach is also capable of accurately estimating the driving requirements of EV users and allocating sufficient battery charge before each journey. Kasturi et al. (2019) contributed to the use of EVCSs deployed at various sites to provide V2G support and vehicle charging to assist the grid during various needs. Vehicle charging, harmonic removal, and reactive power compensation in the case of an EVCS deployed in the Kochi Metro Rail System have been modeled, with findings described through the waveform. In order to maximize the benefits of EVs in a predetermined timetable, Kumar M. et al. (2019) suggested a charging–discharging method that considers the most efficient ways to drive. The charging and discharging of EVs are permitted at any location served by the same bus in a radial distribution network, including the workplace and residence. For mitigation of the negative effects of EV charging and discharging on the grid and the costs associated with the operation for the owners of EVs and utilities, a multi-objective multiverse optimization (MOMVO) algorithm is employed to determine the bus where the EVs should be connected and the optimal number of EVs to use. In the V2G mode, EVs are able to contribute reactive electricity to the grid and primarily discharge at peak load periods to increase efficiency and reliability. (Jaiswal and Ballal, 2018), offer V/f regulation, control the intermittent nature of renewable energy, and load balancing services using demand side management (DSM) as a set of potential ancillary services. The number of lost life cycles and the deterioration of batteries are two issues linked with the V2G system. Other challenges include the need for modifications to be made in the architecture and equipment of the grid, the loss of energy, and the enormous investment cost. This study describes the benefits and drawbacks of the V2G technology for EV owners and grid operators. The implementation of Plug-in hybrid electric vehicles (PHEVs) into the current grid infrastructure entails significant changes in terms of load scheduling, grid stability, peak-to-average demand ratio (PAR), and energy cost. In recent research, multi-objective optimization approaches have been employed to address these needs. With the use of a smart scheduling vector, the optimization approach (Javed et al., 2020) seeks to reduce demand while increasing PHEV charging and discharging availability and lowering energy costs. For the utility goal of minimizing PAR, this study suggests a distinct schedule vector for PHEV owners’ charging and discharging to decrease total energy costs. The V2G design and the important concerns (Mousavi-Khademi et al., 2020) related to V2G are comprehensively examined in this study by the focused control and management of the battery system, as well as battery deterioration and bi-directional chargers. Furthermore, V2G problems, benefits, and technologies are covered in addition to the financial costs and income of the EV owners and the power grids. For conventional test networks, the effects of V2G on the power system are examined in this study.
Vita and Koumides (2019) provided a unique strategy based on value-based pricing for the deployment of DGs and V2G parking lots simultaneously. Consideration of the network’s technical challenges, such as increasing the voltage profile and minimizing losses, is addressed by establishing the optimal capacity of EV parking facilities and distributed production resources by taking into account value-based pricing to attract network investment. Thus, the study’s key contribution is the inclusion of value-based pricing of DGs and V2Gs during their optimum placement and the suggested optimal search algorithm (PSA). The results might be outstanding if EVs are integrated into a smart grid with supporting technologies such as V2G architecture and RES. It is possible to supply DG and energy storage and stabilize the variable load demand using these solutions. EVs and RES have been studied by Shariff et al. (2019), analyzing the existing status of the V2G system research. The methods used for power flow under the V2G system were described by Jawale et al. (2022). Commercial V2G adoption is also explained in detail. Vehicle-to-vehicle (V2V) and vehicle-to-house (V2H) state-of-the-art comparisons are also provided. With the help of a network of charging stations, Bibak and Tekiner-Mogulkoc (2021) developed an efficient bidirectional V2G system that connects an EV fleet to a distributed power grid in both directions. Through frequency and voltage control services, the system may lower EV ownership charging costs by scheduling EV charging and discharging for the next day. According to the suggested method, the day-ahead energy projection is updated based on real-time EV consumption data, allowing for further optimization of the use of EVs to support both voltage and frequency management. Initial battery charge, EV charging, battery deterioration, charging costs, and regulation pricing are factors that should be considered in a V2G scheduling strategy. The effects of EVs and V2G on grid reliability, costs, and emissions are examined using a precise and effective technique (Golla et al., 2022a). The strength of this research lies in the adaptability of the proposed technique to power grids with various patterns and characteristics with regard to the generation of power through RES by considering the stochastic factors forming demand and supply trends on a daily basis. We provide two innovative indexes, continuous and random power supply, for evaluating the availability of RESs in power supply systems and assessing the grid stability. To account for every scenario, a variety of cases based on various EV and V2G penetration rates, charging methods, locations, and schedules are defined. In every instance, the effects of the scenarios under consideration on the grid dependability, emissions, and cost are assessed using a Monte Carlo simulation. Babu and Swarnasri (2020) focused on optimizing EVCS and DG location and size simultaneously using multi-objective optimization. Optimization of actual power losses, AVDI, and VSI of the DS is formulated as the objective problem. The conventional test systems for the IEEE 33-bus and IEEE 69-bus were used for simulation. Here, TLBO and Harries Hawk optimization (HHO) algorithms were used to test the efficacy. Bagheri Tookanlou et al. (2020) developed a scheduling strategy that provides all V2G and G2V agent incentives. Using the shortest driving route and the EVCS cost–benefit analysis, EVs plan for the charging and discharging mode. EVCSs identify the optimal ES to buy wholesale power. Three optimization issues consider all agent V2G and G2V rewards. A cloud scheduling system acquires input from all agents, solves optimization problems, and sends the findings to relevant agents to apply the recommended strategy. Three agents achieve optimal hourly electricity pricing. Simulations of nine EVCSs and three ESs are used to display and evaluate the modeled findings. Bagheri Tookanlou et al. (2020) suggested a new multi-level V2G scheduling technique, which allows the V2G approach to operate and regulate EV users in an efficient manner. An innovative economic dispatch optimization model for EVs was also proposed in this study to keep the operational costs of the regional V2G system to a minimum. Using an IEEE 33-bus system with 100 EVs, an analysis demonstrates that this idea is viable and can be eliminated by properly managing the overall EV battery capacity and ramping it up and down. Khasanov et al. (2021) analyzed the yearly operation and advantages of EVs and V2G technology in a microgrid setting and exhibited the various modes of operation. Studying various EV charging scenarios and their impact on V2G operations is one of the most significant contributions of this study. The primary goal of the scheduling strategy of this study is to ensure that all agents taking part in V2G and G2V operations will get their incentives. According to the suggested technique, EVs individually schedule their charging and discharging based on the best driving route and the cost–benefit ratio provided by EVCSs (Chippada and Reddy, 2022). The best energy supplier (ES) to buy power from the wholesale market is also found by each EVCS. The formulation of three optimization problems accounts for the advantages of all agents in EV V2G and G2V operation. In order to evaluate the seasonal features of V2G operations, the study examines interactions between V2G, intermittent renewable production, and utility-scale batteries throughout periodic simulations. The findings of O’Neill et al. (2022) implied that the most recent EV technological developments increased the economic sustainability of V2G and its potential to increase grid efficiency by offering more storage space and peak demand control.
In terms of efficiently balancing grid power generation and demand, providing grid-support services, reducing GHG emissions, producing economic benefits, and meeting critical energy needs during outages, PEVs with bidirectional V2G capability offer utility grid operators significant techno-economic-environmental benefits (Viswanath et al., 2022). In order to reduce grid peak demand and environmental damage, PEV with V2G optimization has thus become crucial for utility grid operation. The intelligent technique for parking lot location and pricing scheduling is a two-layer methodology proposed in this research. Parking lots have been strategically placed in the first layer while minimizing active power loss and adhering to voltage profile restrictions. EV charging schedule has been carried out in the second layer while ensuring minimal charging prices (Pal et al., 2022). The G2V and V2G modes (Dharavat et al., 2022) were considered while performing these procedures. In order to put EV owners in a profitable position, soft computing techniques have been used to determine the best spots for parking lots and calculate the entire cost of charging. The extensive features of the EV sector and its infrastructure for charging are covered by Dharavat et al. (2023). Moreover, it offers a step-by-step process for putting the V2G deployment into practice, explains how artificial intelligence may be used to use data recorders to power an EV battery, and analyzes the costs and benefits of using the V2G technique effectively. The role of aggregators and customers is also discussed, as well as the numerous facets of the EV industry. The relationship between the transport and electricity systems is analyzed using V2G at corresponding peak times. By incurring extra delay expenses and changing their departure time, commuters who choose to discharge get benefits. Drivers’ decisions about discharge and departure time are characterized using a bottleneck model (Ding et al., 2022). We generate potential queuing patterns and occurrence conditions. When commuters who choose to discharge arrive at the bottleneck sooner than those who choose not to discharge, traffic congestion is shown to be minimized compared to the scenario without V2G. Table 1 represents the techno-economic analysis of DS with the integration of DGs and EVs.
After reviewing the existing literature, it is evident that a scheduling strategy that incorporates the integration of both DGs and EVCS is necessary. The studies mentioned previously focused on the importance of the placement of DGs. Due to the growing demand for electrical energy, DGs have grown in importance within DS. The power DS losses and voltages will be impacted by the positioning and capacity of DGs. In certain cases, when demand is naturally intermittent, DGs alone will not be able to provide it. Power engineers have supplemented the system with a backup unit, which is a battery energy storage system (BESS), to alleviate the intermittency. Because of the volatility of EV load demand, SoC has to accommodate a wide variety of charging patterns. The identification of an off-load and peak load during a period of time is also challenging. The precise planning of EVs to charge and discharge with a smart charging system based on the intermittent load demand is important. Here, V2G plays a vital role, with proper planning and effective utilization of V2G methodology as one of the DGs to the grid-connected system is a major concern for the stable and reliable operation of the grid. In most studies, the authors focus on the system performance, such as reducing the losses and enhancing the voltage level due to the penetration of the EVs. Some of them addressed the end user point on measures for reducing the charging cost, maximizing the profits with priority-based charging without compromising on the standards of quality in charging. The research on considering the factors of system performance and the end user benefits is in the development phase, which is considered in the proposed objective.
The contribution of the article is as follows:
1) Optimal placement of EVCS in the strong bus and DGs in the weak bus using the loss sensitivity factor approach.
2) Smart charging framework based on the off-load and peak load for a 24-h duration, with the simultaneous placement of EVCS and DGs along with V2G in the DS using the operational constraints of SoC, load, and power.
3) Cost–benefit analysis with the charging and discharging patterns of the end user.
3 Problem formulation
The major objective of this research is to provide a smart EV charging scheduling that considers the effective use of the V2G model for the DG-integrated grid-connected system with EVCS. The operational constraints of EV are considered for increasing the voltage profile and minimizing network losses of power in the DS. Customer priorities are taken for the technical and economic validation of the V2G’s ability to deliver grid services.
First, to minimize the total real power losses, V2G acting as a source with its bidirectional model is shown as follows:
where PL is the real loss of power, I is the current, R is the resistance, and N is the number of buses.
The power balance equation for the EV acting as a load is shown as follows:
The power balance equation for the EV acting as a source (DG) is shown as follows:
where
3.1 Limits on charging and discharging for EVs
where
Each electric vehicle battery’s state of charge (SoC) must be kept within certain ranges as follows:
The boundary limits of DG are as follows:
Here, the limits are in kW.
4 Methodology for scheduling of EVCS and DGs
4.1 Optimal positioning of EVCS and DGs
The planning framework for charging stations includes identifying the optimal position for the EVCS. Strong buses are identified for the location of EVCS using the loss sensitivity factor (LSF) approach. Considering the LSF and the nominal voltage severity factor in the analysis, the weak buses are identified for the placement of DGs.
4.2 Loss sensitivity factor
Loss sensitivity factors are determined to choose the potential node DG placement. The search space may be decreased by estimating these sensitive nodes. The LSF values for each line are arranged in decreasing order so that the buses with higher values have a better probability of being chosen as a potential site for the placement of DGs.
The magnitudes of the normalized voltages are determined for each of the buses from the following formula:
Buses with normalized values below 1.01 are regarded as potential nodes in need of compensation. The positioning of the buses to be considered for compensation is determined by loss sensitivity factors, and the necessity for compensation for each bus is determined by the normalized voltage values.
To assess the impact of EVCS demand along with the existing base load in the distribution system and to compensate for the demand to some extent, the proposed simultaneous integration of EVCS and DGs to the distribution system is analyzed along with G2V and V2G methodology based on the off-load and peak load for stable operation of the distribution system as shown in Figure 1.
4.3 V2G framework in the distribution system
Due to the intermittent nature of DGs integrated into the grid-connected system, in the upcoming generation, the analysis of V2G technology plays a vital role in being one of the sources to act as an additional DG to the existing grid-connected system. With the bi-directional converter, the EVCS can operate in dual mode as a load and source at times based on the shown off-load and peak load. By the effective utilization of EVs as one of the sources based on the intermittent load demand with smart charging, the losses will be further reduced and enhance the voltage profile. The DGs and EV batteries are perceived as negative loads and are thus mitigated for the objective of minimizing losses. The optimal DG size and location are decided by the position with the minimum losses over the respective sizes.
The following are the possible cases for the EV battery to operate under intermittent load demand based on the operational constraints of SoC level, demand, and power flow shown in Figure 2.
Case 1: When the demand is greater than the generation and the battery’s SoC is lower than the battery’s minimum SoC or in contrast to demand, the generation is larger and the SoC of the battery is greater than the SoC maximum. Under this circumstance, it is not good to adhere to charging or discharging represented in Equations 9, 10. Therefore, the EV will be in the neutral mode, denoted with 1:
Case 2: If the demand is lower than the generation and the SoC of the battery is below the SoC maximum, then the charging operation takes place as follows, denoted with 2:
Case 3: If the demand is greater than the generation and the SoC of the battery is greater than the SoC minimum, then the discharging operation takes place as follows, denoted with 3:
The SoC at each hour based on the mode to charge and discharge is represented by Equations 13, 14. The battery power is given by Equation (15). The battery is charged at its rated capacity, which is the initial difference between generation and demand shown in Equation (16). The efficiency during charging and discharging is assumed to be 90 percent:
Here, SoC(t) is SoC in the current hour,
SoC (t-1) is SoC in the past hour,
ηc, ηd represent efficiency under the charging and discharging modes,
E is the energy of the battery in kWh,
and ∆t is the respective time interval,
The range of SoC is between 0.2 and 0.9 pu.
Benefit factor = (power/SoC) *demand
Total electricity price at each hour in cents/kWh is given by
Ep = (mean price of ES to EVCS) + (benefit factor* mean price of ES to EVCS)
Net profit earned in cents/kWh = Ep − mean price of ES to EVCS
5 Results and discussion
In the proposed method, an IEEE 33 bus system was considered to assess the performance of the V2G smart charging system. The configuration of the 33 radial bus system is determined by the “total lines in number: 32, Slack bus: 1, base case voltage: 12.66 kV, MVA: 100 MVA, the total true power: 3.715 MW, and reactive power: 2.295 Mvar.” The base load of the conventional IEEE 33 bus system is 3.715 MW, and the true power loss without connecting the load was 0.203 MW. The planning framework for charging stations includes identifying the optimal position for the EVCS. Using the loss sensitivity factor (LSF) approach, the strong buses are identified for the location of EVCS. Here, the four locations of EVs are discovered for the IEEE 33 bus system’s optimal location of EVCS (Golla et al., 2022b). For an IEEE 33 bus system, the total load was raised to 4.735 MW by connecting the EV load to each of the four buses (10, 14, 17, and 30) shown in Table 2, and the corresponding increases in losses reached 0.374 MW, which was raised to 45.7 percent.
Here, seven DG locations are determined to be the weak buses for the optimal positioning of DGs using the LSF approach. With the integration of seven DGs (5, 8, 11, 15, 18, 28, and 32), the losses decreased to 0.141 MW in the system. With the installation of EVs and DGs on the IEEE 33 bus simultaneously, the entire increase in load was 4.735 MW, whereas the losses were reduced to 0.163 MW owing to the integration of DG, which dropped to 56.4%.
Figure 3 shows the variation of real power losses for the potential EV and DG scenarios in possible cases.
Figure 4 depicts the voltage profile in the IEEE 33 bus system for the base case, with EV load, with EV and DG placed simultaneously. This was accomplished by placing 4 EVs and 7 DGs simultaneously. Figure 5 shows the layout of the 33 bus system integrated with EVCS and DGs with G2V and V2G modes.
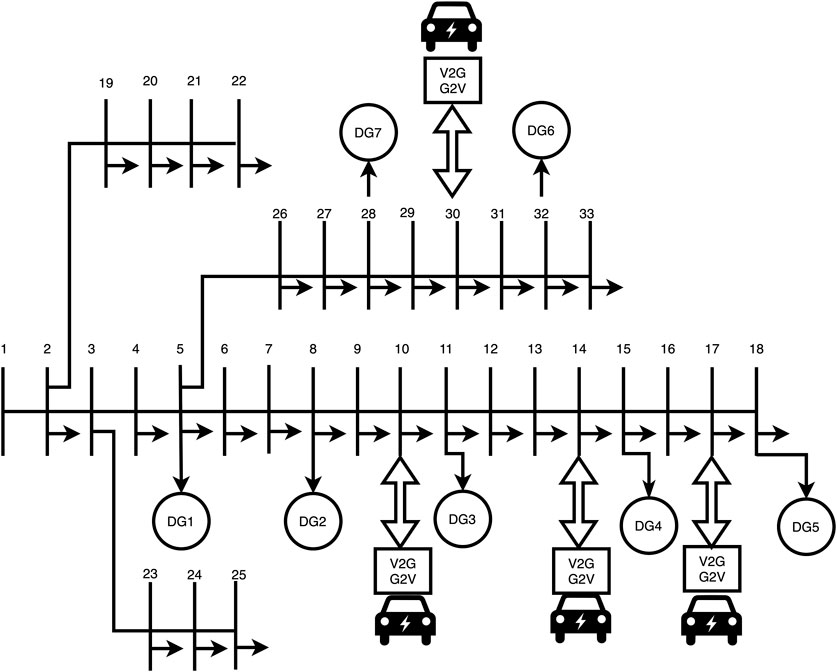
FIGURE 5. Simultaneous integration of EVCS and DGs in the radial 33 bus system operating in G2V and V2G modes.
The real power constraints of the DG placement for the respective buses along with EVs as a source are in the range given in Table 3.
5.1 Smart charging technique with EVCS and DG along with V2G
The load demand is estimated per unit for a horizon of a day of 24 h to analyze the smart charging framework from the EV and DG integration in the DS at the same time. The SoC, demand, and power are determined at each EVCS to examine the off-load and peak load demand for the efficient operation of the smart charging technique. Based on the load demand and the SoC level of the EV battery, the charging, discharging, and neutral operation takes place in EVCS, shown in Table 4.
Figure 6A shows the variation of SoC, demand, and power curve with respect to time for the EVCS operating at the 17th bus. Initially, the demand is constant, and the generation is high. Therefore, the SoC of the battery operates in a neutral mode; at the 6th hour, demand increases, and the SoC of the battery starts discharging. From the 9th hour, the demand is high, and SoC is minimum, operating in a neutral position. At the 11th hour, for a considerable change in demand, the SoC of the battery starts charging. At the 16th hour, the battery’s SoC rises to its highest point, and the demand is low, so it operates in a neutral position. Finally, at the 21st hour, demand starts increasing, and the SoC of the battery starts discharging. The same process continues for all the remaining EVCS. Figures 6B–D show the variation in demand, SoC, and power for a 24-h horizon at remaining buses of 10, 14, and 30.
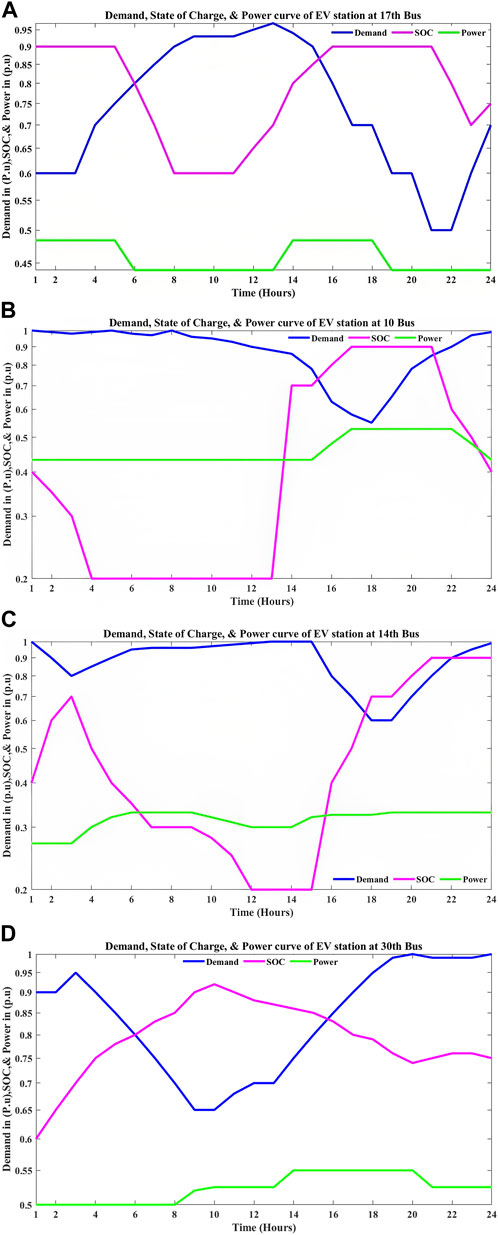
FIGURE 6. (A) SoC, demand, and power curve at the 17th bus. (B) SoC, demand, and power curve at the 10th bus. (C) SoC, demand, and power curve at the 14th bus. (D) SoC, demand, and power curve at the 30th bus.
5.2 Cost analysis of G2V and V2G modes
The extreme peak load on the electric system mandates ineffective peak power facilities, raises grid operational costs, and brings system stability at risk. Peak shaving or minimizing the amplitude of daily peaks may save grid operators time and money while lowering consumer prices. The economic benefit of the end user of an EV is considered based on the demand, SoC, and power over a period of time. For each hour, it is expected that there exist EVs in a certain region with known origin and destination, as well as initial SoC. The optimal hourly electricity price supplied by the ES to the EVCS for a 24-h horizon is given in Figure 7.
The mean of the electricity price supplied by the ES to each EVCS is varied due to the intermittent load demand; at the 30th bus, it is 20.07 cents/kWh.
The simulation is carried out for cost–benefit analysis on each EVCS based on smart charging. Figure 8 shows the variation of cost analysis for EVCS at the 30th bus on each hour, with the EVs connected to the respective charging station by the G2V and V2G methodology. The percentage increase in profit by G2V methodology at first hour is 42.8% and by V2G at 19th hour is about 41.7%. Table 5 gives the net profit earned by EVCS in the G2V and V2G modes from smart charging methodology.
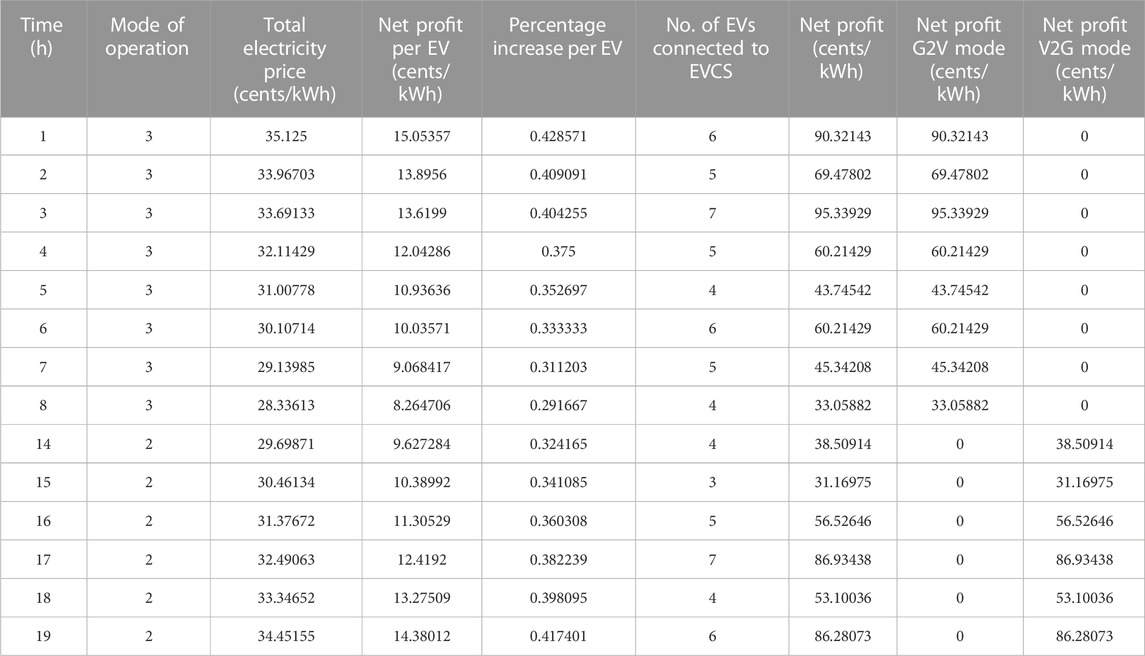
TABLE 5. Net profit earned by EVCS at the 30th bus in 24-h horizon by G2V and V2G modes by smart charging.
The net profit earned by the respective EVs at the 30th bus undergoing V2G methodology during the 14th, 15th, 16th, 17th, 18th, and 19th hours is shown in Figure 9. The highest profit is seen to be earned at the 17th hour, which is 86.93 cents/kWh. Table 6 shows the maximum percentage increase in the profit earned by the EVCS and end user by smart charging at a particular hour of the day for the modes of G2V and V2G.
5.3 Impact of losses and voltage in the distribution system by V2G mode
The simultaneous deployment of four EVs and seven DGs in the IEEE 33 bus system, also considering each among four EVCSs and the combination of two, three, and four EVCSs acting as a DG by the V2G method using smart charging based on the load demand and power availability. The simulation is carried out, and it is observed that the losses were reduced further compared with the optimal placement of EVs and DGs represented in Table 7 and the variations of power loss shown in Figures 10, 11 for the possible test cases.
Case 1: The base loss for the standard IEEE 33 bus test system is 0.203 MW; with the four EVCSs (10th, 14th, 17th, and 30th buses) connected to the 33 bus system, the losses increased to 0.374 MW.
Case 2: With the simultaneous integration of four EVCSs and seven DGs (5th, 8th, 11th, 15th, 18th, 28th, and 32nd buses) integrated into the 33 bus system, the losses were reduced to 0.163 MW.
Case 3: With the simultaneous placement of four EVCSs, among the EVCS connected to the 10th bus acting as DG (V2G mode) in addition to the seven DGs integrated into the 33 bus system, the losses were reduced to 0.0638 MW.
Case 4: With the simultaneous placement of four EVCSs, among the EVCS connected to the 14th bus acting as DG (V2G mode) in addition to the seven DGs integrated into the 33 bus system, the losses were reduced to 0.0801 MW.
Case 5: With the simultaneous placement of four EVCSs, among the EVCS connected to the 17th bus acting as DG (V2G mode) in addition to the seven DGs integrated into the 33 bus system, the losses were reduced to 0.0631 MW.
Case 6: With the simultaneous placement of four EVCSs, among the EVCS connected to the 30th bus acting as DG (V2G mode) in addition to the seven DGs integrated into the 33 bus system, the losses were reduced to 0.0793 MW.
Case 7: With the simultaneous placement of four EVCSs, among the EVCS connected to the 10th and 14th buses acting as DGs (V2G mode) in addition to the seven DGs integrated into the 33 bus system, the losses were reduced to 0.0401 MW.
Case 8: With the simultaneous placement of four EVCSs, among the EVCS connected to the 10th, 14th, and 17th buses acting as DGs (V2G mode) in addition to the seven DGs integrated into the 33 bus system, the losses were reduced to 0.0576 MW.
Case 9: With the simultaneous placement of four EVCSs, among the EVCS connected to the 10th, 14th, 17th, and 30th buses acting as DGs (V2G mode) in addition to the seven DGs integrated into the 33 bus system, the losses were reduced to 0.062 MW.
Simulation is carried out for the four EVs and seven DGs in the IEEE 33 bus simultaneously, also considering each among the four EVCSs and the combination of 2, 3, and 4 EVCSs acting as a DG by the V2G method using smart charging, and the improvement in the voltage profile is shown in Figure 12.
The simulation results revealed that with the efficient exploitation of the IEEE 33 bus system, with EVs and DGs along the V2G method with smart charging, the overall losses with (7 + EV1 + EV2) DGs were reduced to 0.0401 MW (i.e., reduced to 75.4%) and improved the voltage profile, compared to the losses with EV and DG of 0.163 MW. Due to the intermittent nature of EVCS for load demand, the simulation results show that the smart utilization of charging, discharging, and neutral operation over a period of time based on the SoC of the battery and demand will make the distribution stable and enhance the reliable operation of the grid.
6 Conclusion and future scope
The rapid penetration of EVs in the market leads to an increase in the distribution network capacity of EVCS for charging purposes, resulting in network performance degradation due to high power losses, system voltage violations, and network asset congestion. As a result, it is critical to effectively regulate the supply due to the increase in the charging demand by the network. Various charging frameworks in the literature provide controlled charging to reduce the EV charging demand. However, with only the EVCS connected to the 33 bus system, the losses increased to 0.374 MW. With the simultaneous placement of EVCS and DGs for an integrated grid-connected system, the losses were reduced to 0.163 MW, and the simulation results based on the operational constraints of SoC, load demand, and power for the proposed V2G smart charging framework reduced the losses to 0.0401 MW in the network and enhanced the voltage level in various possible cases tested for the IEEE 33 bus system. In addition, the cost–benefit analysis was based on the 24-h horizon carried out for the G2V and V2G methodology. Furthermore, the research can be carried out by considering the driving behavior of the end-user and the degradation of the EV battery.
Data availability statement
The original contributions presented in the study are included in the article/supplementary material. Further inquiries can be directed to the corresponding author.
Author contributions
All authors listed have made a substantial, direct, and intellectual contribution to the work and approved it for publication.
Conflict of interest
The authors declare that the research was conducted in the absence of any commercial or financial relationships that could be construed as a potential conflict of interest.
Publisher’s note
All claims expressed in this article are solely those of the authors and do not necessarily represent those of their affiliated organizations or those of the publisher, the editors, and the reviewers. Any product that may be evaluated in this article, or claim that may be made by its manufacturer, is not guaranteed or endorsed by the publisher.
References
Ahmadi, S. E., Kazemi-Razi, S. M., Marzband, M., Ikpehai, A., and Abusorrah, A. (2023). Multi-objective stochastic techno-economic-environmental optimization of distribution networks with G2V and V2G systems. Electr. Power Syst. Res. 218, 109195. doi:10.1016/j.epsr.2023.109195
Al-Ammar, E. A., Farzana, K., Waqar, A., Aamir, M., Saifullah, , Ul Haq, A., et al. (2021). ABC algorithm based optimal sizing and placement of DGs in distribution networks considering multiple objectives. Ain Shams Eng. J. 12 (1), 697–708. doi:10.1016/j.asej.2020.05.002
Amamra, S. A., and Marco, J. (2019). Vehicle-to-Grid aggregator to support power grid and reduce electric vehicle charging cost. IEEE Access 7, 178528–178538. doi:10.1109/ACCESS.2019.2958664
Babu, P. V. K., and Swarnasri, K. (2020). Multi-objective optimal allocation of electric vehicle charging stations in radial distribution system using teaching learning based optimization. Int. J. Renew. Energy Res. 10 (1), 366–377. doi:10.20508/ijrer.v10i1.10453.g7882
Bagheri Tookanlou, M., Marzband, M., Al Sumaiti, A., and Mazza, A. (2020). “Cost-benefit analysis for multiple agents considering an electric vehicle charging/discharging strategy and grid integration,” in 2020 IEEE 20th Mediterranean Electrotechnical Conference ( MELECON), 19–24. doi:10.1109/MELECON48756.2020.9140637
Bagherzadeh, L., Shahinzadeh, H., and Gharehpetian, G. B. (2019). “Scheduling of distributed energy resources in active distribution networks considering combination of techno-economic and environmental objectives,” in 2019 International Power System Conference (PSC), 687–695. doi:10.1109/PSC49016.2019.9081477
Bibak, B., and Tekiner-Mogulkoc, H. (2021). Influences of vehicle to grid (V2G) on power grid: An analysis by considering associated stochastic parameters explicitly. Sustain. Energy, Grids Netw. 26, 100429. doi:10.1016/j.segan.2020.100429
Chai, Y. T., Tan, W. N., Gan, M. T., and Yip, S. C., (2019). “An optimal charging and discharging schedule to maximize revenue for electrical vehicle,” 2019 IEEE Conference on Sustainable Utilization and Development in Engineering and Technologies (CSUDET). 240–245. doi:10.1109/CSUDET47057.2019.9214678
Chippada, D., and Reddy, M. D. (2022). Optimal planning of electric vehicle charging station along with multiple distributed generator units. Int. J. Intell. Syst. Appl. 14 (2), 40–53. doi:10.5815/ijisa.2022.02.04
Chtioui, H., and Boukettaya, G. (2020). “Vehicle-to-grid management strategy for smart grid power regulation,” in 2020 6th IEEE International Energy Conference (ENERGYCon), 988–993. doi:10.1109/ENERGYCon48941.2020.9236530
Dharavat, N., Sudabattula, S. K., and Velamuri, S. (2021). “Integration of distributed generation and electric vehicles in a distribution network using political optimizer,” in 2021 4th International Conference on Recent Developments in Control, Automation and Power Engineering (RDCAPE), 521–525. doi:10.1109/RDCAPE52977.2021.9633448
Dharavat, N., Sudabattula, S. K., Velamuri, S., Mishra, S., Sharma, N. K., Bajaj, M., et al. (2022). Optimal allocation of renewable distributed Generators and electric vehicles in a distribution system using the political optimization algorithm. Energies 15, 6698–6718. doi:10.3390/en15186698
Dharavat, N., Golla, N. K., Sudabattula, S. K., Velamuri, S., Kantipudi, M. V. V. P., Kotb, H., et al. (2023). Impact of plug-in electric vehicles on grid integration with distributed energy resources: A review. Front. Energy Res. 10 (1), 1–13. doi:10.3389/fenrg.2022.1099890
Ding, Y., Li, X., and Jian, S. (2022). Modeling the impact of vehicle-to-grid discharge technology on transport and power systems. Transp. Res. Part D. Transp. Environ. 105, 103220. doi:10.1016/j.trd.2022.103220
Golla, N. K., Sudabattula, S. K., and Suresh, V. (2022a). Optimal placement of electric vehicle charging station in distribution system using meta-heuristic techniques. Math. Model. Eng. Probl. 9 (1), 60–66. doi:10.18280/mmep.090108
Golla, N. K., Sudabattula, S. K., and Suresh, V. (2022b). Optimal placement of charging station and distributed generator along with scheduling in distribution system using arithmetic optimization algorithm. Int. J. Renew. Energy Res. 12 (2), 970–980. doi:10.20508/ijrer.v12i2.12964.g8480
Jaiswal, S., and Ballal, M. S. (2018). “Optimal load management of plug-in electric vehicles with demand side management in vehicle to grid application,” 2017 IEEE Transp. Electrif. Conf. ITEC-India 2017. 1–5. doi:10.1109/ITEC-India.2017.8356942
Javed, M., Deb, S., Alam, M. S., Rafat, Y., and Hameed, S. (2020). “Impact of vehicle to grid on power system,” in 2020 5th IEEE International Conference on Recent Advances and Innovations in Engineering (ICRAIE), 1–5. doi:10.1109/ICRAIE51050.2020.9358388
Jawale, S. A., Singh, S. K., Singh, P., and Kolhe, M. L. (2022). Priority wise electric vehicle charging for grid load minimization. Processes 10, 1898–1899. doi:10.3390/pr10091898
Kasturi, K., Nayak, C. K., and Nayak, M. R. (2019). Electric vehicles management enabling G2V and V2G in smart distribution system for maximizing profits using MOMVO. Int. Trans. Electr. Energy Syst. 29 (6), 1–17. doi:10.1002/2050-7038.12013
Khasanov, M., Kamel, S., Rahmann, C., Hasanien, H. M., and Al-Durra, A. (2021). Optimal distributed generation and battery energy storage units integration in distribution systems considering power generation uncertainty. IET Gener. Transm. Distrib. 15 (24), 3400–3422. doi:10.1049/gtd2.12230
Kumar, M., Vyas, S., and Datta, A. (2019a). “A review on integration of electric vehicles into a smart power grid and vehicle-to-grid impacts,” in 2019 8th International Conference on Power Systems (ICPS), 2019. doi:10.1109/ICPS48983.2019.9067330
Kumar, S., Mandal, K. K., and Chakraborty, N. (2019b). Optimal DG placement by multi-objective opposition based chaotic differential evolution for techno-economic analysis. Appl. Soft Comput. 78, 70–83. doi:10.1016/j.asoc.2019.02.013
Mazumder, M., and Debbarma, S. (2021). EV charging stations with a provision of V2G and voltage support in a distribution network. IEEE Syst. J. 15 (1), 662–671. doi:10.1109/JSYST.2020.3002769
Mehrjerdi, H., and Rakhshani, E. (2019). Vehicle-to-grid technology for cost reduction and uncertainty management integrated with solar power. J. Clean. Prod. 229, 463–469. doi:10.1016/j.jclepro.2019.05.023
Memon, Z. A., Hassan, M. Y., Said, D. M., Leghari, Z. H., and Shaikh, P. H. (2022). “A systematic approach to accommodate plug-in electric vehicles in distribution networks with optimal integration of distributed generation,” in Proceedings of the 6th International Conference on Electrical, Control and Computer Engineering, 339–352. doi:10.1007/978-981-16-8690-0_31
Mousavi-Khademi, M. R., Chamorro, H. R., Zareian-Jahromi, M., Sood, V. K., Guerrero, J. M., and Martinez, W (2020). “Optimal value-based prices placement of der and V2G using planet search algorithm,” in 2020 IEEE Electric Power and Energy Conference (EPEC), 1–6. doi:10.1109/EPEC48502.2020.9320044
O’Neill, D., Yildiz, B., and Bilbao, J. I. (2022). An assessment of electric vehicles and vehicle to grid operations for residential microgrids. Energy Rep. 8, 4104–4116. doi:10.1016/j.egyr.2022.02.302
Pal, A., Das, S., Chakraborty, A. K., Acharjee, P., and Bhattacharya, A. (2022). “Optimal allocation of parking lot with intelligent charging scheduling of electric vehicles in distribution system,” in 2022 1st International Conference on Sustainable Technology for Power and Energy Systems (STPES), 1–6. doi:10.1109/STPES54845.2022.10006566
Raveendran, V., Krishnan, N. N., and Nair, M. G. (2017). “Vehicle-to-grid support by electric vehicle charging stations operated at airports and metro rail stations,” in 2017 International Conference on Technological Advancements in Power and Energy ( TAP Energy), 1–6. doi:10.1109/TAPENERGY.2017.8397241
Rocky Mountain Institute and Niti Aayog (2019). India’s Electric Mobility Transformation: Progree to date and future opportunities. p. 56, Available at: http://niti.gov.in/writereaddata/files/document_publication/NITI-RMI-Report.pdf.
Sami, I., Ullah, Z., Salman, K., Hussain, I., Ali, S. M., Khan, B., et al. (2019). “A bidirectional interactive electric vehicles operation modes: Vehicle-to-grid (V2G) and grid-to-vehicle (G2V) variations within smart grid,” in 2019 International Conference on Engineering and Emerging Technologies (ICEET), 1–6. doi:10.1109/CEET1.2019.8711822
Shariff, S. M., Iqbal, D., Saad Alam, M., and Ahmad, F. (2019). A state of the art review of electric vehicle to grid (V2G) technology. IOP Conf. Ser. Mater. Sci. Eng. 561, 012103. doi:10.1088/1757-899X/561/1/012103
Singh, J., and Tiwari, R. (2020). Cost benefit analysis for V2G implementation of electric vehicles in distribution system. IEEE Trans. Ind. Appl. 56 (5), 5963–5973, Sep. doi:10.1109/TIA.2020.2986185
Sovacool, B., Axsen, J., and Kempton, W. (2017). Tempering the promise of electric mobility? A sociotechnical review and research agenda for vehicle-grid-integration (VGI) and vehicle-to-grid (V2G). Annu. Rev. Environ. Resour. 42 (8), 16.1–16.30. doi:10.1146/annurev-environ-030117-020220
Subbaramaiah, K., and Sujatha, P. (2023). Optimal DG unit placement in distribution networks by multi-objective whale optimization algorithm and its techno-economic analysis. Electr. Power Syst. Res. 214, 108869. doi:10.1016/j.epsr.2022.108869
Tarroja, B., Shaffer, B., and Samuelsen, S. (2015). The importance of grid integration for achievable greenhouse gas emissions reductions from alternative vehicle technologies. Energy 87. 504–519. doi:10.1016/j.energy.2015.05.012
Velamuri, S., Cherukuri, S. H. C., Sudabattula, S. K., Prabaharan, N., and Hossain, E. (2022). Combined approach for power loss minimization in distribution networks in the presence of gridable electric vehicles and dispersed generation. IEEE Syst. J. 16 (2), 3284–3295. doi:10.1109/JSYST.2021.3123436
Viswanath, B., Khatod, D. K., and Padhy, N. P. (2022). Vehicle-to-Grid (V2G) optimization for grid peak demand reduction and decarbonization: A state-of-the-art review,” in 2022 IEEE 10th Power India International Conference (PIICON), 1–6. doi:10.1109/PIICON56320.2022.10045118
Keywords: electric vehicle charging station, distributed generator, vehicle to grid, grid to vehicle, cost–benefit analysis
Citation: Golla NK, Dharavat N, Sudabattula SK, Velamuri S, Kantipudi MVVP, Kotb H, Shouran M and Alenezi M (2023) Techno-economic analysis of the distribution system with integration of distributed generators and electric vehicles. Front. Energy Res. 11:1221901. doi: 10.3389/fenrg.2023.1221901
Received: 13 May 2023; Accepted: 19 June 2023;
Published: 03 July 2023.
Edited by:
Arvind R. Singh, University of Pretoria, South AfricaReviewed by:
Rajeev Kumar, KIET Group of Institutions, IndiaSalem Al-Ameri, Curtin University Sarawak, Malaysia
Copyright © 2023 Golla, Dharavat, Sudabattula, Velamuri, Kantipudi, Kotb, Shouran and Alenezi. This is an open-access article distributed under the terms of the Creative Commons Attribution License (CC BY). The use, distribution or reproduction in other forums is permitted, provided the original author(s) and the copyright owner(s) are credited and that the original publication in this journal is cited, in accordance with accepted academic practice. No use, distribution or reproduction is permitted which does not comply with these terms.
*Correspondence: Mohammed Alenezi, QWxlbmV6aW0xQGNhcmRpZmYuYWMudWs=