Development of a supply chain model for the production of biodiesel from waste cooking oil for sustainable development
- 1Department of Mechanical Engineering, University of Engineering and Technology Lahore, Lahore, Pakistan
- 2Department of Industrial Engineering, College of Engineering, King Saud University, Riyadh, Saudi Arabia
- 3Department of Agricultural Engineering, Bahauddin Zakariya University, Multan, Pakistan
- 4Department of Mechanical Engineering, College of Engineering and Technology, University of Sargodha, Sargodha, Pakistan
- 5Faculty of Science and Technology, Jan Dlugosz University in Czestochowa, Czestochowa, Poland
The increasing demand for energy and the severe environmental and economic repercussions have contributed to the development of renewables options. The scarcity of fossil fuels and their negative effect on the environment have sparked an alarming situation for alternative energy sources that are cleaner and more sustainable. Waste cooking oil is a valuable feedstock for biodiesel production, but it is often disposed of improperly, causing environmental pollution and health hazards. The current waste cooking oil supply chain in Pakistan and other countries is fragmented, inefficient, and often unregulated, leading to a lack of standardization and quality control. The study aims to develop a comprehensive supply chain model that integrates waste cooking oil collection, transportation, processing, and biodiesel production to create a sustainable value chain that benefits the environment, the economy, and society as a whole. The proposed optimization approach reduces the total expenses associated with the activities of the biodiesel supply chain. Modified possibilistic chance constrained programming (MPCCP) is used as a solution technique to represent this uncertainty. The MPCCP model is solved with the assistance of Lingo 18.0, while fuzzy logic demand forecasting was done using MATLAB. Accordingly, the fuzzy logic designer (FLD) simulation was conducted to demonstrate the applicability and effectiveness of FLD simulation for the particular kind of issue being considered. The research, not only focuses on mitigating environmental and health risks associated with improper waste cooking oil disposal, resulting in reduced pollution and a cleaner environment but it also advocates for the efficient utilization of waste cooking oil as a valuable feedstock for biodiesel production, thereby promoting a more sustainable and renewable energy source. By optimizing supply chain activities and minimizing costs, the research contributes to enhancing economic growth and efficiency within the biodiesel industry. This research encourages further exploration and collaboration among researchers and stakeholders to expand the applications of the proposed model in waste management, renewable energy, and supply chain optimization.
1 Introduction
Global energy consumption is increasing exponentially, especially in developing nations where it plays an important role in socioeconomic development (Habib et al., 2021). Scientists have been looking for alternative energy sources due to issues including global warming, energy scarcity, and food scarcity, as well as the strong desire for a better quality of life (Wang P. et al., 2022). Renewable energy has gained the attention of academic and industrial experts in order to secure future energy reliability and sustainability (Shang et al., 2023). As a result, a sustainable approach toward sources of renewable energy is one option to satisfy society’s growing energy demands (Li et al., 2023). Due to technical advancements and the industrialization, globally societies became more dependent on fossil fuels (Wang et al., 2023). Furthermore, the combustion of fossil fuels greatly affects the surroundings by raising carbon emissions and the Earth’s average temperature (Mishra et al., 2020). Even while fossil fuels are essential to society’s development, they have a negative effect on climate change (Xu et al., 2022). Over the past century, the usage of fossil fuels in the manufacturing and building industries has resulted in huge emissions that have sparked the global warming process (E et al., 2019). Traditional fuels could be replaced with agricultural waste, forestry waste, and their byproducts (Wan et al., 2022). As a result, over the past 20 years, interest in biofuels generated from biomass has greatly expanded on a global scale (Guo et al., 2023).
It is evident that adoption of sustainable unconventional energy resources as an alternative to fossil fuels might help to reach the world’s energy demands in the future (Shah et al., 2018). Biodiesel is one of the unconventional fuels that may be used instead of traditional fuels and still be considered environmentally friendly. The popularity of biodiesel as an alternative energy source has a number of advantages over other petroleum-based products, including a higher flash point, increased flowability, and minimal toxicity (Banković-Ilić et al., 2014). It is still debatable whether or not biodiesel made from first-generation sources can be considered sustainable due to the fact that it is more expensive than fossil fuel (Victor T. Wyatta et al., 2005) (Ghaderi et al., 2018). The main reason for the restricted use of biodiesel in business settings is that its manufacturing is rather expensive. The cost of the raw materials accounts for anything between 70 and 95 percent of the entire cost of producing biodiesel. Because diesel fuel derived from petroleum is more cost-effective than biodiesel made from food-grade oils, it is essential to find alternative oily feedstocks that are both new and less expensive in order to produce biodiesel (Gui et al., 2008; Leung et al., 2010; Balat, 2011). It is possible to increase the production economics of a product that is both environmentally friendly and sustainable by making use of inexpensive used-cooking oils, by-products from consumable-oil refineries, and non-consumable oils (Zhang S. et al., 2022). The conversion of used-cooking oil into biodiesel is seen as a good way to deal with issues relating to food security, the energy disaster, and environmental issues (Yu et al., 2023a). Waste cooking oil, on the other hand, has not been recycled for use in industry in a satisfactory manner during the previous several years (Zhang et al., 2017). Researchers have recently been interested in waste cooking oil because of its potential to serve as a feedstock that is economically sustainable (Kleinová et al., 2011). However, it is evident that there is need to focus on how to minimize production costs when using biodiesel made from waste cooking oil (Kim et al., 2011; Ji et al., 2022). According to Singhabhandhu and Tezuka (Singhabhandhu et al., 2010) there are some of the benefits of utilizing waste cooking oil as feedstock as opposed to using other forms of feedstock. For example, as compared to feedstocks, waste cooking oil is more cost-effective in terms of profitability; and easy to dispose and maintain its steady supply. Whereas, due to commercialization, social and environmental issues, desire is to have sustainable development in biodiesel fuels, considering all three components as: economics, environment, and society (Babazadeh et al., 2017). Similarly, having an effective supply chain network (SCND) for biodiesel fuels needs making strategic choices at the level of defining the volume, location, and capacity of manufacturing plants (Babazadeh et al., 2013). This prompted academics to build SCND optimization models so that systematic supply chains from feedstock source locations to demand zones may be built (Qu et al., 2022).
This research is different from the previous one as it introduces a mathematical model that considers waste cooking oil collection and pretreatment, biorefineries, and biodiesel demand zones, with the aim of minimizing costs and the percentage of carbon emissions related to biofuel production and distribution. In addition, to address uncertainties in the optimal solution, a fuzzy modified possibilistic chance constrained programming approach (MPCCP) is proposed. Moreover, the study proposes an interactive fuzzy programming technique to solve the waste cooking oil-based biodiesel supply chain model. The research’s emphasis on reducing costs and carbon emissions in the biofuel supply chain aligns with the objective of fostering responsible consumption and production practices which is very important among sustainable development goals (SDG) given by United Nation (UN). By implementing strategies to optimize efficiency and environmental impact, the study contributes to the promotion of sustainable resource management and sustainable development. Moreover, the research is mapped with another SDG such as climate action as it aims to reduce carbon emissions by integrating a carbon tax and implementing strategies to minimize environmental impact in the biodiesel supply chain. Initially, the relevant literature review is presented in the following Section 2. While Section 3 presents problems at hand. Subsequently, Section 4 presents formulation of desired supply chain model and fuzzy possibilistic programming method to manage uncertainty. Section 5 presents the application of model as a case study. The results obtained and the sensitivity analyses carried out are also presented in this paper in Section 6. Lastly, conclusion, limitations of study and future scopes are presented in Section 7.
2 Literature review
There is a high degree of unreliability in the logistics chain network owing to the capacity limitations of local firms and changes in global market demand (Munir et al., 2022). Various types and degrees of uncertainties may be found throughout the biodiesel supply chain (Geng et al., 2021a). The manufacturing of biodiesel from a huge variety of biofuels, which may be broken down into two categories: edible and non-edible, was the subject of discussion in a number of research articles (Xiao et al., 2022). Biofuel generation from edible fuel sources has been shown to be significantly discouraged due to competition with the food market (Naik et al., 2010; Mohr et al., 2013; Safieddin Ardebili et al., 2018). In recent years, there has been a huge amount of interest in the use of biodiesel as an alternative fuel. This is due to the fact that biodiesel offers a number of benefits over petroleum diesel, particularly in form of how well it performs in engines and how well it is for these kinds of efforts may also assist reduce reliance on oil imported from other countries and the consequences of greenhouse gases. There is a wide range of different non-edible feedstocks that may be found in the study that has been conducted. (Ahmed et al., 2018). developed a mathematical framework for the manufacturing of biodiesel using agricultural residuals as feedstock. This model took into account the fact that both the demand for biodiesel and the availability of feedstock were unknown variables (Ahmed et al., 2018). Under the unpredictability of distribution (Zahraee et al., 2020) created a deterministic planning and forecasting framework. A multiperiod and multilevel (MILP) model was constructed by Giarola et al. (Giarola et al., 2012) in order to handle the issues of organic material allocation, production machinery selection, and plant placement. This model took into consideration the unpredictability of biomass supply as well as a CO2 emission quota trading scheme, with the goal of increasing the total present value of the supply chain and decreasing CO2 emissions (Zhang et al., 2022b). The procurement process has a number of major uncertainty issues, one of the most important of which is the uncertainty over the availability of biomass (Zhang et al., 2022c). On the other hand, the supply of biomass is clearly seasonal since it is dependent on planting and harvesting activities and needs a relatively constant growth cycle (Li et al., 2020). However, there is a regional and temporal variation in how waste cooking oil and other municipal wastes are used by urban inhabitants and the economic growth of the city. Due to competition from the unlawful “gutter oil” manufacturing chain, the real quantity of biomass gathered by refining facilities is further exacerbated by ambiguity about biomass (Nguyen et al., 2018). Biodiesel supply chain network design employing Jatropha carcass L. and cooking oil as feedstocks was created by (Babazadeh et al., 2013) for decreasing SC cost and environment effect in the face of epistemic uncertainties. The authors developed a unique solution methodology to reduce the overall risk values in order to reduce the uncertainty effect on this innovative solution methodology (Kim et al., 2011). (Hu et al., 2017) modelled a supply chain that included a service need, an unpredictable supply and demand, and a profit-sharing agreement. From this model, they were able to extract the best ordering policy for the buyer as well as the ideal supply policy for the provider (Hu et al., 2017).
Supply chain sustainability has emerged as a new technique for making business choices by considering the effects on the environment, economy, and society all at once (Geng et al., 2021b). In a biofuel supply chain network design challenge, (Yu et al., 2023b), used a MILP to improve economic, energy, and environmental goals. Each megajoule of biofuel manufacturing requires a certain amount of fossil fuel energy to produce. In the early stages of an engineering project, attention is often paid to environmental concerns (Wang Y. et al., 2022). As a result of these uncertainties, they used a robust possibilistic programming (RPP) approach in order to reduce the influence of these variables (Mousavi Ahranjani et al., 2018). An economic, social, and environmental MILP model developed by (Ziolkowska, 2013) examined the SC of bioethanol generated from cellulose. A biofuel SCND aimed to reduce costs and CO2-equivalent emissions (Yan et al., 2021). As the bioenergy sector grows, new jobs and economic vibrancy are expected to be created in rural regions, which is good for social sustainability (Baudry, 2018; Gao et al., 2019). Researchers have been pushed to build SCND optimization models as a result of the fast development of operations within the biodiesel sector (Huang et al., 2010; Ghelichi et al., 2018). The ideas and techniques of biodiesel SCND optimization have been the subject of substantial study by academics, particularly in the fields of biodiesel distribution network facility. First, a gap analysis is done by examining the current supply chain studies and discussing essential elements displayed in Table 1.
The commercial dynamics of biofuel supply chain functions are inherently riskier than those inside the conventional supply chain. This results in very uncertain data for parameters whose values are unknown. According to (Pishvaee et al., 2011) there are two primary categories of data uncertainty: Randomness causes (1) a uncertainty (2) Uncertainty originating from a lack of knowledge (epistemic uncertainty). In the first sort of randomness, the parameter itself has an unpredictability of its own. These strategies are most successful if sufficient historical data on parameter distributions are provided (Pishvaee et al., 2012). When dealing with circumstances in which the data acquired is tainted by epistemic uncertainty, the most effective method to use is called “possibilistic programming. To solve the suggested optimization model, a solution approach that is based on possibilistic chance constrained programming is used. The following are some of the ways in which the planned study would add to the current body of knowledge about the biodiesel supply chain:
• Proposing a mathematical model that takes into account the collecting and pretreatment of waste cooking oil, biorefineries, and biodiesel demand zones in order to reduce the costs associated with producing and distributing biofuels.
• For dealing with the unknown factors of the suggested optimal solution, Fuzzy modified possibilistic chance constrained programming approach is provided.
• To solve the waste cooking oil-based biodiesel supply chain model, an interactive fuzzy programming technique is being proposed.
3 Problem statement and adopted supply chain network
The supply chain is composed of three major participants in this research: a waste cooking oil supplier, an integrated biorefinery, and a market zone with its own distinct processes. At supplier’s locations, kitchen trash is generated on a daily basis (restaurants, guest houses, food market, etc.). After that, it is transferred to integrated biorefineries for preprocessing and biodiesel synthesis by small trucks. The biodiesel that was manufactured is ultimately transported to the demand regions. In the future, there will be increased unpredictability around the generation of biodiesel from used cooking oil due to factors such as the availability of feedstock, the demand for biodiesel, and the evolution of rules and policies. According to the data collected over the course of the previous decade, the overall amount of trash produced in kitchens has grown by anywhere from 1 percent to 8 percent. When it comes to planning the whole waste cooking oil supply chain, the aim is to build a possibilistic programming model that takes into account process validation and provides sustainable solutions. The particular difficulties that need to be handled by the (MPCCP) model involve determining the total cost of the waste cooking oil under the agreement that was signed by the restaurants and the biodiesel producer.
Thus, the network of supply chain facilities includes waste cooking oil supply points, waste cooking oil preprocessing units, biodiesel production units, transportation of resources between the various processing facilities and distribution. The following Figure 1 depicts the adopted framework.
In the above supply network, used cooking oil is considered a potential source of feedstock for biodiesel. Waste cooking oil is sourced from processing plants located in various regions across the country. Prior to being transported to biorefineries, the waste cooking oil undergoes preprocessing at a central facility. Following pretreatment, the fat from waste cooking oil is converted into biodiesel at the biorefineries, which is then distributed to regions with demand for the fuel. When planning the transportation of preprocessed oil and biodiesel within the network, road transport is a preferred consideration. The consumption of biodiesel and the availability of feedstock supplies are both highly unpredictable. Additionally, factors like CO2 emissions, shipping, processing, and storage costs are challenging to accurately forecast during the design phase. Fuzzy numbers are employed to represent the parameters in this model due to their ability to handle the ambiguity of the situation.
4 Mathematical modeling
For this proposed framework for waste cooking oil-based biodiesel supply network the notations, variables, objective functions, and constraints, and the proposed modified possibilistic chance constrained programming method are presented.
4.1 Notations and variables
j is index for waste cooking oil Preprocessing unit
k is index for bio refineries
L is index for demand zone area
T represents time period.
4.1.1 Parameters
η Conversion Factor for WCO feedstock.
4.1.2 Decision variables
4.2 Objective functions
Sustainable biodiesel supply chain system’s economical purpose is to reduce the financial cost. The total cost minimization of producing biodiesel from used cooking oil in a supply chain is shown in Eq. 1
Fixed installation cost of biorefineries
Waste cooking oil purchasing and handling cost
Processed waste oil and biodiesel production cost
Transportation cost of transferring biodiesel and processed oil at different locations
CO2 emissions cost during transportation and handling process of biodiesel
Total cost of waste cooking oil-based biodiesel supply chain network
4.3 Constraints
4.3.1 Capacity constraints
Equation 10 shows the quantity of WCO transferred from collection point to biorefineries cannot surpass the available supply of feedstock.
4.3.2 Production capacity constraint
According to Eq. 11 each biorefinery’s processing capability exceeds or equals the complete feedstock amount carried from fat collecting stations to the biorefineries in a particular period.
4.3.3 Conversion constraint
The mass balance parameter for the production of processed feedstock to biodiesel is shown in Eq. 12, which shows that the total waste oil transported from collection units to biorefineries using conversion factor for fat is equal to the entire quantity of biodiesel that is transported from biorefineries to demand regions.
4.3.4 Demand constraint
The total amount of biodiesel produced by all biorefineries in a particular time period must meet the consumer needs, according to Eq. 13 There is a degree of randomness around the demand, which is shown by a fuzzy figure.
4.3.5 Binary constraint
4.3.6 Non-negative constraint
It is evident that in a real-world economic context of waste cooking oil, there exists a notable level of uncertainty regarding factors such as biodiesel demand, availability of waste cooking oil fat, pricing of biofuels, and various associated costs (Ghaderi et al., 2016). These attributes are influenced by epistemic uncertainties due to the limited knowledge and lack of statistical information. To address such uncertainties effectively, the fuzzy possibilistic programming method emerges as a suitable approach (Ahmed et al., 2018).
4.4 Modified possibilistic chance constrained programming
To deal with uncertainties, possibilistic programming is suggested in the past research (Selim et al., 2006; Niakan et al., 2015). The proposed modified possibilistic chance constrained programming (MPCCP) method is as here below:
Equations 17–22 offer the PCCP-based equivalent formulation of the used cooking oil-based biodiesel supply chain system.
5 Implementation and evaluation through case study
Based on the Pakistani context, the Waste cooking oil model’s effectiveness and performance is evaluated. As of right now, Pakistan uses just a small percentage of its renewable sources, the majority of which are first-generation biomass sources. On the other hand, waste cooking oil, which is now put to use in low-cost applications, has the potential to be used as a source in the production of biodiesel. The yearly consumption of edible oil in Pakistan is 3.7 million metric tones (MMT), which suggests that Waste cooking oil-based biodiesel has a considerable ability to greatly reduce the reliance that the nation has on traditional fossil fuels. In addition, the government is promoting the use of renewables to minimize harmful emissions and achieve long-term development objectives. In this regard, this research offers a useful decision-making tool that will make it possible for the government bodies to meet their objective of achieving sustainable biodiesel.
According to the collected data, there are a total of eight waste cooking oil pre-processing units, four potential sites for biorefinery facilities, and five marketplaces for biodiesel have been selected. Four sites in the area are being investigated for biorefineries with varied biodiesel production capacity. All four provinces have been truncated into four zones, which meet all the criteria of local legislation as well as environmental and industrial regulations. Figure 2 depicts the Potential locations of Waste cooking oil-based biodiesel Production facilities all across Pakistan.
6 Result and discussion
6.1 Analytical results
The proposed model for the waste cooking oil-based biodiesel supply chain is precisely formulated using an experimental dataset to obtain the desired outcomes. The mathematical model was constructed utilizing LINGO 18 optimization software. This model consists of 650 constraints, 310 continuous decision variables, and four binary decision variables. Interactive parameters that need to be selected by decision-makers before results may be obtained include z, supply and demand value. Values are defined based on current conditions, which are constantly changing in actual time. Biodiesel demand and waste oil supply may be assumed by DMs to have an 87% confidence level in a given situation. A total cost of $30,159,860 is attained for the biodiesel manufacturing supply chain system in this particular case scenario. For waste cooking oil-based biodiesel processing and marketing networks, the breakdown of total supply chain costs is shown in Supplementary Tables SA1, SA2. Supplementary Table SA9 shows the amount moved from the pre-processing unit (j) to the biorefineries (k) over time (t), whereas Supplementary Table SA6 shows the volume of biodiesel delivered from the refineries (k) to the market zones (l) over time (t).
For the suggested waste cooking oil-based biodiesel supply chain model, a graphical depiction of optimum options throughout period t is presented in Figure 2 and Figure 3 shows the supply chain network design for pre-processing unit, biorefineries & market zones. The most optimum location for a biorefinery is selected from among four possible sites, as shown in a graphical depiction of optimal options. There are preprocessing plants at Nawab Shah and Mirpur Khas that offer preprocessed oil to the Hyderabad biorefinery, while supply points in Multan and Faisalabad primarily provide preprocessed oil to the Sheikhupura biorefinery. The pre-processed oil from other provinces is sent to the biorefinery closest to the collecting locations. Though it is possible to cut per ton biodiesel production costs by selecting a bigger biorefinery production capacity, the suggested optimization model prefers biorefinery sites with low processing capacity, despite this fact. If chosen, this would greatly raise overall supply chain costs in terms of shipping costs as well as environmental effects. On the other hand, the biodiesel that is generated by the Kohat Biorefinery is only distributed to the marketplaces of Peshawar throughout all times of the year, whilst the Sheikhupura Biorefinery satisfies the need of the marketplaces of Lahore and Islamabad.
6.2 Senstivity analysis for waste cooking oil based biodiesel supply chain model
Following the dynamics of a real-time event, a sensitivity analysis is conducted on the provided model to present a set of optimum options from which to choose. The primary goal of this scenario is to assess the degree of sensitivity of a supply chain dependent on waste cooking oil. We analysed how the negotiated total cost of the supply chain and the structure of the network varied in response to varying objective coefficients for waste pretreatment rates.
6.3 Simulation of model results
To evaluate the collecting point capacity, biorefinery capacity, biodiesel purchase and production cost, and cost per quantity of preprocessed oil and biodiesel demasnd in the market, a fuzzy logic model has been developed. The model was operated using MATLAB 2019a for fuzzy logic simulation. Table 2 shows the quantity of Pre-processed oil supplied by each WCO Pre-processing unit to biorefinery. Similarly Table 3 shows the gallons of biodiesel supplied by each biorefinery to market areas.
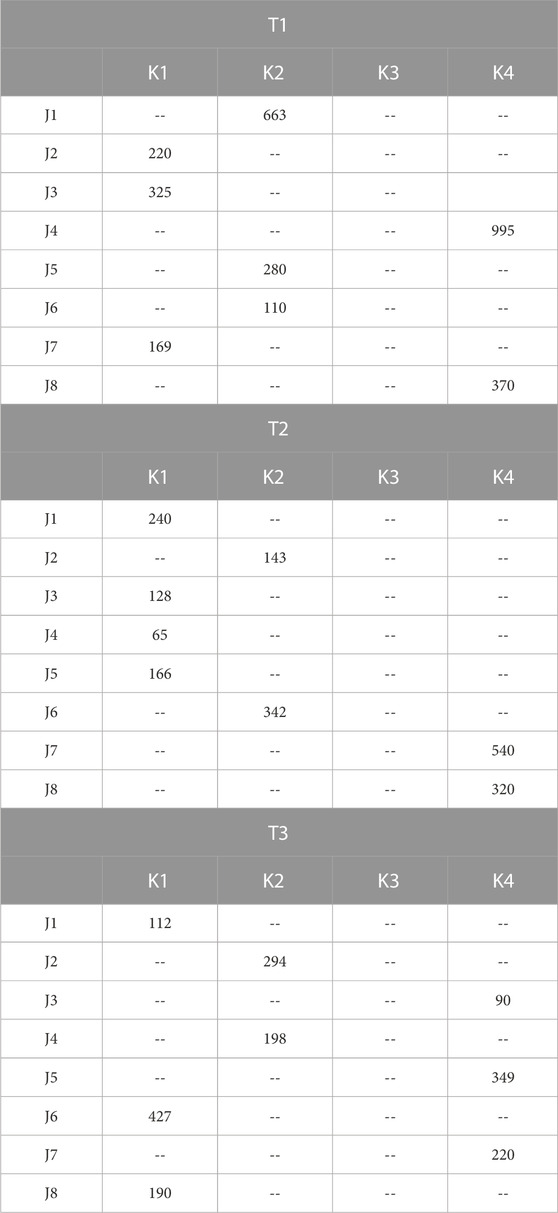
TABLE 2. Quantity of Pre-processed oil supplied by each WCO Pre-processing unit to biorefinery (tons).
According to the established input indicators, the findings reveal that the optimal transfer of preprocessed oil from the collection point to the biorefinery unit occurs when the collection capacity is 70% and the biorefinery capacity is 80%. When the cost of buying is 60% and the cost of producing biodiesel is 70%, the greatest amount of biodiesel has been transferred from the biorefinery to the demand unit. As a result, if you base your calculations on the judgments that were made, K5 and L3 have the highest amount of preprocessed oil and biodiesel. As a result, stakeholders must make the necessary management choices to reduce the risks associated with these outcomes. Table 4 shows the detail of sensitivity report for waste cooking oil based bio diesel supply chain model. Similarly Table 5 provides Fuzzy logic results.
6.3.1 Fuzzy inference system
Decision making is the core function of the Fuzzy Inference System, which is the central component of a fuzzy logic system. Decisions are made using “IF … THEN” logic and the connectors “OR” and “AND”.
6.3.2 Mamdani fuzzy inference system
The Mamdani fuzzy inference can be utilized for the development of a control mechanism. In a Mamdani system, each rule’s output is represented as a fuzzy set. Mamdani systems are particularly suitable for expert system applications, such as medical diagnostics, where rules are derived from human expert knowledge. This is due to their rule bases being more intuitive and easier to comprehend.
6.3.3 Sugano inference system
In Sugano fuzzy inference, the output membership functions use singletons, which can be either constant or linear functions of the inputs. A Sugano system’s defuzzification method employs a weighted average or weighted sum of a few data points rather than computing a centroid of a two-dimensional region, making it more computationally efficient. Use the convert To Sugano function to convert from a Mamdani system to a Sugano system. The output membership functions of the Sugeno system correspond to the Mamdani output membership functions’ centroids.
Fuzzy Logic Toolbox is used to construct the fuzzy logic hierarchical system in the Matlab environment. A Mamdani and sugano-type fuzzy inference system is used to build each of the four subsystems. Figure 4 shows the 3D inference surfaces for the four fuzzy logic subsystems.
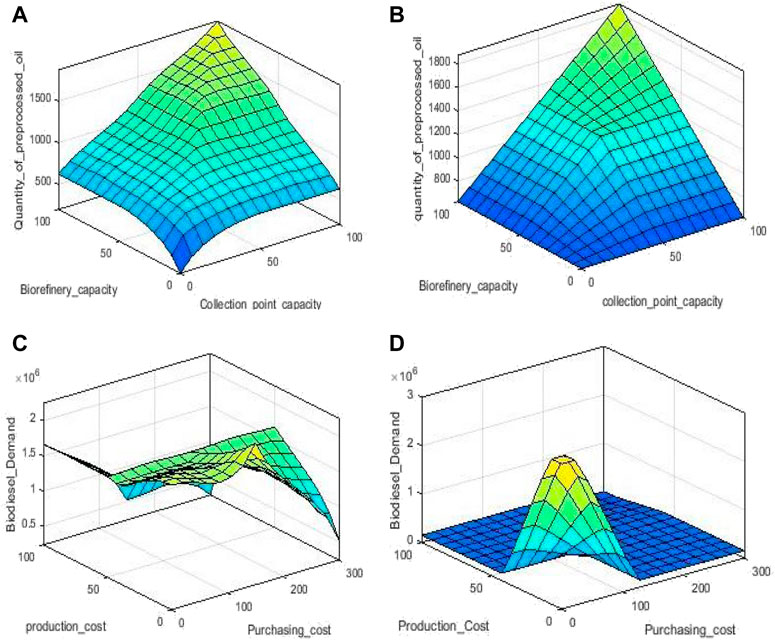
FIGURE 4. (A) Mamdani surface viewer. (B) Sugano surface viewer. (C) Mamdani surface viewer. (D) Sugano surface viewer.
7 Conclusion
The increasing demand for alternative energy sources has been prompted by the rapid exhaustion of fossil fuels. A multi-period supply chain model for biodiesel synthesis is introduced in this study for reducing the entire supply chain cost. In order to reduce carbon emissions in the biodiesel manufacturing and distribution supply chain, a carbon tax has been implemented. This information is polluted by intrinsic epistemic uncertainty due to the changing nature of the business climate for biodiesel SC and the early stages of biodiesel use in Pakistan. The MPCCP based solution approach that offers economic viability to the derived solutions is used because it is effective in dealing with epistemic uncertainty.
While Lingo 18.0 is utilized to solve the deterministic, static, multi-echelon MPCCP model, MATLAB is used for demand forecasting based on fuzzy logic. MATLAB 2019a is used to simulate FLD, demonstrating the usefulness and effectiveness of fuzzy logic designer simulation for this issue. The case-specific SC network and efficient product flow between warehouses and distributors are both developed using the output data. In addition, it is shown that the FLD simulation may be employed in place of analytical calculations, guaranteeing a reduced representation for this approach and saving time.
For this particular scenario, it was shown that by spending an additional 18 percent in costs, the strategic choices of the suggested waste cooking oil-based supply chain model may be protected from the intrinsic epistemic uncertainty. Moreover, the economic objectives analysis shows that logistics activities associated costs are the second most significant expenditure after facility construction costs. Most of the inventory cost is accounted for by logistics operations. To minimize overall logistic cost and the carbon tax paid owing to carbon emissions caused by transportation, facility placement choices play an important role.
The suggested model was tested in a real-world scenario in Pakistan and found to be accurate. However, this study has some limitations. The findings are based on a specific context in Pakistan, which may limit the generalizability of the proposed model to other regions or industries. Furthermore, the study highlights the significance of logistics activities and transportation costs in the overall supply chain costs. However, it would be beneficial to delve deeper into other cost factors and explore potential trade-offs between various objectives such as environmental sustainability and economic viability. Overall, the study provides valuable insights and lays the foundation for further research in optimizing waste cooking oil-based supply chains. Future studies could consider expanding the scope beyond a specific region, evaluating the long-term implications of the proposed model, and exploring additional cost factors and objectives for a more comprehensive analysis.
Data availability statement
The original contributions presented in the study are included in the article/Supplementary Material, further inquiries can be directed to the corresponding author.
Author contributions
MM and HL conceptualized the idea and drafted the manuscript. SI, MF, and AH were the research supervisors and provided guidance for the collection of relevant data. AR, MS, QA, and JK helped in developing frameworks and also contributed for the graphical data and figures. All authors contributed to the article and approved the submitted version.
Funding
This paper is part of the work supported by the Higher Education Commission Pakistan Project number (NRPU 20-12879/NRPU/R&D/HEC/2020). And this research is also funded by the Researchers Supporting Project number (RSPD 2023R701), King Saud University, Riyadh, Saudi Arabia.
Acknowledgments
The authors extend their appreciation to the Researchers supporting Project number (RSPD2023R701), King Saud University, Riyadh, Saudi Arabia.
Conflict of interest
The authors declare that the research was conducted in the absence of any commercial or financial relationships that could be construed as a potential conflict of interest.
The handling editor MR declared a past collaboration with the author MF.
Publisher’s note
All claims expressed in this article are solely those of the authors and do not necessarily represent those of their affiliated organizations, or those of the publisher, the editors and the reviewers. Any product that may be evaluated in this article, or claim that may be made by its manufacturer, is not guaranteed or endorsed by the publisher.
Supplementary material
The Supplementary Material for this article can be found online at: https://www.frontiersin.org/articles/10.3389/fenrg.2023.1222787/full#supplementary-material
References
Ahmed, W., and Sarkar, B. (2018). Impact of carbon emissions in a sustainable supply chain management for a second generation biofuel. J. Clean. Prod. 186, 807–820. doi:10.1016/j.jclepro.2018.02.289
Awudu, I., and Zhang, J. (2012). Uncertainties and sustainability concepts in biofuel supply chain management: A review. Renew. Sustain. Energy Rev. 16 (2), 1359–1368. doi:10.1016/j.rser.2011.10.016
Babazadeh, R., Razmi, J., and Ghodsi, R. (2013). Facility location in responsive and flexible supply chain network design (SCND) considering outsourcing. Int. J. Operational Res. 17 (3), 295. doi:10.1504/ijor.2013.054437
Babazadeh, R., Razmi, J., Pishvaee, M. S., and Rabbani, M. (2017). A sustainable second-generation biodiesel supply chain network design problem under risk. Omega 66, 258–277. doi:10.1016/j.omega.2015.12.010
Balat, M. (2011). Potential alternatives to edible oils for biodiesel production – a review of current work. Energy Convers. Manag. 52 (2), 1479–1492. doi:10.1016/j.enconman.2010.10.011
Banković-Ilić, I. B., Stojković, I. J., Stamenković, O. S., Veljkovic, V. B., and Hung, Y.-T. (2014). Waste animal fats as feedstocks for biodiesel production. Renew. Sustain. Energy Rev. 32, 238–254. doi:10.1016/j.rser.2014.01.038
Baudry, G. (2018). How the cap limit for food-crop-based biofuels may affect France’s stakeholders by 2030? A range-based multi-actor multi-criteria analysis. Transp. Res. Part D Transp. Environ. 63, 291–308. doi:10.1016/j.trd.2018.05.012
Gao, E., Sowlati, T., and Akhtari, S. (2019). Profit allocation in collaborative bioenergy and biofuel supply chains. Energy 188, 116013. doi:10.1016/j.energy.2019.116013
Geng, N., Fu, Q., Sun, Y., and chen, x. (2021a). Stochastic programming of sustainable waste cooking oil for biodiesel supply chain under uncertainty. J. Adv. Transp. 2021, 1–18. doi:10.1155/2021/5335625
Geng, N., Sun, Y., and Wang, T. (2021b). Multiobjective optimization of sustainable WCO for biodiesel supply chain network design. Discrete Dyn. Nat. Soc. 2021, 1–16. doi:10.1155/2021/6640358
Ghaderi, H., Moini, A., and Pishvaee, M. S. (2018). A multi-objective robust possibilistic programming approach to sustainable switchgrass-based bioethanol supply chain network design. J. Clean. Prod. 179, 368–406. doi:10.1016/j.jclepro.2017.12.218
Ghaderi, H., Pishvaee, M. S., and Moini, A. (2016). Biomass supply chain network design: An optimization-oriented review and analysis. Industrial Crops Prod. 94, 972–1000. doi:10.1016/j.indcrop.2016.09.027
Ghelichi, Z., Saidi-Mehrabad, M., and Pishvaee, M. S. (2018). A stochastic programming approach toward optimal design and planning of an integrated green biodiesel supply chain network under uncertainty: A case study. Energy 156, 661–687. doi:10.1016/j.energy.2018.05.103
Giarola, S., Zamboni, A., and Bezzo, F. (2012). Environmentally conscious capacity planning and technology selection for bioethanol supply chains. Renew. Energy 43, 61–72. doi:10.1016/j.renene.2011.12.011
Gui, M. M., Lee, K. T., and Bhatia, S. (2008). Feasibility of edible oil vs. non-edible oil vs. waste edible oil as biodiesel feedstock. Energy 33 (11), 1646–1653. doi:10.1016/j.energy.2008.06.002
Guo, Z., Zhan, R., Shi, Y., Zhu, D., Pan, J., Yang, C., et al. (2023). Innovative and green utilization of zinc-bearing dust by hydrogen reduction: Recovery of zinc and lead, and synergetic preparation of Fe/C micro-electrolysis materials. Chem. Eng. J. 456, 141157. doi:10.1016/j.cej.2022.141157
Habib, M. S., Asghar, O., Hussain, A., Imran, M., Mughal, M. P., and Sarkar, B. (2021). A robust possibilistic programming approach toward animal fat-based biodiesel supply chain network design under uncertain environment. J. Clean. Prod. 278, 122403. doi:10.1016/j.jclepro.2020.122403
Habib, M. S., Tayyab, M., Zahoor, S., and Sarkar, B. (2020). Management of animal fat-based biodiesel supply chain under the paradigm of sustainability. Energy Convers. Manag. 225, 113345. doi:10.1016/j.enconman.2020.113345
Hu, B., and Feng, Y. (2017). Optimization and coordination of supply chain with revenue sharing contracts and service requirement under supply and demand uncertainty. Int. J. Prod. Econ. 183, 185–193. doi:10.1016/j.ijpe.2016.11.002
Huang, Y., Chen, C.-W., and Fan, Y. (2010). Multistage optimization of the supply chains of biofuels. Transp. Res. Part E Logist. Transp. Rev. 46 (6), 820–830. doi:10.1016/j.tre.2010.03.002
Ji, Y., Li, H., and Zhang, H. (2022). Risk-averse two-stage stochastic minimum cost consensus models with asymmetric adjustment cost. Group Decis. Negot. 31 (2), 261–291. doi:10.1007/s10726-021-09752-z
Jiaqiang, E., Zhao, X., Liu, G., Zhang, B., Zuo, Q., Wei, K., et al. (2019). Effects analysis on optimal microwave energy consumption in the heating process of composite regeneration for the diesel particulate filter. Appl. Energy 254, 113736. doi:10.1016/j.apenergy.2019.113736
Kim, J., Realff, M. J., and Lee, J. H. (2011). Optimal design and global sensitivity analysis of biomass supply chain networks for biofuels under uncertainty. Comput. Chem. Eng. 35 (9), 1738–1751. doi:10.1016/j.compchemeng.2011.02.008
Kleinová, A., Vailing, I., Lábaj, J., Mikulec, J., and Cvengroš, J. (2011). Vegetable oils and animal fats as alternative fuels for diesel engines with dual fuel operation. Fuel Process. Technol. 92 (10), 1980–1986. doi:10.1016/j.fuproc.2011.05.018
Leung, D. Y. C., Wu, X., and Leung, M. K. H. (2010). A review on biodiesel production using catalyzed transesterification. Appl. Energy 87 (4), 1083–1095. doi:10.1016/j.apenergy.2009.10.006
Li, H.-M., Li, G.-X., and Li, L. (2023). Comparative investigation on combustion characteristics of ADN-based liquid propellants in inert gas and oxidizing gas atmospheres with resistive ignition method. Fuel 334, 126742. doi:10.1016/j.fuel.2022.126742
Li, Q. K., Lin, H., Tan, X., and Du, S. (2020). H∞ consensus for multiagent-based supply chain systems under switching topology and uncertain demands. IEEE Trans. Syst. Man, Cybern. Syst. 50 (12), 4905–4918. doi:10.1109/tsmc.2018.2884510
Mishra, U., Wu, J.-Z., and Sarkar, B. (2020). A sustainable production-inventory model for a controllable carbon emissions rate under shortages. J. Clean. Prod. 256, 120268. doi:10.1016/j.jclepro.2020.120268
Mohr, A., and Raman, S. (2013). Lessons from first generation biofuels and implications for the sustainability appraisal of second generation biofuels. Energy Policy 63 (100), 114–122. doi:10.1016/j.enpol.2013.08.033
Mousavi Ahranjani, P., Ghaderi, S. F., Azadeh, A., and Babazadeh, R. (2018). Hybrid multiobjective robust possibilistic programming approach to a sustainable bioethanol supply chain network design. Industrial Eng. Chem. Res. 57 (44), 15066–15083. doi:10.1021/acs.iecr.8b02869
Munir, M. A., Habib, M. S., Hussain, A., Shahbaz, M. A., Qamar, A., Masood, T., et al. (2022). Blockchain adoption for sustainable supply chain management: Economic, environmental, and social perspectives. Front. Energy Res. 10. doi:10.3389/fenrg.2022.899632
Naik, S. N., Goud, V. V., Rout, P. K., and Dalai, A. K. (2010). Production of first and second generation biofuels: A comprehensive review. Renew. Sustain. Energy Rev. 14 (2), 578–597. doi:10.1016/j.rser.2009.10.003
Nguyen, D. H., and Chen, H. (2018). Supplier selection and operation planning in biomass supply chains with supply uncertainty. Comput. Chem. Eng. 118, 103–117. doi:10.1016/j.compchemeng.2018.07.012
Niakan, F., and Rahimi, M. (2015). A multi-objective healthcare inventory routing problem; a fuzzy possibilistic approach. Transp. Res. Part E Logist. Transp. Rev. 80, 74–94. doi:10.1016/j.tre.2015.04.010
Pishvaee, M. S., Rabbani, M., and Torabi, S. A. (2011). A robust optimization approach to closed-loop supply chain network design under uncertainty. Appl. Math. Model. 35 (2), 637–649. doi:10.1016/j.apm.2010.07.013
Pishvaee, M. S., Razmi, J., and Torabi, S. A. (2012). Robust possibilistic programming for socially responsible supply chain network design: A new approach. Fuzzy Sets Syst. 206, 1–20. doi:10.1016/j.fss.2012.04.010
Qu, S., Shu, L., and Yao, J. (2022). Optimal pricing and service level in supply chain considering misreport behavior and fairness concern. Comput. Industrial Eng. 174, 108759. doi:10.1016/j.cie.2022.108759
Safieddin Ardebili, S. M., and Khademalrasoul, A. (2018). An analysis of liquid-biofuel production potential from agricultural residues and animal fat (case study: Khuzestan Province). J. Clean. Prod. 204, 819–831. doi:10.1016/j.jclepro.2018.09.031
Selim, H., and Ozkarahan, I. (2006). A supply chain distribution network design model: An interactive fuzzy goal programming-based solution approach. Int. J. Adv. Manuf. Technol. 36 (3-4), 401–418. doi:10.1007/s00170-006-0842-6
Shah, S. H., Raja, I. A., Rizwan, M., Rashid, N., Mahmood, Q., Shah, F. A., et al. (2018). Potential of microalgal biodiesel production and its sustainability perspectives in Pakistan. Renew. Sustain. Energy Rev. 81, 76–92. doi:10.1016/j.rser.2017.07.044
Shang, Y., Lian, Y., Chen, H., and Qian, F. (2023). The impacts of energy resource and tourism on green growth: Evidence from Asian economies. Resour. Policy 81, 103359. doi:10.1016/j.resourpol.2023.103359
Singhabhandhu, A., and Tezuka, T. (2010). Prospective framework for collection and exploitation of waste cooking oil as feedstock for energy conversion. Energy 35 (4), 1839–1847. doi:10.1016/j.energy.2010.01.004
Wan, Z., Zhang, T., Liu, Y., Liu, P., Zhang, J., Fang, L., et al. (2022). Enhancement of desulfurization by hydroxyl ammonium ionic liquid supported on active carbon. Environ. Res. 213, 113637. doi:10.1016/j.envres.2022.113637
Wang, P., Yu, P., Huang, L., and Zhang, Y. (2022a). An integrated technical, economic, and environmental framework for evaluating the rooftop photovoltaic potential of old residential buildings. J. Environ. Manage 317, 115296. doi:10.1016/j.jenvman.2022.115296
Wang, Y.-n., Wang, Q., Li, Y., Wang, H., Gao, Y., Sun, Y., et al. (2023). Impact of incineration slag co-disposed with municipal solid waste on methane production and methanogens ecology in landfills. Bioresour. Technol. 377, 128978. doi:10.1016/j.biortech.2023.128978
Wang, Y., Cao, Q., Liu, L., Wu, Y., Liu, H., Gu, Z., et al. (2022b). A review of low and zero carbon fuel technologies: Achieving ship carbon reduction targets. Sustain. Energy Technol. Assessments 54, 102762. doi:10.1016/j.seta.2022.102762
Wyatta, Victor T., MelissaHessaFogliaa, A. R. O. D. Thomas A., Haasa, M. J., Marmer, William N., and Marmer, W. N. (2005). Fuel properties and nitrogen oxide emission levels of biodiesel produced from animal fats. J. Am. Oil Chemists' Soc. 82, 585–591. doi:10.1007/s11746-005-1113-2
Xiao, D., Hu, Y., Wang, Y., Deng, H., Zhang, J., Tang, B., et al. (2022). Wellbore cooling and heat energy utilization method for deep shale gas horizontal well drilling. Appl. Therm. Eng. 213, 118684. doi:10.1016/j.applthermaleng.2022.118684
Xu, X., Lin, Z., Li, X., Shang, C., and Shen, Q. (2022). Multi-objective robust optimisation model for MDVRPLS in refined oil distribution. Int. J. Prod. Res. 60 (22), 6772–6792. doi:10.1080/00207543.2021.1887534
Yan, L., Yin-He, S., Qian, Y., Zhi-Yu, S., Chun-Zi, W., and Zi-Yun, L. (2021). Method of reaching consensus on probability of food safety based on the integration of finite credible data on block chain. IEEE Access 9, 123764–123776. doi:10.1109/access.2021.3108178
Yu, D., Guo, J., Meng, J., and Sun, T. (2023a). Biofuel production by hydro-thermal liquefaction of municipal solid waste: Process characterization and optimization. Chemosphere 328, 138606. doi:10.1016/j.chemosphere.2023.138606
Yu, D., Wan, X., and Gu, B. (2023b). Bi-objective optimization of biomass solid waste energy system with a solid oxide fuel cell. Chemosphere 323, 138182. doi:10.1016/j.chemosphere.2023.138182
Zahraee, S. M., Shiwakoti, N., and Stasinopoulos, P. (2020). Biomass supply chain environmental and socio-economic analysis: 40-Years comprehensive review of methods, decision issues, sustainability challenges, and the way forward. Biomass Bioenergy 142, 105777. doi:10.1016/j.biombioe.2020.105777
Zhang, S., Zhou, Z., Luo, R., Zhao, R., Xiao, Y., and Xu, Y. (2022a). A low-carbon, fixed-tour scheduling problem with time windows in a time-dependent traffic environment. Int. J. Prod. Res., 1–20. doi:10.1080/00207543.2022.2153940
Zhang, X., Ma, F., Dai, Z., Wang, J., Chen, L., Ling, H., et al. (2022b). Radionuclide transport in multi-scale fractured rocks: A review. J. Hazard Mater 424 (Pt C), 127550. doi:10.1016/j.jhazmat.2021.127550
Zhang, X., Wang, Z., Reimus, P., Ma, F., Soltanian, M. R., Xing, B., et al. (2022c). Plutonium reactive transport in fractured granite: Multi-species experiments and simulations. Water Res. 224, 119068. doi:10.1016/j.watres.2022.119068
Zhang, Y., and Jiang, Y. (2017). Robust optimization on sustainable biodiesel supply chain produced from waste cooking oil under price uncertainty. Waste Manag. 60, 329–339. doi:10.1016/j.wasman.2016.11.004
Keywords: supply chain, biodiesel, waste cooking oil, sustainable development, waste management
Citation: Munir MA, Imran S, Farooq M, Latif H, Hussain A, Rehman AU, Sultan M, Ali Q and Krzywanski J (2023) Development of a supply chain model for the production of biodiesel from waste cooking oil for sustainable development. Front. Energy Res. 11:1222787. doi: 10.3389/fenrg.2023.1222787
Received: 15 May 2023; Accepted: 15 June 2023;
Published: 26 June 2023.
Edited by:
Mohammad Rehan, King Abdulaziz University, Saudi ArabiaReviewed by:
Muhammad Shahbaz, Hamad bin Khalifa University, QatarAlberto Pettinau, Sotacarbo S.p.A., Italy
Muhammad Waqas, Kohat University of Science and Technology, Pakistan
Copyright © 2023 Munir, Imran, Farooq, Latif, Hussain, Rehman, Sultan, Ali and Krzywanski. This is an open-access article distributed under the terms of the Creative Commons Attribution License (CC BY). The use, distribution or reproduction in other forums is permitted, provided the original author(s) and the copyright owner(s) are credited and that the original publication in this journal is cited, in accordance with accepted academic practice. No use, distribution or reproduction is permitted which does not comply with these terms.
*Correspondence: M. Farooq, engr.farooq@uet.edu.pk