- 1Laboratoire d’Ecologie, de Botanique et de Biologie végétale (LEB), Université de Parakou, Unité de Recherche en Biologie forestière et Modélisation Ecologique (UR-BioME), Parakou, Benin
- 2Department of Plant and Soil Sciences, University of Pretoria, Pretoria, South Africa
- 3Laboratoire de Recherche sur l’Innovation pour le Développement Agricole (LRIDA), Université de Parakou, Parakou, Bénin
- 4Inspection Forestière du Borgou, Direction Générale des Eaux, Forêts et Chasse, Parakou, Benin
Understanding the spatial variation of factors driving interest in bioenergy is important for designing effective energy policies. Although previous authors have broadly explored the determinants of biomass supply intentions in the field of bioenergy, the potential spatial variation of these factors has received limited consideration from bioenergy research. We performed a structural equation modeling based on the theory of planned behavior to investigate the impact of spatial disparities on factors determining people’s intentions to supply forestry residues for clean energy. Our data were generated through a survey of 247 participants randomly sampled from two contrasting geographic regions (north and south) of the Republic of Benin. We found that spatial disparities altered the effects of attitude and knowledge of renewable energy while income and perceived behavioral control exhibited consistent positive effects across geographical regions. Our findings imply that ignoring spatial disparities when analyzing bioenergy acceptance can lead to distorted inferences. These findings can be used as a baseline information by policymakers to develop region-specific actions for achieving local transition toward clean energy in the Republic of Benin.
1 Introduction
The success of bioenergy promotion and development significantly depends on people’s awareness, acceptance, and willingness to adopt, use, and pay for such energy (Liobikienė et al., 2021). In the field of bioenergy, social opinion analysis generally seeks to identify and explain the determinants of respondents’ attitudes and intentions or willingness towards bioenergy adoption, encompassing aspects ranging from biomass supply to energy consumption. This is typically a theory-driven analysis of key human internal dimensions (attitudes, intentions, subjective norms, and perceived behavioral control), social dimensions (socioeconomic or sociodemographic characteristics), and external dimensions (energy price and accessibility). Numerous authors have extensively explored the determinants of biomass supply decisions (Al-Mulali et al., 2016; Baležentis et al., 2019; Liobikienė et al., 2021). However, they overlooked the potential spatial variation of these factors, which is receiving increasing attention in bioenergy research (Brunson and Shindler, 2004). According to the first law of geography (Miller, 2004), the geographical distance between locations is an important factor that can affect stakeholders’ decision-making and their behavior. As a result, the intentions to supply biomass resources for bioenergy may differ among geographically distant regions primarily due to variations in resource availability, competing resource uses, socioeconomic conditions, and cultural factors. This implies that spatial variation in biomass supply intention could also correlate with key intention predictors such as attitude, environmental concern (EC), knowledge of renewable energy (KRE), subjective norms (SN), and perceived behavioral control (PBC). Investigating this aspect in the field of the bioenergy is important for several reasons. First, it can help policymakers to identify the specific needs and challenges for each region and design more effective and region-specific initiatives to promote bioenergy adoption (He et al., 2022). Second, it can guide the development of supportive policy frameworks that consider unique spatial contexts, enabling the creation of incentives, infrastructure, and supportive measures that encourage bioenergy production (Skevas et al., 2018; Khanna et al., 2021). Third, by considering the specific spatial characteristics, such as biomass resources availability, land use patterns, and socioeconomic factors, stakeholders can make informed decisions regarding the development of bioenergy projects, distribution networks, and infrastructures (Thomas et al., 2014; Kabir, 2021).
To address the question of spatial variation or spatial interdependence in decision-making within the field of bioenergy, various methodological frameworks have been employed in the literature, including correlation tests (Brunson and Shindler, 2004), Bayesian frameworks (Holloway et al., 2007; Skevas et al., 2018), spatially lagged explanatory variable (SLX) and spatial Durbin error probit models (SDEM), as discussed by Storm et al. (2015). However, we did not use any of these approaches because Bayesian frameworks, SLX, and SDEM are more commonly employed for spatial interdependence analysis, while simple correlation tests fail to account for multiple causal relationships, including mediation effects.
In this study, we employed Structural Equation Modeling (SEM) based on the Theory of Planned Behaviour (TPB) as a methodological framework. This framework is powerful in designing a structural model that links the three human dimensions (psychological, social, and external) to test their direct or mediation effects on intentions or behaviors (Ajzen, 1991). The TPB postulates that intention is positively predicted by three main latent variables: attitude, SN and PBC (Ajzen, 1991; Fishbein and Ajzen, 2010). The TPB has been widely used in the field of bioenergy (Collins and Carey, 2007; Leitch et al., 2013; Yee et al., 2022) and continues to evolve through incorporation of new predictor variables such as EC, KRE and sociodemographic or socioeconomic factors such as age, sex, and income (Loera et al., 2022). Based on this background, we expanded the TPB initial model by adding two latent factors (EC and KRE) and two socioeconomic variables (age and income) in this study. These new variables are increasingly being used as key predictors of attitude and intentions in the bioenergy field (Lai et al., 2015).
The main objective of this study is to examine whether the intentions to supply forestry residues for clean energy purposes and the factors determining these intentions are influenced by spatial disparities. Spatial disparities refers to all inequality in the distribution of geographical variables across different spatial units (Kanbur and Wan, 2006). In our study context, the concept of “spatial disparities” refers to the divergence in multiple socioeconomic factors such as income, economic potential, education, poverty, urbanization, and competition for the use of forestry residues between two distant geographical regions (northern vs. southern region) in the Republic of Benin. The northern region is less urbanized and has a lower economic potential and education level compared to the south. However, it has a higher poverty level and is a significant source of woody biomass (stemwood, logging and wood processing byproducts, and stumps) due to its higher forest cover compared to the south (Ahononga et al., 2020; Biaou et al., 2021).
2 Materials and methods
2.1 An overview of the TPB
The TPB has been instrumental in understanding the multiple factors that drive human behaviors. Particularly, in the bioenergy sector, researchers frequently employ TPB to identify and enhance elements that encourage public intentions to support bioenergy initiatives. The TPB was first proposed by Ajzen (1991) and can be defined as an updated model of the Reason Action Theory. The TPB postulates that the intentions of a person is an accurate measure of his behaviors and that his intention is determined by his attitude, SN, and PBC. In this line, the theory posits that people evaluate the salient consequences (advantages and drawbacks) of the behaviors they want to perform before engaging in them and they are more likely to perform behaviors associated with desirable advantages (Ajzen and Fishbein, 1975; Bang et al., 2000). A behavior’s performance by a person is also determined by his motivations to comply with related people’s opinions and his ability to manage barriers against that behavior and opportunities.
Mathematically, attitude is defined as the sum of the product of the beliefs (bi) about the behavioral object and the valence evaluation (ei) of the beliefs (Figure 1). The theory posits that a person with strong beliefs about the advantages of a particular behavior have a higher intention to perform it. One can mathematically define SN as the sum of the product of what other people think about the behaviors that a person intends to perform (NBi) and his motivations to comply with those beliefs (MCj). The theory also posits that persons with strong normative beliefs are more likely to perform a behavior than not perform it. The PBC can be defined as the level of confidence of a person to perform a behavior. It is a person’s ability to challenge hindrances against behavioral performance by using the internal facilitators (skills, knowledge, and experience) and external facilitators (information and opportunities) of a behavior (Ajzen, 1991). PBC is the sum of the product of a person’s control of his behaviors (ci) and his perceived control (pi). As predicted by TPB, persons with strong beliefs about particular behavioral facilitators are more likely to perform them.
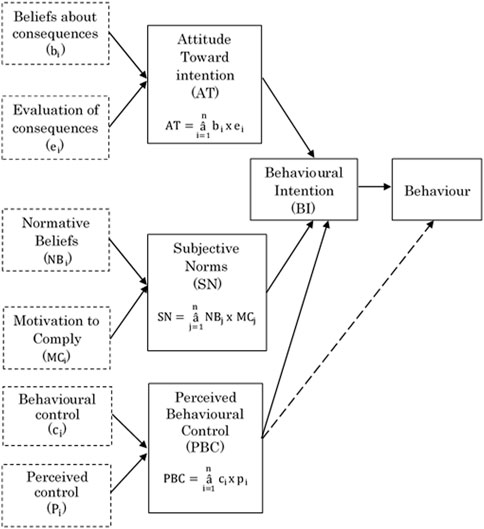
FIGURE 1. Representation of the theory of planned behavior (Ajzen, 1991).
2.2 Modification of the original TPB model and research hypotheses
We expanded the Ajzen (1991) initial TPB model by including EC and KRE as key latent variables, as well as age and income (monthly income) as socioeconomic variables (Figure 2). In fact, previous authors have reported a positive correlation between biomass supply intentions and EC or KRE (Lai et al., 2015; Ali et al., 2023). Positive correlations were also found between both EC, KRE and attitude (Liobikienė et al., 2021). It was also found that the effect of EC or KRE on intentions may be mediated by attitude (Li et al., 2019). Age and income were previously used as key socioeconomic and demographic predictors of intentions (Liu et al., 2013; Dagiliūtė, 2023). Age-intention relationship is reportedly negative across the literature (Joshi and Mehmood, 2011; Liu et al., 2013) whereas income was positively correlated with intentions (Liu et al., 2013; Karanja and Gasparatos, 2019). Based on the predictions from the TPB and previous findings, we tested the following four hypotheses.
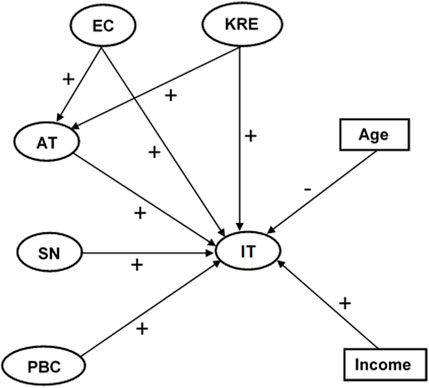
FIGURE 2. Hypothesized structural equation model: SN, Subjective norms; PBC, Perceived behavioral control; AT, Attitude towards intention; EC, Environmental concern; KRE, Knowledge about renewable energy; IT, Intention to supply forestry residues for bioenergy. The sign + indicates a positive effect and the sign–indicates a negative effect. The arrows towards IT and AT indicate the causality path between the two variables and their predictor variables.
H1: Respondents with a more positive attitude towards forestry residues supply for clean energy production, a stronger alignment with SN, and greater PBC, particularly, those who are environmentally friendly or have more KRE, are likely to exhibit higher intentions to supply forestry residues for clean energy production.
H2: Demographic characteristics, such as having a higher income or being younger, are positively associated with the intentions to supply forestry residues for clean energy production.
H3: The effect of EC and KRE on the intentions to supply forestry residues for clean energy production is mediated by the attitude towards these intentions.
H4: Spatial disparities have a significant influence on the determinants of people intentions to supply forestry residues for clean energy production.
2.3 Study area
The study was carried out in the Republic of Benin, a West African country located between 6°15′ and 12°25′ N and 0°40′ and 3°45′E (Figure 3). The country covers an area of 114 763 km2 with a population density of 87.2 inhabitants per km2 (11 186 785 inhabitants) in 2017 (INSAE and ICF, 2019). The south is more populated (57.60 inhabitants per km2) compared to the north (29.59 inhabitants per km2). About 42% of the population of Benin is poor, with a higher rate of poverty in the north (60% of the population) compared to the south (35% of the population). The level of education is higher in the south, with 76% of the population having received at least a primary education, in contrast to the north, where only 40% have reached the same educational level (INSAE, 2015; INSAE and ICF, 2019). Approximately half of the country’s households (50%) used fossil fuel for lighting, with a higher rate in the south (59.22%) compared to the north (20.82%). About a third of the country’s households (33.42%) purchase electricity from the government (Agbandji et al., 2020). The south is more electrified (34.55% of the households) than the north (18.52%) (Yatokpa et al., 2010). Charcoal is commonly used for cooking across the country, but it is more commonly used in the south (28.6% of households) than in the north (18.97%). The use of renewable energy sources, such as solar power and residue-derived energy (from forestry and agriculture), is currently limited. The rainfall varies from 800 mm in the Sudanian zone (north) to 1300 mm in the Guinean zone (south) (Adomou, 2005; Akoègninou et al., 2006). Soils are ferruginous in the north and ferralitic in the south. The vegetation is mainly composed of savannas. The north has a higher forest coverage and encompasses 92.5% of the country’s forest cover (Ahononga et al., 2020). Like many West African countries, Benin highly depends on energy, with 100% of fossil fuels and 90% of power imported from Nigeria and Ghana (Yatokpa et al., 2010). Yet, only a small percentage (6.7%) of rural households have access to electricity (Yatokpa et al., 2010) although a huge amount of crop and wood residues is available. The survey area covered eight representative localities (two in the north and six in the south) in terms of forestry residues production in Benin Republic (Figure 3).
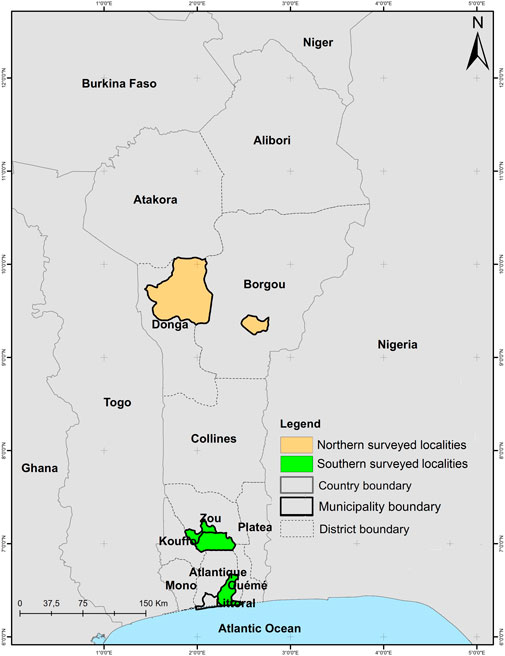
FIGURE 3. Map showing the surveyed localities in the Republic of Benin. Localities surveyed in the north are characterized by low competition for the use of forestry residues, low urbanization level, low economic development, low population density, low education level but high woody biomass availability, and high poverty level. In contrast, localities surveyed in the south are characterized by high competition for forestry residues, high urbanization level, high economic development, high population density, high education level, but low woody biomass availability, and low poverty level.
2.4 Data collection
2.4.1 Questionnaire design
We first drafted a questionnaire composed of 63 items which were hypothesized to measure six constructs (attitude, SN, PBC, EC, KRE, and intention). The questionnaire also included two key socioeconomic variables (age and income). These items were generated using a 5-point Likert scale (Supplementry Table SA1). To ensure that our items meet the key principles for successful item writing (Saville and MacIver, 2017), the questionnaire was first sent to an expert panel for the review of the selected items. Subsequently, a pilot survey was conducted by administering the questionnaire to a sample of 20 respondents. This pilot survey was relevant to test the quality of the questionnaire and to redefine items that were not comprehensible, ambiguous, or asked more than a single question (Streiner et al., 2015). The updated questionnaire was entered on a smart phone using KoBo Toolbox, a digital tool recommended for survey data collection (Sherin et al., 2018).
2.4.2 Sampling and questionnaire administration
We conducted a cross-national survey by administering the KoBo questionnaire to a random sample of 247 participants. This sample size is appropriate for performing SEM (Wolf et al., 2013). Additionally, the sample size is close to the ideal size of 300 recommended by various scholars (Comrey and Lee, 2013; Kyriazos and Stalikas, 2018). We conducted the survey in eight localities where forestry residues are abundantly generated (logging and wood processing residues, stumps) across the country. These localities were selected by interviewing the head of the national office of wood (https://cadredevie.gouv.bj/structure/onab/). The localities were selected based on the abundance of wood processing industries and short rotation plantations in the area. In each selected locality, we randomly administered the questionnaire to residues producers (sawmill and plantation owners), sellers (bulk sellers and detailers), and consumers (households, poultry farmers, bakers, and restaurant owners) representing respectively 51.82%, 17% and 31.17% of the surveyed participants. Data recorded on each participant included sociodemographic traits (age, sex, occupation, education level, and income) and the item score responses.
2.5 Statistical analysis
2.5.1 Factor analysis
First, we ran a factor analysis (FA) using the “factanal” function with varimax rotation in R 4.1.0 (R Core Team, 2021) to find out the groups of items and their underlying common factors from the initial pool of 63 items. Given that six latent variables were considered in our TPB model, we assumed that a six-common factor model was sufficient to reduce the number of items in the dataset. This assumption was tested in R using the chi-square fit statistic. The six-factor model is validated when the probability (p) of the chi-square fit statistic is greater than 0.5 (p ≥ 0.5). Based on the first FA output, we ran a second FA using items that are highly intercorrelated (Pearson r2 > 0.20) and showed a loading greater than 0.60 on each common factor (Kyriazos, 2018). We ran this second FA with the expectation that each common factor would display a minimum of three items of high quality (Streiner et al., 2015; DeVellis and Thorpe, 2021). We measured the internal reliability of each common factor using Cronbach’s alpha index (Cronbach, 1951). The Cronbach’s alpha was computed in R using the package “scales” (Wickham and Seidel, 2020). An alpha value greater than 0.70 indicates that the scale is reliable to measure the construct (Kyriazos, 2018). The Kaiser-Meyer-Olkin’s (KMO) and the Bartlett’s test of sphericity (Bartlett, 1951) were also computed to evaluate the quality of the FA. Bartlett’s test of sphericity was performed using the “RedaS” package (Maier, 2015). The desirable KMO for the whole data should be greater than 0.5 (Kyriazos, 2018). The FA was performed using the data from 247 informants without any missed values.
2.5.2 Confirmatory factor analysis and structural equation modeling
A confirmatory factor analysis (CFA) was performed to validate the common factor model. CFA is a theory-driven technique that seeks to test whether the theoretical relationships between the items and underlying factors are reliable. Technically, it is about comparing a population covariance matrix with the observed covariance matrix (Schreiber et al., 2006). We applied the diagonally weighted least squares (WLSMV) method to estimate CFA parameters (Li, 2016). The CFA was performed using the package “lavaan” (Rosseel, 2012) in R.
After construct validation, we performed a SEM using the lavaan package to test the structural relationship between the variables. We first performed the SEM for each geographical region separately and next run it again using pooled data from the two regions. In the SEM, the measurement model was simply the well-fit CFA model. In the regression part of the SEM, we tested the effect of attitude, SN, PBC, KRE, and EC on intentions and the effect of KRE and EC on attitude. We also included the mediation effect of KRE and EC through attitude. Like in CFA, we used the diagonally weighted least squares (WLSMV) method for estimating the model parameters. We computed the R2 of each regression model to appreciate the model explanatory power. The convergence of the CFA and SEM models was verified based on the standards recommended by Hu and Bentler (1999) and Brown (2005): RMSEA (≤0.06, 90% CI ≤ 0.06), SRMR (≤0.08), CFI (≥0.95), TLI (≥0.96), and the chi-square/df ratio (less than 3) (Kline, 2016).
3 Results
3.1 Sociodemographic characteristics of respondents
The surveyed respondents were on average 43 years old (40 in the north to 45 in the south). The majority were men (84.61%) and belonged to the Fon ethnic group (66.80%), the dominant ethnic group across the country. In the northern region, approximately 17% of the survey respondents were women, compared to 83% of men. Similarly, 14% of respondents were women in the south, compared to 86% of men. Approximately 49.79% (half) and 29.95% of the respondents reached secondary and primary school, respectively. Only 5.26% of them reached university, and 14.98% did not have any formal education. There were more respondents with no formal education in the south (50.20%) than in the north (34.82%). The monthly income of respondents was 53510.16 CFA on average. This income is lower in the north (48,674.53 CFA) compared to the south (57,171.43 CFA).
3.2 FA output and descriptive statistics of reliable items
In line with our assumption, a six-factor model was sufficient to reduce the number of variables in our dataset (chi-square fit statistic = 91, p = 0.7). The six factors accounted for 76.3% of the whole variance in the dataset (Table 1). We got a reliable KMO (0.87) indicating that our FA model is appropriate. The Bartlett’s test was also significant (chi-square = 7282.738, DF = 595, p < 0.05). The value of the Cronbach alpha (α) was reliable and acceptable for all the common factors (α = 0.60–0.96).
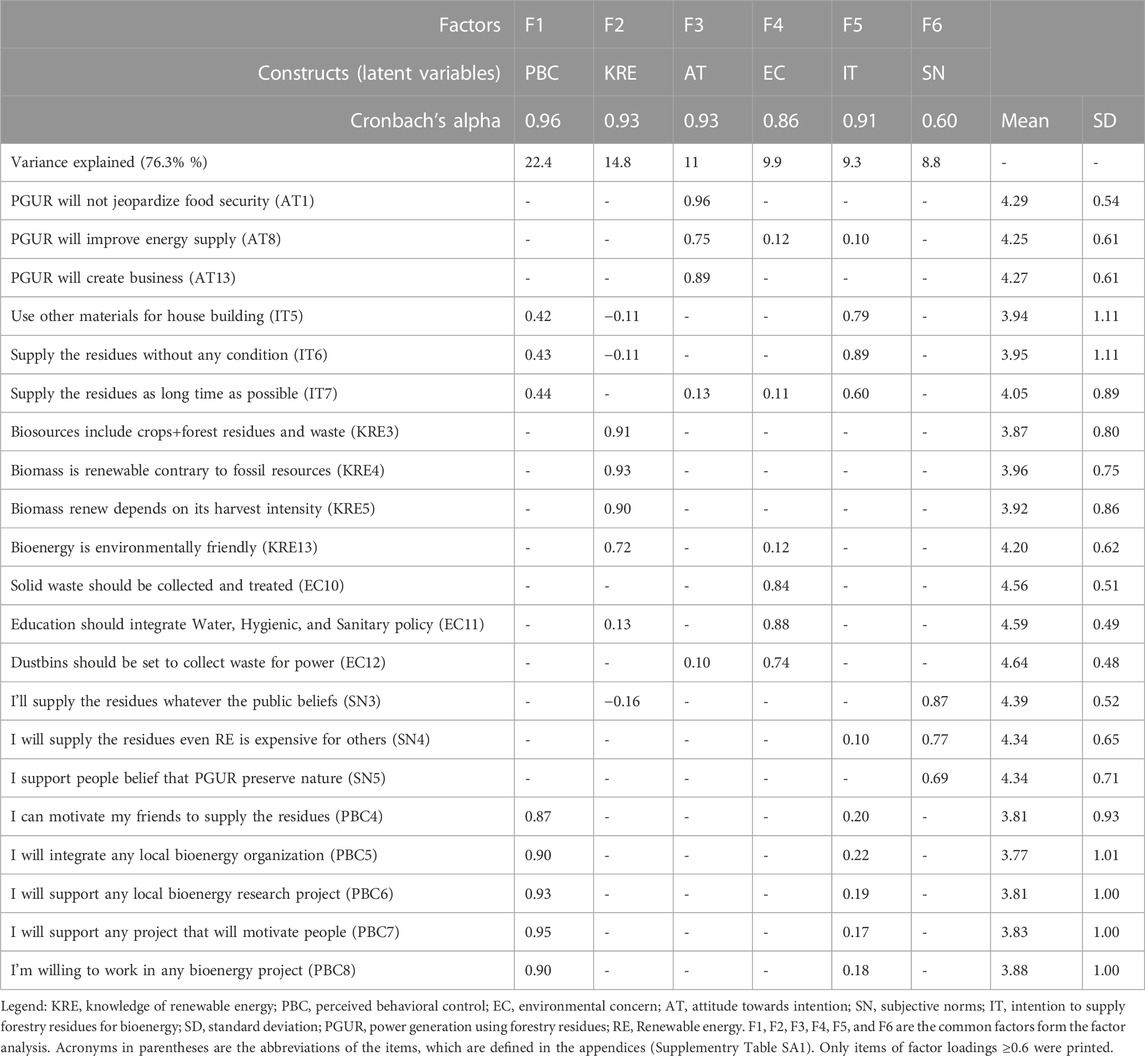
TABLE 1. Factor loadings from factor analysis (FA), Cronbach’s alpha and descriptive statistics of high loading items.
The first factor (F1) accounts for 22.4% of the variance in our data and is strongly related to PBC items (Table 1). Factor 2 (F2) explained 14.8% of the total variance and is strongly related to KRE items. Factor 3 (F3) explained 11% of the total variance and underlined attitude items. Factors 4 (F4) explained 9.9% of the total variance and is linked to EC items. Factor 5 (F5) explained 9.3% of the total variance and reflects the items that underlie the intention to supply woody residue. Factor 6 (F6) explained 8.8% of the variance and is strongly related to SN.
The descriptive analysis showed that respondents had favorable opinions about the valorization of forestry residues for bioenergy purposes. Overall, all the constructs’ items received high scores from the respondents (3.77 ± 1.01 to 4.64 ± 0.48, Table 1). With regard to the attitude construct, respondents mostly had strong beliefs that the use of residues for power or gas production will improve food security (4.29 ± 0.54), the quantity of energy supplied (4.25 ± 0.61) and business opportunities for young people (4.27 ± 0.61). Considering the construct on intentions, respondents mostly accepted to use alternate materials for building houses and supply the residues for bioenergy projects (3.94 ± 1.11). Additionally, they strongly agreed to supply the residues without any conditions (3.95 ± 1.11) and supply them for as long as possible (4.05 ± 0.89). Respondents’ knowledge about biomass and bioenergy was also acceptable. Respondents’ understanding of biomass and bioenergy concepts was also acceptable (3.87 ± 0.80). They mostly accepted that bioenergy is environmentally friendly (4.20 ± 0.62) and its sources are renewable, contrary to fossil sources (3.96 ± 0.75). They also supported the idea that the renewability of bioenergy sources will depend on their harvest intensity. Respondents strongly cared more about the environment. They strongly supported solid waste recycling (4.56 ± 0.51), the consideration of Water, Sanitary, and Hygienic (WASH) policy by the education system (4.59 ± 0.49) and waste mobilization for power generation (4.64 ± 0.48). In terms of normative beliefs, they strongly accepted to supply the residues independently of public opinions (4.34 ± 0.65 to 4.39 ± 0.52) and complied with the public opinions that using residues for bioenergy purposes will preserve nature (4.34 ± 0.71). Respondents manifested acceptable control beliefs. They showed such strong self-reliance that they could motivate their friends (3.81 ± 0.93) and support any bioenergy projects in terms of organization, research, and sensitization (3.77 ± 1.01 to 3.88 ± 1.00).
3.3 Goodness-of-fit of CFA and SEM models
All the comparative fit index values for the CFA and SEM were acceptable and showed a good fit. The CFI and TLI were 1 (>0.95) and 1.01 (>0.96) respectively. The RMSEA value (0.00) was under the recommended threshold (0.06) as were the SRMR (0.05 < 0.09) and the chi-square/df ratio (1.37 < 2). The Squared Multiple Correlation (SMC) ranged from 0.43 to 0.90 (Table 2), indicating a high association between items and latent variables.
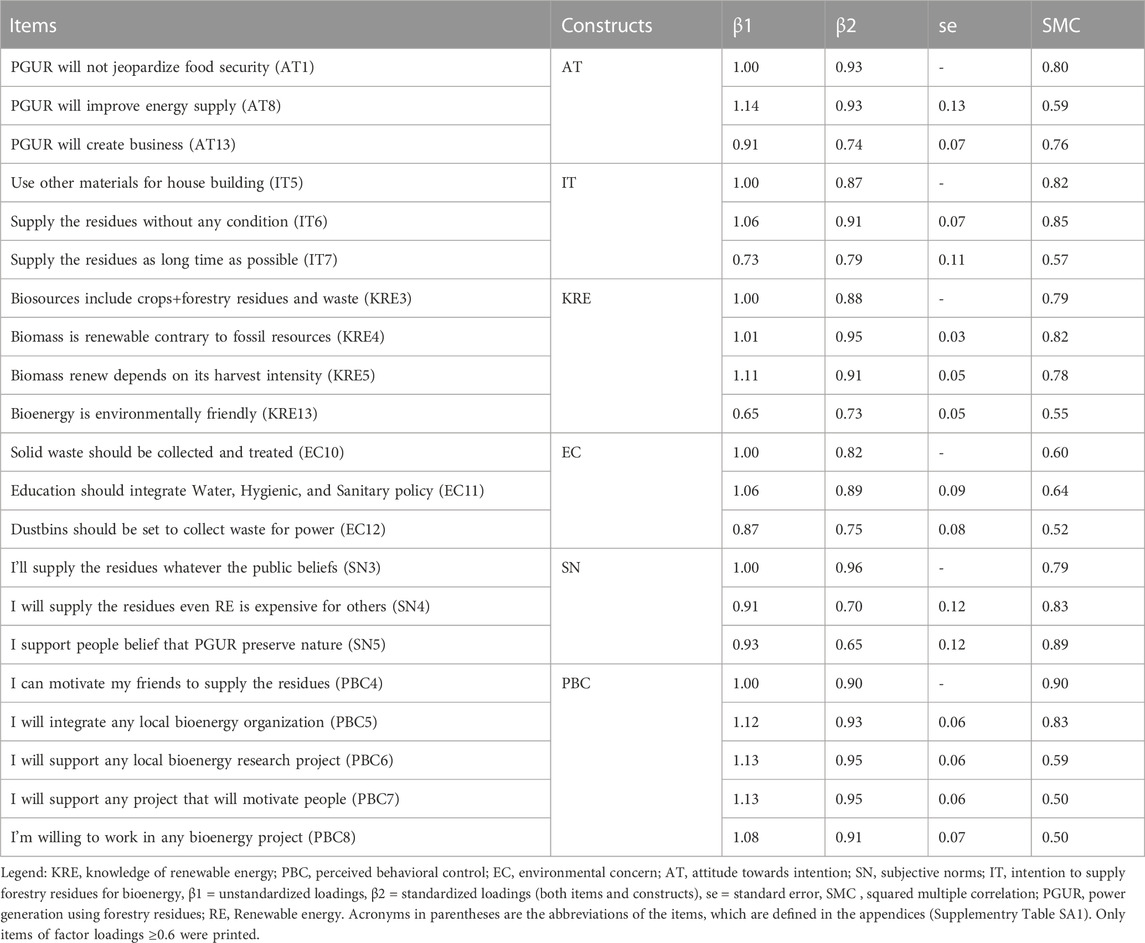
TABLE 2. Standardized and unstandardized coefficients form the confirmatory factor analysis, item variance explained by the construct and squared multiple correlation values.
3.4 Determinants of the intentions to supply forestry residues for clean energy
When spatial disparities were ignored by aggregating the data from the two regions, we found significant correlations between intentions and several hypothesized predictor variables. All the model predictors explain 55% (R2 = 0.55, Figure 4) of the variance in respondents’ intentions. This value indicates the acceptable explanatory power of this model. Respondents’ intentions were positively correlated with attitude, PBC, and income (Figure 4; Supplementry Table SA2 in the Appendices). This outcome validates our first and second hypotheses (H1 and H2) that respondents with a more positive attitude towards supplying forestry residues, a higher PBC and a higher income are likely to have a higher intention to supply forestry residues. We surprisingly found a negative correlation between intentions and KRE (Figure 4; Supplementry Table SA2 in the Appendices) which contrast with our assumption that respondents who are more knowledgeable about renewable energy are likely to have a greater willingness to supply woody residues. The assumption that a stronger alignment with SN, a higher environmentally friendly, or being younger are positively associated with the intentions was not met. In fact, no significant correlation was found between intentions and SN, EC, and age (Figure 4; Supplementry Table SA2 in the Appendices). Interestingly, EC was positively correlated with attitude but not with KRE (Figure 4; Supplementry Table SA2). In terms of covariance, KRE and SN shared negative covariance, while the covariate relationship between age and income was positive (Figure 4).
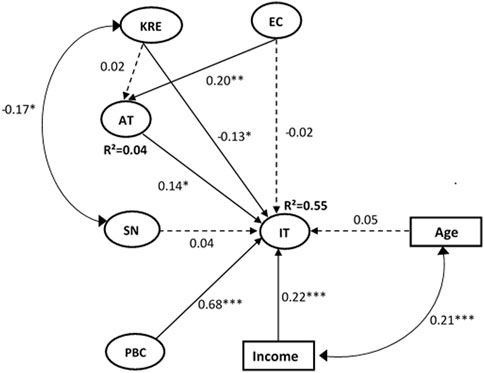
FIGURE 4. Results of the regression models. Solid black arrow means the relationship is significant (p < 0.05) and dash arrows indicate non-significant relationships (p ≥ 0.05). KRE, Knowledge of renewable energy; PBC, Perceived behavioral control; EC, Environmental concern; AT, Attitude towards intention; SN, Subjective norms; IT, Intention to supply forestry residues for bioenergy. R2 is the power of the regression model (the variation in IT or AT accounted by the predictors). The solid black arrow denotes a significant relationship, whereas the dash arrow indicates a non-significant relationship. Solid double arrow indicates a significant covariate relationship. Path coefficients (standardized values) followed by asterisks are significant: ***p < 0.001, **p < 0.01, *p < 0.05.
Considering the region-specific data, we found acceptable explanatory power of the model across the geographical regions, with higher fit in the north (R2 = 0.62) than in the south (R2 = 0.51). We found evidence of spatial variation in this study. KRE failed to determine intentions in the north but exhibited a positive association with intentions in the south (Figures 5A, B). Additionally, the KRE effect on attitude also demonstrated spatial variation, with a positive effect in the north (Figure 5A) and a negative effect in the south (Figure 5B). No spatial variation was found regarding the effects of PBC, EC, SN, attitude, income, and age on intentions. The intentions to supply the residues were positively predicted by PBC and income consistently across geographical regions (Figures 5A, B; Supplementry Table SA2 in the Appendices). The effects of EC, SN, and attitude were not significant across geographical regions. We also found spatial variation in the covariance between KRE and EC with a positive covariate link in the north (Figure 5A) and a negative link in the south (Figure 5B). Predictor variables like SN, KRE, and age had no significant effect on intentions across the spatial gradients (Figures 5A, B).
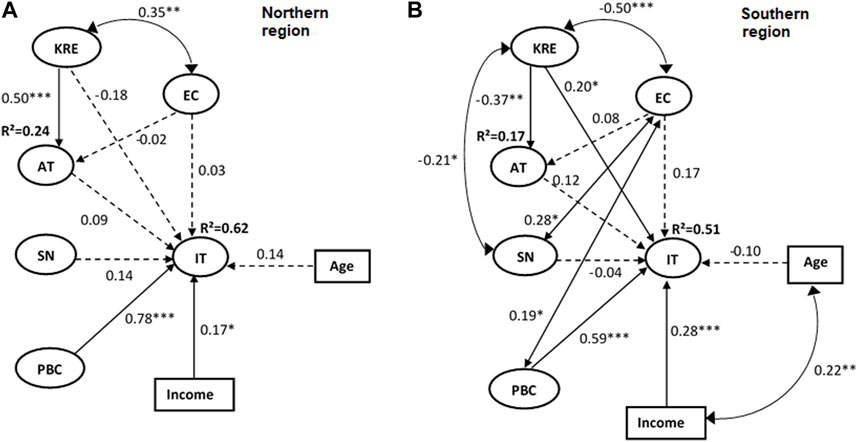
FIGURE 5. Results of structural equation modeling (SEM) showing the spatial variation in the intention predictors between (A) northern region and (B) southern region of the Republic of Benin. Solid black arrows indicate significant (p < 0.05) relationships and dash arrows indicate non-significant relationships (p ≥ 0.05). KRE = Knowledge of renewable energy, PBC, Perceived behavioral control; EC, Environmental concern; AT, Attitude towards intention; SN, Subjective norms; IT, Intention to supply forestry residues for bioenergy. R2 is the power of the regression model (the variation in IT or AT accounted for by the predictors). The solid black arrow denotes a significant relationship, whereas the dash arrow indicates a non-significant relationship. The solid double arrow indicates a significant covariate relationship. Path coefficients (standardized values) followed by asterisks are significant: ***p < 0.001, **p < 0.01, *p < 0.05.
Our findings validate our fourth hypothesis (H4) that the determinants of the intention to supply forestry residues for clean energy are influenced by spatial disparities.
Considering the mediation effects, EC and KRE had no indirect effect on intention through attitude (Table 3). This outcome rejects our third hypothesis (H3) that the effect of EC and KRE on the intention to supply the residues is mediated by attitude.
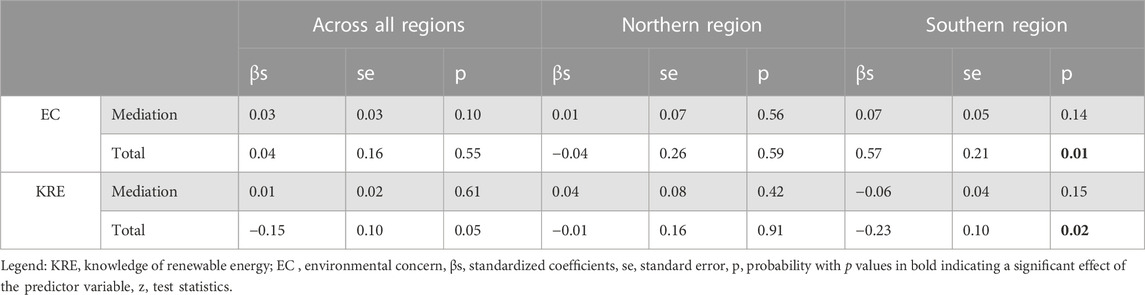
TABLE 3. Mediation and total effects of environmental concern and knowledge of renewable energy on the intention to supply forestry residues for clean energy through attitude towards the intention.
4 Discussion
This study applied the TPB to assess the intentions to supply forestry residues for bioenergy purposes (gas and electricity). We performed an expanded TPB model to identify the major determinants of intention and examined whether the model is shaped by spatial disparities. The R2 values (0.51–0.62) of all our TPB models were over the threshold value of 0.35 recommended (Anderson and Gerbing, 1988) indicating a strong explanatory power of the models and enables the interpretations of the outputs.
We first hypothesized that respondents with a more positive attitude, a stronger alignment with SN, and greater PBC, particularly, those who are environmentally friendly or have more KRE, are likely to have a higher intention to supply forestry residues for clean energy (H1).
Our results revealed that people’s intentions to supply the residues is positively predicted by attitude and PBC, while it had a negative and negligible association with KRE and SN, respectively. This finding partly supports our first hypothesis and is consistent with the predictions from the theory of planned behavior (Ajzen, 1991). The positive effect of attitude and PBC on intention was reported by many other studies in the literature (Halder et al., 2016; Adepoju and Akinwale, 2019; Jabeen et al., 2019). According to the behavioral attitude theory (Fishbein and Ajzen, 2010), attitude is considered as the first important variable in explaining people’s intentions. Attitude positively guides intentions when it is driven by experience (Radics et al., 2016). Because attitude and EC were positively correlated, we believe that EC might be the key driver of the positive effect of attitude on intention in this study. This translates the attitude improvement role of EC towards the social support of bioenergy. This finding aligns with others studies in the literature (Liobikienė et al., 2021) and suggests that raising people’s awareness about the environment would increase their intention to supply residues for bioenergy projects.
The positive relationship between PBC and intention aligns with the prediction from the theory of planned behavior (Ajzen, 1991) and demonstrates stakeholders’ self-reliance to supply the residues for bioenergy projects. Indeed, our data show that most respondents are strongly confident that public beliefs will not divert their opinions to supply the residues. They also showed strong willingness to support renewable energy initiatives by motivating their relevant peers, participating in renewable energy projects, and substituting residues for alternative sources. This proves their strong ability to secure their intentions and focus on opportunities that may make renewable energy projects successful. Contrary to our expectation in the first hypothesis, KRE negatively relates to intention. This is probably because an increase in KRE might raise people awareness of the limitations of bioenergy, such as its purchase price, potential impact on food and land use (Nazir and Tian, 2022; Asif et al., 2023).
The correlation between SN and intention was not significant, contrary to our expectation in the first hypothesis. This finding translates the non-significance of social pressure (friends and family pressure) on intention. Additionally, this finding supports the prediction from the theory of planned behavior (Ajzen, 1971) that perceived societal opinions may lead or not to behavioral performance. SN played an insignificant role in this study, probably because respondents are indifferent to the opinions of their relatives. Based on the theory of Maslow (Maslow, 1943), this may be explained by the fact that most of the respondents have fundamental needs rather than higher-level needs and, they, will not intentionally follow social consent or support. Therefore, social pressures will have limited influence on respondents’ decisions to supply the residues. Another reason could be that the concept of renewable energy is only minimally incorporated into educational curriculums and has yet to gain cultural acceptance throughout the country. Our finding is similar to that of previous studies (Bang et al., 2000; Jiang et al., 2018; Kowalska-Pyzalska, 2018; Lopes et al., 2019). However, significant links were reported in several studies (Saleh et al., 2014; Halder et al., 2016; Jabeen et al., 2019; Ashinze et al., 2021; Mäntymaa et al., 2023).
We hypothesized that demographic characteristics, such as having a higher income or being younger, are positively associated with the intention to supply forestry residues for clean energy (H2). Our results confirmed the expectation of a positive effect of income but failed to support the effect of age. The most plausible explanation is that respondents with higher incomes can purchase alternative materials as a substitute for the residues. As reported by Rezaei and Ghofranfarid (2018), higher financial means tend to raise rural households’ willingness to support renewable energy projects. Similar finding was also previously reported (Karanja and Gasparatos, 2019; Nigussie et al., 2021; Okereke et al., 2023). The non-significant correlation between intention and age contrasts with the common opinion that formal education and outdoor environmental activities can catalyze the willingness to support bioenergy projects at a young age (Ashinze et al., 2021). The relationship between intention and age appeared to be neutral, likely due to the limited exposure to renewable energy concepts in educational institutions, as well as the infrequent discussions about them in home settings. This finding is supported by the observations of François et al. (2023), who revealed no correlation between respondents’ age and their willingness to use biogas based on human excreta.
We examined whether the determinants of the intention to supply the residues exhibited any spatial variation. We hypothesized that intention predictors are affected by spatial disparities (H4). We found a shift in the effect of KRE between the two regions, but spatial disparities did not impact the other variables. KRE exhibits a null effect in the north but a positive effect in the south. Additionally, KRE significantly influenced attitudes, but this effect shifted from positive in the north to negative in the south. We assumed that the higher education level in the south was the main reason for this spatial variation. In theory, education can raise people’s awareness about the benefits of using bioenergy (Karytsas and Theodoropoulou, 2014; Van Dael et al., 2017) which might increase their intentions to support bioenergy projects. Education can also increase communities KRE by providing them with in-depth knowledge on renewable bioenergy, including renewable types, properties, and potential uses, which may increase people’s confidence in bioenergy as a viable alternative to traditional energy sources (Karanja and Gasparatos, 2019). The spatial variation of the KRE effect on attitude may be associated with disparities in geographical characteristics between regions. The negative influence of KRE on attitudes in the south may result from this region’s higher economic potential, which leads to higher competition for forestry residues for various uses compared to the north.
Spatial disparities did not impact the effect of PBC and income, probably because respondents shared similar socioeconomic and psychological values between the two regions (Skevas et al., 2018). The positive effect of income underlines the importance of raising people’s financial capacity for a sustainable adoption of bioenergy (Nigussie et al., 2021). This emphasizes the major role of incentives to make bioenergy projects more financially viable (Nayar, 2016; Yu et al., 2020). The consistent and positive effect of PBC across regions may be the result of respondents self-efficacy, which would raise their ability to overcome any hindrances or challenges in supplying the residues for bioenergy (Alam et al., 2014; Yu et al., 2020). Also, social support and encouragement from close relatives (friends, family, and communities) and bioenergy projects might increase respondents’ perceived control, which would have raised their intentions to supply the residues (Pagiaslis and Krontalis, 2014; Xie and Zhao, 2018; Karasmanaki and Tsantopoulos, 2019; Liobikienė et al., 2021). It may also be driven by EC, as the two variables share positive covariance. Indeed, several authors reported that people who are environmentally concerned may feel a moral obligation to support bioenergy projects (Pagiaslis and Krontalis, 2014; Xie and Zhao, 2018; Karasmanaki and Tsantopoulos, 2019; Liobikienė et al., 2021) which may increase their perceived control.
5 Conclusion and policy implications
We employed SEM based on the TPB to assess the determinants of the intentions to supply forestry residues for bioenergy and test the impact of spatial variation on the determinants of the intentions to supply residues for bioenergy. Our findings trigger important conclusions. The first is that when spatial variation is ignored, the intentions to supply residues is determined by attitude, income, PBC, and KRE. The second is that when spatial variation is considered, we found a shift in KRE effect between regions, while PBC and income exhibited a positive effect regardless of regions.
Our study outcome has important policy implications. The consistent effect of income implies that policymakers should provide incentives to encourage citizens’ involvement in bioenergy projects. This will raise people’s control beliefs and subsequently, their willingness to supply the residues for bioenergy. Policymakers are invited to prioritize the northern region of Benin Republic while providing incentives because of the higher poverty rates in this region that may hinder the adoption of renewable energy due to cost barriers (Aly et al., 2019; Xu et al., 2022). Policymakers should also offer loans and generate employment opportunities to raise citizens’ income and enhance their control beliefs. Additionally, the positive covariance between EC and PBC implies that raising people’s environmental awareness can increase their control beliefs and their intentions to supply the residues. The spatial disparities impact on the KRE effect is relevant empirical information that can be used by policymakers to tailor actions to each geographical region. For example, policymakers can change people’s attitude in the north through KRE improvement actions. They can also raise residues supply intentions in the south by improving people’s KRE in the south. The success of these actions requires the incorporation of renewable energy into educational curriculums and awareness campaigns through media, and workshops. Another implication is that studies on biomass supply intentions for bioenergy should consider the spatial drivers of variation in intentions to make accurate inferences. This will enable more targeted bioenergy policies and expedite the transition towards pro-environmental behavior and renewable energy adoption. Overall, by addressing these policy domains related to education, incentives, and research, the promotion and adoption of sustainable bioenergy projects can be effectively facilitated, contributing to a cleaner and more sustainable energy future.
Data availability statement
The original contributions presented in the study are included in the article/Supplementary Material, further inquiries can be directed to the corresponding author.
Ethics statement
The studies involving humans were approved by the Research Planning and Value Creation of Research Outcomes; University of Parakou, Benin; Approved by Professor Mohamed Nasser BACO, Email: dmplYW5ib3Njb0BnbWFpbC5jb20=, Tel: +229 97 93 00 87. The studies were conducted in accordance with the local legislation and institutional requirements. The participants provided their written informed consent to participate in this study.
Author contributions
SB and RB conceived the ideas of this study and designed the methodology. RB collected the data and conducted the statistical analysis with assistance from EA, IM-M, and AS. RB drafted the first version of the manuscript. All authors contributed to the article and approved the submitted version.
Funding
This study was supported by International Foundation for Science (grant number D/6155-1) and my research unit UR-BioME.
Acknowledgments
We are grateful to Nicaise K. M. Oguidi and Issa Amadou for field assistance, Enagnon B. Olivier Ahouandjinou, and Florent Noulekoun for providing valuable comments on the manuscript.
Conflict of interest
The authors declare that the research was conducted in the absence of any commercial or financial relationships that could be construed as a potential conflict of interest.
Publisher’s note
All claims expressed in this article are solely those of the authors and do not necessarily represent those of their affiliated organizations, or those of the publisher, the editors and the reviewers. Any product that may be evaluated in this article, or claim that may be made by its manufacturer, is not guaranteed or endorsed by the publisher.
Supplementary material
The Supplementary Material for this article can be found online at: https://www.frontiersin.org/articles/10.3389/fenrg.2023.1239710/full#supplementary-material
Abbreviations
KRE, Knowledge of renewable energy; EC, Environmental concern; PBC, Perceived behavioral control; SN, Subjective norms.
References
Adepoju, A. O., and Akinwale, Y. O. (2019). Factors influencing willingness to adopt renewable energy technologies among micro and small enterprises in Lagos State Nigeria. Int. J. Sustain. Energy Plan. Manag. 19, 69–82. doi:10.5278/ijsepm.2019.19.7
Adomou, A. C. (2005). Vegetation patterns and environmental gradients in Benin: implications for biogeography and conservation. PhD thesis. Wageningen, Netherland: Wageningen University.
Agbandji, L., Behanzin, P., Dossou, G., and Saïnou, J. (2020). Déterminants de la demande résidentielle de l’énergie électrique au Bénin: une étude empirique Repères. Perspect. Econ. 4 (2), 126. doi:10.34874/IMIST.PRSM/RPE/21647
Ahononga, F. C., Gouwakinnou, N. G., Biaou, S., and Biaou, S. (2020). Vulnérabilité des terres des écosystèmes du domaine soudanien au Bénin de 1995 à 2015. Bois For. Trop. 346, 35–50. doi:10.19182/bft2020.346.a36295
Ajzen, I. (1971). Attitudinal vs. Normative messages: an investigation of the differential effects of persuasive communications on behavior. Sociometry 34, 263–280. doi:10.2307/2786416
Ajzen, I. (1991). The theory of planned behavior. Organ. Behav. Hum. Decis. process. 50, 179–211. doi:10.1016/0749-5978(91)90020-t
Ajzen, I., and Fishbein, M. (1975). A Bayesian analysis of attribution processes. Psychol. Bull. 82, 261–277. doi:10.1037/h0076477
Akoègninou, A., Van der Burg, W. J., and Van der Maesen, L. J. G. (2006). Flore analytique du Bénin. Leiden, Netherlands: Backhuys Publishers.
Alam, S. S., Nik Hashim, N. H., Rashid, M., Omar, N. A., Ahsan, N., and Ismail, M. D. (2014). Small-scale households renewable energy usage intention: theoretical development and empirical settings. Renew. Energy 68, 255–263. doi:10.1016/j.renene.2014.02.010
Ali, S., Yan, Q., Irfan, M., and Fahad, S. (2023). Relating biogas technology and environmental impact assessment: a roadmap towards clean energy for environmental sustainability. Environ. Sci. Pollut. Res. 30, 72832–72853. doi:10.1007/s11356-023-27553-6
Al-Mulali, U., Ozturk, I., and Solarin, S. A. (2016). Investigating the environmental Kuznets curve hypothesis in seven regions: the role of renewable energy. Ecol. Indic. 67, 267–282. doi:10.1016/j.ecolind.2016.02.059
Aly, A., Moner-Girona, M., Szabó, S., Pedersen, A. B., and Jensen, S. S. (2019). Barriers to large-scale solar power in Tanzania. Energy sustain. Dev. 48, 43–58. doi:10.1016/j.esd.2018.10.009
Anderson, J. C., and Gerbing, D. W. (1988). Structural equation modeling in practice: a review and recommended two-step approach. Psychol. Bull. 103, 411–423. doi:10.1037/0033-2909.103.3.411
Ashinze, P. C., Tian, J., Ashinze, P. C., Nazir, M., and Shaheen, I. (2021). A multidimensional model of sustainable renewable energy linking purchase intentions, attitude and user behavior in Nigeria. Sustainability 13, 10576. doi:10.3390/su131910576
Asif, M. H., Zhongfu, T., Ahmad, B., Irfan, M., Razzaq, A., and Ameer, W. (2023). Influencing factors of consumers' buying intention of solar energy: a structural equation modeling approach. Environ. Sci. Pollut. Res. Int. 30 (11), 30017–30032. doi:10.1007/s11356-022-24286-w
Baležentis, T., Streimikiene, D., Zhang, T., and Liobikiene, G. (2019). The role of bioenergy in greenhouse gas emission reduction in EU countries: an Environmental Kuznets Curve modelling. Resour. Conserv. Recycl. 142, 225–231. doi:10.1016/j.resconrec.2018.12.019
Bang, H.-K., Ellinger, A. E., Hadjimarcou, J., and Traichal, P. A. (2000). Consumer concern, knowledge, belief, and attitude toward renewable energy: an application of the reasoned action theory. Psychol. Mark. 17, 449–468. doi:10.1002/(sici)1520-6793(200006)17:6<449::aid-mar2>3.0.co;2-8
Bartlett, M. S. (1951). The effect of standardization on a χ 2 approximation in factor analysis. Biometrika 38, 337–344. doi:10.2307/2332580
Biaou, S., Gouwakinnou, G. N., Biaou, H. S. S., Tovihessi, M. S., Awessou, B. K., Ahononga, F. C., et al. (2021). Identifying the land use and land cover change drivers: methods and case studies of two forest reserves in Northern Benin. Environ. Dev. Sustain. 24, 9885–9905. doi:10.1007/s10668-021-01849-4
Brown, D. G., Johnson, K. M., Loveland, T. R., and Theobald, D. M. (2005). Rural land-use trends in the conterminous United States, 1950-2000. Ecol. Appl. 15, 1851–1863. doi:10.1890/03-5220
Brunson, M. W., and Shindler, B. A. (2004). Geographic variation in social acceptability of wildland fuels management in the western United States. Soc. Nat. Resour. 17, 661–678. doi:10.1080/08941920490480688
Collins, S. E., and Carey, K. B. (2007). The theory of planned behavior as a model of heavy episodic drinking among college students. Psychol. Addict. Behav. 21, 498–507. doi:10.1037/0893-164X.21.4.498
Comrey, A. L., and Lee, H. B. (2013). A first course in factor analysis. Hillsdale, NJ: Lawrence Erlbaum: Psychology Press.
Cronbach, L. J. (1951). Coefficient alpha and the internal structure of tests. psychometrika 16, 297–334. doi:10.1007/bf02310555
Dagiliūtė, R. (2023). Influence of negative and positive perceptions about renewable energy on intention to use bio—and other renewable energy sources. Environ. Dev. Sustain. doi:10.1007/s10668-022-02731-7
DeVellis, R. F., and Thorpe, C. T. (2021). Scale development: theory and applications. 4th Edn. SAGE
Fishbein, M., and Ajzen, I. (2010). Predicting and changing behavior: the reasoned action approach. New York, NY: Psychology Press.
François, M., Lin, K. S., Vaincoeur, E., Rachmadona, N., and Khoo, K. S. (2023). Haitians’ perceptions of biogas produced via human excreta: an approach to the democratization of energy systems. Chemosphere 334, 138986. doi:10.1016/j.chemosphere.2023.138986
Halder, A., Kandambeth, S., Biswal, B. P., Kaur, G., Roy, N. C., Addicoat, M., et al. (2016). Decoding the morphological diversity in two dimensional crystalline porous polymers by Core planarity modulation. Angew. Chem. Int. Ed. 55, 7806–7810. doi:10.1002/anie.201600087
He, K., Ye, L., Li, F., Chang, H., Wang, A., Luo, S., et al. (2022). Using cognition and risk to explain the intention-behavior gap on bioenergy production: based on machine learning logistic regression method. Energy Econ. 108, 105885. doi:10.1016/j.eneco.2022.105885
Holloway, G., Lapar, M., and Lucila, A. (2007). How big is your neighbourhood? Spatial implications of market participation among Filipino smallholders. J. Agric. Econ. 58, 37–60. doi:10.1111/j.1477-9552.2007.00077.x
Hu, L., and Bentler, P. M. (1999). Cutoff criteria for fit indexes in covariance structure analysis: conventional criteria versus new alternatives. Struct. Equ. Model. Multidiscip. J. 6, 1–55. doi:10.1080/10705519909540118
INSAE and ICF (2019). République Du Bénin Ciquième Enquête Démographique et de Santé au Bénin (EDSB-V) 2017-2018.
Jabeen, G., Yan, Q., Ahmad, M., Fatima, N., and Qamar, S. (2019). Consumers’ intention-based influence factors of renewable power generation technology utilization: a structural equation modeling approach. J. Clean. Prod. 237, 117737. doi:10.1016/j.jclepro.2019.117737
Jiang, X.-L., An, M., Zheng, S.-S., Deng, M., and Su, Z.-H. (2018). Geographical isolation and environmental heterogeneity contribute to the spatial genetic patterns of Quercus kerrii (Fagaceae). Heredity 120, 219–233. doi:10.1038/s41437-017-0012-7
Joshi, O., and Mehmood, S. R. (2011). Factors affecting nonindustrial private forest landowners’ willingness to supply woody biomass for bioenergy. Biomass Bioenergy 35, 186–192. doi:10.1016/j.biombioe.2010.08.016
Kabir, Z. (2021). “Chapter 12 - social, economic, and environmental aspects of bioenergy resources,” in Bioenergy resources and technologies. Editors A. K. Azad, and M. M. K. Khan (Academic Press), 349–381. doi:10.1016/B978-0-12-822525-7.00003-2
Kanbur, R., and Wan, G. (2006). “Spatial disparities in human development: an overview of the Asian evidence” in Spatial Disparities in Human Development: Perspectives from Asia, Editor R. Kanbur, A. J. Venebles, and G. Wan (Tokyo-New York-Paris: United Nations University Press), 1–6.
Karanja, A., and Gasparatos, A. (2019). Adoption and impacts of clean bioenergy cookstoves in Kenya. Renew. Sustain. Energy Rev. 102, 285–306. doi:10.1016/j.rser.2018.12.006
Karasmanaki, E., and Tsantopoulos, G. (2019). Exploring future scientists’ awareness about and attitudes towards renewable energy sources. Energy Policy 131, 111–119. doi:10.1016/j.enpol.2019.04.032
Karytsas, S., and Theodoropoulou, H. (2014). Socioeconomic and demographic factors that influence publics’ awareness on the different forms of renewable energy sources. Renew. Energy 71, 480–485. doi:10.1016/j.renene.2014.05.059
Khanna, M., Chen, L., Basso, B., Cai, X., Field, J. L., Guan, K., et al. (2021). Redefining marginal land for bioenergy crop production. GCB Bioenergy 13 (10), 1590–1609. doi:10.1111/GCBB.12877
Kline, R. B. (2016). in Principles and practice of structural equation modeling. Editor T. D. Little Fourth.
Kowalska-Pyzalska, A. (2018). An empirical analysis of green electricity adoption among residential consumers in Poland. Sustainability 10, 2281. doi:10.3390/su10072281
Kyriazos, T. A. (2018). Applied psychometrics: sample size and sample power considerations in factor analysis (EFA, CFA) and SEM in general. Psychology 9, 2207–2230. doi:10.4236/psych.2018.98126
Kyriazos, T. A., and Stalikas, A. (2018). Applied psychometrics: the steps of scale development and standardization process. Psychology 9, 2531–2560. doi:10.4236/psych.2018.911145
Lai, I. K. W., Liu, Y., Sun, X., Zhang, H., and Xu, W. (2015). Factors influencing the behavioural intention towards full electric vehicles: an empirical study in Macau. Sustainability 7, 12564–12585. doi:10.3390/su70912564
Leitch, Z. J., Lhotka, J. M., Stainback, G. A., and Stringer, J. W. (2013). Private landowner intent to supply woody feedstock for bioenergy production. Biomass Bioenergy 56, 127–136. doi:10.1016/j.biombioe.2013.04.017
Li, C.-H. (2016). The performance of ML, DWLS, and ULS estimation with robust corrections in structural equation models with ordinal variables. Psychol. Methods 21, 369–387. doi:10.1037/met0000093
Li, S., Peng, S., Jin, B., Zhou, J., and Li, Y. X. (2019). Multi-scale relationship between land use/land cover types and water quality in different pollution source areas in Fuxian Lake Basin. PeerJ 7, e7283. Available at:. doi:10.7717/peerj.7283
Liobikienė, G., Dagiliūtė, R., and Juknys, R. (2021). The determinants of renewable energy usage intentions using theory of planned behaviour approach. Renew. Energy 170, 587–594. doi:10.1016/j.renene.2021.01.152
Liu, W., Wang, C., and Mol, A. P. (2013). Rural public acceptance of renewable energy deployment: the case of Shandong in China. Appl. Energy 102, 1187–1196. doi:10.1016/j.apenergy.2012.06.057
Loera, B., Murphy, B., Fedi, A., Martini, M., Tecco, N., and Dean, M. (2022). Understanding the purchase intentions for organic vegetables across EU: a proposal to extend the TPB model. Br. Food J. 124 (12), 4736–4754. doi:10.1108/bfj-08-2021-0875
Lopes, J. R. N., Kalid, R. de A., Rodríguez, J. L. M., and Ávila Filho, S. (2019). A new model for assessing industrial worker behavior regarding energy saving considering the theory of planned behavior, norm activation model and human reliability. Resour. Conserv. Recycl. 145, 268–278. doi:10.1016/j.resconrec.2019.02.042
Maier, M. J. (2015). REdaS: companion package to the book “R: einführung durch angewandte Statistik.”.
Mäntymaa, E., Kaseva, J., Hiedanpää, J., and Pouta, E. (2023). Residents’ interest in landscape value trade related to wind energy: application of the attitude–behavior framework to willingness to pay. Ecosyst. People 19 (1), 2212797. doi:10.1080/26395916.2023.2212797
Miller, H. J. (2004). Tobler’s first law and spatial analysis. Ann. Assoc. Am. Geogr. 94, 284–289. doi:10.1111/j.1467-8306.2004.09402005.x
Nazir, M., and Tian, J. (2022). The influence of consumers’ purchase intention factors on willingness to pay for renewable energy; mediating effect of attitude. Front. Energy Res. 10, 1–13. doi:10.3389/fenrg.2022.837007
Nigussie, Z., Tsunekawa, A., Haregeweyn, N., Tsubo, M., Adgo, E., Ayalew, Z., et al. (2021). Small-scale woodlot growers’ interest in participating in bioenergy market in rural Ethiopia. Environ. Manage. 68, 553–565. doi:10.1007/s00267-021-01524-4
Okereke, C., Onyeneke, R. U., Ijeoma, S., Fadero, T., Ahanotu, K., and Anieze, E. E. (2023). Attitude, knowledge and perception of choice of cooking fuels: evidence from two large communities in South-east Nigeria. Environ. Prog. Sustain. Energy 42 (1), e13983. doi:10.1002/ep.13983
Pagiaslis, A., and Krontalis, A. K. (2014). Green consumption behavior antecedents: environmental concern, knowledge, and beliefs. Psychol. Mark. 31, 335–348. doi:10.1002/mar.20698
Radics, R. I., Dasmohapatra, S., and Kelley, S. S. (2016). Public perception of bioenergy in North Carolina and Tennessee. Energy sustain. Soc. 6, 17. doi:10.1186/s13705-016-0081-0
R Core Team (2021). R: a language and environment for statistical computing. Vienna, Austria: R Foundation for Statistical Computing. URL https://www.R-project.org/.
Rezaei, R., and Ghofranfarid, M. (2018). Rural households’ renewable energy usage intention in Iran: extending the unified theory of acceptance and use of technology. Renew. Energy 122, 382–391. doi:10.1016/j.renene.2018.02.011
Rosseel, Y. (2012). lavaan: an R package for structural equation modeling. J. Stat. Softw. 48, 1–36. doi:10.18637/jss.v048.i02
Saleh, M. al, Owaidah, T., Musa, A. A., Al-Zahrani, H., Al-Sweedan, S., Joseph, N., et al. (2014). Rare inherited clotting factor deficiencies in paediatric population single tertiary centre experience in Saudi arabia. Blood 124, 5064. doi:10.1182/blood.V124.21.5064.5064
Saville, P., and Maclver, R. (2017). “A very good question” in Psychometric Testing: Critical Perspectives, Editor B. Cripps (West Sussex, United Kingdom: John Wiley & Sons, Ltd.), 29–42.
Schreiber, J., Nora, A., Stage, F., and Barlow, E. (2006). Presentación de informes de modelos de ecuaciones estructurales y análisis factorial confirmatorio: una revisión. Rev. Investig. Educ. 99, 323–338.
Sherin, S., Mathew, P., Johns, F., and Abraham, J. (2018). The feasibility of using remote data collection tools in field surveys. Int. J. Community Med. Public Heal 5, 81–85. doi:10.18203/2394-6040.ijcmph20175514
Skevas, T., Skevas, I., and Swinton, S. M. (2018). Does spatial dependence affect the intention to make land available for bioenergy crops? J. Agric. Econ. 69, 393–412. doi:10.1111/1477-9552.12233
Storm, H., Mittenzwei, K., and Heckelei, T. (2015). Direct payments, spatial competition, and farm survival in Norway. Am. J. Agric. Econ. 97, 1192–1205. doi:10.1093/ajae/aau085
Streiner, D. L., Norman, G. R., and Cairney, J. (2015). Health measurement scales: a practical guide to their development and use. Oxford University Press.
Thomas, M. A., Ahiablame, L. M., Engel, B. A., and Chaubey, I. (2014). Modeling water quality impacts of growing corn, switchgrass, and miscanthus on marginal soils. J. Water Resour. Prot. 06, 1352–1368. doi:10.4236/jwarp.2014.614125
Van Dael, M., Lizin, S., Swinnen, G., and Van Passel, S. (2017). Young people’s acceptance of bioenergy and the influence of attitude strength on information provision. Renew. Energy 107, 417–430. doi:10.1016/j.renene.2017.02.010
Wickham, H., and Seidel, D. (2020). scales: scale functions for visualization. R. Package Version 1, 1.
Wolf, E. J., Harrington, K. M., Clark, S. L., and Miller, M. W. (2013). Sample size requirements for structural equation models: an evaluation of power, bias, and solution propriety. Educ. Psychol. Meas. 73, 913–934. doi:10.1177/0013164413495237
Xie, B.-C., and Zhao, W. (2018). Willingness to pay for green electricity in Tianjin, China: based on the contingent valuation method. Energy Policy 114, 98–107. doi:10.1016/j.enpol.2017.11.067
Xu, X., Liu, G., and Mola-Yudego, B. (2022). Barriers and opportunities for bioenergy expansion in Chinese rural areas. Energy sustain. Dev. 70, 181–193. doi:10.1016/j.esd.2022.06.012
Yatokpa, A. B., Mahman, S., and Abble, K. (2010). Identification des potentialités et modalités d’exploitation des sources d’énergies renouvelables sur l’ensemble du territoire national. Rapp. Bénin.
Yee, C. H., Al-Mulali, U., and Ling, G. M. (2022). Intention towards renewable energy investments in Malaysia: extending theory of planned behaviour. Environ. Sci. Pollut. Res. 29, 1021–1036. doi:10.1007/s11356-021-15737-x
Keywords: geographic heterogeneity, behavioral decision, forestry biomass supply, sustainable energy transition, Benin
Citation: Balagueman RO, Assèdé ESP, Moumouni-Moussa I, Soumanou AA and Biaou SSH (2023) Spatial disparities altered the determinants of biomass supply intention for clean energy: policy implications. Front. Energy Res. 11:1239710. doi: 10.3389/fenrg.2023.1239710
Received: 13 June 2023; Accepted: 13 November 2023;
Published: 23 November 2023.
Edited by:
Rizwan Rasheed, Government College University, Lahore, PakistanReviewed by:
Richa Arora, Punjab Agricultural University, IndiaDilip Khatiwada, Royal Institute of Technology, Sweden
Copyright © 2023 Balagueman, Assèdé, Moumouni-Moussa, Soumanou and Biaou. This is an open-access article distributed under the terms of the Creative Commons Attribution License (CC BY). The use, distribution or reproduction in other forums is permitted, provided the original author(s) and the copyright owner(s) are credited and that the original publication in this journal is cited, in accordance with accepted academic practice. No use, distribution or reproduction is permitted which does not comply with these terms.
*Correspondence: Rodrigue Ogoulonou Balagueman, cm9kcmlndWViYWxhZ3VlbWFuQGdtYWlsLmNvbQ==
†These authors have contributed equally to this work