Design-based system performance assessment of a combined power and freshwater cogeneration system
- 1Department Teknik Elektro, Universitas Negeri Padang, Padang, Indonesia
- 2Energy Department, Universidad de la Costa, Barranquilla, Colombia
- 3Department of Electrical Engineering, Prince Sattam Bin Abdulaziz University, College of Engineering, Alkharj, Saudi Arabia
- 4Computer Technical Engineering Department, College of Technical Engineering, The Islamic University, Najaf, Iraq
- 5College of Technical Engineering, Al-Farahidi University, Baghdad, Iraq
- 6Docente Titular, Universidad de Investigación y Tecnología Experimental Yachay: Escuela de Ciencias Biológicas e Ingeniería, Urcuquí, Ecuador
- 7School of Environmental Management, Universidad Internacional del Ecuador: Escuela de Gestión Ambiental, Quito, Ecuador
- 8Department of Mechanics, Islamic Azad University, Tehran, Iran
- 9Department of Electrical Engineering, University of Engineering and Technology, Peshawar, Pakistan
- 10Islamic University Centre for Scientific Research, The Islamic University, Najaf, Iraq
- 11Department of Computer Engineering, Jeju National University, Jeju City, Republic of Korea
- 12Department of Business, University of South-Eastern Norway, Norway
In this research, the design and use of combined systems for the simultaneous production of water, heat, and energy have been proposed, and, to fulfill the water, electricity, and heat demands of a hotel, modeling of the multi-effect evaporative desalination (MED) and combined heat and power (CHP) generation system was done. Then, the design of these two systems was administered in a combined way. This design was applied in order to evaluate the economy of the combined system compared to separate systems. The performed scenario was executed every 24 h during the two seasons of the year. The genetic algorithm was used to optimize this system, and it was considered the objective function to minimize the annual costs. The results showed that the nominal capacity of the gas turbine and backup boiler in the CHP + MED + thermal energy storage (TES) system was (14%) larger and (8.2%) smaller, respectively, compared to the CHP+ MED system. In addition, by using the energy storage tank in the combined CHP + MED system, 5.1% of the annual costs were reduced.
1 Introduction
Nowadays, optimizing the performance of energy systems, reducing costs, and decreasing the pollutant of production is a serious trouble for designers and engineers, for which many solutions have been proposed. One of the best solutions is simultaneous production systems which are widely used in many industrial factories due to their higher efficiency (Baniassadi et al., 2016). In these systems, heat and electricity are produced simultaneously in one process, which can be used for domestic hot water, space heating, swimming pool heating, laundry processes, and absorption cooling (Rosen, 2021). Providing water and energy for the growing population of the world is a big challenge for human beings. Furthermore, due to the large amount of salt water worldwide, desalination is a suitable solution for the production of freshwater (Pugsley et al., 2016; Wang et al., 2019). Recently, the integration of water desalination systems with power generation systems has been taken into consideration by many researchers (Bai et al., 2023). Michael et al. evaluated the efficiency of combining the evaporative desalination system with the power and heat generation plant. In this article, a multi-effect evaporative desalination-thermal vapor compression (MED-TVC) desalination plant with a capacity of 36,000 m3/day was studied. Based on the development model found in EES software, sensitivity analysis was performed on it. The effect of several design and operation parameters on the fraction of the energy output evaluated as “efficient cogeneration” was investigated. The results showed that this fraction increases almost linearly with the number of MED units which were supplied with steam (Tamburini et al., 2016). Baniassadi et al. (2016) investigated the potential of exhaust waste heat recovery of a microturbine for seawater desalination by using an organic Rankine cycle (ORC). The heat obtained from the microturbines was used by operating the ORC, and the generated power was transferred to the reverse osmosis (RO) desalination water plant. Furthermore, the biological absorption of CO2 through microalgae cultivation by exploiting microturbine exhaust gases was considered (Tajik Mansouri et al., 2020). Cardona et al. integrated a small thermal desalination system MEE with small size (2000 m3/day) with one system SWRO single-stage seawater reverse osmosis. The heat and electricity needed for the system was provided by a gas engine. The results of 30% and 8% reduction in carbon dioxide emissions showed the carbon and unit cost of freshwater (Demir and Dincer, 2022). Salimi and Amidpour contributed toward modeling, simulation, parametric study, and economic evaluation of the internal combustion engine with desalination (Salimi and Amidpour, 2017). A multipurpose water desalination system, with the MED-TVC thermal steam concentrator, was simulated and optimized for economic analysis with a new approach in MATLAB software using the extra heat. A steam generator, with HRSG heat recovery, was used to provide the required heat for steam production (Harandi et al., 2021). The multiobjective optimization was done by using genetic algorithms based on the NSGA II non-major sorting. Finally, it is evident that the heating steam temperature is more influenced by GOR than by other decision variables.
Jamshidian et al. (2022) developed a solar hybrid desalination system that is independent of any fossil-based power source. They found that compared to a single multieffect desalination plant, the hybrid system could increase the total recovery by 15%. Moreover, an approximately 8% drop in the final cost of 1 m3 of produced freshwater was observed. Ghiasirad et al. (2021) proposed a multigeneration system fueled by a geothermal source integrated with a humidification–dehumidification desalination cycle. They reported that the overall system performance in winter was better than that in summer. Furthermore, the system had considerably high heating, cooling, and freshwater capacities. Moreover, thermoeconomic indexes were notably lower than those of similar systems. Zuo et al. (2020) investigated the numerical simulations for the wind supercharging solar chimney power plant integrated with a seawater desalination unit and a gas waste heat cycle. They considered the effect of the flue gas jet on the flow field in the chimney and the influence of rotational speed on the power output and freshwater yield. The power generation and hourly freshwater rate for that plant were approximately 0.38 MW and 20.3 ton/h, respectively. Shahsavari et al. (2022) developed the optimum sizing of a hybrid CHP and desalination unit as a polygeneration plant for supplying rural demands. As observed from the results, that system had a ∼58.5% lower carbon dioxide emission than conventional natural gas-driven plants and less than 27 km of grid breakeven distance. Moreover, the CHP reduced the annual fuel consumption by almost 225 m3/yr. Catrini et al. (2017) developed a CHP plant coupled with the MED unit considering the exergetic and exergoeconomic costs for all the material streams. In the aforementioned plant, the concentrated brine was alternatively considered a residue to be disposed or a resource to be exploited. Moreover, reverse electrodialysis was adopted for exergy recovery from the concentrated brine. They reported that the high unit costs were determined for the material streams and energy flows. You et al. (2020) developed a combined cooling, heating, and power system integrated with an MED system, a fuel cell, and a microgas turbine. They reported that the most exergy degradation of components is mainly due to endogenous components with gas turbines, inverters, and air compressors. Tawalbeh et al. (2023) reported that the desalination integrated with salinity gradient solar ponds was reported to exceed 50% exergy efficiency. Furthermore, those plants could show enhanced overall efficiency (approximately 30%) in hybrid power systems. However, the environmental concerns and large-scale requirements are still among the main challenges.
Mario Gorta et al. evaluated the production of power and freshwater with a gas turbine, S-CO2 supercritical cycle, and ORC with a water unit. An RO desalination unit was added to the power generation cycle to produce freshwater at a low cost. The results showed that the proposed combined system with excess heat recovery leads to high efficiency with a low cost of energy and freshwater (Manesh et al., 2021). Benalcazar determined the optimal size of thermal energy storage systems for CHP plants according to specific investment costs. The results showed that the integration of TES leads to a significant reduction in the use of steam boilers and reduces fuel costs and environmental impact (Benalcazar, 2021). Lepixar et al. improved the CHP system by integrating TES technology into the heat and power generation energy system. The investigated solutions included the connection of CHP with electric boilers and TES which helps balance heat and electricity loads and provides the possibility of creating an RES in the system (Lepiksaar et al., 2021). Haj Abdullahi evaluated the use of cooling and heating energy storage tanks in the optimization of the multiple production system. The optimization of the heating, cooling, and CCHP generation system by using a gas engine as the main driver is presented in this work. It was determined that using TES + CES tanks improved the optimal TAP by 9.48%, 5.19%, and 2.23%, respectively, compared to not using storage tanks (Hajabdollahi, 2015a).
This research suggests using a thermal energy storage tank to store excess heat and use it during necessary hours, reducing system costs and pollutants. The system includes a gas turbine for power generation and a backup boiler for heat supply if needed.
2 Materials and methods
2.1 Gas turbine—multieffect evaporative distillation hybrid system
Figure 1 shows a general schematic of the simultaneous production system of heat, electricity, and desalinated water CHP + MED. In this system, the required electricity is supplied from the main driver, which is the gas turbine, and in case of shortage, it is purchased from the network. The produced heat, which is discharged to the external environment through the exhaust, is recovered by using a heat exchanger with 90% efficiency and is used to provide the required heat for desalination and building. This heat exchanger is usually part of the drive system (Sanaye and Hajabdollahi, 2016). If the heat produced by the driver is less than the total requirement, the backup boiler will supply it.
Figure 2 shows the general schematic of the heat, electricity, and desalination system using the thermal energy storage tank CHP + MED + TES. If there is excess heat in the system, it would use it and would not waste on the environment like the previous system (Ma Y. et al., 2023; Gu et al., 2023; Tian et al., 2023). The working method of the energy storage tank is such that the excess heat of the stimulus is stored and used in the next timesteps according to the considered strategy, if necessary. The existence of this energy storage tank not only prevents the backup boiler from working more but also leads to the production of fewer pollutants.
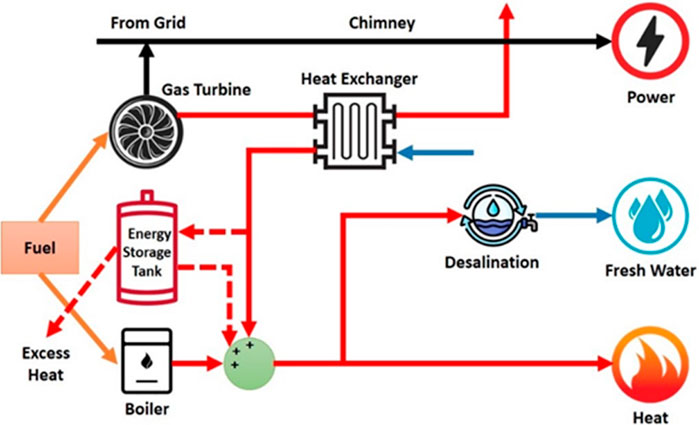
FIGURE 2. Novel gas turbine multieffect evaporative distillation hybrid system with an energy storage tank.
Figure 3 shows a typical example of an MED-TVC desalination plant. Its main components consist of N number of evaporators, a condenser, and a thermal vapor compressor (ejector). Their most common arrangement is parallel feeding (He et al., 2022; Wu et al., 2022; Han et al., 2023). In the parallel feeding arrangement, the flow direction of water vapor and liquid is similar to each other and is simpler than that of the other two arrangements. In general, the structure of this type of desalination is such that the steam produced in each effect is used as a driving fluid in the next effect. As shown in Figure 3, after the feed water enters the condenser, it exchanges heat with the steam coming out of the last heat exchanger and then exits at the temperature
2.2 Thermodynamic modeling of the CHP + MED + TES system equipment
The freshwater system has gained a lot of importance recently and is expected to increase in the future, so its thermodynamic modeling is presented with more complete details. The CHP system energy modeling is done based on existing relationships for efficiency and performance of equipment including the gas turbine and backup boiler at different partial loads. The energy storage tank is also modeled in terms of energy by considering it as a control volume whose enthalpy is checked at its input and output (Ashour, 2003; Al-Mutaz and Wazeer, 2014; Sanaye and Hajabdollahi, 2016; Esrafilian and Ahmadi, 2019; Xu et al., 2021; Zhao et al., 2022; Zhu et al., 2022; Luo et al., 2023).
2.3 Desalination modeling (MED-TVC)
In this section, the equations governing multieffect evaporative desalination with a thermal steam condenser have been described. Modeling is done by applying energy and mass conservation equations in evaporators, condensers, thermal vapor compressors (ejectors), and flash chambers. The design variables include the number of effects (n), driving steam pressure (
Assuming that the temperature difference is constant in all effects, so the following equation is used to calculate it (Al-Mutaz and Wazeer, 2014):
The compressed steam temperature
The steam temperature in the last heat exchanger is obtained from the following equation (Al-Mutaz and Wazeer, 2014):
The specific heat capacity of water, which is a function of water temperature and salinity, is obtained from the following equation (Al-Mutaz and Wazeer, 2014):
The pressure of condensed steam
Pressure is in kilopascals and temperature is in degree Celsius (Gao et al., 2021; Lin et al., 2022a; Lin et al., 2022b; Lu L. et al., 2022). The expansion ratio in the ejector can be obtained with Equation 7, and the compression ratio in the ejector can be obtained with Equation (8) (Al-Mutaz and Wazeer, 2014):
If the driving flow and the suction fluid is water vapor, the model equation for calculating the suction ratio
The amount of steam drawn is determined by the following equation:
The brine temperature in each heat exchanger is lower than the previous effect by
The steam temperature in each heat exchanger is calculated as follows:
The feedwater flow rate for each heat exchanger is obtained from the following equation:
The steam condensation temperature
The latent heat of driving steam, steam in the evaporator, and condenser are obtained from the following relationships:
In the aforementioned equations, the temperature is given in degree Celsius (Chen et al., 2022; Zhang et al., 2022; Li et al., 2023; Xia et al., 2023) and the latent heat is given in kJ/kg. The steam produced in the first effect is obtained by using the law of conservation of energy as follows (Al-Mutaz and Wazeer, 2014):
The saltwater concentration balance is estimated from the following equation:
The steam produced in the second heat exchanger is estimated from the following equation (Al-Mutaz and Wazeer, 2014):
The temperature and salinity of the heat exchanger output brine are calculated from the following equations, (Al-Mutaz and Wazeer, 2014):
The flash efficiency for 2 to n heat exchangers is obtained from the following equation (Sanaye and Hajabdollahi, 2016):
The cooling air temperature when entering into the heat exchanger can be calculated using the following equation (Al-Mutaz and Wazeer, 2014):
Equation 15 is used for the latent heat of vaporization at
In order to calculate the
The condensation temperature of the vapors entering the flash chambers can be estimate using the following equation:
The flashed steam in flash chambers is estimated as follows (Al-Mutaz and Wazeer, 2014):
The amount of steam created from the effect of 3 to
The total amount of condensation is obtained by the following equation (Al-Mutaz and Wazeer, 2014):
The output brine and salinity of converter 3 to n are calculated from the following equations, respectively (Al-Mutaz and Wazeer, 2014):
The vapors created in the last heat exchanger are divided into two parts: one part enters the
The overall heat transfer coefficient is estimated from the following equation (Al-Mutaz and Wazeer, 2014):
The heat transfer level for the first exchanger is estimated from the following equation:
The heat transfer level for the 2 to
The total surface heat transfer effects are estimated using the following equation:
The average logarithmic temperature difference and the overall heat transfer coefficient of the condenser can be calculated from the following two equations (Al-Mutaz and Wazeer, 2014):
The heat transfer level of the condenser is estimated from the following equation:
The flow rate of cooling water is obtained from the law of conservation of energy as follows (Al-Mutaz and Wazeer, 2014):
The specific heat transfer level is calculated as the sum of the heat transfer level of the exchangers and the condenser over the total produced water as follows (Li P. et al., 2022; Duan et al., 2023):
The performance of the MED-TVC system under the title of GOR is defined as follows (Al-Mutaz and Wazeer, 2014):
One of the most important features of thermal freshwater is specific heat consumption, which is defined as the amount of thermal energy consumed by the system to produce 1 l of freshwater (Deng et al., 2023). This relationship is calculated from the first law of thermodynamics as follows:
2.4 CHP system modeling
The total heating demand (building heating and desalination) is estimated from the following relationship (Hajabdollahi et al., 2015):
The first term of the equation is related to the required energy consumption for evaporative desalination.
By using the following relationship, the fuel consumption of the backup boiler is obtained as follows (Hajabdollahi et al., 2015):
where
The heat changes that can be recovered from the gas turbine exhaust in terms of partial load are calculated from the following function (Hajabdollahi, 2015b):
in which
The nominal fuel consumption
The thermal efficiency of the boiler is assumed to be a function of the partial load as follows (Hajabdollahi et al., 2015):
The efficiency of the gas turbine is estimated according to the nominal capacity of the following function (Sanaye and Hajabdollahi, 2016):
The modeling of the energy storage tank by choosing it as a volume to control the input and output enthalpies in it is checked as follows (Hajabdollahi et al., 2015):
Here,
When the drive heat recovery is greater than the total heating load, the excess heat is stored in the energy storage tank (Hajabdollahi et al., 2015):
2.5 Optimization
In this study, the minimization of the total annual cost is as follows (Hajabdollahi et al., 2015):
where
where
In this research, selling electricity to the grid is not allowed, and the excess electricity in each stage must be 0 or negative
Buying electricity is equivalent to a negative amount of electricity. In order to ensure that the temperature of the smoke is maintained above the dew point temperature and to prevent the formation of sulfuric acid and corrosion of the chimney, the following condition is considered:
The cost of gas fuel is 0.01054 $/kWh, purchase of electricity from the grid is 0.071667 $/kWh, and the emission penalty is 0.02086 $/kg of carbon dioxide (Sanaye and Hajabdollahi, 2016). The genetic algorithm is one of the oldest and most powerful optimization algorithms. According to the literature, acceptable optimal results can be achieved by using this algorithm to optimize energy conversion systems (Miao et al., 2021; Yuan and Yang, 2022; Zhang Z. et al., 2023).
2.6 Case studies
The study case is the proposed method of adding a thermal energy storage tank to the CHP + MED system for a commercial building in Ahvaz city to supply heat, electricity, and freshwater simultaneously (Lu et al., 2017). This building has 250 rooms, with an average area of 40 m2 for each room. It is to be noted that for simulation and mathematical modeling of the studied system, MATLAB and EES software were used.
In general, any study based on numerical evaluation and simulation has limitations compared to experimental and practical work. In this regard, the following assumptions were considered to simplify the simulation (Kaheal et al., 2023; Mousavi Rabeti et al., 2023; Yi et al., 2023):
1. The hybrid energy system works under steady-state conditions.
2. The temperature difference is the same in all converters.
3. Distilled water is free of salt.
4. Boiling point increase for all effects is 0.8.
5. Thermodynamic losses are negligible.
6. The temperature difference in all effects of the desalination unit is constant.
7. The flow rate of all effects of the desalination unit is the same.
8. The thermal efficiency of the boiler is assumed to be a function of partial load.
9. Components under constant isentropic efficiency are considered.
10. The system can work without any restrictions in the considered studied region and can be implemented.
11. The cost of replacing spare parts and insurance are considered 1.5% and 0.05% of the total direct cost, respectively.
12. The owner’s cost rate is 10% of the direct material and labor costs.
3 Results
3.1 Validation
The investment cost of system equipment is shown in Table 1. To validate the code developed in MATLAB software, in the multieffect evaporative desalination model with a thermal steam condenser, reference input parameters (You et al., 2020) in Table 2 have been used. The output results from the computational code with the modeling available in reference (You et al., 2020) compared the allowable error in an engineering range, which was than 2%, as shown in Table 3.
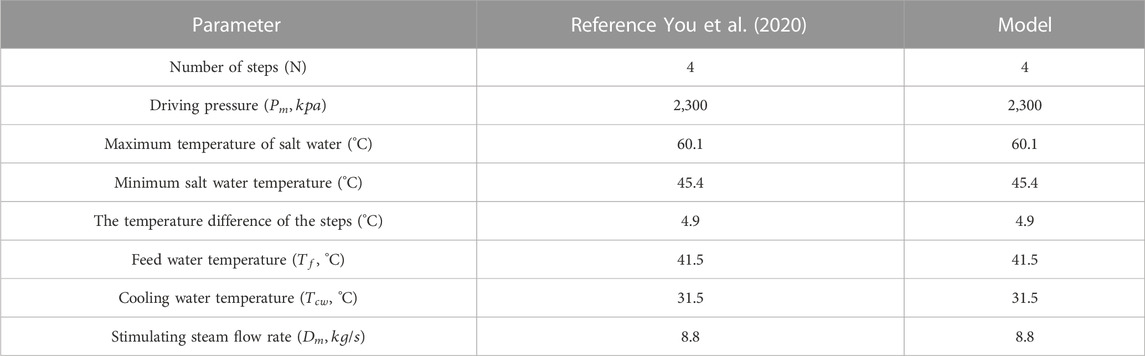
TABLE 2. Working conditions of desalination in the reference (You et al., 2020) and this research.
The energy required for desalination is provided from the heat recovered from the gas turbine and the storage tank in case of excess heat and finally from the backup boiler in case of shortage. For this purpose, first, the calculation code governing on the problem is developed in MATLAB software, and then it is optimized with a genetic algorithm. The number of design variables for combining the CHP + MED-TVC + TES system is 31 items, including gas turbine capacity, backup boiler capacity, energy storage tank capacity, and engine partial load every 2 hours out of every 24 h. Furthermore, a total of 24 items, the number of desalination effects, driving steam pressure in the first effect, flow rate feed water, and driving steam flow rate are selected to reduce the annual cost. In the combination of the CHP + MED-TVC system, all the mentioned variables, except the capacity of the energy storage tank, a total of 30 cases have been selected. The strategy used in the system is that for every 24 h of the two seasons of the year, the demand for heat, electricity, and freshwater for the whole year should be satiated, according to Figure 4.
The new strategy presented by many cases can be examined, which is also evaluated with a specific case study. The number of chromosomes of the genetic optimization algorithm, 300 mutation probability, 0.02 integration probability (Meng and Suzuki, 2016; Wang J. et al., 2022; Sun et al., 2023; Yang et al., 2023), and 1,000 generations were considered. Step design variables and their range in Table 4, as well as the optimal design variables for CHP + MED system composition in both cases, are given in Table 5:
3.2 CHP + MED + TES system results
As can be seen in Figure 5, the heat required for heating the building and desalination water throughout day and night is equal to the total heat produced by the driver, backup boiler, and storage tank, in which the heat produced by the driver is more than the total requirement. This excess heat is stored in the energy storage tank, and it is used when necessary and prevents the boiler from working or working more in the following hours. Figure 4 shows that in spring and summer, the heat demand is lower than the electricity demand throughout day and night.
In these two seasons, according to Figure 5, from 2 to 10 o’clock, the heat produced by the driver is more than the total heat requirement of the building and the desalination water requirement, and in this way, the excess heat is stored in the storage tank. Again, from 12:00 to 14:00, the production heat of the stimulator increases and reaches full load by 24:00, and the desalination energy consumption also increases (Min et al., 2023). From 12:00 p.m. to 6:00 p.m., the total heat required for desalination and heating is greater than the heat produced by the driver, so the storage tank starts to discharge.
During the autumn and winter seasons, according to Figure 4, the need for electricity decreases from 02:00 to 10:00 AM and increases from 12:00 to 18:00, and its peak continues until 24:00. It is clear that the actuator produces more heat at a higher partial load and less heat at a lower partial load. According to Figure 5, from 4:00 to 4:00 p.m., not only the generator works at partial load but also the heat demand is almost high in the early hours of this time, as a result of which the heat in the generator is not excessive and the back-up boiler is used to provide all the heat needs (Zhang X. et al., 2023). The boiler is turned off from 14:00 to 24:00, and additional heat is stored again from 16:00 to 24:00.
The heat produced by the engine is 87.226 MWh for the whole year, and the fuel consumed by the engine and boiler is 4,815,669 and 336,064 kg/year, respectively. The optimal parameters include the rated capacity of the gas turbine 2,500, backup boiler 2,600, and heat storage tank 2.3 Mw/hr. Furthermore, for desalination, the number of stages is 11, and the driving steam pressure is 1,000 kPa, the flow rate of seawater feed is 200, and the flow rate of driving steam is 1 kg/s.
Figure 6 shows that the electricity produced by the gas turbine provides an optimal part of the total electricity requirement. The shortage of this electricity is purchased from the network. After optimizing the desalination parameters, including the suction ratio in the ejector of 0.94, the performance coefficient of 12.26, and the specific heat consumption of 200.82 kJ/kg, the total purchase of electricity from the network is 13.527 MWh and the electricity produced by the generator is 49.772 MWh for the whole year (Chen et al., 2023a). The total electricity required in the whole year is 633 MWh. The generator provides 78.62% of the total electricity requirement, and the rest is purchased from the network. The penalty for pollutant emissions produced by the booster and backup boiler is 2.40292*105 $/year.
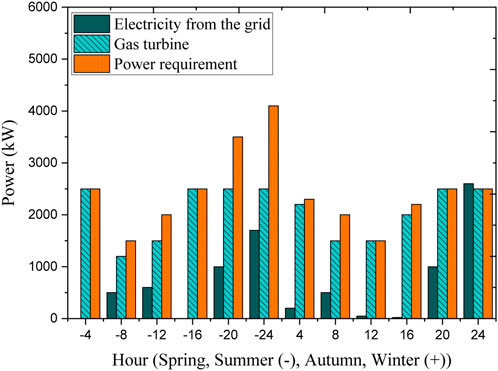
FIGURE 6. Electricity produced or consumed by the CHP + MED + TES system equipment throughout the year.
Figure 7 shows the freshwater production and demand by desalination throughout the year. Because in the CHP+MED system combination mode with and without the thermal energy storage tank, the freshwater is assed in (Chen et al., 2023b; Yin et al., 2023).
3.3 The results of the CHP + MED system
In this section, the results related to the modeling and optimization of the combination of the CHP + MED system without a storage tank are presented. In this system, the total heat required at all hours of the day and night for the building, and desalination is supplied from the generator and in case of shortage from the backup boiler because the heat required in autumn and winter is more than that in spring and summer (especially from 4:00 to 12:00 p.m.).
According to Figure 4, the electricity demand is low in winter and autumn from 8:00 a.m. to 2:00 p.m., 12 is almost uniform. Figure 8 shows that the actuator is on partial load and produces relatively lower heat. Therefore, the backup boiler produces more heat during these hours than during other hours. For the spring and summer seasons, the backup boiler provides the shortage of heat to meet the total heating and desalination needs at all hours. In this system, the heat produced by the driver is 81.022 Mwh/year, the fuel consumed by the driver is 4,506.129, and for the backup boiler, 1,075 tons has been obtained for the whole year.
After optimizing the rated driving capacity of 2.2 MWh, the rated capacity of the backup boiler is 2.8 MWh, also for desalination, the number of stages, driving steam pressure of 4 MPa, seawater flow rate of 300, and driving steam flow rate of 1 kg/s have been achieved.
As can be seen in Figure 9, after optimization, a part of the electricity demand is purchased by the supply driver, and the remaining power purchased from the grid. The total electricity requirement is 633 MWh/year, in which 47.416 MWh or 76.3% is purchased by the generator and the remaining 15,884 kWh is purchased from the grid.
The optimal capacity of the driver is 300 kW or 13.5% compared to the CHP + MED + TES system, and its fuel consumption is reduced by 7.1%. The fine for pollutant emissions produced by the booster and backup boiler 2.6032*105 $/yr has been obtained.
The reason for making the system cheaper by using a storage tank is the choice of a driver with a higher nominal capacity, which leads to more electricity production and a 4.01% reduction in electricity purchase. On the other hand, the heat produced by the drive in the CHP + MED + TES system is 8.02% more than that in the CHP + MED system, which makes the fuel consumption of the backup boiler decrease by 70.35% throughout year. The surcharge of pollutant emission for this system has increased by 8.58% compared to the CHP + MED + TES system.
Figure 10 shows the effect of fuel price changes, electricity purchase from the grid, and pollutant emission fines on the total annual cost. With the help of the following figure, considering the different prices in different cities, the total annual cost is obtained.
One of the influencing parameters on the amount of freshwater produced is the fuel flow rate fed to the energy system. Figure 11 shows the effect of the fuel flow rate fed to the energy system on the amount of freshwater produced and total annual cost. By increasing the flow rate of fuel fed to the process, more thermal energy can be available for the cycle, which consequently improves the thermal energy administered into the desalination unit. Therefore, the rate of freshwater produced can increase. However, due to the increase in cost related to the increase in fuel consumption, the total annual cost of the energy system will relatively increase. Figure 11 confirms that by increasing the rate of fuel input into the system from 1 to 5 kg/s, the rate of freshwater produced and the total annual cost will increase by 33.2% and 4.6%, respectively.
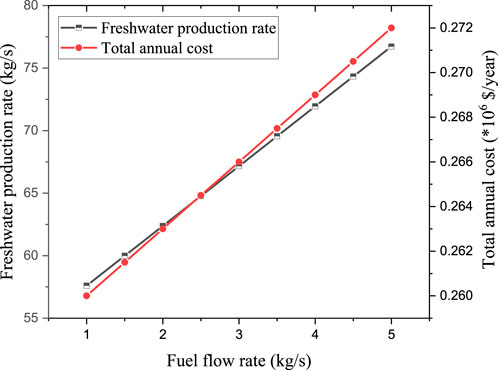
FIGURE 11. Effect of the fuel flow rate fed to the energy system on the amount of freshwater produced and the total annual cost.
Another parameter that affects the performance of the energy system is the pressure of the input flow to the gas turbine. Figure 12 shows the effect of gas turbine inlet flow pressure on the amount of freshwater produced and the total annual cost. Although increasing the pressure of the gas turbine inlet flow can increase the production power rate of the turbine, it is due to the reduction of the enthalpy of the turbine output flow (in fact, the reduction of the waste heat of the gas turbine), which results in less heat being available to the desalination unit. As a result, the amount of freshwater produced decreases. In addition, increasing the pressure of the input flow to the turbine requires spending additional costs for the compression process, which causes the growth of the overall annual cost of the energy system. Figure 12 exhibits that by increasing the pressure of the input flow to the turbine from 1 to 5 MPa, the rate of freshwater produced and the total annual cost will decrease and increase by 16.9% and 8.18%, respectively.
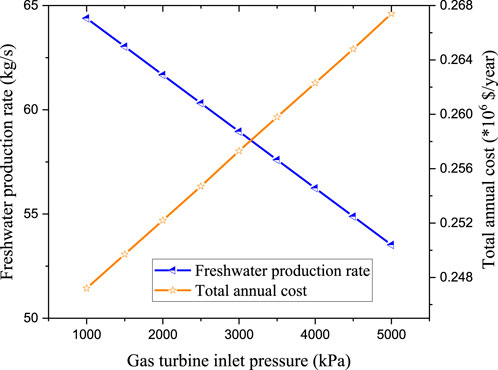
FIGURE 12. Effect of gas turbine inlet flow pressure on the amount of freshwater produced and the total annual cost.
Increasing the effect number of the MED unit is another critical parameter that can affect the total annual cost of the energy system. Increasing the effect number of the MED unit can increase the rate of freshwater production due to increasing the number of desalination stages. However, this can increase the total annual cost of the energy system. However, it is expected that the unit cost of freshwater production will decrease with the increase in the production rate of freshwater. This requires further analysis in future research. The influence of effect number of the MED unit on the amount of freshwater produced and the total annual cost of the energy system is displayed in Figure 13. As seen, by increasing the effect number of the MED unit from 4 to 7, the rate of freshwater produced and the total annual cost will increase by 58.3% and 2.1%, respectively.
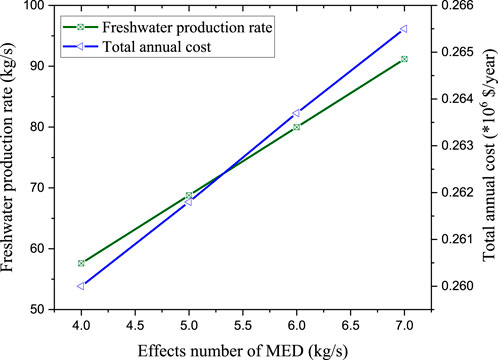
FIGURE 13. Influence of effect number of the MED unit on the amount of freshwater produced and the total annual cost of the energy system.
3.4 Comparative analysis
In order to verify the obtained results and also highlight the advantages of the proposed energy system, the overall result of the research has been compared with the results reported in similar studies in the form of a comparative evaluation. Table 6 summarizes the comparative analysis. As can be seen, the hybrid energy system can achieve a lower total annual cost (and in some cases, competitive results) than other similar technologies for producing power and freshwater. However, it is recommended that in future studies, the experimental and environmental behaviors of the proposed energy system should be studied and analyzed carefully so that an eco-friendly and efficient system can be addressed.
4 Conclusion
Nowadays, optimizing the performance of energy systems, reducing costs, and decreasing pollutant of production is a serious trouble for designers and engineers for which many solutions have been proposed. One of the ways to reduce the cost of energy systems, which is proportional to the increase in efficiency, is the use of combined heat and power production systems. In this research, the modeling and investigation of the combined gas turbine and desalination system for the production of heat, electricity, and freshwater has been done.
In this study, some of them used the excess heat of the drive system for the required heat of the desalination water, and the others used it to feed the Rankine organic power generation cycle, which seems necessary to check the energy storage tank to use this heat for the CHP and freshwater system. To increase the efficiency and evaluate the reliability of the studied system, the effect of the thermal storage tank on the overall efficiency of the system has been evaluated.
In order to reduce the operating costs of the CHP and water desalination system, the studied system has been optimized using the intelligent algorithm. The results showed that the use of the thermal storage tank has a direct relationship with the amount of fuel consumption in the gas turbine, and this parameter will have a direct effect on the emission of pollutants so that the use of the thermal tank will increase the efficiency of the system and reduce the fuel consumption in the gas turbine and reduce the costs of the system.
The optimal technical results in these systems showed that in the combined heat, power, and water desalination system, the gas turbine with a nominal capacity of 14% is larger, the heat production is 8.2% higher, and its fuel consumption is increased by 7.1.
Suggestions for future work include the following (Liao et al., 2023):
Performance evaluation of the three latent heat storage designs.
Performance analysis of a co-generation system using solar energy.
Thermo-economic analysis of a combined based power and freshwater cogeneration system.
Data availability statement
The original contributions presented in the study are included in the article/Supplementary Material, further inquiries can be directed to the corresponding authors.
Author contributions
EE: conceptualization, formal analysis, and writing–original draft. JN: data curation, investigation, project administration, software, and writing–original draft. AC: methodology, project administration, resources, software, and writing–original draft. IA-K: resources, software, validation, and writing–review and editing. MJ: investigation, methodology, supervision, conceptualization, formal analysis, and writing–original draft. PY-M: data curation, validation, visualization, and writing–original draft. RA: conceptualization, data curation, methodology, project administration, and writing–review and editing. IK: methodology, project administration, software, supervision, and writing–review and editing. DM: formal analysis, funding acquisition, investigation, methodology, project administration, resources, supervision, software, and writing–review and editing.
Funding
The author(s) declare that the financial support was received for the research, authorship, and/or publication of this article. This research was financially supported by the Ministry of Small and Medium-sized Enterprises (SMEs) and Startups (MSS), Korea, under the “Regional Specialized Industry Development Plus Program (R&D, S3246057)” supervised by the Korea Technology and Information Promotion Agency for SMEs (TIPA). This work was also financially supported by the Ministry of Trade, Industry, and Energy (MOTIE) through the fostering project of the establishment project of industry–university fusion district.
Conflict of interest
The author declares that the research was conducted in the absence of any commercial or financial relationships that could be construed as a potential conflict of interest.
Publisher’s note
All claims expressed in this article are solely those of the authors and do not necessarily represent those of their affiliated organizations, or those of the publisher, the editors, and the reviewers. Any product that may be evaluated in this article, or claim that may be made by its manufacturer, is not guaranteed or endorsed by the publisher.
References
Ahmadi, P., Fakhari, I., and Rosen, M. A. (2022). A comprehensive approach for tri-objective optimization of a novel advanced energy system with gas turbine prime mover, ejector cooling system and multi-effect desalination. Energy 254, 124352. doi:10.1016/j.energy.2022.124352
Al-Mutaz, I. S., and Wazeer, I. (2014). Development of a steady-state mathematical model for MEE-TVC desalination plants. Desalination 351, 9–18. doi:10.1016/j.desal.2014.07.018
Ashour, M. M. (2003). Steady state analysis of the Tripoli West LT-HT-MED plant. Desalination 152 (1), 191–194. doi:10.1016/s0011-9164(02)01062-7
Bai, L., Asadollahzadeh, M., Chauhan, B. S., Abdrabboh, M., Fayed, M., Ayed, H., et al. (2023). A new biomass-natural gas dual fuel hybrid cooling and power process integrated with waste heat recovery process: exergoenvironmental and exergoeconomic assessments. Process Saf. Environ. Prot. 176, 867–888. doi:10.1016/j.psep.2023.06.037
Baniassadi, A., Momen, M., Shirinbakhsh, M., and Amidpour, M. (2016). Application of R-curve analysis in evaluating the effect of integrating renewable energies in cogeneration systems. Appl. Therm. Eng. 93, 297–307. doi:10.1016/j.applthermaleng.2015.09.101
Benalcazar, P. (2021). Optimal sizing of thermal energy storage systems for CHP plants considering specific investment costs: A case study. Energy 234, 121323. doi:10.1016/j.energy.2021.121323
Cai, T., Dong, M., Chen, K., and Gong, T. (2022). Methods of participating power spot market bidding and settlement for renewable energy systems. Energy Rep. 8, 7764–7772. doi:10.1016/j.egyr.2022.05.291
Catrini, P., Cipollina, A., Micale, G., Piacentino, A., and Tamburini, A. (2017). Exergy analysis and thermoeconomic cost accounting of a Combined Heat and Power steam cycle integrated with a Multi Effect Distillation-Thermal Vapour Compression desalination plant. Energy Convers. Manag. 149, 950–965. doi:10.1016/j.enconman.2017.04.032
Chen, C., Wu, X., Yuan, X., and Zheng, X. (2022). A new technique for the subdomain method in predicting electromagnetic performance of surface-mounted permanent magnet motors with shaped magnets and a quasi-regular polygon rotor core. IEEE Trans. Energy Convers. 38, 1396–1409. doi:10.1109/TEC.2022.3217042
Chen, J., Liu, Z., Yin, Z., Liu, X., Li, X., Yin, L., et al. (2023a). Predict the effect of meteorological factors on haze using BP neural network. Urban Clim. 51, 101630. doi:10.1016/j.uclim.2023.101630
Chen, J., Wen, L., Bi, C., Liu, Z., Liu, X., Yin, L., et al. (2023b). Multifractal analysis of temporal and spatial characteristics of earthquakes in Eurasian seismic belt. Open Geosci. 15 (1). doi:10.1515/geo-2022-0482
Demir, M. E., and Dincer, I. (2022). An integrated solar energy, wastewater treatment and desalination plant for hydrogen and freshwater production. Energy Convers. Manag. 267, 115894. doi:10.1016/j.enconman.2022.115894
Deng, W., Zhang, Y., Tang, Y., Li, Q., and Yi, Y. (2023). A neural network-based adaptive power-sharing strategy for hybrid frame inverters in a microgrid. Front. Energy Res. 10. doi:10.3389/fenrg.2022.1082948
Du, S., Yin, J., Xie, H., Sun, Y., Fang, T., Wang, Y., et al. (2022). Auger scattering dynamic of photo-excited hot carriers in nano-graphite film. Appl. Phys. Lett. 121 (18), 181104. doi:10.1063/5.0116720
Duan, Y., Zhao, Y., and Hu, J. (2023). An initialization-free distributed algorithm for dynamic economic dispatch problems in microgrid: modeling, optimization and analysis. Sustain. Energy, Grids Netw. 34, 101004. doi:10.1016/j.segan.2023.101004
El-Dessouky, H. T., and Ettouney, H. M. (2002). Fundamentals of salt water desalination. Amsterdam, Netherlands: Elsevier.
Esrafilian, M., and Ahmadi, R. (2019). Energy, environmental and economic assessment of a polygeneration system of local desalination and CCHP. Desalination 454, 20–37. doi:10.1016/j.desal.2018.12.004
Gao, Y., Doppelbauer, M., Ou, J., and Qu, R. (2021). Design of a double-side flux modulation permanent magnet machine for servo application. IEEE J. Emerg. Sel. Top. Power Electron. 10 (2), 1671–1682. doi:10.1109/JESTPE.2021.3105557
Ghamari, V., Hajabdollahi, H., and Dehaj, M. S. (2021). Comparison of gas turbine and diesel engine in optimal design of CCHP plant integrated with multi-effect and reverse osmosis desalinations. Process Saf. Environ. Prot. 154, 505–518. doi:10.1016/j.psep.2021.07.030
Ghiasirad, H., Asgari, N., Khoshbakhti Saray, R., and Mirmasoumi, S. (2021). Thermoeconomic assessment of a geothermal based combined cooling, heating, and power system, integrated with a humidification-dehumidification desalination unit and an absorption heat transformer. Energy Convers. Manag. 235, 113969. doi:10.1016/j.enconman.2021.113969
Gu, Q., Li, S., Gong, W., Ning, B., Hu, C., and Liao, Z. (2023). L-SHADE with parameter decomposition for photovoltaic modules parameter identification under different temperature and irradiance. Appl. Soft Comput. 143, 110386. doi:10.1016/j.asoc.2023.110386
Hajabdollahi, H. (2015a). Evaluation of cooling and thermal energy storage tanks in optimization of multi-generation system. J. Energy Storage 4, 1–13. doi:10.1016/j.est.2015.08.004
Hajabdollahi, H., Ganjehkaviri, A., and Jaafar, M. N. M. (2015). Assessment of new operational strategy in optimization of CCHP plant for different climates using evolutionary algorithms. Appl. Therm. Eng. 75, 468–480. doi:10.1016/j.applthermaleng.2014.09.033
Hajabdollahi, H. (2015b). Investigating the effects of load demands on selection of optimum CCHP-ORC plant. Appl. Therm. Eng. 87, 547–558. doi:10.1016/j.applthermaleng.2015.05.050
Han, Y., Chen, S., Gong, C., Zhao, X., Zhang, F., and Li, Y. (2023). Accurate SM disturbance observer-based demagnetization fault diagnosis with parameter mismatch impacts eliminated for IPM motors. IEEE Trans. Power Electron. 38 (5), 5706–5710. doi:10.1109/TPEL.2023.3245052
Harandi, H. B., Asadi, A., Rahnama, M., Shen, Z. G., and Sui, P. C. (2021). Modeling and multi-objective optimization of integrated MED–TVC desalination system and gas power plant for waste heat harvesting. Comput. Chem. Eng. 149, 107294. doi:10.1016/j.compchemeng.2021.107294
He, Y., Wang, F., Du, G., Pan, L., Wang, K., Gerhard, R., et al. (2022). Revisiting the thermal ageing on the metallised polypropylene film capacitor: from device to dielectric film. High. Volt. 8, 305–314. doi:10.1049/hve2.12278
Huang, N., Chen, Q., Cai, G., Xu, D., Zhang, L., and Zhao, W. (2021b). Fault diagnosis of bearing in wind turbine gearbox under actual operating conditions driven by limited data with noise labels. IEEE Trans. Instrum. Meas. 70, 1–10. doi:10.1109/TIM.2020.3025396
Huang, S., Huang, M., and Lyu, Y. (2021a). Seismic performance analysis of a wind turbine with a monopile foundation affected by sea ice based on a simple numerical method. Eng. Appl. Comput. fluid Mech. 15 (1), 1113–1133. doi:10.1080/19942060.2021.1939790
Jamshidian, F. J., Gorjian, S., and Shafieefar, M. (2022). Techno-economic assessment of a hybrid RO-MED desalination plant integrated with a solar CHP system. Energy Convers. Manag. 251, 114985. doi:10.1016/j.enconman.2021.114985
Jiang, J., Zhang, L., Wen, X., Valipour, E., and Nojavan, S. (2022). Risk-based performance of power-to-gas storage technology integrated with energy hub system regarding downside risk constrained approach. Int. J. Hydrogen Energy 47 (93), 39429–39442. doi:10.1016/j.ijhydene.2022.09.115
Kaheal, M. M., Chiasson, A., and Alsehli, M. (2023). Component-based, dynamic simulation of a novel once through multistage flash (MSF-OT) solar thermal desalination plant. Desalination 548, 116290. doi:10.1016/j.desal.2022.116290
Lepiksaar, K., Mašatin, V., Latõšov, E., Siirde, A., and Volkova, A. (2021). Improving CHP flexibility by integrating thermal energy storage and power-to-heat technologies into the energy system. Smart Energy 2, 100022. doi:10.1016/j.segy.2021.100022
Li, M., Yang, M., Yu, Y., and Lee, W. (2021). A wind speed correction method based on modified hidden Markov model for enhancing wind power forecast. IEEE Trans. Industry Appl. 58 (1), 656–666. doi:10.1109/TIA.2021.3127145
Li, P., Hu, J., Qiu, L., Zhao, Y., and Ghosh, B. K. (2022b). A distributed economic dispatch strategy for power–water networks. IEEE Trans. Control Netw. Syst. 9 (1), 356–366. doi:10.1109/TCNS.2021.3104103
Li, R., Wu, X., Tian, H., Yu, N., and Wang, C. (2022a). Hybrid memetic pretrained factor analysis-based deep belief networks for transient electromagnetic inversion. IEEE Trans. Geoscience Remote Sens. 60, 1–20. doi:10.1109/TGRS.2022.3208465
Li, S., Chen, J., He, X., Zheng, Y., Yu, C., and Lu, H. (2023). Comparative study of the micro-mechanism of charge redistribution at metal-semiconductor and semimetal-semiconductor interfaces: pt(Ni)-MoS2 and Bi-MoS2(WSe2) as the prototype. Appl. Surf. Sci. 623, 157036. doi:10.1016/j.apsusc.2023.157036
Liao, K., Lu, D., Wang, M., and Yang, J. (2022). A low-pass virtual filter for output power smoothing of wind energy conversion systems. IEEE Trans. Industrial Electron. 69 (12), 12874–12885. doi:10.1109/TIE.2021.3139177
Liao, Q., Li, S., Xi, F., Tong, Z., Chen, X., Wan, X., et al. (2023). High-performance silicon carbon anodes based on value-added recycling strategy of end-of-life photovoltaic modules. Energy 281, 128345. doi:10.1016/j.energy.2023.128345
Lin, L., Shi, J., Ma, C., Zuo, S., Zhang, J., Chen, C., et al. (2023b). Non-intrusive residential electricity load decomposition via low-resource model transferring. J. Build. Eng. 73, 106799. doi:10.1016/j.jobe.2023.106799
Lin, L., Zhang, J., Gao, X., Shi, J., Chen, C., and Huang, N. (2023a). Power fingerprint identification based on the improved V-I trajectory with color encoding and transferred CBAM-ResNet. PloS one 18 (2), e0281482. doi:10.1371/journal.pone.0281482
Lin, X., Liu, Y., Yu, J., Yu, R., Zhang, J., and Wen, H. (2022b). Stability analysis of Three-phase Grid-Connected inverter under the weak grids with asymmetrical grid impedance by LTP theory in time domain. Int. J. Electr. Power & Energy Syst. 142, 108244. doi:10.1016/j.ijepes.2022.108244
Lin, X., Yu, R., Yu, J., and Wen, H. (2022a). Constant coupling effect-based PLL for synchronization stability enhancement of grid-connected converter under weak grids. IEEE Trans. Industrial Electron. 70, 11310–11323. doi:10.1109/TIE.2022.3227268
Liu, G. (2023). A Q-Learning-based distributed routing protocol for frequency-switchable magnetic induction-based wireless underground sensor networks. Future Gener. Comput. Syst. 139, 253–266. doi:10.1016/j.future.2022.10.004
Liu, S., and Liu, C. (2021b). Direct harmonic current control scheme for dual three-phase PMSM drive system. IEEE Trans. Power Electron. 36 (10), 11647–11657. doi:10.1109/TPEL.2021.3069862
Liu, S., and Liu, C. (2021a). Virtual-vector-based robust predictive current control for dual three-phase PMSM. IEEE Trans. Industrial Electron. 68 (3), 2048–2058. doi:10.1109/TIE.2020.2973905
Liu, Y., and Xu, K. (2023). Millimeter-wave bandpass filters using on-chip dual-mode resonators in 0.13-m SiGe BiCMOS technology. IEEE Trans. Microw. Theory Tech. 71, 3650–3660. doi:10.1109/TMTT.2023.3242317
Lu, B., Meng, X., Tian, Y., Zhu, M., and Suzuki, R. O. (2017). Thermoelectric performance using counter-flowing thermal fluids. Int. J. Hydrogen Energy 42 (32), 20835–20842. doi:10.1016/j.ijhydene.2017.06.132
Lu, C., Zhou, H., Li, L., Yang, A., Xu, C., Ou, Z., et al. (2022a). Split-core magnetoelectric current sensor and wireless current measurement application. J. Int. Meas. Confed. 188, 110527. doi:10.1016/j.measurement.2021.110527
Lu, L., Wu, W., Gao, Y., Pan, C., Yu, X., Zhang, C., et al. (2022b). Study on current discrepancy and redistribution of HTS non-insulation closed-loop coils during charging/discharging and subsequent transient process toward steady-state operation. Supercond. Sci. Technol. 35 (9), 095001. doi:10.1088/1361-6668/ac7dfe
Luo, Z., Cai, S., Hao, S., Bailey, T. P., Luo, Y., Luo, W., et al. (2023). Extraordinary role of Zn in enhancing thermoelectric performance of Ga-doped n-type PbTe. Energy Environ. Sci. 15, 368–375. doi:10.1039/d1ee02986j
Lv, S., Zhang, B., Ji, Y., Ren, J., Yang, J., Lai, Y., et al. (2023). Comprehensive research on a high performance solar and radiative cooling driving thermoelectric generator system with concentration for passive power generation. Energy 275, 127390. doi:10.1016/j.energy.2023.127390
Ma, X., Wan, Y., Wang, Y., Dong, X., Shi, S., Liang, J., et al. (2023b). Multi-parameter practical stability region analysis of wind power system based on limit cycle amplitude tracing. IEEE Trans. Energy Convers., 1–13. doi:10.1109/TEC.2023.3274775
Ma, Y., Zhu, D., Zhang, Z., Zou, X., Hu, J., and Kang, Y. (2023a). Modeling and transient stability analysis for type-3 wind turbines using singular perturbation and lyapunov methods. IEEE Trans. Industrial Electron. 70 (8), 8075–8086. doi:10.1109/TIE.2022.3210484
Manesh, M. K., Firouzi, P., Kabiri, S., and Blanco-Marigorta, A. (2021). Evaluation of power and freshwater production based on integrated gas turbine, S-CO2, and ORC cycles with RO desalination unit. Energy Convers. Manag. 228, 113607. doi:10.1016/j.enconman.2020.113607
Meng, X., and Suzuki, R. O. (2016). Helical configuration for thermoelectric generation. Appl. Therm. Eng. 99, 352–357. doi:10.1016/j.applthermaleng.2016.01.061
Miao, Z., Meng, X., and Liu, L. (2021). Design a new thermoelectric module with high practicability based on experimental measurement. Energy Convers. Manag. 241, 114320. doi:10.1016/j.enconman.2021.114320
Min, C., Pan, Y., Dai, W., Kawsar, I., Li, Z., and Wang, G. (2023). Trajectory optimization of an electric vehicle with minimum energy consumption using inverse dynamics model and servo constraints. Mech. Mach. Theory 181, 105185. doi:10.1016/j.mechmachtheory.2022.105185
Mousavi Rabeti, S. A., Khoshgoftar Manesh, M. H., and Amidpour, M. (2023). An innovative optimal 4E solar-biomass waste polygeneration system for power, methanol, and freshwater production. J. Clean. Prod. 412, 137267. doi:10.1016/j.jclepro.2023.137267
Nazari, N., and Porkhial, S. (2020). Multi-objective optimization and exergo-economic assessment of a solar-biomass multi-generation system based on externally-fired gas turbine, steam and organic Rankine cycle, absorption chiller and multi-effect desalination. Appl. Therm. Eng. 179, 115521. doi:10.1016/j.applthermaleng.2020.115521
Pugsley, A., Zacharopoulos, A., Mondol, J. D., and Smyth, M. (2016). Global applicability of solar desalination. Renew. Energy 88, 200–219. doi:10.1016/j.renene.2015.11.017
Rosen, M. A. (2021). “Exergy analysis,” in Design and performance optimization of renewable energy systems (Amsterdam, Netherlands: Elsevier), 43–60.
Salimi, M., and Amidpour, M. (2017). Modeling, simulation, parametric study and economic assessment of reciprocating internal combustion engine integrated with multi-effect desalination unit. Energy Convers. Manag. 138, 299–311. doi:10.1016/j.enconman.2017.01.080
Sanaye, S., and Hajabdollahi, H. (2016). Comparison of different scenarios in optimal design of a CCHP plant. Proc. Institution Mech. Eng. Part E J. Process Mech. Eng. 230 (4), 247–262. doi:10.1177/0954408914547070
Shahsavari, A., Vaziri Rad, M. A., Pourfayaz, F., and Kasaeian, A. (2022). Optimal sizing of an integrated CHP and desalination system as a polygeneration plant for supplying rural demands. Energy 258, 124820. doi:10.1016/j.energy.2022.124820
Sun, S., Liu, Y., Li, Q., Wang, T., and Chu, F. (2023). Short-term multi-step wind power forecasting based on spatio-temporal correlations and transformer neural networks. Energy Convers. Manag. 283, 116916. doi:10.1016/j.enconman.2023.116916
Tajik Mansouri, M., Amidpour, M., and Ponce-Ortega, J. M. (2020). Optimization of the integrated power and desalination plant with algal cultivation system compromising the energy-water-environment nexus. Sustain. Energy Technol. Assessments 42, 100879. doi:10.1016/j.seta.2020.100879
Tamburini, A., Cipollina, A., Micale, G., and Piacentino, A. (2016). CHP (combined heat and power) retrofit for a large MED-TVC (multiple effect distillation along with thermal vapour compression) desalination plant: high efficiency assessment for different design options under the current legislative EU framework. Energy 115, 1548–1559. doi:10.1016/j.energy.2016.03.066
Tawalbeh, M., Javed, R. M. N., Al-Othman, A., and Almomani, F. (2023). Salinity gradient solar ponds hybrid systems for power generation and water desalination. Energy Convers. Manag. 289, 117180. doi:10.1016/j.enconman.2023.117180
Tian, H., Liu, J., Wang, Z., Xie, F., and Cao, Z. (2023). Characteristic analysis and circuit implementation of a novel fractional-order memristor-based clamping voltage drift. Fractal Fract. 7 (1), 2. doi:10.3390/fractalfract7010002
Vazini Modabber, H., and Khoshgoftar Manesh, M. H. (2021). Optimal exergetic, exergoeconomic and exergoenvironmental design of polygeneration system based on gas Turbine-Absorption Chiller-Solar parabolic trough collector units integrated with multi-effect desalination-thermal vapor compressor-reverse osmosis desalination systems. Renew. Energy 165, 533–552. doi:10.1016/j.renene.2020.11.001
Wang, H., Wang, B., Luo, P., Ma, F., Zhou, Y., and Mohamed, M. A. (2022a). Association of low-carbohydrate-diet score and cognitive performance in older adults: national Health and Nutrition Examination Survey (NHANES). CSEE J. Power Energy Syst. 8 (4), 983–992. doi:10.1186/s12877-022-03607-1
Wang, H., Wu, X., Zheng, X., and Yuan, X. (2022b). Model predictive current control of nine-phase open-end winding PMSMs with an online virtual vector synthesis strategy. IEEE Trans. Industrial Electron. 1, 2199–2208. doi:10.1109/TIE.2022.3174241
Wang, J., Liang, F., Zhou, H., Yang, M., and Wang, Q. (2022c). Analysis of position, pose and force decoupling characteristics of a 4-UPS/1-RPS parallel grinding robot. Symmetry 14 (4), 825. doi:10.3390/sym14040825
Wang, X., van Dam, K. H., Triantafyllidis, C., Koppelaar, R. H., and Shah, N. (2019). Energy-water nexus design and operation towards the sustainable development goals. Comput. Chem. Eng. 124, 162–171. doi:10.1016/j.compchemeng.2019.02.007
Wang, Y., He, H., Xiao, X., Li, S., Chen, Y., and Ma, H. (2023b). Multi-stage voltage sag state estimation using event-deduction model corresponding to EF, EG, and EP. IEEE Trans. Power Deliv. 38 (2), 797–811. doi:10.1109/TPWRD.2022.3198854
Wang, Y., Ma, H., Xiao, X., Wang, Y., Zhang, Y., and Wang, H. (2023a). Harmonic state estimation for distribution networks based on multi-measurement data. IEEE Trans. Power Deliv. 38 (4), 2311–2325. doi:10.1109/TPWRD.2023.3239479
Wu, Z., Lin, B., Fan, J., Zhao, J., Zhang, Q., and Li, L. (2022). Effect of dielectric relaxation of epoxy resin on dielectric loss of medium-frequency transformer. IEEE Trans. Dielectr. Electr. Insulation 29 (5), 1651–1658. doi:10.1109/TDEI.2022.3193652
Xia, H., Zan, L., Yuan, P., Qu, G., Dong, H., Wei, Y., et al. (2023). Evolution of stabilized 1T-MoS2 by atomic-interface engineering of 2H-MoS2/Fe-nx towards enhanced sodium ion storage. Angew. Chem. Int. Ed. 62 (14), e202218282. doi:10.1002/anie.202218282
Xiao, S., Wang, Z., Wu, G., Guo, Y., Gao, G., Zhang, X., et al. (2023). The impact analysis of operational overvoltage on traction transformers for high-speed trains based on the improved capacitor network methodology. IEEE Trans. Transp. Electrification, 1. doi:10.1109/TTE.2023.3283668
Xu, K., Guo, Y., Liu, Y., Deng, X., Chen, Q., and Ma, Z. (2021). 60-GHz compact dual-mode on-chip bandpass filter using GaAs technology. IEEE Electron Device Lett. 42 (8), 1120–1123. doi:10.1109/LED.2021.3091277
Yang, M., Wang, Y., Wang, C., Liang, Y., Yang, S., Wang, L., et al. (2023). Digital twin-driven industrialization development of underwater gliders. IEEE Trans. Industrial Inf. 19, 9680–9690. doi:10.1109/TII.2023.3233972
Yi, S., Lin, H., Abed, A. M., Shawabkeh, A., Marefati, M., and Deifalla, A. (2023). Sustainability and exergoeconomic assessments of a new MSW-to-energy incineration multi-generation process integrated with the concentrating solar collector, alkaline electrolyzer, and a reverse osmosis unit. Sustain. Cities Soc. 91, 104412. doi:10.1016/j.scs.2023.104412
Yin, L., Wang, L., Ge, L., Tian, J., Yin, Z., Liu, M., et al. (2023). Study on the thermospheric density distribution pattern during geomagnetic activity. Appl. Sci. 13 (9), 5564. doi:10.3390/app13095564
You, H., Han, J., and Liu, Y. (2020). Conventional and advanced exergoeconomic assessments of a CCHP and MED system based on solid oxide fuel cell and micro gas turbine. Int. J. Hydrogen Energy 45 (21), 12143–12160. doi:10.1016/j.ijhydene.2020.02.138
Yu, D., Guo, J., Meng, J., and Sun, T. (2023). Biofuel production by hydro-thermal liquefaction of municipal solid waste: process characterization and optimization. Chemosphere 328, 138606. doi:10.1016/j.chemosphere.2023.138606
Yuan, H., and Yang, B. (2022). System dynamics approach for evaluating the interconnection performance of cross-border transport infrastructure. J. Manag. Eng. 38 (3). doi:10.1061/(ASCE)ME.1943-5479.0001015
Zhang, X., Yue, X., Pan, Y., Du, H., and Liu, B. (2023b). Crushing stress and vibration fatigue-life optimization of a battery-pack system. Struct. Multidiscip. Optim. 66, 48. doi:10.1007/s00158-023-03510-2
Zhang, X., Wang, Y., Yuan, X., Shen, Y., Lu, Z., and Wang, Z. (2022). Adaptive dynamic surface control with disturbance observers for battery/supercapacitor-based hybrid energy sources in electric vehicles. IEEE Trans. Transp. Electrification, 1. doi:10.1109/TTE.2022.3194034
Zhang, Z., Altalbawy, F. M. A., Al-Bahrani, M., and Riadi, Y. (2023a). Regret-based multi-objective optimization of carbon capture facility in CHP-based microgrid with carbon dioxide cycling. J. Clean. Prod. 384, 135632. doi:10.1016/j.jclepro.2022.135632
Zhao, P., Ma, K., Yang, J., Yang, B., Guerrero, J. M., Dou, C., et al. (2022). Distributed power sharing control based on adaptive virtual impedance in seaport microgrids with cold ironing. IEEE Trans. Transp. Electrification 9, 2472–2485. doi:10.1109/TTE.2022.3211204
Zhu, L., Li, Z., and Hou, K. (2022). Effect of radical scavenger on electrical tree in cross-linked polyethylene with large harmonic superimposed DC voltage. High. Volt. 8, 739–748. doi:10.1049/hve2.12302
Keywords: optimization, desalination, combined system, gas turbine, heat synchronization
Citation: Elfizon E, Nuñez Alvarez JR, Chammam A, Al-Kharsan IH, Jweeg MJ, Yánez-Moretta P, Alayi R, Khan I, Byun Y-C and Madsen DØ (2023) Design-based system performance assessment of a combined power and freshwater cogeneration system. Front. Energy Res. 11:1265309. doi: 10.3389/fenrg.2023.1265309
Received: 27 July 2023; Accepted: 15 September 2023;
Published: 02 October 2023.
Edited by:
Arman Oshnoei, Aalborg University, DenmarkReviewed by:
Sina Ghaemi, Aalborg University, DenmarkHoda Sorouri, Aalborg University, Denmark
Omid Sadeghian, University of Tabriz, Iran
Copyright © 2023 Elfizon, Nuñez Alvarez, Chammam, Al-Kharsan, Jweeg, Yánez-Moretta, Alayi, Khan, Byun and Madsen. This is an open-access article distributed under the terms of the Creative Commons Attribution License (CC BY). The use, distribution or reproduction in other forums is permitted, provided the original author(s) and the copyright owner(s) are credited and that the original publication in this journal is cited, in accordance with accepted academic practice. No use, distribution or reproduction is permitted which does not comply with these terms.
*Correspondence: José Ricardo Nuñez Alvarez, jnunez22@cuc.edu.co; Yung-Cheol Byun, ycb@jejunu.ac.kr; Dag Øivind Madsen, dag.oivind.madsen@usn.no