- 1Civil Engineering Department, College of Engineering, Najran University, Najran, Saudi Arabia
- 2Architectural Engineering Department College of Engineering, Najran University, Najran, Saudi Arabia
- 3Graduate School of Education, Department of Architecture (Doctorate-English) Okan Universitesi Istanbul, Istanbul, Türkiye
- 4Grupo de Investigación en Deterioro de Materiales, Transición Energética y Ciencia de datos DANT3, Facultad de Ingenieria y Urbanismo, Universidad Señor de Sipán, Chiclayo, Peru
- 5Department of Civil Engineering, Iran University of Science and Technology, Tehran, Iran
This study was carried out to investigate the impact of building insulation, a method of reducing energy consumption, on the amount of energy consumed in a building, as well as its impact on cooling and heating loads and carbon emission. A residential structure was designed in Revit, and DesignBuilder determined the cooling and heating loads, as well as the energy consumption. Under three distinct climate conditions, the impact of the environment on the energy-carbon emission nexus of residential buildings was assessed. The cold mountain climate of Koick, Slovakia; the arid desert climate of Ha’il, Saudi Arabia; and the tropical monsoon climate of Borneo, Indonesia were chosen. During the design stage, the Particle Swarm Optimization (PSO) method was used to minimize the energy consumption cost (ECC) and CO2 emissions. Over the course of 24 h, the cooling and heating loads decreased by 2.51 kW and 1.9 kW, respectively. When the two modes in Ha’il were combined, the heating load was reduced to 850 kWh and the cooling load was reduced to 650 kWh, according to the results. In Borneo, the heating load was reduced by 200 kWh, while in Koick, it was reduced by 2,000 kWh. The cooling load was reduced by 550 and 50 kWh in Borneo and Koick, respectively. This system appears to perform better in arid and hot climates in terms of both heating and cooling loads. However, energy losses in the arid and hot climate (Ha’il) are greater than in other climates. This could be due to temperature and humidity differences between the inside and outside. According to the findings of the PSO evolutionary algorithm optimization, the building can be constructed to reduce ECC by 19% by taking into account input characteristics such as Wind-to-Wall Ratio (WWR), wall, glazes, and weather conditions. This research provides useful insights into the practical application of optimization methods for reducing CO2 emissions, paving the way for more sustainable and eco-conscious architectural practices.
1 Introduction
The advent of modernity has witnessed a surge in housing construction, which can be attributed to the proliferation of technologies reliant on fossil fuels (Mobaraki et al., 2012; Tajfar et al., 2023). The industrial revolution has shifted men from rural to urban areas (Ramakrishna et al., 2020). With the advancement of technology, human lifestyles shifted to the point where fossil fuels were used as heaters (Kalair et al., 2021). As a result, the world’s energy needs have grown, but the world’s energy resources are running out (Taner et al., 2018; Molajou et al., 2021; Afshar et al., 2022). The new energy shock in 2000 has coincided with an increase in energy consumption in China and India, resulting in a significant increase in global energy demand and prices (Wang and Li, 2016). However, owing to the non-renewability of fossil fuels, new and more severe shocks are likely (Liu et al., 2021; Yang and Dian, 2022). Energy consumption management has been proposed as a means of mitigating the impact of this potential event on communities (Gopinath et al., 2020).
Integrating renewable energy and fuel cells into the built environment has garnered attention to address these challenges (Taner, 2018). Techno-economic analysis determines the feasibility and viability of such technologies, enabling sustainable energy transition decision-making (Taner et al., 2019). Solar panels, wind turbines, and geothermal systems can reduce building energy use and environmental impact (Taner and Sivrioglu, 2017; A; Jasim et al., 2023; Alaiwi et al., 2023). Alternative energy solutions like fuel cells can boost energy efficiency and reduce environmental impact (Taner, 2018). A comprehensive cost analysis is needed to assess the financial costs of installing, maintaining, and operating these technologies to ensure long-term economic and environmental sustainability (Tehranian, 2023b). Additionally, transportation energy consumption must be addressed. Energy-efficient transportation systems, public transportation, and electric vehicles can reduce carbon emissions and energy use (Tehranian, 2023a).
The building sector accounts for approximately 40% of global energy consumption in the whole world (Pérez-Lombard et al., 2008). According to this statistic, this sector consumes more energy than transportation and industry combined (Bahramian et al., 2021). This energy is used to power mechanical and electrical devices in buildings, which provide air conditioning in the form of cooling, heating, ventilation, and lighting (Zhou et al., 2007). Thus, by following these principles and making a small initial investment, the energy consumption of the construction industry can be reduced by 5–30 percent. Implementing these changes determines the effects of minor and major design changes on the energy consumption or savings of a building or any of its spaces (Carvalho et al., 2021; Khan and Panuwatwanich, 2021). To reduce energy consumption, techniques such as selecting materials with high thermal resistance, thermal insulation, multiplane windows, solar systems, renewable energy sources, and high-efficiency air conditioning systems should be used (Boddapati et al., 2021). To calculate the cooling and heating loads, it is critical to accurately model and simulate the energy consumption (Fan and Ding, 2019).
Active and passive methods are frequently employed to optimize energy consumption during the construction phase owing to the significant energy requirements for achieving sustainable buildings (Balali et al., 2020; Yu et al., 2020). The goal of the active method is to substitute renewable energy sources with nonrenewable energy sources and fossil fuels. Renewable energy sources are an important component of sustainable development and can be effectively used in urban environments under certain conditions (Gholamian et al., 2022). Renewable energy sources are used to generate mechanical, electrical, and thermal energy. Solar energy, wind energy, water energy, energy derived from the Earth’s core heat, biofuel energy, tidal energy, and ocean wave energy are all growing in popularity (Darko et al., 2020; Shacheri et al., 2022; Yavari et al., 2022). The passive method expresses optimal design, climate compatible, and achieves environmental and design potentials, such as maximizing natural light use or interior space arrangement (Zhang et al., 2022). The optimal use of energy in passive method was achieved using design methods, basic principles, and natural elements. This method provides recommendations for building design, so the architectural design of the building should be as environmentally friendly as possible to take advantage of the desired natural conditions and facilities. This architectural design technique reduced the amount of energy required to maintain thermal comfort. Building orientation, volume, general shape, interior space, light walls, canopies, thermal inertia, and natural ventilation are all elements effect the energy consumption factors in buildings (Ghedas, 2021; Tushar et al., 2021; Arumugam et al., 2022).
Owing to the rapid advancement of computer software and its application in building design, engineers and architects are now designing air-conditioning systems and building facilities in new ways (Zhou et al., 2007). Historically, air conditioning system design and facility calculations were based on the estimation of maximum loads, which were calculated using simple and manual methods (Huang and Li, 2021). In addition to calculating loads, the energy analysis must be considered during the building design phase. In other words, to reduce energy consumption, the values of the parameters affecting the building energy must be calculated (Guo et al., 2021; Liu and Jiang, 2021).
Building Information Modelling (BIM) is the process of creating and deploying a computer model to simulate, plan, design, construct, and operate facilities (Utkucu and Sözer, 2020). The resulting model is a building information model with numerous intelligent and parametric datasets. By extracting and analyzing this information in the output, the user can make better and more accurate decisions, thereby improving the running process (Boddupalli et al., 2019; Rausch and Haas, 2021). BIM refers to the virtual model examination of a building prior to construction to investigate problems and simulate and analyze the underlying effects (Elnabawi, 2020; Pellegrini et al., 2020). It also aids in the reduction of errors by displaying execution and construction management errors as well as ambiguous information, paving the way for project calculation and control. Energy modeling, as part of BIM, is one of the foundations of building design quality (Di Biccari et al., 2022). Energy modeling can provide an accurate assessment of energy consumption, as well as the cost of operation, system selection, design, and proper optimization of heating, refrigeration, and lighting systems. As a result, energy modeling should be synchronized with BIM to consider all the project’s decisions (Sharif and Hammad, 2019; Abdel-Mawla et al., 2022).
BIM has been linked to building energy modeling through academic and corporate efforts. The most important stage in BIM-based energy simulation is information transfer between BIM software and energy simulation software, with as little information loss as possible. Gao et al. (Gao et al., 2019) looked into the relationship between building information model-ing and energy simulation models. They developed a BIM-based BEM classification system for information exchange (building energy Modelling). Data are typically transmitted using Industry Foundation Classes (IFC) or green building XML (gbXML). A similar study was conducted by Sanhudo et al. (Sanhudo et al., 2018) who concluded that the fundamental problem is information loss during data transfer between BIM and building energy simulation, which necessitates the re-entry of a large amount of previously stored data into the building energy model. Furthermore, despite the issue of information loss, they discovered that there are significant benefits of using BIM in retrofit projects because of the ease of building information management. By transferring data via the BIM-to-BEM Interoperability Process (BBIP), Kamal and Memari (Kamel and Memari, 2019) investigated the link between BIM and building energy simulations. They discovered that the benefit of BIM for energy modeling is the ease of data management if proper communication and retrieval of relevant files are performed using BEM tools. If this issue is addressed, energy consumption modeling may result in the automation of building energy modeling and simulation, the clear visualization of simulation results, and the capacity to manage building data, particularly real-time data, to maintain an energy model. Updating the degree of accuracy and reliability of energy consumption modeling in structures relies on the reduction of errors in BIM-to-BEM communication. Choi et al. (Choi et al., 2019) developed a Python tool that uses IFC data to perform an ISO 13790:2008-compliant calculation. They believed that this technology is less prone to mistakes than manual entry caused by human error.
Effective energy management has the potential to significantly reduce the energy consumption considering environmental issues (Balouch et al., 2022). From this perspective, a reduction in energy consumption and CO2 emission as well as a precise analysis of energy-carbon emission nexus in the building are necessary (Liu et al., 2020). The purpose of this study is to optimize the energy consumption, energy costs, and CO2 emissions in a residential building during construction and operation. BIM and Revit Autodesk 2020 were used to design the residential structure, and DesignBuilder was used to calculate the cooling and heating loads, as well as the energy consumption. BIM reduces inefficiency, boosts productivity, and lowers project cost. To achieve optimal conditions, the characteristics of the building elements and environmental conditions were numerically modeled. Furthermore, the effects of exterior wall insulation and lack of insulation on energy loss were evaluated hourly throughout the day and monthly under various weather conditions as a novel approach. To accomplish this goal, three distinct weather conditions were chosen: a cold mountain climate, dry desert climate, and tropical monsoon climate. This study can help managers and construction companies to understand how to design a net-zero energy consumption building. The design parameters were evaluated using the PSO algorithm to minimize the energy consumption cost (ECC) and CO2 emissions in the building to determine whether the lowest amount of energy consumption is achievable in the design mode.
2 Methodology
In this study, residential buildings were examined from two perspectives. First, the external walls of the building were considered without insulation, and the energy consumption and cooling and heating loads were calculated. Next, polyurethane insulation was applied to the external walls, and energy analysis was repeated. Finally, the two cases were examined and analyzed simultaneously. Additionally, three weather conditions were investigated. Cold mountain climate (Košický, Slovakia), arid desert climate (Ha’il, Saudi Arabia), and tropical monsoon climate (Borneo, Indonesia) were selected. The main weather data are shown in Figure 1.
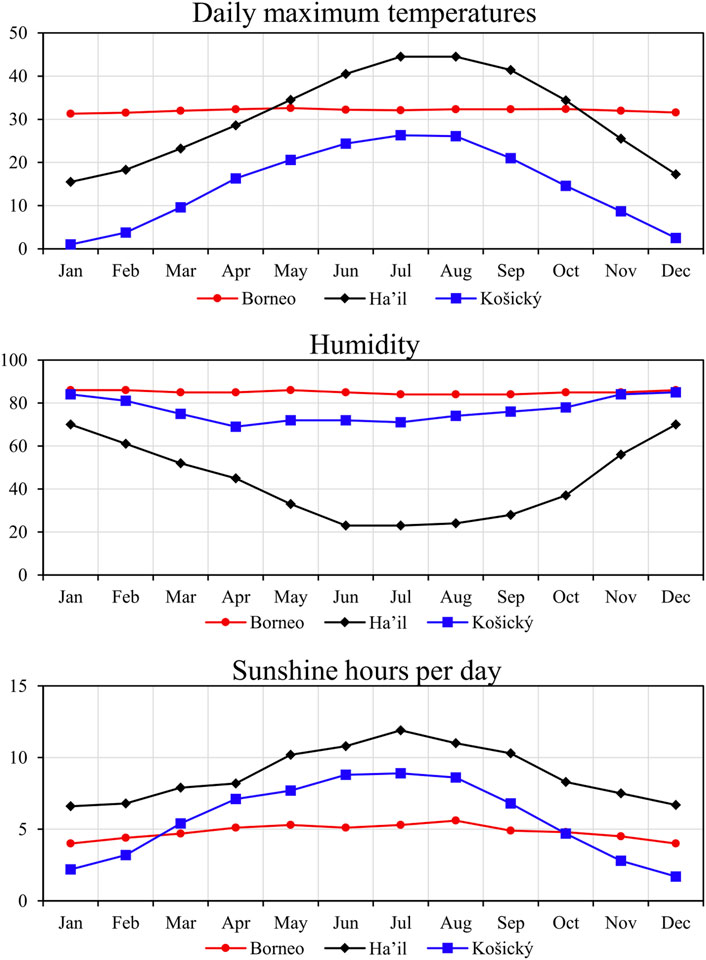
FIGURE 1. A comparison of the weather condition for Košický, Slovakia, as cold mountain climate, Ha’il, Saudi Arabia, as arid desert climate, and Borneo, Indonesia, as tropical monsoon climate.
As shown in Figure 1, the highest daytime temperatures in Ha’il were reached in July, with an average of 45.7°C. On the other hand, the coldest month is January, with an average of just 17.0°C. In Košický, July is the warmest month, at 26.3°C. There, it is coolest in January, with an average of 1.0°C. However, the highest daytime temperatures in Borneo were reached in May, with an average of 32.6°C. On the other hand, the coldest month is January, with an average of just 31.3°C.
2.1 Residential building details
The intended building is a building with a 2-story residential use; the amount of area and use of each part could be seen in Figure 2.
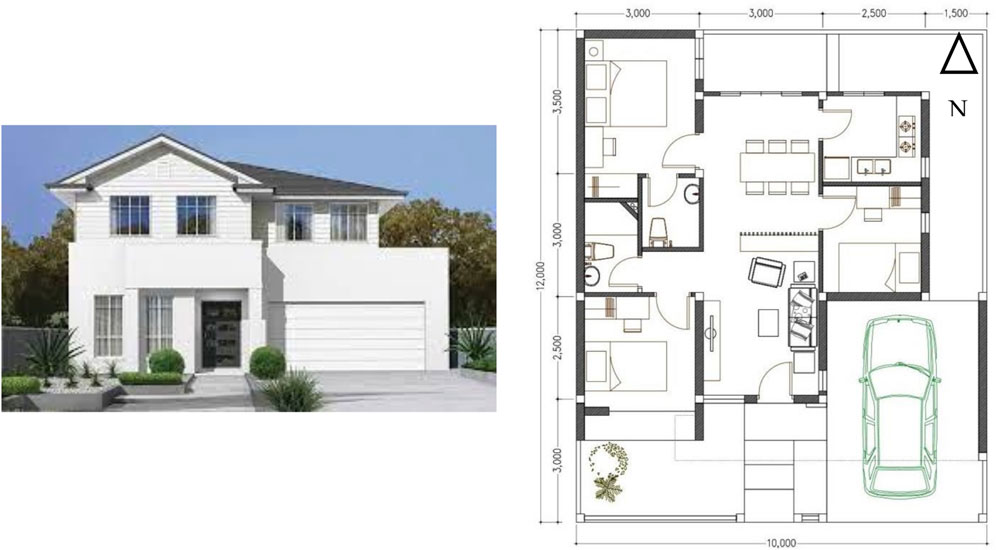
FIGURE 2. Schematic of the residential building in this study and floor plan with the size of the premises.
Tables 1, 2 list the materials used in exterior walls and ceilings with thermal properties that result in the first case (exterior walls of the building without insulation) (De Lieto Vollaro et al., 2015). In the second case, the outside walls of the building were made with the materials listed in Tables 1, 2, plus a 5 cm layer of polyurethane insulation. Weather data was also used in DesignBuilder.
2.2 Energy consumption simulation
The existing emulation software implements shared computing engines. For instance, the Energy Plus tool computing engine is utilized in a variety of simulation tools such as the recently released DesignBuilder. DesignBuilder not only has highly accurate calculations, but also has an abundance of features compared to other modeling software currently available (such as eQUEST, KEP-IYTE-ESS, BEMOSS, etc.). The most significant advantage of DesignBuilder is its ability to model all aspects of a building, including building physics (building materials), building architecture, cooling and heating systems, and lighting systems. When changes are made during the building design and modeling phases, the function of this software becomes more evident (Pinheiro et al., 2018). The extensive library of software also helps obtain more accurate outcomes. In addition, given that the novelty of this study is to analyze the decrease in heating and cooling energy consumption during different months of the year, the software stated is well suited to achieve the objectives of the study.
DesignBuilder was used to simulate the cooling and heating loads as well as the energy consumption in the two previously mentioned modes (insulated outer shell with and without insulation). After creating a three-dimensional model of the building, the spaces that require ventilation are defined in the software and output in the Revit software in gbxML format, and its model is imported into the DesignBuilder software. Finally, the energy consumption of the building was calculated. A fan coil system also provides thermal comfort to the building. Following that, information such as space usage, number of occupants, lighting type, electrical equipment, amount of air infiltration, and heat transfer coefficients are entered, all of which are useful for calculating cooling and heating loads (Salimzadeh et al., 2020; Tan et al., 2021).
2.3 Optimization method
PSO is a method that was utilized for the aim of this inquiry in order to produce the most optimal design. This investigation uses a modernized version of the PSO method, which permits quicker advancement toward the goal of global convergence. Similar to other collective algorithms, the particle community algorithm maintains a set of potential solutions until the optimal solutions are found and the end conditions are met. They continued their search for space. The efficiency of the particle community algorithm is determined by two primary factors: the algorithm itself and the population size. The coefficients of the algorithm are a collection of real numbers that appear in the equation of the algorithm and determine the path of the particle movement in the search space. These coefficients are typically fixed or variable in common methods (Wang et al., 2018). In the PSO algorithm, the particles move in the search space, and each particle saves its best personal experience, including the position or velocity vector, whereas the group saves its best collective experience. At every instant, the velocity vector is updated based on the three velocity factors from the previous step, the best personal experience, and the best collective experience. Each particle’s position is then updated based on this new velocity. If
where
The Eqs 1, 2 for velocity update and position update in multi objective PSO (MOPSO) are identical to those for PSO. Except for the objective function, every explicitly stated parameter is identical. Eq. 3 represents a function of objectives comprising numerous goals. Multi-objective optimization typically involves the incorporation of constraints alongside multiple objectives. All solutions, including the optimal one, must adhere to the limitations in order to be deemed practical. The flowchart of the MOPSO algorithm, which operates under the dominance criterion, is depicted in Figure 3.
The goal of the optimization component is to reduce the Energy Consumption Cost (ECC) and CO2 emission. The ECC includes costs associated with both the construction of the building and its ongoing operation. Because ECC considers cash flows at a number of different stages, it is necessary to compute the value of money across time. As a result, the values of each ECC element were revised to reflect the present. Using Eq. 4, it can be achieved the actual ECC.
Mj is the quantity of the j-th material; Ij is the energy intensity of the j-th material; and m is the operation time for the project.
Input variables are also essential components of the optimization process. In this investigation, the design factors that had the greatest impact on lighting and thermal performance were selected as the choice variables. According to Liu and Ning (Liu and Ning, 2019), the most significant factors to consider when designing a building are the types of walls, glazing, window-to-wall ratio (WWR), and climate conditions which identical by humidity and temperature (Table 3).
3 Results and discussion
This section examines the amount of cooling and heating load in different building environments in 24 h and the amount of cooling and heating load in different months of the year in the two normal modes and with polyurethane. In addition, the energy consumption of each structure was compared.
3.1 Cooling load
Figures 4, 5 were utilized to illustrate the cooling loads associated with various building components during a hot day. Through a comprehensive analysis, it was determined that the most significant heat loss is observed in the outer shell walls of the building, particularly between 16 and 19 h. Notably, the uninsulated wall exhibited a heat loss of 9.67 kW, which reduced to 7.59 kW with the incorporation of insulation in the outer shell. This signifies a decrease in heat loss by 2.08 kW, representing a substantial 21.5% reduction, emphasizing the crucial role of external wall insulation in energy conservation.
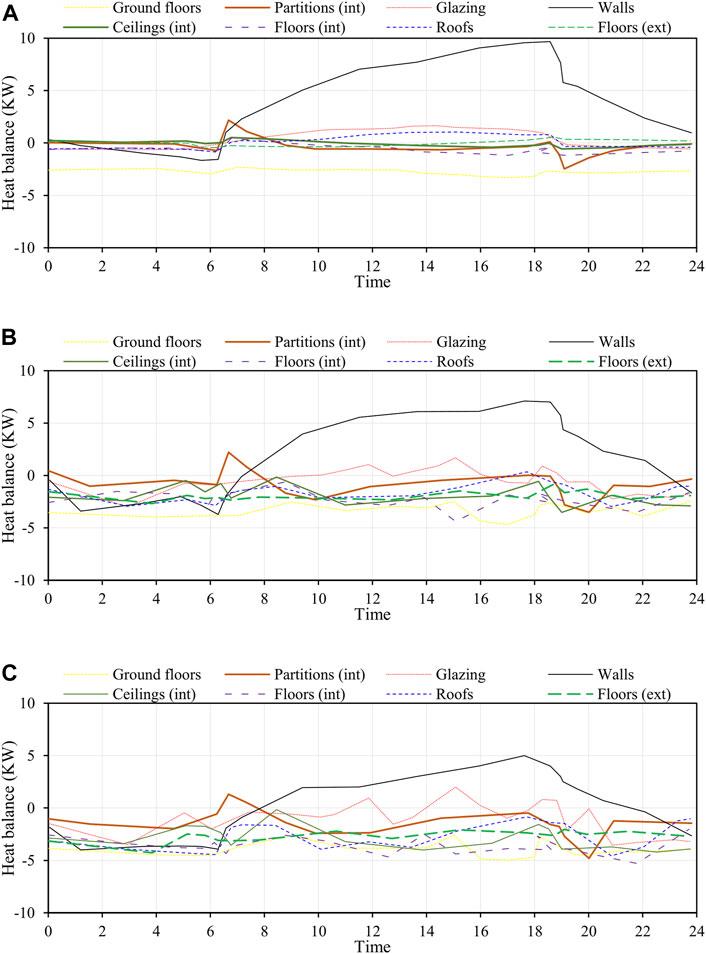
FIGURE 4. Cooling load and waste in different parts of the building (outer shell without insulation). (A) Ha’il, Saudi Arabia, (B) Borneo, Indonesia, and (C) Košický, Slovakia.
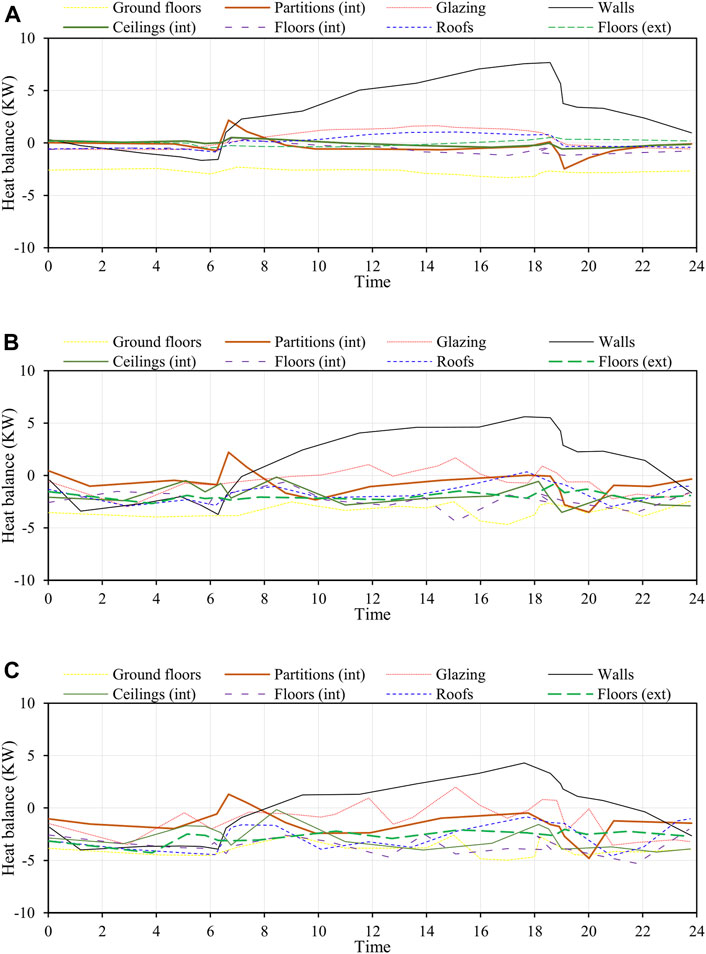
FIGURE 5. Cooling load and waste in different parts of the building (insulated outer shell). (A) Ha’il, Saudi Arabia, (B) Borneo, Indonesia, and (C) Košický, Slovakia.
Moreover, the findings from Figures 4, 5 emphasize that the highest energy absorption occurred during the construction of the outer shell, while the basement exhibited the least energy losses. It is important to note that energy losses are comparable across walls, windows, floors, ceilings, and roofs. Additionally, the study area’s dry and hot climate, as observed in Ha’il, resulted in higher energy losses compared to other climates. This disparity may potentially be attributed to the lower humidity levels indoors relative to the outdoor environment. Considering the journal’s emphasis on the depth of analysis, these results necessitate further exploration and detailed examination of the analytical findings.
3.2 Heating load
Figure 6 highlights the heating load variations across different building components with and without exterior shell insulation. The results illustrate that in various materials and climate settings, more heat dissipation occurs through the walls compared to other building components, aligning with the observations made in the cooling load analysis. Specifically, Figure 6A demonstrates an 11.2 kW heat loss through exterior walls without insulation in arid and hot climates, whereas Figure 6B shows a reduced heat loss of 9.8 kW through insulated exterior walls, indicating a significant reduction of 1.4 kW or approximately 12.5%.
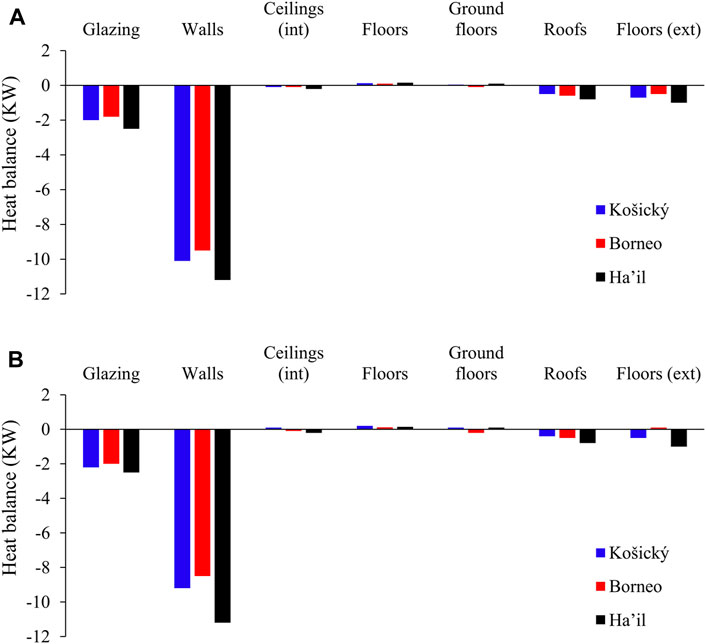
FIGURE 6. Heating load and amount of waste in different parts of the building (A) outer shell without insulation, (B) insulated outer shell.
Conversely, in cold weather conditions, exterior walls without insulation exhibited a heating load of 10.1 kW, whereas those with insulation exhibited a reduced heating load of 9.2 kW. Similarly, in tropical climates, the heating load for external walls without insulation was 9.5 kW, whereas with insulation, it was reduced to 8.5 kW. These findings suggest that buildings in tropical and humid climates demonstrate greater adaptability and superior heat transfer performance, emphasizing the crucial role of exterior wall insulation in maintaining energy efficiency.
Furthermore, the study noted that in a dry and hot climate, the cooling load amounted to 19.84 kW, while the heating load in the absence of external shell insulation reached 15.45 kW. However, with well-insulated building exteriors, the cooling and heating loads decreased to 17.33 kW and 13.55 kW, respectively, marking a considerable reduction of 2.51 kW and 1.9 kW from their uninsulated counterparts. These findings underline the significant impact of exterior insulation on enhancing the energy efficiency of buildings across various climates, prompting further examination and exploration into the intricacies of the heating load analysis.
3.3 Comparison of cooling and heating load in different months of the year
Figures 7, 8 provide detailed insights into the cooling and heating loads across different months of the year. Figure 7 presents a comprehensive view of the total cooling and heating loads, alongside the apparent load within the building’s uninsulated exterior shell, under three distinct climate scenarios. Notably, the findings indicated that in Ha’il, the total heating load in January amounted to 6,200 kWh, while in Borneo and Košický, it was 1,200 kWh and 8,500 kWh, respectively. The month characterized by the coldest weather in the Northern Hemisphere contributed significantly to the overall total, leading to the highest combined load across all scenarios.
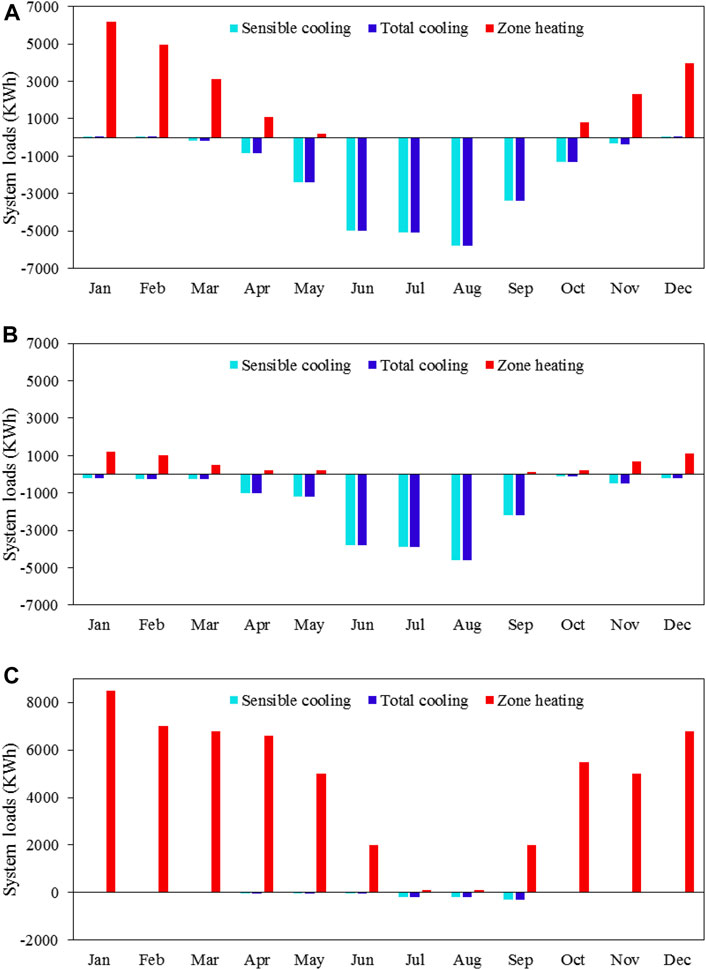
FIGURE 7. Cooling and heating load and sensible cooling load in different months of the year outer shell without insulation (A) Ha’il, Saudi Arabia, (B) Borneo, Indonesia, and (C) Košický, Slovakia.
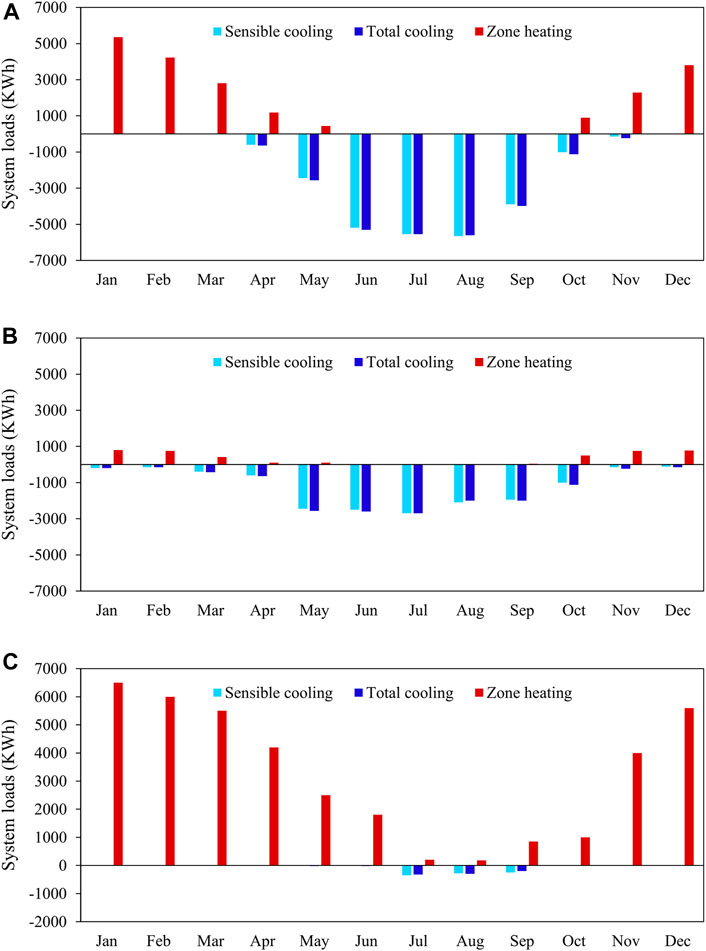
FIGURE 8. Cooling load and waste in different parts of the building outer shell without insulation (A) Ha’il, Saudi Arabia, (B) Borneo, Indonesia, and (C) Košický, Slovakia.
Additionally, during August, Ha’il registered a total cooling load of 5,790 kWh, with Borneo and Košický recording 3,210 kWh and 385 kWh, respectively. Remarkably, the sensible cooling loads in Ha’il amounted to 5,780 kWh, 3,250 kWh in Borneo, and 400 kWh in Košický, marking the highest cumulative values observed throughout the entire year. Figure 8 further illustrates the cooling and heating loads, along with the apparent load, for an insulated exterior shell. Comparing the two modes in Ha’il revealed a reduction in heating load to 850 kWh and a reduction in cooling load to 650 kWh. Similarly, the heating loads in Borneo and Košický experienced reductions of 200 kWh and 2,000 kWh, respectively. In Borneo, the cooling load diminished by 550 kWh, while in Košický, it decreased by 50 kWh.
Evidently, this system exhibits superior performance in arid and hot environments, significantly reducing heating and cooling loads. Notably, the analysis of cooling and heating energy loads in the case studies highlighted that in arid climates like Ha’il and Saudi Arabia, a significant portion of cooling energy consumption occurs between April and October, spanning 7 months of the year. Meanwhile, Borneo, Indonesia, characterized by consistently high temperatures, maintains relatively stable cooling and heating energy consumption throughout the year. Conversely, in the cold and dry environment of Košický, Slovakia, buildings typically require cooling energy for the majority of the year, spanning approximately 10 months. These results underscore the dynamic nature of energy consumption in diverse climates and emphasize the critical role of climate-specific strategies in optimizing energy usage throughout the year.
3.4 Sensitivity analysis
There are various methods for performing sensitivity analysis. Methods based on regression and methods based on variance. Variance-based methods are superior because they are more accurate. The Sobol method, which is based on the separation of the objective function, is one of the methods based on variance that can provide an accurate sensitivity response. The Monte Carlo sampling method is used in this numerical method. The Sobol variance method is used in this article. This method was originally used for uniform distribution. Arwade et al. (2010) also developed it for normal distribution and used it in structural problems. Figure 9 depicts the sensitivity analysis of the investigated parameters on the building’s energy consumption and annual CO2 emissions. Figure 9 shows that WWR has the greatest impact on energy consumption and CO2 emissions.
3.5 Optimization results
Table 4 provides a comprehensive overview of the optimal design solutions derived from the PSO optimization model, considering energy consumption and CO2 emission parameters under diverse weather conditions. The comparison between the standard design conditions, focused solely on wall insulation, and the optimal settings is presented. The application of the optimization model, incorporating the variables examined in this study, resulted in a notable reduction in energy consumption costs, ranging between 14.5% and 19%, depending on the specific weather conditions.
According to the results of a study by Goia (2016), adjusting the WWR of a building in Rome, Italy showed that the optimal WWR, which was determined to be 35%–45%. Shaeri et al. (2019) determined that the building exhibited the lowest energy consumption when the WWR was between 20% and 60%. In cold climates, the optimal WWR range was found to be 20%–40%, while in hot climates. Based on the findings displayed in Table 4, it can be concluded that the proposed optimization model yields an optimal WWR ranging from 40% to 50% for energy consumption under various meteorological conditions.
Furthermore, the investigation of CO2 emissions for the study building across three distinct climates revealed significant reductions through the implementation of an optimization approach in the structure’s design and operation. Specifically, the optimized approach in Košický, Slovakia, led to an 8% reduction in CO2 emissions. Similarly, in Ha’il, Saudi Arabia, a reduction of 7.2% was achieved, while in Borneo, Indonesia, the emission reduction reached 11.1%.
These results underscore the efficacy of the optimization method in effectively mitigating the environmental impact of the structure, emphasizing the critical role of tailored strategies that account for climate-specific factors. Notably, the findings highlight the importance of incorporating optimized approaches to reduce carbon emissions, thereby contributing significantly to sustainable construction practices and the global effort to combat climate change. The successful application of these strategies showcases their potential to foster environmentally conscious building practices and promote a more sustainable future.
4 Conclusion
The growing global concerns regarding population growth, amplified global warming, escalating greenhouse gas levels, and the overreliance on fossil fuels have precipitated critical challenges for humanity. Mitigating this crisis necessitates a concerted effort to curtail energy consumption, with buildings representing a significant portion of energy usage. Leveraging BIM as a practical approach in energy research and analysis, this study scrutinized the energy consumption of a building, particularly focusing on cooling and heating losses, along with the impact of exterior insulation on energy usage.
By employing Revit Autodesk 2020 for building design and DesignBuilder for energy consumption calculations, this study provided a comprehensive exploration of how to minimize heating and cooling energy consumption across different months and climates. Notably, the examination of various weather conditions highlighted the superior performance of the system under both heating and cooling load scenarios in arid and hot climates. It is crucial to note that energy losses are notably higher in the arid and hot climate of Ha’il compared to other climates.
The application of the PSO evolutionary algorithm optimization revealed that by incorporating input characteristics such as WWR, wall compositions, glazing, and weather conditions, a potential 19% reduction in ECC can be achieved. This reduction can be attributed to the temperature and humidity differentials between the building’s interior and exterior. Notably, the integration of external wall insulation parameters highlighted the significance of incorporating additional factors and strategies prior to construction, effectively reducing energy consumption and costs.
Furthermore, the successful reduction of CO2 emissions and ECC underscored the potential of optimization techniques in facilitating environmentally sustainable and cost-effective designs and operational practices. Emphasizing the importance of integrating such methodologies into future construction projects, this study advocates for a harmonious balance between meeting building requirements and minimizing ecological footprints. The insights provided here serve as a valuable guide for the practical implementation of optimization methods, offering a promising pathway toward fostering sustainable and ecologically conscious architectural practices.
Data availability statement
The original contributions presented in the study are included in the article/Supplementary material, further inquiries can be directed to the corresponding author.
Author contributions
AA: Conceptualization, Data curation, Methodology, Software, Validation, Writing–original draft. YD: Conceptualization, Data curation, Methodology, Software, Validation, Writing–original draft. AN: Visualization, Writing–original draft, Writing–review and editing. AA-M: Conceptualization, Investigation, Methodology, Validation, Writing–review and editing. AM: Data curation, Investigation, Software, Writing–review and editing.
Funding
The author(s) declare that no financial support was received for the research, authorship, and/or publication of this article.
Acknowledgments
Authors would like to acknowledge the support of the Deputy for Research and Innovation- Ministry of Education, Kingdom of Saudi Arabia for this research through a grant (NU/IFC/2/SERC/-/14) under the Institutional Funding Committee at Najran University, Kingdom of Saudi Arabia.
Conflict of interest
The authors declare that the research was conducted in the absence of any commercial or financial relationships that could be construed as a potential conflict of interest.
Publisher’s note
All claims expressed in this article are solely those of the authors and do not necessarily represent those of their affiliated organizations, or those of the publisher, the editors and the reviewers. Any product that may be evaluated in this article, or claim that may be made by its manufacturer, is not guaranteed or endorsed by the publisher.
References
Abdel-Mawla, M. A., Hassan, M. A., and Khalil, A. (2022). Phase change materials in thermally activated building systems: a comprehensive review. Int. J. Energy Res. 46, 11676–11717. doi:10.1002/er.8035
Afshar, A., Soleimanian, E., Akbari Variani, H., Vahabzadeh, M., and Molajou, A. (2022). The conceptual framework to determine interrelations and interactions for holistic Water, Energy, and Food Nexus. Environ. Dev. Sustain. 24, 10119–10140. doi:10.1007/s10668-021-01858-3
Alaiwi, Y., Taha, A., and Aljumaili, Y. (2023). Enhancement of the polycrystalline solar panel performance using a heatsink cooling system with PCM. Int. J. Eng. Artif. Intell. 4, 24–34. doi:10.55923/jo.ijeal.4.1.103
Arumugam, P., Ramalingam, V., and Vellaichamy, P. (2022). Effective PCM, insulation, natural and/or night ventilation techniques to enhance the thermal performance of buildings located in various climates – a review. Energy Build. 258, 111840. doi:10.1016/j.enbuild.2022.111840
Arwade, S. R., Moradi, M., and Louhghalam, A. (2010). Variance decomposition and global sensitivity for structural systems. Eng. Struct. 32, 1–10. doi:10.1016/j.engstruct.2009.08.011
Bahramian, F., Akbari, A., Nabavi, M., Esfandi, S., Naeiji, E., and Issakhov, A. (2021). Design and tri-objective optimization of an energy plant integrated with near-zero energy building including energy storage: an application of dynamic simulation. Sustain. Energy Technol. Assessments 47, 101419. doi:10.1016/j.seta.2021.101419
Balali, A., Hakimelahi, A., and Valipour, A. (2020). Identification and prioritization of passive energy consumption optimization measures in the building industry: an Iranian case study. J. Build. Eng. 30, 101239. doi:10.1016/j.jobe.2020.101239
Balouch, S., Abrar, M., Abdul Muqeet, H., Shahzad, M., Jamil, H., Hamdi, M., et al. (2022). Optimal scheduling of demand side load management of smart grid considering energy efficiency. Front. Energy Res. 10. doi:10.3389/fenrg.2022.861571
Boddapati, V., Sree Ram Nandikatti, A., and Arul daniel, S. (2021). Design and feasibility analysis of a solar PV array installation during the construction of high-rise residential buildings. J. Energy Syst. 5, 60–79. doi:10.30521/jes.811978
Boddupalli, C., Sadhu, A., Rezazadeh Azar, E., and Pattyson, S. (2019). Improved visualization of infrastructure monitoring data using building information modeling. Struct. Infrastructure Eng. 15, 1247–1263. doi:10.1080/15732479.2019.1602150
Carvalho, J. P., Bragança, L., and Mateus, R. (2021). Sustainable building design: analysing the feasibility of BIM platforms to support practical building sustainability assessment. Comput. Industry 127, 103400. doi:10.1016/j.compind.2021.103400
Choi, J.-W., Jun, Y.-J., Yoon, J., Song, Y., and Park, K.-S. (2019). A study of energy simulation integrated process by automated extraction module of the BIM geometry module. Energies 12, 2461. doi:10.3390/en12132461
Darko, A., Chan, A. P. C., Yang, Y., and Tetteh, M. O. (2020). Building information modeling (BIM)-based modular integrated construction risk management – critical survey and future needs. Comput. Industry 123, 103327. doi:10.1016/j.compind.2020.103327
De Lieto Vollaro, R., Guattari, C., Evangelisti, L., Battista, G., Carnielo, E., and Gori, P. (2015). Building energy performance analysis: a case study. Energy Build. 87, 87–94. doi:10.1016/j.enbuild.2014.10.080
Di Biccari, C., Calcerano, F., D’Uffizi, F., Esposito, A., Campari, M., and Gigliarelli, E. (2022). Building information modeling and building performance simulation interoperability: state-of-the-art and trends in current literature. Adv. Eng. Inf. 54, 101753. doi:10.1016/j.aei.2022.101753
Elnabawi, M. H. (2020). Building information modeling-based building energy modeling: investigation of interoperability and simulation results. Front. Built Environ. 6. doi:10.3389/fbuil.2020.573971
Fan, C., and Ding, Y. (2019). Cooling load prediction and optimal operation of HVAC systems using a multiple nonlinear regression model. Energy Build. 197, 7–17. doi:10.1016/j.enbuild.2019.05.043
Gao, H., Koch, C., and Wu, Y. (2019). Building information modelling based building energy modelling: a review. Appl. Energy 238, 320–343. doi:10.1016/j.apenergy.2019.01.032
Ghedas, H. B. (2021). Skylight as a passive design strategy in Tunisian dwelling using BIM technology. Int. J. BIM Eng. Sci., 18–25. doi:10.54216/IJBES.040102
Gholamian, E., Bagheri Barmas, R., Zare, V., and Ranjbar, S. F. (2022). The effect of Incorporating phase change materials in building envelope on reducing the cost and size of the integrated hybrid-solar energy system: an application of 3E dynamic simulation with reliability consideration. Sustain. Energy Technol. Assessments 52, 102067. doi:10.1016/j.seta.2022.102067
Goia, F. (2016). Search for the optimal window-to-wall ratio in office buildings in different European climates and the implications on total energy saving potential. Sol. Energy 132, 467–492. doi:10.1016/j.solener.2016.03.031
Gopinath, R., Kumar, M., Prakash Chandra Joshua, C., and Srinivas, K. (2020). Energy management using non-intrusive load monitoring techniques – state-of-the-art and future research directions. Sustain. Cities Soc. 62, 102411. doi:10.1016/j.scs.2020.102411
Guo, K., Li, Q., Zhang, L., and Wu, X. (2021). BIM-based green building evaluation and optimization: a case study. J. Clean. Prod. 320, 128824. doi:10.1016/j.jclepro.2021.128824
Huang, Y., and Li, C. (2021). Accurate heating, ventilation and air conditioning system load prediction for residential buildings using improved ant colony optimization and wavelet neural network. J. Build. Eng. 35, 101972. doi:10.1016/j.jobe.2020.101972
Jasim, A., Ahmed, M. K., and Alaiwi, Y. (2023). Performance of solar stills integrated with PV/Thermal solar collectors: a review. NTU J. Renew. Energy 4, 97–111. doi:10.56286/ntujre.v4i1.456
Kalair, A., Abas, N., Saleem, M. S., Kalair, A. R., and Khan, N. (2021). Role of energy storage systems in energy transition from fossil fuels to renewables. Energy Storage 3. doi:10.1002/est2.135
Kamel, E., and Memari, A. M. (2019). Review of BIM’s application in energy simulation: tools, issues, and solutions. Automation Constr. 97, 164–180. doi:10.1016/j.autcon.2018.11.008
Khan, S., and Panuwatwanich, K. (2021). Applicability of building information modeling integrated augmented reality in building facility management. Lect. Notes Civ. Eng., 2139–2148. doi:10.1007/978-981-15-8079-6_196
Liu, B., Chen, J., Wang, H., and Wang, Q. (2020). Renewable energy and material supply risks: a predictive analysis based on an LSTM model. Front. Energy Res. 8. doi:10.3389/fenrg.2020.00163
Liu, S., and Ning, X. (2019). A two-stage building information modeling based building design method to improve lighting environment and increase energy efficiency. Appl. Sci. 9, 4076. doi:10.3390/app9194076
Liu, Z., and Jiang, G. (2021). Optimization of intelligent heating ventilation air conditioning system in urban building based on BIM and artificial intelligence technology. Comput. Sci. Inf. Syst. 18, 1379–1394. doi:10.2298/CSIS200901027L
Liu, Z., Lu, Y., Shen, M., and Peh, L. C. (2021). Transition from building information modeling (BIM) to integrated digital delivery (IDD) in sustainable building management: a knowledge discovery approach based review. J. Clean. Prod. 291, 125223. doi:10.1016/j.jclepro.2020.125223
Mobaraki, O., Mohammadi, J., and Zarabi, A. (2012). Urban form and sustainable development: the case of urmia city. J. Geogr. Geol. 4. doi:10.5539/jgg.v4n2p1
Molajou, A., Afshar, A., Khosravi, M., Soleimanian, E., Vahabzadeh, M., and Variani, H. A. (2021). A new paradigm of water, food, and energy nexus. Environ. Sci. Pollut. Res. 30, 107487–107497. doi:10.1007/s11356-021-13034-1
Pellegrini, L., Campi, S., Locatelli, M., Pattini, G., Di Giuda, G. M., and Tagliabue, L. C. (2020). Digital transition and waste management in architecture, engineering, construction, and operations industry. Front. Energy Res. 8. doi:10.3389/fenrg.2020.576462
Pérez-Lombard, L., Ortiz, J., and Pout, C. (2008). A review on buildings energy consumption information. Energy Build. 40, 394–398. doi:10.1016/j.enbuild.2007.03.007
Pinheiro, S., Wimmer, R., O’Donnell, J., Muhic, S., Bazjanac, V., Maile, T., et al. (2018). MVD based information exchange between BIM and building energy performance simulation. Automation Constr. 90, 91–103. doi:10.1016/j.autcon.2018.02.009
Ramakrishna, S., Ngowi, A., Jager, H.De, and Awuzie, B. O. (2020). Emerging industrial revolution: symbiosis of industry 4.0 and circular economy: the role of universities. Sci. Technol. Soc. 25, 505–525. doi:10.1177/0971721820912918
Rausch, C., and Haas, C. (2021). Automated shape and pose updating of building information model elements from 3D point clouds. Automation Constr. 124, 103561. doi:10.1016/j.autcon.2021.103561
Salimzadeh, N., Vahdatikhaki, F., and Hammad, A. (2020). Parametric modeling and surface-specific sensitivity analysis of PV module layout on building skin using BIM. Energy Build. 216, 109953. doi:10.1016/j.enbuild.2020.109953
Sanhudo, L., Ramos, N. M. M., Poças Martins, J., Almeida, R. M. S. F., Barreira, E., Simões, M. L., et al. (2018). Building information modeling for energy retrofitting – a review. Renew. Sustain. Energy Rev. 89, 249–260. doi:10.1016/j.rser.2018.03.064
Shacheri, F., Nikou, S. R., Ziaei, A. N., and Saeedi, M. (2022). Surface dense discharge from rectangular and trapezoidal channels. Flow Meas. Instrum. 87, 102213. doi:10.1016/j.flowmeasinst.2022.102213
Shaeri, J., Habibi, A., Yaghoubi, M., and Chokhachian, A. (2019). The optimum window-to-wall ratio in office buildings for hot‒humid, hot‒dry, and cold climates in Iran. Environments 6, 45. doi:10.3390/environments6040045
Sharif, S. A., and Hammad, A. (2019). Simulation-based multi-objective optimization of institutional building renovation considering energy consumption, life-cycle cost and life-cycle assessment. J. Build. Eng. 21, 429–445. doi:10.1016/j.jobe.2018.11.006
Tajfar, I., Pazoki, M., Pazoki, A., Nejatian, N., and Amiri, M. (2023). Analysis of heating value of hydro-char produced by hydrothermal carbonization of cigarette butts. Pollution 9, 1273–1280. doi:10.22059/poll.2023.335704.1293
Tan, T., Mills, G., Papadonikolaki, E., and Liu, Z. (2021). Combining multi-criteria decision making (MCDM) methods with building information modelling (BIM): a review. Automation Constr. 121, 103451. doi:10.1016/j.autcon.2020.103451
Taner, T. (2018). Energy and exergy analyze of PEM fuel cell: a case study of modeling and simulations. Energy 143, 284–294. doi:10.1016/j.energy.2017.10.102
Taner, T., Naqvi, S. A. H., and Ozkaymak, M. (2019). Techno-economic analysis of a more efficient hydrogen generation system prototype: a case study of pem electrolyzer with Cr-C coated SS304 bipolar plates. Fuel Cells 19, 19–26. doi:10.1002/fuce.201700225
Taner, T., and Sivrioglu, M. (2017). A techno-economic & cost analysis of a turbine power plant: a case study for sugar plant. Renew. Sustain. Energy Rev. 78, 722–730. doi:10.1016/j.rser.2017.04.104
Taner, T., Sivrioğlu, M., Topal, H., Dalkılıç, A. S., and Wongwises, S. (2018). A model of energy management analysis, case study of a sugar factory in Turkey. Sādhanā 43, 42. doi:10.1007/s12046-018-0793-2
Tehranian, K. (2023a). Can machine learning catch economic recessions using economic and market sentiments? SSRN Electron. J. doi:10.2139/ssrn.4553506
Tehranian, K. (2023b). Monetary policy & stock market. Available at: http://arxiv.org/abs/2305.13930.
Tushar, Q., Bhuiyan, M. A., Zhang, G., and Maqsood, T. (2021). An integrated approach of BIM-enabled LCA and energy simulation: the optimized solution towards sustainable development. J. Clean. Prod. 289, 125622. doi:10.1016/j.jclepro.2020.125622
Utkucu, D., and Sözer, H. (2020). Interoperability and data exchange within BIM platform to evaluate building energy performance and indoor comfort. Automation Constr. 116, 103225. doi:10.1016/j.autcon.2020.103225
Wang, D., Tan, D., and Liu, L. (2018). Particle swarm optimization algorithm: an overview. Soft Comput. 22, 387–408. doi:10.1007/s00500-016-2474-6
Wang, Q., and Li, R. (2016). Drivers for energy consumption: a comparative analysis of China and India. Renew. Sustain. Energy Rev. 62, 954–962. doi:10.1016/j.rser.2016.04.048
Yang, F., and Dian, J. (2022). Macro-economic impact of policies for controlling fossil energy consumption in China. Energies 15, 1051. doi:10.3390/en15031051
Yavari, F., Salehi Neyshabouri, S. A., Yazdi, J., Molajou, A., and Brysiewicz, A. (2022). A novel framework for urban flood damage assessment. Water Resour. Manag. 36, 1991–2011. doi:10.1007/s11269-022-03122-3
Yu, C. R., GuoSenWang, H. Q. C., and Chang, R. D. (2020). Revealing the impacts of passive cooling techniques on building energy performance: a residential case in Hong Kong. Appl. Sci. Switz. 10, 4188. doi:10.3390/APP10124188
Zhang, X., Qu, Q., Zhou, A., Wang, Y., Zhang, J., Xiong, R., et al. (2022). Core-shell microparticles: from rational engineering to diverse applications. Adv. Colloid Interface Sci. 299, 102568. doi:10.1016/j.cis.2021.102568
Keywords: energy consumption cost, energy-carbon emission nexus, building information modelling, cooling and heating loads, optimization
Citation: Alhamami AH, Dodo YA, Naibi AU, Alviz-Meza A and Mokhtarname A (2023) Energy-carbon emission nexus in a residential building using BIM under different climate conditions: an application of multi-objective optimization. Front. Energy Res. 11:1326967. doi: 10.3389/fenrg.2023.1326967
Received: 24 October 2023; Accepted: 14 November 2023;
Published: 27 November 2023.
Edited by:
Mohammad Hossein Ahmadi, Shahrood University of Technology, IranReviewed by:
Hossein Moayedi, Southern Illinois University Edwardsville, United StatesTolga Taner, Aksaray University, Türkiye
Hasan Yildizhan, Adana Alparslan Turkes Science and Technology University, Türkiye
Fathollah Pourfayaz, University of Tehran, Iran
Copyright © 2023 Alhamami, Dodo, Naibi, Alviz-Meza and Mokhtarname. This is an open-access article distributed under the terms of the Creative Commons Attribution License (CC BY). The use, distribution or reproduction in other forums is permitted, provided the original author(s) and the copyright owner(s) are credited and that the original publication in this journal is cited, in accordance with accepted academic practice. No use, distribution or reproduction is permitted which does not comply with these terms.
*Correspondence: Aníbal Alviz-Meza, alvizanibal@crece.uss.edu.pe